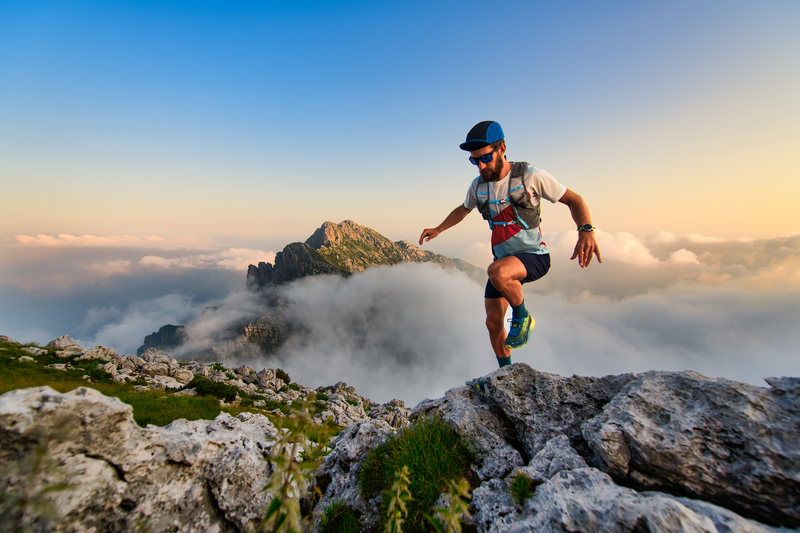
95% of researchers rate our articles as excellent or good
Learn more about the work of our research integrity team to safeguard the quality of each article we publish.
Find out more
ORIGINAL RESEARCH article
Front. Educ. , 20 June 2022
Sec. STEM Education
Volume 7 - 2022 | https://doi.org/10.3389/feduc.2022.892312
Concept maps are graphical tools for organizing and representing knowledge. They are recommended for biology learning to support conceptual thinking. In this study, we compare concept map construction (CM-c, i.e., creating concept maps) and concept map study (CM-s, i.e., observing concept maps). Existing theories and indirect empirical evidence suggest distinct effects of both formats on cognitive, metacognitive and emotional aspects of learning. We developed a CM-c training, a CM-s training, and a brief introduction to concept maps (control training) for junior high school students. We investigated effects on learning performance, concept map quality, cognitive load (cognitive effects), accuracy of self-evaluation (metacognitive effects) and enjoyment (emotional effects) of these trainings in a subsequent learning phase (CM-c learning vs. CM-s learning) in a quasi-experimental two-factorial study with 3 × 2 groups (N = 167), involving the factors training type and learning type. Results reveal that CM-c training increased learning performance and concept map quality. Effects of CM-c training on learning performance transferred onto learning with CM-s. Self-evaluation was slightly more accurate after CM-c training than CM-s training. Students reported moderate, and highly varying enjoyment during CM-c and CM-s learning. The superiority of CM-c over CM-s in learning performance and concept map quality probably lies in its characteristic of being an active learning strategy. We recommend practitioners to favor CM-c training over CM-s training, and foster students’ active engagement and enjoyment.
Natural sciences deal with the description, explanation and prediction of natural phenomena. Inherent to understanding the natural sciences is conceptual thinking. Conceptual thinking involves organization of new knowledge and the integration of it into already existing knowledge. Modern biology lessons aim to provide opportunities for students to develop skills in conceptual thinking, and educate students to apply these skills to become solution-focused problem solvers. While conceptual thinking can be challenging for students (OECD, 2016; Ekinci and Şen, 2020), it can be encouraged through many different learning strategies. Working with concept maps provides such a learning strategy (e.g., Tseng, 2020). Concept maps (CMs) are network-like diagrams for organizing and representing knowledge. They summarize and visualize the most important concepts of a topic and the relationships between these concepts. Concepts are linked with labeled arrows whereas the direction of the arrowheads specify the reading direction. Concept map construction (CM-c) is the process of creating a concept map (mostly) based on textual material by self-organizing concepts and arrows. Concept map study (CM-s), on the other hand, is the process of viewing a previously designed (expert-)concept map without additional textual material.
Concept maps have been intensively examined and further developed since their introduction in the 1970s by Joseph Novak. Many recommendations were given for their use (see e.g., Schroeder et al., 2018 for a recent overview). Heterogeneous results regarding the learning effectiveness of concept maps are often explained by the notion that the learners had different expertise in the use of concept maps. Up to now, it is controversially discussed whether concept map training is necessary in order to use concept maps successfully and how this training should be structured. While previous studies primarily focused on cognitive aspects of learning with concept maps (e.g., learning performance and concept map quality), metacognitive and emotional aspects have scarcely been addressed. However, learning processes are generally accompanied by metacognitive and emotional activities (e.g., self-evaluation and enjoyment) whilst directly or indirectly influencing learning outcome.
This study presents and examines two concept map trainings, focusing on concept map construction on the one hand and concept map study on the other. The aim of this study was to (1) develop a training structure based on theoretical foundation and empirical evidence, (2) examine aspects of cognitive, metacognitive, and emotional effects of familiarity with concept maps on the learning process, and (3) investigate to what extent expertise with one learning format (e.g., concept map study) is conducive to the use of the other format (here: concept map construction). We specifically aim at deriving implications for practitioners and future research from our study.
CM-c and CM-s are regularly used in classrooms and empirical comparison of their effects on learning seems valuable. Learning with concept maps can yield improved learning outcome (Visible Learning MetaX Research Base ®, 2021). This is especially prevalent when CM-c and CM-s are compared with other learning strategies. Learners who constructed concept maps outperformed learners who took notes (Reader and Hammond, 1994), created summaries, discussed with fellow students (Chularut and DeBacker, 2004), marked texts (Amer, 1994), and read texts or attended a lecture (Nesbit and Adesope, 2006; Woldeamanuel et al., 2020; Hwang et al., 2021). Learners who studied (animated) concept maps outperformed others who studied texts (Rewey et al., 1989; Patterson et al., 1992; O’Donnell et al., 2002; Nesbit and Adesope, 2011), lists (Lambiotte et al., 1993), or outlines (Salata, 1999). Meta-analyses report mixed findings when comparing CM-c and CM-s based on effect sizes. Horton et al. (1993) observed greater benefits for CM-s than for CM-c. In contrast, Adesope and Nesbit (2013) and Schroeder et al. (2018) observed greater benefits for CM-c than CM-s. The more recent meta-analysis including more studies and larger sample sizes, provide evidence for superiority of CM-c over CM-s in learning performance. We are not aware of empirical studies that directly compared the effects of CM-c and CM-s on learning outcome. Comparing CM-c and CM-s will offer insight into the robustness of theory-driven cognitive mechanisms of learning with concept maps. Findings might also provide guidance for practitioners to make decisions about learning strategy use.
Based on Ausubel’s theory on learning (Ausubel et al., 1978), it is argued that concept maps promote meaningful learning (Novak and Cañas, 2008; Schroeder et al., 2018). Meaningful learning is taking place when new knowledge is created or assimilated into existing interconnected knowledge structures through cognitive elaboration (Novak and Cañas, 2008). Meaningful learning involves well-organized, relevant knowledge structure and emotional commitment to integrate new knowledge with existing knowledge (Novak and Cañas, 2008). Potential cognitive effects of learning with concept maps are proposed (Nesbit and Adesope, 2006; Schroeder et al., 2018). They include: (1) Dual coding through visual and verbal information in concept maps supports effective retrieval, (2) Cognitive load is reduced and overloading of the memory system is prevented, (3) Centralization of the key concept allows for better semantic integration, (4) Semantic structure is marked more clearly compared to text formats, (5) Simple syntax allows for easy access to learners with yet poor reading and writing abilities, (6) Greater elaborative thinking is promoted through decision making processes, and (7) Greater elaborative thinking is promoted through higher degree of concision and summarization.
With respect to these proposed cognitive effects, a distinction must be made between different concept map formats. CM-c and CM-s differ particularly in their degree of elaborative thinking and cognitive load (mechanisms 2, 6, and 7). CM-c is presumed to promote learners’ active engagement with the interconnections of the content (Hardy and Stadelhofer, 2006; Freeman et al., 2014); it is more cognitively demanding, supports deeper engagement, and fosters a higher level of elaborative thinking than CM-s (Schroeder et al., 2018). Taken together, enhanced learning performance through CM-c than CM-s can be assumed. The impact on other relevant learning variables is likely to differ between CM-c and CM-s, too.
