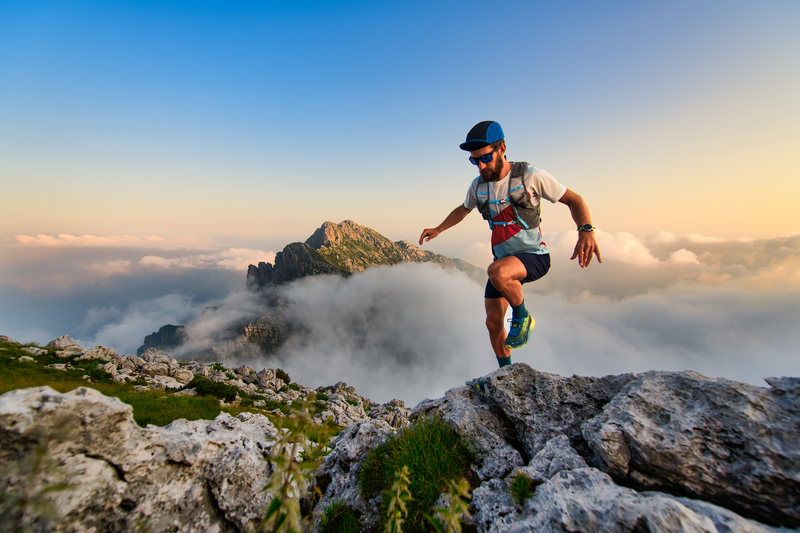
94% of researchers rate our articles as excellent or good
Learn more about the work of our research integrity team to safeguard the quality of each article we publish.
Find out more
HYPOTHESIS AND THEORY article
Front. Educ. , 06 July 2022
Sec. Digital Education
Volume 7 - 2022 | https://doi.org/10.3389/feduc.2022.906195
This article is part of the Research Topic Insights in Digital Education: 2022 View all 9 articles
The educational implications of COVID-19 have shaken both practitioners and researchers alike. Practitioners were expected to use technologies instantaneously, and this set up trauma in individuals. The ramification of understanding people’s response to why technologies are accepted/not accepted and used/not used has significant implications for education. This conceptual paper sets out the process used to develop a theoretical framework based on the technology acceptance model (TAM). The constructs within the original TAM and extended TAM, were explored to understand “why” practicing teachers would choose to use technologies for educational purposes at primary and secondary school levels during the COVID-19 pandemic period. The TAM has been criticized for being simplistic and narrowly focused. Many researchers criticize TAM because their finding cannot be confirmed or that the constructs don’t fit their needs. This paper challenges these critiques. The theoretical framework suggested in this paper represents a view of reality of the relational and influencing effects of variables that potentially moderate or control affective and cognitive responses. It contributes to the existing literature through a comprehensive reviewing of concepts, constructs and COVID-19 “event” contextual realities. The findings offered are that: contextual realities and application often require a grounded theoretical framework to unravel complex questions and answers; the suggested unidirectional influence of perceived ease of use (PEOU) on perceived usefulness (PU) can be challenged through a dispositional rationale; neglecting non-use as a reality severely hampers TAMs applicability in studies focused on theory testing, and TAM provides sufficient flexibility by leaving the doors open for adaptation, and this flexibility is an asset in social science research.
Research agendas are generally informed by examples such as, current events and difficulties (COVID-19; non-use of technology), political imperatives and redress (decolonization; rural educational access) and educational needs (enhancing learning and teaching; twenty-first century skills). Focused research into technology adoption, in an educational context, has once more reared its head in a somewhat forced situation owing to the recent COVID-19 pandemic (2020…). The use of educational technologies for teaching and learning is not pervasive in all social and educational context. The COVID-19 pandemic period thus challenges the relationship between teaching and educational technologies in the pre-and-during COVID-19 period. In this regard, governments and institutional COVID-19 policies forced many educational practitioners to provide emergency online remote teaching and variations of hybrid methodologies. This then fundamentally implied the use of technologies for teaching and learning. Furthermore, the relationship between technologies and teaching during this period suggested an rapid change in practices, which in many cases may not have been the familiar practices of teachers, especially those at primary and secondary schools.
Technological developments and people’s needs and wants can be located as “events” in time. Many previous “events” through history significantly changed the world and its people. For example: Bubonic plague (fourteenth century); Spanish flu (1918–1920); Asian bird flu (H5N1: 1959–1991) and other events which include the industrial revolutions’ age of mechanization (eighteenth to mid-nineteenth century); two major world wars; the silicon revolution (1947s onward); the age of electricity and the development of sea, air, road, rail travel, etc. COVID-19 has become one of these “events” and this paper adopts the concept of “events” to typify the COVID-19 context. The implication of the COVID-19 event’s, policy compulsion in educational context, suggests that there would be changes in behavior regarding technologies adoption and use. Some of these changes could be instantaneous and some temporal, while some could become permanent and some provisional. Behavioral changes would consequently be based on personal choices for basic survival and sophisticated needs for self and learners.
Studies on technology adoption, have since the late 1800s been guided in many instances by the technology adoption model (TAM) and its derivatives. It has been used in various sectors such as education, information systems, agriculture, health, e-Commerce, financial services and, also to examine aspects such as attitudes to technology use, technology use for specific subjects, mobile technology use, and social networking service use. Some research example included:
• Davis (1993) looked at the acceptance of IT in the workplace; Fariza et al. (2021): evaluated teachers’ views on the use of learning technologies in mathematics lessons in preschool and primary schools.
• Jarvenpaa and Staples (2000) and Dasgupta et al. (2002) adapted TAM for technology adoption in a Web 2.0 environments; Sánchez-Franco (2009) Enhanced TAM model with the effect of perceived affective quality; Hossain and de Silva (2009) Extended TAM by considering social ties for understanding social networking systems; Cheung and Vogel (2013) Extended TAM to explain the factors that influence the acceptance of applications for collaborative learning; Pituch and Lee (2006) Added system and learner characteristics as external variables to TAM; Diop et al. (2019) Extended TAM for understanding travelers’ adoption of variable message signs.
• Gong et al. (2004) and Sánchez-Franco (2009) Developed theoretical models to understand behaviors associated with adoption of learning technologies among students; Lee et al. (2005) Integrated TAM with motivational theory; Liu et al. (2005) Used flow theory with TAM to understand systems learning.
• Sayel and Rahman (2003); Porter and Donthu (2006), Li and Kirkup (2007) and Edmunds et al. (2012) explored internet use in universities; Sánchez and Hueros (2010) looked at the acceptance of Moodle; Fathema et al. (2015) Expanded TAM to examine faculty use of LMSs in higher education institutions.
• Tsai (2015): applied TAM to explore the effects of a CMS writing instruction; Ajibade (2018) explored the Limitations of TAM in Practical Applications and Use in Technology-related Studies; Wannapiroon et al. (2021) Explored Technology acceptance of online instruction for vocational instructors in new normal education.
Since its inception TAM has been both lauded and criticized. Many of the critics note that TAM is overly simplistic and takes a narrow perspective which focuses only on individual adopters’ beliefs, perceptions, and usage intention. This paper is not a criticism of TAM per say, but rather a deeper exploration of implicit adoption decisions that could explain underlying decisions and behaviors in contextually different situations.
