- 1Graduate School of Education, The University of Tokyo, Tokyo, Japan
- 2College of Education, Yokohama National University, Yokohama, Japan
Many previous studies related to self-regulated learning have demonstrated that students with higher academic achievement tend to use varied and effective learning strategies. However, they cannot acquire these strategies in a short period. If learning strategies are acquired and used gradually, it may help specify individual levels of use and teach its usage in a reasonable order. Therefore, this study analyzed data on strategy use with item response theory (IRT) to specify students’ level of strategy acquisition and determine how they are ordered. Questionnaire data on the frequency of learning strategy use were obtained from students in five universities (N = 472) and analyzed using the IRT model. It was shown that the average levels of students’ strategy acquisition were related to their universities’ academic achievement rankings.
Introduction
Life in modern society is highly unpredictable, and people need to be proactive in deciding their behavior. Therefore, fostering self-regulated learners is one of the most important goals in education. Self-regulated learning is defined as involving “metacognitive, motivational, and behavioral processes that are personally initiated to acquire knowledge and skills, such as goal setting, planning, learning strategies, self-reinforcement, self-recording, and self-instruction” (Zimmerman, 2015, p. 541). This implies that these learners have sufficient skills to earn a living or acquire the necessary competencies. To deal with society’s unpredictability and decide how to live proactively, students need to develop self-regulated learning skills. This idea is consistent with discussions on the importance of developing learning skills from the perspectives of key competencies, proposed by the Organization for Economic Cooperation and Development (OECD; Rychen and Salganik, 2003) and from the “21st century skills” movement (e.g., Griffin et al., 2012). The key competencies and 21st century skills are related to the cultivation of necessary student skills that will be useful in and out of school and in future contexts.
In considering how self-regulated learners can be cultivated, an important aspect is promoting the use of effective learning strategies (e.g., Uesaka, 2012; Manalo et al., 2017). Many reviews and theoretical papers in the self-regulated learning research area have shown (Zimmerman and Schunk, 1989; Schunk and Zimmerman, 1994, 1998; Dignath et al., 2008) that these learners use effective strategies that might positively affect life-long learning. Based on this idea, various studies have examined factors that promote learning strategy use (Ames and Archer, 1988; Nolen, 1988; Zimmerman and Martinez-Pons, 1990; Lynch, 2006) and proposed educational methods that enhance students’ skills by promoting the effective use of these strategies (Harris and Graham, 1999; Cleary and Zimmerman, 2004; Guthrie et al., 2004; Camahalan, 2006; Ramdass and Zimmerman, 2008).
If learning strategy use can be considered an important skill for students to become self-regulated learners, identifying the level of learning strategy use can help in considering how to promote their development. For example, as described later, some studies on knowledge acquisition with worked examples, proposed and examined the necessity of specifying students’ knowledge level, which has been used to improve students’ knowledge acquisition (e.g., Schwonke et al., 2007; Salden et al., 2010). To achieve these goals, computer-adaptive testing (CAT) has confirmed the effectiveness of many good practices and methodological bases of statistics for analyzing student levels. Item response theory (IRT) is an effective method for analyzing students’ levels. For example, their level of English skills in second-language acquisition was analyzed using this method (e.g., Min and Aryadoust, 2021). Previous research in self-regulated learning has not applied such an idea to analyze individual differences in learning strategy use. However, specifying them regarding learning strategies using this method may facilitate the development of more effective instructional strategies for cultivating self-regulated learners.
In detail, learners may be more likely to acquire learning strategies more effectively if they receive advice and guidance that is aligned with their specific learning strategy acquisition. In fact, learning strategies range from relatively easy to difficult, and if they are not taught according to their acquisition level, they are likely to be either too easy or too difficult for the learner to acquire. However, many learning strategy teaching studies, as discussed below, teach the same strategy(ies) to participants, and such level-appropriate teaching is not currently possible. To operationalize learning strategy support in CAT, research is required to identify the underlying learning strategy and learner level. However, to date, no published study has provided the basis for operationalizing the support of such learning strategies in CAT.
The level of acquisition of the learning strategy of a student needs to be identified to take it into account for future initiatives and to advise on appropriate learning strategies. Furthermore, the difficulty level of the learning strategy also needs to be identified (for example, which learning strategies are acquired first and which later). Additionally, it is necessary to map that level to the learner’s level of acquisition of the learning strategy. If a new framework is proposed that allows this, it could enable the guidance of strategy instruction by the learner’s level of acquisition of the learning strategy. Therefore, this study adopted IRT analysis, which can deal with the difficulty level of the learning strategies and the learner’s level of acquisition on a unified scale.
In conducting this study, four considerations were taken into account. First, as this is the first study to determine the extent to which effective learning strategies are acquired, learning strategies that may not necessarily be initially effective were included. Learning strategies such as simple repetition are known to be less effective than those that use metacognition or elaboration organization (for example, Ramsden, 1988); however, the possibility remains that these strategies may be used in the early stages of learning. Initially, they should be seen as integrative, and if they are qualitatively different, a procedure should be followed to exclude them. Second, while using a theoretical framework, we analyzed IRT by preparing items that were in line with specific subject areas. Theoretical strategy studies are often worded in such a way that they are not subject-specific, as will be discussed later in this section. However, it is easier for learners and teachers to give advice on specific learning strategies if they are structured in a subject-specific manner. Therefore, it was decided to list and use learning strategies based on theory but also in a form that is specific to the subject areas.
Third, when analyzing the students’ responses to the use of the learning strategies with IRT, we analyzed them with both polytomous and binary IRT and compared the two. Whereas polytomous IRT is an in-depth analysis that uses all the information, it is not always easy to interpret when considering the use of learning strategies. Therefore, if the results of both were found to be similar, we decided to report the results in the binary type, which is more commonly used in IRT. Finally, for this study, participants were drawn from higher-, middle-, and lower-ranked Japanese universities. University level is indicative of academic achievement in a broad sense. Even if it is difficult to verify the nature of the relationship between individual performance data and the level of learning strategies identified in this study, it is possible to argue that learning styles may underlie academic performance if even a small relationship can be demonstrated.
Variation of effective learning strategies and possibility of cultivation
In a seminal study on learning strategy use conducted by Marton and Säljö (1976), the authors interviewed university students to understand their learning processes and discovered two types: surface-level processing and deep-level processing. The former includes simple repetition (such as repeated reading and writing to remember the material), whereas the latter employs extra activities to improve the understanding of the material (such as elaboration of information). Successive studies have shown that learners who use deep-level processing strategies perform better (e.g., Ramsden, 1988).
Since the above-mentioned research, various effective learning strategies have been identified by questionnaire-type studies (Weinstein and Mayer, 1986; Pintrich and de Groot, 1990; Zimmerman and Martinez-Pons, 1990). Of these, the Motivated Strategies for Learning Questionnaire is one of the most well-known and frequently used methods (Pintrich et al., 1993). Prior studies have also attempted to categorize learning strategies. For example, Pintrich et al. (1993) proposed dividing them into three categories: cognitive, metacognitive, and resource management strategies. Accordingly, this study also used the framework of effective strategies considering these three main categories.
The two types of learning strategies categorizations are surface-level processing/deep-level processing and cognitive/metacognitive/resource management. These two categories are closely connected and, therefore, are not independent frameworks. Murayama (2007) indicated that cognitive strategies can be divided into two types: shallow (e.g., rehearsal and repetition) and deep cognitive strategies (e.g., elaboration and organization). Their structure is shown in Table 1. Shallow-level processing is a learning method that emphasizes simple repetition (Murayama, 2007). By contrast, here, deep-level processing is a learning method that is based not on simple repetitive processes but rather on cognitive devices in some sense. For example, it includes deep cognitive learning strategies that focus on understanding meaning in relation to existing knowledge, metacognitive strategies that focus on one’s own cognitive state, and learning methods that utilize external resources such as other people and diagrams.
