- Institute of Educational Research, Johannes Gutenberg University of Mainz (JGU), Mainz, Germany
Cognitive student activation describes the mental stimulation of learners to engage in a deeper mental investigation of the subject matter within the learning context. Based on the “Angebots-Nutzungs-Modell” (Supply-Use Model), a lesson that is cognitively activating can be understood as a learning opportunity provided by the teacher, which learners can make use of based on their individual ability. This paper focuses on the side of the users, i.e., the cognitive activity of students during phases of collaborative work. Based on a videotaped English lesson of a 10th-grade class (N = 18), low- and medium-inference, indicator-based coding and rating systems were used to investigate the micro level of student-teacher and student-student interactions. The focus of the analysis was to examine triggers of student’s individual high cognitive activity during group work phases. After a basic coding to achieve a precise description of students activities, the level of cognitive activity in selected sequences was coded by a medium-inference rating system. Subsequently the sequences in which a student’s cognitive activity had previously been rated as high were assessed in a content analysis (Mayring, 2015) to determine what triggered a particular phase of high cognitive activity. Analyses show that the students are cognitively engaged at varying degrees and at different times. It is interesting to note that high cognitive activity is not necessarily interrelated with the frequency of verbal participation. Even students with little verbal participation several times exhibit high cognitive engagement. This can be attributed to different aspects and triggers: On the one hand, students are cognitively activated by working with the material and by stimuli provided by the teacher. On the other hand, they engage in cognitive activity among themselves by asking one another questions or by benefiting from the discussion between other group members.
Introduction
Cognitive activation, one of the three basic dimensions of teaching quality (Klieme et al., 2006), is regarded in the discourse on teaching quality in the German-speaking countries as a significant factor for initiating student learning processes (e.g., Klieme et al., 2001; Kunter and Trautwein, 2013; Fauth et al., 2019; Kleickmann et al., 2020; Praetorius et al., 2020b). Its effects are well documented empirically (e.g., Pauli et al., 2008; Lipowsky et al., 2009; Kunter and Voss, 2013; Fauth et al., 2014b). Existing studies, however, focus primarily on task types and teacher activities (e.g., Seidel et al., 2006; Pauli et al., 2008; Kleinknecht, 2010; Lotz et al., 2013). Video-based studies often offer overall judgments of cognitive activation potentials based on an entire lesson or teaching unit, but in general fail to examine cognitive activation and the resultant cognitive activity (Fauth and Leuders, 2018) at the individual level. However, this latter aspect is of special interest if one seeks to take the holistic nature of these learning processes into consideration (Praetorius and Charalambous, 2018). Based on the supply-use model1 (Helmke, 2012), therefore, the question is whether and how the impulses for cognitive activation (supply) are taken up and processed by the students (use).
The present paper focuses on the cognitive activity of students at the micro level of teacher-student and student-student interaction on the basis of a videotaped English lesson in the tenth grade. We observed individual students during phases of group work and focused on behaviors that allowed us to draw conclusions about their cognitive activity. Using low and medium inference coding and rating systems, we explore the question at what moments individual students exhibit high cognitive activity in the course of group work. Subsequently, we analyze the reasons why students engage particularly intensely with their subject at these specific moments, i.e., what the concrete triggers are that elicit individual cognitive activity.
Theoretical Background
Identifying the characteristics of high-quality teaching is a core concern of teaching research and has been addressed and intensely discussed in multiple studies (for summaries, Hattie, 2009; Scheerens, 2013). The three basic dimensions of teaching quality (Klieme et al., 2006) have been the focus of special attention in German speaking scientific community (Praetorius et al., 2018, 2020b; Kleickmann et al., 2020). This model groups generic aspects of effective classroom teaching into three basic dimensions: “classroom management,” “supportive classroom climate,” and “cognitive activation.” There is definite overlap between these dimensions and the three components of the internationally discussed Classroom Assessment Scoring System (CLASS) (Hamre and Pianta, 2010), which are defined as “emotional support,” “classroom organization,” and “instructional support” (Praetorius and Charalambous, 2018; Praetorius et al., 2020b). Parallels can also be drawn with other models of international classroom research, such as the Australian Quality Teaching Model, which comprises the three dimensions of “intellectual quality,” “quality learning environment,” and “significance” (Amosa et al., 2007), or the Dynamic Model of Educational Effectiveness (Creemers and Kyriakides, 2010), which is widely applied in international research.
While the international discourse uses various concepts—such as “higher order thinking” (Newmann, 1988), “thoughtful discourse” (Brophy, 2002), “instructional support” (Hamre and Pianta, 2010), or “deeper learning” (Meyer et al., 2018)—the concept of “cognitive activation” has gained general currency in Germany (Kunter and Voss, 2013; Praetorius et al., 2018). Within the framework of the model of the three basic dimensions of teaching quality, cognitive activation is the dimension that describes the stimulation of students to a deeper mental engagement with the subject at a level that is optimal for individual learning (e.g., Kunter et al., 2005; Hugener et al., 2009; Leuders and Holzäpfel, 2011; Lipowsky, 2020). This is achieved on the one hand by means of a complex task that challenges learners to develop an independent solution (Hugener et al., 2007; Kleickmann, 2012; Fauth and Leuders, 2018; Lipowsky, 2020). On the other hand, the teacher’s supervision of task completion is significant (Lipowsky et al., 2009; Kunter and Trautwein, 2013). The teacher plays an active role by, for example, triggering cognitive conflicts, emphasizing differences in ideas and attitudes, or encouraging students to relate their answers to one another (Lipowsky, 2020). Furthermore, cooperative learning settings are assumed to be effective in stimulating students’ cognitive activities. The juxtaposition of different opinions might provoke cognitive conflicts, so as the collaborative discussion encourages the students to build up new concepts or redefine existing beliefs. Similarly, the verbalization of questions and explanations can be an effective method of elaboration (van Boxtel et al., 2000; Kunter and Trautwein, 2013; Kyndt et al., 2013; Bleck and Lipowsky, 2021). According to a constructivist interpretation of teaching and learning processes, however, the students’ uptake of learning opportunities is crucially important. In connection with the supply-use model (Helmke, 2012), teaching can, in terms of the theory of action, be regarded as the offer of an opportunity which becomes effective only when individual students make use of it. This use can be suggested, but not determined either by the offer or by the actions of the teacher (Klieme, 2019; Vieluf et al., 2020).