Despite a small number of studies concluding that a short introduction to concept maps is sufficient or that learning with concept maps does not need to be practiced at all (Ruiz-Primo, 2004; Ifenthaler, 2011; Karpicke and Blunt, 2011), research predominantly recommends concept map practice. Most scholars in the field support the notion that the learning effectiveness of concept maps depends on the degree of familiarity with this learning method (Holley and Dansereau, 1984; Renkl and Nückles, 2006; Correia et al., 2008; Mintzes et al., 2011; Aguiar and Correia, 2017; Großschedl and Tröbst, 2018). Trainings (i.e., extended periods of practice) increase familiarity and hence support learning effectiveness. It was shown that CM-c trainings improve the ability to construct concept maps (den Elzen-Rump and Leutner, 2007; Jin and Wong, 2010; Sumfleth et al., 2010; Leopold and Leutner, 2015; Becker et al., 2021). In line with this, it was observed that expertise in the use of knowledge maps (Chmielewski and Dansereau, 1998) and concept maps (Chang et al., 2002) improves knowledge structuring and information encoding when summarizing texts. CM-s training increased level of expertise measured through eye movement (Lenski and Großschedl, im Druck). For untrained students, on the other side, CM-c yielded negative effects on learning performance (Neuroth, 2007).
These negative effects are probably due to excessive cognitive load. Learners’ working memory may get overloaded when processing two types of information simultaneously: strategy-related information about concept mapping and learning-related information about learning contents. Learners might experience a so-called map shock when studying concept maps. This is characterized by “bewilderment of not knowing where to start or how to penetrate the topography of the map” (Blankenship and Dansereau, 2000; p. 294).
Theoretically, memory resources can be occupied by three types of cognitive load: intrinsic, germane, and extraneous load (Sweller, 2010). Intrinsic load arises from the difficulty and complexity of the task. It depends on the number of interacting elements (element interactivity) and learners’ prior knowledge. Intrinsic load can be manipulated by activating the learners’ prior knowledge or simplifying the learning content (Klepsch and Seufert, 2020).
Intrinsic load cannot be altered directly by the design of learning material. On the other side, extraneous load is caused by suboptimal design of learning material (e.g., plain, text-based learning materials; e.g., Poppenk et al., 2010; Orru and Longo, 2018). A reduction in extraneous load could free resources to be available for acquiring and automating schemes in long-term memory (germane load). Germane load refers to the learning-related load and comprises resources that are available for acquiring and automating schemes in long-term memory.
Increasing the familiarity with concept maps through training could result in a reduction of intrinsic and extraneous load; and prevent a map shock. Greater familiarity with the task could reduce the amount of new strategy-related information, simplify the learning process and reduce the perceived difficulty (intrinsic load, Young et al., 2014). As a consequence, more cognitive resources for content-related processes (germane load) will be available (Mayer and Moreno, 2003).
We presume intrinsic (H1.3a) and extraneous cognitive load (H1.3b) to be reduced and germane load (H1.3c) to be increased through both, CM-c training and CM-s training. We expect this effect to be evident compared to a control training.
Furthermore, we assume that learners who are trained in the use of CM-c or CM-s, show improved skills in constructing concept maps (concept map quality) (H1.2) and increased learning performance compared to untrained learners (H1.1a).
We additionally aim at understanding whether skills acquired through training in one specific format of working with concept maps impact working with another format. Although both learning formats are somewhat similar, it needs to be assumed that different skills are needed for each type of learning, e.g., CM-c learning requires learners to (re-)structure, CM-s learning requires learners to recognize information and compare new knowledge with already existing knowledge. We address the question whether CM-c training is conducive to CM-s and vice versa. If such a transfer effect exists, we might see similar results in learning performance when learning with CM-c and CM-s after CM-c training. We assume that CM-c training has higher transfer potential on CM-s learning than CM-s training has on CM-c learning, because concept mapping skills are probably transferred from the (more) active type of use to the (more) passive type of use (H1.1b). Taken together, an advantage of CM-c training on cognitive measurements is expected.
The accuracy of self-evaluation refers to the congruency of objective and subjective performance evaluation. Self-evaluation is conceptually placed within the frameworks of metacognition and self-regulation (see Flavell, 1979; Panadero, 2017). Both frameworks refer to abilities that include planning, monitoring and evaluating one’s own learning processes (Schraw, 1998; Panadero, 2017). Metacognition emphasizes the observer’s perspective and is described as “thinking about thinking” (Flavell, 1979). One’s own thoughts become objects of thoughts themselves. Accuracy of self-evaluation is placed within the evaluation aspect of self-regulation and metacognition.
Accuracy of self-evaluation is pivotal when practicing a new learning strategy, because it might determine appropriate adjustment of learning efforts toward a learning goal. Following Zimmerman’s idea of a circular learning process (Zimmerman, 2000) accurate self-evaluation leads to adapted planning behavior. This means, high congruency of self-evaluation results in more appropriate planning behavior by students and goal attainment of the learning goal becomes more likely. However, accurate self-evaluation is not always naturally existent. Empirical studies suggest that some students overestimate and others underestimate their abilities in various skills (Kruger and Dunning, 1999). The Kruger-Dunning effect was shown to be less evident after improving these skills (Kruger and Dunning, 1999). We assume that the Kruger-Dunning effect probably occurs in working with concept maps as well, and can be overcome by CM training. Through CM trainings, students acquire necessary declarative and procedural skills. Hence, student’s ability to accurately self-evaluate their own skills is likely to improve. While we assume that both trainings (CM-c and CM-s) improve student’s self-evaluation, we expect higher accuracy following a CM-c training (H2). We expect this because of a higher degree of procedural concept map experience in CM-c training.
According to Ausubel et al. (1978), emotional commitment is an inherent part of meaningful learning. Emotional commitment to a learning task is reflected in the construct of enjoyment. Enjoyment can be defined as an activity related affective state (Pekrun et al., 2006). It is experienced when the activity or the learning material is positively valued and perceived as controllable by the learner (Pekrun et al., 2006). Experiencing enjoyment increases task engagement and supports persistent use of a learning strategy beyond training or a formal research study. A few studies report insights into the perception of enjoyment during concept map tasks. Romero et al. (2017) observed that students largely enjoy working with concept maps. Percentages of 77.8 and 88.2% of two groups of 13 to14 year old students stated to “like working on the subject through concept mapping experience.” A study with university students indicates that enjoyment differs between learning formats (Blunt and Karpicke, 2014). Students gave higher reports of enjoyment for constructing concept maps after reading a text compared to summarizing the same text in a paragraph (while the text is still present). In this study moderate enjoyment was reported (29 to 51 on a scale from 0 = “not at all” to 100 = “totally”).
CM-trainings have the potential to increase enjoyment. Negative affective states which accompany (potential) excessive cognitive demands might be reduced as a consequence of familiarity with concept maps. Learners will be more likely to perceive the task as controllable. We assume that CM-c and CM-s trainings increase familiarity with concept maps, reduce cognitive demands and therefore increase enjoyment with working with concept maps (H3). Potential differences between the learning formats (CM-c learning, CM-s learning) are of equal interest in this study.
We investigate the effects of concept map trainings (CM-c training, CM-s training, control training) and concept map learning type (CM-c learning, CM-s learning) on cognitive (learning performance, concept map quality, cognitive load), metacognitive (accuracy of self-evaluation) and emotional aspects (enjoyment) through a direct comparison.
Based on the theoretical foundation, the following hypotheses arise:
H1.1: We assume that learners who are trained in the use of CM-c or CM-s show increased learning performance compared to untrained learners (a). Furthermore, we assume that CM-c training has higher transfer potential on CM-s learning than CM-s training has on CM-c learning, because concept mapping skills are probably transferred from the (more) active type of use to the (more) passive type of use (b).
H1.2: We hypothesize that learners who are trained in the use of CM-c or CM-s, show improved skills in constructing concept maps (concept map quality).
H1.3: We presume intrinsic (a) and extraneous cognitive load (b) to be reduced and germane load (c) to be increased through both, CM-c training and CM-s training compared to a control training.
H3: We assume that CM-c and CM-s trainings increase familiarity with concept maps, reduce cognitive demands and therefore increase enjoyment with working with concept maps.
