- 1Department of Biology Education, Centre of Math and Science Education, University of Bayreuth, Bayreuth, Germany
- 2Interdisciplinary Centre for Security, Reliability, and Trust, FINATRAX Research Group, University of Luxembourg, Esch-sur-Alzette, Luxembourg
The relevance of English language competencies in authentic, discipline-specific contexts at school is increasingly acknowledged outside of English-speaking countries. Since any understanding of complex scientific problems requires the combination of scientific literacy with other competencies, such as scientific modelling, the appropriate application of Content and Language Integrated Learning (CLIL) is of great importance. The present study focuses on an established, hands-on outreach genetic education module on DNA structure, which it extends with a bilingual adaption to examine the influence of non-CLIL and CLIL learning on students’ scientific modelling skills and model understanding. When comparing non-CLIL learners (n = 149) and CLIL learners (n = 316), the former received higher scores in the assessment of model-related self-evaluation sheets and built better models. We also found that non-CLIL learners achieved better temporary knowledge of “DNA as a model” scores and, for model evaluation, were more reflective in determining similarities and differences between their hand-crafted model and a commercial DNA school model. However, CLIL learners performed better in comparing their model sketches with their hand-crafted models. They also used different approaches to develop models and conceptualize integral components of models, as reflected in their advanced model understanding. We conclude that CLIL influences modelling qualities on different levels, by fostering modelling practice, and in particular, model understanding.
Introduction
English has become the internationally acknowledged lingua franca of science and often requires English language competencies beyond levels commonly achieved in school language lessons (Rodenhauser and Preisfeld, 2015). In response, the European Commission (Hrsg.) (2004), among other authorities, has emphasized the need for Content and Language Integrated Learning (CLIL) to prepare young students for the demands of a globalized society (Canz et al., 2021; Coyle and Meyer, 2021). At German schools, CLIL subject teaching is increasingly applied in science education. Thereby, students are encouraged to understand and apply scientific content in English at the same time as achieving an appropriate level of scientific literacy (Lemke, 1990; Klieme et al., 2010; Canz et al., 2021).
Definitions of scientific literacy commonly fit into two complementary groups: (1) understanding scientific content and working scientifically, enabling one’s development as a future scientist, and (2) understanding science and using scientific information to make informed decisions as a conscientious citizen (Ke et al., 2020). Thus, scientific literacy goes beyond language alone but requires knowledge of relevant vocabulary, and an understanding of scientific practices that enable and encourage in-depth analyses of observed phenomena (Passmore and Svoboda, 2012; Mendonça and Justi, 2013). One such practice is scientific modelling (Ke et al., 2020), which helps break down complex phenomena in the search for possible explanations and encourages scientific discourse (Lee et al., 2015).
Modelling has received considerable attention in science education classrooms. As “an explanatory system that represents objects or phenomena via discourse, writing, behaviour, and drawing” (Lee et al., 2015, p. 234), a model engages—and, potentially, fosters—various scientific competencies. This, however, only holds true “if students understand the nature and the purpose of models in science, as well as comprehend how models are constructed” (Sins et al., 2009, p. 1206). The fact that their use encourages students to actively discuss scientific findings and ideas makes models a particularly important tool for achieving scientific literacy. In combination with CLIL learning, scientific modelling may advance many competencies required to be successful in an international academic environment (Grandinetti et al., 2013), such as in-depth understanding of scientific phenomena, and the capability to effectively communicate this understanding (Passmore and Svoboda, 2012; Mendonça and Justi, 2013; Ke et al., 2020).
To explore the effect of CLIL learning on knowledge of “DNA as a model,” model understanding, and the ability of students to develop and evaluate models, we piloted a CLIL genetics outreach learning module structurally identical to a previously conducted non-CLIL outreach learning module (Roth et al., 2020). The module lasted for 1 day, which authentically reproduces the overall very little time that teachers in regular science classrooms have to foster model understanding—irrespective of CLIL or non-CLIL. In the course of 2 months with between three to four visiting classes and respective 1-day modules per week, we focused on the recently hypothesized relationship between scientific modelling and scientific literacy (Ke et al., 2020) to explore the influence of this relationship on model understanding. Specifically, we examine the changing nature of models (CNM) as a subscale of model competency (Treagust et al., 2002). While the model-of-approach of our module only covers the most basic level of model learning, even at this level, students often do not display model understanding. Prior studies by, for instance, Treagust et al. (2002) and Krell et al. (2012), indicated that many students understand the multiplicity of models but still perceive them as an exact replica of the scientific phenomenon. Such weak epistemological understanding may—if not improved—prevent students from developing content knowledge or understanding (Schwarz and White, 2005; Sins et al., 2009). We also investigate knowledge of “DNA as a model” scores yet do so with the understanding that both modelling and language learning as well as the development of scientific literacy require a high degree of mental capacity, which could result in a mental trade-off (Grandinetti et al., 2013). Other studies, such as Lo and Lo (2014) and Piesche et al. (2016) have already indicated that this trade-of could have adverse effects on academic performance. Moreover, we explicitly do not focus on language learning as an outcome of the CLIL module but—due to the increasing adoption of CLIL outside of English-speaking countries—particularly examine the potential positive and negative outcomes combining CLIL and model understanding. To explore these possible mutual influences, we first outline the relevant theoretical background, including the basic principles of CLIL learning and model learning, and the connection between model learning and scientific literacy.
Theoretical Background
Content and Language Integrated Learning
“Reading, writing, and oral communication are critical literacy practices for participation in a global society. [They] support learners by enabling them to grapple with ideas, share their thoughts, enrich understanding and solve problems” (Krajcik and Sutherland, 2010, p. 456). The central importance of written and oral language competencies makes scientific literacy in English indispensable (Yore and Treagust, 2006; Coyle and Meyer, 2021; Pfenninger, 2022). Thus, efforts to improve scientific literacy in English need to be increased at school (Virida, 2021). European language policies support this endeavour by encouraging English language learning in regular school curricula outside of English language learning classes via CLIL (European Commission, 2012). Used effectively, CLIL combines language learning and content learning so that neither proficiency in English nor the respective subject area is a prerequisite for successful learning (Stoddart et al., 2002; European Commission (Hrsg.), 2004).
Content and language integrated learning “encompasses any activity in which a foreign language is used as a tool in the learning of a non-language subject in which both language and subject have a joint role” (Marsh, 2002, p. 58). Although CLIL learning, by definition, requires content and language to be equally integrated into lesson planning, the scientific language often acts only as a catalyst (Rodenhauser and Preisfeld, 2015). That is, the attention is primarily on the content—similar to regular science teaching—and the language only plays a minor role (Nikula et al., 2016). Few teachers are aware of the intricate connection between language and content (Meskill and Oliveira, 2019; Pfenninger, 2022). The one-dimensional view of language and content learning fails to acknowledge that content and its contextualization induce an act of language, while language gives meaning to content (Stoddart et al., 2002; Luykx et al., 2008). This connective approach is particularly relevant for CLIL in STEM subjects. More specifically, scientific practice and discourse, for instance the explanation of a phenomenon or the development of a respective model, encourages the use of language as a mediator of meaning (“what language does”) and not solely as a structural body of grammar and words without contextualization (“what language is”) (Lee and Stephens, 2020, p. 429). A recent study by Tagnin and Ní Ríordáin (2021) confirms the need for scientific discourse in CLIL and emphasized the importance of higher-order-thinking questions. This notion is grounded in information processing theories (Craik and Lockhart, 1972) that assume high cognitive engagement in fostering understanding to be the basis of deep learning. Yet, a high cognitive engagement due to language and content learning in tandem, can also have adverse effects on academic achievements. Studies such as Lo and Lo (2014) or Piesche et al. (2016) show that CLIL students often display lower content knowledge scores, since CLIL students require more effort and different learning strategies to understand the same information as non-CLIL students. This leaves a research gap as to what beneficial effects the application of CLIL can have on different kinds of science learning. Building Craik and Lockhart (1972) as well as Vygotsky (1986) CLIL proof itself for deep learning tasks as opposed to common content learning exercises. In particular, authentic settings, wherein “visual cues, concrete objects, and hands-on activities” (Stoddart et al., 2002, p. 666) are supplied can may provide the necessary basis for this kind of learning (Meskill and Oliveira, 2019). Accordingly, enriching such settings with CLIL may encourage scientific literacy in both the native language and English (via the regular practice) (Gonzalez-Howard and McNeill, 2016; Tolbert et al., 2019) and foster deep learning. This may, however, also be very dependent on the country and precise learning context (Virida, 2021).
Examples from practice (Lam et al., 2012) confirm that “the creation of immersive environments where students participate in discourse patterns that mirror those seen in the scientific community” has a positive effect on scientific literacy and content learning (Quarderer and McDermott, 2020, p. 3). Such environments are often created through inquiry learning, which combines hands-on activities with experiments and the exploration of a scientific phenomenon (Hampton and Rodriguez, 2001; Campillo-Ferrer and Miralles-Martínez, 2022; Roth et al., 2022). Thereby, students engage in meaningful scientific practice, such as describing observed phenomena, formulating hypotheses, assessing results, and reflecting on findings, which demands the use of a discipline-specific register (Stoddart et al., 2002; Satayev et al., 2022). To foster such practice in science classrooms, Tolbert et al. (2019) suggested four dimensions of instruction, including contextualization, scientific reasoning, scientific discourse and scientific literacy. This way, language is inherently contextualized—no longer a mere catalyst—and helps students to structure and communicate acquired information (Lemke, 1990; Hampton and Rodriguez, 2001; Satayev et al., 2022). A study by Piacentini et al. (2022) showed that in addition to science and language learning rewards for students, CLIL also benefitted teachers to recognize pattern among their students that they have been oblivious to previously.
Since CLIL learning requires considerable mental capabilities to manage content and language teaching simultaneously, effective scaffolding is necessary to decrease the cognitive load (Grandinetti et al., 2013; Tolbert et al., 2019). Scaffolding is “a type of teacher assistance that helps students learn new skills, concepts, or levels of comprehension of material” (Maybin et al., 1992, p. 188). Such scaffolding activities are particularly important for the discipline-specific register of the foreign language, as the “language provides learners with meaningful cues that help them interpret the content being communicated” (Stoddart et al., 2002, p. 666). Gottlieb (2016) describes four possible scaffolding dimensions: linguistic, graphic, sensory, and interactive. In science subjects such as biology, graphic or visual scaffolding involving diagrams, graphs, or charts are commonly used tools. These have also proven useful for language learning (Kress, 2003; Evnitskaya and Morton, 2011). Other forms of scaffolding that may come in handy for CLIL science teaching include linguistic scaffolding, which can provide definitions of key terms, language frames, or bridging and prompting to foster scientific literacy (France, 2019).
Model Learning
One increasingly popular inquiry method for fostering scientific literacy is scientific modelling (Akerson et al., 2009; Ke et al., 2020). Models are commonly used to visualize or explain phenomena (Krajcik and Merritt, 2012). The process skills of scientific modelling include revealing underlying mechanisms, showing causal links, raising questions, and testing multiple hypotheses (Akerson et al., 2009). Whenever a prediction proves incorrect or new evidence emerges, a model can be adapted and refined accordingly (Passmore et al., 2009).
This adaptability of models to different theoretical perspectives is described as the changing nature of models (CNM) in the SUMS questionnaire (Students’ Understanding of Models in Science), which was designed to gain insights into students’ understanding of models (Treagust et al., 2002). It is an essential aspect of modelling practice since most students believe models to be exact replicas (EM) “of reality that embody different spatio-temporal perspectives [in contrast to] constructed representations that may embody different theoretical perspectives” (Grosslight et al., 1991, p. 799). A study by Krell et al. (2012) confirmed these findings. Treagust et al. (2002) initially hypothesized that the analysis of more abstract concepts would automatically lead to a more abstract perception of scientific models, but their results indicated that this does not hold true. Also, Schwarz et al. (2009) alongside Sins et al. (2009) and Louca and Zacharia (2012) questioned the development of epistemic knowledge about models and decontextualized understanding of models. In classroom teaching, scientific models often lack contextualization and are only introduced superficially. Thus, students do not usually understand the value of scientific models for explaining phenomena, appreciate the adaptability of models to emerging evidence, or recognize differences between models and explained phenomena (Krajcik and Merritt, 2012; Ke et al., 2020).
Yet, establishing model understanding in classroom practice is no easy feat (Gilbert and Justi, 2002; Sins et al., 2009), which is why research often focusses on influencing factors to improve scientific modelling and model understanding (Passmore and Svoboda, 2012; Mendonça and Justi, 2013). One influencing factor may be the positive relationship between modelling and content knowledge due to the application of in-depth strategies inherent to deep learning, such as cognitive reasoning through metacognition (Sins et al., 2009; Mendonça and Justi, 2013). Reflective thinking to evaluate possible learning outcomes and the generation of explanations for observed phenomena are particularly salient for deep learning strategies (Lee et al., 2015). Such strategies require communicative action between students to convey their thoughts and build valid arguments that explain the observed phenomenon (Passmore and Svoboda, 2012; Ke et al., 2020). The use of modelling thus provides students with an experience that mirrors those of scientists, who also have to evaluate, modify, and argue in support of their models when presenting them to their peers (Mendonça and Justi, 2013; Ke and Schwarz, 2020). Students, thereby, “do not just describe empirical experiences [when they engage in modelling in science lessons] as they would with experiments and observations; they can also reason, explain, and communicate phenomena or systems using empirical experiences as evidence” (Lee et al., 2015, p. 236).
The scientific discourse associated with modelling is also what renders models useful agents of CLIL. Modelling as a deep-learning practice allows students a stepwise approach to understanding the scientific phenomenon in question (Ke et al., 2020). At the same time, modelling as a discursive activity also fosters understanding of different models, their scope, and limitations. Boulter (2000) drafts the different dependencies and interactions between language and modelling as observed in classroom discourse. She describes language as an instrument of contextualization that initiates, directs and informs knowledge or ideas in a didactic, socratic, or dialogic manner that can be summarized in, for instance, visual-graphic or verbal-metaphoric models. The visual thinking connected to modelling is often also regarded as a scaffolding-tool in language learning (Fernández-Fontecha et al., 2020). Based on the dual-coding theory by e.g., Clark and Paivio (1991) and context-availability method by e.g., Aslandag and Yanpar (2014) both the verbal and non-verbal code embedded into a larger context are believed to mediate the understanding of difficult information and the uptake of contextualized vocabulary (Fernández-Fontecha et al., 2020). In turn, the scientific discourse and vocabulary are believed to encourage model-understanding of students (Ke and Schwarz, 2020). The negotiation involved in constructing own models also requires students to answer more general questions regarding the nature, use, and criteria of models (Ke et al., 2020). However, in how far deep-learning processes of CLIL (Virdia and Wolff, 2020) combined with a concrete scientific phenomenon that is to be understood and modelled by students encourages model-understanding even further, remains to be determined.