The supply-use model distinguishes conceptually between cognitive activation as an offer directed at students from the outside and between the students’ actual cognitive activity, i.e., the use which learners make of the offer (Fauth and Leuders, 2018). The term “cognitive activity” encompasses all the students’ learning activities (Fauth and Leuders, 2018, p. 5) in the context of engaging with the school subject. In the present paper, we focus on the user side, i.e., the individual cognitive activity of learners.
State of Research
The importance of cognitive activation for learning, students’ motivation and subject-specific interest has already been studied frequently in relation to different grade levels and diverse subject cultures, with a focus on mathematics and science subjects (e.g., Klieme and Rakoczy, 2003; Lipowsky et al., 2009; Kunter and Voss, 2013; Fauth et al., 2014b; Praetorius et al., 2018; Grünkorn et al., 2020).
The findings to date predominantly indicate a positive relationship between cognitive activation in the classroom and student performance (e.g., Klieme et al., 2001; Pauli et al., 2008; Lipowsky et al., 2009; Baumert et al., 2010). However, some studies report only a weak correlation or none at all between cognitive activation and learning achievement or the development of subject-related interest (e.g., Hugener et al., 2007; Fauth et al., 2014a,b; Praetorius et al., 2020a). Therefore, the picture that emerges of the impact of cognitively activating teaching is heterogeneous. This may be attributable to the differences in conceptualization and operationalization of cognitive activation as well as to the use of different methodological approaches (Leuders and Holzäpfel, 2011; Praetorius et al., 2018; Lipowsky and Bleck, 2019), which range from assessing the cognitive activation potential of tasks (e.g., Büchter and Leuders, 2005; Drollinger-Vetter, 2006; Kleinknecht, 2010; Baumert and Kunter, 2011) to surveying teachers and students (e.g., Baumert and Kunter, 2011; Fauth et al., 2014a) to analyzing classroom videos using high-inference rating procedures (e.g., Kobarg and Seidel, 2003; Lipowsky et al., 2009; Kleinknecht, 2010; Reusser and Pauli, 2010; Lotz, 2016; Grünkorn et al., 2020).
The divergent findings may also be explained by the fact that the effects of cognitive activation in teaching do not unfold until learners make use of the opportunity. The operationalization of cognitive activation by the teacher may not be sufficient to capture the level of information processing and the depth of the students’ intellectual engagement with the subject matter (Lipowsky and Bleck, 2019; Praetorius et al., 2020b).
In addition to analyzing the cognitively activating behavior of the teacher, some researchers have conducted surveys with students to include their perspective on cognitive activation (e.g., Baumert and Kunter, 2011; Fauth et al., 2014b). Some video-based classroom studies also take into account additional aspects, such as identifying the frequency, duration and nature of student contributions, in order to draw conclusions about the characteristics of cognitive activation (e.g., Seidel et al., 2003; Pauli et al., 2008; Stahns, 2013). It is unclear, however, to what extent these approaches to operationalization are suitable for validly assessing the cognitive activity of students in the classroom (Fauth and Leuders, 2018; Lipowsky and Bleck, 2019). Detailed analyses focusing on micro-processes of student engagement with the subject using videoed classroom observations are only available in isolated cases so far (e.g., Ranger, 2017; Grünkorn et al., 2020). These studies assess cognitive activity at classroom level and with respect to longer teaching units. They do not analyze the micro level of individual interactions, although it is precisely observation of individual engagement with the learning opportunity at specific moments of the learning process that could provide additional insights into the utilization and impact of cognitively activating teaching (Praetorius and Charalambous, 2018). It can be assumed that different learners make different use of cognitively activating elements depending on their individual prerequisites (Lipowsky, 2020) and that they therefore exhibit different intensities of cognitive activity at different points in time. Similarly, it can be assumed that a state of high cognitive activity cannot be sustained through an entire lesson and that phases of low cognitive activity alternate with phases of high activity.
Generally, the assumption when empirically observing cognitive activation is that the more pronounced the cognitive activation potential of the teaching, the more likely it is that students will display cognitive activity. What remains open is the question of what specifically triggers cognitive activity in the learning process for individual students. By taking the assumed effects of a cognitively activating instruction into account, triggers can be anticipated at the micro level of the interaction. Thus, it is conceivable that a verbal impulse from the teacher (e.g., Kleinknecht, 2010; Kunter and Trautwein, 2013; Lipowsky, 2020) or cognitively stimulating teaching materials (Büchter and Leuders, 2005; Drollinger-Vetter, 2006; Kleinknecht, 2010; Baumert and Kunter, 2011; Lipowsky, 2020; Herbert and Schweig, 2021) elicit cognitive activity in individual students. Similarly, exchanges in the context of group work can be assumed to trigger cognitive activity (e.g., van Boxtel et al., 2000; Kunter and Trautwein, 2013; Renkl, 2015; Hess and Lipowsky, 2020).
Research Questions
Of particular interest, but not addressed in the literature to date, is the question of how high cognitive activity is triggered in individual students at the micro level of interaction. To explore this issue, it is necessary to begin by identifying phases of high cognitive activity by individual students in the course of group work. Subsequently, the sequences can be analyzed to identify possible triggers for high cognitive activity. For the present paper, therefore, the following research questions arise:
1) To what extent does the level of cognitive activity vary from student to student in the course of group work? (RQ1)
2) What are the triggers of high cognitive activity at the micro level of teacher-student and student-student interactions? (RQ2)
Materials and Methods
Sample
The research questions were studied using a videoed English lesson in the tenth grade of an integrated comprehensive school. The videos were created in the context of “Qualitätsoffensive Lehrerbildung,” a nationwide program promoting reforms in teacher training funded by the German Ministry of Education and Research. In a cooperative seminar on English teaching methodology and educational sciences forming part of the project “Das Lehr-Lern-Forschungslabor” (Teaching-learning research laboratory) at Johannes Gutenberg University of Mainz, a group of three teacher trainees developed lesson content as a cognitively activating learning unit. In close collaboration with the English teacher of the cooperating school, they put their self-developed materials into practice [in the so-called Teaching and Learning Research Lab (Groß-Mlynek et al., 2018)] and teach a 90-min English lesson in a tenth-grade class. Students worked in small groups of two or four. The group composition was self-selected by students, in some cases the teacher assigned individual students to their groups. Ecological validity was thus given, but no composition effects could be controlled. Each student had the use of a tablet for the duration of the learning unit. The aim of the lesson was to use an app to create a concept map (Novak, 2002) about the production process of a pair of jeans. The teacher encouraged the students to work in groups on a collaborative concept map, although it was not a strong prompt. The necessary information was taken from an English-language interactive website. It is assumed that concept mapping is especially conducive to cognitive activation because constructing a concept map requires an intense cognitive engagement with the contents of the task and challenges students to link elements of knowledge and integrate newly acquired knowledge into an overall context (Schroeder et al., 2018; Graf et al., 2021). The students were experienced tablet users and had been given an introductory lesson on the app and the concept mapping method prior to the exercise.