This study was conducted at non-academic track schools during regular school days and term. One instructor conducted the study in all classes and was assisted by one of three assistants. All assistants received the same instructions and performed the same tasks. Both, the instructor and the assistants supported students in case instructions or clarification are needed. We followed the respective local school law agreements (North Rhine-Westphalian Ministry of Education Science and Research, 2005) and the ethical principles and guidelines for the protection of human subjects of research (Department of Health, Education, and Welfare, 2014).
Schools were contacted via e-mail, flyer or personally. Classes were invited to take part in the quasi-experimental intervention study. We received the greatest response from non-academic track schools. The study covered a period of about 3 weeks and was carried out in regular biology or natural science lessons (see Figure 1). The study involved three main phases: firstly, a pretesting phase; secondly, a training phase, and thirdly, a combined learning and testing phase. The entire study comprised six lessons of 45 min each with visiting times of two lessons each week. Pretesting phase, in which demographic data were gathered, took place in the first school lesson. It was identical for all participants. Subsequently, entire classes were randomly assigned to one of the trainings by drawing lots. Entire classes underwent either a CM-c training, a CM-s training or a control training. Training phase lasted for three lessons. After the training phase, students were randomly assigned to either one of two types of learning. Within one class, half of the students studied through CM-c learning and the other half studied through CM-s learning. Students studied with individual workbooks. In this learning and testing phase, students’ ability to develop knowledge through CM-learning was measured. A second set of workbooks was used to assess the effects of training and learning. In these textbooks, students provided answers to test questions and variables of interest. Learning and testing phase lasted for two lessons. The stepwise randomization (first step: class level, second step: student level) resulted in a two-factorial design with 3 × 2 groups. Of 58 students that took part in the CM-c training, 31 students studied through CM-c learning and 27 students studied through CM-s learning in the learning and testing phase. Of 59 students that took part in the CM-s training, 29 students studied through CM-c learning and 30 students studied through CM-s learning in the learning and testing phase. Of 50 students that took part in the control training, 20 students studied through CM-c learning and 30 students studied through CM-s learning in the learning and testing phase. Supplementary Material 1 shows resulting groups.
Figure 1. Study design and procedure. Participant allocation can be obtained from Supplementary Material 1.
A total of 201 eighth-graders from nine classes (between 12 and 35 students per class) at non-academic track schools in North Rhine-Westphalia, Germany participated in this study. The 8th grade was chosen because, according to the curriculum, method training can be integrated well here. Supplementary Material 1 gives an overview of participant allocation, exclusion criteria and the variables analyzed. We excluded thirty-four students from data analyses because crucial parts of the study were missed. Eighteen students were excluded because they took part in less than two out of three training sessions. Sixteen students were excluded because they were late for class and missed parts of the learning and testing phase. The remaining N = 167 participants were on average M = 14.05, SD = 0.82 years old. Of all participants, 47.3% were female and 44.9% were male (7.8% did not provide an answer). A percentage of 52.1% were German native speakers and 25.7% stated another language than German as their first language (22.2% did not provide an answer). Reading fluency was lower (80.42 ± 13.62) than in norm samples (100 ± 15) as assessed by Salzburger Lesescreening (Auer et al., 2005). The average biology grade was 2.68 (grading scale from 1 = “very good” to 6 = “insufficient”). Students were informed that this study will not affect their academic reports. In one class, only a small number of students gave evaluable answers to the questions regarding cognitive load, self-evaluation and enjoyment leading to reduced sample sizes for these variables (see Supplementary Material 1). We note that the instruction was disregarded by the students.
During pretesting phase, we gathered students’ demographic information including age and gender, reading fluency and prior knowledge about ecosystems to account for individual differences potentially influencing learning performance. Reading fluency was assessed through the Salzburger Lesescreening 5–8 with reported reliability of rtt = 0.89 (SLS 5–8; Auer et al., 2005). This test measures reading speed and reading comprehension by means of a list of simple sentences. Students are asked to read these sentences as quickly as possible and determine their truthfulness. The test can be assessed in class and takes about 10 min to execute. Prior knowledge about ecosystems in general and the ecosystem lake was evaluated in a written test including single and multiple-choice questions (see Supplementary Material 2). The questionnaire consisted of six self-developed questions and two modified questions obtained from Keusch and Telaak (2017). Additionally, three questions were obtained from the third International Mathematics and Science Study TIMSS (Harmon et al., 1997; Baumert et al., 1998), as the items were validated for grade eigth and cover the topic ecosystems (see Supplementary Material 2, items taken from the TIMSS study are marked accordingly). An item on general knowledge about ecosystems includes, for example, the task of filling in an incomplete food chain (Supplementary Material 2, p. 3, item 4). An item focusing on the lake ecosystem covers, for example, the limnetic zone of a lake (Supplementary Material 2, p. 5, item 6). Test scores were transformed into a percentage value with 100% indicating solely correct answers. We report a Cronbach’s α of 0.36.
All trainings were based on cognitive theories as recommended by Collins et al. (1988); Klauer (1988), and Renkl (2010). The theory of adaptive control of thought (ACT; Anderson, 1983) recommends to teaching declarative knowledge (e.g., facts, ideas, and rules) followed by procedural knowledge (knowledge of how an activity is performed) to acquire competence in a certain process. Based on this, all trainings began with a 25-min introduction to concept mapping. This introduction included declarative knowledge about concept maps, the general idea of concept maps and the use of this new learning method. In CM-c and CM-s training, procedural knowledge about CM-c and CM-s was conveyed. The cognitive apprenticeship theory (CAT; Collins et al., 1988) is a constructivist approach to instruction. Cognitive and metacognitive processes which take place during the execution of complex tasks are made visible. This is done by an instructor who verbalizes these processes while the task is performed and provides support and feedback for the learners when performing the task on their own.
Based on this, students underwent four phases (modeling, scaffolding, fading, and coaching). The modeling phase was administered for declarative introduction (instructor constructs a sample concept map on the blackboard) whereas the remaining three phases were only carried out in the CM-c and the CM-s trainings but not for the control training. Students in the control training did not receive any further instruction or in-depth information on concept maps beyond the 25-min introduction to concept maps. Instead, students took part in a non-academic social training (team building activity) which did not include a learning activity (see Supplementary Material 3 for detailed description of the trainings and their theoretical foundation). In Lenski and Großschedl (2021), the complete teaching concept for the construction training in German including all necessary materials is available.
In the learning and testing phase, we examined students’ ability to develop knowledge through CM-c learning and CM-s learning. Students studied the topic “ecosystem lake” in three subtopics (“living organism in a lake,” “zones of a lake,” “limnetic zones of a lake”) through either CM-c learning or CM-s learning. The three subtopics were studied consecutively with a learning period of 20 min each with individual workbooks. During CM-c learning, students constructed concept maps based on learning texts. Stickers with concepts were provided to promote and simplify the construction of concept maps (for a similar approach see Gehl, 2013). During CM-s learning, students were asked to study expert designed concept maps. These concept maps had been designed based on the same textual material as used in CM-c learning. Validity was secured through three independent raters with content equivalence of o Fleiss’ κ = 0.96 for concept map 1 (“living organisms in a lake”), of Fleiss’κ = 1 for concept map 2 (“zones of a lake”), and of Fleiss’κ = 0.82 for concept map 3 (“limnetic zones of a lake”).
After students studied each subtopic, we measured learning performance, concept map quality (only for CM-c learning, not CM-s learning), cognitive load, self-evaluation, and enjoyment. This resulted in three measurements for all variables providing more valid data than one measurement.
We assessed learning performance on the topic ecosystem lake by a paper-based questionnaire with open-ended and single choice questions. The questionnaire can be obtained from Supplementary Material 4. This questionnaire comprised five self-developed questions, two questions from the TIMSS study (Harmon et al., 1997) and 16 modified questions based on Keusch and Telaak (2017). Test scores were transformed into a percentage value with 100% indicating solely correct answers. We report internal consistency of Cronbach’s α = 0.75.
