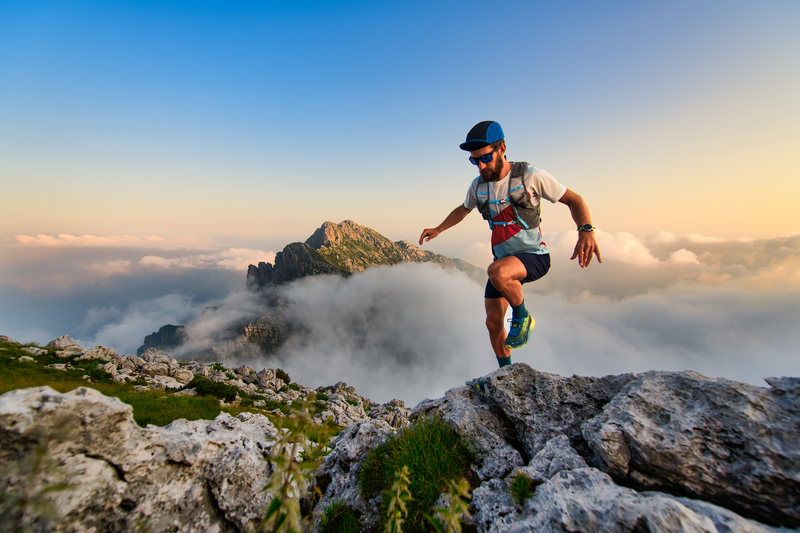
94% of researchers rate our articles as excellent or good
Learn more about the work of our research integrity team to safeguard the quality of each article we publish.
Find out more
ORIGINAL RESEARCH article
Front. Educ. , 20 September 2022
Sec. Higher Education
Volume 7 - 2022 | https://doi.org/10.3389/feduc.2022.881449
Students of color, particularly women of color, face substantial barriers in STEM disciplines in higher education due to social isolation and interpersonal, technological, and institutional biases. For example, online exam proctoring software often uses facial detection technology to identify potential cheating behaviors. Undetected faces often result in flagging and notifying instructors of these as “suspicious” instances needing manual review. However, facial detection algorithms employed by exam proctoring software may be biased against students with certain skin tones or genders depending on the images employed by each company as training sets. This phenomenon has not yet been quantified nor is it readily accessible from the companies that make this type of software. To determine if the automated proctoring software adopted at our institution and which is used by at least 1,500 universities nationally, suffered from a racial, skin tone, or gender bias, the instructor outputs from ∼357 students from four courses were examined. Student data from one exam in each course was collected, a high-resolution photograph was used to manually categorize skin tone, and the self-reported race and sex for each student was obtained. The likelihood that any groups of students were flagged more frequently for potential cheating was examined. The results of this study showed a significant increase in likelihood that students with darker skin tones and Black students would be marked as more in need of instructor review due to potential cheating. Interestingly, there were no significant differences between male and female students when considered in aggregate but, when examined for intersectional differences, women with the darkest skin tones were far more likely than darker skin males or lighter skin males and females to be flagged for review. Together, these results suggest that a major automated proctoring software may employ biased AI algorithms that unfairly disadvantage students. This study is novel as it is the first to quantitatively examine biases in facial detection software at the intersection of race and sex and it has potential impacts in many areas of education, social justice, education equity and diversity, and psychology.
Retaining and graduating students of color in STEM fields is a high priority for many institutions. Students of color still comprise a strong minority in many STEM fields. For example, though Black students make-up ∼11% of the bachelor’s degrees earned from 4-year colleges and universities, they only comprise ∼9% and ∼4% in science and engineering fields, respectively (National Center for Science and Engineering Statistics, 2021). The barriers faced by students of color are many-fold. First, students of color are often socially isolated from peers and fail to have a sense of belongingness that is common among their White counterparts (Ong et al., 2018). Second, students of color are subject to explicit and implicit biases, sometimes in the form of microaggressions, from peers and faculty that further demean and demoralize these students. Finally, universities can contribute to systemic biases that further marginalize students of color through policies designed to support White students, the presentation of non-inclusive materials, or the presence of racist representations (e.g., United States confederate statues). Further, universities can support systemic racism through policies that are not immediately obvious or thoroughly tested. One such example would be supporting the use of biased technologies. It is of the utmost importance that universities identify and cease the use of technologies that disadvantage women or students of color, especially for those technologies that relate to academic assessment or performance, such as test proctoring software.
Women also may still face barriers in higher education. Women comprise 57.1% of the total enrollment in United States colleges in 2020 (National Center for Science and Engineering Statistics, 2021) but only earn 34% of the STEM degrees. The lack of females in these fields can lead to feeling of isolation and a lack of retention (White and Massiha, 2016). This further bleeds into the retention of women in STEM fields post-graduation as well. Biases that women may encounter in higher education STEM settings include, but are not limited to, drops in self-confidence, feelings of isolation, lack of female mentors, and traditional stereotypes that suggest that women are not as good as men in technical or more rigorous curricula (Brainard and Carlin, 1997; White and Massiha, 2016). These intrinsic factors are experienced in conjunction with more implicit or subtle forms of bias against women, such as in technologies employed by universities. Therefore, it is of the utmost importance that universities identify technologies that disadvantage women or students of color, especially for those technologies that relate to academic assessment or performance, such as online proctoring software.
Automated exam proctoring software systems experienced a surge in usage worldwide as remote learning becomes more commonplace in higher education settings. These systems are designed to allow students to take exams remotely while maintaining the academic integrity of a test without the use of live proctors. These software employ artificial intelligence (AI) or machine learning (ML) algorithms to monitor and record students during online exams and detect behaviors that may indicate cheating (e.g., a face is not detected, a second face is detected, or talking is detected). After exams, instructors are provided a report with a summary and details of suspicious, or flagged, behaviors as well as video recordings of students’ testing sessions. The report is intended to eliminate the need of the instructor to review the entirety of each video session for cheating by identifying specific portions of videos from the assessment of individual students for manual review by the instructor.
Despite the software’s potential utility, proctoring software built on AI or ML may come with high costs–particularly to students in demographic groups who already face barriers in higher education, including STEM fields (National Center for Science and Engineering Statistics, 2021). A widely known concern with AI and ML algorithms is the issue of fairness and algorithmic bias (Cramer et al., 2018; Turner Lee, 2018; Amini et al., 2019; Bird et al., 2019). If left unchecked, algorithms can have unequal, negative impact on individuals from historically disadvantaged groups and can perpetuate systemic biases (Barocas and Selbst, 2016). Amazon.com’s résumé review system that treated women as ineligible for tech positions (Dastin, 2018) and an automated Twitter account that learned and perpetuated stereotypes in its posts (Fosch-Villaronga et al., 2021) are two well-known examples in which automated systems discriminated against certain groups.
