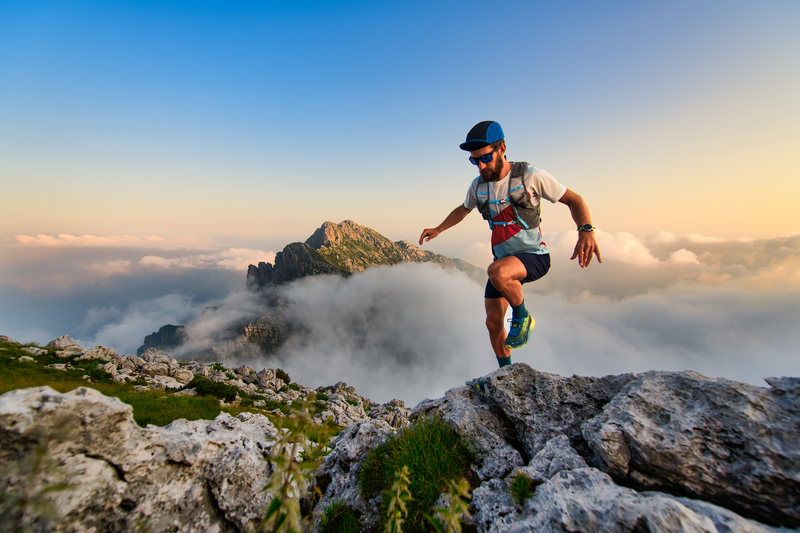
95% of researchers rate our articles as excellent or good
Learn more about the work of our research integrity team to safeguard the quality of each article we publish.
Find out more
ORIGINAL RESEARCH article
Front. Educ. , 18 August 2022
Sec. Educational Psychology
Volume 7 - 2022 | https://doi.org/10.3389/feduc.2022.920498
This article is part of the Research Topic Progress Monitoring and Data-Based Decision-Making in Inclusive Schools View all 14 articles
Digital learning platforms (DLP) provide various types of information about student learning when used for learning and practice. This learning data holds potential for individualized instruction, which has become increasingly necessary for adequately addressing learners’ individual needs. For primary schools in particular, this is important for developing inclusive schools. However, despite the potential of DLP and the learning data that can be obtained from them, they are rarely used by teachers. Furthermore, little is known about factors that lead teachers to use learning data for instruction and individual support. To address this research gap, we conducted an online cross-sectional survey study of N = 272 primary school teachers in Germany. After describing the respondents’ current and previous usage of learning data from DLP, we used structural equation modeling (SEM) to test the influence of predictors on respondents’ intention to use as well as their usage of learning data from DLP. Finally, we discuss the need for increased usage of learning data in teacher education and training, contributing to ongoing debates about the usage of digital learning data in educational research and practice.
Today, teachers face a variety of challenges in their daily school life, including an increased number of administrative tasks, a heterogeneous student population, and the digitization of schools (Schmid et al., 2017; Tondeur et al., 2018). Overcoming these challenges can be exhausting, but also holds potential for the further development of schools and teaching. More precisely, combining different challenges can provide additional opportunities for development. For example, digital learning platforms (DLP) can support the development of individual learning requirements in the context of inclusive education. This is especially relevant for primary schools due to their high heterogeneity (Schwab et al., 2017). The usage of DLP contributes to the ability of all learners to participate in the classroom (Vanbecelaere et al., 2020; Schaumburg, 2021). Regarding the support of learners, DLP hold an added value of particular importance: the availability of learning data. Using this learning data, teachers can track and reflect on individual learning processes and implement appropriate learning support (FitzGerald et al., 2018). To date, such data usage is found primarily in research on Data-Based Decision Making (DBDM) and Learning Analytics (e.g., Mandinach and Schildkamp, 2020; Blumenthal et al., 2021; Krein and Schiefner-Rohs, 2021). Although the benefits of using (digital) learning data have been highlighted in previous research, the factors that promote or hinder primary school teachers’ usage of learning data from DLP, especially in Germany, remain mostly unconsidered. Therefore, based on the Theory of Planned Behavior (TPB) (Ajzen, 1991) and in consideration of further potentially influential factors, we conducted a cross-sectional survey study among German primary school teachers to investigate the antecedents of their intention to use and usage of learning data from DLP.
First, we consider digital media which collect learning data for the teacher, DLP, and their potential for individualized practice. We then address the usage of learning data in the context of instructional design. These two topics provide the contextual basis for the study to examine the intention to use and usage of learning data from DLP. Second, the introduction of the TPB will allow us to predict the intention to use as well as the usage of learning data from DLP based on an established model.
According to Böhme et al. (2020), the aims of using digital media in schools are, on the one hand, the promotion of a critical use of digital media and, on the other hand, the support of learning. Especially in highly heterogeneous inclusive school settings, there is a great potential of digital media, as digital media have the potential to increase the participation of all students in the classroom (Vanbecelaere et al., 2020; Schaumburg, 2021). In this context, digital media can support teachers with diagnostic information and thus foster individualized learning offers (Schaumburg, 2021).
There are several types of digital media that can improve student learning, such as intelligent tutorial systems, drill-and-practice programs, or learning management systems (Nattland and Kerres, 2009; Petko, 2014). However, in the context of this study, we did not study a specific type of digital media. Reinhold et al. (2020, p. 1) emphasize “that it is not the mere medium that does have an effect on learning outcomes, but rather the appropriate way of implementing it into the classroom as well as certain features that technology enhanced learning environments can offer.” Therefore, the focus is on digital media that are used for individualized practice. To specify these, the term DLP is used comprehensively. As examples for DLP Anton or Bettermarks can be mentioned (Holmes et al., 2018; Schaumburg, 2021). Here, DLP contain the following characteristics: First, DLP include practice exercises that students can work on (Greller et al., 2014; Daniela and Rūdolfa, 2019). During the assignment, DLP analyze the students’ input and provide them with direct, formative feedback (Daniela and Rūdolfa, 2019; Hillmayr et al., 2020). At the same time, student results (as an example for learning data) are stored and displayed on a teacher-dashboard within the DLP (Greller and Drachsler, 2012; Greller et al., 2014). Furthermore, DLP create interaction with students and teachers as well as between them (Faustmann et al., 2019; Hillmayr et al., 2020). In the best case, DLP include the possibility of adaptive adjustments by the system itself or the teacher (Daniela and Rūdolfa, 2019). In particular, by using learning data provided by DLP, teachers have the opportunity to provide individualized instruction to their students. The usage of learning data for instructional design—with both digital and analog data—has already been addressed in several research fields, which are presented in the following chapter.
Under the term of DBDM—which refers to “the systematic collection and analysis of different kinds of data to inform educational decisions” (Mandinach and Schildkamp, 2020, p. 1)—learning data in teaching and learning processes became a major focus of research. Among others, DBDM can help teachers determine instructional steps that meet learners’ diverse needs (Mandinach and Gummer, 2016; Prenger and Schildkamp, 2018; Peters et al., 2021). Because DBDM focuses on every child, not just children with identified special educational needs, it is consistent with the idea of an inclusive school environment (Mandinach and Gummer, 2013; Knickenberg et al., 2020). DBDM assumes that teachers collect a variety of data (i.e., quantitative, qualitative; analog, digital) in their daily practice. However, as a large amount of data is available, especially in the age of digitalization, teachers should consider which data they want to use and for what purpose (Schildkamp, 2019). Since the usage of DLP should be embedded in a pedagogical concept, the usage of learning data and the resulting decision making evokes pedagogical actions (Molenaar and Knoop-van Campen, 2017; Kerres, 2018). Therefore, on the one hand, teachers need pedagogical knowledge, perceptions, and an openness to the fact that pedagogy changes with the integration of digital media and learning data. A positive attitude toward the usage of learning data is considered essential (Blumenthal et al., 2021). On the other hand, teachers need data literacy to analyze and appropriately interpret learning data and to set and implement learning goals (Schildkamp and Kuiper, 2010; Mandinach and Gummer, 2016; Molenaar and Knoop-van Campen, 2017; Krein and Schiefner-Rohs, 2021).