As a theoretical desktop study using secondary sources, this paper employed a grounded approach, taking a broad-based view of TAM, theories underpinning TAM, and subsequent iterations of TAM, toward understanding individual behavior related to technology adoption/non-adoption and use/non-use. The focus of the paper asks the “WHY” question. According to Sadeck (2016, p. 222) “WHY” is a complex question and…complex questions provide complex answers.” Bagozzi (2007, p. 244) noted earlier that “…little theoretical insight is provided into the mechanism, or “the why,” behind proposed interaction effects.” The aim of this paper is to demystify complex answers free of academic rhetoric toward a simple way of understanding the complexities of technology adoption/non-adoption and use/non-use in an educational context.
In the context of this paper technologies (available to primary and secondary schools) is regarded as a plurality and is taken to represent:
• Systems and services such as collective social networking services (SNS); video conferencing facilities; learning management systems (LMS); etc. and,
• Applications/software such as videos; animations; simulations; audio clips; gaming apps; virtual reality; etc. and,
• Physical hardware devices such as computers, tablets, mobile phones, data projectors, interactive white boards, etc.
Two broad research foci (see Figure 1) include theory testing (test/explore TAM constructs to confirm or deny the applicability of all or some of its core constructs in the context of a study), or theory generation (to “understand” technology adoption/non-adoption through TAM as a reference framework). The response in this paper is through a focus on theory generation: WHAT the ultimate reason for selecting and using technologies might be, HOW are the most appropriate technologies choices made and, WHY would educational practitioners respond in particular ways in particular educational contexts. Three interrelated elements: technological affordances; psychological dispositions and pedagogical reasoning constitute the focal lenses. The following section will present a background on TAM, its developments and criticism.
This section will present briefly a broad-based synthesis of the following: Theory of Reasonable Action (TRA; Fishbein and Ajzen, 1975); Theory of Planned Behavior (TPB; Ajzen, 1985, 1991); the original and extended Technology Acceptance Model (TAM; Davis, 1986; Davis et al., 1989), Technology Acceptance Model 2 (TAM2; Venkatesh and Davis, 2000); Unified Theory of Acceptance and Use of Technology (UTAUT; Venkatesh et al., 2003) and, the Technology Acceptance Model 3 (TAM3; Venkatesh and Bala, 2008).
Fred Davis who conceived the original Technology Acceptance Model (TAM: developed 1985—published 1986, see Figure 2) stated two objectives: First “it should improve our understanding of user acceptance processes, providing new theoretical insights into the successful design and implementation of information systems. Second, TAM should provide the theoretical basis for a practical “user acceptance testing” methodology” (Davis, 1986, p. 7). Aligned with these objectives, Meerza (2017, p. 52471) explains it as “to determine the likelihood of end users adopting a particular technology…understanding of the internal and external factors influencing specific groups of users to either adopt or abandon specific technologies.”
Figure 2. Original technology acceptance model (Davis, 1985, p. 24).
An analysis shows that two specific belief constructs, i.e., Perceived Usefulness (PU) and Perceived Ease of Use (PEU) are the cornerstone determinants of TAM and its subsequent iterations. The logic presented (see Figure 2) is that “cognitive response factors (PU and PEU)…“impact the attitude toward using, as determinants of affective response (attitude toward using), which leads to behavioral response actual system use”(Go et al., 2020, p. 629). In the original TAM, design features (X1, X2, and X3), are suggested to directly influence PU and PEU. Both PU and PEU are located squarely as a cognitive process.
The TAM is widely acknowledged as an expansion and leveraging of two models from the field of psychology. These are the Theory of Reasoned Action (TRA—Fishbein and Ajzen, 1975) and the Theory of Planned Behavior (TPB; Ajzen, 1985, 1991). At the core of TRA, attitudes (A) toward a behavior together with subjective norms (SN) are used to explain how attitudes influence behavior (toward an anticipated outcome (Castiblanco Jimenez et al., 2021, p. 3). The Theory of Planned Behavior is a derivative of TRA and adds a third concept, i.e., Perceived Behavioral Control (PCB), which according to Ajzen (1991, p. 183) refers to people’s perception of the ease, difficulty, or capability to perform behaviors. Lai (2017) refers to this as “the control which users perceive that may limit their behavior.” Thus, TPB suggest that subjective norms, with attitudes, alongside PBC directly determine user’s intentions which then influence behavior. Castiblanco Jimenez et al. (2021, p. 3) speaks of these as “two complementary theories formulate that there is a sequence of causal relationships among what individuals believe about using a system and the actual use.” Davis (1986, p. 26) noted that “the subjective norm and behavioral intention variables, although central elements of the Fishbein paradigm, are omitted from the present (Davis, 1986) model.”
Two adaptations are noted in the extended TAM (Figure 3). Behavioral intention (BI) was reintroduced from TRA (excluded in Davis, 1986 TAM) “as a new variable to mediate the relationship, wherein BI was considered part of the attitude factor” (Meerza, 2017, p. 52472). Davis et al. (1989, p. 985) state that “…BI is viewed as being jointly determined by the person’s attitude toward using the system (A [attitude]) and perceived usefulness (U [PU]).” Aligned with the notion of “affective response” (see Figure 2), Go et al. (2020, p. 630) notes that “a person’s behavior can be determined by the person’s behavioral intention to execute the behavior, based on the theory reasoned action.”
Figure 3. Extended TAM (Davis et al., 1989, p. 985).
The second adaption is the substitution of the design features (X1, X2, and X3; see Figure 2) with “external variables” (see Figure 3). The extended TAM excludes the construct of TRA’s “subjective norm (SN) as a determinant of BI” (Davis et al., 1989, p. 986). According to Davis et al. (1989, p. 985) “A key purpose of TAM, therefore, is to provide a basis for tracing the impact of external factors on internal beliefs, attitudes, and intentions.” Meerza (2017, p. 52472) echoes Davis et al. (1989) when she states “…depend upon an analysis of internal factors, as impacted by external or mediating factors, in order to determine attitudes and outcomes.”
However, another version of TAM emerged in 1996 by Venkatesh and Davis (see Figure 4). This emerged “after the main finding of both perceived usefulness (PU) and perceived ease of use (PEOU) were found to have a direct influence on behavior intention (BI), thus eliminating the need for the attitude (A) construct” (Lai, 2017).
Figure 4. Technology Acceptance Model (TAM) (Venkatesh and Davis, 1996).
Following the 1986–1996 period of TAM revisions, we experienced an 8 year span of 3 further modifications to TAM (2000-TAM2, 2003-UTAUT and 2008-TAM3).
The TAM2 (Venkatesh and Davis, 2000) is a response to criticism that TAM did have significant limitations for explaining the reasons why an individual would perceive a given system as useful, as the focus was primarily on PU only. Meerza (2017, p. 52473) noted that a range of additional external variables were presented in TAM 2 that “drew direct links between these and the PU factor; [importantly] ignoring any possible effect of these external variables on the PEOU factor.” An analysis showed how 7 additional constructs were added. These are 5 constructs to mediate PU, and a further 2 constructs to mediate BI through subjective norms.
The UTAUT (Venkatesh et al., 2003) response was a move away from the cognitive domain to concentrate on the affective aspects of technology adoption and use. The focus in this iteration of TAM was on BI and use behavior (Shachak et al., 2019, p. 1; Go et al., 2020, p. 630). In this model a further 8 constructs were added. Three constructs: social influence, performance expectancy and effort expectancy were added to mediate BI and, 1 construct: facilitation conditions to further mediate use behavior and, 4 control determinants: gender, experience, age and voluntariness of use to assist mediating the effects of the entire process (Shachak et al., 2019).