Objectives of the Study
The present study aims to contribute to the current body of literature by providing insights into how a short-term CLIL module can foster deep-learning of specific complex scientific phenomena as an addition to regular classroom teaching. We, therefore, focus on the following research questions:
• RQ1: How does a 1-day CLIL science module influence students’ knowledge of “DNA as a model” throughout the hands-on laboratory?
• RQ2: How does CLIL influence students’ general understanding of models and modelling?
• RQ3: How does CLIL influence students’ ability to evaluate models—specifically, the two implemented evaluation phases (evaluation-1 and evaluation-2)?
Materials and Methods
Treatment Comparability
Following our previous German gene-technology module design that particularly focused on model-understanding and the knowledge of “DNA as a model” (Mierdel and Bogner, 2019; Roth et al., 2020), we wanted to explore the impact of deep-learning processes associated with CLIL on these two parameters. Thus, we retained the design and four intervention phases of the German gene-technology module, while carefully integrating CLIL (Table 1). Treatment comparability could be retained by focusing on the same age group, form of school, and region of participants of our previous module (Roth et al., 2020).
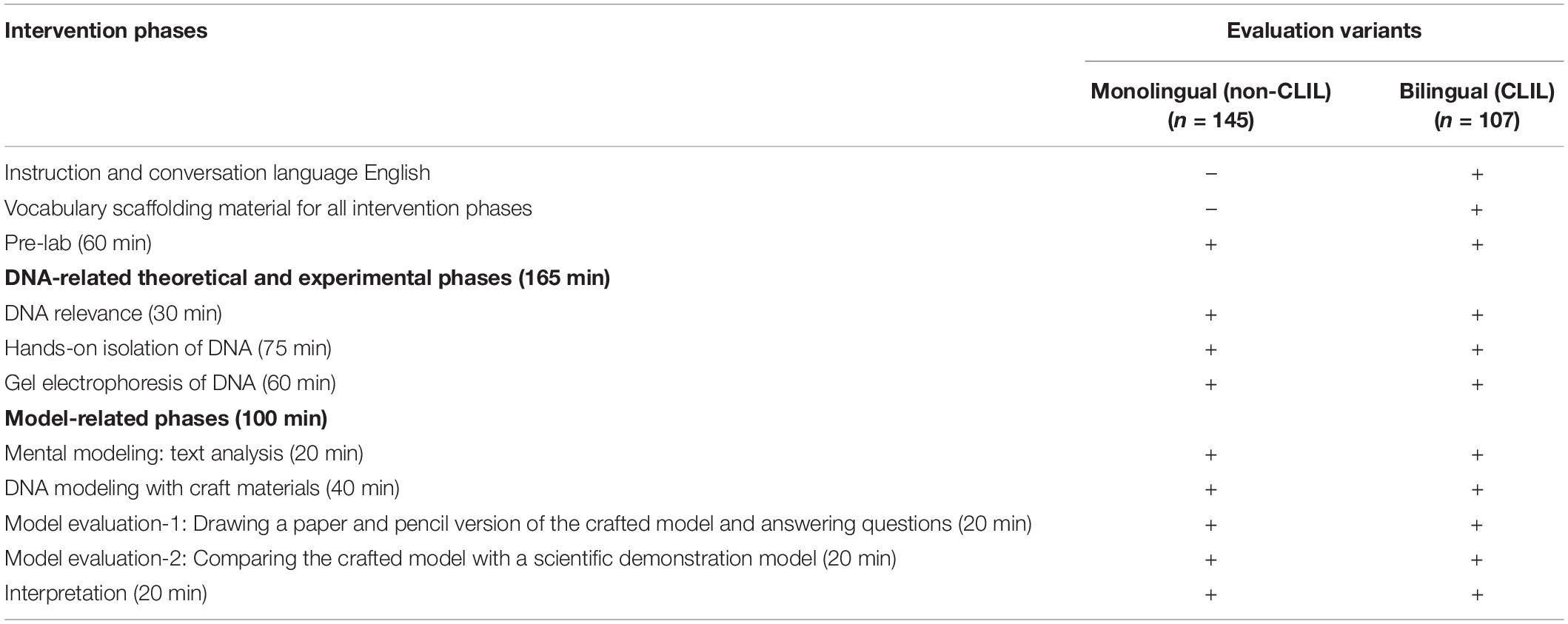
Table 1. Quasi-experimental intervention design [adapted from Roth et al. (2022)].
Analogous to the German module, we provided students with the same laboratory manuals but written in English. The laboratory manual was designed and adapted over the course of four laboratory evaluations (Goldschmidt and Bogner, 2016; Langheinrich and Bogner, 2016; Mierdel and Bogner, 2019; Roth et al., 2020). Only key terms had additional German translations [code-switching; Cheshire and Gardner-Chloros (1998)]. Content scaffolding in the laboratory manual, interactive smart-board presentations, and the interactive poster were retained across both modules. However, we created a special linguistic scaffolding workbook for the CLIL module to reduce difficulties in understanding and support content learning in a foreign language while fostering scientific literacy and language learning. The book contained language-specific riddles, such as word search puzzles and crossword puzzles, allocating words to English definitions. Students were also asked to match the words and their definitions with German translations or to translate the words into German. At all times, students were allowed to use English-English and English-German dictionaries available in the laboratory. For each phase of the intervention, we had one specific language scaffolding exercise (see Supplementary Datasheet 1). This is in line with supportive lexical focus on form (FonF) in CLIL environments to “[draw] learners’ attention to vocabulary items if they “are necessary for the completion of a communicative, or an authentic language task”” (Morton, 2015, p. 256). Since students did not have any previous laboratory experience and were not familiar with the terminology connected to DNA, the vocabulary scaffolding connected to the different phases throughout the laboratory intended to reduce language-related cognitive load (Mahan et al., 2018; Mahan, 2022).
Participants
Altogether, 465 ninth graders (higher secondary school) participated in our study (girls 50.8%, boys 49.2%; Mclass size = 21.9, SD = 4.6; Mage = 14.7, SD = 0.7), which lasted over 2 months and hosted between three to four classes each week. Seven intact classes from different schools took part in our non-CLIL German intervention (n = 149) in 2019 and 14 intact classes from different schools in our CLIL intervention (n = 316) in 2020. The disparity in participating classes of the two interventions was the result of a sudden increase in volunteering schools. Classes were assigned in a quasi-experimental research design, which excluded randomization and accounted for non-equivalence of groups (Taylor and Medina, 2011). Non-CLIL students teamed up in overall 69 two-person groups (and three three-person groups, two students working individually due to illness) and CLIL students in overall 151 two-person groups (and four three-person groups, two students working individually due to illness) while retaining class integrity.
Content and language integrated learning students were from the same schools in the same school district as the non-CLIL students. Classes were largely homogenous regarding gender ratio, social-income ratio, and cultural background. All students had only little prior experience with laboratory experimentation and exposure to English outside of the classroom. Teachers were explicitly asked to postpone content about DNA until after participation. All schools followed the same curriculum and had teachers from either a Biology-Chemistry, Biology-Math, or biology-English background.
Participation was voluntary. Written parental consent was given before students participated in our study, although the data collection was pseudo-anonymous, and students could not be identified. The study was designed in accordance with the World Medical Association (2013), and the state ministry approved the questionnaires used.
Treatment Description
Our 1-day, hands-on CLIL module offered inquiry-based learning activities focused on the structure of DNA, model-understanding, and CLIL adapted to the capabilities of ninth graders. The module’s content is in line with the state’s syllabus and follows national competency requirements (KMK, 2005). While CLIL may add another layer of complication to the already demanding goal of developing students’ modelling practice, such practice is very authentic with the increasing adoption of CLIL outside of English-speaking countries. The language of instruction was English, yet students could indicate with colour-cards in green, orange, and red if the instructions were easy to understand, required renewed explanation, or code-switching into German (Cheshire and Gardner-Chloros, 1998).
One of the authors instructed and guided the students throughout the laboratory. Teachers, who accompanied their classes either alone or with another colleague, participated in the laboratory as students or observed. Students participated in intact class-groups and worked in pairs to complete their tasks. Classes were assigned randomly after their confirmation of participation (Cook and Campell, 1979). Except for the theoretical and explanatory phases, the instructor acted as a learning guide and did not interfere with the students’ experiments. The students worked self-reliantly with the help of their laboratory manuals and linguistic scaffolding workbooks and only asked for explanations or assistance when required.
Intervention Phases
Pre-lab Phase
Both the German and CLIL module started with a pre-lab phase. After a short introduction to the rules of safety in a gene-technology laboratory, we familiarized students with the laboratory equipment, techniques of use and theoretical concepts connected to experimentation (e.g., Sarmouk et al., 2019). That is, students learned how to handle laboratory equipment—such as micropipettes and centrifuges—necessary for experimentation. The theoretical part was provided by the instructor with an interactive smartboard presentation including, for instance, the matching of laboratory equipment with the correct terminology or the matching of the correct micropipette to a given quantity of liquid. For the practical part of pipetting and centrifuging, the instructor acted as a guide and students derived their instructions from the laboratory manual.
DNA-Related Theoretical and Experimental Phases
After a short break, the pre-lab phase was followed by another theoretical phase that alerted students to the relevance of DNA-analyses in criminal investigations. To foreshadow the subsequent experimental phases, the instructor invited the students to be criminal investigators for 1 day (DNA relevance, Table 1). This also entailed a short recapitulation of previous knowledge about the structure of cells and an introduction to the scientific concepts of DNA isolation (Mierdel and Bogner, 2019; Roth et al., 2020). After successful extraction of DNA from oral mucosal cells, students assembled in the back of the laboratory where a poster and the gel-electrophoresis-devices were positioned. The poster was designed as a cloze. Together with the instructor, students filled in the cloze with knowledge they already had obtained from the workbook or derived from other science disciplines. To help students understand the very complex concept of gel-electrophoresis even better, the poster made use of visuals and code-switching. Subsequent to the theoretical phase, students further processed their DNA sample and applied to the gel of the gel-electrophoresis-device. Our decision to provide information in a series of theoretical phases ensured that students were not overwhelmed by the amount of information and we able to focus on the forthcoming experimental phases.
For the experimental phases, we used an evidence-based, two-step approach (Mierdel and Bogner, 2019; Roth et al., 2020). That is, students first answered questions in their laboratory manuals and considered subsequent experimental procedures. In addition, they filled in the linguistic exercises in the linguistic scaffolding workbook. Then, they worked in pairs to discuss each step before carrying out experiments. Such practice effectively combined hands-on and minds-on activities and required them to do more than simply follow instructions (Mierdel and Bogner, 2019; Roth et al., 2020). For our hands-on approach, we provided sufficient scaffolding material in the form of visuals in our laboratory manual to enable independent experimentation and self-reliant protocolling of their observations, as well as the materials necessary for modelling [same for both German and CLIL module; Mierdel and Bogner (2019); Roth et al. (2020)].
Model-Related Phases
Both model-related phases directly followed and built on the experimental, DNA-related phases. We subdivided our model-related phases into a mental modelling phase involving text analysis. This either consisted of the construction and discussion of a purely mental model or a rough sketch. Building on their mental model, students constructed a model from craft materials, which they evaluated in a detailed and labelled sketch (model evaluation-1 phase), and later compared to a commercially available DNA model and Watson and Cricks model in model evaluation-2 phase (Table 1).
We based our model-related phases on the four main stages of the Model of Modelling (Gilbert and Justi, 2002, p. 370 ff.). Mental modelling was, thereby, the key to providing a theoretical basis for experimental findings. In our module, a text about the discovery of the DNA’s structure (Usher, 2013) provided fundamental knowledge about the necessary components of the DNA. Students could then apply this knowledge to their simplified mental models, which either remained imaginary or were roughly sketched on paper (e.g., Franco and Colinvaux, 2000; Mierdel and Bogner, 2019). Our “model-of-approach,” which focused on conveying CNM instead of EM, may not be popular (Gouvea and Passmore, 2017), but followed the curriculum and laid the foundation for “model-for-approaches” by partially developing a DNA model from experimentation with DNA and Crick’s letter to his son about the discovery of DNA as well as students’ critical reflection on their model (ISB, 2019).
That is, after determining the DNA’s representation in a mental model and building the hand-crafted model from the text, we conducted a model evaluation-1 phase as a reciprocal self-evaluation. As combining sketching and hand-crafting models proved to be important (e.g., Prabha, 2016), students evaluated their hand-crafted DNA model based on a detailed paper-and-pencil version. Another effective method for encouraging self-evaluation is reflective writing (Kovanović et al., 2018). Open-ended questions about model-related components encouraged students to rethink and reassess certain steps and decisions in developing the mental model into its physical counterpart (Roth et al., 2020). In evaluation-2 phase, students assessed their hand-crafted DNA models using a comparison-based self-evaluation with a commercially available DNA demonstration model.
Interpretation Phase
In this final phase, the instructor collected different ideas of the “DNA as a model,” before revealing several different yet scientifically adequate models. This helped students understand that models can have different levels of complexity. A short recapitulation of history also showed that models may change with new scientific evidence and are, thus, not ER. Moreover, the instructor revealed the results of the gel-electrophoresis and explained the meaning of the different bars.
Dependent Variables
As dependent variables, we examined students’ knowledge of “DNA as a model” scores and model understanding in a repeated measurement design: a pre-test (T0) 2 weeks before the intervention, a post-test (T1) directly after the module, and a retention test 6 weeks later (T2). We examined students’ sketches and their responses to the open questions as part of the evaluation-1 phase, as well as students’ models and their self-evaluation sheets as part of the evaluation-2 phase.
