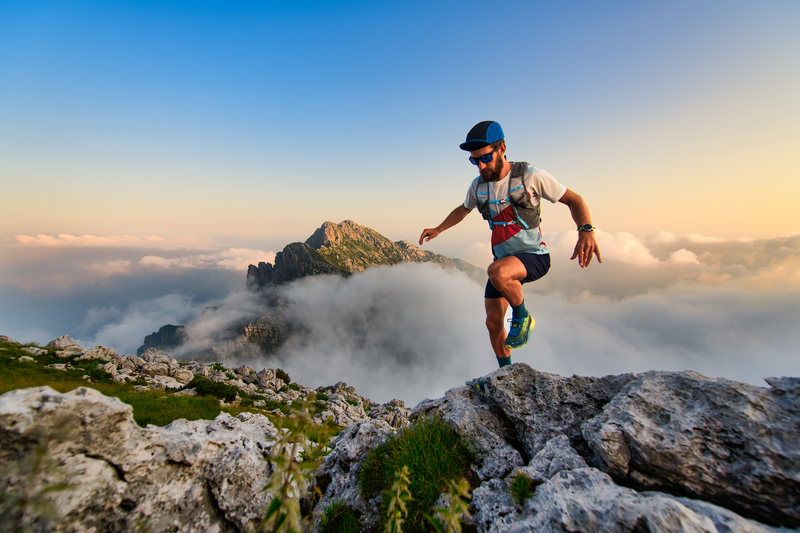
95% of researchers rate our articles as excellent or good
Learn more about the work of our research integrity team to safeguard the quality of each article we publish.
Find out more
ORIGINAL RESEARCH article
Front. Educ. , 06 July 2022
Sec. Educational Psychology
Volume 7 - 2022 | https://doi.org/10.3389/feduc.2022.917944
This article is part of the Research Topic Progress Monitoring and Data-Based Decision-Making in Inclusive Schools View all 14 articles
In intervention research, single-case experimental designs are an important way to gain insights into the causes of individual changes that yield high internal validity. They are commonly applied to examine the effectiveness of classroom-based interventions to reduce problem behavior in schools. At the same time, there is no consensus on good design characteristics of single-case experimental designs when dealing with behavioral problems in schools. Moreover, specific challenges arise concerning appropriate approaches to analyzing behavioral data. Our study addresses the interplay between the test power of piecewise regression analysis and important design specifications of single-case research designs. Here, we focus on the influence of the following specifications of single-case research designs: number of measurement times, the initial frequency of the behavior, intervention effect, and data trend. We conducted a Monte-Carlo study. First, simulated datasets were created with specific design conditions based on reviews of published single-case intervention studies. Following, data were analyzed using piecewise Poisson-regression models, and the influence of specific design specifications on the test power was investigated. Our results indicate that piecewise regressions have a high potential of adequately identifying the effects of interventions for single-case studies. At the same time, test power is strongly related to the specific design specifications of the single-case study: Few measurement times, especially in phase A, and low initial frequencies of the behavior make it impossible to detect even large intervention effects. Research designs with a high number of measurement times show robust power. The insights gained are highly relevant for researchers in the field, as decisions during the early stage of conceptualizing and planning single-case experimental design studies may impact the chance to identify an existing intervention effect during the research process correctly.
While experimental group designs are the most common way of testing educational and psychological research hypotheses, single-case experimental designs (SCED) experienced a renaissance over the last decades (Smith, 2012). In intervention research, SCEDs are a vital way to gain insight into the causes of individual changes that yield high internal validity (Kratochwill et al., 2010; Shadish et al., 2015). Among others, SCEDs are commonly applied to examine the effectiveness of classroom-based interventions to reduce behavioral problems in schools. Several literature reviews of SCED behavioral intervention studies have been published in the past few years. For example, Briesch and Briesch (2016) summarize the findings of single-case research on 48 behavioral self-management intervention studies. Soares et al. (2016) synthesized results of 28 single-case studies focusing on the effect size of token economy use in classroom settings. More recently, Moeyaert et al. (2021) summed up the body of research on the effects of peer-tutoring on academic and social-emotional outcomes and included 46 single-case studies. Several additional examples of the application of SCED in similar fields can be identified (e.g., Busacca et al., 2015; Harrison et al., 2019). However, at the same time, there is no consensus on good design characteristics of SCED when dealing with count data. Moreover, specific challenges arise concerning appropriate approaches to analyzing behavioral SCED data.
This paper aims to clarify these questions by specifying which factors (hereafter design specifications) influence the chance (i.e., statistical test power) of detecting an intervention effect in a single-case behavioral intervention study. In addition, based on the results gained, we aim to provide recommendations for SCED or at least to identify criteria for a researcher to consider when planning a single-case study.
The most basic structure of a SCED consists of time series measurements on one individual divided into two phases: Continuous measurements occur before the start of a specific event (phase A) and continuous measurements taken after the event, e.g., the manipulation of an independent variable (phase B). This design can be extended to numerous variations regarding the number and order of phases (e.g., ABAB or AB1B2B3) based on specific research questions and assumptions on the nature of the behavior and the resulting data (Nock et al., 2007). Following the experimental logic of counterfactual thinking, the data of phase A serve as a reference for what would have happened in phase B if no intervention had taken place. Therefore, the level and development in phase B are compared to the level and development in phase A.
Despite the usefulness and importance of such SCEDs in applied research, researchers have to find common ground on how many measurements and phases should be included in SCED. Kratochwill et al. (2013) provide an overview of single-case intervention research design standards developed by a panel of experts in SCED methodology. However, these important design recommendations include only very general design specifications and do not consider the specific characteristics of the measured feature (scaling and distribution). In contrast, we hypothesize that recommendations should be different when the measurements are count data (e.g., problem or error frequencies, which are Poisson distributed) or standardized scales (e.g., T or Z test scores, which are Gaussian distributed). We also hypothesize that choosing a particular SCED design depends on several design specifications (see Figure 1): the initial problem intensity at the start of a study, the intervention effect’s expected strength (a level or a slope effect), and an expected data trend.