In recent years, the research field of Learning Analytics evolved. This can be seen as a further development of research on DBDM. Here, the usage of learning data is considered only in a digital context. Learning Analytics help teachers and learners to individualize learning processes based on digital learning data (Krein and Schiefner-Rohs, 2021). The idea of Learning Analytics grew due to the large amount of learning data collected with the help of digital technologies (Greller et al., 2014). Learning Analytics deal with digitally generated data that is analyzed and presented in real time (Ifenthaler and Drachsler, 2020). Learning Analytics and DBDM pursue the same goal: Both concepts aim to support teachers in making pedagogical decisions based on learning data and not only on experience and intuition, for example, to enable individualized learning processes (Schildkamp and Kuiper, 2010; Greller et al., 2014). Digital learning data, as collected by DLP, include, for example, how long students practiced with the DLP, how many tasks they worked on, and whether they solved the tasks correctly or incorrectly.
Despite the potential of learning data for instructional design, it is, yet, not being used to a great extent for decision making among teachers (Schildkamp and Kuiper, 2010; Kippers et al., 2018). Especially in Germany, few teachers and schools have practiced DBDM to date. However, in other countries such as the United States and the Netherlands, DBDM is already being implemented more frequently (Blumenthal et al., 2021; Schaumburg, 2021). Studies of DBDM have found positive effects for students and teachers in primary schools. For example, Keuning et al. (2019) showed that teachers’ data usage had a positive impact on student achievement in mathematics and spelling. Anderson et al. (2020) found that progress monitoring—which is also a DBDM concept to identify learning problems—can help students acquire reading skills and help teachers to address student heterogeneity. Further, Souvignier et al. (2021) reported that student achievement in reading and mathematics improved after a progress monitoring intervention. Peters et al. (2021) identified the potential of DBDM for teachers dealing with particularly low-performing students. Molenaar and Knoop-van Campen (2018) observed teachers’ usage of learning data from DLP and found that Dutch teachers referred to the learning data multiple times during their instruction and that the data influenced their pedagogical actions. Although some studies have addressed DBDM and Learning Analytics in school contexts, there is still a need for further research. The usage of learning data retrieved especially from DLP and the intention to use the learning data for individualized instruction has not been covered empirically. Therefore, a need for research is indicated (Molenaar and Knoop-van Campen, 2017; Blumenthal et al., 2021; Schaumburg, 2021).
In order to better understand why primary school teachers use data from DLP (or not), further studies are needed. To gain insights into the factors which are associated to teachers’ intention to use and their usage of learning data from DLP, we refer to the TPB (Figure 1; Ajzen, 1991) as a theoretical framework. Also, teacher-specific factors are considered additionally. Since this research focuses on the usage of learning data from DLP and not on the usage of the DLP as a technology, the TPB is preferred over models that address acceptance of technology, such as UTAUT (Venkatesh et al., 2003).
Figure 1. The Theory of Planned Behavior [oriented to Ajzen (1991, p. 182)].
The TPB was developed as an extension of the Theory of Reasoned Action (Fishbein and Ajzen, 1975) and aims to explain and predict human behavior in various situations (Ajzen, 1991). Fundamental to the explanation of behavior is that actual behavior is predicted by intended behavior. A high behavioral intention increases the probability for actual behavior. In some instances, there is a gap between intention and behavior. Accordingly, though a person might have a specific intention, the behavior is not always performed (Sheeran, 2002). The TPB attempts to explain this intention-behavior-gap by considering the factors that influence intention as well as by examining the relationship between the intention and the actual behavior (Sheeran, 2002). According to the TPB, an intention is predicted by attitudes toward the behavior, subjective norms, and perceived behavioral control (Ajzen, 1991). Attitude refers to a person’s positive or negative feelings toward a behavior. The subjective norm includes the expectations a person has about the reactions of others. Finally, perceived behavioral control contains the estimation of the person’s skills, competencies, and resources to perform the behavior (Nistor, 2020).
In educational research, TPB has proven to be a useful instrument to explain teachers’ intentions and actual behavior. TPB has been used in (primary) teaching studies on inclusive education and individualized student support (Hellmich et al., 2019; Knauder and Koschmieder, 2019), DBDM (Pierce et al., 2013; Prenger and Schildkamp, 2018), and technology acceptance (Teo and Tan, 2012). In a cross-sectional survey study on N = 290 German primary school teachers, Hellmich et al. (2019) identified the school principal’s expectations as indicators of subjective norms as the largest factor influencing teachers’ intentions to implement inclusive education. Positive attitudes toward inclusion and teachers’ self-efficacy beliefs about organizing inclusive education were also, but were found to be less important. Knauder and Koschmieder (2019) applied the TPB to investigate teachers’ intrinsic and extrinsic motivational intentions to support students individually as well as to predict teachers’ individualized support and lesson design in a cross-sectional survey study involving N = 488 Austrian primary school teachers. For the intrinsic motivational intention, they found a strong association between attitude and individualized support, but no significant influence of subjective norms and perceived behavioral control (Knauder and Koschmieder, 2019). This was different for extrinsic motivational intention. Here, school as a factor of subjective norms had a significant influence on the intention to support students, whereas attitude and perceived behavioral control did not predict their extrinsic motivational intention to support students individually (Knauder and Koschmieder, 2019). For the context of DBDM, the TPB model was also able to explain teachers’ intention to use data from different sources to inform their teaching. Also, in a cross-sectional survey study of approximately 1,000 Australian teachers, Pierce et al. (2013) used the TPB model to gain insights into teachers’ perceptions of factors influencing their intention to use data from national testing in their lesson planning, confirming the usefulness of the TPB model in explaining teachers’ intention to use data. Prenger and Schildkamp (2018) tested an extended version of the TPB model (affective and instrumental attitude, subjective norms, perceived behavioral control, self-efficacy, collective efficacy) to explain teachers’ intentions as well as their instructional data usage related to curriculum assessments. They conducted a cross-sectional survey study with N = 131 primary school teachers in the Netherlands. Perceived behavioral control predicted instructional data usage, whereas, intention to use data was significantly predicted only by affective attitude and instrumental attitude (Prenger and Schildkamp, 2018). Teo and Tan (2012) reported that TPB is a useful instrument for explaining technology acceptance in educational contexts. In a cross-sectional survey study of N = 293 Singapore pre-service teachers, attitude toward technology were found to have the greatest influence on intention to use technology. Perceived behavioral control and subjective norms were also identified but were found to be less important predictors (Teo and Tan, 2012). The abovementioned studies indicate the relevance of the TPB model in educational research. To date, however, no study has used TPB to examine teachers’ usage of learning data received from digital media such as DLP in the context of individualization.