Students’ Content Knowledge Regarding the Structure of DNA
We applied an ad-hoc knowledge test comprising eighteen multiple-choice questions about content knowledge related to the different model phases regarding the structure of DNA. Students had to select one correct answer out of four possible answers. To avoid response patterns, we randomly rearranged questions and possible answers for each testing time (Lam et al., 2012; Scharfenberg and Bogner, 2013). The complete questionnaire can be found in Supplementary Datasheet 2. Content validity was a given as the items were consistent with the state syllabus. Likewise, construct validity was confirmed based on the items’ heterogeneity in relation to complex constructs, such as building up content knowledge and understanding of scientific concepts (Rost, 2004). Cronbach’s alpha values of 0.70 (T0), 0.71 (T1), and 0.69 (T2) indicate acceptable internal consistency.
Students’ Model Understanding
Following Mierdel and Bogner (2019), we deployed a shortened version of the SUMS questionnaire (Treagust et al., 2002) focusing on the subscales of models as exact replicas (ER) and the changing nature of models (CNM), which was based on a five-point Likert-Scale ranging from strongly disagree (1) to strongly agree (5). The complete questionnaire can be found in Supplementary Datasheet 2. ER and CNM best address the two dimensions that form the focus of our modelling phases. As ER is a conceptual error, low scores are desirable. CNM, on the other hand, shows true model understanding, meaning that high scores are considered beneficial (Treagust et al., 2002). Cronbach’s alpha values above 0.7 (T0 = 0.81, T1 = 0.76, T2 = 0.70) indicate acceptable internal consistency (Lienert and Raatz, 1998). The SUMS questionnaire uses a 5-point Likert scale ranging from strongly disagree (1) to strongly agree (5).
Evaluation-1 Phase
To compare the effects of both treatment approaches on the evaluation-1 phase, we assessed students’ model sketches after all participating schools have visited the laboratory [changed after Langheinrich and Bogner (2016); for definitions, examples, and frequencies, see Supplementary Datasheet 3]. We then randomly selected 38 out of 231 drawings for a second scoring (16.4%). Cohen’s kappa coefficient (Cohen, 1968) scores of 0.90 and 0.85 for intra-rater and inter-rater reliability indicated an “almost perfect” rating (Wolf, 1997, p. 964).
Using content analysis (Bos and Tarnai, 1999), we iteratively categorized the statements made by students in response to the open questions. For the first question, “Which features of the original DNA molecule are simplified in your model,” we assigned each answer to one of four categories: level of DNA, level of substance, level of particles, and level of structure (for definitions, examples, and frequencies, see Supplementary Datasheet 4). We then randomly selected 76 out of 474 statements for a second scoring (16.0%). We computed Cohen’s kappa coefficient (Cohen, 1968) scores of 0.97 and 0.84 for intra-rater and inter-rater reliability, which indicated an “almost perfect” rating (Wolf, 1997, p. 964). For the second question, “Explain why one might create different models of one biological original (in our case, the structure of the DNA)?,” we applied the adapted category system developed by Langheinrich and Bogner (2016) and Mierdel and Bogner (2019) and identified five categories: individuality of DNA, different interpretation, different model design, different focus, and different research state (for definitions, examples, and frequencies, see Supplementary Datasheet 5). We then randomly selected 61 out of 416 statements for a second scoring (14.7%). We computed Cohen’s kappa coefficient (Cohen, 1968) scores of 0.89 and 0.72 for intra-rater and inter-rater reliability, which showed a “substantial” to “almost perfect” rating (Wolf, 1997, p. 964).
Evaluation-2 Phase
A three-step approach was applied to evaluate evaluation-2 phase (Table 2). Similar to evaluation-1 phase, the assessment of evaluation-2 phase was conducted after all participating schools have visited the laboratory.
• Documentation of the students’ self-evaluation: We counted each box that students had ticked on their self-evaluation sheet as one point (maximal score 14 points, for description, see Table 2).
• Assessment of students’ self-evaluation sheets: We analyzed the conformity of ticked boxes on self-evaluation sheets using the respective models. Where ticks matched the models, students received one point each.
• Assessment of students’ models: We independently assessed the models. Correct features received one point each, whether or not they had been identified (maximal score 14 points).
Both assessors randomly selected 30 self-evaluation sheets, from a total of 231, and 30 models, from a total of 231, for a second scoring (13.0%, either). Cohen’s kappa coefficient (Cohen, 1968) scores of 0.90 and 0.89 for intra-rater and of 0.77 and 0.79 for inter-rater reliability showed “substantial” to “almost perfect” ratings (Wolf, 1997, p. 964).
Comparing documented boxes and assessing the self-evaluation sheets helped us determine the degree to which students were correctly evaluating the models. Lower assessment scores from students’ self-evaluation sheets indicate that students had performed poorly in their evaluations of models, yet these scores also imply that students may have documented model features that were not given. High model scores may, in contrast, reveal that students did not correctly identify existent model features.
Comparison of Students’ Evaluation Phases
To compare students’ evaluation-1 and evaluation-2 phases, we scored their sketches, with a maximum possible score of 14 points, by adding scores of their self-evaluation sheets and our assessment of their models. When compared to our model scores, scores of the recoded sketches indicate the quality of their evaluation-1 phase, while scores of their self-evaluation sheets indicate the quality of their evaluation-2 phase.
Statistical Analysis
Overall Tests
Statistical tests were conducted using SPSS Statistics 27. We applied nonparametric methods due to the non-normal distribution of variables [Kolmogorov-Smirnov test (Lilliefors modification): partially p < 0.001], and, consequently, use boxplots to illustrate our results. Intra-group differences over the three test dates were analyzed using the Friedman test (F) combined with a pairwise analysis from T0 to T1 and T2, and from T1 to T2, using the Wilcoxon (W) signed-rank test. Mann–Whitney U tests (MWU) were used to evaluate inter-group differences. To account for the use of multiple testing methods, we applied a Bonferroni correction and decreased the Alpha level to 0.017, respectively (Field, 2012). In case of significant results, effect sizes r (Lipsey and Wilson, 2001) were calculated with small (>0.1), medium (>0.3), and large (>0.5) effect sizes. For contingency analyses, we calculated the adjusted Pearson’s contingency coefficient (C; Pearson, 1904). Since Pearson’s C is a member of the r effect size family (e.g., Ellis, 2010), we also treat it as an effect size score.
Factor Analysis Model Understanding
We used a principal component analysis (PCA) with subsequent varimax rotation, which reduces the data’s dimensionality while retaining its variation (Bro and Smilde, 2014), to evaluate the shortened SUMS scale. Following the Kaiser-Guttman-Criterion (Kaiser, 1970), it divided into two factors: the Kaiser-Meyer-Olkin (KMO = 0.80, χ2 = 599.2) values being acceptable to good, indicating that conducting a factor analysis with our dataset was feasible. The analysis of the scale’s applicability with PCA of seven items from the SUMS questionnaire, involving varimax rotation, resulted in two factors based on eigenvalues >1.0. The Kaiser–Meyer–Olkin measure verified the sampling adequacy (KMO = 0.796), which is well above the acceptable lower limit of 0.5 (Field, 2012). Bartlett’s test of sphericity (χ2 = 599.224, p < 0.001) indicated that correlations between items were sufficient for performing a PCA. Examination of the Kaiser–Guttman criterion yielded empirical justification for the retention of two factors (Figure 1), which explained 63.69% of the total variance.
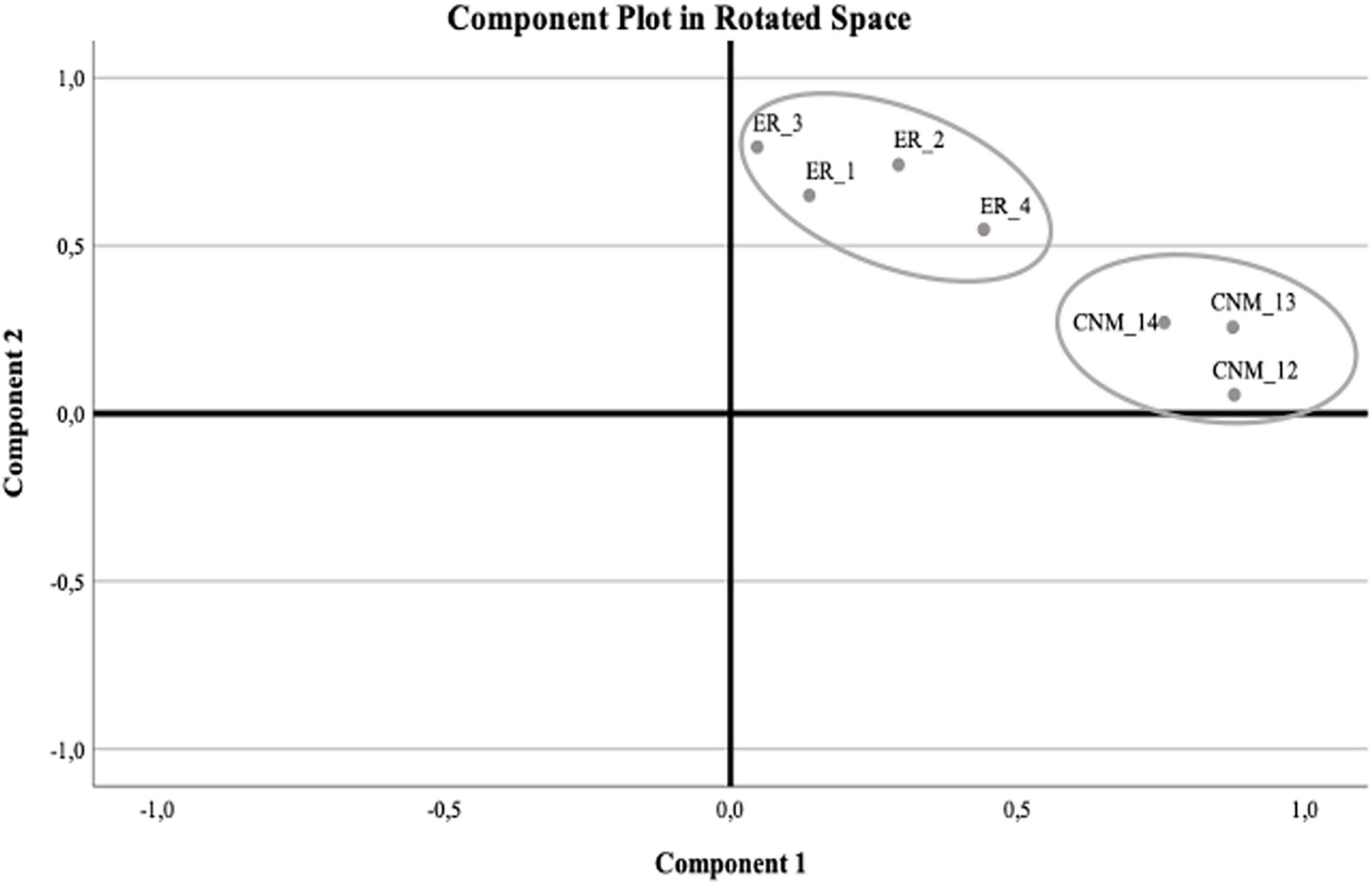
Figure 1. Component plot in rotated space after principal component analysis with clearly distinct subscales “models as exact replicas” (ER) and “changing nature of models” (CNM).
Results
We first provide an overview of our intra-group and inter-group analyses with regard to students’ knowledge of “DNA as a model” and model understanding. This overview is followed by a detailed assessment of the evaluation-1 and evaluation-2 phases.
Influence of Content and Language Integrated Learning Module on Students’ Knowledge of “DNA as a Model”
Intra-Group Analyses of Students’ Knowledge of “DNA as a Model”
Analyses revealed changes for both non-CLIL and CLIL learners’ scores [F: χ2non–CLIL/CLIL (2, n = 149/316) = 202.94/114.71, p < 0.001]. With each approach, knowledge initially increased before dropping between T1 and T2, but not below levels of T0 (Figure 2; WT0/T1: non-CLIL/CLIL Z = 0-10.07/–8.64; p < 0.001; WT0/T2: non-CLIL/CLIL Z = –9.77/–7.43, p < 0.001; WT1/T2: non-CLIL/CLIL Z = –8.06/–2.62, p < 0.001/ = 0.009). This suggests that students gained short-term and mid-term knowledge of “DNA as a model” throughout the intervention.
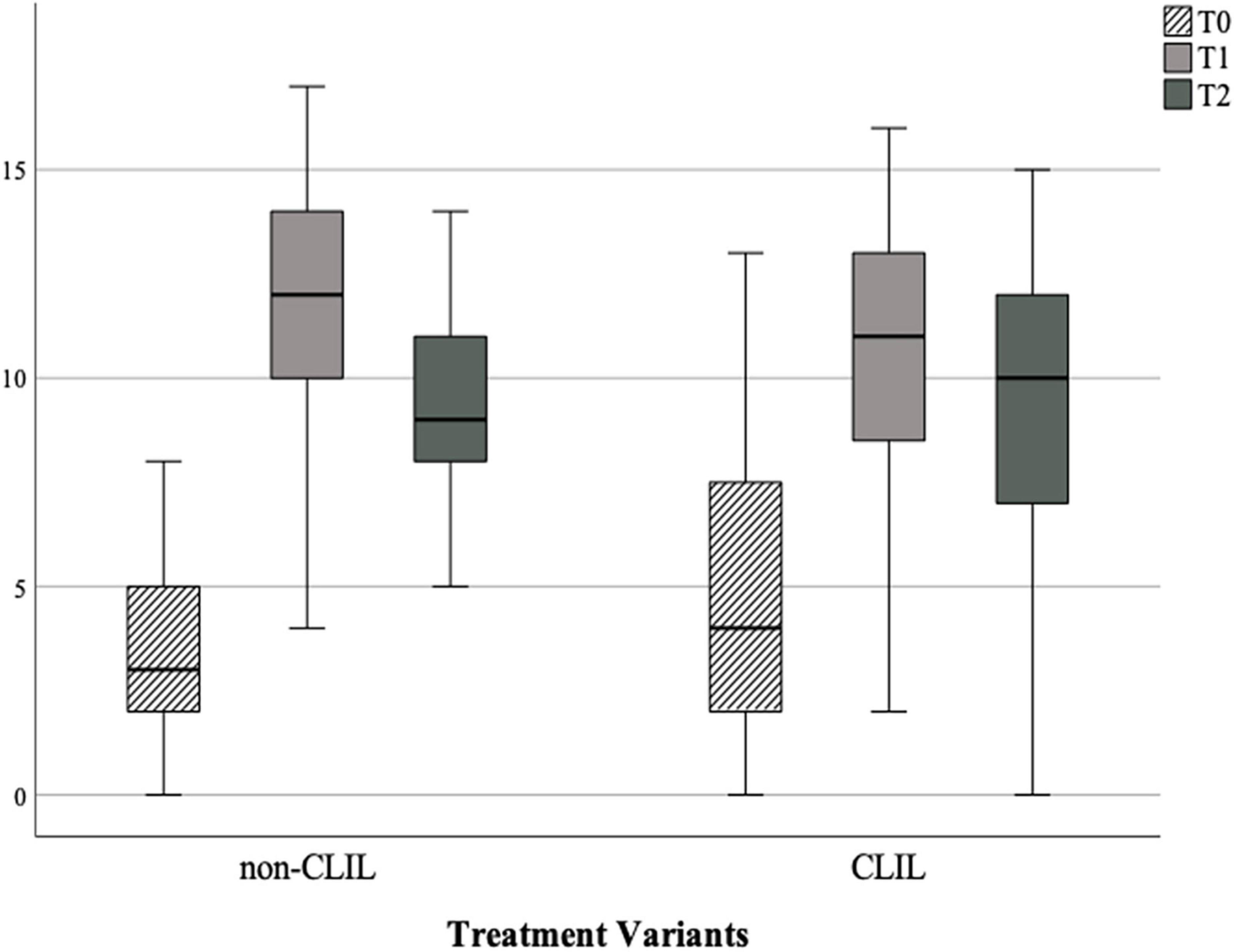
Figure 2. Differences between non-CLIL and CLIL learners for knowledge of “DNA as a model” at the three different testing times.