In contrast with TAM 2, the TAM3 (Venkatesh and Bala, 2008), responded to the impact of antecedent factors on the PEOU variable. In effect TAM 3 further delved into atomized possible influential variables (on PEOU) to explain the “external variables.” Go et al. (2020, p. 630) reports that TAM 3 uses 6 additional factors to measure PEOU: perceived enjoyment, computer playfulness, computer anxiety, objective usability, computer self-efficacy, and user control.
So, the “simple” TAM model comprising 6 core constructs (Figure 3; EV; PU; PEOU; A; BI; U) has over a period of time exploded to 11 constructs in TAM2, 14 constructs in UTAUT and, 12 constructs in TAM3—It’s no wonder researchers are at sevens with TAM. According to Benbasat and Barki (2007, p. 211) “The independent attempts by several researchers to expand TAM in order to adapt it to constantly changing IT environments have led to a state of theoretical chaos and confusion in which it is not clear which version of the many iterations of TAM is the commonly accepted one.” Needless to say, the versions of TAM in a 10-year span (1986–1996) have left not only left novice researchers, but seasoned researchers, somewhat bewildered. This has given rise to much criticism of TAM, which will be discussed in the next section.
Researchers using TAM have a propensity to use the extended TAM (Davis et al., 1989 version) as opposed to the original TAM (Davis, 1986), which excludes BI and external variables, and the later Venkatesh and Davis (1996) version which excludes BI. Fewer researchers appear opt for TAM2, UTAUT and TAM3. Aligned with King and He’s (2006, p. 741) categories of modification, Castiblanco Jimenez et al. (2021, p. 3) suggested that the research foci determine the version of TAM leveraged. The foci could be on one or both of the following: A technology-centric cognitive focus through factors predicting PU and PEOU, or, focus on external variables potential moderating or controlling effect, such as contextual factors and focus on behavioral response based on affective and cognitive responses. This paper responds to the second focus.
Models and frameworks are embodiments of underlying theories, and the TAM is backed by physiological theories. Conspicuous by their absence and/or their exclusion and reinstatement in the different versions are the constructs of “attitude” (A) and “behavioral intent” (BI). Technology adoption is inherently a human activity and processes that inform decision-making cannot be left out of any adoption model even if it is considered to “partially” mediate. The 6 TAM core constructs are inherently sound to explore and understand technology adoption, all be it that I set them out in an atomized and non-academic manner hereunder.
The “EXTERNAL VARIABLES” are those aspects that are not technology centric. The “PEOU” and “PU” constructs are contingent on the technologies being interrogated in terms of navigation, operation, etc. and the specific attributes of the technologies. The “ATTITUDE” and “BEHAVIORAL INTENT” constructs are linked to decisions to consider using/not using and these are attitudinal decisions and are characteristically human decisions informed by cognitive factors. The “USE” construct is a suggested end point as a culmination of the preceding constructs.
The TAM offers a particular view of reality, and this is noted by Goodhue (2007) as a consideration of relationships among important factors of technology adoption, “while simultaneously blurring others” (Goodhue, 2007, p. 220). He further states that “TAM has left us with some significant blind spots,” and is furthermore “limited in the questions it poses, such as: What causes users to utilize a technology?” This question is similar to and aligned with the “why” people choose to use technology question which is the focus of this paper. These and other statements offering critiques of TAM are relatively broad and in themselves do not offer insights into the issues of applicability, validity, and reliability of the TAM constructs. For example:
Chao (2019, p. 2) note the disadvantages of TAM as: “not providing adequate insight into individuals’ perspectives of novel systems; neglecting its indicators and directly investigating the external variables of perceived ease of use (PEOU) and perceived usefulness (PU); and ignoring the relationship between usage attitude and usage intention.” Furthermore Shachak et al. (2019, p. 1) reports that the criticism of TAM and UTAUT is that it is “overly simplistic…taking a narrow perspective, which focuses only on individual adopters’ beliefs, perceptions and usage intention,” suggesting that TAM is relegated to the use of “three constructs only: perceived usefulness, perceived ease of use, and usage intention.” The critique thus leveled is the lack of insight, into technology adoption and use, through little explanatory detail.
According to Shachak et al. (2019, p. 2) this could be on account of the fact that “they adopt a social psychology view, which focuses on the individual adopter and assumes a direct causal influence of most of the predictors of use as mediated through usage intention.” In other words, the BI, which is not in the original TAM, and then conveniently omitted in other iterations. The influence of BI on actual use could be contentious. Sadeck (2016, p. 79) stated that “A teacher could also have an unfavorable disposition, and this could result in non-adoption. However, a teacher may still engage in an action even if he/she holds an unfavorable attitude toward it. [Furthermore], the use of ICT may be mandatory, and the teacher must use it whether he/she likes it or not. While attitude is a determinant of intent (to use or not to use), its application as a predictor of a teacher’s action is not considered to be reliable across all contexts.” Interestingly Shachak et al. (2019, p. 2) provides a novel critique when they note that their “main criticism of TAM and UTAUT is that their contribution to our current knowledge has reached a plateau.”
Why then do novice and experiences researchers choose to use TAM or its extensions? King and He (2006) suggest that it is due to the simplicity of the framework and its capacity to elucidate individual behavior in relation to technology, that TAM and its extensions have been widely used in ICT research. The original/extended TAM, without additional constructs is considered stable for research into technology adoption and use. Meerza (2017, p. 52473) notes that there is “significant applicability and validity attributed to TAM in most experienced research in education.” Furthermore, Chuttur (2009, p. 17) concludes that “future research will focus in developing new models that will exploit the strengths of the TAM model while discarding its weaknesses.” The ensuing iterations of TAM have, over time, in alignment with Chuttur’s statement above, added and/or omitted mediating constructs which could be significant to progress our understanding of an individual’s technology adoption/non-adoption and use/non-use. In this regard, Bagozzi contends that “consideration of moderating variables is one way of deepening any model, but introductions of these should be grounded in theory…” (Bagozzi, 2007, p. 244).
This paper examines the “adoption/non-adoption and use/non-use” of technologies in the context of the COVID-19 period. The focus was to understand to what extent, technological affordances, disposition, and pedagogical reasoning at both primary and secondary school levels influence implementation (use/non-use). Following Wiggins and McTighe’s (2005) backward mapping this paper started with the endpoint, i.e., Technologies were being used/not-used during the COVID-19 period…so the question is why was this happening? The matter-of-fact reasoning that guided this examination is:
• that a teacher will use/not use any of the technologies based on there being a need, and that
• any of the technologies are suitable to address the need, and that
• their beliefs and attitudes predispose them to deciding to use/not use the technologies.
The approach taken was to examine the TAM constructs suggested in both the original and extended TAM as representations of the TAM theory.
• The external variable (E) was taken as the contextual influence, i.e., the COVID-19 compulsion, which gave rise to a NEED—the need represents the pedagogical reasoning.
• Perceived usefulness (PU) was taken to represent the technologies affordances.
• The attitude (A) construct was taken as the disposition.
The researchers’ matter-of-fact reasoning of “suitability of technologies” and “beliefs and attitudes” alongside “TAM constructs” is discussed under affordances and dispositions in this paper. In examining TAM toward progressing its applicability for this paper, existing theoretical works on affordance and cognitive information-processing were employed.
The following sections delve into pedagogical reasoning, technological affordances, and psychological dispositions.
The external variables (E) (see Figure 3) represent the NEED—What would the technologies be needed for in the context of this paper. COVID-19 restrictions necessitated a need for curriculum recovery and for teaching and learning to continue at both primary and secondary schools. This is taken to refer to a pedagogical need, premised on the interplay among teaching, learning and assessment. This interplay suggest teacher’s Pedagogical Content Knowledge (PCK) and more relevantly in the context of this paper teacher’s Technological Pedagogical and Content Knowledge (TPACK).
