- 1Department of Education and Child Studies, Leiden University, Leiden, Netherlands
- 2Leiden Institute for Brain and Cognition (LIBC), Leiden University, Leiden, Netherlands
- 3Graduate School of Teaching (ICLON), Leiden University, Leiden, Netherlands
In this exploratory descriptive study, we use eye-tracking technology to examine teachers’ visual inspection of Curriculum-Based Measurement (CBM) progress graphs. More specifically, we examined which elements of the graph received the most visual attention from teachers, and to what extent teachers viewed graph elements in a logical sequence. We also examined whether graph inspection patterns differed for teachers with higher- vs. lower-quality graph descriptions. Participants were 17 fifth- and sixth-grade teachers. Participants described two progress graphs while their eye-movements were registered. In addition, data were collected from an expert to provide a frame of reference for interpreting the teachers’ eye-tracking data. Results revealed that, as a group, teachers devoted less visual attention to important graph elements and inspected the graph elements in a less logical sequence than did the expert, however, there was variability in teachers’ patterns of graph inspection, and this variability was linked to teachers’ abilities to describe the graphs. Directions for future studies and implications for practice are discussed.
Introduction
Teachers are increasingly expected to use data to guide and improve their instructional decision-making. In general education, this data-use process often is referred to as Data- Based or Data-Driven Decision Making (e.g., see Mandinach, 2012; Schildkamp et al., 2012). In special education it is referred to as Data-Based Instruction or Individualization (e.g., see Kuchle et al., 2015; Jung et al., 2017). Despite differences in terminology, researchers in general and special education draw upon similar data-use models, which typically include the following steps: (a) identify and define the problem; (b) collect and analyze data; (c) interpret/make sense of the data; (d) make an instructional decision (e.g., see Mandinach, 2012; Deno, 2013; Beck and Nunnaley, 2021; Vanlommel et al., 2021). It is not only the data-use models that are similar across general and special education, but also the concerns about teachers’ ability to successfully implement the models, especially their ability to implement steps (c) and (d). In both general and special education, research has shown that teachers have difficulty interpreting data and making effective instructional decisions based on these interpretations (e.g., see Stecker et al., 2005; Datnow and Hubbard, 2016; Gleason et al., 2019; Espin et al., 2021a; Mandinach and Schildkamp, 2021).
Although it is clear from the research that teachers have difficulty interpreting data and making instructional decisions, it is not clear why teachers have such difficulties. Answering the why question requires an understanding of the processes underlying teachers’ data-based decision making. In the current study, we examine the processes underlying teachers’ data-based decision making, most specifically, the processes underlying teachers’ ability to interpret or make sense of data. The data that teachers interpret in the current study are Curriculum-Based Measurement (CBM) data.
Curriculum-Based Measurement
CBM is a system that teachers use to monitor the progress of and evaluate the effectiveness of interventions for students with learning difficulties (Deno, 1985, 2003). CBM involves frequent, repeated, administration of short, simple measures that sample global performance in an academic area such as reading. CBM measures have been shown to be valid and reliable indicators of student performance and progress (see, for example, Wayman et al., 2007; Yeo, 2010; Shin and McMaster, 2019).
To assist teachers in interpreting the data, CBM scores are placed on a progress graph that depicts student growth over time in response to various iterations of an intervention (see Figure 1). The graph consists of: (a) baseline data, representing the student’s beginning level of performance in comparison to peers; (b) a long-range goal, representing the desired level of performance at the end of the school year; (c) a goal line drawn from the baseline to the long-range goal, representing the desired rate of progress across the year; (d) data points representing the student’s performance on weekly measurement probes; (e) phases of instruction separated by vertical lines, representing the initial intervention and adjustments to that intervention, and; (f) slope lines, representing the student’s rate of growth within each instructional phase.
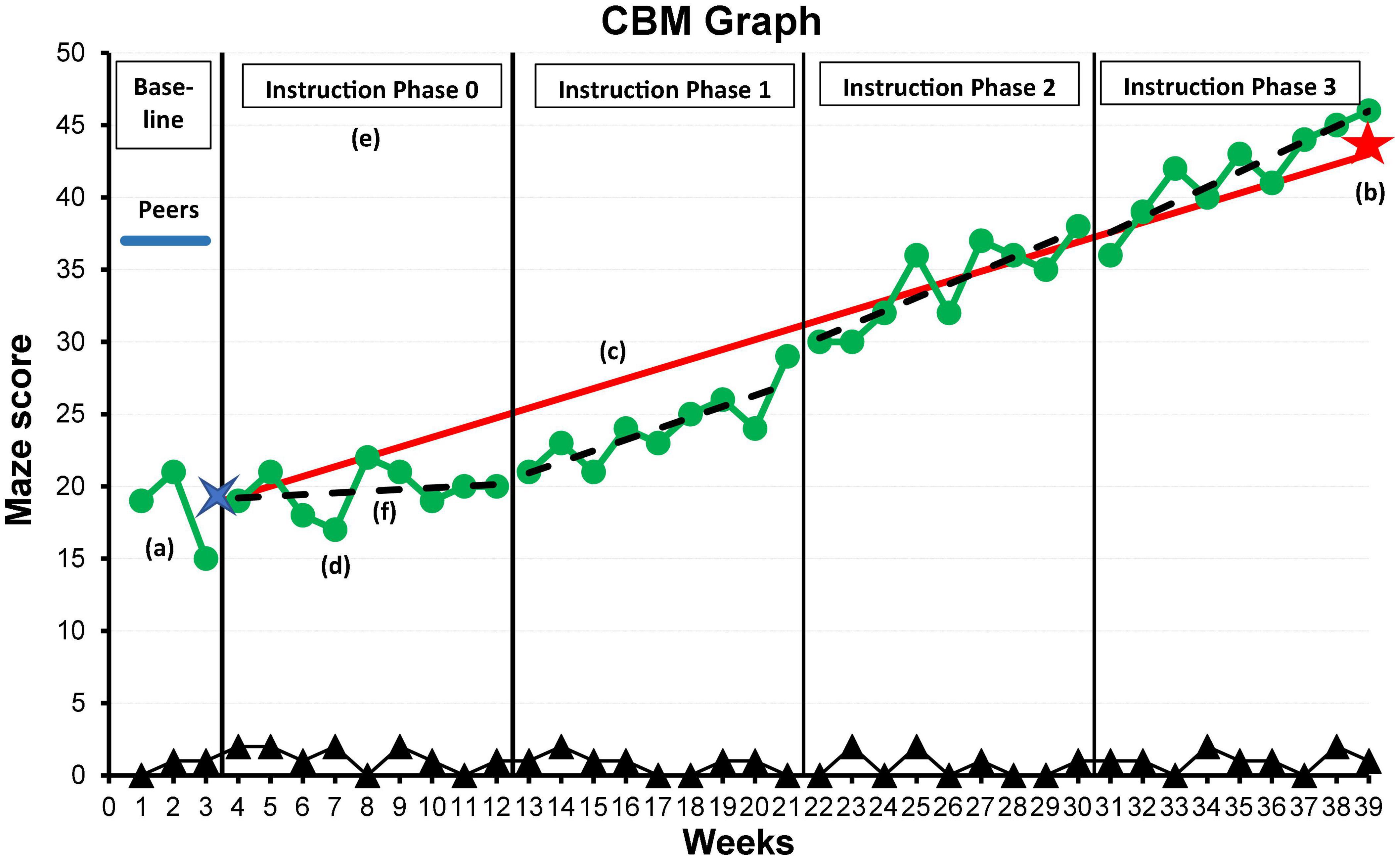
Figure 1. Sample CBM progress graph: (a) baseline data; (b) long-range goal; (c) goal line; (d) data points; (e) phase of instruction; (f) slope (growth) line.
The progress graph lies at the heart of CBM because it guides teachers’ instructional decision-making (Deno, 1985). When using CBM, teachers regularly inspect the CBM graph to evaluate student progress within each phase of instruction. Based on their interpretation of the data, teachers make one of the following instructional decisions:
(1) Modify/adjust the intervention, when the slope line is below and/or less steep than the goal line, indicating that the student is performing below the expected level and/or progressing at a rate slower than expected;
(2) Continue the intervention as is, when the slope line is at a level equal to and parallel to the goal line, indicating that the student is progressing at the expected level and rate of progress;
(3) Raise the goal, when the slope line is above and parallel to or steeper than the goal line, indicating that the student is progressing above the expected level and/or progressing more rapidly than expected.