Inter-Group Analyses of Students’ Knowledge of “DNA as a Model”
Non-CLIL and CLIL students started with similar scores (T0, Figure 2; MWU: Z = –2.27; p = 0.023). After the intervention, with a small-to-medium effect, non-CLIL students scored higher than CLIL students (T1, Figure 2; MWU: Z = –2.47; p = 0.013; r = 0.218). In the follow-up test, scores of both treatment variants decreased to similar levels (T2, Figure 2; Z = –2.09; p = 0.036). Yet, participants in both groups increased their understanding of the “DNA as a model” based on the knowledge obtained throughout the different experimental steps and modelling activities.
Influence of the Content and Language Integrated Learning Module on Students’ General Understanding of Models and Modelling
Inter-Group Analysis Model Understanding
To account for differences in students’ understanding of models with regard to ER and CNM between non-CLIL and CLIL learners, we calculated mean scores for all testing times. Starting at similar levels (T0, MWU: Z = –1.750, p = 0.80), we found differences for T1 (MWU: Z = –7.845, p < 0.001, r = 0.513) and T2 (MWU: Z = –8.339, p < 0.001, r = 0.543) regarding CNM (Figure 3, left part) and ER (Figure 3, right part) between non-CLIL and CLIL learners with large effect sizes (Lipsey and Wilson, 2001).
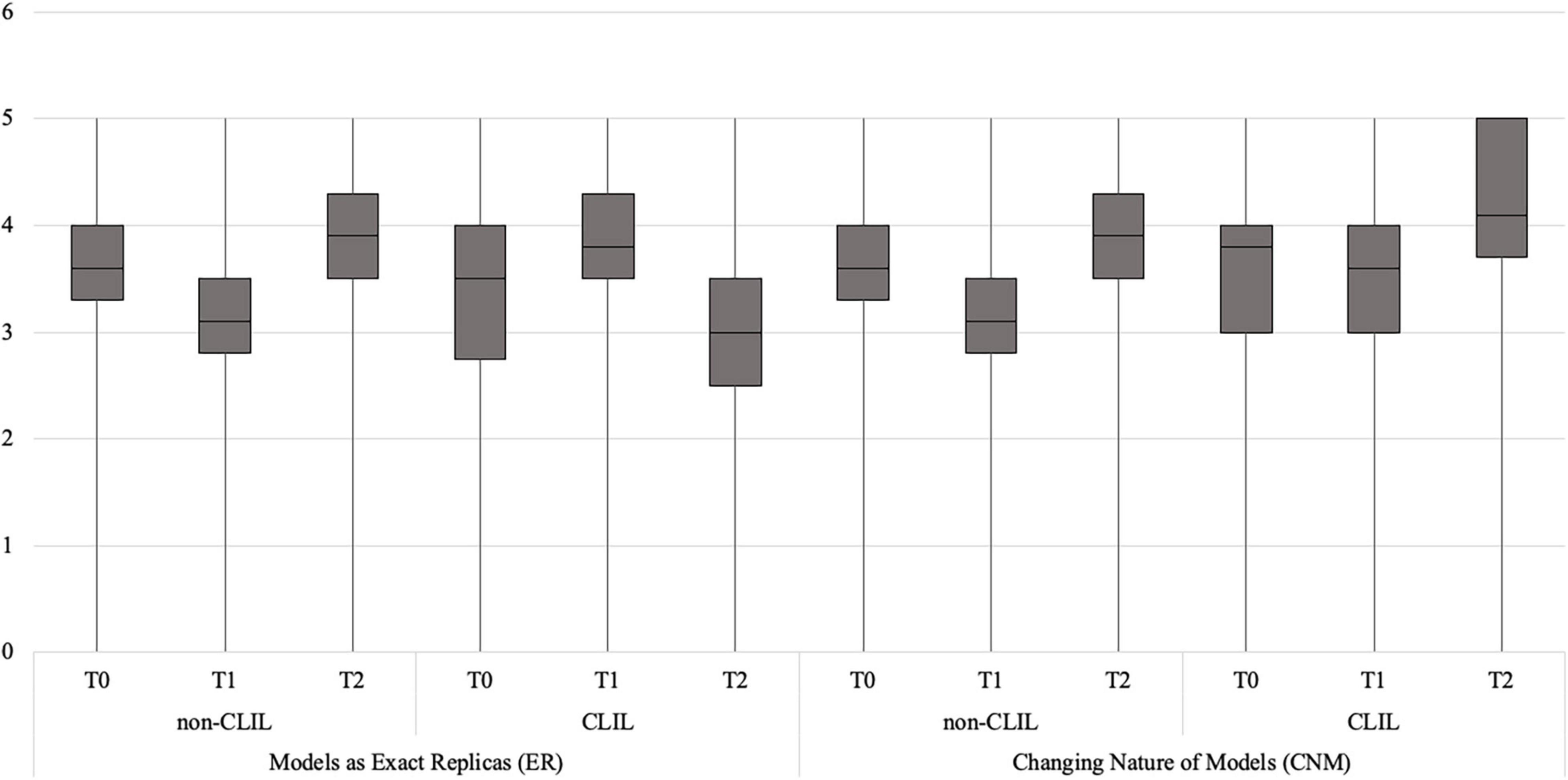
Figure 3. Differences between non-CLIL and CLIL learners in “models as exact replicas” and “changing nature of models” across testing points.
Intra-Group Analysis Model Understanding
Analyses revealed changes for non-CLIL and CLIL learners for model understanding [F: χ2non–CLIL/CLIL (5, n = /149316) = 152.41/125.94, p < 0.001]. Non-CLIL learners changed their notions of models from ER to CNM after participation (T1) but returned to ER after another 6 weeks (T2). Among CLIL learners, in contrast, perception of models as ER was reinforced by participation (T1) but changed to CNM after a 6 week reflection phase (T2) (Figure 3; WT0/T1: non-CLIL/CLIL ER Z = –4.74/–4.71; p < 0.001; WT1/T2: non-CLIL/CLIL ER Z = –7.90/–7.06, p < 0.001; WT0/T2: non-CLIL/CLIL ER Z = –2.96/–4.54, p = 0.003/ p < 0.001; WT0/T1: non-CLIL/CLIL CNM Z = –2.42/–1.65; p = 0.015/ p = 0.098; WT1/T2: non-CLIL/CLIL CNM Z = –6.60/–5.39, p < 0.001; WT0T2: CLIL/CLIL CNM Z = 2.91/–4.46, p = 0.004/ p < 0.001). Thus, the long-term effect of participation on mode-understanding yielded more desirable results for CLIL learners despite the initial retention of ER.
Influence of the Content and Language Integrated Learning Module on Students’ Ability to Evaluate Models
Assessment of Evaluation-1 Phase
We compared sketches of both language variants and identified significantly better results with a medium effect size (MWU: Z = –6.574, p < 0.001, r = 0.304; for details, see Supplementary Datasheet 3) for the non-CLIL approach (Figure 4).
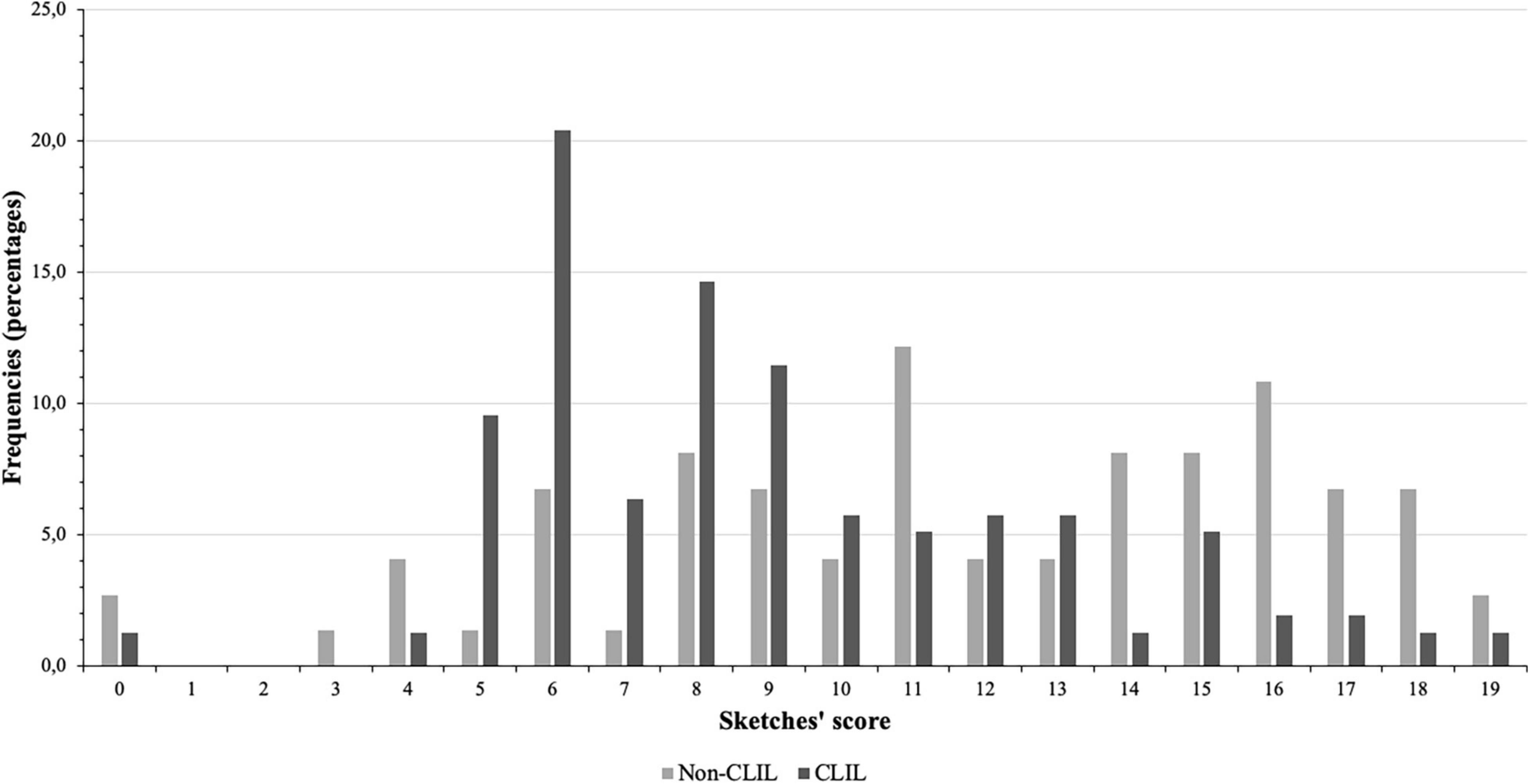
Figure 4. Category frequencies after analyses of students’ responses to the open questions for both non-CLIL and CLIL learners.
Comparing the two treatment groups in terms of category frequencies and students’ responses to the open questions, we found differences for both questions (Figure 5; C = 0.426 and C = 0.445, p < 0.001 in both cases). In their answers to the question “Which features of the original DNA molecule are simplified in your model,” CLIL learners focused almost entirely on the structural level category (C = 0.335, p < 0.001) while non-CLIL learners also pointed to the categories DNA and particle levels (C = 0.427/0.211, p < 0.001).