The teaching and learning environment incorporating technology integration comprises a wide range of technologically based pedagogical practices and technologies (Barr and Miller, 2013). According to Steele et al. (2019), pedagogical practices cover instructional strategies, interactions with technology, and content delivery methods, dynamically enabling improved learning experiences. There is thus a compelling need to deconstruct pedagogical needs in this paper, and this was explored through the nature of teaching and learning with technologies using Laurillard’s (2012) conversational framework (see Figure 5) designed to support teachers in designing online pedagogical practices).
Figure 5. Reconceptualization of Laurillard’s (2012) conversational framework.
Figure 5 shows, what Laurillard refers to as, the learner’s internal learning cycle where they learn and practice/apply concepts, that is they learn and do. The teacher’s role in the process is to stimulate and facilitating learning in this internal learning cycle. This cycle suggest that learners could be hearing, reading, observing, attending to explanations, watching demonstrations, etc. This learning cycle will be explored in the section on learning events.
The teacher’s role in this framework requires three elements, which are communication, practice, and modeling. Simplistically the communication element is achieved by the teacher explaining concepts, provoking questions, providing feedback, etc. (Laurillard, 2012, p. 87). The practice element focuses on the teacher providing an environment for learners to generate actions, i.e., to practice and apply learnings, again with continued feedback from the teacher (Laurillard, 2012, p. 89). The modeling environment is dependent on the way the teacher performs the learning cycle, i.e., the best methods to understand the concepts (Laurillard, 2012, p. 162–163). The three teacher elements influence and are mediators of technologies selection based on pedagogical reasoning of learning events.
A third component in the framework are the peer communication and collaborating elements represented as an external learning cycle. According to Laurillard (2012, p. 91), this is facilitated through the teacher’s role in encouraging meta-cognitive learning through exchange of ideas and practice through peer interaction. This is often articulated through individual students and the peers engaging in reflection, discussions or collaborating of issues. The communicative and collaborative aspects of meta-cognitive learning could be enabled through activities that are structured or [semi]structured and could be stand-alone or embedded within other methods. They could be either singularly or combined student-led, teacher-led and in small or large groups. The three components of the framework thus depict pedagogical activities. The next section delves into the nature of the activities toward an understanding of the technologies connections.
Both Laurillard (2002) and Leclercq and Poumay (2005) speak of events of learning. Learning events are representative of what a learner could be “doing” during learninging. Table 1 depicts congruence and deviations between the authors regarding learning events. This paper focuses on Laurillard’s learning events only.
Table 1. Pedagogical actions (based on Laurillard’s, 2002; Leclercq and Poumay’s, 2005 learning events).
The different learning events shown in Table 1 provide insights into the type of learner engagements in the learning cycle. Pedagogical decisions and reasoning are contingent on the concepts being studied and the best way to achieve learning is through an understanding of a particular/group of learning events through relevant activities for primary and secondary school learners. For example, if need is for learners to collaborative discuss a topic, or experiment with a scientific concept, etc., the teacher considers two questions, i.e., 1. How can the concept be best understood and learned? and, 2. Which technologies would be most appropriate to enable this learning experience? Similarly, if the learner is to experiment as the most appropriate way to learn about e.g., the Doppler Effect using technologies, then the teacher could decide to use a simulation. Addressing the pedagogical needs is based squarely on pedagogical decisions which encompasses attention to pedagogical practices and learning events. The next section links pedagogical reasoning to technologies through technological affordances.
Lee et al. (2014, in Shin, 2022, p. 2) refer to affordances as “the features of an object that indicate which actions a user can take and signify how they can interact with the object.” Affordances according to Anderson and Robey (2017, in Shin, 2022, p. 2) is a “trait or property of a technology that presents a cue to what can be done with an artifact. Shin (2021, in Shin, 2022, p. 2) provides insights from an interactive perspective, when he notes that “affordances operate as “prompts that provide input on how users can interact with it [technology].” The literature supports this paper’s conceptualized difference between affordance and attributes. The perceived usefulness construct (PU) (see Figures 2, 3) in this paper represents the TECHNOLOGICAL AFFORDANCES.
Engaging with this construct requires us to understand how any of the available technologies can satisfy the need. In other words what usefulness does it offer, i.e., not the attributes of the technologies, but specifically their affordances for the pedagogical reason identified by the teacher in the need. In the literature on affordances in Hwang and Shin (2020, p. 916) salient nuances of the concept of affordances are offered. These include “educational affordance (Shin, 2019), emotional affordance, technological affordance (Velasco, 2016) and motivational affordance (Jung et al., 2010), affective affordances (Zhao et al., 2013). The various lenses on affordances are aptly summed up as having to be “properly perceived for the user to recognize the potential for action (Jung et al., 2010).
The distinction that this paper makes between attributes and affordances is best understood through paradigmatic dispositions which all technologies possess. Alvarez (2017, p. 5) explains paradigmatic dispositions as “a property of an object, defined by its manifestation but ontologically independent of its ever being manifested.” For example, a Learning Management System (LMS e.g., Moodle) possess attributes to enable online learning through media tools. As a technology the LMS’s attributes are dormant until leveraged for a purpose. In the context of this paper, there is a need for a stimulus conditions (the pedagogical need (E) to trigger the LMS attributes to manifest as action. The attribute (paradigmatic disposition) become active (manifest) when applied for learning activities, thus changing inherent potential into affordances.
The affordances (PU) assume five different media a form, which Laurillard (2002) identifies as: narrative, interactive, communicative, adaptive, and productive. Drawing on Conole and Fill’s (2005) narrative on these media types we can understand them as:
1. Narrative media such as text, image, etc. show the learner something.
2. Search engines, quizzes, simple models some examples are of Interactive media which afford limited interaction for learners.
3. Communicative media facilitate interactions between and among learners and teachers through technologies such as, discuss forums, online chats, email.
4. Greater interaction and engagement in learning can be found in Adaptive media in technologies such as simulations, virtual worlds.
5. Productive media provide opportunities for learners to generatively produce something when using technologies such as word processors, graphics programs, and spreadsheet.
Laurillard (2002) argues that different media forms [technology attributes] have different affordances as they provide different levels of support for diverse learning experiences. Table 2 shows Laurillard’s (2002) depiction of learning events with corresponding technologies affordances. The purpose of this is to link the learning activity to technologies.
Table 2. Technological affordances for pedagogical needs (based on Laurillard’s (2002) learning events).
For example, in the pedagogical need of “practice,” where the learner needs to experience a concept to understand how results are influenced by variables, the teacher considers a range of technologies (different attributes) that best allow for learning to take place. The teacher selects simulations, because it allows for true interactivity and authentic engagement with variables in the concept (individual learning) and combines this with a discussion forum for learners to discuss their discoveries with peers (collaborative learning) (leveraging the affordances of these two technologies). The teacher could have elected to use only one of the technologies or selected different technologies such as a video demonstration or a narrated presentation.
The choices made are based on a learning need and not on any of the inherent attributes of the technologies, but more on what how it will enable the learning (the affordances). This paper offers that the selection and use of technologies is implicit through pedagogical needs and design and, in this context, not attributes of technologies, but rather their relevant affordances toward achieving the pedagogical need through delivery. The strong direct relationship between E and PU in the context of this paper is argued as the usefulness in practice is contingent on the need.