Whereas the structure of these learning strategies provides useful information, it is unclear which type is best in the early stages of learning and which in the later stages. Although many studies that have identified these structures also consider learning strategies in a domain-general manner (for example, “When reading I try to connect the things I am reading about with what I already know”), it is difficult to ascertain solely from these items what specific learning strategies should be developed in each subject area.
Another branch of learning strategy research has revealed other variables related to its use (Ames and Archer, 1988; Nolen, 1988; Shell and Husman, 2008; Tabachnick et al., 2008; Kesici et al., 2009). Furthermore, concrete programs to cultivate learning strategy skills to enhance student performance in a specific subject have been conducted. Reading literacy is one of the most active research areas. Concept-oriented reading instruction, proposed by Guthrie et al. (1998, 2004), and self-regulated strategy development, proposed by Sawyer et al. (1992) and Harris and Graham (1999), were seminal works. For example, Guthrie et al. (2004) focused on promoting reading strategies among third-grade students across 12 weeks and demonstrated that their program enhanced reading performance, motivation, and strategy use among the participants. In addition to reading, strategy training has been provided in other subject areas; for example, in mathematics. Ramdass and Zimmerman (2008) provided self-correction strategy training for middle school students and demonstrated improvement in the accuracy of self-evaluation of efficacy and mathematics performance in division learning. These studies showed that learning strategy use can be enhanced by implementing thoughtful programs that are carefully planned by researchers.
Whereas these studies are unique in that they examine the promotion of specific learning strategies in a subject area, leading to actual strategy support, the idea of varying study skills interventions according to a student’s level of learning strategy has been overlooked. Most studies that have supported learning strategies, even with targeted groups of participants, have provided the same instructions and materials to all participants (Sawyer et al., 1992; Guthrie et al., 1998, 2004; Harris and Graham, 1999). However, students’ spontaneous use of learning strategies differs considerably according to their learning level. Students in the early stages of learning development might use easier strategies, whereas those in the latter stage might use more complex strategies. This was demonstrated by Uchida (2021), who showed that early stage learners mainly used shallow-level strategies in vocabulary learning, whereas latter stage learners used deeper-level strategies. Therefore, students might be placed on a continuum from early to late-stage acquisition of learning strategy use. However, none of the previous studies have been based on the idea of identifying the level of learning strategies within a single scale, and then identifying the learner’s level within that same scale. This would make it possible to support level-based learning strategies. Therefore, in this study, based on the structure of Table 1, learning strategies for specific subjects are placed on the same scale. In addition, we aim to position the learners on the scale by using IRT, which will be described later. This will make it possible to provide support according to the level of learning strategy.
Computer-adaptive testing and adjusting instruction according to individual levels by item response theory
Although learning strategy research has not analyzed students’ levels of learning strategy use, other research areas have conducted such an analysis to adjust for instructions and individual learning circumstances. For example, CAT is a well-known technique for language acquisition among second-language learners, in which items are adapted according to test-takers’ responses; for example, following a student’s response to the first item (e.g., failure or success in solving the first task), the test identifies the acquisition level and provides one of several alternative types of tasks as the second item. This is rooted in IRT, a unique test theory that calculates individual knowledge levels and hence item difficulties as probabilities based on item responses.
To explain IRT in more detail, the knowledge acquisition dimension is represented by θ, and test responses to each item are modeled by a mathematical item response function; using students’ responses to each item, their acquisition levels (called the “trait value” in IRT) are also specified. In the two-parameter model, the function in equation (1) was used. In this analysis, student knowledge acquisition and item difficulty levels are represented using θ. In the two-parameter model, parameter bi is called “item difficulty” of item i and is defined as the θ score of a population in which 50% of participants pass the item. Higher scores indicate more difficult items. Parameter ai is called “item discrimination,” of item i and refers to the θ score that can be most effectively distinguished using this item. D is a constant 1.702 to approximate the curve to the cumulative normal distribution (see de Ayala, 2009, for basic information on IRT). When analyzing with IRT, a graph of the relationship between θ and the percentage of correct responses (the item characteristic curve, ICC) is drawn for each item. IRT was developed based on the use of binary data, and in the case of binary data, a single ICC is drawn for each item. However, an IRT for analyzing multi-valued data has been proposed (for example, Tang, 1996). In the polytomous-type IRT for analyzing multi-valued data, multiple ICCs are drawn for each item for each response value, such as 1, 2, 3, or 4.
Computer-adaptive testing, as well as various other tools and studies, has used IRT as an assessment of competencies. For example, in the OECD’s Programme for International Student Assessment, students’ achievement levels were represented by IRT analysis-based scores. Similarly, Brackenbury et al. (2017) developed a screening test using IRT to detect speech-sound disorders, and Balsis et al. (2017) utilized it to conduct complex personality disorder research. IRT also holds promise in many other areas.
A unique feature of IRT is that item difficulty and participant level can be represented by the same dimension (θ). In other words, it is characterized by the ability to select items based on the learner’s level. With this feature, it can be used it as a basis for determining which item (in this case, the learning strategy) would be appropriate when teaching a learner at a particular level.
Overview and questions of the present study
This study aims to provide a foundational framework toward a future in which learners can be supported according to their level of use of learning strategies. Currently, the idea of adjusting instruction and assessment according to the level of the student is uncommon. However, identifying students’ level of mastery of learning strategies and adjusting instruction accordingly may be important for improving effective learning strategy instruction to foster self-regulated learning.
The IRT framework allows for a unified discussion of the relationship between the difficulty level of a learning strategy and the learner’s level of mastery of the learning strategy; when IRT identifies the difficulty level of a strategy and the student’s learning strategy level on the same dimension, the result is that appropriate learning strategies can be taught. Therefore, we aim to identify the difficulty level of the learning strategy as a continuum using IRT and represent the learner’s use of the learning strategy on the same dimension. These can be used to coordinate instruction to promote the use of learning strategies in the future.
In addition to these objectives, this study examined whether there is a relationship between the level of strategy use and academic performance, which would validate the use of the continuum of individual differences obtained in the IRT analysis in educational settings.
The following steps were taken in this study. First, a list of effective learning strategies for specific subjects was made, using the framework in Table 1. If questionnaires were already available, they were used, and if they were missing, we added them. At this stage, even shallow-level learning strategies were included, as they may be used in the early stages of learning. Factor analysis would then be used to confirm the unidimensionality that is the premise of the IRT analysis. If the results of the factor analysis indicated that the shallow-level learning strategies were qualitatively different from the deep-level learning strategies, only deep-level learning strategies were used and shallow-level learning strategies were excluded. If the unidimensionality was not confirmed and the learning strategies were separated by subject area, IRT was used for each one. However, if the items were not grouped by subject but constituted a single dimension, we assumed that effective learning strategies were unidimensional at a deep cross-subject area and proceeded to the next step in the analysis. Second, IRT was applied to items that were confirmed to have a one-factor structure, and acquisition scores were calculated to represent each participant’s strategy acquisition level (θ). When analyzing the results with IRT, both polytomous and dichotomous IRT analyses are conducted; if the two results are compared and it is confirmed that there is no significant difference between those two, the results analyzed with dichotomous IRT are reported, giving priority to ease of interpretation. Finally, the relationship between academic achievement and participants’ acquisition scores was analyzed. If students who showed higher academic performance demonstrated higher acquisition scores, the strategy acquisition level might be valid for predicting underlying high academic performance. To this end, in this study, students were reminded about the learning methods used in high school. In Japan, the university entrance examinations are very competitive. Students need to use effective learning strategies acquired during their high school years to achieve academic excellence. As Shimoyama (1984) argues, Japanese students select universities that match their academic abilities. Therefore, this study examined learning strategies during high school and university levels to test their validity.