Algorithms that process facial images do so for the purpose of identifying a person (i.e., facial recognition), or identifying a human (i.e., facial detection). These algorithms compare an image to a data set (e.g., training images of faces) and are unfortunately fraught with error. In fact, a recent study of 189 commercially available automated face recognition systems conducted by the National Institute of Standards and Technology showed that majority of systems produced differential error rates across demographic groups based on age, gender, and race (Grother et al., 2019). For many of the systems, the lowest accuracy (i.e., higher false positive rates) were observed for those with the darkest skin tones and for women. Another study found unequal performance on gender classification such that systems were most accurate for White males and least accurate for Black females (Buolamwini and Gebru, 2018). Moreover, it has been demonstrated anecdotally that an automated face detection system can fail to detect a face with darker skin (Sandvig et al., 2016). However, most of these studies focus on systems trained to recognize faces (i.e., facial recognition) or classify images based on characteristics, rather than simply detecting the presence of a face at all. Tackling this potential issue with exam proctoring software in higher education, which has the potential to impact thousands of students, and generating quantifiable data on whether biases in exam proctoring software exist, therefore, becomes of the utmost importance.
Whether there is bias in exam proctoring software is a particularly important question for several reasons. First, some colleges and universities have abandoned automated proctoring software due to concerns about fairness and privacy and in response to student pushback (Kelley, 2020; Chin, 2021). Concerns have also resulted in Senatorial inquiries into the industry (Blumenthal et al., 2020; Nash, 2020). Yet, colleges and universities need a fair, effective, and rigorous mechanism for remote testing (Chin, 2021) given the rise of online education and need for flexibility in testing methods, as was seen on a large scale during the COVID-19 pandemic (Sandvig et al., 2016; Grother et al., 2019). Second, underrepresented students already face many barriers and challenges in higher education, including a lack of representation at college and in many fields of study (National Center for Science and Engineering Statistics, 2021), stereotypes depicting inferior academic performance [i.e., stereotype threat, (Pennington et al., 2016)], microaggressions, and health disparities (Lipson et al., 2018), to name a few. Additional harm could be inflicted on historically marginalized, underrepresented students if the testing software disproportionally “flags” them as being at high risk for cheating more often than their lighter-skinned peers (Buolamwini and Gebru, 2018; Kelley, 2020).
Therefore, it is imperative that instructors examine and continually test the products used in their classrooms for bias, especially when proctoring software companies fail to provide data on this very topic or to conduct tests on their software in all areas where bias could be present. In the present study, we investigated whether skin tone, race, sex assigned at birth (gender was not assessed as this information was not available from the institution), or intersectional disparities were observed in facial detection software by evaluating the output of the automated exam proctoring software predominantly used at our university during the Fall 2020 semester. Data were collected from four large, STEM courses and analyzed for a variety of outputs given by the automated test proctoring software. The research described in this study reflects the experience of the instructor when analyzing the outputs from this type of software, rather than the student experience, but instructor reports are the basis for where biases may be first observed and where accusations of cheating may arise.
The automated test proctoring software evaluated in the present study, Respondus Monitor, is a commercially available software solution commonly used by colleges and universities. At the time of the present study, it was the primary automated proctoring software used by our university. The software uses proprietary AI and ML algorithms to monitor students’ behavior in real time during the testing session and flags anomalous behaviors. Red flags are produced for general anomalous events detected, such as loss of internet connection or video frame rate lowered due to quality of internet connection, as well as events or behaviors that could be indicative of cheating, such as a student missing from the frame or a different person in the frame. Based on the red flag data, the software’s algorithm computes a “priority score” for each student that indicates relative likelihood of cheating. At the end of each testing session, the software provides an instructor report that includes details about each student’s session. The report consists of students’ names, pictures and videos from the session, general information about the exam session such as time in exam, whether an internet interruption occurred or the video frame rate was the lowered due to quality of internet connection, as well as indicators of possible cheating including priority score and red flag data.
Data collected from the instructor report for this study included: a high-resolution image of each student captured during the initiation sequence for skin tone analysis (below), total time in test, priority score (high, medium, or low), total number of flags, total flagged time, and percent of time during the assessment that there was a face detected (facial detection%). The number of different flagged events including “missing from frame,” “different person in frame,” “failed facial detection check,” “student turned off facial detection alerts,” “an internet interruption occurred,” “low facial detection,” and “video frame rate lowered due to quality of internet connection” were also included in the study (Supplementary Table 1). In addition, for a set of exploratory analyses, videos of students’ testing sessions were viewed and coded by the researchers for possible cheating behaviors, internet interruptions, environmental distractions, and lighting issues.
Student skin tone was classified by comparing the digital high-resolution image of each student, primarily the forehead, cheeks, and area underneath the nose when visible, to the expanded Fitzpatrick skin tone scale Previously published skin tone classifications, including the original Fitzpatrick Scale, which is based on six broad color categories, have been recently criticized for failing to have enough differentiation in darker skin tones (1). Therefore, to obtain more accurate classifications, we used an expanded version of the Fitzpatrick Scale, which includes multiple tonalities within each category (see Supplementary Figure 1).
A trained researcher, who identifies as a person of color, initially classified all the student skin tones on a scale of 1–6. A second trained researcher, who identifies as White, classified 40 randomly selected de-identified images for reliability purposes on a different computer (due to COVID-19 isolation). The two researchers indicated the exact same skin tone categories 47% of the time and were within a single classification 97.5% of the time, with most discrepancies in categories labeled 3 and 4. Because interrater reliability was low at the precise level, skin tone classifications were merged into 3 skin-tone groups as follows: Darker (groups 1 and 2, n = 41), Medium (groups 3 and 4, n = 187), and Lighter (groups 5 and 6, n = 129).
Sex assigned at birth data for each student was obtained from the University of Louisville’s Office for Academic Planning & Accountability. Gender identity information is not collected by the university. One hundred twenty-four students self-described their sex assigned at birth as male and 233 students indicated female. There were no intersexed or other sexes indicated in this dataset. These classifications were combined with skin tone classifications to generate the intersectionality data. There were 24 females and 17 males with the darker skin tones, 120 females and 67 males with medium skin tones, and 89 females and 40 males with the lightest skin tones. Race was not used in intersectionality data due to low numbers in some groups which reduced the power of the statistical analyses. Sex and intersectionality data was analyzed as described above for skin tone and race using similar methods.