People’s decisions are located in the psychological domain and are informed by cognitive stimuli. Having established the NEED (pedagogical) and the TECHNOLOGIES (affordances) for teaching and learning in the previous sections, we turn to the teacher as the human element in TAM. The focus of the approach is to understand and explain why something happened/is happening through people’s disposition (dispositional properties, Alvarez, 2017, p. 1). This is examined primarily through TAM’s attitude (A), the perceived ease of use (PEOU) and behavioral intent (BI) constructs.
According to Alvarez (2017, p. 1), “human actions, especially intentional actions [and] aims and goals…are generally thought of as dispositions” [and] “are often explained by citing psychological factors.” A psychological disposition may be understood as a person being disposed to act in a particular way based on his/her beliefs and needs/wants. For example, a person’s aim of wanting to achieve a goal speedily, disposes such a person to use selected technologies that he/she thinks are beneficial for getting a task done quickly. Thus, one might consider that the person’s attitude (A) to the technologies favors it affordance to enable speedy outcomes. Castiblanco Jimenez et al. (2021, p. 3) similarly, when reporting on how attitudes influence behavior, leverage TRA to explain how a user’s intention (BI) is a natural flow of the relationship between a person’s attitudes (A) and subjective norms (SN) toward an outcome. This rationale is supported by Shin (2021, p. 8) who notes that “multiple emotions make up attitudes.” He (Shin, 2021, p. 8) further notes that when users see inherent “value of a system [technologies],” their emotional outlook “becomes more positive,” given that dispositions are contingent on human emotions.
Alvarez (2017, p. 6) speaks of understanding dispositions as a multi-track, i.e., “a disposition to certain “inner” phenomena such as thinking, judging, reasoning, desiring and feeling in certain ways” and, “a disposition to engage in a variety of “overt” [or covert] behavior.” The interrelations between the two tracks (attitude/intent and behavior/action) become obvious to us through their manifestations. However, such manifestations are contingent on certain stimulus conditions. A strong stimulus is often the push-pull/cause-effect/if-then relationship between needs/wants (external variable (E) and technologies affordances (PU), that possess a power for an attitude and a behavior. The determination of attitudes and outcomes is an interplay reported as a dependency of “an analysis of internal factors, as impacted by external or mediating factors, in order to determine attitudes and outcomes” (Meerza, 2017, p. 52472).
The critique in literature of the relationship between attitude (A) and behavioral intent (BI) culminating in use (U) may be viewed through a dispositional lens. A person may possess the capabilities to use technologies to complete tasks but does not have any disposition to do so for a variety of reasons. The necessary self-efficacy and experience (often aligned with the PEOU construct) could be inherent to the person’s disposition, but this does not manifest in action. We may accordingly consider the debate on the linear progression of TAM differently. The attitude (A) a person holds as a result of cognitive reasoning (based on the technologies affordances to potentially progress toward achieving a goal, coupled with his/her beliefs of PEOU and personal self-efficacy regarding the technologies), manifests firstly as behavioral intent (BI), and secondly in covert or overt use/non-use behavior.
Consequently, we find a range of dispositions on a spectrum, with people located at different points. It is also conceivable that a person will use technologies even though they are not technologically inclined and conversely, we could see a technologically savvy person elect not to use any technologies. People’s dispositions are innate and not immutable. Given this and in the context of this paper, the dispositional outcomes may be temporal and is subject to change should the external variable or need change. In a post-COVID-19 period, there is a chance that teachers may revert to non-technology integrated teaching and learning, irrespective of the technologies affordances (PU) their perceived ease of use/their self-efficacy (PEOU), favorable attitudes (A) and their behavioral intent to use (BI).
While in agreement with Shachak et al. (2019, p. 1) that in “many studies, the model [TAM] is reduced to three constructs only: perceived usefulness [PU], perceived ease of use [PEOU], and usage intention [BI], which makes outcomes such as intended or perceived use become the endpoint rather than actual use of the technology,” this paper focuses on understanding the journey to the end point. It thus challenges Shachak et al. (2019, p. 1) contention that the focus on selected TAM constructs “lowers its explanatory power and provides little insight.”
Using an adaptation of Burrell and Morgan’s (1979, p. 24) concept of dimensional space, this paper mapped the relevant key TAM constructs into the quadrants of inquiry for this paper two-dimensionally. The key TAM constructs are:
• External Variables (E) (representing pedagogical NEED)
• Perceived Usefulness (PU) (representing technologies affordances)
• Attitude (A) (representing disposition)
• With Behavioral Intent (BI) (supporting Attitudes—toward satisfying the NEED)
• Perceived Ease of Use (PEOU) (supporting Perceived Usefulness toward satisfying the NEED)
In the original TAM, design features (X1, X2, and X3) and, external variables (extended TAM), are suggested to directly influence PU and PEOU. Both PU and PEOU are located squarely as a cognitive process which this paper acknowledges as useful for understanding what triggers decisions. We are drawn to the fact that there is an outcome on the horizon, and reminded that technology affordance progresses toward this outcome, when looking to understand any reasoning behind why a technology is considered “useful.” The PEOU relationship with PU is evidenced in Davis (1993, p. 477) who stated that “the one [technology] that is considered easier to use will be will be considered more useful.” Pintrich (2003, p. 673) later leveraged extrinsic motivation to argue that PU leans toward “the purpose of a result, external reason or instrumental value.”
Castiblanco Jimenez et al. (2021, p. 12) report that “A weaker but still significant influence of PEOU on PU was found in theoretical models and in cases-study evaluating the acceptance of a bundle of technologies.” Davis (1993,p. 477) contends that PU is not considered to have an impact on PEOU, because PU is about what the outcome will be of using the technology, and PEOU is the level of difficulty to use the system. This paper accepts that PU justifiably is about the outcome (through technologies affordances) and offers a different view on PEOU alongside the traditional notion of “level of difficulty.” Levels of difficulty are associated more with the attributes of a technology and to a lesser extent to its affordances. When PEOU is considered through its manifestation, it ought to be viewed relationally between affordance-action such as (1) ease of use for the teacher to achieve the outcome and (2) ease of use for the learners to learn the concept.
This paper further challenges the notion that PU is contingent on PEOU as suggested in TAM through a unidirectional cause-effect of PEOU on PU (see Figures 2, 3) and argues that it could be the other way around. The usefulness of technologies (PU) toward outcomes may not be considered “difficult to use”—the teacher might have the necessary self-efficacy beliefs, but still does not consider the technologies “easy” to use, because it is not easily applicable in the teaching/learning situation [its usefulness]. For example WhatsApp is considered easy to use as an application—WhatsApp could have the affordances when used to provide collaboration opportunities, but the teacher might note that the nature of the discussion should not be asynchronous. This can be supported from Hwang and Shin’s (2020, p. 916) statement that “Affordance is an interpretative connection between users and objects that occurs during the users’ interaction with technology in real contexts. From this viewpoint PEOU does not influence the inherent usefulness (PU) of the technologies. The decisions going forward are more influenced by, the pedagogical need and the technologies affordances, as opposed to PEOU. Theoretically, as in this paper, the suggestion is that technologies affordances (PU) exerts a significantly stronger influence on PEOU. Both PU and PEOU relationally exert influences on attitudes toward enabling a user to achieve the outcome (pedagogical needs). This logic is noted by Castiblanco Jimenez et al. (2021, p. 12) who confirmed that “the external variables have different effects on PU and PEOU.”