Terminology used in the current study
As shown in Figure 1, terminologies different from the traditional IRT framework were used. While IRT analysis has primarily been used for academic achievement tests, some terminologies do not fit the context of learning strategy use. Thus, certain phrases were modified to suit the context of the study. For example, the traditional term “trait value” for the abscissa (θ) was replaced by “strategy acquisition level,” which represents the extent to which each student acquired higher strategies. The calculated values representing the students’ levels were called “strategy acquisition scores.”
We retained the same term, “item difficulty” according to the IRT framework, for parameter b. Theoretically, we perceived it to represent an “order of acquisition” (the ranking of strategy items on the θ scale in the IRT analysis) of each learning strategy. However, IRT analysis is not evidence of the temporal and developmental order of strategy acquisition. Therefore, the same term for “item difficulty” was used.
Methods
Participants
To cover a wide range of learning strategy levels of Japanese university students, participants were drawn from a balanced mix of Japan’s higher-, middle-, and lower-ranked universities. A total of 472 Japanese university students (female = 180, male = 275, unknown = 17) from five universities in Tokyo and Kanagawa prefectures (mean age = 19.81 years, SD = 2.07) were recruited. The universities represented different rankings in Japan; Universities A and B were part of the higher-ranked universities, Universities C and D were in the middle ranks, and University E was in the lower-ranked category (Benesse Corporation, 2017). The number of students in each school was as follows: University A had 165 students, University B had 67 students, University C had 59 students, University D had 127 students, and University E had 54 students. All participants were recruited from educational psychology courses, as these courses are compulsory for students aiming for teacher qualification. Furthermore, those studying educational psychology belong to diverse disciplines, from science and technology to the humanities and economics. All students in this study participated voluntarily. We obtained ethics approval to conduct this study from the Life Sciences and Research Ethics and Safety Committee of the University of Tokyo (approval number: 18–16).
Materials and procedure
As stated at the beginning of this manuscript, the purpose of this study is to create a foundation for specifically advising students on what learning strategies to learn next, depending on their level of learning strategy. To achieve this objective, it is necessary to list specific subject learning strategies while maintaining a theoretical foundation. Therefore, we have listed specific subject learning strategies, using the structure of Table 1 as a foundation. Specifically, we took five subjects (mathematics, English, Japanese, social studies, and science) that are relatively commonly taught in Japanese junior high and high schools and selected items in an unbiased manner, considering how the learning strategies of cognition, metacognition, and resource management can be embodied in each. In selecting items, we referred to previous studies on learning strategies (for example, Inuzuka, 2002; Murayama, 2003; Ichihara and Arai, 2006; Oyama, 2009), case reports on cognitive counseling, and educational practices using psychology (for example, Ichikawa, 1998; Uesaka et al., 2017). Where studies could not be found, we added items ourselves, taking theory into account.
Table 2 lists labels, definitions, and specific examples for each of the subcategories in Table 1. The first group is the (shallow) cognitive learning strategy group, which is a learning method that emphasizes simple repetition (Murayama, 2007). Examples of the items are “When trying to remember English words, I memorized them by writing them repeatedly” (English) and “When I learned mathematics, I remembered the procedure as much as possible” (mathematics). The second group is the (deep) cognitive learning strategy group, which is a learning method that focuses on understanding meaning in relation to existing knowledge (Murayama, 2007). Strategies such as “elaboration” and “organization” as discussed in psychology are also included in this category. Examples of the items are “When trying to remember mathematical formulas, I considered how to get the formula” (mathematics) and “When I learned social science, I considered why such a historical event happened” (social science). The third group is the metacognitive learning strategy group, which is a learning method that monitors one’s own cognitive state and controls the learning behavior based on it. Examples of the items are “When I learned science, I tried to make clear the points that I did not understand” (science) and “When learning mathematics, to prevent failure, I wrote points for attention in my notebook” (mathematics). The final category is the external resource utilizing strategy, which is a method of learning that involves actively using things and people around oneself. Examples of the items are “When I found difficult words while reading (Japanese), I looked them up in the dictionary” (language learning), and “I tried to participate in scientific learning programmes held in the area or in the school” (science). All items used are listed in Table 3.
Participants answered the questionnaires on a four-point Likert-type scale to reflect their learning strategies in high school: “1: do not use at all,” “2: use sometimes,” “3: use often,” and “4: always use.” Another choice, “not taking the course,” was also included for those who had not taken a course in the subject area.
The questionnaire included other items that were not relevant to this study’s aim, such as items on beliefs about learning. Along with these and the focal items, the booklet additionally asked the participants to report to their university to check the validity of the connection between the level of strategy acquisition and academic achievement. Participants responded at their pace; the questionnaire took approximately 20–30 min to complete.
Results
Results of factor analysis to check one-dimensionality
A factor analysis was preliminarily conducted to choose one-dimensional items before the IRT analysis. R 3.4.4 with the “psych” package was employed to perform the factor analysis (Revelle, 2018). Two items that many participants responded to without having taken the course (over 10%) were excluded.
A factor analysis was conducted to determine the items to be analyzed in the IRT. Specifically, we tested the possibility that the items of the learning strategies prepared for this study would be grouped by subject area, theoretical grouping, and shallow and deep level, or as one cohesive whole. The decay of eigenvalues was as follows: 10.09, 5.10, 2.99, 2.67, 1.97, and so on. Harris–Kaiser independent cluster rotation (Harris and Kaiser, 1964) was used as the rotation method to obtain a simple structure. As shown in Table 3, a two-factor model was understandable from the perspective of theories and findings in cognitive psychology. As shown in Figure 2, the results of the factor analysis demonstrated that learning strategies do not cohere by five subject areas. It was also shown that they do not cohere according to theoretical groupings (shallow cognitive learning, deep cognitive learning, metacognitive learning, and resource managing strategies). However, it is difficult to understand them as a single factor as a whole; it is more appropriate to understand them as two cohesive groups: deep-level and shallow-level learning strategies, as will be discussed below. Therefore, two factors were considered appropriate and adopted in this study.
A more detailed look at the results of the factor analysis shows that items that load highly on the first factor are generally deeper-level learning strategies. For example, “When trying to remember mathematical formulas, I considered how to get the formula” and “When I learned social science, I considered why such a historical event happened” were among the items categorized as deep cognitive learning strategies; “When I learned mathematics, I tried to conside why I failed to solve the problems” and “When I read (Japanese), I set myself some questions to check my understanding” were metacognitive learning strategies; and items such as “When I read English text, I looked words up in the dictionary if I found unknown words” and “When I found unclear points when learning science, I asked my friends and teachers” were categorized as utilizing external resource learning strategies. As indicated in the classification in Table 1, these are all positioned as deep-level learning strategies.
However, items that load highly on the second factor are deemed shallow-level learning strategies. For example, “When I learned science, I memorized the content without considering the reason” and “When I learned history, I memorized years of historical events or the events.” As shown by Ramsden (1988), deep and metacognitive learning strategies are more effective than shallow ones. The correlation between the first and second factors was very low (r = 0.02).
The results indicate that shallow-level learning strategies are qualitatively different from deep-level learning strategies. Therefore, since the purpose of this study was to identify the level of effective learning strategies, rather than using a shallow-level in the early stages of learning that gradually transforms into a deep level, shallow-level learning strategies that were determined not to constitute unidimensionality with deep-level learning strategies were excluded from subsequent analyses.
Again, the factor analysis was also conducted for the 45 items loading on the first factor, with three excluded due to the small factor loadings (the criterion for exclusion was set as a factor loading value under 0.25). The decay of eigenvalues was as follows: 9.84, 2.82, 2.41, 2.07, 1.84, 1.65, and so on. The factor loading values, which are highly correlated with the first-factor loading values obtained from all items, are shown in the right column of Table 3. Thus, only those items were used for subsequent IRT analysis. The alpha coefficient of these 45 items was 0.92, indicating high internal consistency.