Figure 1. Design specifications potentially influencing the test power of single-case experimental designs (SCEDs).
In addition to the design specifications, we also need to determine the method of data analysis since not all methods have the same sensitivity (or power). If someone decides to base the data analysis solely on visual inspection, one might recommend a different design than if the data analysis is based on a piecewise regression model.
Traditionally, single-case data have been analyzed through visual analysis (Parker and Brossart, 2003). Specifically, visual analysis is based on visual inspection of graphed time-series data where patterns related to level, trend, and overlapping/non-overlapping phases are evaluated to determine intervention effects (e.g., Parker and Vannest, 2012). Critics point out that visual analysis is overly subjective, vulnerable to misinterpretations due to data trends or outliers, and has less power (an increased type II error risk) compared to statistical analyses (Greenwald, 1976; Jones et al., 1978; Keppel, 1982; Matyas and Greenwood, 1990; Allison, 1992; Klapproth, 2018; Wilbert et al., 2021). There is evidence that agreement among multiple analysts and the consistency of their conclusions could be increased by using systematic protocols (Maggin et al., 2013; Wolfe et al., 2019).
Several statistical analysis techniques have been developed to overcome these critics throughout the last decades, either as a complement or a substitute for visual analysis. These procedures comprise overlapping indices (see Parker and Brossart, 2003) and “classical” statistical tests for comparing differences between groups like Student’s t-tests and Mann-Whitney U-tests. These approaches have both benefits and significant limitations (e.g., not addressing autocorrelation and the existence of a trend throughout the data). Consequently, more complex statistical approaches have been applied to single-case data. These primarily include regression-based accounts (Huitema, 1986; Beretvas and Chung, 2008), randomization tests (Edgington and Onghena, 2007; Dugard et al., 2012; Heyvaert and Onghena, 2014), and mixed-effect models (Davis et al., 2013; Shadish et al., 2013; Moeyaert et al., 2014). These approaches address many of the former shortcomings like autocorrelation and the existence of a trend throughout the data (piecewise-regression models and randomization test), differentiate between immediate and continuous effects of an intervention (piecewise regression models), and allow the mutual analysis of several SCEDs (mixed models).
Many criteria considered in visual analysis are included and modeled in these more sophisticated statistical approaches (e.g., immediate and evolving intervention effects, data trends, data variability, complex phase contrasts). Other criteria specific to visual inspection may have to be investigated in more detail so they can be added to the statistical models explicitly (e.g., non-linearity of effects, outliers, lagged onset of intervention effects).
It is not easy to decide which approach is the “best” for analyzing single-case data. The underlying approaches to data analyses and statistics are fundamentally different: Piecewise regression analyses model data according to a complex theoretic model about the structure of single cases. Conversely, visual inspection relies on human expertise, pattern recognition, and intuition while overlap indices are targeted toward practitioners as an easy and accessible way to calculate effect sizes to validate their subjective judgment.
Based on the abovementioned arguments and studies, we consider piecewise regression models as one potentially appropriate and versatile approach among other alternatives. Notwithstanding, applying regression-based analyses (piecewise regression models and mixed models) comes with additional questions about the adequate distribution for modeling the dependent variable (more precisely, the error term) and the proper link function. Most implementations of regression analyses for SCED data are based on OLS estimators (e.g., Huitema and Mckean, 2000) or generalized models with ML estimators based on Gaussian distributions (Ferron, 2002; Beretvas and Chung, 2008). While these estimators are adequate when the measured variable is continuous and normally distributed (e.g., a score in a standardized math test), they are less suitable for analyzing count data.
However, in single-case research, there are multiple types of dependent measures including count or frequency data. This is predominantly the case in SCEDs focusing on behavioral problems in schools: the dependent variable is often conceptualized as the frequency of a specific behavior within a certain period (e.g., disruptive or aggressive behavior). Frequencies are discrete numbers in nature; the Gaussian distribution models continuous values. Furthermore, frequencies can never be negative. Nevertheless, all negative numbers are modeled with a certain probability in a Gaussian probability density function. In line with this, Shadish and Sullivan (2011), in their overview of published SCED studies, argue:
Of particular interest is the fact that nearly all outcome variables were some forms of a count. Most parametric statistical procedures assume that the outcome variable is normally distributed. Counts are unlikely to meet that assumption and, instead, may require other distributional assumptions. In some cases, for example, the outcome is a simple count of the number of behaviors emitted in a session of a fixed length, which has a Poisson distribution (p. 979).
Binomial and Poisson distributions might be adequate alternatives. Binomial distributions display the probability of an outcome frequency given the number of events and the probability of an outcome for each event. Therefore, they are adequate for modeling count data and proportions (e.g., the frequency of behaviors). In cases where the occurring number of events is low, but the potential number of events is high, Poisson distributions are a viable alternative. These distributions depict a binomial distribution when the number of potential events approximates infinity, and the expected frequency of an outcome (λ) is given. While a binomial distribution gives the probabilities of frequencies in the case of a finite exact number of possible occurrences, the Poisson distribution depicts the expected frequencies of an outcome when the number of possible occurrences approximates infinity. Such conditions are often met when behavioral data are measured. Consider, for example, a researcher investigating the occurrence of inappropriate behavior. At its extreme, a student might show inappropriate behavior at any second. At the same time, it is also realistically possible that no inappropriate behavior occurs at all.
Despite these arguments, piecewise Poisson-regression models are not widespread in SCED research. This depicts a potential limitation to existing studies as effects might not have been adequately identified as relying on flawed distributional assumptions impacts the power of the chosen analytical approach. In addition, the use of Poisson distributions in regression models as means of analyzing SCED has not been examined in detail. Insights into test power and alpha error rate are lacking. However, such insights might yield crucial additional information on the adequacy of the design specifications of SCED.