This paper views attitudes (A) and behavioral intent (BI) as dispositional elements in a symbiotic relationship. They are both directly influenced by the relational effects of the pedagogical need (E) and technological affordances/ease of use (PU/PEOU). In the extended TAM, BI is said to be influenced by attitudes (A) and furthermore suggested to be directly influenced by PU. Behavioral intent (BI) is often associated with use and seldom with non-use. This paper suggest that the close association between A and BI warrants consideration given a traditional acceptance that favorable attitudes may yield positive intentions to use, and unfavorable attitudes may yield negative intentions resulting in non-use.
The general acceptance that a favorable attitude results in positive use behavior is challenged by a favorable attitude that does not culminate in actual use behaviors. According to Sadeck (2016, p. 79) “a teacher may still engage in an action even if he/she holds an unfavorable attitude toward it.” Ajzen and Fishbein (2005, p. 20–21) offer that it is the “qualities of the attitude itself…that may moderate the strength of the attitude-[intent]-behavior relation.” In risking any direct claim that internal and environmental factors determine behavior from a social cognitive theory perspective this paper notes Bandura’s (1989) articulation that, “people are neither driven by inner forces nor automatically shaped and controlled by the environment…they function as contributors to their own motivation, behavior, and development within a network of reciprocally interacting influences (Bandura, 1989, p. 8). Hence the critique of Shachak et al. (2019, p. 1) that TAM “focuses only on individual adopters’ beliefs, perceptions and usage intention” is addressed jointly through three rationalities:
(1) Desired outcomes in COVID-19 context,
(2) Social cognition,
(3) The focus of this paper, i.e., The WHY behind individual behaviors.
The COVID-19 context reiterated an educational pedagogical need, and this need was driven by a compulsion to adopt and use technologies. The deep-set attitudes of teachers, toward technologies use, are thus tempered through the mandatory (policy like) expectation of educational authorities. That is that the teacher must use technologies whether he/she likes it or not. While attitude is a determinant of intent (to use/not use), its clinical application as a predictor of behavior is not unidirectional across all contexts. As individuals, teacher’s responses and practices would have evolve differently in response to the COVID-19 contextual imperatives. Teachers are more likely to alter their attitudes toward a more flexible adaptation of their traditional practices in teaching and learning situations. The desired outcome in the COVID-19 context is educational, and if teachers perceive gains toward this outcome, they will more likely be users and those who do not are less likely to be users of technologies (Gellerstedt et al., 2018, p. 14). This is aligned with (Leonardi, 2013; Hwang and Shin, 2020, p. 916) who notes that “affordances are thus properties of the environment that enable action on the part of those who perceive them. The technological affordances/ease of use (PU/PEOU) TAM constructs are thus related to the pedagogical need (E) in varying intencies of influence. This can be supported by the notion that “many teachers do not have any problems using the technology, but don’t use it anyway, since they lack pedagogical incentives” (Gellerstedt et al., 2018, p. 14).
The “network of reciprocally interacting influences” referred to by Bandura (1989, p. 8) influence behaviors. This network includes contextual factors, cognitive factors, societal norms and dispositions. In dispositional speak, teachers that are less technologically inclined are likely to “take risks” even though statistical research relegates such persons as less likely to adopt and use technologies. The contextual realities to “get on with it” combined with personal and educational needs influence deep-seated attitudes that spur teachers into actions that may not normally be in their repertoire.
Teachers are “helped” during these decision-making phases by advice and opinions within their social environments. In this regard Castiblanco Jimenez et al. (2021, p. 12) note that “in some cases, people may use a technology based on others’ perceptions about the convenience of the use over his or her own emotions and beliefs.” Institutional pressures resulting from governance (such as stipulations of which technologies must be used) coupled with social pressure of “tried/tested” technologies (I find Facebook and Zoom the best to get learners learning) are some examples of societal norms that a teacher is faced with. The constant push-pull of interacting influences means that the individual is repeatedly looking for and making decisions that may not necessarily native to him/her as a person. Social cognition in the context of this paper may be summed up as reported by Castiblanco Jimenez et al. (2021, p. 14) that, “social pressure can have a more significant influence on users’ perceptions of usefulness than in PEOU.”
This paper is not intended to generalize to large populations, but rather to what individual processes, logic and realities inform a person’s behavior. This is explored through understanding WHY an individual is likely or less likely to consider using or not using technologies at both primary and secondary school levels during the COVID-19 pandemic period.
With reference to Figure 6 (theoretical framework for this paper), Figure 2 (original TAM) and Figure 3 (extended TAM):
Figure 6. Theoretical framework for this paper (based on conceptual space for the analysis of social theory, Burrell and Morgan, 1979, p. 24).
Relational aspects in the cognitive domain. Incorporation of the joint influences of perceived usefulness (PU—technological affordances) and perceived ease of use (PEOU) on the external variables (E—pedagogical need).
Relational aspects in the cognitive/affective domains. Strengthens the linkage between attitudes (A—psychological dispositions) and perceived usefulness (PU—technological affordances) toward achieving the outcomes (E—pedagogical need).
Relational aspects in the affective/cognitive domains. Unites the relationship between attitudes (A—psychological dispositions) and intentions (BI—toward use/non-use behavior).
Relational aspects in the cognitive/affective/behavioral domains. This incorporates action/non-action in relation to outcomes. Ultimately representative of the actualization of BI cyclically linked back to the ultimate pedagogical needs (E).
The quadrants distilled into an adapted representation of TAM are set out in Figure 7 below.
The contextual problem driving this theoretical desktop study is the COVID-19 “event.” It sought to understand WHY teachers would consider using technologies for teaching and learning, through an exploration of the TAM. This paper contributes to the existing literature through the comprehensive reviewing of concepts, constructs, and contextual realities in a policy like compulsion to spring into action and use technologies brought about by the COVID-19 “event.” In line with various research/authors, this paper acknowledges the TAM constructs: E, PU, PEOU, A, BI and U as robust and capable for both understanding and predicting behavior in different contexts. This paper offers that the domain phases: affective, cognitive, and behavioral are relationally connected and, influences and contingencies among the constructs, play out in different intensities.
This paper concludes that:
1. Contextual realities and application often require a grounded theoretical framework to unravel complex questions and answers.
2. The traditionally accepted unidirectional influence of PEOU on PU can be challenged through a dispositional rationale.
3. Neglecting non-use as a reality severely hampers TAMs applicability in studies focused on theory testing.
4. The TAM provides sufficient flexibility by leaving the doors open for adaptation, and this flexibility is an asset in social science research.
So ‘Is use/non-use a case of technological affordances or psychological disposition or pedagogical reasoning?’ This paper suggests “YES to all three”—through a simple understanding of the complexities of the answer via logical exploration of influencing and relational links among different elements in specific contexts.
Two suggestions are offered:
1. Toward application of the suggested theoretical framework: research seeking to understand “WHY” something is happening should consider the nature of external variables to understand the context-dependency for technologies use.
2. Toward future technology adoption research: this theoretical model has not been tested empirically, and future qualitative and quantitative research studies could be undertaken to validate its rigor.
The original contributions presented in the study are included in this article/supplementary material, further inquiries can be directed to the corresponding author.
The author confirms being the sole contributor of this work and has approved it for publication.
The author declares that the research was conducted in the absence of any commercial or financial relationships that could be construed as a potential conflict of interest.
All claims expressed in this article are solely those of the authors and do not necessarily represent those of their affiliated organizations, or those of the publisher, the editors and the reviewers. Any product that may be evaluated in this article, or claim that may be made by its manufacturer, is not guaranteed or endorsed by the publisher.