Since “teaching is an activity where teachers enact their conceptions about teaching and learning” (Yan et al., 2021, p. 229), it might be useful to consider other factors besides the TPB Model in order to gain more insight. The TPB model includes only a few personal factors and these factors are particularly related to the investigated behavior. However, a systematic review demonstrated the relevance of other factors, such as teaching beliefs, to the implementation of formative assessment (Yan et al., 2021). Accordingly, it can be assumed that the didactic context in which learning data from DLP is used should be considered. Learning data from DLP is related to the usage of DLP, is embedded in practice, contains feedback, is used for individualization, and is to be regarded overall in the context of data-based instructional design. Other studies that have examined the usage of media didactics in the classroom in general hypothesized that didactical concepts have an influence on the intention to use digital media in schools (Tappe, 2017; Gellerstedt et al., 2018). This assumption is also adopted in the present study and will be tested to predict teachers’ intention to use learning data obtained from DLP.
The theoretical overview above illustrated that digital media, especially DLP, are suitable to address the challenges of heterogeneous groups of students and to support and encourage learners individually. Learning data from DLP are of great importance in this context. Research on DBDM and Learning Analytics highlights the utility of learning data for instructional design. However, the reasons why teachers intend to use, use or do not use learning data from DLP have not yet been investigated. Therefore, this study—with reference to the TPB—seeks to answer the following research questions:
1. To what extent do primary school teachers in Germany use learning data from DLP for individualization?
2. What predicts teachers’ intentions to use and the usage of learning data from DLP?
To gain insights into German primary school teachers’ technology and data acceptance, we conducted an online cross-sectional survey study using LimeSurvey from October to December 2021. The study was developed from a psychological and educational perspective as part of the Competencies for Digitally-Enhanced Individualized Practice (CODIP) project. In this article, we focus on clarifying teachers’ intentions to use and their usage of learning data from DLP. An examination of the acceptance of DLP itself will be provided in another publication. Primary school teachers from the northern German federal states Bremen, Hamburg, Mecklenburg-Western Pomerania, Lower Saxony, and Schleswig Holstein were recruited by sending emails to their schools. In addition, teachers were reached via social media, and we also commissioned a market research panel to recruit teachers. Participation in the online survey took an average of 20 min.
The study was preregistered at Open Science Framework before we accessed the research data.1 Additionally, because the study involved human subjects, it was reviewed and approved by the Ethics Committee of the Leuphana University Lüneburg. Furthermore, the study was approved by the respective education offices of each involved federal state.
To find participants, 2,684 schools in northern Germany were contacted. The resulting total sample of the study consisted of N = 272 primary school teachers who were predominantly female (86%). This distribution corresponds to the findings obtained by the survey of the Federal Statistical Office for the school year 2020/21 (Federal Statistical Office of Germany, 2022). Most participants were between 40–49 and 50–59 years old (each 28%), 19% were between 30–39 years, 13% were older than 60 years, and 12% were younger than 30 years. The distribution is roughly in line with the information from the Federal Statistical Office, in which the 40–49-year age group is the largest, with the 30–39-year age group second, followed by the 50–59-year age group (Federal Statistical Office of Germany, 2022). Work experience was also captured by time ranges. Most of the teachers had 11–20 years of work experience (28%), followed by 26% with 21–30 years of work experience, while 20% of the teachers were career starters with up to 5 years of work experience. The remaining teachers had 6–10 years (13%), 31–40 years (11%), and more than 40 years (2%) of work experience.
The online survey started with an introductory video. This self-created video included a definition of DLP and its resulting learning data. The definitions were intended to ensure that all participants had the same understanding of DLP and learning data from DLP. Similarly, teachers who do not have experience with learning data from DLP can answer the questionnaire based on the video. The questions were divided into four sections: (1) DLP, (2) learning data from DLP, (3) digital media, and (4) didactical concepts. The sections relevant for this article Sections “Purpose and research questions,” Materials and methods,” and “Results” will be described in detail below. All scales used in the survey were adapted from existing measures or were based on theoretical assumptions. The item wordings were altered to the context of usage of data from DLP. Table 1 summarizes all characteristics of the scales used within this research context.
Following TPB (Ajzen, 1991) as a theoretical framework to explain teachers’ intentions to use learning data from DLP, we used scales to assess teachers’ self-reported data usage, their intentions to use learning data from DLP, their attitude toward learning data from DLP, their perceived behavioral control regarding learning data from DLP and their subjective norm regarding learning data from DLP.
To assess data usage in the context of individualization we developed items on purpose-related usages like using data from DLP for identifying student needs, setting learning goals, or revising lessons based on individual needs. Because to date no study had examined teachers’ usage of learning data from DLP, we adapted items on general usage of learning data to this context. This also applies to the following scales concerning learning data from DLP.
Within this questionnaire, intention to use data from DLP was measured using three different stages. Thus, the items addressing the intention to use included thinking about a behavior, planning the behavior, as well as the determination of the intention to actually perform the behavior. Still, if teachers answered that they have not used learning data from DLP yet, they got an additional information before answering the items regarding the intention to use learning data from DLP. We asked teachers to answer the next items to the best of their ability, and to think about potential use if necessary.
Based on theoretical background, attitude, perceived behavioral control, and subjective norm should predict teachers’ intention to use learning data from DLP and their usage. Regarding attitudes toward learning data from DLP, items were devoted to the benefits of data usage for the teacher, as well as items focused on improvements for students. The items on perceived behavioral control regarding learning data from DLP captured how teachers assessed their own ability to use learning data from DLP. Here, using learning data was again focused on aspects of individualization and additionally on aspects of instructional design. Within the items on subjective norm regarding learning data from DLP, teachers were asked to rate how much they think other professionally relevant groups of people (students, parents, colleagues, school administrators) expect them to use learning data from DLP. We also provided an option to give no answer as the items of these scales were mandatory to be able to proceed the questionnaire.
In addition to the TPB model, research data on other factors were collected to elucidate the intention to use learning data from DLP. For this purpose, we asked teachers about the digital media they use. Teachers had to indicate for ten different digital media whether they use them as part of their teaching. The focus was less on technical devices and more on applications such as learning management systems or learning videos. A sum score was calculated across the ten items to indicate teachers’ proneness to usage of digital media for instruction. Additionally, the questionnaire contained items regarding teachers’ attitudes toward digital media. These items also contained positive attitudinal statements regarding benefits to teachers and students, but with focus on digital media in general.
When using DLP and the resulting data, the pedagogical context requires closer consideration. Therefore, we included items on didactical concepts in our survey instrument. Related to our research aim to better understand teachers’ intention to use learning data from DLP we integrated items regarding practice, individual support, feedback, and data-based instructional design within our study. The didactic concepts were not related to digitalization in order to find out how important these concepts were for teachers in their lessons independently from the usage of data from DLP.
The scale Practice consisted of items assessing automated and elaborated practice as those are different ways to practice. Since individual instructional design can benefit from the usage of learning data from DLP, items on Individual support were used to its importance for teachers’ instruction. The scale Feedback inquired the extent to which teachers provide feedback to their students in the classroom. With the scale Data-driven instructional design, we determined whether teachers also use learning data without a digital medium to design their lessons and to encourage and challenge learners. A mean value was calculated for each scale.
Non-response occurred when participants did not answer all items of a scale or used the alternative response option, I cannot tell. To cope with missing data, we used multiple imputation (Van Buuren, 2012), using the mice package in R-Studio. The multiple imputation was based on a created predictor matrix with 10 iterations.