For each student, 56 min of video material from a group work phase was available. Two cameras were used to film the group tables from two angles to ensure that all their facial expressions and gestures were recognizable and that the tablets and all other teaching materials were visible at all times. A total of 18 students were videoed at five different group tables.
On the one hand, the videos serve as a basis for reflection on the performed lesson. On the other hand, we use the video material to address further research questions.
Analysis
Since mental engagement with the subject is not directly visible, observational studies are confined to drawing conclusions about individual cognitive activity by analyzing visible behavior, especially the verbalizations of the students. Therefore, the analysis of cognitive activity in group work phases is beneficial in two respects. Besides to the assumption that cooperative learning phases are cognitively activating (Kunter and Trautwein, 2013; Kyndt et al., 2013), learners are encouraged by communication in the group to verbalize mental processes, so that these become observable and accessible for analysis (cf. van Boxtel et al., 2000; Minnameier et al., 2015; Ranger, 2017). This does not rule out the possibility that learners are cognitively highly activated even during periods of individual work, but without externalization in the form of interaction with others, mental activity remains inaccessible to an outside observer. Thus, the present analysis focuses on observable student-student and teacher-student interactions.
Basic Coding
We began with basic coding of all the material in order to get an overview of students activities during the entire lesson. A low-inference category system with several levels was deductively and inductively developed for the purpose. Level 1 consists of interaction types in the categories “individual work,” “interaction with other students,” and “interaction with teachers.” The latter category also includes students’ interactions with the trainee teachers who supported the subject teacher in supervising group work. Level 2 comprises the students’ verbal and non-verbal activities, broken down into more nuanced categories: “explaining,” “asking,” “commenting,” “listening/observing,” “working on the material,” and “off-task” (see Supplementary Table 1).
As the basic coding was geared toward achieving a precise description of behavior at the individual student level in the course of the entire lesson, the coding used a time-sampling procedure with 10-s intervals (Hugener et al., 2006; Lotz et al., 2013). Categorization was performed separately for each student by two independent coders (student teachers at an advanced stage of their studies) who were trained by the team of authors. The video analysis program Mangold Interact (version 17.1.11.0) was used for coding and analyzing the data.
The interrater reliability of the low-inference coding of nominal scale variables was assessed in terms of the kappa coefficient (κ) (Cohen, 1960). The coding congruence of the reliability coefficients is very good at level 1 (0.89 ≤ κ ≤ 0.99) and level 2 (0.79 ≤ κ ≤ 0.89) (Wirtz and Caspar, 2002) (see Table 1).
Rating: Level of Cognitive Activity
In order to take a closer look at individual cognitive activity in relation to the subject of the lesson, all sequences of the categories “explaining,” “asking,” and “commenting” (see section Basic Coding, Level 2) relating to the subject were subsequently selected. A 1-day training was held for the coding of the subject-specific sequences. Two project team members and one additional rater (student teachers at an advanced stage of their studies) coded the material. The interrater reliability yielded good congruencies (see Table 1).
The level of cognitive activity on the part of individual students in these subject-specific sequences was estimated using a three-level (high, medium, low) medium-inference rating system comprising 26 indicators. Based on Lipowsky (2020), various student behaviors suggesting high cognitive activity were incorporated into the rating system as indicators. These include verbalizations with which the learners explain their solution processes, exchange arguments, make references to other students’ contributions, refer back to prior knowledge, transfer their knowledge to other fields, formulate hypotheses and assumptions, ask questions about the subject matter, create comparisons, and draw conclusions. These indicators were supplemented with elaborated explanations according to Renkl (1997), who refers to the repetition of content in the student’s own words and to rephrasing interrelationships in order to help his or her peers to understand it (see Supplementary Table 2).
Phases with high cognitive activity (3) are characterized by a reflective engagement with information and by explanations of the students’ own thoughts and suggested solutions. Students arrange knowledge modules into a superordinate structure which creates links to other knowledge, so that an independent knowledge structure becomes discernible (Lipowsky, 2020). Phases of medium cognitive activity (2) are characterized by approaches indicating an independent engagement with the subject matter, but not one that goes into greater depth. For example, the student might state an approach to solving the problem without explaining it in detail or placing it into context with other aspects. Phases of low cognitive activity (1) comprise sequences in which a rather superficial engagement can be assumed. This may be the case when students adopt problem-solving approaches or arguments from their peers without further thought and do not contribute their own ideas. Stating that they do not understand something without making an effort to understand similarly indicates the absence of independent engagement with the subject matter (for examples see Supplementary Table 3).
The interrater reliability for cognitive activity was calculated using kappa statistics and indicated a good level of agreement (see Table 1).
Content Analysis and Coding: Triggers of Cognitive Activity
Subsequently, student-teacher and student-student interactions were exploratively studied at the micro level to understand what happens in the respective group before individual students exhibit high cognitive activity. For this purpose, the sequences in which a student’s cognitive activity had previously been rated as high (see section Rating: Level of Cognitive Activity) were assessed in a content analysis (Mayring, 2015). We went back up to 30 s to identify what triggered a high cognitive activity phase. On the basis of this analysis, various triggers were identified and clustered into four categories that we derived from theory and from the material. Subsequently, the four categories were inserted into a low-inference category system and the material was coded once again. The category system was developed by two project team members and then applied with help from another rater (student teacher) after a 2-day training period (for interrater reliability see Table 1).
Results
Labeling Phases of Cognitive Activity
Two levels were considered during basic coding: On Level 1 (L1), we coded the times when students were interacting with others and when they were engaging in individual work. On Level 2 (L2) we examined interaction sequences more closely and differentiated between various activities (see section Basic Coding).
On Level 1, “type of interaction” (see section Basic Coding), it becomes clear that students are very different in the ways they respond to offers of cooperation embedded in the tasks assigned to them (Figure 1). While working on a joint concept map, students in groups 3 and 5 engaged in verbal and non-verbal interactions for significantly more than half the lesson time (55.98% and 71.43% respectively). In contrast, students in the other three groups interacted with other group members for only about one-quarter of the lesson time (between 20.48% and 28.14%), instead completing the task mostly by themselves (between 65.03% and 71.30%). In all groups, interaction with the teacher accounted for a relatively small proportion of M = 7.65% on average (SD = 2.52%).