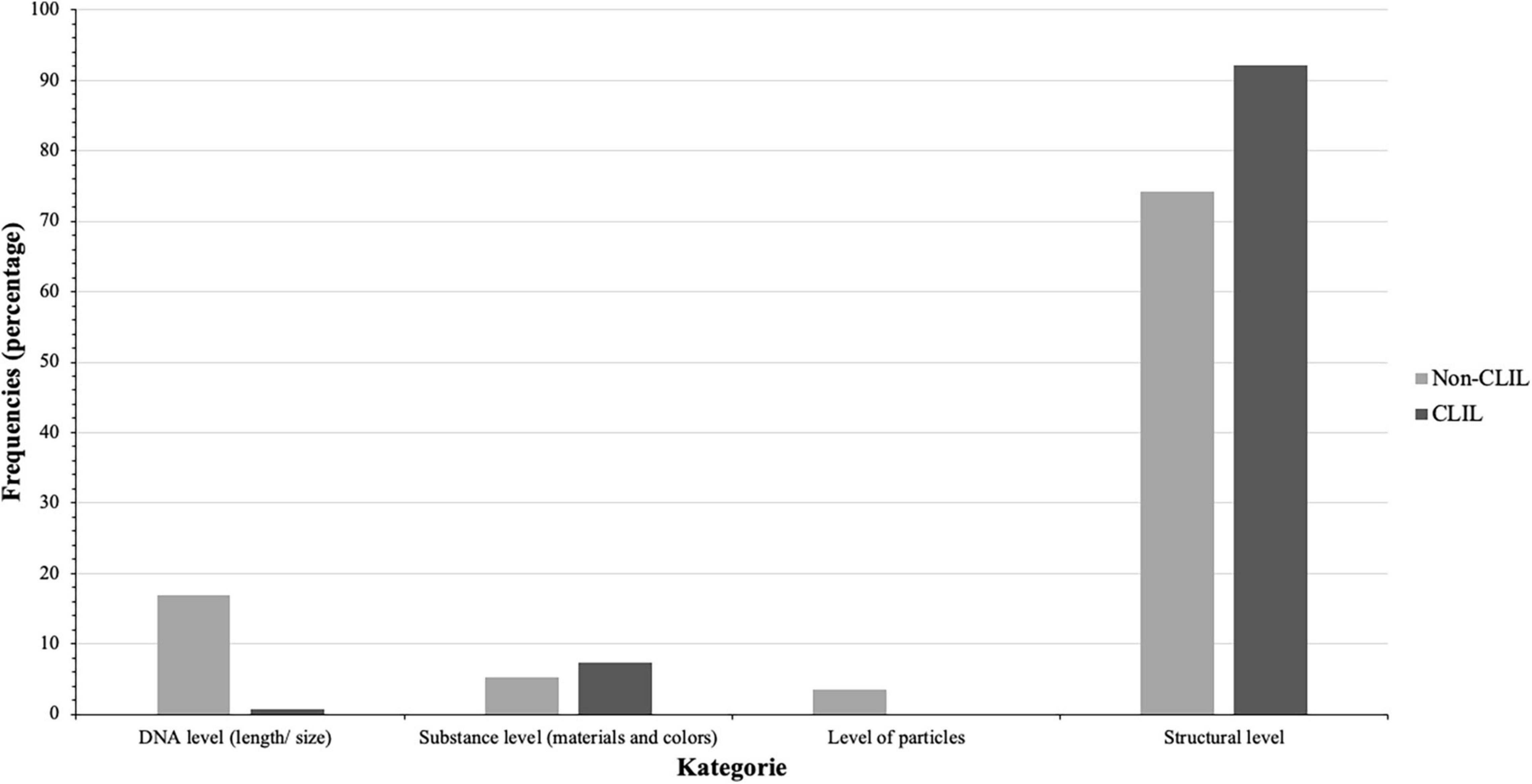
Figure 5. Category frequencies of the open question “Which features of the original DNA molecule are simplified in your model” for non-CLIL and CLIL learners.
In their answers to the question “Explain why one might create different models of one biological original (in our case, the structure of the DNA),” non-CLIL learners commonly pointed to the category Individuality of DNA (C = 0.372, p < 0.001) while CLIL learners focused instead on different research states as explanations for different DNA models (C = 0.322, p < 0.001). That is, CLIL learners focused on CNM also in the open questions while non-CLIL learners pointed to more phenomenon-related reasons (Figure 6).
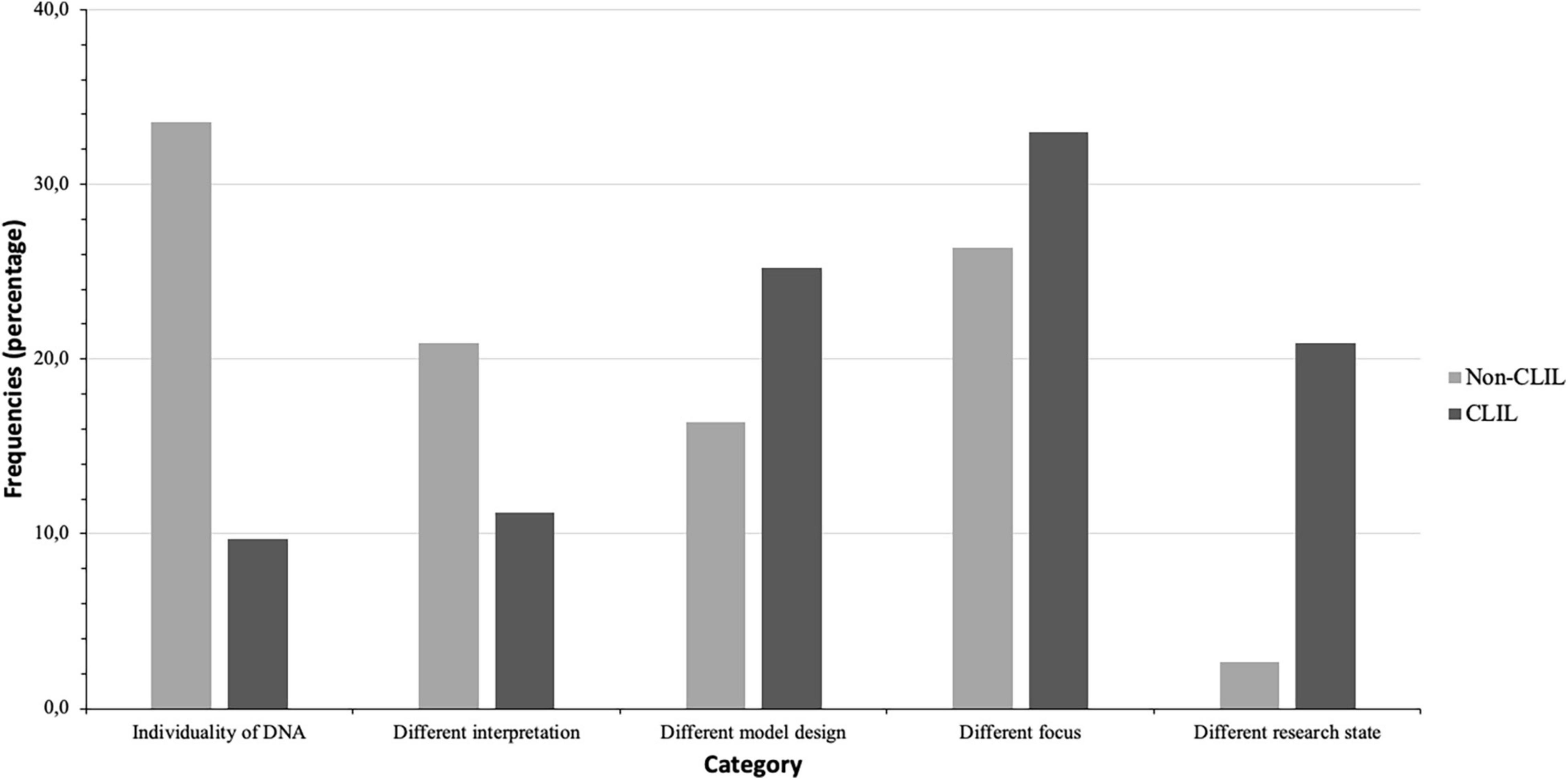
Figure 6. Category frequencies of the open question “Explain why one might create different models of one biological original (in our case, the structure of the DNA)” for non-CLIL and CLIL learners.
Assessment of Evaluation-2 Phase
We compared students’ self-evaluation sheets, our assessment of their sheets, and our assessment of their models. For non-CLIL learners, we identified higher scores both for the assessment of students’ self-evaluation sheets (MWU: Z = –3.711, p < 0.001; r = 0.171, small-to-medium effect) and for the assessment of their models (MWU: Z = –8.576, p < 0.001; r = 0.396, medium-to-large effect). Students’ self-evaluation sheets did not differ significantly (MWU: Z = –0.893, p = 0.372; see Figure 7 and for details Table 3).
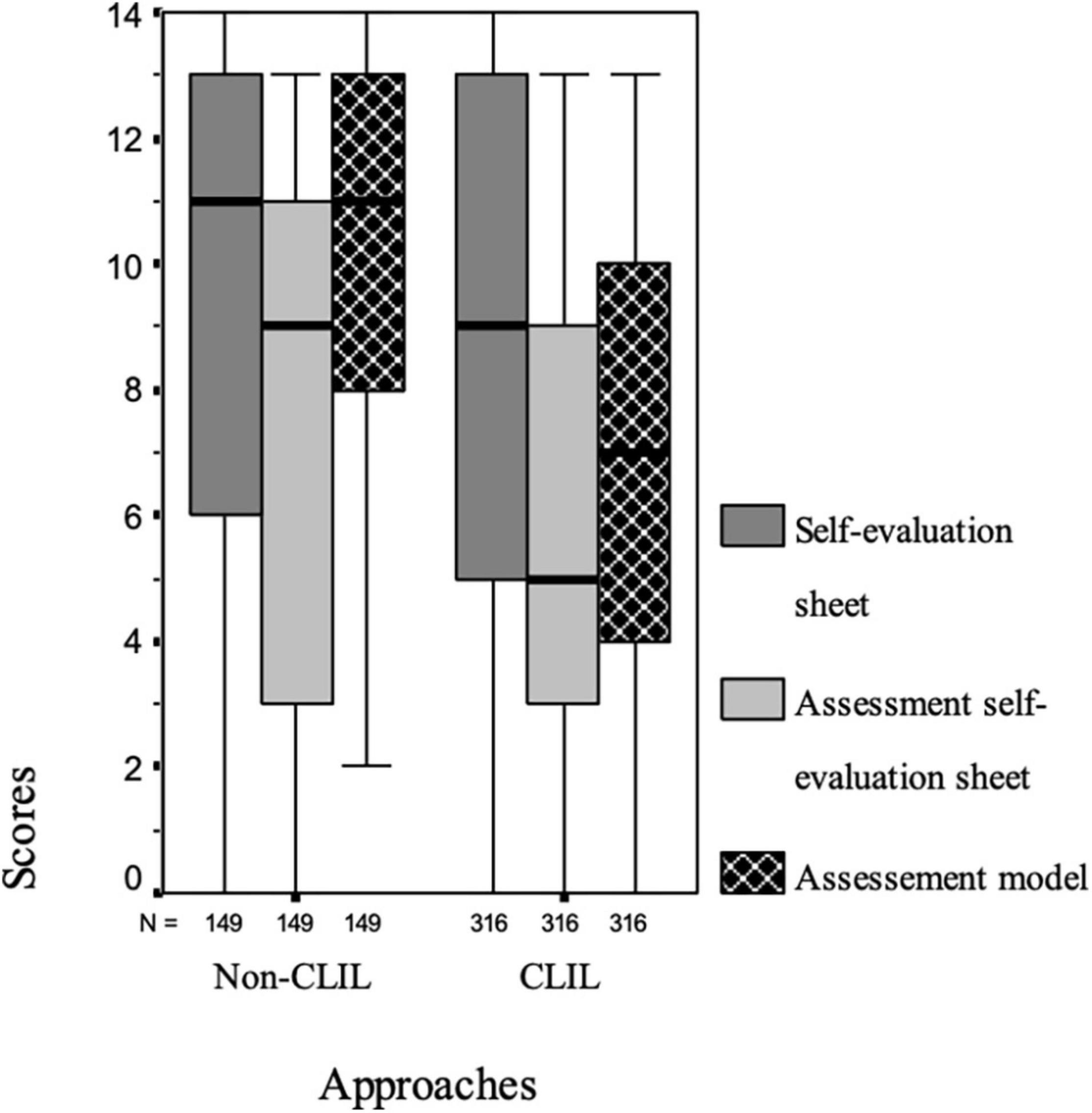
Figure 7. Differences between non-CLIL and CLIL learners in scores for self-evaluation sheets, subsequent model assessment and reassessment of the students’ self-evaluation sheets.
Intra-group analyses of both treatment variants indicated similar differences between students’ self-evaluations and our assessment of their self-evaluation sheets and assessment of their model [Table 3; F: χ2non–CLIL/CLIL (2, n = 149/316) ≥ 121.68, p < 0.001]. The pairwise analysis revealed lower scores for our assessment of their self-evaluation sheets compared to their own self-evaluated scores, with large effects (W: Z ≤ -8.818, p < 0.001; r ≥ 0.722). Thus, students identified features as correct that were not given in their model. This discrepancy was evident across all analyses of all sections in both treatment variants [except for the section primary structure; Table 3; F: χ2non–CLIL/CLIL (2, n = 149/316) ≥ 16.92, p < 0.001]; W: Z ≤ -2.762, p ≤ 0.006, r ≥ 0.326 medium-to-large effects). In consequence, students believed to build better models than they did.
In addition to these beliefs, the assessed models scored higher than the assessed self-evaluation sheets (W: Z ≤ -8.280, p < 0.001; r ≥ 0.504, medium-to-large effect). That is, students did not identify all the correctly modelled features. This phenomenon was also evident across all analyses of sectors in both treatment variants [except for the sector bases and secondary structures in the CLIL variant; Table 3; F: χ2non–CLIL/CLIL (2, n = 149/316) ≥ 16.92, p < 0.001]; W: Z ≤ -3.191, p ≤ 0.001, in each case; r ≥ 0.180, small-to-medium effects]. Thus, students also built better models than they believed.
Comparison of Evaluation-1 and Evaluation-2 Phases
In both language variants, we compared the scores of recorded sketches, which represent the quality of evaluation-1 phase, and the scores of students’ self-evaluation sheets, which reflect the quality of their evaluation-2 phase, with our model scores (Figure 8).