To answer the first research question, the research data on usage of learning data from DLP were analyzed descriptively. Descriptive statistics were compiled for all scales in preparation for explaining the intention to use and the usage of learning data from DLP.
To answer the second research question, we used structural equation modeling (SEM). The SEM method was chosen as it enables to consider all variables in one model at once, acting as both independent and dependent variables. SEM combines factor and path analysis in order to separate measurement error influences from true influences. In addition, SEM can be used to check the fit of a model with the data set (Schumacker and Lomax, 2010; Eid et al., 2017).
We first examined the TPB model as our Model 1. Since this was applied to a novel context, we checked all connections of the variables (i.e., also attitude and subjective norm for usage and not only for intention to use). In another SEM, Model 2, we tested the extended TPB model that included additional predictors. Following Tappe (2017) and Gellerstedt et al. (2018), the additional predictors are tested only in relation to the intention to use learning data from DLP and not in relation to the self-reported usage. We specified that the difference in the explained variance between both models (ΔR2) must be ≥0.05 for the extended model to provide a meaningful improvement compared to Model 1. For the purpose of this article, we used model fit values according to the following guidelines: As a criterion for the acceptance of the overall model, we assumed that χ2/df should be ≤3.00 (Homburg and Giering, 1997). For the comparative fit index (CFI), values ≥0.90 indicate a good fit (Garson, 2009). Additionally, the root mean square error of approximation (RMSEA) should be ≤0.05 to be accepted as a good model fit or ≤0.08 for an acceptable model fit. The associated p-value of RMSEA must be ≥0.05 (Browne and Cudeck, 1993). All analyses were conducted using R-Studio.
It was part of the survey to ask teachers about their previous and current usage of learning data from DLP for five different purposes of individualization (Figure 2). The self-reported usage is utilized as a dependent variable in the main analyses, but to answer research question 1 it is also examined descriptively.
Figure 2 shows that the teachers were divided into data users and non-users of roughly equal size across all five purposes. Up to 50% of the teachers had never used learning data from DLP for either of the specific purposes targeting individualization. The majority of the teachers (58.5%) had used learning data from DLP to tailor instruction to individual students’ needs. Of these, 48.9% claimed to currently use the learning data occasionally or regularly. Further, 46.7% of teachers reported that they currently used learning data from DLP to identify the learning needs of struggling students occasionally or regularly. Another 10.7% of participants had used the learning data at an earlier time. The third most common reason for using learning data from DLP was to set learning goals for individual students. Here, 11.0% of teachers had used learning data in the past and 43.0% currently did so. A total of 51.9% of teachers had previously used (10.3%) or currently use (41.6%) learning data from DLP to determine whether they needed to re-teach certain concepts and skills. Teachers were least likely to use learning data from DLP to identify the learning needs of students who were not struggling. Here, exactly 50.0% of the participants used learning data for this reason and correspondingly, the same number of participants did not. As with the previous purpose, 10.3% of teachers had used the learning data at an earlier time. The remaining teachers (39.7%) currently used the learning data at the time of the survey. The results showed that learning data from DLP were used by teachers to similar extents for different purposes.
In preparation for the main analyses, we analyzed the descriptive statistics and reliability of all scales (Table 2). Since the reliability analyses yielded acceptable to excellent values for all scales, this issue is not considered further.
Results of the descriptive statistics showed that the mean score for intention to use learning data from DLP was M = 2.75. That is, on average, participants were slightly more likely to imagine using learning data from DLP within the school year to design their lessons than to imagine not using them. A closer look at the evaluation of the items for the intention showed that 78% of teachers had an intention to use learning data from DLP. Thereby, the intention to use learning data differed among these teachers regarding its intensity. The remaining 22% of teachers had no intention to use learning data from DLP. An example item to assess the intention to use was “I plan to use learning data from digital learning platforms for my teaching within the current school term.” Even though the items on the self-reported usage of learning data from DLP have already been considered in more detail (Section “To what extent do German primary school teachers use learning data from digital learning platforms for individualization?”), the mean value should also be mentioned here (M = 2.07). An example item for the usage scale was “I use data from digital learning platforms to set learning goals for individual students.”
On average, the participants reported more positive than negative attitudes toward learning data from DLP (M = 2.75). This implies, for example, that teachers agreed that they find learning data from DLP useful. The mean for all participants regarding the scale perceived behavioral control regarding learning data from DLP was about moderate (M = 2.53). Thus, we could not make a clear determination about whether or not teachers might be able to deal with learning data from DLP. While some teachers stated that they are already able to use learning data from DLP other stated they cannot. For the subjective norm regarding learning data from DLP scale, the average answer showed a tendency toward negative response options (M = 2.22). This suggests that teachers were not particularly influenced by, for example, their colleagues to use learning data from DLP.
With regard to the usage of digital media, the teachers were asked about the use of ten different types of digital media in their lessons. We then calculated the sum score with a mean of M = 5.19. This showed that the teachers on average used about half of the digital media given. Teachers, on average, highly approved items regarding the attitude toward digital media (M = 3.03). That is, teachers, on average, rather liked working with digital media in the classroom.
For most teachers, the didactical concepts considered, i.e., practice, individual support, feedback, and data-based instructional design certainly mattered in their teaching. Practicing was considered relevant by teachers on average (M = 3.21). For example, practice could be represented by the following item “I let my students practice with tasks where I can see particularly well whether the essentials have been understood.” Participants also perceived individual support to be relevant to their teaching with M = 3.35. For example, teachers occasionally to regularly gave their students tasks that fit their needs. Giving feedback appeared to be the most relevant didactic concept for teachers, as indicated by the mean of M = 3.51. On average, teachers occasionally to regularly told their students in which areas they could improve. For data-based instructional design, M = 3.21 showed the same mean value as for practicing. Accordingly, teachers tended to use learning data occasionally; for example, as a basis for conversations with parents.
To finally answer the second research question, we predicted teachers’ intention to use learning data from DLP as well as their self-reported usage of learning data from DLP by firstly their attitude, perceived behavioral control, subjective norm and secondly as well by their usage of digital media, attitude toward digital media, practice, individual support, feedback, and data-based instructional design. The correlation matrix of all predictors with the intention to use and the usage of learning data from DLP is shown in Table 3. Cohen (1988) was followed in interpreting the correlation coefficients. Low to moderate significant correlations with intention to use learning data from DLP were found for all independent variables except for practice. Attitude, perceived behavioral control, usage of digital media, and attitude toward digital media showed moderate correlations with teachers’ intentions to use learning data from DLP. A high positive correlation was found for intention to use learning data from DLP with usage of learning data from DLP. Additionally, the correlation matrix showed low to high correlations with usage of learning data from DLP and the other variables except for practice. Here, perceived behavioral control showed a high correlation with teachers’ intentions to use learning data from DLP whereas moderate correlations were found for attitude, subjective norm, usage of digital media, and attitude toward digital media.
To examine the association of all variables in one model, we performed SEM. First, we considered the TPB model in its original form, but considered attitude and subjective norm as predictors for teachers’ usage of data from DLP as well (Figure 3). Secondly, we extended the TPB model with additional variables: usage of digital media, attitude toward digital media, and didactical concepts (Figure 4). The influence of the additional variables was only tested regarding the intention to use data from DLP. Table 4 provides the standardized beta values of all relationships for both models.