The present paper aims to investigate the test power of piecewise regression analyses for analyzing SCEDs with count data. Thereby, we aim to address the impact of essential design specifications of SCEDs on test power. More specifically, we examine the influence of the following aspects on the test power:
(1) The initial frequency of the (problem) behavior,
(2) The strength of the intervention effect,
(3) The number of measurement times in phase A (baseline) and phase B (intervention),
(4) The interaction between initial frequency, the strength of the intervention effect, and the number of measurement times,
(5) The interaction of the number of measurement times in phase A and phase B, and the initial frequency of the behavior,
(6) The presence of a trend in the data,
(7) The interaction of a trend in the data, the strength of the intervention effect, and the number of measurement times.
Besides the test power, we will also report the alpha-error probabilities (type I errors) for all investigated conditions. Our regression approach will extend the piecewise regression model proposed by Huitema and Mckean (2000) to include Poisson distributed dependent variables. These insights might depict an important orientation for deriving design principles of adequate SCED in the context of behavioral data.
To answer the research questions mentioned above, we set up several Monte-Carlo simulation studies that focused on specific design specifications of SCEDs. The general idea behind such simulations is to generate a high number of random single-case datasets with specified conditions (e.g., a specific intervention effect). Afterward, these datasets are analyzed (here, using a piecewise Poisson-regression model). Comparing the results of each analysis to the initial setup of the random case generates four results:
(1) True-positive: The initial setup contained an intervention effect, and the analysis found a significant effect.
(2) True-negative: The initial setup did not contain an intervention effect, and the analysis did not find a significant effect.
(3) False-positive: The initial setup did not contain an intervention effect, and the analysis found a significant effect.
(4) False-negative: The initial setup did contain an intervention effect, and the analysis did not find a significant effect.
The proportion of true positive results is the power, and the proportion of the false-positive results is the alpha error probability of a test for the given design specifications.
The data simulation followed the rationale elaborated below. For any studies applying a Monte-Carlo approach, the validity of the findings and their relevance to practice depend on the characteristics of the data generated. Therefore, we paid particular attention to aligning the simulated data, if reasonable, with the reality of published SCED studies.
AB-Designs are the simplest form of a SCED comprised of a baseline (phase A) and an intervention phase (phase B). At the same time, AB depicts the building block for any multiple-phase design, and the multiple baseline design (MBD) – the most frequent SCED (Shadish et al., 2014). Therefore, we decided to choose an AB design as the underlying phase design of the simulated data.
We were particularly interested in analyzing intervention studies in which a teacher or researcher attempts to reduce a specific (problematic) behavior during classroom learning. Here, the target behavior is captured through systematic direct observations (e.g., Hintze et al., 2002; Lane and Ledford, 2014; Ledford et al., 2018), which are the “most widely used outcomes in single-case research” (Pustejovsky, 2018, p. 100). Thus, we used Poisson-regression models. The simulated data should represent count data (frequency of the observed behavior).
Another potential factor influencing the test power and alpha-error probability of the analyses is the frequency of the dependent variable. The behavior of interest to the particular research question may be scarce (e.g., self-harming behavior during class) or widespread (e.g., disturbing behavior). Hence, the problem behavior frequency depends on the behavior of interest and the exact operationalization. Therefore, we decided to set up a simulation where we vary the expected problem intensity starting with a low frequency of 5 to a high frequency of 30. These frequencies follow the mean baseline frequencies of adverse valence outcomes described in the overview of 303 published SCEDs provided by Pustejovsky et al. (2019, p. 24). In simulations where we did not focus on the relevance of behavior frequencies, we chose an expected behavior frequency of 15.
A certain proportion of published SCED studies include fewer than three phase A measurement times (Pustejovsky et al., 2019). This contradicts both current recommendations (e.g., Kratochwill et al., 2013) and the basic requirements of regression methods. We simulated single-case data using a minimum of three measurements per phase following usual conventions (e.g., Hitchcock et al., 2014). Further, Pustejovsky et al. (2019) found that the number of phase A measurements was below 20 for the overwhelming majority of SCED studies. Most studies had between 2 and 15 phase A measurement times. Therefore, we set up a simulation varying the length of phase A between 3 and 19 measurements. In line with Smith (2012), who found an average of 10.2 phase A observations in their review of 400 published SCED studies, we used 10 phase A measurements for the other simulations.
In addition to varying phase A (baseline) lengths, the number of measurement times in phase B (intervention) also varies, for example, due to the number of sessions of an implemented intervention. We, therefore, varied the number of measurement times (the length) of phase B in one simulation. Usually, the length of phase B exceeds the length of phase A. We took this into account by setting the minimum length of phase B to 10 measurements and the maximum to 50. We set 20 phase B measurements as a fixed value for the other simulations.
Another essential characteristic of SCED studies is the strength of the intervention effect (i.e., the reduction of the problem behavior). Most of the published research using SCEDs usually reports quite significant effects; however, it needs to be considered that this might also be due to a publication bias (Travers et al., 2016; Dowdy et al., 2022). In addition, the majority of the published SCED studies report different measures of effect sizes (such as overlap indices). Only a few studies report effect sizes associated with regression analysis. Therefore, it is difficult to derive an expected “mean” intervention effect from existing studies. We addressed this challenge by setting up a simulation with varying intervention effects employing the level effect between 20% and 80% problem reduction. We used a reduction of the dependent variable by 50% for the other simulations. In practice, behavior reductions of this magnitude are considered substantial (Vannest and Sallese, 2021, p. 17). We further assumed that, on average, no additional slope effect would be present in the data, but we included a slope effect for each case randomly drawn from a gaussian distribution with a mean of zero and a standard deviation of 10% of the initial problem behavior frequency. We considered that an intervention does not exactly exert the same effects on every individual.