Once the teacher has made an instructional decision, the teacher implements the decision, and then continues to collect data to evaluate the effects of the decision on student progress. This ongoing cycle of data interpretation, instructional decision-making, data interpretation, instructional decision-making, etc. is an integral part of Data-based Instruction (DBI; Deno, 1985; National Center on Intensive Intervention, 2013). When implemented appropriately, DBI results in individually tailored interventions for students with learning difficulties that, in turn, lead to significant improvements in the academic performance of the students (Filderman et al., 2018; Jung et al., 2018). Implementing DBI “appropriately,” however, requires that teachers accurately read and interpret the CBM progress graphs.
Curriculum-Based Measurement graph comprehension
The ability to read and interpret—to “derive meaning from”—graphs is referred to as graph comprehension (Friel et al., 2001, p. 132). Graph comprehension can be influenced by both the characteristics of the graph and the viewer (Friel et al., 2001). Regarding the viewer—which is the focus of the present study—research has demonstrated that preservice and inservice teachers have difficulty describing CBM graphs in an accurate, complete, and coherent manner (Espin et al., 2017; van den Bosch et al., 2017; Wagner et al., 2017; Zeuch et al., 2017). For example, van den Bosch et al. (2017) found that inservice teachers were less complete and coherent in describing CBM graphs than CBM experts, and were less likely than the experts to compare student data to the goal line, to compare data across instructional phases, and to link data to instruction. Making such comparisons and links are essential for using CBM data to guide instruction.
Although research has made it clear that teachers have difficulty comprehending CBM graphs, it has not made clear why teachers have such difficulties. Little is known about the processes underlying teachers’ ability to read and interpret CBM progress graphs. Knowing more about these processes might help to pinpoint where problems lie and might provide insights into how to improve teachers’ graph comprehension. One technique for gaining insight into the processes underlying completion of visual tasks such as graph reading is eye- tracking.
Eye-tracking
Eye-tracking is a technology used to register people’s eye movements while completing a visual task. Eye-movements reveal how attention is allocated when viewing a stimulus to complete a task and provide insight into the cognitive strategies used to complete the task (Duchowski, 2017). Eye-tracking has been used in reading to gain understanding of and insight into the processes underlying the reading of text (e.g., see Rayner, 1998; Rayner et al., 2006). Specific to teacher behaviors, eye-tracking has been used to study teachers’ visual perception of classroom events (van den Bogert et al., 2014), awareness of student misbehavior (Yamamoto and Imai-Matsumura, 2012), and perceptions of problematic classroom situations (Wolff et al., 2016). In the area of graph reading, eye-tracking has been used to gain understanding into the processes underlying interpretation of graphs and to examine differences in processes related to the type and complexity of the graph (Vonder Embse, 1987; Carpenter and Shah, 1998; Okan et al., 2016).
The current study is to the best of our knowledge the first to use eye-tracking to study teachers’ reading of CBM progress graphs. As such, it is an exploratory, descriptive study. Because there were no previous studies to guide us, the first challenge we faced in designing the study was to know what to expect of teachers. To address this challenge, we collected eye-tracking data from a member of the research team with expertise in CBM (see section “Materials and method”) to provide a frame of reference for interpreting the teachers’ data. A second challenge was to determine which variables to consider when analyzing the eye-tracking data. To address this challenge, we drew upon previous eye-tracking studies that compared experts’ and novices’ eye movements.
Eye-movements: Experts vs. novices
Across a wide variety of fields including medicine, sports, biology, meteorology, forensics, reading, and teaching, eye-tracking has been used to examine differences between experts and novices in their comprehension of visual stimuli and their use of strategies used to complete visual tasks (e.g., see Canham and Hegarty, 2010; Jarodzka et al., 2010; Al-Moteri et al., 2017; Watalingam et al., 2017; Beach and McConnel, 2019). A consistent finding to emerge from these studies is that experts devote more attention to task-relevant parts of visual stimuli and approach visual tasks in a more goal-directed or systematic manner than do novices. Similar findings have emerged from eye-tracking research on the comprehension of graphs. For example, Vonder Embse (1987) found that experts fixated significantly longer on important parts of mathematical graphs than did novices, and that these differences were related to overall comprehension of the graphs. Similarly, Okan et al. (2016) found that viewers with high graph literacy devoted more time to viewing relevant features of graphs than participants with low graph literacy.
Drawing upon this previous body of eye-tracking research, we decided to examine the extent to which teachers devoted attention to various elements of CBM progress graphs and the extent to which they viewed the graphs in a systematic, orderly manner.
Purpose of the study
This study was an exploratory, descriptive study aimed at describing teachers’ patterns of visual inspection when reading and interpreting CBM progress graphs. Teachers viewed CBM progress graphs and completed a think-aloud in which they described what they were looking at. As they completed their think-alouds, teachers’ eye- movements were registered. Results from the think-aloud portion of the study have been reported elsewhere (van den Bosch et al., 2017). In this paper, we focus on the eye-tracking data. Our overall purpose is to develop and illustrate a method that can be used to examine teachers’ inspection of CBM progress graphs and to delineate potential patterns of visual inspection that can be more closely examined in future research.
Our general research question was: What are teachers’ patterns of visual inspection when reading and interpreting CBM progress graphs? We addressed three specific research questions:
1. To what extent do teachers devote attention to various elements of CBM graphs?
2. To what extent do teachers inspect the elements of CBM graphs in a logical, sequential manner?
3. Do the visual inspection patterns examined in research questions 1 and 2 differ for teachers with higher- vs. lower-quality graph descriptions (i.e., think-alouds)?
Materials and methods
Participants
Teachers
Participants were 17 fifth- and sixth-grade teachers (15 female; Mage = 42.9 years, SD = 11.77, range: 26–60) from eight different schools in the Netherlands, who were recruited via convenience sampling. The original sample consisted of 19 teachers. Inspection of the demographic data collected from the teachers revealed that two of the teachers had completed a university course on CBM prior to the study. Because none of the other participating teachers had prior knowledge of or experience with CBM, we decided to exclude the data for these two teachers from the study.
Participating teachers had all completed a teacher education program and held bachelor’s degrees in education. One teacher also held a master’s degree in psychology. Teachers had on average 17.82 years (SD = 10.11, range: 5–37) of teaching experience. All teachers had students with reading difficulties/dyslexia in their classes. Although the 17 participating teachers were not familiar with CBM prior to the start of the study, they were familiar with the general concept of progress monitoring because Dutch elementary-school teachers are required to monitor the progress of their students via standardized tests given one to two times per school year.
Curriculum-Based Measurement expert
To provide a frame of reference for interpreting the teachers’ data, a member of the research team with expertise in CBM completed the same eye-tracking task as the teachers prior to the start of the study. The CBM expert was a university professor in the area of learning disabilities, with a Ph.D in educational psychology/special education, and with more than 23 years of experience conducting research and training on CBM, and with more than 40 publications focused on CBM and/or reading interventions for students with learning disabilities.
Materials: Curriculum-Based Measurement graphs
Two researcher-made CBM graphs were used in the study. The graphs depicted fictitious but realistic student data and were designed to capture data patterns often seen in CBM progress graphs. The data points and data patterns differed across the two graphs, but the set-up for each graph was the same, and included baseline data for the student and peers, a long-range goal, a goal line, five phases of instruction (labeled as Phases 0–4), data points, slope (growth) lines drawn through the data points within each phase, and a legend (see sample graph, Figure 2; note that the graphs shown to the participants did not have any shaded areas). The order in which the two graphs were presented was counterbalanced (AB vs. BA) across teachers. The graphs for this study were modified versions of those used in Wagner et al. (2017). The graph titles, scales, and labels were changed to reflect CBM maze-selection rather than reading-aloud and were written in Dutch.