We assessed concept map quality through a scoring system as suggested by Clausen and Christian (2012). It allows evaluation of concept map structure and content. Students in CM-c learning condition constructed three concept maps on three subtopics of the “ecosystem lake.” Numbers between one and five were assigned for each proposition accounting for the type of relation, labels and connecting structures; 0 = two linked concepts without substantial relation, 1 = two linked concepts, arrow without label but with substantial relation, 2 = two linked concepts with labeled arrow and descriptive relation, 3 = two linked concepts with hierarchical relation, 4 = cause-effect relation without labeled arrow, 5 = cause-effect relation with labeled arrow. Numbers were added to a sum-score. Two rating teams evaluated ten percent of all maps while one rating team rated the entire material. We report an interrater reliability of Cohen’s κ = 0.75 for concept map 1 (“living organisms in a lake”), of Cohen’s κ = 0.94 for concept map 2 (“zones of a lake”), and of Cohen’s κ = 0.94 for concept map 3 (“limnetic zones of a lake”). One overall mean value of all three concept map-sum-scores was calculated for each student.
We assessed cognitive load via the seven-item version of a self-reporting questionnaire designed by Klepsch et al. (2017). We measured extraneous (ECL), intrinsic (ICL) and germane load (GCL). Questionnaire statements were modified only by the replacement of “the task” with “the concept map” (e.g., “When looking at concept maps, many things needed to be kept in mind simultaneously.”). Students rated statements on a seven-point Likert scale ranging from “I fully disagree” to “I fully agree.” Mean values for the subscales over all three times of assessments were computed. We report the following internal consistencies: extraneous load (ECL, Cronbach’s α = 0.68–0.78), intrinsic load (ICL, Cronbach’s α = 0.55–0.75), germane load (GCL, Cronbach’s α = 0.75–0.78).
Self-evaluation on students’ concept map skills was measured with five statements; “I read the text thoroughly,” “I used all the concept stickers,” “I paid attention to the direction of the arrows.”, “I labeled all the arrows.” and “I understood connections between concepts.” Students rated their agreement on a three-stepped emoticon-based scale (joyful, indifferent, sad smiley) according to den Elzen-Rump and Leutner (2007). We report internal consistencies for self-evaluation for each subtopic (concept map 1: Cronbach’s α = 0.68, concept map 2: Cronbach’s α = 0.77, concept map 3: Cronbach’s α = 0.76).
Enjoyment was measured with a single question in reference to Blunt and Karpicke (2014). Enjoyment was measured three times after each of the three learning periods (“living organism in a lake,” “zones of a lake,” “limnetic zones of a lake”). We asked students to answer the question “How much did you enjoy this task?” on a written scale from 0 to 100% in increments of 10%.
Preliminary tests were carried out at an α-level of 0.10 to determine potentially existing differences between training groups before students’ participation in the intervention. Choosing an α-level of 0.10 allows to indirectly minimize the β-error in statistical analyses in which the null hypothesis is “favored.” The null hypothesis is “favored” in preliminary tests because we assume no differences between training groups at baseline. One-way analyses of variance (ANOVAs) and a chi-square test were carried out. Results indicated that there were no differences between training groups in reading fluency, F(2,130) = 2.04, p = 0.135, prior knowledge about ecosystems, F(2,152) = 0.76, p = 0.471 or gender proportions, χ2(2) = 1.34, p = 0.513 but in age, F(2,152) = 2.98, p = 0.054 (for descriptive data see Supplementary Material 5). As we perceive reading fluency and prior knowledge as greater predictors of learning performance than age, we did not regard the age difference between training groups as substantial. For most variables, analyses on standard distribution and outliers (>3× interquartile range) did not yield unusual data distribution. Alternative tests were used in the case of a violation of assumptions (see section “Results” for specific tests applied).
Throughout the results section we use the terms “TRAINING” and “LEARNING” for the two independent variables. “TRAINING” relates to the type of training, which students took part in: CM-c training, CM-s training, control training. “LEARNING” relates to the type of learning phase, which students underwent subsequently to training. Students studied either through CM-c or CM-s. All main hypotheses were tested at an α-level of 0.05. We applied two-way analysis of variances to investigate differences in learning performance and enjoyment through CM training and learning (H1.1a.b; H3). We ran one-way analyses of variances to determine differences in concept map quality between training groups (H1.2). We used two-way multivariate analyses of variances to investigate differences in cognitive load (resp. extraneous, intrinsic, germane cognitive load) through CM training and learning (H1.3a – c). Bonferroni corrections were applied as post hoc analyses for statistically significant results following analyses of variances. We ran Spearman correlations for ordinal data with self-evaluation and concept map quality to determine accuracy of self-evaluation (H2). Correlations allow us to determine congruency of two variables with each other. If not provided by IBM SPSS Statistics (version 24.0), effect sizes were calculated according to Lenhard and Lenhard (2016). Because of missing data in the control group and potential distorting statistical results, we interpret statistical results for cognitive load, self-evaluation and enjoyment in both training groups but not in the control group.
To investigate whether training type (CM-c training, CM-s training, control training) and type of learning (CM-c learning, CM-s learning) influenced learning performance, we ran a two-way analysis of variance on learning performance. Table 1 and Figure 2 show means and standard deviations of learning performance.
Table 1. Means and standard deviations for learning performance, concept map quality, cognitive load, self-evaluation and enjoyment separate for training type and learning and testing phase.
We observed that learning performance was higher after CM-c training (62.58 ± 20.52%) compared to CM-s training (52.65 ± 17.89%); FTRAINING(2, 161) = 4.03, p = 0.020, η2p = 0.05 with post hoc analyses (Bonferroni) resulting in p = 0.017, d = 0.52 (partially support for H1.1a). We observed no differences between the control training (54.74 ± 18.73%) and both CM trainings (pCM–c training = 0.105; pCM–s training = 1.00). We did not find that the type of learning impacted learning performance (CM-c learning: 59.21 ± 20.28%, CM-s learning: 54.44 ± 18.50%); FLEARNING (1,161) = 2.03, p = 0.157. We did not observe an interaction of training type with type of learning; FTRAINING X LEARNING (2, 161) = 0.01, p = 0.989 (support for H1.1b).
To examine differences in concept map quality between training groups (CM-c training, CM-s training, control training) during CM learning, we ran a one-way analysis of variance. Table 1 and Figure 3 show means and standard deviations for concept map quality. Results showed that concept map quality was higher following CM-c training (29.88 ± 15.58) compared to CM-s training (20.03 ± 13.90), F(2,77) = 6.47, p = 0.003, η2p = 0.14 with post hoc analyses (Bonferroni) of p = 0.033, d = 0.67. Concept map quality was also higher following CM-c training compared to the control training (15.77 ± 14.09; p = 0.004, d = 0.95) (partially support of H1.2). There was no difference between CM-s training and the control training (p = 0.956).
To investigate whether training type (CM-c training, CM-s training), and type of learning (CM-c learning, CM-s learning) influenced cognitive load, we ran a two-way multivariate analysis of variance on cognitive load including extraneous (ECL), intrinsic (ICL) and germane load (GCL). Table 1 shows means and standard deviations. Results of the multivariate analysis revealed no difference in cognitive load between training groups FTRAINING (3, 109) = 0.45, p = 0.715, Wilks’ Λ = 0.99, η2p = 0.12, but a difference between type of learning phase FLEARNING (3, 109) = 5.25, p = 0.002, Wilks’ Λ = 0.87, η2p = 0.13. This effect did not reach statistical significance after post hoc testing [FICL (1, 111) = 3.63, p = 0.059, η2p = 0.032; FGCL (1, 111) = 0.61, p = 0.437, η2p = 0.005; FECL (1, 111) = 1.20, p = 0.277, η2p = 0.011].
No interaction of training type with type of learning phase was evident FTRAINING × LEARNING (3, 109) = 1.55, p = 0.205. Taken together, training type (CM-c training, CM-s training) and type of learning did not differ in their impact on students’ cognitive load (lack of support of H1.3a-c).
We investigated whether CM trainings influenced accuracy of students’ self-evaluation.
In our study, accuracy of self-evaluation is reflected in the congruency of students’ self-evaluation (evaluation of concept map skills) and objective assessment (concept map quality). As a measurement of congruency, we ran Spearman correlations for ordinal data with self-evaluation and concept map quality for each training group. High correlations indicate high accuracy of self-evaluation. Correlations reveal highest accuracy after CM-c training (rs = 0.66, p < 0.001, n = 30), followed by CM-s training (rs = 0.52, p = 0.004, n = 28) and the control training (rs = 0.60, p < 0.159, n = 7; partially support for H2). Table 1 shows means and standard deviations for self-evaluation and concept map quality. We observed that only a small number of participants in the control training provided answers to self-evaluation questions. Only a comparison between correlations after CM-c training and CM-s training is legitimate.