Another common feature of single-case data is the presence of a trend effect in the data. This trend indicates an overall development in the problem behavior, which already appears in phase A (baseline) and is independent of the intervention. This trend might be positive (increasing the problematic behavior frequency across time) or negative (reducing the problematic behavior) and depends on many individual variables (e.g., additional support from home; negative peer influence; maturation). Therefore, we set up a simulation for positive and negative trend effects by varying the trend’s strength between a decrease of 60% to an increase of 60% of the problem behavior frequency throughout all measurements. For all the other simulations, we included a random trend effect for each simulated single-case drawn from a gaussian distribution with a mean of zero and a standard deviation of 10% of the initial problem behavior.
We conducted three simulations. Each simulation varied specific SCED specifications.
For simulation 1, we varied the intervention effect (4 iterations: −0.2; −0.4; −0.6; −0.8), the number of measurement times in phases A (9 iterations: 3, 5, 7, 9, 11, 13, 15, 17, 19), and the number of measurement times in phase B (7 iterations: 10, 15, 20, 25, 30, 40, 50), resulting in 4 × 9 × 7 = 252 design conditions.
For simulation 2, we varied the initial frequency of the behavior (6 iterations: 5; 10; 15, 20; 25; 30), the intervention effect (4 iterations: −0.2; −0.4; −0.6; −0.8), and number of measurement times (6 iterations: 15, 21, 27, 33, 39, 45 where 1/3 of the measurements belong to phase A and 2/3 to phase B), resulting in 6 × 4 × 6 = 144 design conditions.
For simulation 3, we varied the initial frequency of the behavior (6 iterations: 5; 10; 15, 20; 25; 30), the trend effect (5 iterations: −0.6; −0.4; 0; 0.4; 0.6), and number of measurement times (6 iterations: 15, 21, 27, 33, 39, 45 where 1/3 of the measurements belong to phase A and 2/3 to phase B), resulting in 6 × 5 × 6 = 180 design conditions.
For each design condition within each simulation, 10,000 random single cases with the respective design specifications were generated (the generation algorithm below). Each case was analyzed with a piecewise Poisson-regression model (see below). The proportion of significant intervention effects in these analyzes is the test power for the respective attributes for that design condition.
In a second step, another 10,000 random single-cases were created for each design condition. This time, the intervention effect was set to zero for all cases. Again, each case was analyzed with a piecewise Poisson-regression model. The proportion of significant intervention effects detected in these analyses is the design condition’s alpha-error probability.
Preparatory tests have shown that we need a rather high number of 10 000 cases per variant to achieve a stable estimate. This is due to various random parameters and several interactions that go into the data generation algorithm (see section “Results” and Table 1).
The random cases were generated with the R package scan (Wilbert and Lüke, 2022). The same package was used for calculating the test power and alpha error probability. The source code for all analyzes is available as an online supplement to this paper1.
Firstly, a random single-case was created by calculating the expected behavior frequency (λ) for each measurement (i). The formula adapts a piecewise-regression model for single cases:
where,
i = The index of a measurement.
start = The initial problem frequency at the start of the study.
phase = A variable with 0 for phase A and 1 for phase B measurements.
level = The change of expected problem behavior frequency due to the intervention (e.g., −0.5 for a 50% reduction).
mt = The measurement time.
trend = A trend effect leading to a change in problem behavior frequency for each measurement. Calculated by .
slope = A change of expected problem behavior frequency for each measurement that starts with the onset of phase B. For simulations 1 and 2 calculated by .
MTA = The number of measurement times of phase A.
Second, the observed values for each measurement y were drawn from a Poisson distribution with the expected probability:
Depending on the respective aim of the simulation, start, MTA, MTB (the total number of measurements – MTA), level, and trend were varied.
Figure 2 shows three corresponding examples of single cases.
Each randomly generated case was re-analyzed with a piecewise regression model (Huitema and Mckean, 2000) adapted for Poisson distributed data:
Table 2 shows an example of a piecewise Poisson-regression analysis for the first example case of Figure 2. Here, the level phase B effect is significant (B = −1.18, p < 0.01). As the original construction algorithm for that single case entailed an intervention effect, the result of this analysis is true-positive.
Table 2. Piecewise Poisson-regression table, for case 1 in Figure 2.
In the present study, we investigated how various specifications of SCEDs affect the statistical power of regression-based analyses assuming Poisson-distributed behavioral data. In addition, we focused on those design parameters that we believe are most frequently discussed and most likely to be influenced by researchers when planning a SCED. All figures in this paper are created with the software packages ggplot (Wickham, 2016) and scplot (Wilbert, 2022). All data and analyses are reproducible and made available on the project page (see text footnote 1).
First, we examined the statistical power as a function of the intervention effect and the number of measurement times in phases A and B. The initial frequency of the behavior is kept constant, and the trend- and slope effect sizes are randomly generated for each case with an expected value of zero (see Table 1).
Figures 3A-D depict the power (blue lines) and alpha-error probability (red lines) for all design conditions. The figures also include lines marking the usually recommended minimal power level of 80% and the maximum alpha-error probability of 5%. The length of phase A is plotted on the x-axis (between 3 and 19). The shape of the dots describes the respective length of phase B (between 10 and 50). The facets (Figures 3A-D) refer to the strength of the intervention effect (between −0.2 and −0.8).
Figure 3. Power and alpha error rates (line colour) for different intervention effect sizes (part) and measurement times per phase (dot shape and x-axis) (simulation 1).
No relevant power is obtained for a small intervention effect (20% reduction of the problem behavior) regardless of the number of measurement times in phases A and B (Figure 3D). With a reduction of problem behavior by 40% at the beginning of phase B (Figure 3C), a significant power of more than 80% is only achieved with a large number of measurement times; more precisely, with 11 measurement times in phase A and ≥50 measurement times in phase B, as well as with 13 measurement times or more in phase A and ≥40 measurement times in phase B. If the intervention reduces the problem behavior by 60% (Figure 3B), sufficient power is achieved with designs of ≥5 measurement times in phase A and ≥15 measurement times in phase B, improving further with ≥11 measurement times in phase A. For designs with ≤ 10 measurement times in phase B, sufficient power is achieved only with ≥9 measurement times in phase A. With an 80% reduction of the problem behavior with the intervention’s start, statistical power is satisfactory across all design conditions (Figure 3A). In particular, with ≥15 measurement times in phase B, the probability of detecting an intervention effect is high regardless of the number of measurement times in phase A.