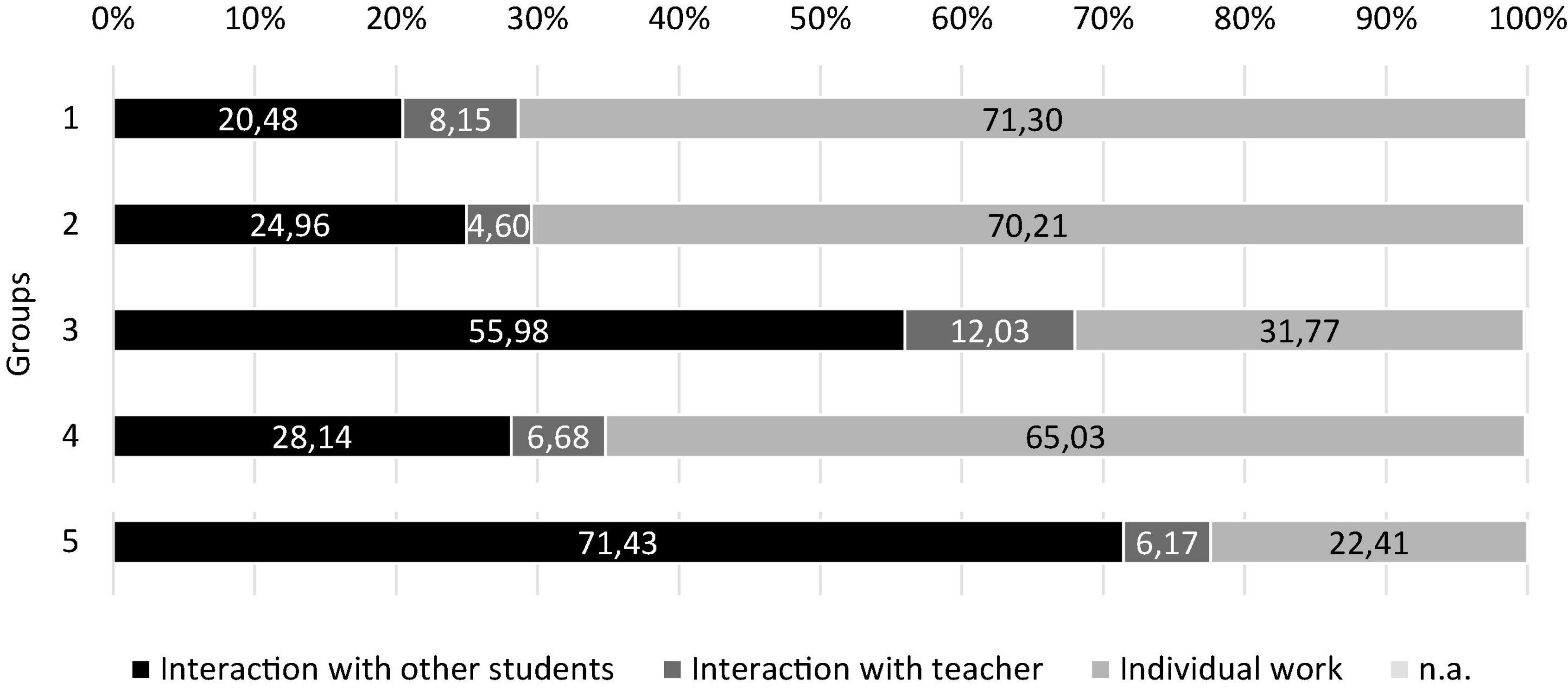
Figure 1. Interaction type (L1) by student groups. 100% represents recorded teaching time per student group (M = 55.91 min, SD = 0.33 min). n.a., category not assignable (less than 1% per group). In the interests of clarity, figures under 5% are not shown.
An examination of the level of activities (L2) compared to total lesson time reveals that students in all groups were on-task and engaging with the material for over 83% of lesson time (Figure 2). Students in groups 3 and 5 exhibited high proportions of verbal (explaining, asking, commenting) and non-verbal (listening, observing) activities compared to the other groups. Overall, non-verbal activities represent a significant proportion of total interactions in all groups.
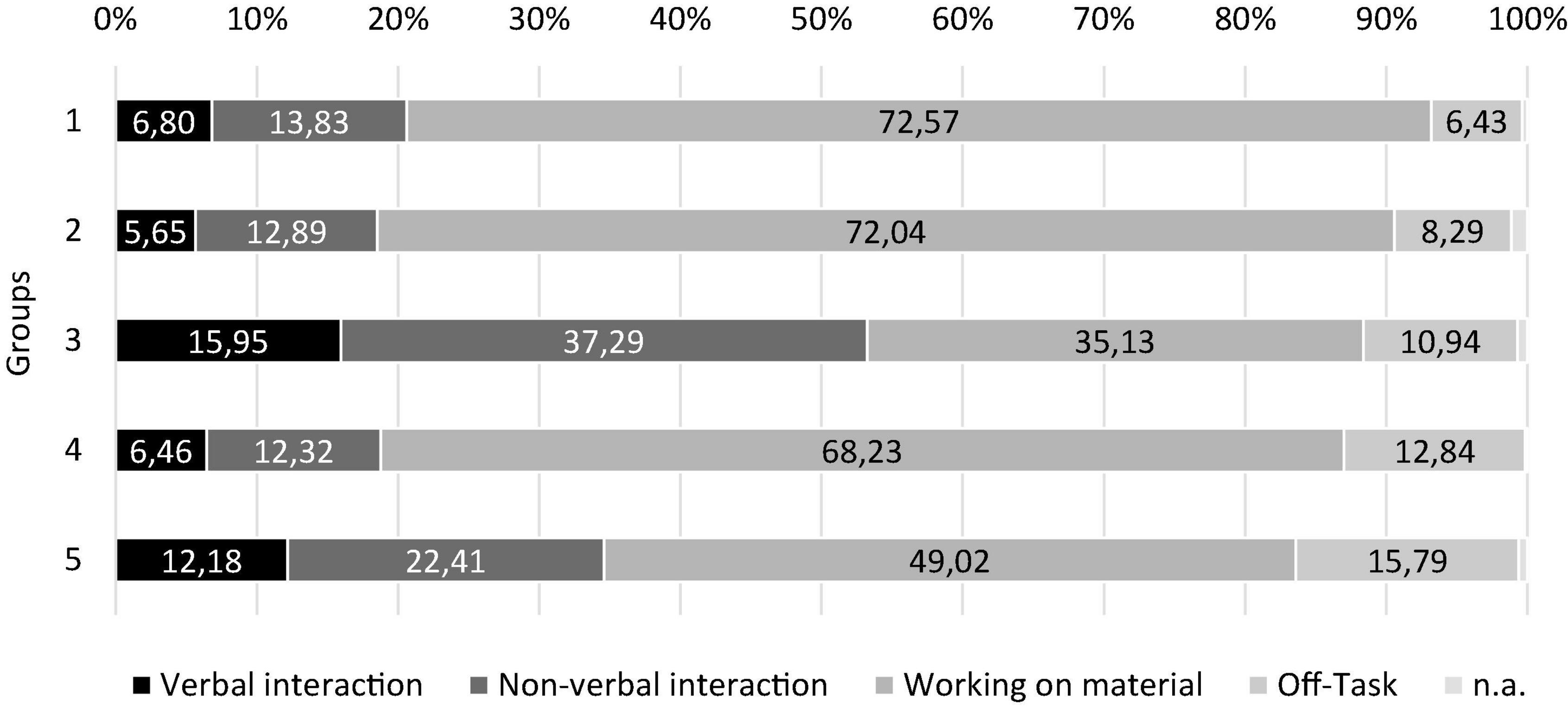
Figure 2. Activities (L2) by student groups. 100% represents recorded teaching time per student group (M = 55.91 min, SD = 0.33 min). n.a., category not assignable (between 0.37% and 1.95%). In the interests of clarity, figures under 5% are not shown.