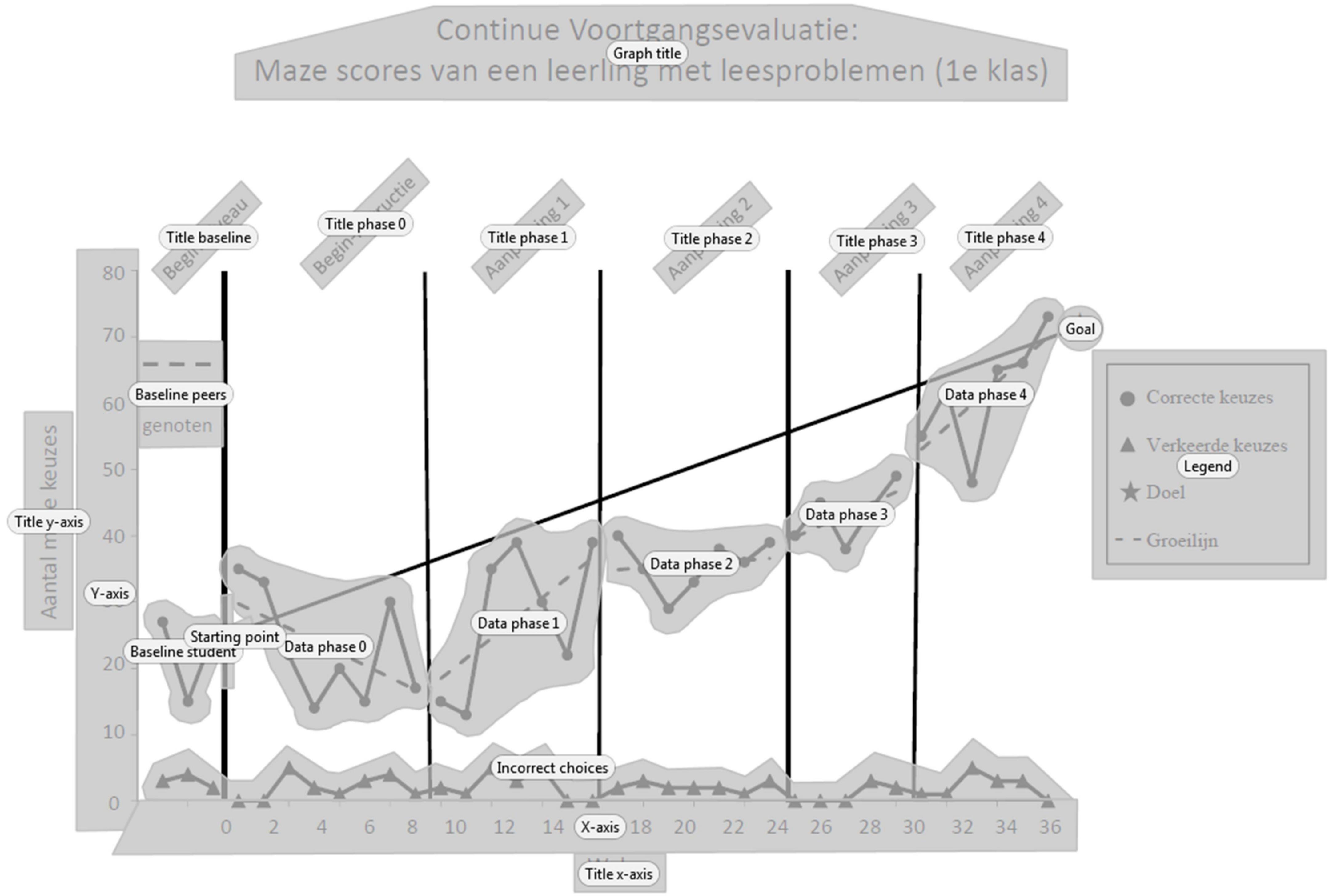
Figure 2. Sample CBM graph with the following AOIs (as depicted by the shaded areas): Framing (i.e., graph title, titles axes, x- and y-axis, legend), Baseline (i.e., title, data student, data peers), Starting point (begin point goal line), Instructional phases 0–4 (i.e., titles, data points, and slope lines), Incorrect choices (triangles at bottom of graph), and Long-range goal (end point goal line). Figure adapted from Wagner et al. (2017).
Eye-tracking procedures
To examine teachers’ patterns of graph inspection, their eye-movements were registered as they described each graph. Prior to describing the graphs, teachers were shown a sample CBM graph and given a short description of the graph. They were told that the graph depicted the reading progress of one student receiving intensive reading instruction, and that the scores on the graph represented the student’s correct and incorrect choices on weekly administered 2-min maze-selection probes. Each graph element was identified and described briefly to the teachers (see van den Bosch et al., 2017, for the full description).
Teachers were then positioned in front of the eye-tracker screen. They were told that they would be shown a CBM graph and that they would be asked to “think out loud” while looking at the graph. They were asked to tell all they were seeing and thinking, including what they were looking at and why they were looking at it. After calibrating the eye-tracker, instructions were repeated, and the first graph was presented. After teachers had described the first graph, the graph was removed from the screen, the instructions were again repeated, and the other graph was presented. There were no time limits for the graph descriptions.
Data were collected in individual sessions at the teachers’ schools by trained doctoral students and a trained research assistant. Two data collectors were present during each data collection session. One data collector operated the eye-tracker while the other instructed the participant and audio-taped the think-aloud. Instructions were read aloud from a script.
Eye-tracking apparatus and software
To register the eye movements of the participants, a Tobii T120 remote eye tracker was used. The Tobii T120 Eye Tracker is robust with regard to participants’ head movements and its calibration procedure is quick and simple (Tobii Technology, 2010). Participants were positioned in front of the Tobii eye-tracker screen so that the distance between their eyes and the screen was approximately 60 cm. The data sampling rate was set at 60 Hz. The accuracy of the Tobii T120 Eye Tracker typically is 0.5 degrees, which implies an average error of 0.5 centimeter between the measured and the actual gaze direction (Tobii Technology, 2010).
Tobii Studio 3.4.8 and IBM SPSS Statistics 23 were used to process and to descriptively analyze the eye-tracking data.
Eye-tracking data
Establishing areas of interest
To analyze the eye-tracking data, Areas of Interest (AOIs) were defined for the graphs (see Figure 2, shaded areas). We categorized the AOIs into 10 graph elements: (1) Framing (areas related to the graph set-up, including graph title, x- and y-axes and titles, the legend); (2) Baseline (areas related to baseline data, including title, baseline student, baseline peers); (3) Starting point (beginning point of the goal line), (4)–(8): Instructional phases 0, 1, 2, 3, and 4, respectively (areas related to instructional phases, including titles, data points, and slope lines within each phase), (9) Incorrect choices (triangles at the bottom of graph), and (10) Long-range goal (end point of the goal line). These ten graph elements were similar to those identified in previous research on CBM graph comprehension (Espin et al., 2017; Wagner et al., 2017), and to those coded in the think-aloud portion of the study (see van den Bosch et al., 2017). Due to the nature of CBM graphs in which different graph elements are near each other, some of the AOIs were adjacent to each other, and in some instances, overlapped slightly.
Fixation duration and fixation sequence
Two types of eye-tracking data were examined in this study: fixation duration data and fixation sequence data. Fixations serve as measures of visual attention and are defined as a short period of time in which the eyes remain still to perceive a stimulus, that is, to cognitively process the stimulus (Holmqvist et al., 2011). Fixation duration is the sum of the duration of all fixations within a particular area of the stimulus and fixation sequence is the order in which participants look at each area.
Fixation duration served as an indicator of participants’ distribution of visual attention. The minimal fixation duration setting was set to 200 ms, meaning that a fixation was not registered unless the participant looked at a specific point for at least 200 ms. This cutoff point was chosen because typical values for fixations range from 200 to 300 ms (Holmqvist et al., 2011). For each participant the total duration of fixations (in sec.) was computed for each AOI via the eye-tracker software, after which the percentage of visual attention devoted to each of the 10 graph elements was calculated.
Fixation sequence served as an indicator of the extent to which teachers inspected CBM graph elements in a logical, sequential manner. Fixation sequences revealed the order in which participants viewed the CBM graph elements. For each participant, the sequence of fixations was computed via the eye-tracker software. The fixation sequence data were provided in the form of strings of graph element names (e.g., Baseline, Phase 1, Phase 2, Phase 1, etc.).
Coding teachers’ visual inspection of Curriculum-Based Measurement graphs
Attention devoted to elements of the graph
To address research question 1, to what extent teachers devoted attention to various elements of the CBM graph, for each teacher, the total duration of fixations (in sec.) was computed for each AOI via the eye-tracker software, after which the percentage of visual attention devoted to each graph element was calculated. Percentages were then totaled across teachers.