At the time of this analysis, only 298 videos remained available to the researchers (due to students either graduating or leaving the university–their data is expunged from the software when this happens) so all 298 videos were coded. When students had multiple videos, all videos were assessed. The environmental check videos were used to estimate the type of computer used for the video(s). Student lighting was assessed during multiple points in the video(s) and coded as back-lit, side-lit, front-lit, top-lit, or bottom-lit, though no student was coded as bottom-lit and this category was later removed from analysis. Videos were then analyzed for evidence of cheating. This was categorized as the presence of another screen, students looking off camera repeatedly, students talking to someone else about the exam specifically, etc. If the reviewer was not sure, this was noted but marked as no cheating. Videos were also coded for noise interruptions by listening at multiple points in the video for interior or exterior noises (e.g., television noise or ambulance sirens, respectively) or the observation of someone else in the room. Videos were also coded for whether the student left the field of the camera, looked down or looked up at any point in the video and this was coded as fully missing from frame. Those students that obscured part of their face with their hands, water bottles, clothing, etc., or those that moved their face partially out of frame (but not entirely) were coded as partially missing from frame. The total number of times the students were fully or partially missing from frame was not quantified and thus direct comparisons to the exam proctoring flags were not analyzed. Intersectional analyses were also not completed due to a lack of statistical power.
All statistical analyses were run in GraphPad Prism v 5.04 and selected datasets were verified in SPSS. All data sets were checked for normality and none fit a normal distribution. Therefore, only non-parametric tests were used. One-way ANOVAs (Kruskal Wallis) were run and Dunn’s post-test pairwise comparisons between all pairs of data sets were used for the indicated analyses. Chi-square tests with a 95% confidence interval were used on categorical data from the analysis of student videos.
After a student completes an assessment, the automated proctoring software generates an analysis report that includes various metrics about the exam itself and summarizes the total flagged data. Additionally, the output also presents a high-resolution image students take at the beginning of the exam; thumbnails of timespoints in the video recording; and a timeline of the assessment with flagged time shown. For each video, the software then produces a priority score, which summarizes their proprietary algorithm analysis to categorize the captured recording as high (needs review), medium (may need review), or low (may not need review). Videos with high priority scores are moved to the top of the page to emphasize their importance to the instructor.
To assess whether students with darker skin tones faced an algorithmic bias, we first assessed skin tone classifications and the summative priority score for each student. Overall, most students had low priority scores with 293 of 358 students having a low priority for review (82%). Overall, there were fewer students in the medium (19, 5%) or high (46, 13%) priority score categories. However, students that had darker skin tones were significantly more likely to have medium or high priority scores than the students in the groups with medium or lighter skin tones (p < 0.05, Figure 1A). Further, students with medium skin tones were also more likely to be flagged as medium or high priority compared to students with lighter skin tones though these differences were not significant (p > 0.05). Descriptive statistics on the data can be found in Supplementary Table 2. This metric was also examined for bias based on race. As shown in Panel 1B, there were significant differences in the median values between the 5 well-represented racial datasets (p = 0.0038). Pairwise analysis between the different racial datasets revealed a significant difference only between Black students and White students, whose means were 1.60 vs. 1.23, respectively (p < 0.01).
Figure 1. Overall metrics by the automated test proctoring software based on skin tone classification or self-reported race. (A,B) Priority scores based on skin tone classification are shown with priority scores of 1, 2, and 3 indicating low, medium, and high priority for review. (C,D) The total number of flags given to a student during the assessment. (E,F) The total amount of time during the assessment the student was flagged for any reason. (G,H) Percent of the assessment students spent being flagged by the AI algorithm. (A,C,E,G) Show the data based on the skin tone analysis. (B,D,F,H) Show the data based on student’s self-reported race. Box plots represent 25 and 75% confidence intervals with whiskers represent Tukey’s distributions and dots represent data outliers. Lines inside the boxes represent the median values and + indicate the mean values. Note the divided y-axis scales on some plots to accommodate the wide range of values. Panels (A,B) Chi-squared p-values shown. Panels (C–H)–Kruskal Wallis non-parametric tests yielded an overall p-values indicated on each plot and Dunn’s post-test pairwise comparisons shown with brackets (*p > 0.05, **p < 0.01, ***p < 0.001).
The total number of flags were collected from each automated proctoring video output. Flags are given for a variety of reasons including student faces disappearing from the frame, multiple people in the video frame, internet interruptions, video frame rate reductions, etc. We chose to analyze several of these individually but also chose to examine the data in aggregate as well. We compared the number of collective flags for each student and their skin tone classification. Students with darker skin tones were more likely to have a higher number of flags on average (Figure 1C) but there are also significant differences between all three skin tone classifications. Students with darker skin tones had, on average, 6.07 flags per assessment which was more than twice that of students with medium and lighter skin tones had 1.91 and 1.19 flags/assessment, respectively (Supplementary Table 1). Using race data, once again, the pairwise data analysis revealed significant differences in the number of flags given to Black students compared to White students (p < 0.05, Figure 1D). Other racial pairwise comparisons did not significantly differ in their mean values.
Another metric from the automated proctoring software is the total amount of time during the assessment that the student was flagged. This is expressed in minutes. Students whose face is not detected, therefore, should spend more of their assessment being flagged. We assessed whether students with darker skin tones were flagged for long periods of time than students with lighter skin. Students with darker skin tones were flagged for significantly longer periods than lighter skin students (p < 0.0001) (Figure 1E and Supplementary Table 1). Using racial data, similar results were observed in that there were significant differences in the medians for the racial groups (p = 0.0037) and, as before, when examining the pairwise comparison of individual groups, the only statistical significantly different groups were Black students (mean: 2.80 min, Supplementary Table 2) and White students (mean: 0.85 min).
Perhaps these data reflect differences in the amount of time taken for the assessments by each student. In fact, there were small but significant differences in the amount of time that students of different skin tone category or race took on the assessments (Supplementary Figure 2 and Supplementary Tables 1, 2). As assessments analyzed varied in length both from a class standpoint and from a student standpoint, the total flagged time was normalized for each student based on the time they had taken for the assessment to generate a percent time flagged variable to determine whether students with darker skin tones spent more of their time being flagged was assessed. Even with this normalized data, students with darker and medium skin tones were significantly more likely to be flagged for a greater percentage of their assessment compared to their White peers (Figure 1G and Supplementary Table 2). Students with the darkest skin tones spent on average 7.64% of their time being flagged by the software compared to 3.33 or 1.56% for students with medium or lighter skin tones, respectively. Similarly, when these outputs were examined and compared to student’s self-reported race, the mean values for all outputs were significantly different (p < 0.0001). In pairwise tests, students that reported their race as Black were more likely to be given a higher priority score (p < 0.01, Figure 1B), more flagged events (p < 0.05, Figure 1D), flagged for a greater amount of time and percent of time for their assessment (both p < 0.05, Figures 1F,H) compared to their White counterparts. We conclude from these data analyses that the automated proctoring software AI algorithms show a clear bias against students with darker skin tones and those that report themselves as Black.