There are also noticeable differences at the individual level of individual students (see Table 2). Students 2 and 4 in group 3 and student 2 in group 5 exhibit high scores (52, 54, and 65 sequences) in the “explaining” category. Of particular interest is student 4 in group 3, who was very active during group work and achieved the highest scores in her group in all categories of verbal activities (asking, explaining, commenting), but devoted the least attention to her fellow students in the form of non-verbal activities (listening, observing) compared to other group members. In contrast, student 3 in group 3 displayed little verbal activity, but was the most active participant (182 sequences) in the group process at the non-verbal level by primarily listening and observing. An examination of the students in the other group reveals similar patterns: Student 3 in group 1, student 2 in group 4, and student 2 in group 5 participated verbally in their group work particularly frequently (42.06% to 50.77% total verbal activities of asking, explaining, and commenting), while non-verbal activities predominated among the rest of the students with a frequency of over 60% to 86.26%.
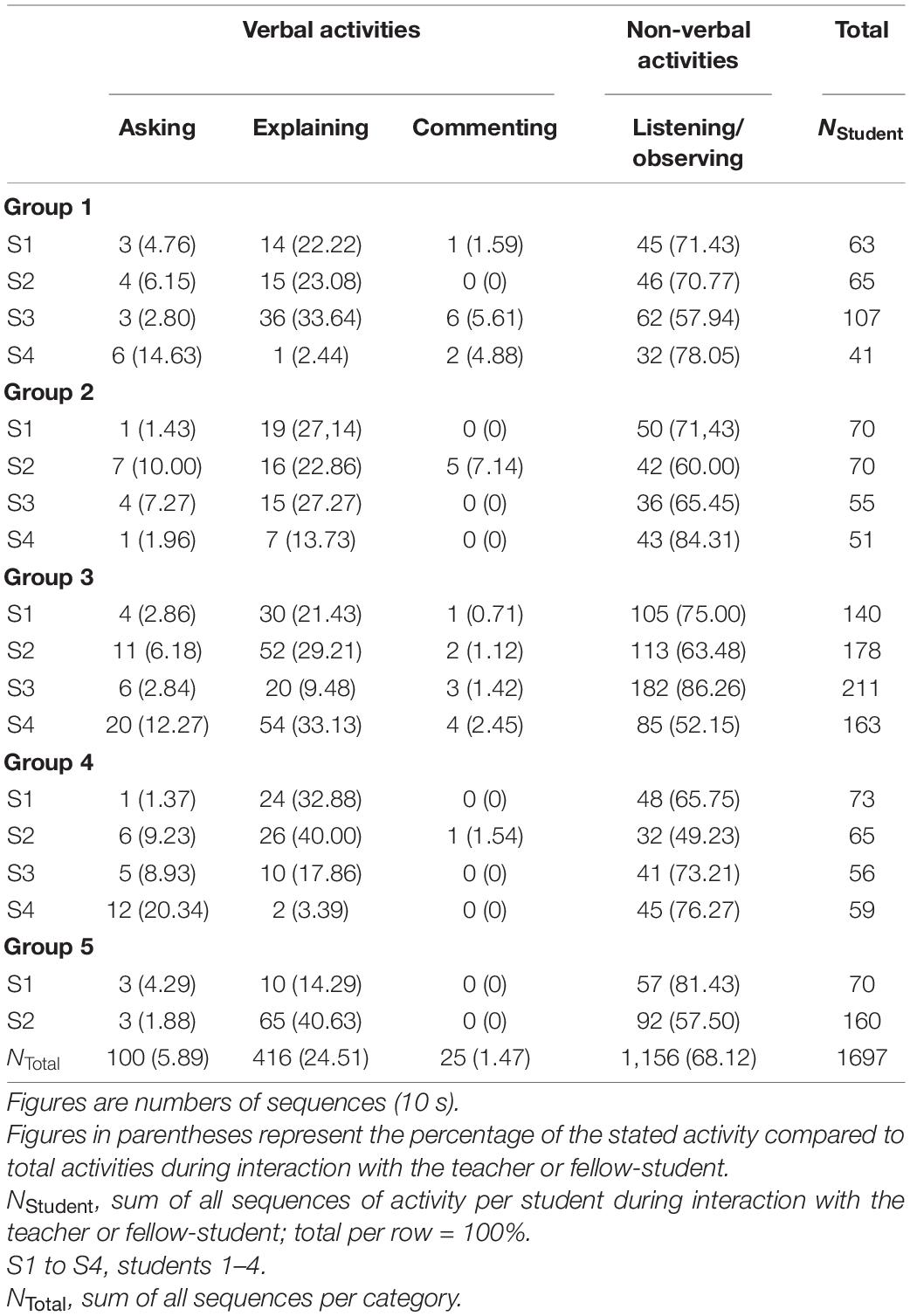
Table 2. Verbal and non-verbal activities of students during interactions with the teacher or another student.