Figure 3. Results of the structural equation modeling (SEM) for the theory of planned behavior (TPB) model in case of data from digital learning platforms (DLP). N = 272; *p < 0.05, **p < 0.01, and ***p < 0.001.
Figure 4. Results of the structural equation modeling (SEM) for the extended theory of planned behavior (TPB) model in case of data from digital learning platforms (DLP). N = 272; *p < 0.05, **p < 0.01, and ***p < 0.001.
Based on the confirmatory factor analysis, a good model fit could be established for Model 1: χ2(242) = 385.65, χ2/df = 1.60, p ≤ 0.001. With a CFI = 0.95, the value represented a good model fit. The RMSEA = 0.05, with p = 0.73, and 90% CI [0.04, 0.06], could also be classified as good. Taking a closer look at the results of the first SEM, the intention to use data from DLP was mostly significantly predicted by teachers’ attitudes toward learning data from DLP. Thus, teachers with a positive attitude toward the usage of learning data from DLP showed a higher intention to use it. Additionally, the perceived behavioral control regarding learning data from DLP also significantly predicted the intention to use learning data from DLP. Similarly, teachers who assessed their skills in using learning data from DLP as good showed a higher usage intention. In contrast, no significant associations were found for subjective norm regarding learning data from DLP. Regarding the usage of data from DLP, teachers’ intentions to use learning data from DLP as well as their perceived behavioral control regarding learning data from DLP and subjective norm regarding learning data from DLP turned out as significant predictors. In this context, perceived behavioral control was most significant in explaining the model. Thus, we found that teachers use learning data from DLP when they perceive themselves as competent enough to do so or when other persons like colleagues influenced teachers’ interest in using such learning data. With Model 1 we were able to explain 30% of the variance of the intention to use data from DLP and 51% of the variance of the usage of learning data from DLP.
Also for Model 2 a good model fit was established: χ2(1277) = 1971.79, p ≤ 0.001, χ2/df = 1.60. Even though the CFI of 0.90 was a bit lower here, it could still be described as good. The RMSEA = 0.05, with p = 0.99, and 90% CI [0.04, 0.05], could also be classified as good. Model 2 explained 38% of the variance of the intention to use data from DLP and 52% of the variance of the usage of learning data from DLP. As the additional predictors were tested only in relation to the intention to use learning data from DLP, only the consideration of ΔR2 for intention was interesting. Following our default that an increase in explained variance becomes practically relevant only when ΔR2 ≥ 0.05, a ΔR2 = 0.08 shows that the second model differed meaningfully from the first model. Therefore, Model 2 should be considered. In addition to the TPB variables, this model contained digital media (usage, attitude) and didactical concepts (practice, individual support, feedback, data-based instructional design). Again, attitude toward learning data from DLP most strongly predicted the intention to use learning data from DLP. In contrast to Model 1, perceived behavioral control regarding learning data from DLP did not predict teachers’ intentions to use learning data from DLP. Of the added factors, usage of digital media was found to be a significant predictor of teachers’ intentions to use learning data from DLP. Thus, in addition to teachers’ attitudes, the usage of several types of digital media was predictive for their intention to use learning data from DLP. Other didactical concepts showed no significant association with the intention to use data from DLP. The previously identified predictors for the usage of data from DLP remained the same: intention to use data from DLP, perceived behavioral control regarding data from DLP, and subjective norm regarding data from DLP.
The presented study provides a valuable insight into German primary school teachers’ intention to use and usage of data from DLP. In this cross-sectional survey study, on the one hand, we were able to describe the usage of learning data from DLP for purposes of individualization. On the other hand, we predicted teachers’ intention to use learning data from DLP as well as their usage with variables from the established TPB model (Model 1) as well as an extended TPB model (Model 2).
Regarding the first research question, about half of all participants indicated that they were already using learning data from DLP for various purposes of individualization. For example, identifying struggling students’ learning needs led to great consent among the teachers. This emphasizes the added value of DBDM in the school context: Theoretical articles and empirical studies have cited the determination of appropriate instructional steps for students’ individual learning needs as a reason for data usage (Mandinach and Gummer, 2016; Prenger and Schildkamp, 2018; Peters et al., 2021). Similarly, research on educational technologies has reported on the potential of DLP to provide information about students’ needs from learning data (Greller et al., 2014; Schaumburg, 2021). Nevertheless, half of all teachers who participated in the survey did not use learning data from DLP. A non-use of learning data was also found in other studies (Kippers et al., 2018; Blumenthal et al., 2021). For this reason, it was important to further investigate the reasons for the intention to use and usage of learning data from DLP.
With regard to the second research question on predictors of intention to use and usage of learning data from DLP, teachers’ attitudes toward learning data from DLP proved to be the most relevant predictor for intention to use learning data from DLP in both models. Therefore, teachers need a positive mindset about learning data from DLP in order to consider using them. Teo and Tan (2012) also found the highest influence of attitude as a factor of the TPB model when they predicted teachers’ intentions to use technology in school. Likewise, Blumenthal et al. (2021) identified attitude toward data—independently of TPB—as an important predictor for the intention to use data for educational decisions. In contrast to previous studies, however, subjective norm had no effect on the intention to use learning data from DLP (Teo and Tan, 2012; Hellmich et al., 2019; Knauder and Koschmieder, 2019). This might be explained by the fact that teachers in Germany are quite independent in their lesson planning and often do not receive regulations regarding the choice of their methods (Kerres, 2020). The irrelevance of subjective norm changed when considering the TPB variables in terms of the usage of learning data from DLP. Here, subjective norm regarding learning data from DLP significantly predicted teachers’ usage of learning data from DLP. In return, the attitude toward learning data from DLP had no influence on the explanation of the usage of learning data from DLP. The relevance of perceived behavioral control regarding learning data from DLP and the irrelevance of attitude toward learning data from DLP to the usage of learning data from DLP is consistent with the findings of Knauder and Koschmieder (2019) on the consideration of TPB with respect to individualized instructional design but is also in contrast to the findings of other studies (Prenger and Schildkamp, 2018; Hellmich et al., 2019). As expected, intention to use learning data from DLP had a significant influence on the usage of learning data from DLP. Nevertheless, an intention-behavior gap is evident here as well (Ajzen, 1991; Sheeran, 2002): More teachers have the intention to use, but fewer actually realize the usage of learning data from DLP. This may be due to the fact that it takes more than just a positive attitude to use it. It also requires competencies—expressed here in perceived behavioral control—that must first be acquired. The addition of further variables led to a meaningful improvement of the model, but only the previous usage of digital media could be identified as a significant predictor of the intention to use learning data from DLP. Therefore, it is helpful for teachers to be able to imagine the usage of learning data from DLP if they have already gained experience with other digital media. From this we can assume that there would also be a significant association between the intention to use or the usage of the DLP and the intention to use learning data from DLP. The extension of a predictive model to include didactical concepts, like the importance of feedback or the usage of data-based instructional design, as proposed by Tappe (2017) and Gellerstedt et al. (2018), yielded no success in this study. We conclude that even though the TPB model proved to be very robust and the influence of additional predictors was small, it seems useful to consider the teacher’s instructional context when explaining teachers’ intentions to use learning data from DLP in future studies.
To the best of our knowledge, this is the first study to examine primary teachers’ intention to use and usage of learning data from DLP in the context of individualization in Germany. In this context, an already established theoretical model proved useful in the cross-sectional survey study and was tested with additional factors. Nevertheless, there are some limitations to this study.