To investigate whether training type (CM-c training, CM-s training) and type of learning (CM-c learning, CM-s learning) influenced emotional commitment to learning with CMs, we ran a two-way analysis of variance on enjoyment. Enjoyment was analyzed with Box-Cox transformed data because of a violation of homogeneity of error variances. Table 1 shows untransformed means and standard deviations for enjoyment. We observed moderate enjoyment and high variability across students (38.35 ± 30.09%) with a range of 0 to 100% in enjoyment. Students reported average enjoyment following the CM-c (36.64 ± 32.09%) and CM-s training (37.40 ± 29.33%) with high variability during learning phase. Training type did not influence enjoyment; FTRAINING (1, 111) = 0.40, p = 0.530 (lack of support for H3). We observed no effect of type of learning; FLEARNING (1, 111) = 2.12⋅104, p = 0.988. Training type and type of learning did not interact; FTRAINING X LEARNING, F(1,111) = 3.26, p = 0.074. It needs to be noted that analyses revealed a violation of the assumption of homogeneity of error variances. Box-Cox transformation reduced heterogeneity but did not entirely stabilize data as assessed by Levene’s test, p = 0.036. The unusually dispersed data might have obscured potential effects. Results need to be observed and interpreted with caution.
As expected, results show higher learning performance for students who took part in CM-c training instead of CM-s training (partially support for H1.1b). As we observed that CM-c training improved concept map quality (partially support for H1.2), it is likely that the increased learning performance is a result of improved concept mapping skills.
In line with other findings (Hilbert and Renkl, 2008; Jin and Wong, 2010; Sumfleth et al., 2010), we assume that CM-s training and the control training are not sufficient to enable students to construct concept maps. A specific training in the construction of concept maps is needed to improve students’ ability to construct concept map as suggested by other authors (e.g., den Elzen-Rump and Leutner, 2007; Sumfleth et al., 2010; Großschedl and Tröbst, 2018).
Students were able to apply these skills and to engage more deeply with the learning content. This finding supports the assumption that CM-c promotes elaborative thinking. Elaborative thinking probably takes place to a greater extent in CM-c than in CM-s. We ascribe this superiority of CM-c training in learning performance to its active nature. Active learning tasks are generally associated with increased learning performance (McCagg and Dansereau, 1991; Chang et al., 2002; Freeman et al., 2014).
However, contrary to our hypothesis we did not observe a difference in learning performance between CM trainings and the control training. We assume that students who took part in the control training probably did not acquire the necessary skills to effectively apply CM-c or CM-s during learning. Instead of applying concept mapping skills, students probably used other learning strategies that appeared to be beneficial for them in the past (e.g., repeated reading) (see Wild, 2001 for more information on individual learning strategy use). This is supported by the observation of lower concept quality after the control training. Increase in learning performance following the control training cannot be explained by an increase in concept mapping skills.
In conclusion, in contrast to CM-s training, CM-c training enabled students to apply concept mapping skills to a degree that allowed them to learn effectively with concept maps. Students improved their ability to construct concept maps and they were able to use this learning strategy to acquire similar knowledge as the use of other naïve strategies would. To be able to use concept maps as a more effective way of learning, we suggest practice of more than three lessons. The maximum potential of concept maps as a learning strategy might only be exploited by a prolonged training.
We addressed the questions whether CM-c training impacts CM-s learning and vice versa. Our results show CM-c training increased learning performance irrespective of whether students constructed or studied concept maps in a subsequent learning task (support for H1.1b). Here, the absence of a statistically significant interaction effect suggests the existence of a transfer effect. An evident interaction effect (i.e., higher learning performance after CM-c training for those students who constructed concept maps during learning and testing phase but not for those students who studied concept maps) would have suggested that skills learned through CM-c training are only applied in CM-c learning but not in CM-s learning. We did not observe such an interaction effect and conclude that skills learned through CM-c training are also applied in CM-s learning. The CM-c training most likely altered student’s overall information processing strategies, enabling them to implicitly interact with a different CM learning format. This is in line with previous studies suggesting that the familiarity with particular formats can positively influence learning performance in similar formats (e.g., Royer and Cable, 1976; Royer, 1979). Our results could be explained by the nature of the tasks (passive vs. active learning task). The familiarity in an active learning task (here CM-c) has higher transfer potential compared to the passive learning task. We conclude that CM-c training benefits learning performance regardless of which learning format (CM-c or CM-s) is applied after training.
We expected intrinsic (H1.3a) and extraneous cognitive load (H1.3b) to be reduced and germane load (H1.3c) to be increased through both, CM-c training and CM-s training compared to the control training. Statistical results showed that CM-c training and CM-s training did not differ in their impact on cognitive load. We observed no difference between types of learning.
That cognitive load seemed uninfluenced by training in our study, reflects methodological limitation instead of providing an answer to our research question. We surmise that the used instrument did not differentiate between sources of ECL and ICL as mentioned by Klepsch and Seufert (2020), which was published after the conduction of this study. For settings where ICL and EGL may be intertwined, Klepsch and Seufert (2020) recommend using complex instruments to uncover the underlying processes. We also suspect methodological issues with measuring GCL and agree with the authors of the instrument that the “wording of the current items was ambiguous so learners understood them differently” (Klepsch et al., 2017, p. 9). Therefore, our findings should be treated with caution. Further research is needed to find measurements that reliably assess cognitive load during learning activities. We emphasize that simple and clear language that is comprehensible also for low-achieving students should be used.
We assumed that CM trainings increase accuracy of self-evaluation while we expected that CM-c training has higher influence than CM-s training. Our data only allow a comparison of CM-c and CM-s because of a low number of participants in the control group. Based on effect sizes, results show that accuracy of self-evaluation is improved through CM-c training to a greater extent than CM-s training (partially support for H2). We assume that this outcome is due to higher amount of procedural knowledge acquired through CM-c training. Increased procedural knowledge was shown by the statistical significant difference in concept map quality after CM-c and CM-s training (H1.2). Beyond this, we would like to address the question whether accurate self-evaluation is a premise or a consequence of successful skill acquisition. The answer to this question has relevant implications for practitioners. If accurate self-evaluation is a premise, teachers should include teaching methods that support self-evaluation such as providing opportunities for students to reflect on their current level of task skills. If accurate self-evaluation is a consequence of successful skill acquisition, teachers should focus on students’ skill practice while self-evaluation “automatically” improves. We believe that self-evaluation and skill acquisition could be improved at the same time through specific feedback on task skills.
We suggest that specific feedback on task skills should be given when working with any concept map format including CM-c and CM-s. Based on our data, we cannot conclude whether the Kruger-Dunning effect (Kruger and Dunning, 1999) was overcome by training. Nor can we state whether a Kruger-Dunning effect is evident in working with concept maps.
We hypothesized that CM-c and CM-s trainings increase enjoyment during learning with concept maps compared to a control training. Because of missing data, we are unable to answer this research question. Nevertheless, a comparison of CM-c and CM-s learning is legitimate. CM-c and CM-s did not differ in their degree of enjoyment. In contrast to Romero et al. (2017), but in line with Blunt and Karpicke (2014), we observed merely moderate enjoyment for working with concept maps, while Karpicke and Blunt carried out their study with university students and not school students. We observed in our study higher variability in enjoyment than Romero et al. (2017), who carried out their study with medium to high achieving students. Moderate enjoyment and high variability in our study, lead us to conclude that concept maps should be applied with the aim to enhance enjoyment, especially for those students with yet low to medium academic skills as seen in our study.
Interactive concept maps might provide such an opportunity. Results from meta-analysis have already shown promising effects on learning performance (Schroeder et al., 2018), but the small number of studies does not allow a reliable conclusion. Emotional commitment measured as enjoyment is an integral part of meaningful learning. Based on our findings, we recommend to take high variability in enjoyment into account and support enjoyment for students with the aim to enhance meaningful learning.