Assessment of Cognitive Activity Level
Verbal activities (asking, explaining, commenting) account for 541 of a total of 1,697 sequences (see Table 2). 341 of the verbal sequences (63.03%) were related to the contents of the task and were rated in terms of the cognitive activity of the individual students. Overall, cognitive activity was assessed as high in 177 sequences (51.91%), medium in 133 sequences (39%), and low in 31 sequences (9.09%) (see Table 3).
The percentage distribution of high, medium, and low cognitive activity sequences varies considerably from student to student. Some students, like S1 and S4 in group 3, show a very high percentage of utterances indicating high cognitive activity (69.57% and 74.58% respectively) compared to their fellow-students, indicating that the bulk of their engagement with the subject matter was on a high cognitive level. Others, such as S2 in group 5, predominantly exhibited a medium cognitive level; i.e., they displayed recognizable signs of an independent engagement with the lesson content but did not get into deeper learning processes. Sequences of low cognitive level were comparatively few in number across all students. S2 in group 2 (33.3%) and S3 in group 4 (45.45%) were conspicuous for interacting with their fellow-students particularly often for short questions about the lesson content, but these queries did not lead to a deeper engagement in the course of their interactions.
In an examination of the sequences of high cognitive activity in relation to all verbalizations referring to the subject matter, students S3 and S4 in group 4 stand out (see Supplementary Table 4). The absolute number of their verbalizations is almost equal (15 and 14 sequences respectively) and relatively low compared to their fellow-students. A comparison of the levels of their cognitive activity reveals significant differences. In the case of S3, 64.29% (9 of 14 sequences) of verbalizations related to the subject matter were rated as high cognitive activity, while the same applies to only 25% (3 of 12 sequences) of S4’s verbalizations. A similar pattern is evident in the two students of group 5. While both made a similar number of verbalizations relevant to the subject matter, 60% of S1’s verbalizations and only 42.62% of S2’s were assessed as indicating high cognitive activity (see Supplementary Table 4).
Identifying Triggers
Subsequently triggers were identified for the 177 sequences that had been identified as eliciting high cognitive activity (see Supplementary Table 4).
During content analysis, various triggers were clustered into four categories (see Supplementary Table 4):
(1) Individual engagement with teaching materials on the tablet
(2) Stimuli given by teacher
(3) Questions from fellow-students
(4) Observing fellow-students/listening
Category 1 describes individual engagement with the teaching materials on the tablet as a trigger for high cognitive activity (Drollinger-Vetter, 2006; Kleinknecht, 2010; Baumert and Kunter, 2011; Herbert and Schweig, 2021). Students decide on their own initiative to share their thoughts, and set off an exchange of knowledge within the group. Category 2 comprises classical cognitive activation by the teacher by asking questions, contrasting different opinions, or drawing attention to contradictions (Kleinknecht, 2010; Lipowsky, 2020). Category 3 includes impulses by questions from fellow-students, which are addressed either directly to a specific student or to the group in general (Kunter and Trautwein, 2013; Renkl, 2015; Hess and Lipowsky, 2020). While engagement with the subject matter is usually deliberately prompted by the teacher by means of hints or questions to which the teacher already knows the answer, questions from fellow-students are asked within a joint, open problem-solving process. Category 4 encompasses suggestions from students that elicit after observing other students activities and without recognizable direct verbal prompting. For example, a student who has not previously participated becomes involved after observing or listening to exchanges between two or more other students by contributing a supporting or contradictory argument.
High cognitive activity is observed most frequently (71 sequences) after individual engagement with teaching materials on the tablet (1). The second highest frequency of high cognitive activity in individual students (58 sequences) arise from students observing their fellow-students or listening to what they are saying (4). In 27 sequences, high cognitive activity is triggered by questions from fellow-students (3). In a similar number (21 sequences), impulses provided by the teacher (2) serve as the trigger.
S2 and S4 in group 3 and S2 in group 5 exhibit high scores (17, 19, and 17 sequences) in category 1. In these sequences, observable high cognitive activity is triggered by engagement with the teaching materials. S4 in group 3, additionally, exhibits a high score in category 4 (14 sequences). Thus, high cognitive activity is triggered equally often by engagement with the teaching materials and by observing or listening to exchanges between fellow-students. Similarly, S2 in group 5 is encouraged by fellow group members to a high level of cognitive activity relatively frequently (8 sequences) compared to other students. S1 in group 3 also stands out. This student’s high cognitive activity is almost exclusively triggered by questions from fellow-students (6 sequences) or observing fellow-students and listening to their discussion of the subject matter (9 sequences).
Discussion
The basic coding shows that students spent the bulk of the group work phase engaging with the subject matter and are therefore cognitively active at least on a fundamental level. A total of 12 out of 18 students (three of five groups) chose to work on the task mostly independently (see section “Labelling Phases of Cognitive Activity”) and communicate with fellow-students in order, for example, to clarify their understanding of content or to solve technical difficulties. This typically results in short-term cooperation that do not point to a deeper joint engagement with the subject matter (see section “Assessment of Cognitive Activity Level”). A total of six students (groups 3 and 5) work together in close cooperation, creating a joint final product in their groups and being especially active verbally.
With respect to Research Question 1 (RQ1) we examine the extent to which the level of cognitive activity varies from student to student. We find that students with high verbal participation are assessed by the raters as exhibiting high cognitive activity in many sequences. At first glance, this could be taken as an indication that frequency of verbal participation correlates with cognitive activity. However, when one examines high-cognitive verbalizations in relation to overall verbal interactions, a different picture emerges. Some students who are assessed as displaying high cognitive activity in a particularly high absolute number of sequences (e.g., S4 in group 3) rank in midfield in terms of the ratio of their high-cognitive sequences to their overall verbalizations. Conversely, some students, like S1 in group 3 and S2 in group 5, are less verbally active than the rest of their group, but a large proportion of their infrequent verbalizations are assessed as indicative of high cognitive activity. Thus, assessing the cognitive activity of students in terms of the frequency of their verbal contributions yields an incomplete impression (Pauli and Lipowsky, 2007; Lipowsky and Bleck, 2019). A qualitative assessment as given here for individual student contributions to determine depth of engagement with the subject matter facilitates a detailed look at cognitive activity in specific sequences, resulting in a more nuanced picture.