In this study, the TPB model was considered in terms of both teachers’ intentions and usage of learning data from DLP. However, it is worth noting that teacher respondents were only surveyed at a single point in time, as it is desirable to observe the intentional and behavioral change over a certain time between the first and the second measurement. Accordingly, the results should be confirmed in a longitudinal survey. Nevertheless, for comparability with other studies of the TPB, both intention and usage were included in our analyses. Moreover, the usage—as well as the other items—was only self-reported by the teachers, thus there is a possibility of distortion. The real usage of learning data from DLP, how it is designed, and if it is beneficial for learning of students is left unanswered and should be subject of further research.
Further, although all primary school teachers in Bremen, Hamburg, Mecklenburg-Western Pomerania, Lower Saxony, and Schleswig Holstein were contacted via their schools and invitations were issued on social media to participate in the survey, only a small number of primary school teachers took part in the survey study. Nevertheless, this number met the previously calculated sample size and analyses were conducted. In addition, it can be assumed that the sample is characterized by media-literate teachers, as the respondents were recruited via e-mail and social media and the questionnaire was conducted online. The frequency of usage might be overestimated.
This study was able to explain primary school teachers’ intention to use and the usage of learning data from DLP especially for individualization. Doing so, this study contributes to the growing body of research on the potentials of DBDM and Learning Analytics in the context of inclusive schooling. Nevertheless, further empirical research is needed based on these findings. We have already been able to explain part of the intention to use and the usage of data from DLP, however, some reasons for the (non) use still remain unexplained. These need to be investigated in further studies. In this context, we also recommend qualitative studies, for example interviews with primary school teachers, in order to elaborate further relevant factors. Since students in primary schools are particularly heterogeneous, we focused on primary school teachers. However, an investigation of the model would also be interesting for secondary school teachers. In addition to our findings regarding predictors of intention to use and usage, it would be valuable to better understand what motivates teachers to use or not use learning data from DLP. Moreover, it would be interesting to find out more about how teachers use learning data from DLP, especially in the context of individualization, and whether this use has an impact on learning effectiveness. To this end, qualitative studies like interviews, school-based observation studies, or additional quantitative studies are desirable. Furthermore, it would certainly be worthwhile to take a closer look at teachers’ competencies for using learning data from DLP and to investigate the influence on their intention to use as well as their usage.
The results of this study indicate that it is also necessary to consider its implications for teacher education and training. Consideration needs to be given to how teachers’ attitudes toward the usage of learning data from DLP, as well as their perceived behavioral control, can be fostered in teacher trainings to increase their usage of learning data from DLP. Because DBDM, especially in DLP contexts, can support teachers in establishing individualized learning opportunities, this can help to meet the needs of all students best.
The raw data supporting the conclusions of this article will be provided by the authors upon request.
The studies involving human participants were reviewed and approved by Ethics Committee of the Leuphana University Lüneburg. The participants provided their written informed consent to participate in this study.
AH had the main role in writing the original draft. LK, PK, and DL contributed in editing the manuscript. AH and LK mainly conducted the analyses. All authors contributed to funding acquisition and project administration and approved the submitted version.
This study was funded by the Open Access Publication Fund of the Leuphana University Lüneburg. This study developed within the CODIP project. CODIP is funded by the Quality Initiative Teacher Training (Qualitätsoffensive Lehrerbildung), a joint initiative of Federal Government and the German states. The financial means were provided by the Federal Ministry of Education and Research (BMBF) (Support code: 01JA2002).
We thank the teachers from our development team for their help in revising the questionnaire and our student assistants for their support in data evaluation.
The authors declare that the research was conducted in the absence of any commercial or financial relationships that could be construed as a potential conflict of interest.
All claims expressed in this article are solely those of the authors and do not necessarily represent those of their affiliated organizations, or those of the publisher, the editors and the reviewers. Any product that may be evaluated in this article, or claim that may be made by its manufacturer, is not guaranteed or endorsed by the publisher.
Ajzen, I. (1991). The theory of planned behavior. Organ. Behav. Hum. Decision Process. 50, 179–211. doi: 10.1016/0749-5978(91)90020-T
Anderson, S., Jungjohann, J., and Gebhardt, M. (2020). Effects of using curriculum-based measurement (CBM) for progress monitoring in reading and an additive reading instruction in second classes. Zeitschrift für Grundschulforschung 13, 151–166. doi: 10.1007/s42278-019-00072-5
Baumert, J., Blum, W., Brunner, M., Dubberke, T., Jordan, A., Klusmann, U., et al. (2009). Professionswissen von Lehrkräften, kognitiv aktivierender Mathematikunterricht und die Entwicklung von mathematischer Kompetenz (COACTIV): Dokumentation der Erhebungsinstrumente [Teachers’ professional knowledge, cognitively activating mathematics instruction, and the development of mathematical competence (COACTIV): documentation of survey instruments]. Berlin: Max-Planck-Institut für Bildungsforschung.
Blumenthal, S., Blumenthal, Y., Lembke, E. S., Powell, S. R., Schultze-Petzold, P., and Thomas, E. R. (2021). Educator Perspectives on Data-Based Decision Making in Germany and the United States. J. Learn.. Disabilities 54, 284–299. doi: 10.1177/0022219420986120
Böhme, R., Munser-Kiefer, M., and Prestridge, S. (2020). Lernunterstützung mit digitalen Medien in der Grundschule: Theorie und Empirie zur Wirkweise zentraler Funktionen und Gestaltungsmerkmale [Support Learning with Digital Media in Elementary School: Theory and Empirical Evidence on the Effectiveness of Key Functions and Design Features]. Zeitschrift für Grundschulforschung 13, 1–14. doi: 10.1007/s42278-019-00066-3
Browne, M. W., and Cudeck, R. (1993). “Alternative ways of assessing model fit,” in Testing Structural Equation Models, eds K. A. Bollen and J. S. Long (Newbury Park, CA: Sage Publications), 136–162.
Cohen, J. (1988). Statistical Power Analysis for the Behavioral Sciences. Hillsdale, NJ: L. Erlbaum Associates.
Daniela, L., and Rūdolfa, A. (2019). “Learning platforms: how to make the right choice,” in Didactics of Smart Pedagogy: Smart Pedagogy for Technology Enhanced Learning, ed. L. Daniela (Cham: Springer), 191–209. doi: 10.1007/978-3-030-01551-0
Eid, M., Gollwitzer, M., and Schmitt, M. (2017). Statistik und Forschungsmethoden [Statistics and research methods]. Weinheim, Basel: Beltz.
Faustmann, G., Lemke, C., Kirchner, K., and Monett, D. (2019). “Which factors make digital learning platforms successful,” in Proceedings of the 13th Annual International Technology, Education and Development Conference, Velancia, 6777–6786. doi: 10.21125/inted.2019.1651
Federal Statistical Office of Germany (2022). Allgemeinbildende Schulen: Fachserie 11 Reihe 1 – Schuljahr 2020/2021 [General education schools: Subject-matter series 11 series 1 – School year 2020/2021]. Available online at: https://www.destatis.de/DE/Themen/Gesellschaft-Umwelt/Bildung-Forschung-Kultur/Schulen/Publikationen/_publikationen-innen-schulen-allgemeinbildende.html (Accessed on March 28, 2022).