As common for empirical studies, our results need to be viewed in the context of some limitations. Concerning the measurement of the learning performance, it must be considered that the reliability of the pretest was low (α = 0.36). In this study, we intentionally chose a topic that was still unknown to the students of the eighth grade. This guarantees a similar level of prior knowledge. However, it is known that this can lead to a high guessing probability (e.g., Bergman et al., 2015), which in turn can result in poor reliability of the test. Furthermore, we examined learning performance immediately after training, as most past findings on trainings on graphic strategies did (Moorf and Readence, 1984). However, delayed learning tests are more sensitive to effects of learning compared to immediate tests (Dunlosky et al., 2013). Future studies might consider analyzing long term effects following concept map trainings to unveil potentially delayed learning effects and we also strongly suggest including motivational measurements as control variables. As most instruments were not designed for the application with junior high school students test validity for this age group has to be confirmed. Moreover, we observed high variability in student’s answers, e.g., enjoyment, which reflects “real life” situations but limits options for inferential statistical analyses. Potential effects might be obscured.
Acknowledging the limitations of our study, the direct comparison of CM-c and CM-s allows us to contribute to recent meta-analytical findings (Schroeder et al., 2018). In line with Schroeder et al. (2018) we observed that the construction of concept maps has greater impact on cognitive aspects of learning than the study of concept maps. In detail, we found that training in CM-c compared to CM-s training lead to enhanced learning performance and concept map quality. Concept mapping skills acquired through CM-c training transferred onto learning with CM-s. Students that underwent a CM-c training were able to transfer new skills onto learning with CM-s. We also observed increased accuracy of self-evaluation through CM-c training than CM-s training. Beyond these cognitive and metacognitive outcomes, we add insights into emotional effects of learning with concept maps. We found highly dispersed and overall moderate enjoyment across students. We did not observe statistically significant differences in enjoyment between learning formats after training and learning. Based on the overall results in this study, we conclude that CM-c training has greater effects on cognitive and metacognitive aspects of learning than CM-s training, but not on emotional aspects measured as enjoyment.
For the use in classrooms, we recommend teachers to apply a preceding CM-c training, because it improves learning performance, concept map quality and students’ accuracy of self-evaluation compared to CM-s training. Additionally, concept mapping skills acquired through CM-c are likely to be applied by students in learning with CM-c and CM-s similarly. We advise teachers to promote enjoyment to enhance long-term commitment with this learning strategy. At the same time, we emphasize high interindividual differences in students’ enjoyment that needs to be taken into account by teachers. We advise teachers to seek students’ direct feedback about cognitive load during learning so as to prevent cognitive overload. Concept maps can be applied in many ways and depend on the teacher’s goals and the students’ needs. This study aimed to contribute to recent knowledge about cognitive, metacognitive and emotional aspects of learning with concept maps, providing aid in choosing suitable learning strategies to support conceptual thinking.
Data are openly available in DOI: 10.17605/OSF.IO/MW356.
Ethical review and approval was not required for the study on human participants in accordance with the local legislation and institutional requirements. Written informed consent to participate in this study was provided by the participants’ legal guardian/next of kin.
SL and JG: conceptualization and methodology. SL and SE: formal analysis, writing – original draft preparation, and visualization. SL: investigation. JG: resources, writing, review, editing, and supervision. All authors have read and agreed to the published version of the manuscript.
This study was part of the project “Learning Biology through Concept Mapping: Importance of a learning strategy training for cognitive load, cognitive processes and learning performance,” which was funded by the German Research Foundation (Deutsche Forschungsgemeinschaft; DFG), Grant (GR 4763/2).
The authors declare that the research was conducted in the absence of any commercial or financial relationships that could be construed as a potential conflict of interest.
All claims expressed in this article are solely those of the authors and do not necessarily represent those of their affiliated organizations, or those of the publisher, the editors and the reviewers. Any product that may be evaluated in this article, or claim that may be made by its manufacturer, is not guaranteed or endorsed by the publisher.
The Supplementary Material for this article can be found online at: https://www.frontiersin.org/articles/10.3389/feduc.2022.892312/full#supplementary-material
Adesope, O. O., and Nesbit, J. C. (2013). Animated and static concept maps enhance learning from spoken narration. Learn. Instr. 27, 1–10. doi: 10.1016/j.learninstruc.2013.02.002
Aguiar, J. G., and Correia, P. R. M. (2017). From representing to modelling knowledge: proposing a two-step training for excellence in concept mapping. Knowl. Manag. Elearn Int. J. 9, 366–379. doi: 10.34105/j.kmel.2017.09.022
Amer, A. A. (1994). The effect of knowledge-map and underlining training on the reading comprehension of scientific texts. Engl. Specif. Purp. 13, 35–45. doi: 10.1016/0889-4906(94)90023-X
Auer, M., Gruber, G., Mayringer, H., and Wimmer, H. (2005). Salzburger Lese-Screening für die Klassenstufen 5-8 (SLS 5-8) [Salzburg Reading Screening for Grades 5–8]. Bern: Huber.
Ausubel, D. P., Novak, J. D., and Hanesian, H. (1978). Educational Psychology–A Cognitive View. Holt: Rinehart and Winston.
Baumert, J., Lehmann, R., Lehrke, M., Clausen, M., Hosenfeld, I., Neubrand, J., et al. (1998). Testaufgaben Naturwissenscgaften TIMSS 7./8. Klasse (Population 2) [Test questions natural sciences TIMSS 7./8. Class (population 2)]. Berlin: Max-Planck-Institut für Bildungsforschung.
Bergman, E. M., de Bruin, A. B., Vorstenbosch, M. A., Kooloos, J. G., Puts, G. C., Leppink, J., et al. (2015). Effects of learning content in context on knowledge acquisition and recall: a pretest-posttest control group design. BMC Med. Educ. 15:133. doi: 10.1186/s12909-015-0416-0
Becker, L. B., Welter, V. D. E., Aschermann, E., and Großschedl, J. (2021). Comprehension-oriented learning of cell biology: do different training conditions affect students’ learning success differentially? Educ. Sci. 11:438. doi: 10.3390/educsci11080438
Blankenship, J., and Dansereau, D. F. (2000). The effect of animated node-link displays on information recall. J. Exp. Educ. 68, 293–308. doi: 10.1080/00220970009600640
Blunt, J. R., and Karpicke, J. D. (2014). Learning with retrieval-based concept mapping. J. Educ. Psychol. 106, 849–858. doi: 10.1037/a0035934
Chang, K.-E., Sung, Y.-T., and Chen, I.-D. (2002). The effect of concept mapping to enhance text comprehension and summarization. J. Exp. Educ. 71, 5–23. doi: 10.1080/00220970209602054
Chmielewski, T. C., and Dansereau, D. F. (1998). Enhancing the recall of text: knowledge mapping training promotes implicit transfer. J. Educ. Psychol. 90:407. doi: 10.1037/0022-0663.90.3.407
Chularut, P., and DeBacker, T. K. (2004). The influence of concept mapping on achievement, self-regulation, and self-efficacy in students of English as a second language. Contemp. Educ. Psychol. 29, 248–263. doi: 10.1016/j.cedpsych.2003.09.001
Clausen, S., and Christian, A. (2012). Concept mapping als messverfahren für den außerschulischen Bereich. [Concept mapping for measurement in a non scholar context]. J. Didaktik Biowissenschaft 3, 18–31.
Collins, A. M., Brown, J. S., and Newman, S. E. (1988). Cognitive apprenticeship: teaching the craft of reading, writing and mathematics. Think. J. Philos. Child. 8, 2–10. doi: 10.5840/thinking19888129
Correia, P. R. M., Infante-Malachias, M. E., and Godoy, C. E. C. (2008). “From theory to practice: the foundations for training students to make collaborative concept maps,” in Proceedings of the 3rd International Conference on Concept Mapping, Vol. 2, eds A. J. Cañas, J. D. Novak, P. Reiska, and M. K. Ahlberg (Põltsamaa: Vali Press), 414–421.
den Elzen-Rump, V., and Leutner, D. (2007). “Naturwissenschaftliche sachtexte verstehen–ein computerbasiertes trainingsprogramm für schüler der 10. Jahrgangsstufe zum selbstregulierten lernen mit einer mapping-strategie [Understanding scientific factual texts–a computer-based training program for 10th grade students for self-regulated learning with a mapping strategy],” in Selbstregulation Erfolgreich Fördern [Successfully Promoting Self-Regulation], eds M. Landmann and B. Schmitz (Stuttgart: Kohlhammer), 251–268.
Department of Health, Education, and Welfare (2014). The Belmont report. Ethical principles and guidelines for the protection of human subjects of research. J. Am. Coll. Dent. 81:4.