Item response theory analysis with learning strategy use data
Based on the results of the factor analysis, which showed that items loading on the first factor were deep-level processing learning strategies, 45 items with high loadings on the first factor were used in the IRT analysis. The analysis used both binary and polytomous IRTs. When using a binary IRT, the Likert-type data were converted into binary data. If participants answered “3: use often” or “4: always use” for a given learning strategy, it was coded as “1”; all other responses were coded as “0.” The analysis of both IRTs was conducted using R 3.4.4 and the “irtoys” package (Partchev et al., 2017).
Correlations between students’ mastery scores obtained from the binary IRT and polytomous IRT were analyzed. The results are presented in Figure 3. As shown in the results, the acquisition scores obtained from both analyses are very similar. However, in the case of polytomous IRT, interpretation is difficult because an ICC is given for each response value. Therefore, the present study gives priority to ease of interpretation and reports the results of the binary IRT.
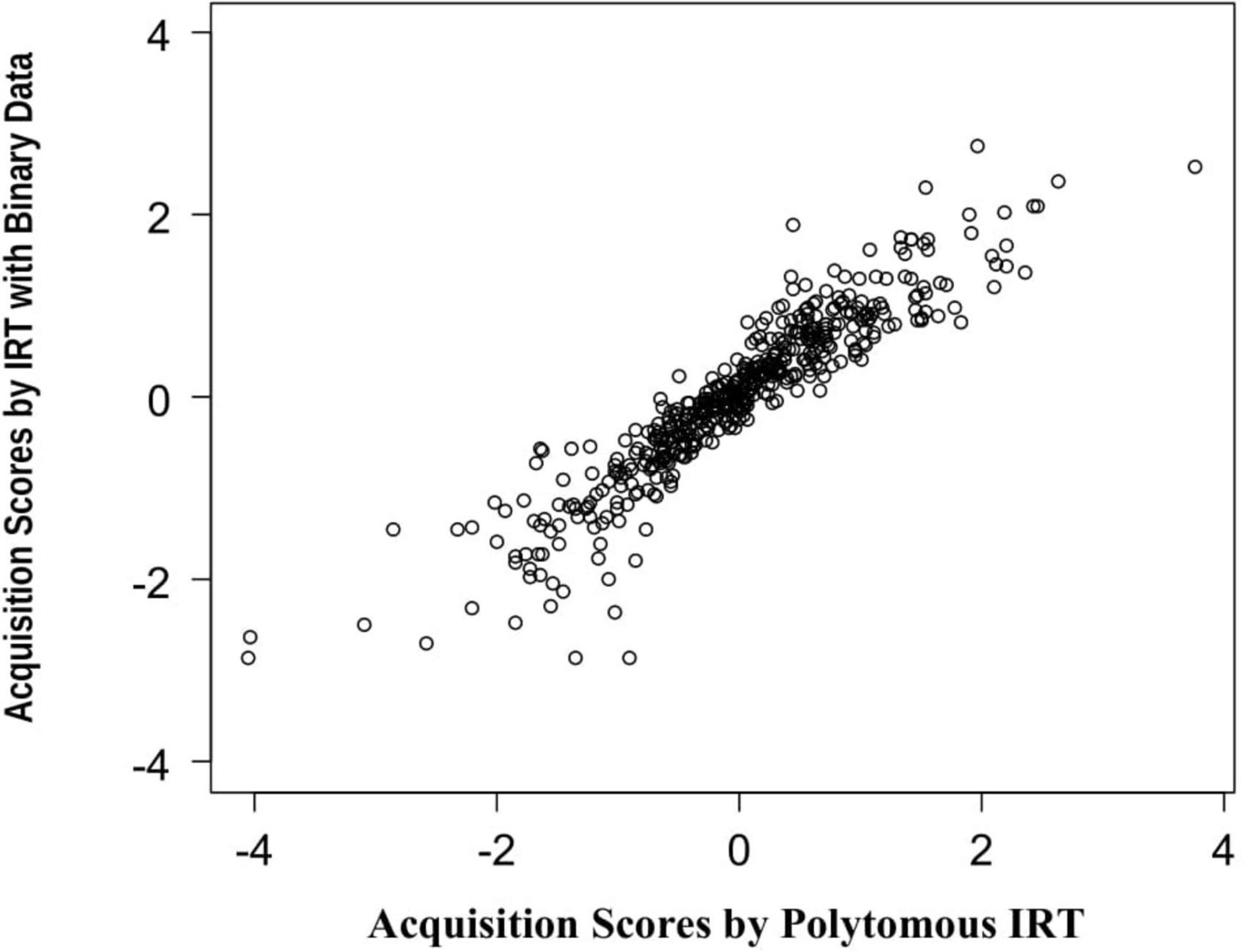
Figure 3. The correlation between students’ acquisition scores obtained from item response theory (IRT) with binary data and polytomous IRT.
Parameters were estimated using a total of 45 items and IRT. The results are presented in Table 4, grouped by subject area for readability; note that IRT is not carried out separately for each subject area. Examples of the ICCs obtained are shown in Figure 4. The acquisition scores were also estimated. The value of the discrimination parameter in all items was greater than 0.55, suggesting that there were no items with a low discrimination parameter value.
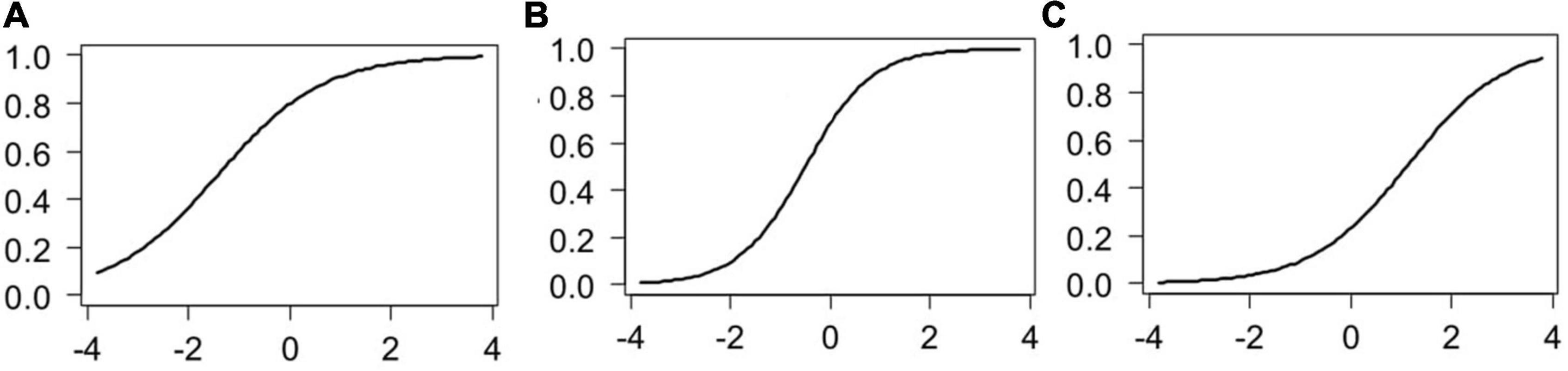
Figure 4. Examples of item characteristic curves (ICCs) in items concerning mathematics. (A) ICC of Item 20 “When I learned mathematics, I checked whether answers were correct after solving.” (B) ICC of Item 18 “When I learned mathematics, I tried again to solve the problems that I had failed to solve.” (C) ICC of Item 23 “When learning mathematics, to prevent failure, I wrote points for attention in my notebook.”
Examination of learning strategies used by each university revealed that students at the higher-performing universities actively used deep cognitive and metacognitive strategies; however, they used strategies that utilized external resources less frequently. For example, learning strategies such as “I tried to participate in scientific learning programmes held in the area or school” or “When I learned English, I read English text in books, magazines, or newspapers” had item difficulty values above 2.0. These strategies were also used less frequently by students at universities with higher academic achievement. However, this should be examined in future studies.