Fishbein, M., and Ajzen, I. (1975). Belief, attitude, Intention and Behaviour: An Introduction to Theory and Research. Reading, MA: Addison-Wesley.
FitzGerald, E., Jones, A., Kucirkova, N., and Scanlon, E. (2018). A literature synthesis of personalised technology-enhanced learning: what works and why. Res. Learn. Technol. 26, 1–16. doi: 10.25304/rlt.v26.2095
Garson, G. D. (2009). Structural Equation Modeling. Asheboro, NC: Statistical Associates Publishers.
Gellerstedt, M., Babaheidari, S. M., and Svensson, L. (2018). A first step towards a model for teachers’ adoption of ICT pedagogy in schools. Heliyon 4, 1–17. doi: 10.1016/j.heliyon.2018.e00786
Greller, W., and Drachsler, H. (2012). Translating learning into numbers: A generic framework for learning analytics. Educ. Tech. Soc. 15, 42–57.
Greller, W., Ebner, M., and Schön, M. (2014). Learning analytics: From theory to practice – data support for learning and teaching. Communicat. Comp. Inform. Sci. 439, 79–87. doi: 10.1007/978-3-319-08657-6_8
Hellmich, F., Löper, M. F., and Görel, G. (2019). The role of primary school teachers’ attitudes and self-efficacy beliefs for everyday practices in inclusive classrooms: A study on the verification of the ‘Theory of Planned Behaviour’. J. Res. Special Educ. Needs 19, 36–48. doi: 10.1111/1471-3802.12476
Hillmayr, D., Ziernwald, L., Reinhold, F., Hofer, S. I., and Reiss, K. M. (2020). The potential of digital tools to enhance mathematics and science learning in secondary schools: A context-specific meta-analysis. Comput. Educ. 153, 1–25. doi: 10.1016/j.compedu.2020.103897
Holmes, W., Anastopoulou, S., Schaumburg, H., and Mavrikis, M. (2018). Technology-enhanced Personalised Learning. Untangling the Evidence. Stuttgart: Robert Bosch Stiftung.
Homburg, C., and Giering, A. (1997). Konzeptualisierung und Operationalisierung komplexer Konstrukte: Ein Leitfaden für die Marketingforschung [Conceptualization and operationalization of complex constructs – A guideline for marketing research]. Marketing 18, 5–24.
Ifenthaler, D., and Drachsler, H. (2020). “Learning Analytics: Spezielle Forschungsmethoden in der Bildungstechnologie [Learning analytics: special research methods in educational technology],” in Handbuch Bildungstechnologie [Educational Technology Handbook], eds H. Niegemann and A. Weinberger (Berlin: Springer), 515–534. doi: 10.1007/978-3-662-54368-9_42
Institute for Quality Development Hessen (2012). Hessischer Referenzrahmen Schulqualität: Dokumentation der Fragebogen [Hessian Reference Framework for School Quality: Documentation of the questionnaires]. Wiesbaden: Institute for Quality Development (IQ) Hessen.
Jäger, R. S., and Helmke, A. (2008). Mathematik-Gesamterhebung Rheinland-Pfalz: Kompetenzen, Unterrichtsmerkmale, Schulkontext (MARKUS) (Version 1) [Mathematics Survey Rhineland-Palatinate: Competencies, Instructional Characteristics, School Context (MARKUS) (Version 1)]. Berlin: IQB – Institute for Educational Quality Improvement, https://doi.org/10.5159/IQB_MARKUS_v1
Kerres, M. (2018). Mediendidaktik. Konzeption und Entwicklung digitaler Lernangebote [Media didactics. Conception and development of digital learning offers]. Berlin: De Gruyter, doi: 10.1515/9783110456837
Kerres, M. (2020). Against All Odds: Education in Germany Coping with Covid-19. Postdigital Sci. Educ. 2, 690–694. doi: 10.1007/s42438-020-00130-7
Keuning, T., van Geel, M., Visscher, A., and Fox, J.-P. (2019). Assessing and validating effects of a data-based decision-making intervention on student growth for mathematics and spelling. J. Educ. Measure. 56, 757–792. doi: 10.1111/jedm.12236
Kippers, W. B., Wolterinck, C. H. D., Schildkamp, K., and Poortman, C. L. (2018). Teachers’ views on the use of assessment for learning and data-based decision making in classroom practice. Teach. Teach. Educ. 75, 199–213. doi: 10.1016/j.tate.2018.06.015
Knauder, H., and Koschmieder, C. (2019). Individualized student support in primary school teaching: A review of influencing factors using the Theory of Planned Behavior (TPB). Teach. Teach. Educ. 77, 66–76. doi: 10.1016/j.tate.2018.09.012
Knickenberg, M., Zurbriggen, C. L. A., Venetz, M., Schwab, S., and Gebhardt, M. (2020). Assessing dimensions of inclusion from students’ perspective: measurement invariance across students with learning disabilities in different educational settings. Eur. J. Special Needs Educ. 35, 287–302. doi: 10.1080/08856257.2019.1646958
Krein, U., and Schiefner-Rohs, M. (2021). Data in Schools: (Changing) Practices and Blind Spots at a Glance. Front. Educ. 6:672666. doi: 10.3389/feduc.2021.672666
Mandinach, E. B., and Gummer, E. S. (2013). A Systemic View of Implementing Data Literacy in Educator Preparation. Educ. Res. 42, 30–37. doi: 10.3102/0013189X12459803
Mandinach, E. B., and Gummer, E. S. (2016). What does it mean for teachers to be data literate: Laying out the skills, knowledge, and dispositions. Teach. Teach. Educ. 60, 366–376. doi: 10.1016/j.tate.2016.07.011
Mandinach, E. B., and Schildkamp, K. (2020). Misconceptions about data-based decision making in education: An exploration of the literature. Stud. Educ. Eval. 2020:100843. doi: 10.1016/j.stueduc.2020.100842
Molenaar, I., and Knoop-van Campen, C. A. N. (2017). Teacher Dashboards in Practice: Usage and Impact, in Data Driven Approaches in Digital Education: EC-TEL 2017. LNCS 10474. Basel: Springer Cham, 15–138. doi: 10.1007/978-3-319-66610-5_10
Molenaar, I., and Knoop-van Campen, C. A. N. (2018). How teachers make dashboard information actionable. IEEE Transac. Learn. Technol. 12, 347–355. doi: 10.1109/TLT.2018.2851585
Moore, R., and Shaw, T. (2017). Teachers‘ Use of Data: An Executive Summary. Available online at: https://www.act.org/content/dam/act/unsecured/documents/R1661-teachers-use-of-data-2017-12.pdf (accessed July 29, 2021).
Nattland, A., and Kerres, M. (2009). “Computerbasierte Medien im Unterricht [Computer-based media in the classroom],” in Handbuch Unterricht [Teaching Handbook], eds K.-H. Arnold, J. Wiechmann, and U. Sandfuchs (Bad Heilbrunn: Klinkhardt), 317–323.
Nistor, N. (2020). “Akzeptanz von Bildungstechnologien [Acceptance of Education Technology],” in Handbuch Bildungstechnologie [Educational Technology Handbook], eds H. Niegemann and A. Weinberger (Berlin: Springer), 535–545. doi: 10.1007/978-3-662-54368-9_46
Peters, M. T., Förster, N., Hebbecker, K., Forthmann, B., and Souvignier, E. (2021). Effects of data-based decision-making on low-performing readers in general education classrooms: cumulative evidence from six intervention studies. J. Learn. Disabilites 54, 334–348. doi: 10.1177/00222194211011580
Petko, D. (2014). Einführung in die Mediendidaktik: Lehren und Lernen mit digitalen Medien [Introduction to Media Didactics: Teaching and learning with digital media]. Weinheim: Beltz.