Ajibade, P. (2018). Technology Acceptance Model Limitations and Criticisms: Exploring the Practical Applications and Use in Technology-related Studies, Mixed-method, and Qualitative Researches. Lincoln, NE: University of Nebraska-Lincoln.
Ajzen, I. (1985). “From Intentions to Actions: A Theory of Planned Behavior,” in Action Control. SSSP Springer Series in Social Psychology, eds J. Kuhl and J. Beckmann (Berlin: Springer), doi: 10.1007/978-3-642-69746-3_2
Ajzen, I. (1991). The Theory of Planned Behaviour. Organization Behaviour and Human Decision Processes. Cambridge, MA: Academic Press, Inc, 179–211. doi: 10.1016/0749-5978(91)90020-T
Ajzen, I., and Fishbein, M. (2005). “The Influence of Attitudes on Behaviour,” in The Handbook of Attitudes, eds D. Albarracín, B. T. Johnson, and M. P. Zanna (Mahwah: Lawrence Erlbaum Associates Publishers).
Alvarez, M. (2017). Are character traits dispositions? R. Inst. Philos. Suppl. 80, 69–86. doi: 10.1017/S1358246117000029
Anderson, C., and Robey, D. (2017). Affordance potency: Explaining the actualization of technology affordances. Inf. Organ. 27, 100–115. doi: 10.1016/j.infoandorg.2017.03.002
Bagozzi, R. P. (2007). The legacy of the technology acceptance model and a proposal for a paradigm shift. J. Assoc. Inf. Syst. 8, 244–254. doi: 10.17705/1jais.00122
Bandura, A. (1989). “Social cognitive theory,” in Annals of child development. Six theories of child development, ed. R. Vasta (Greenwich, CT: JAI Press).
Barr, B., and Miller, S. (2013). Higher Education: The Online Teaching and Learning Experience. Phoenix, AZ: University of Phoenix Faculty School of Advanced Studies.
Benbasat, I., and Barki, H. (2007). ‘Quo vadis, TAM? J. Assoc. Inf. Syst. 8, 211–218. doi: 10.17705/1jais.00126
Burrell, G., and Morgan, G. (1979). Sociological Paradigms and Organizational Analysis. Portsmouth, NH: Heinemann.
Castiblanco Jimenez, I. A., Cepeda García, L. C., Violante, M. G., Marcolin, F., and Vezzetti, E. (2021). Commonly used external TAM variables in e-learning, agriculture and virtual reality applications. Future Internet 13:7. doi: 10.3390/fi13010007
Chao, C. M. (2019). Factors determining the behavioural intention to use mobile learning: an application and extension of the UTAUT Model. Front. Psychol. 10:1652. doi: 10.3389/fpsyg.2019.01652
Cheung, R., and Vogel, D. (2013). Predicting user acceptance of collaborative technologies: an extension of the technology acceptance model for e-learning. Comput. Educ. 63, 160–175. doi: 10.1016/j.compedu.2012.12.003
Chuttur, M. Y. (2009). ‘ Overview of the Technology Acceptance Model: Origins. Bloomington, IN: Indiana University.
Conole, G., and Fill, K. (2005). A learning design toolkit to create pedagogically effective learning activities. J. Interact. Media Educ. 2005:9. doi: 10.5334/2005-8
Dasgupta, S., Granger, M., and McGarry, N. (2002). User acceptance of e-collaboration technology: an extension of the technology acceptance model. Group Decis. Negotiat. 11, 87–100. doi: 10.1023/A:1015221710638
Davis, F. D. (1985). A Technology Acceptance Model for Empirically Testing New End-User Information Systems: Theory and Results. Ph.D. thesis. Cambridge, MA: Massachusetts Institute of Technology.
Davis, F. D. (1986). A Technology Acceptance Model for Empirically Testing New End-User Information Systems: Theory and Results Ph.D. thesis. Cambridge, MA: Massachusetts Institute of Technology.
Davis, F. D. (1993). ‘User acceptance of information technology: System characteristics, user perceptions and behavioural impact’. Int. J. Man Mach. Stud. 83, 475–487. doi: 10.1006/imms.1993.1022
Davis, F. D., Bogozzi, R. P., and Warshaw, P. R. (1989). User acceptance of computer technology: A comparison of two theoretical models. Manage. Sci. 35, 982–1003. doi: 10.1287/mnsc.35.8.982
Diop, E. B., Zhao, S., and Tran, V. (2019). An extension of the technology acceptance model for understanding travelers’ adoption of variable message signs. PLoS One 14:e0216007. doi: 10.1371/journal.pone.0216007
Edmunds, R., Thorpe, M., and Conole, G. (2012). Student attitudes towards and use of ICT in course study, work and social activity: a technology acceptance model approach. Br. J. Educ. Technol. 43, 71–84. doi: 10.1111/j.1467-8535.2010.01142.x
Fariza, K., Assel, A., Gulzhan, Y., and Marzhangul, M. (2021). Evaluation of teachers’ views on the use of learning technologies in mathematics lessons in preschool and primary schools. World J. Educ. Technol. 13, 707–720. doi: 10.18844/wjet.v13i4.6257
Fathema, N., Shannon, D., and Ross, M. (2015). Expanding the Technology Acceptance Model (TAM) to examine faculty use of Learning Management Systems (LMSs) In Higher Education Institutions. J. Online Learn. Teach. 11, 210–233.
Fishbein, M., and Ajzen, I. (1975). Belief, attitude, intention, and behaviour: An introduction to theory and research. Ontario: Addison-Wesley Pub. Co.
Gellerstedt, M., Babaheidari, S. M., and Svensson, L. (2018). A first step towards a model for teachers’ adoption of ICT pedagogy in schools. Heliyon 4:e00786. doi: 10.1016/j.heliyon.2018.e00786
Go, H., Myunghwa, K., and SeungBeum, C. S. (2020). Machine learning of robots in tourism and hospitality: interactive technology acceptance model (iTAM) – cutting edge. Tour. Rev. 75, 629–630. doi: 10.1108/TR-02-2019-0062
Gong, M., Xu, Y., and Yuecheng, Y. (2004). An enhanced technology acceptance model for web-based learning. J. Inf. Syst. Educ. 15, 365–374.
Goodhue, D. L. (2007). Comment on Benbasat and Barki’s “Quo Vadis TAM” article. J. Assoc. Inf. Syst. 8, 220–222. doi: 10.17705/1jais.00125
Hossain, L., and de Silva, A. (2009). Exploring user acceptance of technology using social networks. J. High Technol. Manage. Res. 20, 1–18. doi: 10.1016/j.hitech.2009.02.005
Hwang, Y., and Shin, D. (2020). The role of affordance in the experience of blockchain: the effects of security, privacy and traceability on affective affordance. Online Inf. Rev. 44, 913–932. doi: 10.1108/OIR-01-2019-0013
Jarvenpaa, S., and Staples, D. S. (2000). The use of collaborative electronic media for information sharing: An exploratory study of determinants. J. Strateg. Inf. Syst. 9, 129–154. doi: 10.1016/S0963-8687(00)00042-1
Jung, J., Schneider, C., and Valacich, J. (2010). Enhancing the motivational affordance of Information Systems: the effects of real-time performance feedback and goal setting in group collaboration environments. Manage. Sci. 56, 724–742. doi: 10.1287/mnsc.1090.1129
King, W. R., and He, J. (2006). A meta-analysis of the technology acceptance model. Inf. Manage. 43, 740–755. doi: 10.1016/j.im.2006.05.003
Lai, P. C. (2017). The literature review of technology adoption models and theories for the novelty technology. J. Inf. Syst. Technol. Manage. 14, 21–38. doi: 10.4301/S1807-17752017000100002
Laurillard, D. (2002). Rethinking university teaching, 2nd Edn. London: Routeledge and Francis group. doi: 10.4324/9780203304846
Laurillard, D. (2012). Teaching as a Design Science: Building Pedagogical Patterns for Learning and Teaching. New York, NY: Routledge.