Relationship between learning strategy acquisition and academic achievement
If learning strategy acquisition level highly correlates with academic achievement, it might provide evidence for its validity. The average acquisition scores for each university were calculated to examine the relationship between acquisition level and academic achievement. Note that the study included students from several universities and grades. Therefore, it is not possible to analyze academic achievements using a unified index. However, the university itself is considered to broadly reflect the learner’s level of study. In particular, it has been suggested that in Japan, higher education plans are determined mainly by academic ability and less by other influences (Kariya and Rosenbaum, 1987). Kariya and Rosenbaum (1987) collected data for junior high schools, but the idea is that Japanese students do not change significantly, even when they are aiming to go on to university. Therefore, individual differences in the level of acquisition of learning strategies are related to the level of university, which is considered a manifestation of academic ability in a broad sense. The purpose of this analysis is to show that the theta resulting from this study may provide a background for a certain type of academic ability.
As shown in Figure 5, average acquisition scores, arranged in order, correspond to academic achievement as estimated by the Japanese university rankings (i.e., Universities A and B = comparatively higher-ranked, Universities C and D = middle-ranked, University E = comparatively lower-ranked). The result suggests that the participants’ level of mastery of the use of deep-processing learning strategies may be part of the background to their high academic performance.
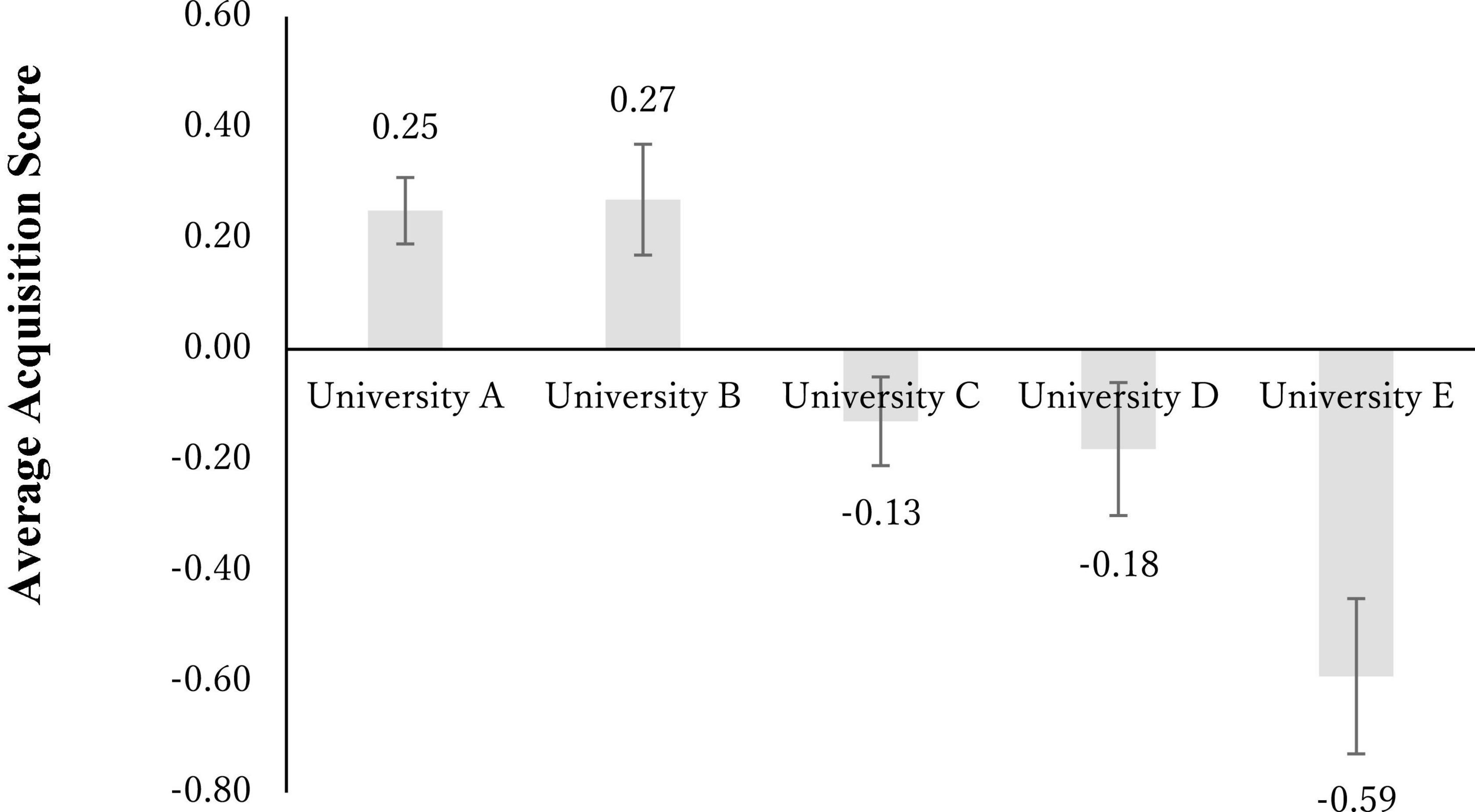
Figure 5. Average acquisition scores for students in each university. The vertical bars show the standard deviations.
Discussion
Summary of the findings in the present research
This study analyzed students’ levels of learning strategies using IRT and specified the ease or difficulty level of each strategy. Traditionally, learning strategy research did not consider the level of learning strategies among students. Moreover, most research, which tried to promote students’ academic performance by encouraging the use of learning strategies, usually provided instructions that were not adjusted to individual levels. This study attempted to shed light on this aspect.
After confirming the one-dimensionality, IRT analysis was applied to all deep-level processing types of items. Moreover, the positive relationship between the acquisition score retrieved from this analysis and academic performance was confirmed. We also specified the appropriate learning strategy for a specific learner level. Although academic achievement was evaluated by the university’s rank, a clear relationship was demonstrated. This means that the θ assessed by the questionnaires might be the basis of academic achievement.
How to utilize the findings of the current study
This manuscript proposes a framework as a basis for ensuring that students can be advised according to their level of acquisition of learning strategies. Although it is limited in that it only proposes a basic framework that has not yet led to actual support, it proposes a direction for the use of this framework in the future.
First, applying this idea in educational practice should involve utilizing it in assessment as well as in the results of personalized interventions. Although current personal tutoring research mainly focuses on instruction in specific subject-related knowledge, such as biology (see the review of Chi et al., 2001; Roscoe and Chi, 2008), some studies and practices focus on improving learning strategy use through personal tutoring; for instance, Craig et al. (2000) demonstrated the enhancement of questioning skills by tutoring. Another personal tutoring study conducted in Japan, referred to as “cognitive counseling” (Ichikawa, 2005; Ichikawa et al., 2017), aimed to develop autonomous learners and utilized cognitive psychology. Currently, counselors with a strong background in cognitive psychology manually assess clients’ learning strategy acquisition levels based on their materials. Based on the findings of this study, tutors or counselors can assess learners’ strategy acquisition levels and teach appropriate learning strategies.
Second, this framework can also be used in classroom instructions to support the complex task of adjusting them according to each student’s needs. This can be done in several ways. For instance, the framework can provide teachers with information on what kinds of learning strategies are used. They can also assess average strategy acquisition levels among students and help teachers adjust their instructions accordingly. Moreover, effective classroom interventions can be implemented, for example, to construct subgroups that share similar levels of learning strategies, and provide tailored instruction to the levels in those respective groups.
Based on the assumption that learning strategies with item difficulties at the same level as acquisition scores are recommended, more concrete suggestions to apply these findings in classroom instruction are reflected below. When students’ average acquisition score is approximately θ = −1.0 (not so high), the following learning strategies might be effective (many of these provide ways to deal with failure in problem-solving or perceived difficulty in learning).
• Item 20: When I learned mathematics, I checked whether answers were correct after solving.