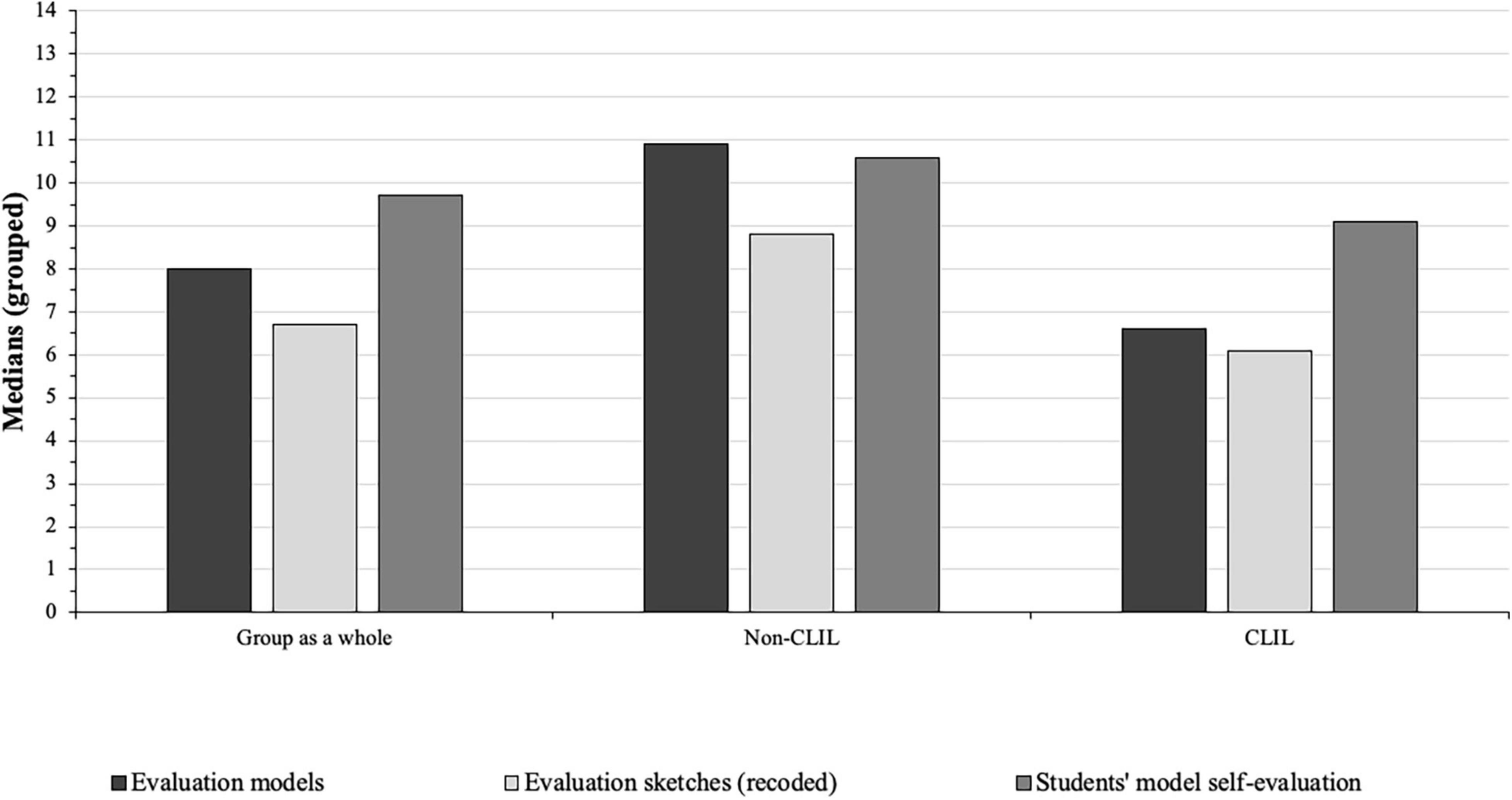
Figure 8. Differences between non-CLIL and CLIL learners for model evaluation, recoded evaluation of students’ sketches and students’ model self-evaluation.
Non-CLIL Learners
Intra-group analyses of non-CLIL students revealed differences between our model scores and the scores for students’ sketches (recoded) and the students’ self-evaluation sheets [F: χ2non–CLIL (2, n = 149) = 10.79, p < 0.001]. Pair-wise analysis revealed lower scores for their sketches (recoded) compared to the model scores (W: Z = –4.051, p < 0.001; r = 0.332, medium effect), but not for their self-evaluation sheets (W: Z = –0.824, p = 0.410). That is, non-CLIL students performed better in evaluation-2 phase than in evaluation-1 phase.
CLIL Learners
Intra-group analyses of CLIL learners revealed differences between our model scores, the scores of students’ sketches (recoded), and their self-evaluation sheets [F: χ2CLIL (2, n = 316) = 109.28, p < 0.001]. Pair-wise analysis displayed similar scores for students’ sketches (recoded) as compared to the model scores (W: Z = –0.352, p = 0.725), but higher scores for their self-evaluation sheets (W: Z = –8.100, p < 0.001, r = 0.456, medium-to-large effect). That is, CLIL learners outperformed non-CLIL learners in evaluation-1 phase. However, CLIL learners also identified features as correct that were not given in their model.
Discussion
Our 1-day outreach module had a positive effect on knowledge of “DNA as a model,” model-understanding, and model evaluation, regardless of non-CLIL or CLIL implementation. Yet, non-CLIL learners outperformed CLIL learners regarding knowledge of “DNA as a model” and model evaluation-2 phase while CLIL learners outperformed non-CLIL learners regarding model-understanding and model evaluation-1 phase. The latter could be explained by the students’ discipline-specific scientific practices involving hands-on and minds-on activities in combination with scientific discourse. This may have led to a deeper understanding of the represented scientific content and models in science (Schwarz et al., 2009), and may also have contributed to scientific literacy (Ke et al., 2020). While the CLIL approach may have triggered higher cognitive involvement (Tagnin and Ní Ríordáin, 2021), it could have also overburdened the mental capacity of students (Grandinetti et al., 2013; Tolbert et al., 2019), explaining the results of the non-CLIL as compared to the CLIL treatment group.
Content Knowledge and Understanding
The influence that our CLIL science module had on content knowledge and understanding regarding “DNA as a model” (RQ1: How does a 1-day CLIL science module influence students’ knowledge of “DNA as a model” throughout the hands-on laboratory?) throughout the hands-on laboratory was in keeping with our previous findings (Roth et al., 2020). That is, non-CLIL learners outperformed CLIL learners regarding short-term knowledge acquisition. We propose that the increased mental load from both content and language learning may have influenced students’ performance. That is, the mental processing of scientific content in a foreign language, accompanied by hands-on experimentation and modelling and model evaluation activities, may have overstrained students’ mental capabilities (Rodenhauser and Preisfeld, 2015; Sweller, 2015). Yet, it mirrors an authentic setting in science classrooms in connecting with the increasing adoption of CLIL outside of English-speaking countries.
Although the performance of the CLIL learners was poorer as compared to non-CLIL learners, there was a significant knowledge acquisition that could be sustained both temporarily and permanently. This confirms studies of, for instance, Campillo-Ferrer and Miralles-Martínez (2022). While the comparably higher mental effort involved in learning scientific content in a foreign language may have influenced overall performance (Rodenhauser and Preisfeld, 2015; Sweller, 2015), the discursive activities—as observed and encouraged by the instructor–involved in the construction and negotiation of an appropriate model may have led to deep-learning (Krell et al., 2015; Ke and Schwarz, 2020; Ke et al., 2020) of information relevant to the design of the DNA model. Thus, the combination of hands-on tasks with minds-on activities in CLIL learning (Glynn and Muth, 1994; Satayev et al., 2022) may have conveyed knowledge of “DNA as a model,” while the negotiation of meaning involved in the process of talking about science could have encouraged retention of this knowledge (Evnitskaya and Morton, 2011). That is, the various scaffolding exercises, which had to be solved in group-work, may have encouraged more and deeper discussion of the DNA-related experiments and ensuing construction of a three-dimensional DNA model (Ke and Schwarz, 2020; Ke et al., 2020).
Model Understanding
The influence that our CLIL science module had on overall model-understanding, compared to the non-CLIL approach (RQ2: How does CLIL influence students’ general understanding of models and modelling?) supports our assumption that the incorporation of scientific discourse into model negotiation and model construction, induced by more extensive scaffolding exercises, encourages deep-learning. In particular, students displayed a significant increase in their awareness for CNM and a respective decrease in the misconception of EM after participation in our CLIL science module. They even answered open questions in accordance with CNM before the post-test was completed. This is in stark contrast to the widespread yet erroneous notion among students, that models are exact copies of real-life phenomena that only differ across time and do not change in response to new evidence (Grosslight et al., 1991; Ke and Schwarz, 2020). In fact, students are largely unaware of the importance of evidence and the constant negotiation of a models’ validity by peer scientists (Treagust et al., 2002; Mendonça and Justi, 2013; Ke et al., 2020). Since the findings of our non-CLIL approach reflect the hypothesized lack of model-understanding—students still perceived models as ER after participation–our CLIL science module appears to have compensated for this lack.
One key aspect that may have influenced model-understanding is discursive action (Passmore and Svoboda). This also includes the analysis and interpretation of data and the re-evaluation of models, if required (Ke et al., 2020). As such, critical reflection of models and the therewith associated re-construction of certain elements may have contributed to CNM (Schwarz et al., 2009). The increased discursive action may have also led to the development of epistemological understanding of modelling processes, hence encouraging deeper cognitive processing of their material (Sins et al., 2009). Yet, discourse and model evaluation were elements of our previous non-CLIL laboratory. Since our participating students were not yet fully fluent in English, and only started developing their scientific literacy, they had to re-read, re-think, and extensively discuss their mental models before they proceeded with construction. This led to several cycles of mental rehearsal (Gilbert and Justi, 2002; Khan, 2011; Oh and Oh, 2010), in the process of which the students could have slowly become aware of the changing nature of models, dependent on the information (Treagust et al., 2002) they extracted from their manuals and the text about the discovery of DNA (Usher, 2013).
This is, of course, only one possible explanation, but it is supported by theories about the development of scientific literacy and associated deep learning. Often, models are simply regarded as representations of a phenomenon and not as a “sense-making tool for considering how and why patterns and mechanisms of phenomena occur” (Ke and Schwarz, 2020, p. 2). Finding answers to the “how” and “why” may move modelling away from purely content-based learning and may focus more on the practice of science. This could have encouraged students to engage in proposing scientific hypotheses and finding scientific arguments to justify these hypotheses. Not only may this stimulate in-depth exploration of the subject at hand, which encourages the development of scientific literacy (France, 2019; Quarderer and McDermott, 2020; Virida, 2021), but it may also promote deep-learning (Lee et al., 2015; Piacentini et al., 2022). Based on the dual-coding theory by e.g., Clark and Paivio (1991) and context-availability method by e.g., Aslandag and Yanpar (2014) both the verbal and non-verbal code embedded into a larger context are believed to mediate the understanding of difficult information and the uptake of contextualized vocabulary (Fernández-Fontecha et al., 2020). That is, “deep-level processing” of the given information is required (Case and Gunstone, 2002, p. 461), which, it seems, our non-CLIL approach did not achieve to the same degree as our CLIL module.
For deep-level processing, students should also understand what they are reasoning about. Since the language of science differs from the language used in everyday settings (Wang and Chen, 2014; Piacentini et al., 2022), scientific literacy in the respective science discipline is indispensable (Prain, 2004). This not only includes vocabulary and hands-on activities but also minds-on activities. Such minds-on activities include the explanation and abstraction of scientific content (Glynn and Muth, 1994). Although minds-on activities were likewise included in our non-CLIL module in the form of open questions in the laboratory manuals encouraging students to summarize observations, we further developed these activities in our CLIL module. As students were still learning English as their second language, we provided additional scaffolding material in the form of a language workbook and tried to ensure that the students had understood the content of our laboratory by encouraging them to summarize the procedures in their own words. We also extended this method to the modelling phases, which is why the purpose of a model may have probably been more clearly understood than in the non-CLIL module. That is, the “verbal representation” of a model may be at least as important as its physical manifestation in explaining and justifying the proposed model (Campbell and Fazio, 2020, p. 2302). Yet, in classroom teaching, such representation of mental models, including the critiquing, revising, and enriching of mental models is often neglected. Specifically, the involvement of teachers to create models from scientific discourse appears to be a key element to model-understanding and modelling (Khan, 2011).
Model Evaluation
The influence that our CLIL science module had on overall model evaluation-1 and model evaluation-2 as compared to the non-CLIL treatment group [RQ3: How does CLIL influence students’ ability to evaluate models—specifically, the two implemented evaluation phases (evaluation-1 and evaluation-2)?] mirrors our findings on knowledge of “DNA as a model.” Non-CLIL learners received higher scores for their model sketches and hand-crafted models and outperformed CLIL learners in model evaluation-2. The better overall performance of non-CLIL learners in model construction, in their sketches and in evaluation-2 may—much like content knowledge and understanding of the scientific concept—be attributed to the increased mental load from both content and language learning for the CLIL learners (Sweller, 2015). As has previously been outlined, the practice of modelling requires an understanding of the content and an extensive period of negotiation (Gilbert and Justi, 2002; Passmore and Svoboda, 2012). The lack of language proficiency in English may, thus, have influenced students’ performance and contributed to an overload of mental demands from the combination of content and language learning (Johnstone and Wham, 1979).
In both non-CLIL and CLIL treatment variants, some students correctly identified and labelled the DNA’s different components, some students identified only a limited number, and some only modelled the DNA’s basic structure. This result is in line with the findings of previous studies (Howell et al., 2019), wherein the researchers described difficulties in students’ understanding of DNA’s structure-function relationships. We also reported such findings in a comparative study of non-CLIL outreach modules (Roth et al., 2020).
Analysis of answers regarding model-related questions on the students’ worksheet of model evaluation-1 may indicate the increased model-understanding of CLIL learners. Sample answers showed that CLIL learners focused, in particular, on the “structural level of DNA” and “state of research” as determinants of how such models are being developed. This may demonstrate a broad understanding of “DNA as a model” and the realization that models are not exact representations of observed phenomena but may change dependent on emerging evidence (Grosslight et al., 1991; Ke and Schwarz, 2020). This awareness is in stark contrast to typical notions among students of the nature of models (Schwarz et al., 2009; Krell et al., 2015). Similar to overall model-understanding, the students’ lack of fluency in English and their developing scientific literacy may have led to more intense re-reading, re-thinking, and extensive discussion of their mental models before they proceeded with their construction. This could have induced several cycles of mental rehearsal (Gilbert and Justi, 2002; Oh and Oh, 2010), which expedited the awareness of CNM (Treagust et al., 2002). Findings from evaluation-1, wherein students were asked to draw sketches of their hand-crafted models, supports this theory. Although the models were certainly not as exact as those built by non-CLIL learners, CLIL learners designed sketches that were far more accurate and labelled components of their models accordingly. This task may demand a deeper model-understanding than simply comparing hand-crafted models to a commercial DNA model and ticking features on a pre-designed list, as was the case in evaluation-2 where non-CLIL learners outperformed CLIL learners. Hence, CLIL learners may not only have understood the importance of CNM but also may have known the function and place of components that they crafted in their models. Yet, further qualitative analysis of observations and more open questions may be required to confirm these first indications.