Leclercq, D., and Poumay, M. (2005). The 8 Learning Events Model and its principles. Release 2005-1. LabSET. Liège: University of Liège.
Lee, K., Lee, S., and Hwang, Y. (2014). The impact of hyperlink affordance, psychological reactance, and perceived business tie on trust transfer. Comput. Hum. Behav. 30, 110–120. doi: 10.1016/j.chb.2013.08.003
Lee, M. K. O., Cheung, C. M. K., and Chen, Z. (2005). Acceptance of Internet-based learning medium: the role of extrinsic and intrinsic motivation. Inf. Manage. 42, 1095–1104. doi: 10.1016/j.im.2003.10.007
Leonardi, P. M. (2013). When does technology use enable network change in organizations? MIS Q. 32, 749–775. doi: 10.25300/MISQ/2013/37.3.04
Li, N., and Kirkup, G. (2007). Gender and Cultural Differences in Internet Use: a Study of China and the UK. Comput. Educ. 48, 301–317. doi: 10.1016/j.compedu.2005.01.007
Liu, D., Zhang, Y., and Zhang, H. (2005). A self-learning call admission control scheme for CDMA cellular networks. IEEE Trans. Neural Netw. 16, 1219–1228. doi: 10.1109/TNN.2005.853408
Meerza, A. (2017). A critical review of the technology acceptance model. Int. J. Curr. Res. 9, 52471–52475.
Pintrich, P. R. (2003). A motivational science perspective on the role of student motivation in learning and teaching contexts. J. Educ. Psychol. 95, 667–686. doi: 10.1037/0022-0663.95.4.667
Pituch, K. A., and Lee, Y.-K. (2006). The influence of system characteristics on e-learning use. Comput. Educ. 47, 222–244. doi: 10.1016/j.compedu.2004.10.007
Porter, C., and Donthu, N. (2006). Using the Technology Acceptance Model to Explain How Attitudes Determine Internet Usage. J. Bus. Res. 59, 999–1007. doi: 10.1016/j.jbusres.2006.06.003
Sadeck, O. (2016). An exploration of e-learning practices of teachers at selected schools in the Western Cape. Belleville: Cape Peninsula University of Technology.
Sánchez, A. R., and Hueros, A. D. (2010). Motivational factors that influence the acceptance of Moodle using TAM. Comput. Hum. Behav. 26, 1632–1640. doi: 10.1016/j.chb.2010.06.011
Sánchez-Franco, M. J. (2009). The Moderating Effects of Involvement on the Relationships Between Satisfaction, Trust and Commitment in e-Banking. J. Interact. Mark. 23, 247–258. doi: 10.1016/j.intmar.2009.04.007
Sayel, A. H., and Rahman, M. N. (2003). Students Use of the Internet: an Extension of TAM in Technical and Vocational Institutions in Brunei Darussalam. Austral. J. Inf. Syst. 10, 91–104. doi: 10.3127/ajis.v10i2.156
Shachak, A., Kuziemsky, C., and Petersen, C. (2019). Beyond TAM and UTAUT: future directions for HIT implementation research. J. Biomed. Inf. 100:103315. doi: 10.1016/j.jbi.2019.103315
Shin, D. (2019). Blockchain: the emerging technology of digital trust. Telemat. Informat. 45, 1–11. doi: 10.1016/j.tele.2019.101278
Shin, D. (2021). The Perception of Humanness in Conversational Journalism: an Algorithmic Information-Processing Perspective. Thousand Oaks, CA: New Media & Society, doi: 10.1177/1461444821993801
Shin, D. (2022). The actualization of meta affordances: Conceptualizing affordance actualization in the metaverse games. Comput. Hum. Behav. 133:107292. doi: 10.1016/j.chb.2022.107292
Steele, J., Holbeck, R., and Mandernach, J. (2019). Defining Effective Online Pedagogy. J. Instr. Res. 8, 5–7. doi: 10.9743/JIR.2019.8.2.1
Tsai, Y.-R. (2015). Applying the Technology Acceptance Model (TAM) to explore the effects of a Course Management System (CMS)-Assisted EFL writing instruction. CALICO J. 32, 153–171. doi: 10.1558/calico.v32i1.25961
Velasco, P. (2016). Sketching Bitcoin: Empirical Research of Digital Affordances. Berlin: Springer International. doi: 10.1007/978-3-319-40700-5_6
Venkatesh, V., and Bala, H. (2008). Technology Acceptance Model 3 and a Research Agenda on Interventions. Decis. Sci. 39, 273–312. doi: 10.1111/j.1540-5915.2008.00192.x
Venkatesh, V., and Davis, F. D. (1996). A model of the antecedents of perceived ease of use: development and Test. Decis. Sci. 27, 451–481. doi: 10.1111/j.1540-5915.1996.tb01822.x
Venkatesh, V., and Davis, F. D. (2000). A Theoretical Extension of the Technology Acceptance Model: four Longitudinal Field Studies. Manage. Sci. 46, 186–204. doi: 10.1287/mnsc.46.2.186.11926
Venkatesh, V., Morris, M. G., Davis, F. D., and Davis, G. B. (2003). User Acceptance of Information Technology: toward a Unified View. MIS Q. 27, 425–478. doi: 10.2307/30036540
Wannapiroon, P., Nilsook, P., Kaewrattanapat, N., Wannapiroon, N., and Supa, W. (2021). Augmented Reality Interactive Learning Model, using the Imagineering Process for the SMART Classroom. TEM J. 10, 1404–1417. doi: 10.18421/TEM103-51
Wiggins, G., and McTighe, J. (2005). Understanding by design, expanded, 2nd Edn. Alexandria VA: Association for Supervision and Curriculum Development.
Keywords: TAM, technological affordance, pedagogical, disposition, COVID-19, grounded in theory
Citation: Sadeck O (2022) Technology Adoption Model: Is Use/Non-use a Case of Technological Affordances or Psychological Disposition or Pedagogical Reasoning in the Context of Teaching During the COVID-19 Pandemic Period? Front. Educ. 7:906195. doi: 10.3389/feduc.2022.906195
Received: 28 March 2022; Accepted: 14 June 2022;
Published: 06 July 2022.
Edited by:
Rob Branch, University of Georgia, United StatesReviewed by:
Clement Simuja, Rhodes University, South AfricaCopyright © 2022 Sadeck. This is an open-access article distributed under the terms of the Creative Commons Attribution License (CC BY). The use, distribution or reproduction in other forums is permitted, provided the original author(s) and the copyright owner(s) are credited and that the original publication in this journal is cited, in accordance with accepted academic practice. No use, distribution or reproduction is permitted which does not comply with these terms.
*Correspondence: Osman Sadeck, T3NhZGVja0BnbWFpbC5jb20=
Disclaimer: All claims expressed in this article are solely those of the authors and do not necessarily represent those of their affiliated organizations, or those of the publisher, the editors and the reviewers. Any product that may be evaluated in this article or claim that may be made by its manufacturer is not guaranteed or endorsed by the publisher.
Research integrity at Frontiers
Learn more about the work of our research integrity team to safeguard the quality of each article we publish.