The automated proctoring software estimates the amount of time each student’s face is detected during the exam and presents a percent facial detection metric to instructors. One hundred percent indicates that a student’s face was detected for the entire assessment. Therefore, we asked whether this metric was biased when correlated with student’s skin tones or race. Students with darker, medium, or lighter skin tones had their faces detected an average of 78, 87, or 92% of their assessment (Figure 2A and Supplementary Table 1). The median values for these groups were significantly different (p < 0.001, Figure 2A). As observed in the previous analyses, Black students, in particular, seem distinctly less recognized than their White counterparts (p < 0.0011, Figure 2B and Supplementary Table 2).
Figure 2. Facial detection outputs from the automated test proctoring software based on skin tone analysis or self-reported race. (A,B) Percent of time a student’s face was detected during the assessment. (C,D) The number of missing from frame flags for each student. (E,F) Number of low face detection flags for each student are shown. (A,C,E) Show the data analyzed based on skin tone categories while (B,D,F) show the data based on self-reported race. Box plots represent 25 and 75% confidence intervals with whiskers represent Tukey’s distributions and dots represent data outliers. Lines inside the boxes represent the median values and + indicate the mean values. Note the divided y-axis scales on some plots to accommodate the wide range of values. Kruskal Wallis tests yielded an overall p-values indicated on each plot with Dunn’s post-test pairwise comparisons shown (*p > 0.05, **p < 0.01, ***p < 0.001).
A very common behavior in students is to rest their hands on their arms or to slouch during an exam, potentially causing their face to only be partially observed by the software. Because this is also a behavior that may also be linked to cheating (e.g., they look down a great deal to look at notes or books), the automated proctoring software analyzes videos for times when student’s faces are not observed. If the algorithm also fails to recognize the faces of people with darker tones, then it is reasonable to hypothesize that students with darker tones will be more likely to receive “missing from frame” flags for their videos. We analyzed the number of missing from frame flags based on skin tone classification. Students with darker skin tones were flagged as missing from frame on average 4.79 times per assessment while students with medium and lighter skin tones were only flagged 1.39 times or 0.83 times, respectively (Figure 2C). This means that darker skin students were flagged >3 times more often than the medium skin tone students and almost 6 times more often than their lighter skin counterparts. For assessments in which the instructors allow the software to let students know when their face is missing from the frame and prompt the students to respond, these frequent notifications can be a significant disruption during an already stressful testing experience.
The automated proctoring software also has another specific type of flag called a “low facial detection” flag that is triggered when the software fails to detect a face for a “significant portion of time” during an assessment. In this dataset, this flagging event was fairly rare. Even so, students with darker skin tones were 2.5 times or 5.6 times more likely to receive a low facial detection flag than medium or lighter skin students correspondingly (p < 0.05 and p < 0.001, respectively, Figure 2E and Supplementary Table 2). Further, students with medium skin tones for this were 2.25 times more likely to be flagged compared to lighter skin students as well.
We also compared this lack of facial detection using race data for each student. As observed in Figures 2B,D,F, these metrics were significantly different for the races. Black students had a significantly lower percentage overall facial detection, more missing from frame flags, and more low facial detection flags compared to their White counterparts (p < 0.001, p < 0.05, p < 0.05, respectively) (Supplementary Table 3). These data further support that automated proctoring software algorithms are biased against detecting the faces of dark skin students, particularly those that are Black.
Many of the issues leading to flagging can also come from poor internet quality, which can lead to internet interruptions or frame rate reductions due to slow internet speeds. Internet interruptions and frame rate reductions cause the automated proctoring software algorithm to have trouble detecting faces due to pixilation of the video or missing segments of videos leading the video to be flagged for review. This may be related to socioeconomic status as students of color are less likely to have broadband internet access and are less likely to have a computer in their home than their White counterparts (Martin, 2021). Thus, these flags may represent a bias against students of lower socioeconomic status rather than directly against skin tone or race. To determine if students with darker skin tones were more likely to receive internet interruption flags or video frame rate reduction flags (which represents the quality of the internet connectivity), we assessed the number of these flags by skin tone classifications. We note here that these flagged events were rare in our dataset. In fact, the automated proctoring software outputs for students with low internet speed were more likely to have no video recorded at all (and thus not included in our dataset) as opposed to individual flags based on low internet connection. In this way, the number of flags due to internet connections was not able to be studied fully.
With the remaining data that could be examined, we observed that the number of internet interruptions was only slightly increased in students of darker skin tones, but it was still significantly greater than students with medium or lighter skin tones (p < 0.05, Figures 2A,C and Supplementary Table 2). Conversely, the number of video frame rate reductions was not significantly different for any color classification. Using race data, internet interruptions and video frame rate reduction flags were not significantly different based on race (Figures 3B,D and Supplementary Table 3). Taken together, this implies that internet disruptions are unlikely to significantly contribute, if any, to the automated proctoring software output discrepancies between students with different skin tones or races.
Figure 3. Internet issues such as interruptions or low speeds outputs from the automated test proctoring software based on skin tone analysis or self-reported race. (A,B) The number of internet interruption flags given to students. (C,D) The number of video frame rate reduction flags for each student. (A,C) Show the data analyzed based on skin tone categories while (B,D) show the data based on self-reported race. Box plots represent 25 and 75% confidence intervals with whiskers represent Tukey’s distributions and dots represent data outliers. Lines inside the boxes represent the median values and + indicate the mean values. Note the divided y-axis scales on some plots to accommodate the wide range of values. Kruskal Wallis tests yielded an overall p-values indicated on each plot with Dunn’s post-test pairwise comparisons shown with brackets (*p > 0.05).