Sequence of visual inspection patterns
To address research question 2, to what extent teachers inspected CBM graph elements in a logical, sequential manner, the extent to which teachers’ sequence of fixations followed a logical, sequential order were examined. As a first step, an “ideal sequence” was created based on the order in which CBM graphs would be used for instructional decision-making (see Espin et al., 2017; Wagner et al., 2017). The teachers’ sequence of fixations was then compared to this ideal sequence. The ideal sequence used in the study was similar to the ideal sequence used to code the think-aloud data from the CBM graph descriptions (see van den Bosch et al., 2017). The ideal sequence for the eye-tracking data was: Framing (i.e., fixating on the elements related to the set-up of the graph), Baseline, Goal setting, Instructional phases 0, 1, 2, 3, and 4, and Goal achievement. The element incorrect choices was not included in the sequential analysis because it spanned multiple phases. Further, because participants could inspect the long-range goal either as a part of goal setting or goal achievement, the following rule was applied: If participants fixated on the long-range goal prior to fixating on any of the instructional phases, the fixation was coded under goal setting. If participants fixated on the long-range goal after fixating on at least one instructional phase, the fixation was coded as goal achievement.
The coding sheet presented in Figure 3 was used to code the percentage of teachers’ sequences following the ideal sequence. Along the top and down the left side of the coding sheet, the graph elements are listed. Sequences between graph elements were recorded using tally marks. To illustrate, let us assume that the viewer examined the graph elements in the following order: Framing—Baseline—Goal setting—Baseline—Phase 0—Phase 1—Phase 2—Phase 1—Phase 3—Phase 4—Phase 3—Goal achievement. The first viewing sequence in this example is Framing (FR) to Baseline (BL). This is recorded in the coding sheet in Figure 3 with a tally mark at the intersection of the FR row and the BL column. The second sequence is Baseline (BL) to Goal setting (GS), which is recorded with a tally mark at the intersection of the BL row and the GS column, and so forth. After all sequences were recorded on the coding sheet, the percentage of sequences following the ideal sequence was calculated.
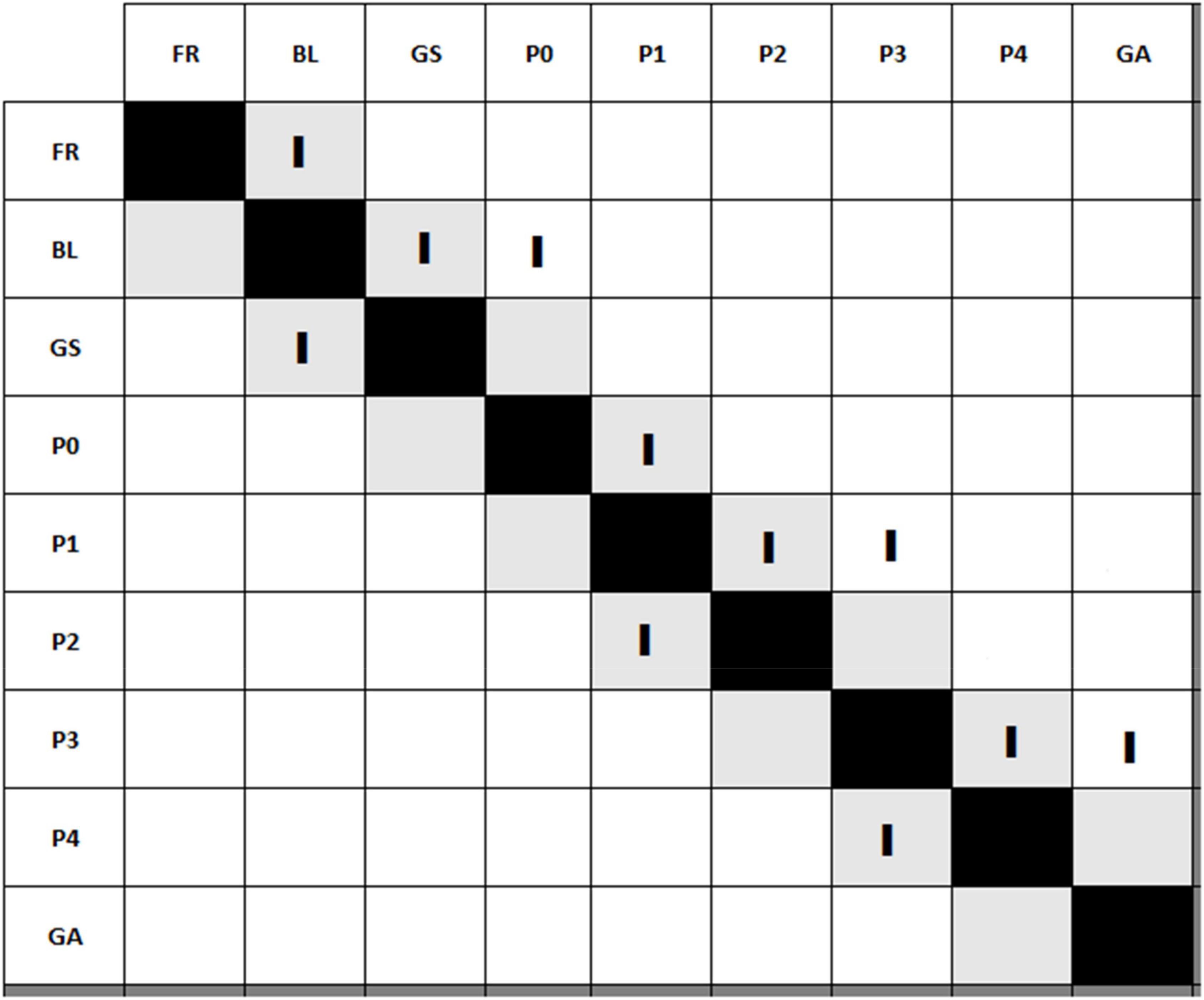
Figure 3. Coding sheet for calculating the logical sequence percentages from the fixation sequence data. FR, Framing; BL, Baseline; GS, Goal setting; P0, Instructional phase 0; P1, Instructional phase 1; P2, Instructional phase 2; P3, Instructional phase 3; P4, Instructional phase 4; GA, Goal achievement. Figure adapted from Espin et al. (2017).
Fixation sequences (strict approach)
The ideal sequence (Framing to Baseline to Goal setting to Instructional phases 0–4, to Goal achievement) is depicted by the light gray boxes above the diagonal in Figure 3. To determine the percentage of sequences following the ideal sequence, the number of tallies in the light gray boxes was divided by the total number of tallies. The greater the percentage of tallies in the light gray boxes above the diagonal, the more closely the participant’s graph inspection matched the ideal sequence. In the example in Figure 3, five of 11 sequences fall in the light gray boxes above the diagonal, resulting in a logical sequence percentage of 45.5%. We refer to this approach as the “strict” calculation approach. After calculating the sequences using this strict approach, we calculated sequences using a more liberal approach that took into account lookbacks between adjacent graph elements.
Fixation sequence (liberal approach)
As we were coding the fixation sequences, we observed that the teachers (as well as the CBM expert) often looked back and forth between adjacent graph elements—in particular, between adjacent instructional phases. Looking back and forth between graph elements (lookbacks) might reflect the fact that a viewer is comparing information across elements. Such comparisons are an important aspect of higher- level graph comprehension (Friel et al., 2001), and are an essential aspect of CBM data-based decision-making (see van den Bosch et al., 2017). We thus decided to calculate the fixation sequences in a second, more liberal, manner that took into account “lookbacks” between adjacent graph elements.
To calculate logical sequences using the liberal approach, we counted the number of tallies in the light gray boxes directly above and below the diagonal, and then divided this number by the total number of tallies. In the example in Figure 3, eight of 11 sequences fell in the light gray boxes either above or below the diagonal, resulting in a logical sequence percentage of 72.7% for the liberal approach. We also counted the subset of tallies between adjacent instructional phases only (as opposed to between all graph elements). Comparing data across adjacent instructional phases (e.g., P1 to P2 or P2 to P1) is essential for determining whether instructional adjustments have been effective. In the example in Figure 3, five of the 11 instances of lookbacks were between adjacent instructional phases, resulting in an instructional phase lookback percentage of 45.5%.