The alpha-error probability is stable at 5% across all design conditions.
Next, we consider the influence of the intervention effect size, the initial behavior frequency, and the total length of the design (see Table 1 for a list of all parameters in this simulation). Figure 4 shows the results and is analogous to Figure 3. The number of measurement time points (1/3 phase A and 2/3 phase B) is plotted on the x-axis (between 15 and 45). The shape of the dots describes the strength of the intervention effect (between −0.2 and −0.8). The facets (Figures 4A–F) refer to the initial frequency of the behavior (between 5 and 30).
Figure 4. Power and alpha error probability (line colour) for different intervention effect sizes (dot shape), initial behavior frequency (part), and number of measurement times (x-axis) (simulation 2).
Both a very low initial behavior frequency and few measurement times lead to poor test power. Regardless of the other specifications of the design, small intervention effects (20% reduction of problem behavior at the beginning of phase B) cannot be detected reliably (Figures 4A-D, lines with crosses). When the intervention reduces the target behavior by 40% (lines with squares), sufficient power is achieved only when the initial behavior frequency and the number of measurement times are high (≥20 initial behavior frequency and ≥33 MT; ≥25 initial behavior frequency and ≥27 MT). Large intervention effects such as an 80% reduction in problem behavior can be reliably detected at initial behavior frequencies of ≥10. For medium-level effects of the intervention (60% reduction; Figure 4, lines with triangles), a sufficient power depends on the combination of the other conditions: If the initial behavior frequency is ≥20, sufficient power is reliably achieved. With ≥30 measurement time points, sufficient power is achieved even with an initial frequency of 10 or 15. With an initial behavior frequency of 5, on the other hand, even a large number of measurement times no longer helps to achieve sufficient power.
The alpha-error probability is stable at 5% for all design conditions.
Finally, we would like to consider in more detail the interplay of the initial behavior frequency, the number of measurement times, and the data trend (see Table 1 for a list of all parameters in this simulation). Figure 5 depicts the results and is built analogous to the previous figures. The number of measurement time points (1/3 phase A and 2/3 phase B) is plotted on the x-axis (between 15 and 45). The shape of the dots describes the strength of the trend effect (between −0.6 and 0.6). The facets (Figures 5A-F) refer to the initial frequency of the behavior (between 5 and 30).
Figure 5. Power and alpha error probability (line colour) for trend effects (dot shape), initial behavior frequency (part), and number of measurement times (x-axis) (simulation 3).
A data trend of 60% reduction in the problem behavior frequency throughout the study (Figure 5, lines with circles) strongly reduces the test power for all design conditions. Only exceptionally high initial levels of the problem behavior (≥25) and large numbers of measurement times (≥33; Figure 5E) show a power level ≥80%. In cases with a weaker, negative data trend (≥−40%), this problem is no longer observed (lines with triangles), and the power is comparable to designs without a trend.
The effect of the initial behavior frequency on the test power described in section “Simulation 3: Initial Frequency of the Behavior, Data Trend, and Number of Measurement Times” can be similarly identified here: Only for designs with an initial behavior frequency ≥15 sufficient power is achieved for most cases. Especially in Figures 5C-E, the interaction between all three parameters becomes apparent: While sufficient power is not achieved for designs with a substantial negative data trend, the detection rate is acceptable for increasing (or stable) problem behavior (≥0) and designs with ≥27 measurement times. In cases with a high initial behavior frequency (≥25; Figure 5E), the power approaches 100% quite rapidly.
Again, the alpha-error probability is stable at 5% for all design conditions.
The goal of the paper at hand was to shed light on the usefulness of applying piecewise Poisson-regression models (in terms of statistical power) to analyze single-case data under varying design specifications. Specifically, we investigated the influence of phase length, intervention effect size, initial frequency of the dependent variable, and the size of a trend effect on test power.
Overall, the results of the conducted simulations indicate that Poisson-regressions have a high potential of identifying (i.e., a test power of 80% or higher) intervention effects. However, at the same time, the test power was low under specific conditions. Hence, following our theoretical assumptions, test power seems to be related to the specific design specifications of the SCED study. The alpha-error probability was 5% for all conditions, even with very strong trend effects. The insights gained are highly relevant for researchers in the field, as design decisions during the early stage of conceptualizing and planning SCED studies might impact the overall potential of correctly identifying an existing intervention effect. Our results might guide researchers on crucial elements of SCEDs to prevent unfavorable decisions.
In our study, the level effect of the intervention had a powerful influence on statistical power. Strong effects, where the behavior was reduced by 60% or higher, were correctly identified under almost all conditions. However, the exact characteristics played a crucial role when the intervention effects were medium or low. Effects that were equivalent to a reduction of 20% could not be correctly identified (independent of the design characteristics). Prior knowledge about the intervention’s expected effect size might help researchers make research design decisions that lead to higher statistical power. However, such knowledge might not be available for all kinds of interventions. Moreover, the expected intervention effect is not something researchers have control over. Therefore, the following discussion will primarily focus on parameters that are at least under partial control of the researcher, designing and conducting the study.
In contrast to the effect size, researchers can influence the operationalization of the outcome variable. A dependent variable can be operationalized differently, leading to different outcome variable frequencies (e.g., a higher sampling rate or larger observation intervals for each measurement time). This is an asset for researchers as the results of our study indicate that the outcome variable frequency has a substantial impact on statistical power, too. Low initial behavior frequencies set high demands on the number of measurement times required to correctly identify effects (especially when the intervention effect is small) or might even wholly prevent its identification (initial behavior frequency ≤ 5). Based on our results, we would recommend targeting operationalizations that allow initial problem behavior frequencies greater than 20. Such frequencies are in line with the existing state of research in SCED (Pustejovsky et al., 2019).