Automated facial recognition software has also been shown to be biased against women, particularly women of color (Buolamwini and Gebru, 2018). To determine if there was bias in the automated test proctoring software algorithms, we obtained the self-reported sex at birth for each student in the dataset and conducted similar analyses to those described for skin tone and race. Our institution does not currently collect gender or gender identity information from students. All 357 students in the dataset self-reported their sex as male or female. The analysis showed that there were no significant differences based on sex in most of the metrics collected including priority score, number of flags, total flagged time, total time taken, percent time flagged, number of missing from frame, face detection, video frame reduction, or internet interruption flags (Figures 4, 5, Supplementary Figures 3, 4 and Supplementary Table 4). The one exception was for percent facial detection in which males were significantly less likely to have their faces recognized (p < 0.05). The mean, standard deviation, median, and ranges for all values for this analysis can be found in Supplementary Table 3.
Figure 4. Overall metrics by the automated test proctoring software based on sex and intersectional data of sex and skin tone. (A,B) Priority scores based on sex or skin tone classification and sex are shown with priority scores of 1, 2, and 3 indicating low, medium, and high priority for review. (C,D) The total number of flags given to a student during the assessment. (E,F) The percent of the assessment students spent being flagged by the AI algorithm. (A,C,E) Show the data based on the self-reported sex of the students. (B,D,F) Show the data based on student’s self-reported sex and skin tone classification. F, female; M, male. Box plots represent 25 and 75% confidence intervals with whiskers represent Tukey’s distributions and dots represent data outliers. Lines inside the boxes represent the median values and + indicate the mean values. Note the divided y-axis scales on some plots to accommodate the wide range of values. Kruskal Wallis non-parametric tests yielded an overall p-values indicated on each plot and Dunn’s post-test pairwise comparisons shown with brackets (*p > 0.05, **p < 0.01, ***p < 0.001).
Figure 5. Face detection metrics by automated test proctoring software based on sex and intersectional data of sex and skin tone. (A,B) The percent facial detection metric (C,D) the number of missing from frame flags given to a student during the assessment, and (E,F) the number of face detection flags for each student are shown. (A,C,E) Show the data based on the self-reported sex of the students. (B,D,F) Show the data based on student’s self-reported sex and skin tone classification. F, female; M, male. Box plots represent 25 and 75% confidence intervals with whiskers represent Tukey’s distributions and dots represent data outliers. Lines inside the boxes represent the median values and + indicate the mean values. Note the divided y-axis scales on some plots to accommodate the wide range of values. Kruskal Wallis non-parametric tests yielded an overall p-values indicated on each plot and Dunn’s post-test pairwise comparisons shown with brackets (*p > 0.05, **p < 0.01, ***p < 0.001).
We next considered the intersectionality of the student population. We assessed the automated outputs based on sex and skin tone analysis. Race was not studied because small group size led lack of statistical power for some of the groups; instead, skin tone was used with sex to assess potential biases in the dataset. In almost all metrics, females with darker skin tones were flagged more often and given higher priority scores compared to lighter skin males and often lighter skin females (Figures 4, 5 and Supplementary Figure 3). For example, darker skin females were 4.36 times more likely to be flagged overall than medium skin tone females (p < 0.05) and 5.6 times more likely to be flagged than lighter skin females (p < 0.001) (Figure 4B and Supplementary Table 5). Similarly, women with darker skin tones had on average 78% facial recognition while women with medium or lighter skin tones had 88 or 92% average facial recognition. Men with darker, medium, or lighter skin tones had 81, 87, or 94% facial recognition values, respectively. In fact, in most cases, male students with darker skin tones did not face the same obstacles as female students with similar skin tones based on the statistical analysis though the mean values are quite different. Female students with medium skin tones too were flagged more often than their lighter skin counterparts as were male students with medium skin tones. When examining metrics that indicate the quality of internet speeds, there were no significant differences between the groups in terms of the number of flags given for video frame rate reductions (p > 0.05) but female students with the darker skin tones were significantly more likely to receive flags for internet interruptions compared to all other groups (p = 0.0008) (Supplementary Figure 4), and, perhaps, this could account for some of the increased flagging but cannot account for all of it as skin tone and racial data does not show significant biases for internet interruptions (Figure 3). The mean, standard deviation, median, and ranges for all values for this analysis can be found in Supplementary Table 5. Taken together, female students with the darkest skin tones face the greatest bias when using this automated test proctoring software, much more than their male counterparts, and students with medium skin tones were also at a disadvantage compared to their lighter skin counterparts. These data further underscore the need for test proctoring software companies to include more women with dark skin tones in their training sets.
One might ask whether students of color were more likely to cheat, have low internet connections, be interrupted, or have lighting issues that caused the proctoring software to flag their videos differentially; thus, maybe the proctoring software is not really biased. We analyzed 298 student videos (83%) for indications of biases in any of these metrics to determine whether the differential patterns we observed were the result of algorithmic bias, actual student behaviors, or environmental differences.
We first assessed cheating behaviors and found no significant differences based on skin tone, race, or sex suggesting that cheating itself cannot underlie any algorithmic bias (Figures 6A,D,G). In fact, of the 12 videos in which the examiners identified actions that they considered behaviors indicative of possible cheating, none were recordings of Black students. Students that displayed possible cheating behaviors were predominantly White (n = 9), in the medium skin tone category (n = 10), and split by sex (m = 5, f = 7), but these behaviors were too rare to provide significant statistical power. The priority scores for these students were predominantly in the Low category (n = 8; Medium, n = 2; High, n = 2). These 12 students had a median number of flags of 2 (range: 0–57), and a median total time flagged of 0.225 min (range: 0–41 min).
Figure 6. Analysis of student videos for student behaviors. Videos of students were coded for potential cheating behaviors (A,D,G), whether interruptions of the videos from inside/outside noises or other people in the same room were observed (B,E,H), and whether students were either fully missing from the frame (e.g., walked away), partially missing from the frame (e.g., covered part of their face, leaned into the camera so that part of the face was missing) at any point in the video (C,F,I). Percentages are shown in each bar and are color-coded for easy comparisons. Chi-squared values from raw data is shown in boxes at the top of each graph. N.D. = not determined due to 0 values in the dataset which did not comply with Chi-squared analysis.
We examined the videos for other behaviors that may have led to flagging events. We observed the recordings for interruptions and noises that may have triggered the exam proctoring software to flag a video. While noises were common (>10% of students had some sort of internal or external noises observed), there was no differential patterns for skin tone, race, or gender (Figures 6B,E,H). Students often moved around during their assessments, and this led to some students obscuring their faces during the video. Many students leaned their heads on their hands, wiped their hands across their faces, or moved so close to the screen that their chin, mouth, and sometimes their noses were not visible, thus making it hard for the facial detection algorithm to recognize a face. Some students also went offscreen during the assessment. However, none of these behaviors either seemed to be significantly different for students with different skin tones, from various races, or based on sex (Figures 6C,F,I). Taken together, these data suggest that student behaviors do not underlie any algorithmic biases observed in the exam proctoring outputs.