Intercoder agreement
The fixation sequence data for all participants were coded by a trained doctoral student and a trained research assistant. Intercoder agreement was 99.94%. There was one disagreement between coders, which was resolved through discussion.
Visual inspection patterns: Higher- vs. lower quality graph descriptions
Question 3 addressed whether the visual inspection patterns examined in research questions 1 and 2 differed for teachers with higher- vs. lower-quality graph descriptions. By addressing this question, we were able to link the eye-tracking data to the think-aloud data. Recall that teachers described the graphs via a think-aloud procedure while their eye-movements were being registered. These think- alouds were then compared to the think-alouds of three CBM experts (different from the expert in this study; see van den Bosch et al., 2017).1 For the current study, we selected the two teachers with the highest- quality think-alouds (i.e., most similar to think-alouds of the experts), and the two teachers with the lowest-quality think-alouds (i.e., least similar to think-alouds of the experts) and compared their patterns of graph inspection.
Results
Fixation duration: Attention devoted to Curriculum-Based Measurement graph elements
The first research question was: To what extent do teachers devote attention to various elements of CBM graphs? Data are reported as average scores across the two graphs. The overall viewing time for the teachers was on average 107.91 sec per graph (SD = 59.83; range 53–252.5 sec). This was compared to 283 sec per graph for the CBM expert.
The percentages of visual attention (i.e., fixation duration) devoted to each graph element for the teachers are reported in Table 1. Data for the CBM expert also are reported to provide a frame of reference (columns 2 and 1, respectively). Teachers devoted a fair amount of visual attention to FR (approximately 26%), which was similar to the value for the CBM expert (approximately 23%). Teachers devoted the largest proportion of visual attention to the five phases of instruction (approximately 58%), and, except for Phase 3, devoted approximately equal amounts of attention to each phase (approximately 11–14%). This pattern was somewhat different from that of the CBM expert. The expert also devoted the largest proportion of visual attention to the phases of instruction, but the percentage was larger than that of the teachers (approximately 70%). In addition, the expert did not devote equal amounts of attention to each phase, but rather devoted an increasing amount of attention across phases, devoting little attention to Phase 0 (approximately 3%), and much more attention to Phase 4 (approximately 25%). Finally, teachers devoted approximately 7.5 and 7% to Baseline and Incorrect choices, respectively, compared to 4 and 0.2% for the CBM expert.
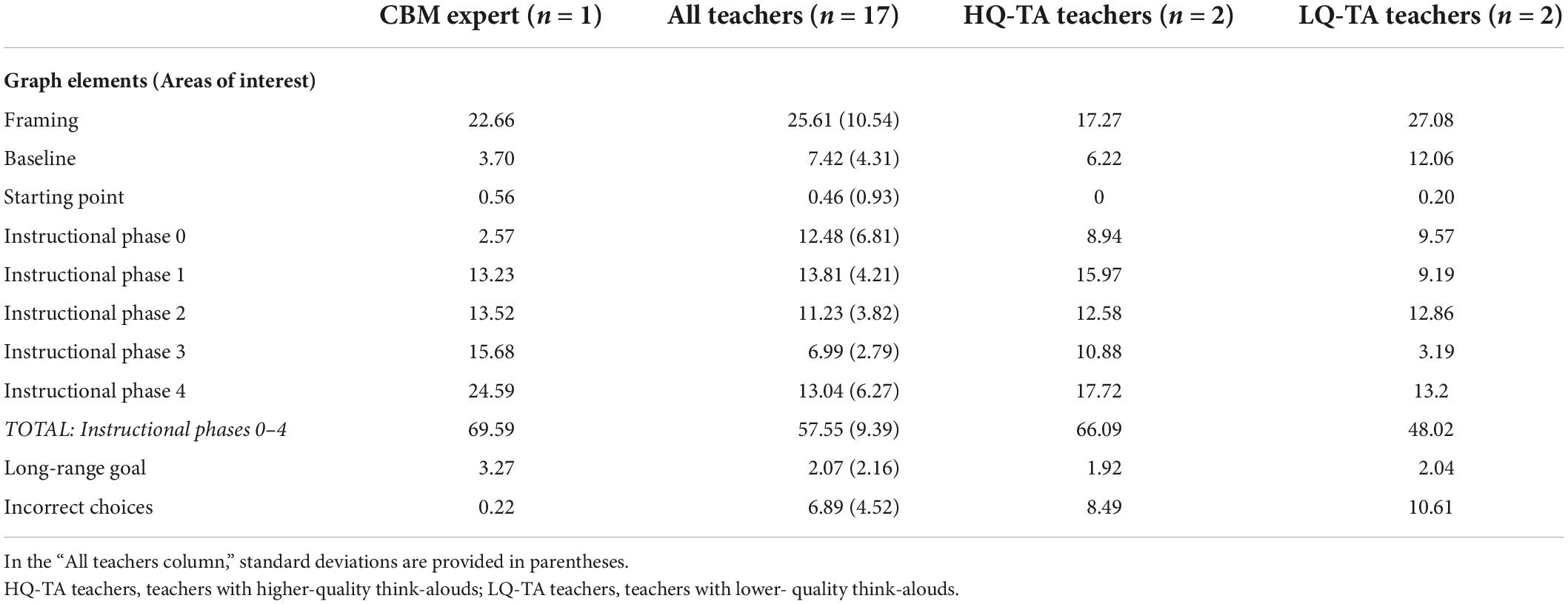
Table 1. Mean percentage of visual attention devoted to graph elements for CBM expert, all teachers, and HQ-TA and LQ-TA teachers.
Fixation sequence: Logical sequence of visual inspection patterns
The second research question of the study was: To what extent do teachers inspect the elements of the CBM graphs in a logical, sequential manner? Recall that we calculated fixation sequence using both a strict and liberal approach, and also calculated the percentage of lookbacks between adjacent instructional phases only. Using the strict calculation approach, the mean logical sequence percentage for the teachers was 24.59% (SD = 5.96, range: 15.78–37.50). Using the liberal calculation approach, it was 40% (SD = 10.8, range: 18.16–56.49). These percentages were smaller than the 40.83 and 74.11% for the CBM expert for the strict and liberal approaches, respectively. The mean percentage of lookbacks between adjacent instructional phases for the teachers was 30.44% (SD = 9.7) compared to 49.62% for the CBM expert.
Visual inspection patterns: Higher- vs. lower quality graph descriptions
Visual attention data for the two teachers with higher- and lower-quality think alouds (HQ-TA and LQ-TA) are reported in the last two columns of Table 1. The data reveal that HQ-TA teachers spent a smaller proportion of time viewing Framing and Baseline than did LQ-TA teachers (approximately 17 vs. 27%, respectively, for Framing, and 6 vs. 12%, respectively, for Baseline), and a larger proportion of time viewing the five instructional phases (approximately 66 vs. 48%, respectively).
We also compared the fixation sequence data for the teachers with higher- and lower- quality think-alouds. Using the strict calculation approach, the mean logical sequence percentage for HQ-TA teachers was 33.57%, compared to 16.94% for the LQ-TA teachers. Using the liberal calculation approach, the mean logical sequence percentage for HQ-TA teachers was 50.39%, compared to 30.19% for the LQ-TA teachers. Finally, the mean percentages of lookbacks between adjacent instructional phases for the HQ-TA was 37.75%, compared to 18.13% for the LQ-TA teachers.
Discussion
The purpose of this study was to examine how teachers visually inspected CBM graphs, and thereby, to gain insight into the processes underlying teachers CBM graph comprehension. The three research questions we addressed in the study were: (1) To what extent do teachers devote attention to various elements of CBM graphs? (2) To what extent do teachers inspect the elements of CBM graphs in a logical, sequential manner? (3) Do the visual inspection patterns examined in research questions 1 and 2 differ for teachers with higher- vs. lower-quality graph descriptions (i.e., think-alouds)? To provide a frame of reference for interpreting the teachers’ data, data also were collected from a member of the research team who was a CBM expert.
Teachers’ visual inspection of Curriculum-Based Measurement graph elements
The overall viewing time per graph for the teachers was about 2.5 times shorter than for the CBM expert. Given that the task had no time limits, the differences are notable, and suggest that teachers inspected the graphs in a less detailed manner than did the expert.