Limitations
Firstly, our study involved ninth-graders, who had little to no prior experience in hands-on experimentation, modelling, and model evaluation. Secondly, the students had little real-life experience of English outside English language lessons, as we learned from conversations with students and teachers. Thirdly, due to Bonferroni corrections, comparisons of T0 and T2 knowledge scores were slightly above the reduced threshold of significance. Therefore, we cannot exclude a potential beta error. However, an additional comparison of different variables only fortified the higher short-term achievement of non-CLIL learners. A lack of commonly agreed standardized instruments for assessing CLIL content learning, which, in our case, also extends to modelling and model evaluation, may impair adequate comparison (Dalton-Puffer, 2007). Fourthly, due to the context-dependency of CLIL learning, results cannot easily be extrapolated (Pérez-Cañado, 2012). As a consequence, generalizing about the success of CLIL learning requires an acknowledgments of the diversity of possible CLIL implementations. Further research in the context of short-term implementations of CLIL in combination with model-related learning is required to pinpoint key challenges and consider possible means to increase its success. That is, the implementation of long-term modules as investigated by, for instance Meyerhöffer and Dreesmann (2019) and confirmed by Haagen-Schützenhöfer et al. (2011), show that the positive outcomes of our module, such as model-understanding, could be enhanced while negative outcomes related to CLIL instruction, such as content knowledge, could be levelled out. Yet, other short-term CLIL modules by, for instance, Rodenhauser and Preisfeld (2015) did not produce significant differences between CLIL and non-CLIL participants. For further implementations, we should consider reducing the extraneous load (Chandler and Sweller, 1991), by providing more scaffolding materials and spreading the module over two consecutive days. This way, and in line with Meyerhöffer and Dreesmann (2019) and Craik and Lockhart (1972) levels of processing theory, CLIL students should succeed and even outperform non-CLIL students also regarding content knowledge, and model building as well as evaluation. Moreover, the inclusion of qualitative discourse analysis in further developments of the module could shed more light on the importance of talking about science in the creation of knowledge and model-understanding.
Conclusion
Our study furthers understanding of the relationship between CLIL learning and model-understanding, which encompasses aspects of language learning and scientific literacy (e.g., Prain, 2004), content learning and language learning (e.g., Stoddart et al., 2002; Gonzalez-Howard and McNeill, 2016), modelling and content learning (e.g., Schwarz et al., 2009; Lee et al., 2015), as well as modelling and scientific literacy (e.g., Ke et al., 2020; Quarderer and McDermott, 2020). While most previous studies have focused, primarily, on only one of these combinations, our module encompasses aspects of them all. Moreover, our study explores the potential of short-term CLIL modules, rather than the long-term CLIL modules more commonly explored by other researchers (Meyerhöffer and Dreesmann, 2019).
Although the CLIL treatment group received overall lower scores than non-CLIL learners, CLIL outreach learning holds the potential to improve model understanding. As the development of model understanding is rather cumbersome, and stimulating environments are difficult to identify (Glynn and Muth, 1994; Schwarz et al., 2009), our CLIL module could provide a possible approach by combining hands-on laboratory experiments with language learning and associated minds-on activities. Although we cannot identify the reasons for lower scores among the CLIL group, we would—in line with cognitive load theory (Sweller, 2015)—encourage the reduction of content in later implementations.
Data Availability Statement
The data are not publicly available due to potentially identifiable information that could compromise the privacy of research participants. The data that support the findings of this study are available on request from the corresponding author (TR).
Ethics Statement
The studies involving human participants were reviewed and approved by Bavarian Ministry of Education and Cultural Affairs (Process number: IV.7-BO5106/149/18). Written informed consent to participate in this study was provided by the participants’ legal guardian/next of kin.
Author Contributions
TR: conceptualization of the CLIL-module based on four previous laboratory interventions and questionnaire, development of scaffolding exercise books tailored to the needs of the CLIL laboratory, investigation, data curation, validation, visualization, and analyses of empirical data, and writing of the original draft. F-JS: formal analysis, visualization, and validation of empirical data and writing of the original draft. FB: supervision and project administration. All authors contributed to the article and approved the submitted version.
Funding
The funding of the research was a joint endeavor of the University of Bayreuth together with the national BMBF’s “Qualitätsoffensive Lehrerbildung” (#01JA1901) and the Horizon 2020 Framework Program CREATIONS (grant number 665917), as well as the Luxembourg National Research Fund FNR and PayPay (P17/IS/13342933/PayPay-FNR/Chair in DFS/Gilbert Fridgen).
Conflict of Interest
The authors declare that the research was conducted in the absence of any commercial or financial relationships that could be construed as a potential conflict of interest.
Publisher’s Note
All claims expressed in this article are solely those of the authors and do not necessarily represent those of their affiliated organizations, or those of the publisher, the editors and the reviewers. Any product that may be evaluated in this article, or claim that may be made by its manufacturer, is not guaranteed or endorsed by the publisher.
Supplementary Material
The Supplementary Material for this article can be found online at: https://www.frontiersin.org/articles/10.3389/feduc.2022.922414/full#supplementary-material
References
Akerson, V. L., Townsend, J. S., Donnelly, L. A., Hanson, D. L., Tira, P., and White, O. (2009). Scientific modeling for inquiry teachers network (SMIT’N): the influence on elementary teachers’ views of nature of science, inquiry, and modeling. J. Sci. Teachers Educ. 20, 21–40. doi: 10.1007/s10972-008-9116-5
Aslandag, B., and Yanpar, T. (2014). Dual-coding versus context-availability: quantitive and qualitative dimensions of concreteness effect. Proc. Soc. Behav. Sci. 116, 4814–4818. doi: 10.1016/j.sbspro.2014.01.1030
Bos, W., and Tarnai, C. (1999). Content analysis in empirical social research. Int. J. Educ. Res. 31, 659–671. doi: 10.1016/S0883-0355(99)00032-4
Boulter, C. J. (2000). “Language, models and modelling in the primary science classroom,” in Developing Models in Science Education, eds J. K. Gilbert and C. J. Boulter (Dordrecht/Boston/London: Kluwer Academic Publishers), 289–305. doi: 10.1007/978-94-010-0876-1_15
Bro, R., and Smilde, A. K. (2014). Principal component analysis. Anal. Methods 6, 2812–2831. doi: 10.1039/C3AY41907J
Campbell, T., and Fazio, X. (2020). Epistemic frames as an analytical framework for understanding the representation of scientific activity in a modeling-based learning unit. Res. Sci. Educ. 50, 2283–2304. doi: 10.1007/s11165-018-9779-7
Campillo-Ferrer, J.-M., and Miralles-Martínez, P. (2022). Primary school teachers’ perceptions of the level of development of low-order cognitive skills under the content and language integrated learning approach. Front. Educ. 7:815027. doi: 10.3389/feduc.2022.815027
Canz, T., Piesche, N., Dallinger, S., and Jonkmann, K. (2021). Test-language effects in bilingual education: evidence from CLIL classes in Germany. Learn. Instruct. 75:101499. doi: 10.1016/j.learninstruc.2021.101499
Case, J., and Gunstone, R. (2002). Metacognitive development as a shift in approach to learning: an in-depth study. Stud. Higher Educ. 27, 459–470. doi: 10.1080/0307507022000011561
Chandler, P., and Sweller, J. (1991). Cognitive load theory and the format of instruction. Cogn. Instruct. 8, 293–332. doi: 10.1207/s1532690xci0804_2
Cheshire, J., and Gardner-Chloros, P. (1998). Code-switching and the sociolinguistic gender pattern. Int. J. Sociol. Lang. 129, 5–34. doi: 10.1515/ijsl.1998.129.5
Clark, J. M., and Paivio, A. (1991). Dual coding theory and education. Educ. Psychol. Rev. 3, 149–210. doi: 10.1007/BF01320076
Cohen, J. (1968). Weighted kappa: nominal scale agreement provision for scaled disagreement or partial credit. Psychol. Bull. 70, 213–220. doi: 10.1037/h0026256
Cook, T. D., and Campell, D. (1979). Quasi-experimentation. Design & Analysis Issues for Field Settings. Chicago, IL: Rand McNally College Publishing Company.
Coyle, D., and Meyer, O. (2021). Beyond CLIL. Pluriliteracies Teaching for Deeper Learning. Cambridge: Cambridge University Press. doi: 10.1017/9781108914505
Craik, F. I. M., and Lockhart, R. S. (1972). Levels of processing: a framework for memory research. J. Verbal Learn. Verbal Behav. 11, 671–684. doi: 10.1016/S0022-5371(72)80001-X
Dalton-Puffer, C. (2007). Discourse in Content and Language Integrated Learning (CLIL) Classrooms. Amsterdam: John Benjamins. doi: 10.1075/lllt.20
Ellis, P. D. (2010). The Essential Guide to Effect Sizes Statistical Power, Meta-analysis, and the Interpretation of Research Results. Cambridge: Cambridge University Press. doi: 10.1017/CBO9780511761676
European Commission (2012). FAQs on Multilingualism and language Learning. Brussels: European Commission.
European Commission (Hrsg.) (2004). Promoting Language Learning and Linguistic Diversity. An Action Plan 2004-06. Luxembourg: Office for Official Publications of the European Communities.
Evnitskaya, N., and Morton, T. (2011). Knowledge construction, meaning-making and interaction in CLIL science classroom communities of practice. Lang. Educ. 25, 109–127. doi: 10.1080/09500782.2010.547199
Fernández-Fontecha, A., O’Halloran, K. L., Wignell, P., and Tan, S. (2020). Scaffolding CLIL in the science classroom via visual thinking: a systemic functional multimodal approach. Linguistics Educ. 55:100788. doi: 10.1016/j.linged.2019.100788
France, A. (2019). Teachers using dialogue to support science learning in the primary classroom. Res. Sci. Educ. 51, 845–859. doi: 10.1007/s11165-019-09863-3
Franco, C., and Colinvaux, D. (2000). “Grasping mental models,” in Developing Models in Science Education, eds J. K. Gilbert and C. J. Boulter (Berlin: Springer), 93–118. doi: 10.1007/978-94-010-0876-1_5
Gilbert, J. K., and Justi, R. S. (2002). Modelling, teachers’ views on the nature of modelling, and implications for the education of modellers. Int. J. Sci. Educ. 24, 369–387. doi: 10.1080/09500690110110142
Glynn, S. M., and Muth, K. D. (1994). Reading and writing to learn science: achieving scientific literacy. J. Res. Sci. Teach. 31, 1057–1073. doi: 10.1002/tea.3660310915
Goldschmidt, M., and Bogner, F. X. (2016). Learning about genetic engineering in an outreach laboratory: Influence of motivation and gender on students’ cognitive achievement. Int. J. Sci. Educ. 6, 166–187. doi: 10.1080/21548455.2015.1031293
Gonzalez-Howard, M., and McNeill, K. L. (2016). Learning in a community of practice: factors impacting English-learning students’ engagement in scientific argumentation. J. Res. Sci. Teach. 53, 527–553. doi: 10.1002/tea.21310
Gottlieb, M. (2016). Assessing English Language Learners: Bridges for Language Proficiency to Academic Achievement. Thousand Oaks: Corwin.
Gouvea, J., and Passmore, C. (2017). ‘Models of’ versus ‘Models for’. towards an agent-based conception of modeling the science classroom. Sci. Educ. 26, 49–63. doi: 10.1007/s11191-017-9884-4
Grandinetti, M. M., Langellotti, M., and Teresa Ting, Y. L. (2013). How CLIL can provide a pragmatic means to renovate science education – even in a sub-optimally bilingual context. Int. J. Bilingual Educ. Bilingualism 16, 354–374. doi: 10.1080/13670050.2013.777390
Grosslight, L., Unger, C., and Jay, E. (1991). Understanding models and their use in science: conceptions of middle and high school students and experts. J. Res. Sci. Teach. 28, 799–822. doi: 10.1002/tea.3660280907
Haagen-Schützenhöfer, C., Mathelitsch, L., and Hopf, M. (2011). Fremdsprachiger physikunterricht: fremdsprachlicher mehrwert auf kosten fachlicher leistungen? auswirkungen fremdsprache- nintegrierten physikunterrichts auf fachliche leistungen [Foreign language physics lessons: added value for language skills at the cost of content achievement? Effects of content-language-integrated physics classes on content achievement]. Zeitschrift Didaktik Naturwissenschaften 17, 223–260.
Hampton, E., and Rodriguez, R. (2001). Inquiry science in bilingual classrooms. Bilingual Res. J. 24, 461–478. doi: 10.1080/15235882.2001.11074463
Howell, M. E., Booth, C. S., Sikich, S. M., Helikart, T., Roston, R. L., Couch, B. A., et al. (2019). Student understanding of DNA structure–function relationships improves from using 3D learning modules with dynamic 3D printed models. Biochem. Mol. Biol. Educ. 47, 303–317. doi: 10.1002/bmb.21234
ISB (2019). Ergänzende Informationen zum LehrplanPLUS [Supplementary Information on the Curriculum]. Available online at: https://www.lehrplanplus.bayern.de/sixcms/media.php/71/B8_10_Info_Modelle_20190916.pdf (accessed 4 May 2021).
Johnstone, A. H., and Wham, A. J. B. (1979). A model for undergraduate practical work. Educ. Chem. 16, 16–17.
Kaiser, H. F. (1970). A second generation little jiffy. Psychometrika 35, 401–415. doi: 10.1007/BF02291817
Ke, L., and Schwarz, C. (2020). “Using epistemic considerations in teaching: fostering students’ meaningful engagement in scientific modeling,” in Towards a Competence-based View on Models and Modeling in Science Education, eds A. Upmeier, D. K. zu Belzen, and J. Van Driel (Berlin: Springer International Publishing), 181–199. doi: 10.1007/978-3-030-30255-9_11
Ke, L., Zangori, L., Sadler, T. D., and Friedrichsen, P. J. (2020). “Integrating scientific modeling and socio-scientific reasoning to promote scientific literacy,” in Socioscientific Issues-Based Instruction for Scientific Literacy Development, eds W. A. Powell (Hershey: IGI Global), 31–56. doi: 10.4018/978-1-7998-4558-4.ch002
Khan, S. (2011). What’s missing in model-based teaching. J. Sci. Teacher Educ. 22, 535–560. doi: 10.1007/s10972-011-9248-x
Klieme, E., Artelt, C., Hartig, J., Jude, N., Köller, O., Prenzel, M., et al. (eds) (2010). PISA 2009: Bilanz Nach Einem Jahrzehnt [PISA 2009: Review after a decade]. Münster: Waxmann.