In a further step we examined the sequences in which individual students displayed high cognitive activity in order to identify possible triggers at the micro level of student-teacher and student-student interaction (RQ2). Four categories of triggers emerged. Category 1, individual engagement with the teaching materials on the tablet, is the most frequent trigger of high cognitive activity in individual students; this finding confirms the relevance of cognitively activating exercises (Drollinger-Vetter, 2006; Kleinknecht, 2010; Baumert and Kunter, 2011). Category 2 reflects the role of the teacher in cognitive activation, which has already been pointed out in other studies (e.g., Clausen et al., 2003; Reusser and Pauli, 2010; Lotz, 2016; Grünkorn et al., 2020). Thus, it can be shown that the teacher and the trainee teachers succeed in prompting high cognitive activity in individual students by asking questions and making comments during the group work phase. It should be stressed that this applies only to individual students; not all students are equally receptive to cognitively activating impulses provided by the teacher. Additionally, the data show that students cognitively activate one another during the group work phase. Thus, the available findings support previous assumptions about the potential of cooperative learning phases for cognitive activation (Kunter and Trautwein, 2013; Renkl, 2015; Hess and Lipowsky, 2020). In these phases, direct questions by fellow-students (category 3) serve as triggers of high cognitive activity, while additional cognitive activation results from observing and listening to fellow-students (category 4), i.e., by an initially passive participation in the exchanges of other group members. An examination of triggers at the level of individual students shows that some students are particularly susceptible to stimulation by certain triggers, supporting the assumption of differential use of learning opportunities depending on individual prerequisites (Praetorius and Charalambous, 2018; Lipowsky, 2020). Overall the results confirm the theoretical assumptions and show that different stimuli can trigger high cognitive activity. It is interesting to note that a specific stimulus does not have the same effect on all students. The assumption that instruction with high cognitive potential leads to increased cognitive activity among students ignores the fact that individual students respond very differently to cognitively activating stimuli. With our differentiated rating for each student (instead of a global rating for a whole lesson or whole class), the individual handling of a potentially cognitively activating lesson is brought more into focus. However, since the individual student data is insufficient in three of five groups, we can only formulate conjectures in this regard, which need to be investigated in further studies. For example, additional variables for factors like performance level, motivation, interest in the subject matter, or group structures could be used to model mediating effects and better explain preferences for certain triggers.
Limitations
The explorative results of the present study must be taken in the context of our small sample of 18 students. The study should be extended to a larger sample and additional subjects and grades in order to verify the findings.
Since direct observation of students’ cognitive activity is fundamentally impossible, the question whether the underlying operationalization and the chosen rating system of the present study are suitable for validly recording cognitive processes must remain unanswered. The fact that cognitive activity can be inferred from the outside only by observing externalized verbalizations and visible behavior limits our perspective and excludes all mental processes that are not verbalized. Thus, it is impossible to conclude that low verbal activity implies low cognitive activity.
However, on the assumption that deeper learning goes hand in hand with appropriate verbalization, i.e., that successful in-depth engagement with the subject matter can be said to have taken place only if learners are capable of verbalizing their knowledge in a manner appropriate to those they are addressing (Meyer et al., 2018), students’ verbalizations can be interpreted as indications that cognitive activity has occurred. Nevertheless, the results of the present study should be taken exclusively as conclusions drawn from a possible manifestation of cognitive activity.
Furthermore, little supplementary information on the goals of the lesson and the students’ individual prerequisites was available, so that an informative contextualization of the analyzed lesson was possible only to a limited extent. An analysis of the concept maps would offer the possibility of assessing the impact of the students’ cognitive activity.
It should also be noted that, while the interrater reliability for assessing cognitive activity levels is satisfactory, it was sometimes difficult to distinguish between high and medium cognitive activity. High cognitive activity is characterized by an intensive engagement with the subject matter, while medium cognitive activity indicates the beginnings of an independent engagement that remains on a more superficial level (see section Rating: Level of Cognitive Activity). The transition from the beginnings of engagement to in-depth engagement, however, is gradual and cannot be clearly delimited, so that assessments of cognitive activity level can diverge at these points.
Another explanation for the difficulty of distinguishing between high and medium cognitive activity could be the fact that assessment of cognitive activity relating to the subject matter is conflated with the assessment of cognitive activity relating to the need to deal with a foreign language.
As an example, a verbalization relating to the subject matter may imply low cognitive activity if it simply repeats information; however, the student may still be engaging in high cognitive activity by virtue of communicating in the foreign language. Pirner (2007) argues that the greater challenge of communicating in another language causes the intensity of engagement with the subject matter to increase because cognitive activity in general is higher, citing indications confirming this assumption in his analyses of bilingual religious education (ibid., p. 47). Thus, we may conjecture that engagement with a foreign language is positively associated with cognitive activity in relation to the subject matter.
Another aspect that could limit the significance of the analyses is the composition of the groups (Kunter and Trautwein, 2013). The intensity of student interaction within the groups varies; for example, the decision by students in groups 3 and 5 to create a joint concept map causes them to engage in particularly intensive verbal exchanges (see section “Labelling Phases of Cognitive Activity”). One should not discount the possibility that, had the groups been put together differently, students would have displayed different behaviors and both our assessments of cognitive activity and our identification of triggers would have yielded different results.
Implications for Research and Teaching
The present approach of observing cognitive activity at the student level takes the students’ individuality into account. Cognitively activating teaching must be tailored toward their individual learning prerequisites. However, students differ not only in the prerequisites they bring to the classroom, but also in their learning processes, in which differences in their uptake of learning opportunities and in their resultant cognitive activity can be observed (Lipowsky, 2020). Cognitively activating elements such as exercise design and impulses provided by the teacher have different effects on different students, so that the use of varied cognitively activating impulses in the classroom appears advisable. The study of a larger number of sequences might enable the development of typologies to reflect the triggers or combinations of triggers causing particularly strong cognitive activation in individual students. Analysis of patterns could also be informative in determining when exactly cognitively activating phases occur.
To encourage mutual cognitive activation during group work phases, intensive exchanges among the students must be stimulated. Greater guidance from the teacher may be one way another may be creating a joint end product of achieving this (Cohen, 1986). From this perspective, it should be asked to what extent the tablets may have inhibited the cooperative construction of knowledge in the lesson we studied, since the availability of one tablet per child might easily tempt students to complete the tasks independently.
Data Availability Statement
The datasets presented in this article are not readily available because the video-based data is not fully anonymized (e.g., pixelating students faces) due to research-related reasons. Requests to access the datasets should be directed to MH.
Author Contributions
All authors listed have made a substantial, direct, and intellectual contribution to the work, and approved it for publication.