Perhaps the students’ environments varied significantly causing the discrepancy in flagging of students from different groups. We assessed the type of computer/camera the students used as this could correlate with lowered video frame rates due to connectivity, the position of students’ faces in the video. Laptops were, by far, the most common type of computer used. There appears to be no significant differences in laptop/desktop usage between students of different skin tones (p = 0.3125), races (p = 0.2756), or sex (p = 0.0723) (Figures 7A,C,E) suggesting that this is not a reason for potential biases. We further analyzed the videos for the type of lighting each student used for their assessments. The exam proctoring software even recommends that students take their exams in well-lit rooms and avoid backlighting for the best facial detection. Students were coded on where the light in the videos came from–either the back, side, front, bottom, or top and all light sources were included in the dataset. We observed a small but significant difference in the distribution of light sources (p = 0.0322) (Figure 7B) with students with darker and medium skin tones being more likely to be lit from the back or side and students with lighter skin tones being more likely to be front-lit. This difference in lighting could contribute to biases observed in the exam proctoring software as having darker or medium skin tones in a backlit setting will make it harder for the facial detection algorithms to detect a face. However, because the significance of this pattern is so small, it may not explain the strong differences observed in the flagging patterns and priority scores (Figures 1–5). In fact, the data does not show a correlation between flagging events and location of light sources for the general student population used in this study (χ2 p = 0.2332) suggesting that this problem is specific to certain skin tone groups only whole. Further, we saw no significant differences in lighting when considering different races (p = 0.6141) (Figure 7D) or sex (p = 0.8995) (Figure 7F) which then cannot explain the significant differences observed between Black and White students in terms of facial detection (Figure 2).
Figure 7. Analysis of student videos for student environments. Videos of students were coded for the type of camera/computer used for the exam (A,C,E), and what type of lighting was used for the exam (B,D,F). For the latter, students could be lit from multiple spots (e.g., from the side and from the computer screen) and this was coded as both when applicable. Percentages are shown in each bar and are color-coded for easy comparisons. Chi-squared p-values from raw data is shown in boxes at the top of each graph.
Understanding the explicit and implicit biases faced by students is critical to ensuring that students from underrepresented groups are given equal educational opportunities as lighter skinned students. The same should be said for women in higher education. This holds true for student retention, particularly those in STEM fields, in which students of color are more likely to change fields/majors during their academic career or leave their university compared to their White counterparts (Riegle-Crumb et al., 2019). In this work, we found significant algorithmic biases against students with darker skin tones, Black students, and female students with darker skin tones. Demographic disparities were found across multiple measures in the software for key metrics related to detecting cheating behaviors, including loss of facial detection, number of red flags, and priority scores although there were no significant differences observed in actual cheating patterns based on skin tone, race, or sex. The fact that disparities found were in measures related to detecting cheating, but we did not observe actual differential rates of cheating, raises concerns about the effects of algorithmic bias in test proctoring software on certain groups, in particular students from historically marginalized groups who already face barriers to success in higher education and in STEM fields. While this data and these conclusions may not be novel to those in the algorithmic bias field, anecdotally it is certainly surprising and disheartening for those faculty members outside of this field, many of whom are unaware that these biases exist in software they use regularly in their classrooms.
Another interesting significant observation from the data in this dataset goes against conventional observations–that men’s faces are recognized more easily than women’s faces (Buolamwini and Gebru, 2018). This is presumably the result of the training sets used by software companies which traditionally contained more images and videos with men than women. In our dataset, we observed that males were slightly less likely to have their faces recognized by the test proctoring software compared to women (Figure 4). This trend appears to be primarily driven by those students with medium skin tones (Figure 5B). Whether this is due to a difference in the number of students that self-reported as males (138) vs. females (233), and thus a bias in the dataset, or is due to true biases in the test proctoring software remain to be seen. But it is interesting to note that perhaps the company behind the software tested in this study may be trying to rectify sex biases in their facial detection algorithms based on this data.
The institutions contracting with companies producing test proctoring software should play an active and major role in training faculty and students about the benefits and limitations of such software. Toward this, universities and accrediting boards should implement mandatory training for faculty that want to use automated proctoring software and make recommendations to faculty to reduce cheating and bias. Further, institutions should also implement implicit bias training for faculty to inform those that use these types of software. However, faculty training does nothing to mitigate the negative experience of having increased scrutiny by the proctoring software on the students themselves. A plethora of studies indicate the academic performance of marginalized students suffer whenever they are reminded of their marginalized status before an exam or other assessment. Stereotype threat is one of the most robust and replicated phenomenon in social psychology (Derks et al., 2008; Schmader et al., 2008; Pennington et al., 2016). Students who are made aware of their membership in a stigmatized group before academic assessment tend to underperform because of the anxiety caused by the fear of confirming the negative performance expectations of their group. Students of color already face an abundance of stereotype threats which can affect overall performance and retention in higher education (Spencer et al., 2016). In addition to impacting the academic performance, engagement in the courses, and retention within a program or within a university by students of color, the physiological responses to the stress of perceiving that they are being treated differently from their peers may negatively impact the general health of these students (Steele and Aronson, 1995; Aronson et al., 2002; Harrell et al., 2011; Whaley, 2018). Therefore, training faculty and instructors on potential biases in automated algorithms that are based on facial detection is not sufficient.
One recommendation made by test proctoring companies to avoid bias in results is for students with darker skin tones to have additional lighting trained on them during the assessment. This recommendation holds true with the data presented in this study in that students with darker skin tones were more likely to be back- or side-lit. However, this requirement automatically informs Black, Indigenous, and people of color (BIPOC) students that the software differentiates between skin color/tone and becomes a stressful reminder of their stereotyped academic inferiority. The injunction that faculty should view videos of darker skinned students more closely to compensate for the false positive cheating flags of these students by the software means that these students are scrutinized more closely than their lighter skinned peers. Consequently, the use of these types of automated test proctoring software can be an automatic trigger of stereotype threat in darker skinned students and result in subpar exam performance as well as potentially subconsciously confirming implicit biases in faculty who see students of color flagged more frequently. According to the concept of stereotype threat, darker skinned students infer from these experiences that their group membership is in a group with a history of substandard academic performance and this group membership overrides their personal ability (Beasley and Fischer, 2012). Stereotype threat can lead to darker skin students leaving higher education because of feelings of alienation and lack of belongingness and contribute to the achievement gap between equal education and marginalized students (Steele and Aronson, 1995; Aronson et al., 2002; Aronson and Inzlicht, 2004). It may also be more stressful to students when a light is shining directly on their faces (Petrowski et al., 2021). Thus, it represents a real and substantial harm to the students and academic institutions that are working hard on retaining BIPOC students.