The number of measurement times is one of the main design elements of SCEDs that the investigator can influence. Our study indicates that the length of phase A has a significant influence on the resulting test power: Low numbers of measurement times in phase A (≤7), which are common, hinder the identification of even strong intervention effects (60% reduction). Nonetheless, such low numbers of measurement times (e.g., 3) depict the lower end of the recommendations in the relevant literature (e.g., Kratochwill et al., 2013). This suggests that many published single-case studies have low power due to a short phase A length. It is better to prolong phase A than phase B in those cases. This seems to be a particularly relevant finding, as researchers might feel forced to begin an intervention (phase B) as quickly as possible due to ethical (stressful classroom situation) or economic (costs which come along with the extension of phase A) reasons. However, our results emphasize the need to extend phase A (even under challenging conditions) as the costs for a short phase A might be the failure to identify a potentially helpful intervention. Extending phase B cannot compensate for a low number of measurement times in phase A. Based on our results, we recommend at least nine measurement times during phase A when the estimated intervention effect is an estimated reduction of 60% or more. When the reduction is between 40% and 60%, collect data for at least 15 measurement times in phase A and extend phase B to at least 30 measurement times.
A similar pattern of results occurs when focusing on the number of measurement times in phase B. Again, an increment in the number of measurement times leads to an overall increase in statistical power. However, the number of measurement times in phase A and intervention effect size seem to be of higher relevance (given a reasonable number of at least 15 measurements in phase B). This implies that extending phase B does not improve statistical power to a sufficient level if the number of measurement times in phase A is too small. For smaller intervention effects (i.e., a reduction of 40%), the length of phase B seems of additional relevance when the length of phase A increases.
Depending on the situation, one can make assumptions about the presence, intensity, and direction of a data trend (e.g., when researchers receive information about the student’s development prior to the study). In many situations, however, trend effects are difficult to predict. Our results suggest that piecewise Poisson-regressions are robust to the possible influence of trend effects (i.e., the results showed no increased alpha error risk even when very strong trend effects were prevalent). Nevertheless, a strong negative trend effect (i.e., a reduction of 60% across all measurements of a single case) affects test power. Since this finding occurs mainly in situations where the initial frequency of the behavior is low, a possible explanation could be a floor effect (e.g., due to the data trend frequencies being so low that the intervention effect cannot develop its full strength). Since trend effects thus might play an important role in predicting test power, it seems crucial to control for the presence of such effects during data analysis. Here, the results of a piecewise regression analysis might help detect a strong trend effect after the data collection. Recognizing a data trend could subsequently serve as further evidence for a potential limitation of test power.
The results of our study clearly emphasize the power of piecewise Poisson-regressions in analyzing SCED studies. Despite the usefulness of the chosen analytical approach, it becomes clear that important design specifications must be considered. Despite our efforts to derive some guiding principles, it becomes clear that the test power depends on an intricate interplay between various design specifications. What an adequate single-case experimental design looks like depends on the context, the type of intervention, and the behavior to address. As with all other hypothesis-testing research designs, researchers planning SCED studies should include power analyses in their research planning. Factors such as the number of measurement times or the precise operationalization of the dependent variable can often be adjusted to improve the design of studies from the very beginning. In addition, post hoc power analyses also help to provide at least a rough estimate of the statistical power and uncover the strength and caveats of a design. Based on our results, it additionally becomes clear that the characteristics of SCEDs that come along with high test power deviate from common practice, especially regarding the number of measurement times.
Despite the insights gained, the study at hand has some limitations. First, our insights are limited to a specific scenario (i.e., count data; an intervention aiming at a frequency reduction), which cannot be generalized to all potential scenarios that might occur in practice. Therefore, additional simulation studies addressing other scenarios are recommended. Specifically, our intervention effect only comprised a level effect and no additional slope effect. However, a slope effect might occur (depending on the interventional approach). Second, we focused on AB designs as the essential ingredient of many SCED variants. In research practice, AB designs only represent one design among other SCEDs. Therefore, the validity of our results is restricted to AB designs.
We focused on the use of regression analysis in this study. Other procedures exist to estimate phase differences in SCED data, such as overlap indices or randomization tests. Our results are not simply generalizable to these procedures. However, we would argue that the power of these procedures is no higher than that of the regression analyses analyzed here. Thus, the requirements for achieving sufficient power are likely to be even higher. Fortunately, software packages are available today to calculate exact power estimations for specific design specifications. All analyses in this study have been calculated with the R package scan (Wilbert and Lüke, 2022), which also allows for calculating the power for different SCEDs (e.g., multiple baseline and multiphase designs; gaussian or binomial distributed data) and other methods of data analysis (e.g., randomization tests or Tau-U).
Based on the result of our analyses, we would like to recommend that researchers conduct a priori power analysis for any SCED they are planning. If the intended research design yields insufficient power (usually below 80%) or the alpha-error probability is too high (usually above 5%), two optional modifications to the SCED can increase the power of the design: (1) Increasing the number of measurement times, especially in phase A (often phase A is too short). (2) Implement a more sensitive operationalization that increases the frequency of the dependent variable (ideally to an initial frequency of at least 20). In addition, conducting a multiple-baseline design with three or more cases/situations or adding a second A and B phase (withdrawal design) may also increase the statistical power of the design.
Researchers cannot and will not always optimize decisions regarding their specific research design in favor of statistical power. Sometimes, the specific circumstances in which SCEDs are applied prevent this (e.g., ethical reasons, opportunities to implement an intervention in the institutional context). Whenever possible, however, we consider it necessary for research in SCEDs to take into account the test power and alpha-error probability and, accordingly, to conduct only those studies that can realistically detect an existing intervention effect. We believe that it would be beneficial in the future to present and demand considerations of statistical power for publications reporting SCEDs as well.
All data and analyses presented in this manuscript are publicly available in the Open Science Framework: https://osf.io/ys3a9/ and https://files.eric.ed.gov/fulltext/ED510743.pdf.