KMK (2005). Beschlüsse der Kultusministerkonferenz – Bildungsstandards im Fach Biologie für den Mittleren Bildungsabschluss [Resolution of the Standing Conference of the Ministers of Education and Cultural Affairs of the Länder in the Federal Republic of Germany - standards of biology education for secondary school]. Luchterhand: Munich.
Kovanović, V., Joksimović, S., Poquet, S., Hennis, T., Dawson, S., Gašević, D., et al. (2018). “Understanding the relationship between technology-use and cognitive presence in MOOCs,” in Proceedings of the Seventh International Conference on Learning Analytics and Knowledge (New York, NY: Association for Computing Machinery). doi: 10.1145/3027385.3029471
Krajcik, J., and Merritt, J. (2012). Engaging students in scientific practices: what does constructing and revising models look like in the science classroom? Sci. Children 49, 10–13.
Krajcik, J. S., and Sutherland, L. M. (2010). Supporting students in developing literacy in science. Science 328, 456–459. doi: 10.1126/science.1182593
Krell, M., Reinisch, B., and Krüger, D. (2015). Analyzing students’ understanding of models and modeling referring to the disciplines Biology, Chemistry, and Physics. Res. Sci. Educ. 45, 367–393. doi: 10.1007/s11165-014-9427-9
Krell, M., Upmeier zu Belzen, A., and Krüger, D. (2012). Students’ understanding of the purpose of models in different biological contexts. Int. J. Biol. Educ. 2, 1–34.
Lam, S. F., Wong, B. P. H., Yang, H., and Liu, Y. (2012). “Understanding student engagement with a contextual model,” in Handbook of Research on Student Engagement, eds S. L. Christenson, A. L. Reschly, and C. Wylie (Boston, MA: Springer US), 403–420.
Langheinrich, J., and Bogner, F. (2016). Computer-related self-concept: the impact on cogni-tive achievement. Stud. Educ. Eval. 50, 46–52. doi: 10.1016/j.stueduc.2016.06.003
Lee, O., and Stephens, A. (2020). English learners in STEM subjects: contemporary views on STEM subjects and language with English learners. Educ. Researcher 49, 426–432. doi: 10.3102/0013189X20923708
Lee, S., Kang, E., and Kim, H.-B. (2015). Exploring the impact of students‘ learning approach on collaborative group modeling of blood circulation. J. Sci. Educ. Technol. 24, 234–255. doi: 10.1007/s10956-014-9509-5
Lienert, G. A., and Raatz, U. (1998). Testaufbau und Testanalyse [Test setup and test analysis], 6th Edn. Weinheim: Psychologie Verlags Union.
Lipsey, M. W., and Wilson, D. (2001). Practical Meta-analysis. Thousand Oaks, CA: Sage Publications.
Lo, Y. Y., and Lo, E. S. C. (2014). A meta-analysis of the effectiveness of English-medium education in Hong Kong. Rev. Educ. Res. 84, 47–73. doi: 10.3102/0034654313499615
Louca, L., and Zacharia, Z. (2012). Modeling-based learning in science education: cognitive, metacognitive, social, material and epistemological contributions. Educ. Rev. 64, 471–492. doi: 10.1080/00131911.2011.628748
Luykx, A., Lee, O., and Edwards, U. (2008). Lost in translation: negotiating meaning in a beginning ESOL science classroom. Educ. Policy 22, 640–674. doi: 10.1177/0895904807307062
Mahan, K. R. (2022). The comprehending teacher: scaffolding in content and language integrated learning (CLIL). Lang. Learn. J. 50, 74–88. doi: 10.1080/09571736.2019.1705879
Mahan, K. R., Breik, L. M., and Ødegaard, M. (2018). Characterizing CLIL teaching: new insights from a lower secondary classroom. Int. J. Bilingual Educ. Bilingualism 24, 401–418. doi: 10.1080/13670050.2018.1472206
Marsh, D. (ed.) (2002). CLIL/EMILE—the European Dimension: Actions, Trends and Foresight. Brussels: European Commission.
Maybin, J., Mercer, N., and Stierer, B. (1992). “Scaffolding: learning in the classroom,” in Thinking Voices. The Work of the National Oracy Project, ed. K. Norman (London: Hodder & Stoughton), 186–195.
Mendonça, P. C. C., and Justi, R. (2013). The relationships between modelling and argumentation from the perspective of the model of modelling diagram. Int. J. Sci. Educ. 35, 2407–2434. doi: 10.1080/09500693.2013.811615
Meskill, C., and Oliveira, A. W. (2019). Meeting the challenges of English learners by pairing science and language educators. Res. Sci. Educ. 49, 1025–1040. doi: 10.1007/s11165-019-9837-9
Meyerhöffer, N., and Dreesmann, D. C. (2019). English-bilingual Biology for standard classes development, implementation and evaluation of an English-bilingual teaching unit in standard German high school classes. Int. J. Sci. Educ. 41, 1366–1386. doi: 10.1080/09500693.2019.1607620
Mierdel, J., and Bogner, F. (2019). Is creativity, hands-on modeling and cognitive learning gender-dependent? Thinking Skills and Creativity 31, 91–102. doi: 10.1016/j.tsc.2018.11.001
Morton, T. (2015). Vocabulary explanations in CLIL classrooms: a conversation analysis perspective. Lang. Learn. J. 43, 256–270. doi: 10.1080/09571736.2015.1053283
Nikula, T., Dafouz-Milne, E., Moore, P., and Smit, U. (2016). Conceptualizing Integration in CLIL and Multilingual Education. Bristol: Multilingual Matters. doi: 10.21832/9781783096145
Oh, P. S., and Oh, S. J. (2010). What teachers of science need to know about models: an overview. Int. J. Sci. Educ. 33, 1109–1130. doi: 10.1080/09500693.2010.502191
Passmore, C., Stewart, J., and Cartier, J. (2009). Model-based inquiry and school science: creating connections. School Sci. Mathemat. 109, 394–402. doi: 10.1111/j.1949-8594.2009.tb17870.x
Passmore, C., and Svoboda, J. (2012). Exploring opportunities for argumentation in modelling classrooms. Int. J. Sci. Educ. 34, 1535–1554. doi: 10.1080/09500693.2011.577842
Pearson, K. (1904). On the Theory of Contingency and its Relation to Association and Normal Correlation. London: Dulau.
Pérez-Cañado, M. L. (2012). CLIL research in Europe: Past, Present, and Future. Int. J. Bilingual Educ. Bilingualism 15, 315–341. doi: 10.1080/13670050.2011.630064
Pfenninger, S. (2022). Emergent bilinguals in a digital world: a dynamic analysis of long-term L2 development in (pre)primary school children. Int. Rev. Appl. Linguistics Lang. Teach. 60, 41–66. doi: 10.1515/iral-2021-0025
Piacentini, V., Marques Vieira, R., and Simões, A. R. (2022). Can “Integrated Learning” with English support science education? a case study in Portugal. EURASIA J. Mathemat. Sci. Technol. Educ. 18:em2114. doi: 10.29333/ejmste/12069
Piesche, N., Jonkmann, K., Fiege, C., and Keßler, J.-U. (2016). CLIL for all? a randomised controlled field experiment with sixth grade students on the effects of content and language integrated science learning. Learn. Instruct. 44, 108–116. doi: 10.1016/j.learninstruc.2016.04.001
Prabha, S. (2016). Laboratory experiences for prospective science teachers: a meta-analytic review of issues and concerns. Eur. Sci. J. 12, 235–250. doi: 10.19044/esj.2016.v12n34p235
Prain, V. (2004). “The role of language in science learning and literacy,” in Writing and Learning in the Science Classroom, eds D. L. Zeidler, J. L. Bencze, M. P. Clough, A. E. K. Fouad, M. Rollnick, T. D. Sadler, et al. (Dordrecht: Springer), 33–45. doi: 10.1007/978-1-4020-2018-6_4
Quarderer, N. A., and McDermott, M. A. (2020). Examining science teacher reflections on argument-based inquiry through a critical discourse lens. Res. Sci. Educ. 50, 2483–2504. doi: 10.1007/s11165-018-9790-z
Rodenhauser, A., and Preisfeld, A. (2015). Bilingual (German-English) molecular biology courses in an out-of-school lab on a university campus: cognitive and affective evaluation. Int. J. Environ. Sci. Educ. 10, 99–110.
Rost, J. (2004). Lehrbuch Testtheorie–Testkonstruktion [Textbook test theory–test construction], 2nd Edn. Bern: Hans Huber.
Roth, T., Conradty, C., and Bogner, F. X. (2022). The relevance of school self-concept and creativity for CLIL outreach learning. Stud. Educ. Eval. 73, 1–12. doi: 10.1016/j.stueduc.2022.101153
Roth, T., Scharfenberg, F.-J., Mierdel, J., and Bogner, F. (2020). Self-evaluative scientific modeling in an outreach gene technology laboratory. J. Sci. Educ. Technol. 29, 725–739. doi: 10.1007/s10956-020-09848-2
Sarmouk, C., Ingram, M. J., Read, C., Curdy, M. E., Spall, E., Farlow, A., et al. (2019). Pre-laboratory online learning resource improves preparedness and performance in pharmaceutical sciences practical classes. Innovat. Educ. Teach. Int. 57, 460–471. doi: 10.1080/14703297.2019.1604247
Satayev, M., Balta, N., Shaymerdenova, I. R., Fernández-Cézar, R., and Alcaraz-Mármol, G. (2022). Content and language integrated learning implementation through team teaching in biology lessons: a quasi-experimental design with university students. Front. Educ. 7:867447. doi: 10.3389/feduc.2022.867447
Scharfenberg, F. J., and Bogner, F. X. (2013). Teaching gene technology in an outreach lab: students’ assigned cognitive load clusters and the clusters’ relationships to learner characteristics, laboratory variables, and cognitive achievement. Res. Sci. Educ. 43, 141–161. doi: 10.1007/s11165-011-9251-4
Schwarz, C., Reiser, B. J., Davis, E. A., Kenyon, L., Achér, A., Fortus, D., et al. (2009). Developing a learning progression for scientific modeling: making scientific modeling accessible and meaningful for learners. J. Res. Sci. Teach. 46, 632–654. doi: 10.1002/tea.20311
Schwarz, C., and White, B. (2005). Metamodeling knowledge: developing students’ understanding of scientific modeling. Cogn. Instruct. 23, 165–205. doi: 10.1207/s1532690xci2302_1
Sins, P., Savelsbergh, E., Van Joolingen, W., and Van Hout-Wolters, B. (2009). The relation between students’ epistemological understanding of computer models and their cognitive processing on a modelling task. Int. J. Sci. Educ. 31, 1205–1229. doi: 10.1080/09500690802192181
Stoddart, T., Pinal, A., Latzke, M., and Canaday, D. (2002). Integrating inquiry science and language development for English language learners. J. Res. Sci. Teach. 39, 664–687. doi: 10.1002/tea.10040
Sweller, J. (2015). In academe, what is learned and how is it learned? Curr. Direct. Psychol. Sci. 24, 190–194. doi: 10.1177/0963721415569570
Tagnin, L., and Ní Ríordáin, M. (2021). Building science through questions in Content and Language Integrated Learning (CLIL) classrooms. Int. J. Stem Educ. 8:34. doi: 10.1186/s40594-021-00293-0
Taylor, P. C., and Medina, M. (2011). Educational research paradigms: from positivism to pluralism. College Res. J. 1, 1–16.
Tolbert, S., Knox, C., and Salinas, I. (2019). Framing, adapting, and applying: learning to contextualize science activity in multilingual science classrooms. Res. Sci. Educ. 49, 1069–1085. doi: 10.1007/s11165-019-9854-8
Treagust, D. F., Chittleborough, G. D., and Mamiala, T. L. (2002). Students’ understanding of the role of scientific models in learning science. Int. J. Sci. Educ. 24, 357–368. doi: 10.1080/09500690110066485
Usher, S. (2013). Letters of Note. Correspondence Deserving of a Wider Audience. Edinburgh: Canongate.
Virida, S. (2021). The (heterogenous) effect of CLIL on content-subject and cognitive acquisition in primary education: evidence from a counterfactual analysis in Italy. Int. J. Bilingual Educ. Bilingualism 25, 1877–1893. doi: 10.1080/13670050.2020.1835805
Virdia, S., and Wolff, D. (2020). Teaching preferences in content and language integrated learning (CLIL): an exploratory study based on the vignette experiment methodology. Sc. Democratica 11, 235–258.
Wang, J.-R., and Chen, S.-F. (2014). Exploring mediating effect of metacognitive awareness on comprehension of science tests through structural equation modeling analysis. J. Res. Sci. Teach. 50, 175–191. doi: 10.1002/tea.21131
Wolf, R. (1997). “Rating scales,” in Educational Research, Methodology and Measurement: an International Handbook, ed. J. Keeves (Oxford: Elsevier), 958–965.
World Medical Association (2013). Declaration of helsinki, world medical association. J. Am. Med. Assoc. 310, 2191–2194. doi: 10.1001/jama.2013.281053
Keywords: CLIL learning, classroom modelling, evaluating models, changing nature of models, gene technology outreach learning, science education
Citation: Roth T, Scharfenberg F-J and Bogner FX (2022) Content and Language Integrated Scientific Modelling: A Novel Approach to Model Learning. Front. Educ. 7:922414. doi: 10.3389/feduc.2022.922414
Received: 17 April 2022; Accepted: 21 June 2022;
Published: 22 July 2022.
Edited by:
Chunxia Qi, Beijing Normal University, ChinaReviewed by:
Xuesong Gao, University of New South Wales, AustraliaMilan Kubiatko, J. E. Purkyne University, Czechia
Copyright © 2022 Roth, Scharfenberg and Bogner. This is an open-access article distributed under the terms of the Creative Commons Attribution License (CC BY). The use, distribution or reproduction in other forums is permitted, provided the original author(s) and the copyright owner(s) are credited and that the original publication in this journal is cited, in accordance with accepted academic practice. No use, distribution or reproduction is permitted which does not comply with these terms.
*Correspondence: Tamara Roth, tamara.roth@uni-bayreuth.de; tamara.roth@uni.lu