This study is not without its limitations. It should be noted that the software tested in this study is reflective of the version available at the time the study was conducted (Fall 2020) and represents only one particular automated test proctoring software on the market. While usage of this software is common, it is not clear whether these biases are also found in other, similar products. It seems like many, if not all, automated test proctoring software companies will have biases for which type of faces are detected as they continue to improve their software. This company may have also made updates to their training sets after the Fall 2020 semester, perhaps to include more diversity in their training set. The authors would also like to note that this study was solely focused on the outputs of this proctoring software. No information was collected about whether the students in this study faced discrimination or bias based on these results at the hands of their instructors. We also did not collect information from instructors about whether they used these outputs to report students for cheating. Further, this study only examined the outputs for four, large, STEM courses with 357 students whose data was included. If more student outputs were examined, the effects could be different (either more or less biased). At the institutional level, we found that this software was never the “primary” means by which instructors identified reported cheating behaviors based on a report generated by the Office of Academic Planning and Accountability at the University of Louisville. Instead, it was used substantiating evidence for the alleged cheating instances in only three situations. In contrast, anecdotal evidence from instructors of these courses and others indicate that the outputs from these types of software are often used as a primary means of identifying cheating behaviors but these are rarely, if ever, reported to the academic grievance committees/offices at the college level for a variety of reasons. Using this type of software to confirm potential cheating and as a cheating deterrent rather than as a screen of all students may mitigate some of the harmful effects of the algorithmic biases on students of color. In fact, given the priority flagging distribution of students identified as displaying behaviors indicative of probable cheating, deterrence rather than detection of cheating may be the most efficacious use of this type of software.
Future studies will focus on understanding other potential biases such as biases against gender, gender identity, or disabilities. For example, are there biases against non-binary students using these types of software? Our observations also included data from a few students with disabilities, such as vision impairments, which may require that they sit at different distances from their screens compared to non-disabled students. Obtaining data on these diverse, and often rare, student groups will require a much larger data set than the one examined here to achieve statistical significance and should be done across academic institutions as well. Additional follow-up studies will examine the effects of online exam proctoring on student’s perceptions, physical and mental health, and performance to assess the effect of stereotype threat, and if there is evidence of disparate outcomes in terms of instances of accused cheating, and overall mental health outcomes.
In summary, studies such as this one are critical to ensuring the highest standards in terms of being able to recognize diverse student populations and reducing systemic bias. Additionally, automated proctoring software companies should be forthcoming about the limitations of their software, produce data using real images from the field to continuously improve their products, and publish the results of their assessments. This will ensure that online testing software using automated proctoring treats students of color equally with their White counterparts and improves the integrity of the software, a new reality in higher education.
The original contributions presented in this study are included in the article/Supplementary material, further inquiries can be directed to the corresponding author/s.
The studies involving human participants were reviewed and approved by the University of Louisville Institutional Review Board. Protocol #20.0757. The patients/participants provided their written informed consent to participate in this study.
DY-H, AA, RH, and PH were responsible for the initial design and implementation of the research. DY-H, AA, KK, RH, PH, CC, RC, and TB were responsible for collecting the data. DY-H and CC were responsible for analyzing the data. DY-H, RH, PH, AA, ER, and CC were responsible for discussion of the data and writing the manuscript. All authors contributed to the article and approved the submitted version.
We are appreciative of the funding support received from the Office of Diversity, Engagement, Culture and Climate in the College of Arts and Sciences at the University of Louisville, who provided the publication fees for this article.
We would like to thank Sherrie Pawson, Alicia Dunlop, Mary Ledbetter, and Karen Chandler for their support and interactions on this research.
The authors declare that the research was conducted in the absence of any commercial or financial relationships that could be construed as a potential conflict of interest.
All claims expressed in this article are solely those of the authors and do not necessarily represent those of their affiliated organizations, or those of the publisher, the editors and the reviewers. Any product that may be evaluated in this article, or claim that may be made by its manufacturer, is not guaranteed or endorsed by the publisher.
The Supplementary Material for this article can be found online at: https://www.frontiersin.org/articles/10.3389/feduc.2022.881449/full#supplementary-material
Amini, A., Soleimany, A. P., Schwarting, W., Bhatia, S. N., and Rus, D. (2019). “Uncovering and mitigating algorithmic bias through learned latent structure,” in Proceedings of the 2019 AAAI/ACM conference on AI, ethics, and society (Honolulu, HI: Association for Computing Machinery). doi: 10.1145/3306618.3314243
Aronson, J., Fried, C. B., and Good, C. (2002). Reducing the effects of stereotype threat on African American college students by shaping theories of intelligence. J. Exp. Soc. Psychol. 38, 113–125. doi: 10.1006/jesp.2001.1491
Aronson, J., and Inzlicht, M. (2004). The ups and downs of attributional ambiguity: Stereotype vulnerability and the academic self-knowledge of African American college students. Psychol. Sci. 15, 829–836. doi: 10.1111/j.0956-7976.2004.00763.x
Barocas, S., and Selbst, A. D. (2016). Big data’s disparate impact. Calif. Law Rev. 104, 671–732. doi: 10.15779/Z38bg31
Beasley, M. A., and Fischer, M. J. (2012). Why they leave: The impact of stereotype threat on the attrition of women and minorities from science, math and engineering majors. Soc. Psychol. Educ. 15, 427–448.
Bird, S., Kenthapadi, K., Kiciman, E., and Mitchell, M. (2019). “Fairness-aware machine learning: Practical challenges and lessons learned,” in Proceedings of the 12th ACM international conference on web search and data mining (Melbourne, VIC: Association for Computing Machinery). doi: 10.1145/3289600.3291383
Blumenthal, R., Wyden, R., Van Hollen, C., Smith, T., Warren, E., and Booker, C. A. (2020). Official communication to Mr. Sebastian Vos, chief executive officer, EXAMSOFT. Washington, DC: U.S. Senate.
Brainard, S. G., and Carlin, L. (1997). “A longitudinal study of undergraduate women in engineering and science,” in Proceedings of the Frontiers in Education 1997 27th Annual Conference. Teaching and Learning in an Era of Change, (Pittsburgh, PA: IEEE). doi: 10.1109/FIE.1997.644826
Buolamwini, J., and Gebru, T. (2018). Gender shades: Intersectional accuracy disparities in commercial gender classification. Proc. Mach. Learn. Res. 81, 1–15.