Examinations of the distribution of visual attention provide more insight into these differences. Teachers devoted most of their visual attention (58%) to the data in the instructional phases, which may not be that surprising given that 5 of the 10 graph elements that were categorized in AOIs were instructional phases. Nonetheless, it is positive that the teachers devoted a considerable amount of time to viewing the data in instructional phases. If teachers are to make sound data-based instructional decisions based on CBM graphed data, they must inspect the data within and between instructional phases to draw conclusions about student progress and the effectiveness of instruction. Despite this positive note, it is important to note the discrepancy between the teachers and CBM expert, who devoted nearly 70% of visual attention to the instructional phases, a much higher percentage than for the teachers. Further, there were differences between the teachers and CBM expert in the distribution of attention across the phases. Except for phase 3, teachers’ attention was fairly evenly distributed across the phases, whereas the expert’s attention increased across phases, from 3% in Phase 0 to 25% in Phase 4.
The discrepancies between the teachers and the CBM expert suggest that the teachers were less likely than the expert to focus attention on the most relevant aspects of the graph, a finding that fits with previous eye-tracking research. Previous research has shown that novices are less likely than experts to focus attention on relevant aspects of visual stimuli within the context of a task and are more likely to skim over non-relevant aspects (e.g., Vonder Embse, 1987; Canham and Hegarty, 2010; Jarodzka et al., 2010; Okan et al., 2016; Al-Moteri et al., 2017). With respect to the CBM graph, the most relevant areas of the graph are the Instructional Phases because they provide information on the effectiveness of instruction, and on the need to adjust that instruction. Within the instructional phases, the final phase is especially relevant because the data in this phase represent the overall success of the teacher’s instruction across the school year, and signal whether the student will achieve the long-range goal.
Supporting the idea that teachers are less likely than the expert to focus on relevant aspects of the graph and more likely to focus on irrelevant aspects of the graph, is the percentages of visual attention devoted to Incorrect choices. Teachers focused nearly 7% of their visual attention on Incorrect choices, compared to 0% for the expert. Within CBM it is the number of correct, not incorrect, choices that reflect growth. The number of incorrect choices is informational, but not as relevant for instructional decision-making as is the number of correct choices. Teachers may have tended to focus on incorrect choices because in typical classroom assessments, incorrect answers are used to calculate grades and to determine where students experience difficulties.
Logical sequence of visual inspection patterns
The second research question addressed the extent to which teachers inspected the CBM graphs elements in a logical (ideal) sequence; that is, a sequence that reflected the order in which CBM graphs would be used for instructional decision-making. For the teachers, 25% of their fixation sequences followed the ideal sequence, whereas for the CBM expert, it was 41%. Using a liberal calculation approach, which took into account looking back and forth between adjacent graph elements, the percentages were 40% for the teachers vs. 74% for the CBM expert. These results reveal that, as a group, teachers viewed the graphs in a less logical, sequential manner than the expert. The results are in line with previous eye-tracking studies comparing experts and novices that have shown that experts are more systematic and goal-directed in completing a visual task than novices (e.g., Jarodzka et al., 2010; Al-Moteri et al., 2017). The results also fit with the think-aloud data from the larger study, and with previous CBM graph comprehension research, which have shown that preservice and inservice teachers describe CBM graphs in a less logical, sequential manner than do CBM experts (van den Bosch et al., 2017; Wagner et al., 2017).
Differences between teachers and the CBM expert also were seen in the percentage of lookbacks between adjacent instructional phases. For teachers, 30% of their fixation sequences involved lookbacks between adjacent instructional phases, whereas for the CBM expert it was 50% of the sequences. These results suggest that the teachers did not often visually compare data points and slope lines between adjacent instructional phases, something that is important for making decisions about the effectiveness of instructional adjustments. These results again mirror the results of the think-aloud data, which showed that teachers were less likely to make data-to-data comparisons than were CBM experts in the larger study (van den Bosch et al., 2017).
In sum, the eye-tracking data indicate which aspects of CBM graph reading may be most problematic for the teachers and most in need of attention when teachers are learning to implement CBM. Specifically, the results suggest that teachers may need to learn to devote more attention to relevant aspects of the graphs such as the instructional phases (especially the later phases), and less attention to irrelevant aspects of the graphs, such as incorrect choices. Furthermore, teachers may need to learn how to view graph elements in a sequence that reflects the time-sensitive nature of the graph. They may also need to learn to compare graph elements, especially how to compare data and slope lines across adjacent phases of instruction, so that they can use the data to evaluate the effects of instruction and of instructional adjustments.
Visual inspection patterns: High- vs. low-quality think-alouds
By comparing visual inspection patterns for teachers with higher- and lower-quality think alouds, we were able to link the eye-tracking data to teachers’ ability to accurately and coherently describe CBM graphs. In general, the results demonstrated that visual inspection patterns for teachers with high-quality think alouds were more similar to those of the expert than visual inspection patterns for teachers with low-quality think alouds. Regarding fixation duration data, the HQ-TA teachers devoted more attention to the data in the instructional phases than did the LQ-TA teachers, with a difference of nearly 18%. With regard to the fixation sequence data, the HQ-TA teachers inspected the CBM graph elements in a more logical sequence, regardless of whether the strict or liberal calculation approach was used, and had a larger percentage of lookbacks between adjacent instructional phases, than did the LQ- TA teachers. Differences between the HQ-TA and LQ-TA teachers were approximately 20% for all three measures.
These data suggest that some teachers struggle more than others in reading, interpreting, and comprehending CBM graphs. The think-aloud data for the LQ-TA teachers (see van den Bosch et al., 2017) had shown that they were not able to describe CBM graphs in a complete and coherent manner and did not make within-data comparisons when describing the graphs. These differences were reflected in these teachers’ patterns of graph inspection. The LQ-TA teachers spent relatively little time on the most relevant aspects of the graph (i.e., instructional phases), and inspected the graphs in a less logical, sequential manner than did the HQ-TA teachers. Further, based on the lookback data, the LQ-TA teachers did not appear to make comparisons between adjacent phases of instruction. In short, although results of this study suggest that all teachers might benefit from specific, directed instruction in reading and comprehending CBM progress graphs, the data comparing the HQ-TA and LQ-TA teachers suggest that some teachers will need more intensive and directed instruction than others.
Limitations
The present study was an exploratory, descriptive study that used eye-tracking technology to examine teachers’ patterns of inspection when reading CBM graphs. Results of this study should be viewed as a springboard for developing future studies with larger and more diverse samples. The study had several limitations. First, the sample was a small sample of convenience, and consisted of teachers with relatively little experience with CBM. Although appropriate for an exploratory study, it is important to replicate the study with a larger more representative sample, and with teachers who have used CBM for an extended period of time. Second, the data used to provide a frame of reference were collected from only one CBM expert, and this expert was a member of the research team who was familiar with the graphs used in the study. Although the graph descriptions of this expert were nearly identical to the graph descriptions given by the three CBM experts from the think-aloud portion of the study [who were not familiar with the graphs (van den Bosch et al., 2017)] it is still a limitation. The study should be replicated with other CBM experts.
Third, the AOIs were in some cases adjacent to each other or even overlapped slightly. We elected to use graphs that were set up identically to those used in the Wagner et al. (2017) so that we could tie our data to that earlier study. These graphs had ecological validity in that they were typical of the type of progress graphs actually seen by teachers when using CBM. That said, bordering/overlapping AOIs are not desirable in analyzing eye-tracking data, and thus the data patterns found in this explorative study should be viewed as suggestive, and should be verified in future research with graphs that are designed so that AOIs do not border on/overlap with each other.
Implications for practice and for future research
Although teachers are expected to closely monitor the progress of students with severe and persistent learning difficulties, and to evaluate the effectiveness of the given instruction for these students with systems like CBM, the results of the present study suggest that teachers have difficulty inspecting CBM graphs, with some teachers having more difficulty than others. Combining the results of the current study with the results of previous think-aloud studies on CBM graph reading (Espin et al., 2017; van den Bosch et al., 2017; Wagner et al., 2017), the results suggest the need to provide teachers with specific, directed instruction on how to inspect, read, and interpret CBM graphs. Unfortunately, such instruction may not typically be a part of CBM professional development training (see Espin et al., 2021b), which is worrisome given that student achievement improves only when teachers adequately respond to CBM data with instructional and goal changes (see Stecker et al., 2005).