Petko, D., Prasse, D., and Cantieni, A. (2018). The interplay of school readiness and teacher readiness for educational technology integration: A structural equation model. Comput. Sch. 35, 1–18. doi: 10.1080/07380569.2018.1428007
Pierce, R., Chick, H., and Gordon, I. (2013). Teachers’ perceptions of the factors influencing their engagement with statistical reports on student achievement data. Aust. J. Educ. 57, 237–255. doi: 10.1177/0004944113496176
PISA (2017). Teacher Questionnaire for Pisa 2018: General Teacher. Main Survey Version. Available online at: https://www.oecd.org/pisa/data/2018database (Accessed on July 29, 2021).
Prenger, R., and Schildkamp, K. (2018). Data-based decision making for teacher and student learning: a psychological perspective on the role of the teacher. Educ. Psychol. 38, 734–752. doi: 10.1080/01443410.2018.1426834
Reinhold, F., Hoch, S., Werner, B., Richter-Gebert, J., and Reiss, K. (2020). Learning fractions with and without educational technology: What matters for high-achieving and low-achieving students? Learn. Instr. 65, 1–19. doi: 10.1016/j.learninstruc.2019.101264
Schaumburg, H. (2021). Personalisiertes Lernen mit digitalen Medien als Herausforderung für die Schulentwicklung: Ein systematischer Forschungsüberblick [Personalized Learning with Digital Media as a Challenge for School Development: A Systematic Research Review]. MedienPädagogik 41, 134–166. doi: 10.21240/mpaed/41/2021.02.24.X
Schaumburg, H., and Prasse, D. (2019). Medien und Schule: Theorie – Forschung – Praxis [Media and school: Theory – Research – Practice]. Bad Heilbrunn: Julius Klinkhardt.
Schildkamp, K. (2019). Data-based decision-making for school improvement: Research insights and gaps. Educ. Res. 61, 257–273. doi: 10.1080/00131881.2019.1625716
Schildkamp, K., and Kuiper, W. (2010). Data-informed curriculum reform: Which data, what purposes, and promoting and hindering factors. Teach. Teach. Educ. 26, 482–496. doi: 10.1016/j.tate.2009.06.007
Schmid, U., Goertz, L., and Behrens, J. (2017). Monitor Digitale Bildung: Die Schulen im digitalen Zeitalter [Digital Education Monitor: Schools in the digital age]. Gütersloh: Bertelsmann Stiftung, doi: 10.11586/2017041
Schumacker, R. E., and Lomax, R. G. (2010). A Beginner’s Guide to Structural Equation Modeling. New York, NY: Routledge.
Schwab, S., Hellmich, F., and Görel, G. (2017). Self-efficacy of prospective Austrian and German primary school teachers regarding the implementation of inclusive education. J. Res. Special Educ. Needs 17, 205–217. doi: 10.1111/1471-3802.12379
Sheeran, P. (2002). Intention—behavior relations: A conceptual and empirical review. Eur. Rev. Social Psychol. 12, 1–36. doi: 10.1080/14792772143000003
Souvignier, E., Förster, N., Hebbecker, K., and Schütze, B. (2021). “Using Digital Data to Support Teaching Practice – quop: An Effective Web-Based Approach to Monitor Student Learning Progress in Reading and Mathematics in Entire Classrooms,” in International Perspectives on School Settings, Education Policy and Digital Strategies: A Transatlantic Discourse in Education Research, eds A. Wilmers and S. Jornitz (Leverkusen: Verlag Barbara Budrich), 283–298. doi: 10.2307/j.ctv1gbrzf4.20
Tappe, E. -H. (2017). Lernen durch Mediengestaltung: Entwicklung eines Konzeptes zur Unterstützung mediendidaktischer Lehre im Schulalltag [Learning through Media Design: Development of a Concept to Support Media Didactic Teaching in Everyday School Life]. [dissertation]. Münster: Westfälische Wilhelms-Universität.
Teo, T., and Tan, L. (2012). The Theory of Planned Behavior (TPB) and Pre-Service Teachers’ technology acceptance: A validation study using structural equation modeling. J. Technol. Teach. Educ. 20, 89–104.
Tondeur, J., Aesaert, K., Prestridge, S., and Consuegra, E. (2018). A multilevel analysis of what matters in the training of pre-service teacher’s ICT competencies. Comput. Educ. 122, 32–42. doi: 10.1016/j.compedu.2018.03.002
Van Buuren, S. (2012). Flexible Imputation of Missing Data. New York, NY: CRC Press Taylor & Francis Group.
Vanbecelaere, S., Cornillie, F., Depaepe, F., Guerrero, R. G., Mavrikis, M., Vasalou, M., et al. (2020). “Technology-mediated personalised learning for younger learners,” in Proceedings of the 2020 ACM Interaction Design and Children Conference: Extended Abstracts, eds S. Price, E. Rubegni, and A. Vasalou (New York, NY: ACM), 126–134. doi: 10.1145/3397617.3398059
Venkatesh, V., Morris, M. G., Davis, G. B., and Davis, F. D. (2003). User acceptance of information technology: Toward a unified view. MIS Q. 27, 425–478.
Wayman, J. C., Wilkerson, S. B., Cho, V., Mandinach, E. B., and Sopovitz, J. A. (2016). Guide to using the Teacher Data Use Survey (REL 2017–166). Washington, DC: U.S. Department of Education, Institute of Education Sciences, National Center for Education Evaluation and Regional Assistance, Regional Educational Laboratory Appalachia.
Keywords: Data-Based Decision Making, digital learning platforms, individualization, Learning Analytics, primary school teacher, structural equation modeling, Theory of Planned Behavior
Citation: Hase A, Kahnbach L, Kuhl P and Lehr D (2022) To use or not to use learning data: A survey study to explain German primary school teachers’ usage of data from digital learning platforms for purposes of individualization. Front. Educ. 7:920498. doi: 10.3389/feduc.2022.920498
Received: 14 April 2022; Accepted: 29 July 2022;
Published: 18 August 2022.
Edited by:
David Scheer, Ludwigsburg University of Education, GermanyReviewed by:
Christine Espin, Leiden University, NetherlandsCopyright © 2022 Hase, Kahnbach, Kuhl and Lehr. This is an open-access article distributed under the terms of the Creative Commons Attribution License (CC BY). The use, distribution or reproduction in other forums is permitted, provided the original author(s) and the copyright owner(s) are credited and that the original publication in this journal is cited, in accordance with accepted academic practice. No use, distribution or reproduction is permitted which does not comply with these terms.
*Correspondence: Alina Hase, aGFzZUBsZXVwaGFuYS5kZQ==
Disclaimer: All claims expressed in this article are solely those of the authors and do not necessarily represent those of their affiliated organizations, or those of the publisher, the editors and the reviewers. Any product that may be evaluated in this article or claim that may be made by its manufacturer is not guaranteed or endorsed by the publisher.
Research integrity at Frontiers
Learn more about the work of our research integrity team to safeguard the quality of each article we publish.