• Item 37: When I read (Japanese) text, I read slowly if I found unclear points.
• Item 18: When I learned mathematics, I tried again to solve the problems that I had failed to solve.
• Item 24: When I found unclear points while learning mathematics, I asked my friends and teachers.
• Item 9: When I read English text, I looked words up in the dictionary if I found unknown words.
If the students’ average acquisition score is approximately θ = 0 (moderate), the following learning strategies might be effective.
• Item 58: When I learned science, I summarized what I learned with tables and figures.
• Item 35: When finding difficult words in (Japanese) text, I paraphrased using easy expressions.
• Item 5: When learning English, I remembered words by identifying prefixes and suffixes.
• Item 4: When trying to remember English words, I paid attention to situations where the words were used.
If students’ average acquisition score is approximately θ = 1.0 (comparatively high), more advanced learning strategies might be appropriate. At this level, advanced strategies that utilize engagement with authentic resources, such as journal articles or community-provided learning programs outside of school, would be useful. The following items provide such examples.
• Item 60: When I learned science, I watched TV programs or read journals concerning science.
• Item 10: When I learned English, I read English text in books, magazines, or newspapers.
• Item 62: I tried to participate in scientific learning programmes held in the area or in the school.
Future directions and questions arising from this study
It is worth discussing the future research directions that emerge from this study. One such direction is to integrate more students as participants. The θ calculated in this study was based on university students’ reflections on their practices and learning during high school. However, high school is not the only stage relevant to this model. For example, Uesaka (2010) instructed junior high school students (eighth grade) in metacognitive learning strategies, reviewing how to analyze failures regarding cognitive counseling, which succeeded in inducing the transfer of learning strategies. Studies in the personal tutoring context and meta-analysis by Dignath et al. (2008) demonstrated the effectiveness of learning strategy instruction at younger school levels, specifically, elementary school. University students should also be instructed in effective learning strategies (Manalo and Henning, 2017). Integrating data right from elementary school to the university level would reveal the learning strategy acquisition order, contributing to high-quality education over the entire learning period.
The second direction is to collect a wider range of items for the analysis. This study applied IRT analysis to items that could be retrieved from previous work and classified them as cognitive, metacognitive, or external resource utilizing strategies. The study could not integrate all types of learning strategies due to time limitation in implementing the questionnaire. However, if the range of participants were expanded, it would be necessary to integrate an increasing number of learning strategies; for example, if we want to cover elementary school students, more items with easier acquisition orders should be integrated. Although this study did not do so, the IRT equating method might be worth using, wherein common items are included for every participant and item parameters are calculated consistently for every age group.
The third direction is to examine in more detail the relationship between data on the level of learning strategies and academic achievement. In this study, we divided the university levels into three categories and examined their relationship to the learning strategy levels obtained. In the future, it will be important to obtain data on individual academic achievement and verify its relationship with learning strategy levels.
Finally, it is worth examining how the use of similar strategies varies across subjects. This study used various types of learning strategies so that the findings could help teachers gain concrete suggestions regarding which strategies should be employed in different subject areas. The results raised the question of whether learning strategy use differed depending on the subject area. For example, as shown in Figure 6, the pair of ICCs comprising Item 24 (“When I found unclear points while learning mathematics, I asked my friends and teachers”) and Item 64 (“When I found unclear points when learning science, I asked my friends and teachers”), and the pair of Item 10 (“When I learned English, I read English text in books, magazines, or newspapers”) and Item 38 (“When I learned social science, I read books or articles on topics I found interesting”) were different, even though the types of learning strategies were similar. However, a pair of ICCs for Item 22 (“When I learned mathematics, I tried to consider why I failed to solve the problems”) and Item 59 (“When I learned science, I tried to consider why I failed to solve the problems”) were similar. A more detailed examination of the relationship between learning strategy use and subject areas is one of the most important topics for future investigation.
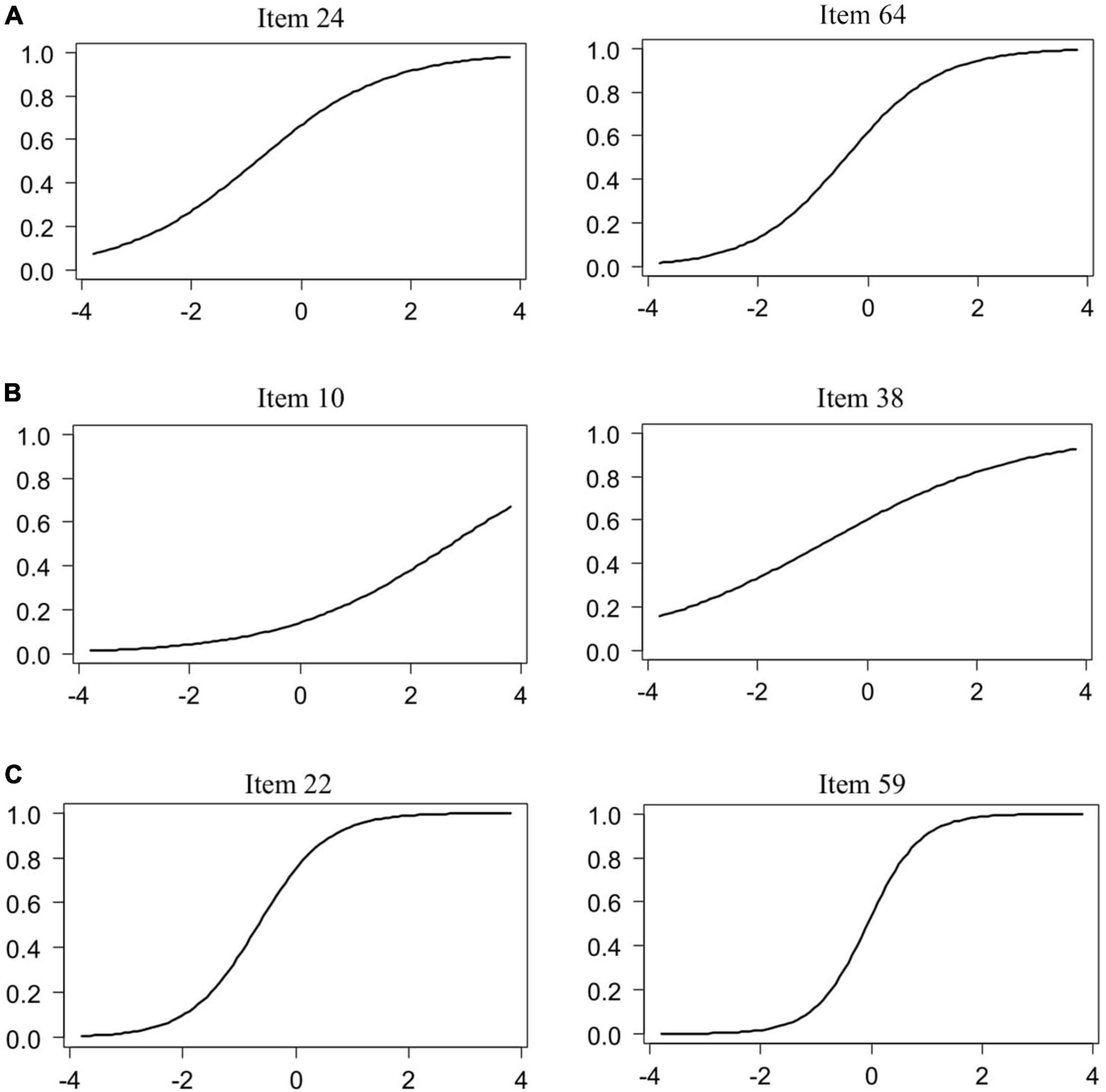
Figure 6. Comparison of item characteristic curves (ICCs) in different subjects. (A) ICCs of Item 24 (“When I found unclear points while learning mathematics, I asked my friends and teachers”) and Item 64 (“When I found unclear points when learning science, I asked my friends and teacher”). (B) ICCs of Item 10 (“When I learned English, I read English text in books, magazines, or newspapers”) and Item 38 (“When I learned social science, I read books or articles on topics I found interesting”). (C) ICCs of Item 22 (“When I learned mathematics, I tried to consider why I failed to solve problems”) and Item 59 (“When I learned science, I tried to consider why I failed to solve problems”).