Dunlosky, J., Rawson, K. A., Marsh, E. J., Nathan, M. J., and Willingham, D. T. (2013). Improving students’ learning with effective learning techniques: promising directions from cognitive and educational psychology. Psychol. Sci. Public Interest 14, 4–58. doi: 10.1177/1529100612453266
Ekinci, S., and Şen, A. Í (2020). Investigating grade-12 students’ cognitive structures about the atomic structure: a content analysis of student concept maps. Int. J. Sci. Educ. 42, 977–996. doi: 10.1080/09500693.2020.1744045
Flavell, J. H. (1979). Metacognition and cognitive monitoring: a new area of cognitive–developmental inquiry. Am. Psychol. 34:906.
Freeman, S., Eddy, S. L., McDonough, M., Smith, M. K., Okoroafor, N., Jordt, H., et al. (2014). Active learning increases student performance in science, engineering, and mathematics. Proc. Natl. Acad. Sci. U.S.A. 111, 8410–8415. doi: 10.1073/pnas.1319030111
Großschedl, J., and Tröbst, S. (2018). Biologie lernen durch concept mapping: Bedeutung eines Lernstrategietrainings für kognitive Belastung, kognitive Prozesse und Lernleistung - Kurzdarstellung des DFG-projekts [Learning biology by concept mapping: the importance of learning strategy training for cognitive load, cognitive processes and learning performance - brief description of the DFG project]. Z. Didaktik Biol. 22, 20–30. doi: 10.4119/zdb-1630
Gehl, D. (2013). Vom Betrachten zum Verstehen [About Viewing to Understanding]. Wiesbaden: Springer. doi: 10.1007/978-3-531-19823-1
Hardy, I., and Stadelhofer, B. (2006). Concept Maps wirkungsvoll als strukturierungshilfen einsetzen: welche rolle spielt die selbstkonstruktion? Z. Pädagog. Psychol. 20, 175–187. doi: 10.1024/1010-0652.20.3.175
Harmon, M., Smith, T. A., Martin, M. O., Kelly, D. L., Beaton, A. E., Mullis, I. V., et al. (1997). Performance Assessment: IEA’s Third International Mathematics and Science Study (TIMSS). Amsterdam: International Association for the Evaluation of Educational Achievement.
Hilbert, T. S., Nückles, M., Renkl, A., Minarik, C., Reich, A., and Ruhe, K. (2008). Concept Mapping zum lernen aus texten: können prompts den wissens- und strategieerwerb fördern? [Concept mapping for learning from texts: can prompts promote knowledge and strategy acquisition?]. Z. pädagog. Psychol. J. Educ. Psychol. 22, 119–125. doi: 10.1024/1010-0652.22.2.119
Hilbert, T. S., and Renkl, A. (2008). Concept mapping as a follow-up strategy to learning from texts: what characterizes good and poor mappers? Instr. Sci. 36, 53–73. doi: 10.1007/s11251-007-9022-9
Holley, C. D., and Dansereau, D. F. (1984). “Networking: the technique and the empirical evidence,” in Spatial Learning Strategies, eds D. F. Dansereau and C. D. Holley (Amsterdam: Elsevier), 81–108.
Horton, P. B., McConney, A. A., Gallo, M., Woods, A. L., Senn, G. J., and Hamelin, D. (1993). An investigation of the effectiveness of concept mapping as an instructional tool. Sci. Educ. 77, 95–111. doi: 10.1002/sce.3730770107
Hwang, G. J., Chang, S. C., Song, Y., and Hsieh, M. C. (2021). Powering up flipped learning: an online learning environment with a concept map-guided problem-posing strategy. J. Comput. Assis. Learn. 37, 429–445. doi: 10.1111/jcal.12499
Ifenthaler, D. (2011). Identifying cross-domain distinguishing features of cognitive structure. Educ. Technol. Res. Dev. 59, 817–840. doi: 10.1007/s11423-011-9207-4
Jin, H., and Wong, K. (2010). Training on concept mapping skills in geometry. J. Math. Educ. 3, 104–119.
Karpicke, J. D., and Blunt, J. R. (2011). Retrieval practice produces more learning than elaborative studying with concept mapping. Science 331, 772–775. doi: 10.1126/science.1199327
Keusch, J., and Telaak, S. (2017). Einfluss der Darstellungsform (Concept Maps vs. Fließtext) auf die Rezeptionsleistung von Schülerinnen und Schülern im Themenbereich „Ökosystem See” [Influence of the Form of Representation (concept maps vs. continuous text) on the Reception Performance of Schoolchildren in the Subject Area “Lake Ecosystem”]. Unpublished master’s thesis. Köln: University of Cologne.
Klauer, K. J. (1988). Teaching for learning-to-learn: a critical appraisal with some proposals. Instr. Sci. 17, 351–367. doi: 10.1007/BF00056221
Klepsch, M., Schmitz, F., and Seufert, T. (2017). Development and validation of two instruments measuring intrinsic, extraneous, and germane cognitive load. Front. Psychol. 8:1997. doi: 10.3389/fpsyg.2017.01997
Klepsch, M., and Seufert, T. (2020). Understanding instructional design effects by differentiated measurement of intrinsic, extraneous, and germane cognitive load. Instr. Sci. 48, 45–77. doi: 10.1007/s11251-020-09502-9
Kruger, J., and Dunning, D. (1999). Unskilled and unaware of it: how difficulties in recognizing one’s own incompetence lead to inflated self-assessments. J. Pers. Soc. Psychol. 77:1121. doi: 10.1037/0022-3514.77.6.1121
Lambiotte, J. G., Skaggs, L. P., and Dansereau, D. F. (1993). Learning from lectures: effects of knowledge maps and cooperative review strategies. Appl. Cogn. Psychol. 7, 483–497. doi: 10.1002/acp.2350070604
Lenhard, W., and Lenhard, A. (2016). Berechnung von Effektstärken [Calculation of Effect Sizes]. Dettelbach: Psychometrica.
Lenski, S., and Großschedl, J. (2021). Concept Maps im Unterricht: eine Trainingseinheit für Schüler*Innen der Sekundarstufe [Concept Maps in the Classroom: a Training Session for Secondary School Students]. Available online at: https://doi.org/https://doi.org/10.17605/OSF.IO/48A5W
Lenski, S., and Großschedl, J. (im Druck). “Biologie lernen mit concept maps: Lässt sich die Expertise im Umgang mit concept maps von den Augen ablesen? [Learning biology with concept maps: can the expertise in dealing with concept maps be read from the eyes?],” in Eye Tracking als Methode in der Mathema-tik- und Naturwissenschaftsdidaktik: Forschung und Praxis, eds P. Klein, M. Schindler, N. Graulich, and J. Kuhn (Cham: Springer).
Leopold, C., and Leutner, D. (2015). Improving students’ science text comprehension through metacognitive self-regulation when applying learning strategies. Metacogn. Learn. 10, 23–27. doi: 10.1007/s11409-014-9130-2
Mayer, R. E., and Moreno, R. (2003). Nine ways to reduce cognitive load in multimedia learning. Educ. Psychol. 38, 43–52. doi: 10.1207/S15326985EP3801_6
McCagg, E. C., and Dansereau, D. F. (1991). A convergent paradigm for examining knowledge mapping as a learning strategy. J. Educ. Res. 84, 317–324. doi: 10.1080/00220671.1991.9941812
Mintzes, J. J., Canas, A., Coffey, J., Gorman, J., Gurley, L., Hoffman, R., et al. (2011). Comment on “Retrieval practice produces more learning than elaborative studying with concept mapping” [Technical Comment]. Science 334, 453–453. doi: 10.1126/science.1203698
Moorf, D. W., and Readence, J. F. (1984). A quantitative and qualitative review of graphic organizer research. J. Educ. Res. 78, 11–17. doi: 10.1080/00220671.1984.10885564
Nesbit, J. C., and Adesope, O. O. (2006). Learning with concept and knowledge maps: a meta-analysis. Rev. Educ. Res. 76, 413–448. doi: 10.3102/00346543076003413
Nesbit, J. C., and Adesope, O. O. (2011). Learning from animated concept maps with concurrent audio narration. J. Exp. Educ. 79, 209–230. doi: 10.1080/00220970903292918
Neuroth, J. (2007). Concept-Mapping als Lernstrategie: Eine Interventionsstudie zum Chemielernen aus Texten [Concept mapping as a Learning Strategy: An Intervention Study on Chemistry Learning from Texts]. Berlin:Logos.