JW did the conceptualization, carried out the data curation, formal analysis, and software, investigated the data, performed the methodology, visualized the data, wrote the original draft, and wrote, reviewed, and edited the manuscript. MB-R and TL did the conceptualization, investigated the data, performed the methodology, wrote the original draft, and wrote, reviewed, and edited the manuscript. All authors contributed to the article and approved the submitted version.
Funded by the Deutsche Forschungsgemeinschaft (DFG, German Research Foundation) – Project number: 491466077.
The authors declare that the research was conducted in the absence of any commercial or financial relationships that could be construed as a potential conflict of interest.
All claims expressed in this article are solely those of the authors and do not necessarily represent those of their affiliated organizations, or those of the publisher, the editors and the reviewers. Any product that may be evaluated in this article, or claim that may be made by its manufacturer, is not guaranteed or endorsed by the publisher.
Allison, D. B. (1992). When cyclicity is a concern: a caveat regarding phase change criteria in single-case designs. Compr. Ment. Health Care 2, 131–149.
Beretvas, S. N., and Chung, H. (2008). A review of meta-analyses of single-subject experimental designs: methodological issues and practice. Evid. Based Commun. Assess. Interv. 2, 129–141. doi: 10.1080/17489530802446302
Briesch, A. M., and Briesch, J. M. (2016). Meta-analysis of behavioral self-management interventions in single-case research. School Psychol. Rev. 45, 3–18. doi: 10.17105/spr45-1.3-18
Busacca, M. L., Anderson, A., and Moore, D. W. (2015). Self-management for primary school students demonstrating problem behavior in regular classrooms: evidence review of single-case design research. J. Behav. Educ. 24, 373–401. doi: 10.1007/s10864-015-9230-3
Davis, D. H., Gagné, P., Fredrick, L. D., Alberto, P. A., Waugh, R. E., and Haardörfer, R. (2013). Augmenting visual analysis in single-case research with hierarchical linear modeling. Behav. Modif. 37, 62–89. doi: 10.1177/0145445512453734
Dowdy, A., Hantula, D. A., Travers, J. C., and Tincani, M. (2022). Meta-analytic methods to detect publication bias in behavior science research. Perspect. Behav. Sci. 45, 37–52. doi: 10.1007/s40614-021-00303-0
Dugard, P., File, P., and Todman, J. (2012). Single-Case and Small-n Experimental Designs: A Practical Guide to Randomization Tests, 2nd Edn. New York, NY: Routledge.
Ferron, J. (2002). Reconsidering the use of the general linear model with single-case data. Behav. Res. Methods Instrum. Comput. 34, 324–331. doi: 10.3758/BF03195459
Harrison, J. R., Soares, D. A., Rudzinski, S., and Johnson, R. (2019). Attention deficit hyperactivity disorders and classroom-based interventions: evidence-based status, effectiveness, and moderators of effects in single-case design research. Rev. Educ. Res. 89, 569–611. doi: 10.3102/0034654319857038
Heyvaert, M., and Onghena, P. (2014). Randomization tests for single-case experiments: state of the art, state of the science, and state of the application. J. Contextual Behav. Sci. 3, 51–64. doi: 10.1016/j.jcbs.2013.10.002
Hintze, J. M., Volpe, R. J., and Shapiro, E. S. (2002). Direct Observation of Student Behavior. Best Pract. School Psychol. 4, 993–1006.
Hitchcock, J. H., Horner, R. H., Kratochwill, T. R., Levin, J. R., Odom, S. L., Rindskopf, D. M., et al. (2014). The what works clearinghouse single-case design pilot standards: who will guard the guards? Remedial Spec. Educ. 35, 145–152.
Huitema, B. E. (1986). “Statistical analysis and single-subject designs,” in Research Methods in Applied Behavior Analysis: Issues and Advances, eds A. Poling and R. W. Fuqua (New York, NY: Plenum), 209–232.
Huitema, B. E., and Mckean, J. W. (2000). Design specification issues in time-series intervention models. Educ. Psychol. Meas. 60, 38–58. doi: 10.1177/00131640021970358
Jones, R. R., Weinrott, M. R., and Vaught, R. S. (1978). Effects of serial dependency on the agreement between visual and statistical inference. J. Appl. Behav. Anal. 11, 277–283.
Keppel, G. (1982). Design and Analysis: A Researcher’s Handbook, 2nd Edn. Englewood Cliffs, NJ: Prentice Hall.
Klapproth, F. (2018). Biased predictions of students’ future achievement: an experimental study on pre-service teachers’ interpretation of curriculum-based measurement graphs. Stud. Educ. Eval. 59, 67–75. doi: 10.1016/j.stueduc.2018.03.004
Kratochwill, T. R., Hitchcock, J., Horner, R. H., Levin, J. R., Odom, S. L., Rindskopf, D. M., et al. (2010). Single-Case Designs Technical Documentation.
Kratochwill, T. R., Hitchcock, J. H., Horner, R. H., Levin, J. R., Odom, S. L., Rindskopf, D. M., et al. (2013). Single-case intervention research design standards. Remedial Spec. Educ. 34, 26–38. doi: 10.1177/0741932512452794
Lane, J. D., and Ledford, J. R. (2014). Using interval-based systems to measure behavior in early childhood special education and early intervention. Top. Early Child. Special Educ. 34, 83–93. doi: 10.1177/0271121414524063
Ledford, J. R., Lane, J. D., and Gast, D. L. (2018). Dependent Variables, Measurement, and Reliability: Single Case Research Methodology. New York, NY: Routledge.