Graph-reading instruction could be improved in different ways. For example, teachers could be taught where to direct their attention when reading CBM graphs. Keller and Junghans (2017) used such an approach for helping viewers to read medical graphs and demonstrated that providing the viewers with written instructions on reading medical graphs while arrows pointed to the task-relevant parts of the graphs increased visual attention for the task-relevant graph parts. Alternatively, teachers could be shown a video of the eye-movements of a CBM expert completing a think-aloud description of a CBM graph. The video would illustrate how to inspect the graph in a detailed, logical, sequential manner. Such Eye Movement Modeling Examples (EMMEs) have been used in other areas such as medical education (Jarodzka et al., 2012; Seppänen and Gegenfurtner, 2012) and digital reading (Salmerón and Llorens, 2019). Teachers’ ability to read and interpret progress graphs could also be improved via specific, directed instruction focused on CBM graph reading, combined with multiple practice opportunities, as demonstrated by van den Bosch et al. (2019).
A final method of improving teachers’ ability to read and interpret CBM progress graphs would be to design the graphs in a way to direct teacher attention to key elements of the graph and to provide graph-reading supports. For example, the slopes could be presented in different colors that correspond to the decision to be made (red for adjusting instruction, green for keeping instruction as is, blue for raising the goal), or graph elements could be hidden or highlighted with a click of the mouse. For a review of graph supports that have been effective in assisting teachers in CBM decision-making (see Stecker et al., 2005; Fuchs et al., 2021).
Conclusion
The results of this exploratory, descriptive study provide insights into how teachers visually inspect CBM progress-monitoring graphs and provide a basis for designing future studies focused on teachers’ ability to read and interpret student progress graphs. The results of the present study revealed differences between teachers and the CBM expert, and between teachers with higher- and lower-quality think-alouds, in terms of how long participants inspected relevant graph elements and the order in which they inspected the elements. In comparison to the expert, teachers as a group were found to be less adept at focusing on the relevant aspects of the CBM graphs, at inspecting the graph elements in a logical sequence, and at comparing data across adjacent instructional phases. However, there were differences between teachers: Teachers in this study who produced better descriptions of the CBM graphs (HQ-TA teachers) were more adept at graph inspection than teachers who produced poorer descriptions of the graphs (LQ-TA teachers). The results of this study, in combination with the results of think-aloud studies of CBM graph comprehension, highlight potential areas of need for teachers, and provide guidance regarding the design of CBM instruction for teachers.
Before making firm conclusions about teachers’ inspection of CBM progress graphs, it will be important to replicate the present study with a larger and more diverse sample, and with independent CBM experts. An important aspect of future research will be to tie teachers’ graph descriptions and patterns of graph inspection to their actual use of CBM data for instructional decision-making, and, ultimately, to student achievement. With a larger data set, statistical models could also be applied to the data (see for example, Man and Harring, 2021) to determine whether particular processing patterns/profiles predict teachers’ CBM graph comprehension, teachers’ appropriate use the data for instructional decision making, and, ultimately, student achievement.
Data availability statement
The data supporting the conclusions of this article will be made available by the authors for specific purposes upon request.
Ethics statement
This study was reviewed and approved by the Ethics Committee, Education and Child Studies. Written informed consent to participate in the study was provided by the participants.
Author contributions
All authors listed have made a substantial, direct, and intellectual contribution to the work, and approved it for publication.
Acknowledgments
We would like to thank the teachers who participated in this research, also Anouk Bakker for her help with the preparation of this manuscript, and Arnout Koornneef for feedback on the design and analysis.
Conflict of interest
The authors declare that the research was conducted in the absence of any commercial or financial relationships that could be construed as a potential conflict of interest.
Publisher’s note
All claims expressed in this article are solely those of the authors and do not necessarily represent those of their affiliated organizations, or those of the publisher, the editors and the reviewers. Any product that may be evaluated in this article, or claim that may be made by its manufacturer, is not guaranteed or endorsed by the publisher.
Footnotes
- ^ Unfortunately, no eye-tracking data could be collected from the three experts involved in the think-aloud portion of the study. For this reason, we collected eye-tracking data from a different CBM expert for the current study.
References
Al-Moteri, M. O., Symmons, M., Plummer, V., and Cooper, S. (2017). Eye tracking to investigate cue processing in medical decision-making: A scoping review. Comput. Hum. Behav. 66, 52–66. doi: 10.1016/j.chb.2016.09.022
Beach, P., and McConnel, J. (2019). Eye tracking methodology for studying teacher learning: A review of the research. Int. J. Res. Method Edu. 42, 485–501. doi: 10.1080/1743727X.2018.1496415
Beck, J. S., and Nunnaley, D. (2021). A continuum of data literacy for teaching. Stud. Educ. Eval. 69:100871.
Canham, M., and Hegarty, M. (2010). Effects of knowledge and display design on comprehension of complex graphics. Learn. Instr. 20, 155–166. doi: 10.1016/j.learninstruc.2009.02.014
Carpenter, P. A., and Shah, P. (1998). A model of the perceptual and conceptual processes in graph comprehension. J. Exp. Psychol. Appl. 4, 75–100. doi: 10.1037/1076-898X.4.2.75
Datnow, A., and Hubbard, L. (2016). Teacher capacity for and beliefs about data-driven decision making: A literature review of international research. J. Educ. Change 17, 7–28. doi: 10.1007/s10833-015-9264-2
Deno, S. L. (1985). Curriculum-based measurement: The emerging alternative. Except. Child. 52, 219–232. doi: 10.1177/001440298505200303
Deno, S. L. (2003). Developments in curriculum-based measurement. J. Spec. Educ. 37, 184–192. doi: 10.1177/00224669030370030801
Deno, S. L. (2013). “Problem-solving assessment,” in Assessment for Intervention: A Problem-Solving Approach, 2nd Edn, eds R. Brown-Chidsey and K. J. Andren (New York, NY: Guilford Press), 10–36.
Duchowski, A. T. (2017). Eye Tracking Methodology: Theory and Practice, 3rd Edn. Cham: Springer International Publishing.
Espin, C. A., Förster, N., and Mol, S. (2021a). International perspectives on understanding and improving teachers’ data-based instruction and decision making: Introduction to the special series. J. Learn. Disabil. 54, 239–242. doi: 10.1177/00222194211017531
Espin, C. A., van den Bosch, R. M., van der Liende, M., Rippe, R. C. A., Beutick, M., Langa, A., et al. (2021b). A systematic review of CBM professional development materials: Are teachers receiving sufficient instruction in data-based decision-making?. J. Learn. Disabil. 54, 256–268. doi: 10.1177/0022219421997103
Espin, C. A., Wayman, M. M., Deno, S. L., McMaster, K. L., and de Rooij, M. (2017). Data- based decision-making: Developing a method for capturing teachers’ understanding of CBM graphs. Learn. Disabil. Res. Pract. 32, 8–21. doi: 10.1111/ldrp.12123
Filderman, M. J., Toste, J. R., Didion, L. A., Peng, P., and Clemens, N. H. (2018). Data-based decision making in reading interventions: A synthesis and meta-analysis of the effects for struggling readers. J. Spec. Educ. 52, 174–187. doi: 10.1177/0022466918790001
Friel, S. N., Curcio, F. R., and Bright, G. W. (2001). Making sense of graphs: Critical factors influencing comprehension and instructional implications. J. Res. Math. Educ. 32, 124–158. doi: 10.2307/749671
Fuchs, L. S., Fuchs, D., Hamlett, C. L., and Stecker, P. (2021). Bringing data-based individualization to scale: A call for the next- generation of teacher supports. J. Learn. Disabil. 54, 319–333. doi: 10.1177/0022219420950654
Gleason, P., Crissey, S., Chojnacki, G., Zukiewicz, M., Silva, T., Costelloe, S., et al. (2019). Evaluation of Support for Using Student Data to Inform Teachers’ Instruction. (NCEE 2019-4008). Washington, DC: U.S. Department of Education, National Center for Education Evaluation and Regional Assistance.
Holmqvist, K., Nyström, M., Andersson, R., Dewhurst, R., Jarodzka, H., and van de Weijer, J. (2011). Eye Tracking: A Comprehensive Guide to Methods and Measures. New York, NY: Oxford University Press.