Data availability statement
The datasets presented in this study can be found in online repositories. The names of the repository/repositories and accession number(s) can be found below: https://doi.org/10.6084/m9.figshare.12502028.v1.
Ethics statement
The studies involving human participants were reviewed and approved by the Life Sciences and Research Ethics and Safety Committee of the University of Tokyo. The patients/participants provided their written informed consent to participate in this study.
Author contributions
YU prepared the questionnaire, collected the data, and wrote the first draft. MS analyzed the data. SI initiated the original idea for this manuscript. All authors contributed to the article and approved the submitted version.
Funding
This research was supported by grants-in-aid (15H01976, 19H00616, and 20K20516) received from the Japan Society for the Promotion of Science.
Acknowledgments
We would like to thank Mikiko Seo of Japan Women’s University and Yoshinori Oyama of Chiba University for supporting data collection, and Emmanuel Manalo of Kyoto University for giving helpful advice on this manuscript.
Conflict of interest
The authors declare that the research was conducted in the absence of any commercial or financial relationships that could be construed as a potential conflict of interest.
Publisher’s note
All claims expressed in this article are solely those of the authors and do not necessarily represent those of their affiliated organizations, or those of the publisher, the editors and the reviewers. Any product that may be evaluated in this article, or claim that may be made by its manufacturer, is not guaranteed or endorsed by the publisher.
References
Ames, C., and Archer, J. (1988). Achievement goals in the classroom: Students’ learning strategies and motivation processes. J. Educ. Psychol. 80, 260–267. doi: 10.1037/0022-0663.80.3.260
Balsis, S., Ruchensky, J. R., and Busch, A. J. (2017). Item response theory applications in personality disorder research. Personal. Disor. 8, 298–308. doi: 10.1037/per0000209
Benesse Corporation (2017). Check from the list of deviation values of the university. Available online at: https://manabi.benesse.ne.jp/ap/daigaku/search/nanido/ (accessed April 1, 2022).
Brackenbury, T., Zickar, M. J., Munson, B., and Storkel, H. L. (2017). Applying item response theory to the development of a screening adaptation of the Goldman-Fristoe Test of Articulation-Second Edition. J. Speech Lang. Hear. Res. 60, 2672–2679. doi: 10.1044/2017_JSLHR-L-16-0392
Camahalan, F. M. G. (2006). Effects of self-regulated learning on mathematics achievement of selected Southeast Asian children. .Instruc. Psychol. 33, 194–205.
Chi, M. T. H., Siler, S. A., Jeong, H., Yamauchi, T., and Hausmann, R. G. (2001). Learning from human tutoring. Cogn. Sci. 25, 471–534. doi: 10.1016/S0364-0213(01)00044-1
Cleary, T. J., and Zimmerman, B. J. (2004). Self-regulation empowerment program: A school-based program to enhance self-regulated and self-motivated cycles of student learning. Psychol. Schools 41, 537–550. doi: 10.1002/pits.10177
Craig, S. D., Gholson, B., Ventura, M., Graesser, A. C., and The, T. R. G. (2000). Overhearing dialogues and monologues in virtual tutoring sessions: Effects on questioning and vicarious learning. Int. J. Artif. Intellig. Educ. 11, 242–253. doi: 10.2190/Q8CM-FH7L-6HJU-DT9W
Dignath, C., Buettner, G., and Langfeldt, H. P. (2008). How can primary school students learn self-regulated learning strategies most effectively? A meta-analysis on self-regulation training programmes. Educ. Res. Rev. 3, 101–129. doi: 10.1016/j.edurev.2008.02.003
Griffin, P., McGaw, B., and Care, E. (eds) (2012). Assessment and teaching of 21st century skills. Dordrecht: Springer. doi: 10.1007/978-94-007-2324-5
Guthrie, J. T., Van Meter, P., Hancock, G. R., Alao, S., Anderson, E., and McCann, A. (1998). Does concept-oriented reading instruction increase strategy use and conceptual learning from text? J. Educ. Psychol. 90, 261–278. doi: 10.1037/0022-0663.90.2.261
Guthrie, J. T., Wigfield, A., Barbosa, P., Perencevich, K. C., Taboada, A., Davis, M. H., et al. (2004). Increasing reading comprehension and engagement through concept-oriented reading instruction. J. Educ. Psychol. 96, 403–423. doi: 10.1037/0022-0663.96.3.403
Harris, C. W., and Kaiser, H. F. (1964). Oblique factor analytic solutions by orthogonal transformations. Psychometrika 29, 347–362. doi: 10.1007/BF02289601
Harris, K. R., and Graham, S. (1999). Programmatic intervention research: Illustrations from the evolution of self-regulated strategy development. Learn. Disabil. Q. 22, 251–262. doi: 10.2307/1511259
Ichihara, M., and Arai, K. (2006). Moderator effects of meta-cognition: A test in math of a motivational model Japanese. J. Educ. Psychol. 54, 199–210. doi: 10.5926/jjep1953.54.2_199
Ichikawa, S. (2005). “Cognitive counseling to improve students’ metacognition and cognitive skills,” in Applied developmental psychology: Theory, practice, and research from Japan, eds D. W. Shwalb, J. Nakazawa, and B. J. Shwalb (Charlotte: Information Age Publishing), 67–87. doi: 10.5014/ajot.2019.031450
Ichikawa, S. (ed.) (1998). Consultation and guidance on learning methods from the perspective of cognitive counseling. New York: Brain-shuppan Inc.
Ichikawa, S., Uesaka, Y., and Manalo, E. (2017). “Three approaches to promoting spontaneous use of learning strategies: Bridging the gap between research and school practice,” in Promoting spontaneous use of learning and reasoning strategies: Theory, research, and practice for effective transfer, eds E. Manalo, Y. Uesaka, and C. A. Chinn (Park Drive: Routledge), 195–210. doi: 10.4324/9781315564029-13
Inuzuka, M. (2002). The structure of reading strategies for understanding expository text. Jap. J. Educ. Psychol. 50, 152–162. doi: 10.5926/jjep1953.50.2_152
Kariya, T., and Rosenbaum, J. E. (1987). Self-selection in Japanese junior high schools: A longitudinal study of students’ educational plans. Sociol. Educ. 60, 168–180. doi: 10.2307/2112274
Kesici, Ş, Erdoğan, A., and Ahmet, K. (2009). Predicting college students’ mathematics anxiety by motivational beliefs and self-regulated learning strategies. College Stud. J. 43, 631–642.