Maggin, D. M., Briesch, A. M., and Chafouleas, S. M. (2013). An application of the what works clearinghouse standards for evaluating single-subject research: synthesis of the self-management literature base. Remedial Spec. Educ. 34, 44–58. doi: 10.1177/0741932511435176
Matyas, T. A., and Greenwood, K. M. (1990). Visual analysis of single-case time series: effects of variability, serial dependence, and magnitude of intervention effects. J. Appl. Behav. Anal. 23, 341–351. doi: 10.1901/jaba.1990.23-341
Moeyaert, M., Ferron, J. M., Beretvas, S. N., and Van den Noortgate, W. (2014). From a single-level analysis to a multilevel analysis of single-case experimental designs. J. Sch. Psychol. 52, 191–211. doi: 10.1016/j.jsp.2013.11.003
Moeyaert, M., Klingbeil, D. A., Rodabaugh, E., and Turan, M. (2021). Three-level meta-analysis of single-case data regarding the effects of peer tutoring on academic and social-behavioral outcomes for at-risk students and students with disabilities. Remedial Spec. Educ. 42, 94–106. doi: 10.1177/0741932519855079
Nock, M. K., Michel, B. D., Photos, V. I., and McKay, D. (2007). “Single-case research designs,” in Handbook of Research Methods in Abnormal and Clinical Psychology, ed. D. McKay (Thousand Oaks, CA: Sage Publications), 337–350.
Parker, R. I., and Brossart, D. F. (2003). Evaluating single-case research data: a comparison of seven statistical methods. Behav. Ther. 34, 189–203.
Parker, R. I., and Vannest, K. J. (2012). Bottom-up analysis of single-case research designs. J. Behav. Educ. 21, 254–265. doi: 10.1007/s10864-012-9153-1
Pustejovsky, J. E. (2018). Using response ratios for meta-analyzing single-case designs with behavioral outcomes. J. School Psychol. 68, 99–112. doi: 10.1016/j.jsp.2018.02.003
Pustejovsky, J. E., Swan, D. M., and English, K. W. (2019). An examination of measurement procedures and characteristics of baseline outcome data in single-case research. Behav. Modif. [Epub ahead of print]. doi: 10.1177/0145445519864264
Shadish, W. R., Hedges, L. V., Horner, R. H., and Odom, S. L. (2015). The Role of Between-Case Effect Size in Conducting, Interpreting, and Summarizing Single-Case Research. Washington, DC: National Center for Education Research.
Shadish, W. R., Hedges, L. V., and Pustejovsky, J. E. (2014). Analysis and meta-analysis of single-case designs with a standardized mean difference statistic: a primer and applications. J. Sch. Psychol. 52, 123–147. doi: 10.1016/j.jsp.2013.11.005
Shadish, W. R., Kyse, E. N., and Rindskopf, D. M. (2013). Analyzing data from single-case designs using multilevel models: new applications and some agenda items for future research. Psychol. Methods 18, 385–405. doi: 10.1037/a0032964
Shadish, W. R., and Sullivan, K. J. (2011). Characteristics of single-case designs used to assess intervention effects in 2008. Behav. Res. Methods 43, 971–980. doi: 10.3758/s13428-011-0111-y
Smith, J. D. (2012). Single-case experimental designs: a systematic review of published research and current standards. Psychol. Methods 17, 510–550. doi: 10.1037/a0029312
Soares, D. A., Harrison, J. R., Vannest, K. J., and McClelland, S. S. (2016). Effect size for token economy use in contemporary classroom settings: a meta-analysis of single-case research. Sch. Psychol. Rev. 45, 379–399. doi: 10.17105/spr45-4.379-399
Travers, J. C., Cook, B. G., Therrien, W. J., and Coyne, M. D. (2016). Replication research and special education. Remedial Spec. Educ. 37, 195–204. doi: 10.1177/0741932516648462
Vannest, K. J., and Sallese, M. R. (2021). Benchmarking effect sizes in single-case experimental designs. Evid. Based Commun. Assess. Interv. 15, 142–165. doi: 10.1080/17489539.2021.1886412
Wilbert, J. (2022). scplot: An R Package for Visualizing Single-Case Data. Potsdam: University of Potsdam.
Wilbert, J., Bosch, J., and Lüke, T. (2021). Validity and judgement bias in visual analysis of single-case data. Int. J. Res. Learn. Disabil. 5, 13–24. doi: 10.28987/ijrld.5.1.13
Wilbert, J., and Lüke, T. (2022). Scan: Single-Case Data Analyses for Single and Multiple Baseline Designs. Potsdam: University of Potsdam.
Keywords: single-case design, single case analysis, Monte-Carlo simulation, behavior problems, special education, research design, single-case experimental design
Citation: Wilbert J, Börnert-Ringleb M and Lüke T (2022) Statistical Power of Piecewise Regression Analyses of Single-Case Experimental Studies Addressing Behavior Problems. Front. Educ. 7:917944. doi: 10.3389/feduc.2022.917944
Received: 11 April 2022; Accepted: 20 June 2022;
Published: 06 July 2022.
Edited by:
Yvonne Blumenthal, University of Rostock, GermanyReviewed by:
Mack Burke, Baylor University, United StatesCopyright © 2022 Wilbert, Börnert-Ringleb and Lüke. This is an open-access article distributed under the terms of the Creative Commons Attribution License (CC BY). The use, distribution or reproduction in other forums is permitted, provided the original author(s) and the copyright owner(s) are credited and that the original publication in this journal is cited, in accordance with accepted academic practice. No use, distribution or reproduction is permitted which does not comply with these terms.
*Correspondence: Jürgen Wilbert, anVlcmdlbi53aWxiZXJ0QHVuaS1wb3RzZGFtLmRl
†ORCID: Jürgen Wilbert, orcid.org/0000-0002-8392-2873; Moritz Börnert-Ringleb, orcid.org/0000-0003-3533-0993; Timo Lüke, orcid.org/0000-0002-2603-7341
Disclaimer: All claims expressed in this article are solely those of the authors and do not necessarily represent those of their affiliated organizations, or those of the publisher, the editors and the reviewers. Any product that may be evaluated in this article or claim that may be made by its manufacturer is not guaranteed or endorsed by the publisher.
Research integrity at Frontiers
Learn more about the work of our research integrity team to safeguard the quality of each article we publish.