Jarodzka, H., Balslev, T., Holmqvist, K., Nyström, M., Scheiter, K., Gerjets, P., et al. (2012). Conveying clinical reasoning based on visual observation via eye- movement modelling examples. Instr. Sci. 40, 813–827. doi: 10.1007/s11251-012-9218-5
Jarodzka, H., Scheiter, K., Gerjets, P., and van Gog, T. (2010). In the eyes of the beholder: How experts and novices interpret dynamic stimuli. Learn Instr. 20, 146–154. doi: 10.1016/j.learninstruc.2009.02.019
Jung, P.-G., McMaster, K. L., and delMas, R. C. (2017). Effects of early writing intervention delivered within a data-based instruction framework. Except. Child. 83, 281–297. doi: 10.1177/0014402916667586
Jung, P.-G., McMaster, K. L., Kunkel, A. K., Shin, J., and Stecker, P. (2018). Effects of data- based individualization for students with intensive learning needs: A meta-analysis. Learn. Disabil. Res. Pract. 33, 144–155. doi: 10.1111/ldrp.12172
Keller, C., and Junghans, A. (2017). Does guiding toward task-relevant information help improve graph processing and graph comprehension of individuals with low or high numeracy? An eye-tracker experiment. Med. Decis. Making 37, 942–954. doi: 10.1177/0272989X17713437
Kuchle, L. B., Edmonds, R. Z., Danielson, L. C., Peterson, A., and Riley-Tillman, T. C. (2015). The next big idea: A framework for integrated academic and behavioral intensive intervention. Learn. Disabil. Res. Pract. 30, 150–158. doi: 10.1111/ldrp.12084
Man, K., and Harring, J. R. (2021). Assessing preknowledge cheating via innovative measures: A multiple-group analysis of jointly modeling item responses, response times, and visual fixation counts. Educ. Psychol. Meas. 81, 441–465.
Mandinach, E. B. (2012). A perfect time for data use: Using data-driven decision making to inform practice. Educ. Psychol. 47, 71–85. doi: 10.1080/00461520.2012.667064
Mandinach, E. B., and Schildkamp, K. (2021). Misconceptions about data-based decision making in education: An exploration of the literature. Stud. Educ. Eval. 69:100842. doi: 10.1016/j.stueduc.2020.100842
National Center on Intensive Intervention (2013). Data-Based Individualization: A Framework for Intensive Intervention. Washington, DC: Office of Special Education, U.S. Department of Education.
Okan, Y., Galesic, M., and Garcia-Retamero, R. (2016). How people with low and high graph literacy process health graphs: Evidence from eye-tracking. J. Behav. Decis. Making 29, 271–294. doi: 10.1002/bdm.1891
Rayner, K. (1998). Eye movements in reading and information processing: 20 years of research. Psychol. Bull. 124, 372–422. doi: 10.1037/0033-2909.124.3.372
Rayner, K., Chace, K. H., Slattery, T. J., and Ashby, J. (2006). Eye movements as reflections of comprehension processes in reading. Sci. Stud. Read. 10, 241–255. doi: 10.1207/s1532799xssr1003_3
Salmerón, L., and Llorens, A. (2019). Instruction of digital reading strategies based on eye-movements modeling examples. J. Educ. Comput. Res. 57, 343–359. doi: 10.1177/0735633117751605
Schildkamp, K., Ehren, M., and Lai, M. K. (2012). Editorial article for the special issue on data- based decision making around the world: From policy to practice to results. Sch. Eff. Sch. Improv. 23, 123–131. doi: 10.1080/09243453.2011.652122
Seppänen, M., and Gegenfurtner, A. (2012). Seeing through a teacher’s eye improves students’ imagining interpretation. Med. Educ. 46, 1113–1114. doi: 10.1111/medu.12041
Shin, J., and McMaster, K. (2019). Relations between CBM (oral reading and maze) and reading comprehension on state achievement tests: A meta-analysis. J. Sch. Psychol. 73, 131–149. doi: 10.1016/j.jsp.2019.03.005
Stecker, P. M., Fuchs, L. S., and Fuchs, D. (2005). Using curriculum-based measurement to improve student achievement: Review of research. Psychol. Sch. 42, 795–819. doi: 10.1002/pits.20113
Tobii Technology (2010). Tobii T/X Series Eye Trackers: Product Description. Available online at: https://www.tobiipro.com/siteassets/tobii-pro/product-descriptions/tobii-pro-tx-product-description.pdf
van den Bogert, N., van Bruggen, J., Kostons, D., and Jochems, W. (2014). First steps into understanding teachers’ visual perception of classroom events. Teach. Teach. Educ. 37, 208–216. doi: 10.1016/j.tate.2013.09.001
van den Bosch, R., Espin, C. A., Chung, S., and Saab, N. (2017). Data-based decision-making: Teachers’ comprehension of curriculum-based measurement progress-monitoring graphs. Learn. Disabil. Res. Pract. 32, 46–60. doi: 10.1111/ldrp.12122
van den Bosch, R. M., Espin, C. A., Pat-El, R. J., and Saab, N. (2019). Improving teachers’ comprehension of curriculum-based measurement progress-monitoring graphs. J. Learn. Disabil. 52, 413–427. doi: 10.1177/0022219419856013
Vanlommel, K., Van Gasse, R., Vanhoof, J., and Van Petegem, P. (2021). Sorting pupils into their next educational track: How strongly do teachers rely on data-based or intuitive processes when they make the transition decision?. Stud. Educ. Eval. 69:100865.
Vonder Embse, C. B. (1987). An Eye Fixation Study of Time Factors Comparing Experts and Novices When Reading and Interpreting Mathematical Graphs. Ph.D. thesis. Columbus, OH: Ohio State University.
Wagner, D. L., Hammerschmidt-Snidarich, S., Espin, C. A., Seifert, K., and McMaster, K. L. (2017). Pre-service teachers’ interpretation of CBM progress monitoring data. Learn. Disabil. Res. Pract. 32, 22–31. doi: 10.1111/ldrp.12125
Watalingam, R. D., Richetelli, N., Pelz, J. B., and Speir, J. A. (2017). Eye tracking to evaluate evidence recognition in crime scene investigations. Forensic Sci. Int. 208, 64–80. doi: 10.1016/j.forciint.2017.08.012
Wayman, M. M., Wallace, T., Wiley, H. I., Tichá, R., and Espin, C. A. (2007). Literature synthesis on curriculum-based measurement in reading. J. Spec. Educ. 41, 85–120. doi: 10.1177/00224669070410020401
Wolff, C. E., Jarodzka, H., van den Bogert, N., and Boshuizen, H. P. A. (2016). Teacher vision: Expert and novice teachers’ perception of problematic classroom management scenes. Instr. Sci. 44, 243–265. doi: 10.1177/0022487114549810
Yamamoto, T., and Imai-Matsumura, K. (2012). Teachers’ gaze and awareness of students’ behavior: Using an eye tracker. Compr. Psychol. 2:6. doi: 10.2466/01.IT.6
Yeo, S. (2010). Predicting performance on state achievement tests using curriculum-based measurement in reading: A multilevel meta-analysis. Remedial Spec. Educ. 31, 412–422. doi: 10.1177/0741932508327463
Keywords: progress monitoring, teachers, graph comprehension, eye-tracking, CBM
Citation: van den Bosch RM, Espin CA, Sikkema-de Jong MT, Chung S, Boender PDM and Saab N (2022) Teachers’ visual inspection of Curriculum-Based Measurement progress graphs: An exploratory, descriptive eye-tracking study. Front. Educ. 7:921319. doi: 10.3389/feduc.2022.921319
Received: 15 April 2022; Accepted: 22 August 2022;
Published: 30 September 2022.
Edited by:
Erica Lembke, University of Missouri, United StatesReviewed by:
Kaiwen Man, University of Alabama, United StatesFrancis O’Donnell, National Board of Medical Examiners, United States
Copyright © 2022 van den Bosch, Espin, Sikkema-de Jong, Chung, Boender and Saab. This is an open-access article distributed under the terms of the Creative Commons Attribution License (CC BY). The use, distribution or reproduction in other forums is permitted, provided the original author(s) and the copyright owner(s) are credited and that the original publication in this journal is cited, in accordance with accepted academic practice. No use, distribution or reproduction is permitted which does not comply with these terms.
*Correspondence: Christine A. Espin, espinca@fsw.leidenuniv.nl