North Rhine-Westphalian Ministry of Education Science and Research (2005). Schulgesetz für das Land Nordrhein-Westfalen [School law for the state of North Rhine-Westphalia]. Available online at: https://recht.nrw.de/lmi/owa/br_vbl_detail_text?anw_nr=6&vd_id=3928&vd_back=N102&sg=&menu=1 (accessed January, 2022).
Novak, J. D., and Cañas, A. J. (2008). The Theory Underlying Concept Maps And How To Construct Them. Technical Report IHMC CmapTools 2006-01 Rev 01-2008. Pensacola, FL: Florida Institute for Human and Machine Cognition.
O’Donnell, A. M., Dansereau, D. F., and Hall, R. H. (2002). Knowledge maps as scaffolds for cognitive processing. Educ. Psychol. Rev. 14, 71–86. doi: 10.1023/A:1013132527007
OECD (2016). PISA 2015 Assessment and Analytical Framework: Science, Reading, Mathematic and Financial Literacy. Paris: OECD Publishing. doi: 10.1787/9789264255425-en
Orru, G., and Longo, L. (2018). “The evolution of cognitive load theory and the measurement of its intrinsic, extraneous and germane loads: a review,” in Proceedings of the International Symposium on Human Mental Workload: Models and Applications, Vol. 1012, eds L. Longo and M. C. Leva (Cham: Springer), 23–48. doi: 10.1007/978-3-030-14273-5_3
Panadero, E. (2017). A review of self-regulated learning: six models and four directions for research. Front. Psychol. 8:422. doi: 10.3389/fpsyg.2017.00422
Patterson, M. E., Dansereau, D. F., and Newbern, D. (1992). Effects of communication aids and strategies on cooperative teaching. J. Educ. Psychol. 84, 453–461. doi: 10.1037/0022-0663.84.4.453
Pekrun, R., Elliot, A. J., and Maier, M. A. (2006). Achievement goals and discrete achievement emotions: a theoretical model and prospective test. J. Educ. Psychol. 98:583. doi: 10.1037/0022-0663.98.3.583
Poppenk, J., Köhler, S., and Moscovitch, M. (2010). Revisiting the novelty effect: when familiarity, not novelty, enhances memory. J. Exp. Psychol. Learn. Mem. Cogn. 36:1321. doi: 10.1037/a0019900
Reader, W., and Hammond, N. (1994). “Computer-based tools to support learning from hypertext: concept mapping tools and beyond,” in Proceedings of the Computer Assisted Learning: Selected Contributions from the CAL’93 Symposium, Pergamon, 99–106. doi: 10.1016/B978-0-08-041945-9.50020-2
Renkl, A. (2010). “Lehren und lernen [Teaching and learning],” in Handbuch Bildungsforschung, eds R. Tippelt and B. Schmidt (Wiesbaden: VS Verlag für Sozialwissenschaften), 737–751. doi: 10.1007/978-3-531-92015-3_39
Renkl, A., and Nückles, M. (2006). “Lernstrategien der externen visualisierung [External visualization learning strategies],” in Handbuch Lernstrategien, eds H. Mandl and H. F. Friedrich (Göttingen: Hogrefe), 135–147.
Rewey, K. L., Dansereau, D. F., Skaggs, L. P., and Hall, R. H. (1989). Effects of scripted cooperation and knowledge maps on the processing of technical material. J. Educ. Psychol. 81, 604–609. doi: 10.1037/0022-0663.81.4.604
Romero, M. d. C., Cazorla, M., and Buzón García, O. (2017). Meaningful learning using concept maps as a learning strategy. J. Technol. Sci. Educ. 7, 313–332. doi: 10.3926/jotse.276
Royer, J. M. (1979). Theories of the transfer of learning. Educ. Psychol. 14, 53–69. doi: 10.1080/00461527909529207
Royer, J. M., and Cable, G. W. (1976). Illustrations, analogies, and facilitative transfer in prose learning. J. Educ. Psychol. 68, 205. doi: 10.1037/0022-0663.68.2.205
Ruiz-Primo, M. A. (2004). “Examining concept maps as an assessment tool,” in Proceedings of the 1st International Conference on Concept Mapping, Pamplona.
Salata, M. W. A. (1999). Concept Maps as Organizers in an Introductory University Level Biology Course. Charlottesville, VA: University of Virginia.
Schraw, G. (1998). Promoting general metacognitive awareness. Instr. Sci. 26, 113–125. doi: 10.1023/A:1003044231033
Schroeder, N. L., Nesbit, J. C., Anguiano, C. J., and Adesope, O. O. (2018). Studying and constructing concept maps: a meta-analysis. Educ. Psychol. Rev. 30, 431–455. doi: 10.1007/s10648-017-9403-9
Sumfleth, E., Neuroth, J., and Leutner, D. (2010). Concept mapping – eine lernstrategie muss man lernen. [Concept mapping – learning strategy is something you must learn]. Chemkon 17, 66–70. doi: 10.1002/ckon.201010114
Sweller, J. (2010). Element interactivity and intrinsic, extraneous, and germane cognitive load. Educ. Psychol. Rev. 22, 123–138. doi: 10.1007/s10648-010-9128-5
Tseng, S.-S. (2020). Using concept mapping activities to enhance students’ critical thinking skills at a high school in taiwan. Asia Pac. Educ. Res. 29, 249–256. doi: 10.1007/s40299-019-00474-0
Visible Learning MetaX Research Base ® (2021). Global Research Data Base. CORWIN. Version 1.1. Updated August 2021. Available online at: https://www.visiblelearningmetax.com/influences (accessed December 21, 2021).
Wild, K.-P. (2001). “Lernstrategien und lernstile [learning strategies and learning styles],” in Handwörterbuch Pädagogische Psychologie [Handbook of Educational Psychology], ed. D. H. Rost (Weinheim: Beltz, Psychologie VerlagsUnion), 309–312.
Woldeamanuel, Y. W., Abate, N. T., and Berhane, D. E. (2020). Effectiveness of concept mapping based teaching methods on grade eight students’ conceptual understanding of photosynthesis at ewket fana primary school, Bahir Dar, Ethiopia. EURASIA J. Math. Sci. Technol. Educ. 16, 1–16. doi: 10.29333/ejmste/9276
Young, J. Q., Van Merrienboer, J., Durning, S., and Ten Cate, O. (2014). Cognitive load theory: implications for medical education: AMEE Guide No. 86. Med. Teach. 36, 371–384. doi: 10.3109/0142159X.2014.889290
Keywords: biology education, concept maps, metacognition, learning, performance, training
Citation: Lenski S, Elsner S and Großschedl J (2022) Comparing Construction and Study of Concept Maps – An Intervention Study on Learning Outcome, Self-Evaluation and Enjoyment Through Training and Learning. Front. Educ. 7:892312. doi: 10.3389/feduc.2022.892312
Received: 08 March 2022; Accepted: 23 May 2022;
Published: 20 June 2022.
Edited by:
Muhammet Usak, Kazan Federal University, RussiaReviewed by:
Taylor Wayne Acee, Texas State University, United StatesCopyright © 2022 Lenski, Elsner and Großschedl. This is an open-access article distributed under the terms of the Creative Commons Attribution License (CC BY). The use, distribution or reproduction in other forums is permitted, provided the original author(s) and the copyright owner(s) are credited and that the original publication in this journal is cited, in accordance with accepted academic practice. No use, distribution or reproduction is permitted which does not comply with these terms.
*Correspondence: Jörg Großschedl, amdyb3Nzc2NAdW5pLWtvZWxuLmRl
†ORCID: Sina Lenski, orcid.org/0000-0001-6379-0941; Stefanie Elsner, orcid.org/0000-0001-7346-8008; Jörg Großschedl, orcid.org/0000-0002-7943-4818
‡These authors share first authorship
Disclaimer: All claims expressed in this article are solely those of the authors and do not necessarily represent those of their affiliated organizations, or those of the publisher, the editors and the reviewers. Any product that may be evaluated in this article or claim that may be made by its manufacturer is not guaranteed or endorsed by the publisher.
Research integrity at Frontiers
Learn more about the work of our research integrity team to safeguard the quality of each article we publish.