Chin, M. (2021). University will stop using controversial remote-testing software following student outcry [Online]. The Verge. Available online at: https://www.theverge.com/2021/1/28/22254631/university-of-illinois-urbana-champaign-proctorio-online-test-proctoring-privacy (accessed June 28, 2021).
Cramer, H., Garcia-Gathright, J., Springer, A., and Reddy, S. (2018). Assessing and addressing algorithmic bias in practice. Interactions 25, 58–63. doi: 10.1145/3278156
Dastin, J. (2018). Amazon scraps secret AI recruiting tool that showed bias against women. Toronto, ON: Thomson Reuters.
Derks, B., Inzlicht, M., and Kang, S. (2008). The neuroscience of stigma and stereotype threat. Group Process. Intergroup Relat. 11, 163–181. doi: 10.1177/1368430207088036
Fosch-Villaronga, E., Poulsen, A., Soraa, R. A., and Custers, B. H. M. (2021). A little bird told me your gender: Gender inferences in social media. Inf. Process. Manage. 58:102541. doi: 10.1016/j.ipm.2021.102541
Grother, P., Ngan, M., and Hanaoka, K. (2019). Face recognition vendor test (FRVT). Part 3: Demographic effects. Gaithersburg, MD: National Institute of Standards and Technology. doi: 10.6028/NIST.IR.8280
Harrell, C. J. P., Burford, T. I., Cage, B. N., Nelson, T. M., Shearon, S., Thompson, A., et al. (2011). Multiple pathways linking racism to health outcomes. Du Bois Rev. Soc. Sci. Res. Race 8, 143–157. doi: 10.1017/S1742058X11000178
Kelley, J. (2020). Students are pushing back against proctoring surveillance apps [Online]. Electronic Frontier Foundation. Available online at: https://www.eff.org/deeplinks/2020/09/students-are-pushing-back-against-proctoring-surveillance-apps (accessed June 28, 2021).
Lipson, S. K., Kern, A., Eisenberg, D., and Breland-Noble, A. M. (2018). Mental health disparities among college students of color. J. Adolesc. Health 63, 348–356. doi: 10.1016/j.jadohealth.2018.04.014
Martin, M. (2021). Computer and internet use in the United States: 2018. A.C.S. reports. Suitland, MD: U.S. Census Bureau.
Nash, J. (2020). US senators ask online proctor firms for evidence they are fighting biometrics bias. Available online at: BiometricUpdate.com (accessed April 23, 2022).
National Center for Science and Engineering Statistics (2021). Women, minorities, and persons with disabilities in science and engineering. Alexandria, VA: National Center for Science and Engineering Statistics.
Ong, M., Smith, J. M., and Ko, L. T. (2018). Counterspaces for women of color in STEM higher education: Marginal and central spaces for persistence and success. J. Res. Sci. Teach. 55, 206–245. doi: 10.1002/tea.21417
Pennington, C. R., Heim, D., Levy, A. R., and Larkin, D. T. (2016). Twenty years of stereotype threat research: A review of psychological mediators. PLoS One 11:e0146487. doi: 10.1371/journal.pone.0146487
Petrowski, K., Buehrer, S., Niedling, M., and Schmalbach, B. (2021). The effects of light exposure on the cortisol stress response in human males. Stress 24, 29–35. doi: 10.1080/10253890.2020.1741543
Riegle-Crumb, C., King, B., and Irizarry, Y. (2019). Does STEM stand out? Examining racial/ethnic gaps in persistence across postsecondary fields. Educ. Res. 48, 133–144. doi: 10.3102/0013189x19831006
Sandvig, C., Hamilton, K., Karahalios, K., and Langbort, C. (2016). Automation, algorithms, and politics | when the algorithm itself is a racist: Diagnosing ethical harm in the basic components of software. Int. J. Commun. 10:19.
Schmader, T., Johns, M., and Forbes, C. (2008). An integrated process model of stereotype threat effects on performance. Psychol. Rev. 115, 336–356. doi: 10.1037/0033-295x.115.2.336
Spencer, S. J., Logel, C., and Davies, P. G. (2016). Stereotype threat. Annu. Rev. Psychol. 67, 415–437. doi: 10.1146/annurev-psych-073115-103235
Steele, C. M., and Aronson, J. (1995). Stereotype threat and the intellectual test performance of African Americans. J. Pers. Soc. Psychol. 69, 797–811. doi: 10.1037//0022-3514.69.5.797
Turner Lee, N. (2018). Detecting racial bias in algorithms and machine learning. J. Inf. Commun. Ethics Soc. 16, 252–260. doi: 10.1108/JICES-06-2018-0056
Whaley, A. L. (2018). Advances in stereotype threat research on African Americans: Continuing challenges to the validity of its role in the achievement gap. Soc. Psychol. Educ. 21, 111–137. doi: 10.1007/s11218-017-9415-9
Keywords: algorithmic bias, test proctoring, skin tone, facial detection, software
Citation: Yoder-Himes DR, Asif A, Kinney K, Brandt TJ, Cecil RE, Himes PR, Cashon C, Hopp RMP and Ross E (2022) Racial, skin tone, and sex disparities in automated proctoring software. Front. Educ. 7:881449. doi: 10.3389/feduc.2022.881449
Received: 22 February 2022; Accepted: 24 August 2022;
Published: 20 September 2022.
Edited by:
Amy Pittenger, University of Minnesota, United StatesReviewed by:
Stacie Craft DeFreitas, Prairie View A&M University, United StatesCopyright © 2022 Yoder-Himes, Asif, Kinney, Brandt, Cecil, Himes, Cashon, Hopp and Ross. This is an open-access article distributed under the terms of the Creative Commons Attribution License (CC BY). The use, distribution or reproduction in other forums is permitted, provided the original author(s) and the copyright owner(s) are credited and that the original publication in this journal is cited, in accordance with accepted academic practice. No use, distribution or reproduction is permitted which does not comply with these terms.
*Correspondence: Deborah R. Yoder-Himes, ZGVib3JhaC55b2Rlci1oaW1lc0Bsb3Vpc3ZpbGxlLmVkdQ==
Disclaimer: All claims expressed in this article are solely those of the authors and do not necessarily represent those of their affiliated organizations, or those of the publisher, the editors and the reviewers. Any product that may be evaluated in this article or claim that may be made by its manufacturer is not guaranteed or endorsed by the publisher.
Research integrity at Frontiers
Learn more about the work of our research integrity team to safeguard the quality of each article we publish.