Lynch, D. J. (2006). Motivational factors, learning strategies and resource management as predictors of course grades. College Stud. J. 40, 423–428. doi: 10.1186/s12913-016-1423-5
Manalo, E., and Henning, M. (2017). “Second language vocabulary learning: Are students cognitive misers and if so, why?,” in Promoting spontaneous use of learning and reasoning strategies: Theory, research and practice for effective transfer, eds E. Manalo, Y. Uesaka, and C. A. Chinn (Park Drive: Routledge), 91–102. doi: 10.4324/9781315564029-7
Manalo, E., Uesaka, Y., and Chinn, C. A. (eds) (2017). Promoting spontaneous use of learning and reasoning strategies: Theory, research, and practice for effective transfer. Park Drive: Routledge. doi: 10.4324/9781315564029
Marton, F., and Säljö, R. (1976). On qualitative differences in learning: I. Outcome and process. Br. J. Educ. Psychol. 46, 4–11. doi: 10.1111/j.2044-8279.1976.tb02980.x
Min, S., and Aryadoust, V. (2021). A systematic review of item response theory in language assessment: Implications for the dimensionality of language ability. Stud. Educat. Evaluat. 68:100963. doi: 10.1016/j.stueduc.2020.100963
Murayama, K. (2003). Test format and learning strategy use. Jap. J. Educ. Psychol. 51, 1–12. doi: 10.5926/jjep1953.51.1_1
Murayama, K. (2007). “Gakushu horyaku: kodomo jishin no jiritsuteki na gakushu wo mezashi te [Learning Strategies: for children’s self-regulated learning],” in Zettai yakudatsu kyoiku shinri gaku, ed. T. Fujita (Kyoto: Mineruvua Shoboo), 85–100
Nolen, S. B. (1988). Reasons for studying: Motivational orientations and study strategies. Cogn. Instruct. 5, 269–287. doi: 10.1207/s1532690xci0504_2
Oyama, S. (2009). Strategies used for learning English vocabulary and occurrence of errors in English. Jap. J. Educ. Psychol. 57, 73–85. doi: 10.5926/jjep.57.73
Partchev, I., Maris, G., and Hattori, T. (2017). irtoys: A collection of functions related to item response theory (IRT). R package version 0.2.1. Available online at: https://CRAN.R-project.org/package=irtoys (accessed April 1, 2022).
Pintrich, P. R., and de Groot, E. V. (1990). Motivational and self-regulated learning components of classroom academic performance. J. Educ. Psychol. 82, 33–40. doi: 10.1037/0022-0663.82.1.33
Pintrich, P. R., Smith, D. A. F., Garcia, T., and McKeachie, W. J. (1993). Reliability and predictive validity of the Motivated Strategies for Learning Questionnaire (MSLQ). Educ. Psychol. Measur. 53, 801–813. doi: 10.1177/0013164493053003024
Ramdass, D., and Zimmerman, B. J. (2008). Effects of self-correction strategy training on middle school students’ self-efficacy, self-evaluation, and mathematics division learning. J. Adv. Acad. 20, 18–41. doi: 10.4219/jaa-2008-869
Ramsden, P. (1988). “Context and strategy,” in Learning strategies and learning styles, ed. R. R. Schmeck (New York, NY: Plenum), 159–184. doi: 10.1007/978-1-4899-2118-5_7
Revelle, W. (2018). psych: Procedures for personality and psychological research. R package version 1.8.4. Available online at: https://CRAN.R-project.org/package=psych (accessed April 1, 2022).
Roscoe, R. D., and Chi, M. T. H. (2008). Tutor learning: The role of explaining and responding to questions. Instruct. Sci. 36, 321–350. doi: 10.1007/s11251-007-9034-5
Rychen, D. S., and Salganik, L. H. (eds) (2003). Key competencies for a successful life and a well-functioning society. Göttingen: Hogrefe.
Salden, R. J. C. M., Aleven, V., Schwonke, R., and Renkl, A. (2010). The expertise reversal effect and worked examples in tutored problem solving. Instruct. Sci. 38, 289–307. doi: 10.1007/s11251-009-9107-8
Sawyer, R. J., Graham, S., and Harris, K. R. (1992). Direct teaching, strategy instruction, and strategy instruction with explicit self-regulation: Effects on the composition skills and self-efficacy of students with learning disabilities. J. Educ. Psychol. 84, 340–352. doi: 10.1037/0022-0663.84.3.340
Schunk, D. H., and Zimmerman, B. J. (eds) (1994). Self-regulation of learning and performance: Issues and educational applications. New York: Lawrence Erlbaum Associates, Inc.
Schunk, D. H., and Zimmerman, B. J. (eds) (1998). Self-regulated learning: From teaching to self-reflective practice. New York, NY: Guilford Publications.
Schwonke, R., Wittwer, J., Aleven, V., Salden, R., Krieg, C., and Renkl, A. (2007). “Can tutored problem solving benefit from faded worked-out examples?,” in Proceedings of EuroCogSci 07. The European Cognitive Science Conference 2007, eds S. Vosniadou, D. Kayser, and A. Protopapas (Erlbaum), 59–64.
Shell, D. F., and Husman, J. (2008). Control, motivation, affect, and strategic self-regulation in the college classroom: A multidimensional phenomenon. J. Educat. Psychol. 100, 443–459. doi: 10.1037/0022-0663.100.2.443
Shimoyama, H. (1984). A longitudinal study on the career decision-making process of a high school. Jap. J. Educ. Psychol. 32, 206–211. doi: 10.5926/jjep1953.32.3_206
Tabachnick, S. E., Miller, R. B., and Relyea, G. E. (2008). The relationships among students’ future-oriented goals and subgoals, perceived task instrumentality, and task-oriented self-regulation strategies in an academic environment. J. Educ. Psychol. 100, 629–642. doi: 10.1037/0022-0663.100.3.629
Tang, K. L. (1996). Polytomous item response theory models and their applications in large-scale testing programs: Review of literature. Princeton: Educational Testing Service.
Uchida, N. (2021). Developmental differences in English vocabulary learning strategies: A cross-sectional study of secondary-level students. Jap. J. Educ. Psychol. 69, 366–381. doi: 10.5926/jjep.69.366
Uesaka, Y. (2010). How learning strategy use transfers across different school subjects: A case study on promotion of spontaneous use of “lesson induction.”. Jap. J. Educ. Psychol. 58, 80–94. doi: 10.5926/jjep.58.80
Uesaka, Y. (2012). Reviews of psychological studies and educational practices focusing on improving student learning skills: Suggestions for addressing the gap between academic studies and classroom practices. Annu. Rep. Educ. Psychol. Japan 51, 105–117. doi: 10.5926/arepj.51.105
Uesaka, Y., Shiba, S., and Ichikawa, S. (eds) (2017). Case studies of learning support at 2017 academic year: Cognitive counseling practices for fostering independent learners. Tokyo: The University of Tokyo.
Weinstein, C. E., and Mayer, R. E. (1986). “The teaching of learning strategies,” in Handbook of research on teaching, ed. M. C. Wittrock (London: Macmillan), 315–327.
Zimmerman, B. J. (2015). “Self-regulated learning: Theories, measures, and outcome,” in International Encyclopedia of the Social & Behavioral Sciences (Second Edition), ed. J. D. Wright (Amsterdam: Elsevier), 541–546. doi: 10.1016/B978-0-08-097086-8.26060-1
Zimmerman, B. J., and Martinez-Pons, M. (1990). Student differences in self-regulated learning: Relating grade, sex, and giftedness to self-efficacy and strategy use. J. Educ. Psychol. 82, 51–59. doi: 10.1037/0022-0663.82.1.51
Keywords: learning strategy use, item response theory (IRT), self-regulated learning, individual differences, students’ learning strategy acquisition
Citation: Uesaka Y, Suzuki M and Ichikawa S (2022) Analyzing students’ learning strategies using item response theory: Toward assessment and instruction for self-regulated learning. Front. Educ. 7:921844. doi: 10.3389/feduc.2022.921844
Received: 16 April 2022; Accepted: 11 July 2022;
Published: 24 August 2022.
Edited by:
Yiming Cao, Beijing Normal University, ChinaReviewed by:
Chongyang Wang, Beijing Normal University, ChinaYi Wang, Beijing Normal University, China
Copyright © 2022 Uesaka, Suzuki and Ichikawa. This is an open-access article distributed under the terms of the Creative Commons Attribution License (CC BY). The use, distribution or reproduction in other forums is permitted, provided the original author(s) and the copyright owner(s) are credited and that the original publication in this journal is cited, in accordance with accepted academic practice. No use, distribution or reproduction is permitted which does not comply with these terms.
*Correspondence: Yuri Uesaka, eXVyaS51ZXNha2FAY3QudS10b2t5by5hYy5qcA==