Funding
This project is part of the “Qualitätsoffensive Lehrerbildung”, a joint initiative of the Federal Government and the Länder which aims to improve the quality of teacher training. The programme is funded by the Federal Ministry of Education and Research. The authors are responsible for the content of this publication.
Conflict of Interest
The authors declare that the research was conducted in the absence of any commercial or financial relationships that could be construed as a potential conflict of interest.
Publisher’s Note
All claims expressed in this article are solely those of the authors and do not necessarily represent those of their affiliated organizations, or those of the publisher, the editors and the reviewers. Any product that may be evaluated in this article, or claim that may be made by its manufacturer, is not guaranteed or endorsed by the publisher.
Supplementary Material
The Supplementary Material for this article can be found online at: https://www.frontiersin.org/articles/10.3389/feduc.2022.873340/full#supplementary-material
Footnotes
- ^ This framework model, widely known and frequently used in German educational studies, provides a schematic representation of the structural and processual properties of teaching. According to the model, teaching is an opportunity created by the teacher which students can utilize based upon their individual preconditions in order to achieve a specific learning outcome (e.g., Baumert et al., 2004; Seidel, 2014; Vieluf et al., 2020).
References
Amosa, W., Ladwig, J., Griffiths, T., and Gore, J. (2007). “Equity effects of quality teaching: closing the gap,” in Proceedings of the Australian Association for Research in Education Research conference (London: Fremantle), 25–29.
Groß-Mlynek, L., Graf, T., Harring, M., and Feldhoff, T. (2018). Unterrichtsvideos als element der Theorie-Praxis-Verzahnung im Lehramtsstudium. J. LehrerInnenbildung 18, 56–61.
Graf, T., Groß-Mlynek, L., Harring, M., and Feldhoff, T. (2021). Kognitive aktivierung mit concept maps. Didaktische überlegungen zum schulischen einsatz. SchulVerwaltung HE/RP. Z. Schulentwicklung Schulmanage. 9, 245–247.
Baumert, J., Blum, W., and Neubrand, M. (2004). Drawing the lessons from PISA 2000. Z. Erziehungswiss. 3, 143–157. doi: 10.1007/978-3-322-86897-8_8
Baumert, J., and Kunter, M. (2011). “Das kompetenzmodell von COACTIV,” in Professionelle Kompetenz von Lehrkräften. Ergebnisse des Forschungsprogramms COACTIV, eds M. Kunter, J. Baumert, W. Blum, U. Klusmann, S. Krauss, and M. Neubrand (Hrsg.) (Münster: Waxmann), 29–53. doi: 10.1007/978-3-658-00908-3_13
Baumert, J., Kunter, M., Blum, W., Brunner, M., Voss, T., Jordan, A., et al. (2010). Teachers’ mathematical knowledge, cognitive activation in the classroom, and student progress. Am. Educ. Res J. 47, 133–180. doi: 10.3102/0002831209345157
Bleck, V., and Lipowsky, F. (2021). “Kooperatives lernen – theoretische perspektiven, empirische befunde und konsequenzen für die Implementierung,” in Handbuch Schulforschung, eds T. Hascher, T.-S. Idel, and W. Helsper (Hrsg.) (Heidelberg: Springer), 1–19. doi: 10.1007/978-3-658-24734-8_44-1
Brophy, J. E. (2002). Teaching. Chicago: University of Illinois, International Academy of Education. Available online at: http://www.ibe.unesco.org/fileadmin/user_upload/archive/Publications/educationalpracticesseriespdf/prac01e.pdf (accessed September 27, 2021)
Büchter, A., and Leuders, T. (2005). Mathematikaufgaben Selbst Entwickeln. Lernen fördern – Leistung überprüfen. Berlin: Cornelsen Scriptor.
Clausen, M., Reusser, K., and Klieme, E. (2003). Unterrichtsqualität auf der Basis hoch-inferenter Unterrichtsbeurteilungen. Ein Vergleich zwischen Deutschland und der deutschsprachigen Schweiz. Unterrichtswissenschaft 31, 122–141. doi: 10.25656/01:6775
Cohen, E. G. (1986). Designing Groupwork: Strategies for the Heterogeneous Classrooms. New York, NY: Teachers College Press.
Cohen, J. (1960). A coefficient of agreement for nominal scales. Educ. Psychol. Meas. 20, 37–46. doi: 10.1177/001316446002000104
Creemers, B., and Kyriakides, L. (2010). School factors explaining achievement on cognitive and affective outcomes: establishing a dynamic model of educational effectiveness. Scand. J. Educ. Res. 54, 263–294. doi: 10.1080/00313831003764529
Drollinger-Vetter, B. (2006). “Kognitiver anspruchsgehalt der aufgabenstellungen,” in Videoanalysen, eds I. Hugener, E. Klieme, and C. Pauli (Hrsg.) (Frankfurt: GFPF), 148–164.
Fauth, B., Decristan, J., Decker, A.-T., Büttner, G., Hardy, I., Klieme, E., et al. (2019). The effects of teacher competence on student outcomes in elementary science education: The mediating role of teaching quality. Teach. Teach. Educ. 86:102882. doi: 10.1016/j.tate.2019.102882
Fauth, B., Decristan, J., Rieser, S., Klieme, E., and Büttner, G. (2014b). Student ratings of teaching quality in primary school: dimensions and prediction of student outcomes. Learn. Instr. 29, 1–9. doi: 10.1016/j.learninstruc.2013.07.001
Fauth, B., Decristan, J., Rieser, S., Klieme, E., and Büttner, G. (2014a). Grundschulunterricht aus Schü ler-, Lehrer- und Beobachterperspektive. Zusammenhänge und Vorhersage von Lernerfolg. Z. Pädagog. Psychol. 28, 127–137. doi: 10.1024/1010-0652/a000129
Fauth, B., and Leuders, T. (2018). Kognitive Aktivierung im Unterricht. Band 2. Stuttgart: Landesinstitut für Schulentwicklung.
Grünkorn, J., Klieme, E., Praetorius, A.-K., and Schreyer, P. (2020). Mathematikunterricht im Internationalen Vergleich. Ergebnisse aus der TALIS-Videostudie Deutschland. Frankfurt: Leibniz-Institut für Bildungsforschung und Bildungsinformation.
Hamre, B. K., and Pianta, R. C. (2010). “Classroom environments and developmental processes: conceptualization and measurement,” in Handbook of Research on Schools, Schooling and Human Development, eds J. L. Meece and J. S. Eccles (Hrsg.) (London: Routledge), 43–59. doi: 10.4324/9780203874844.ch3
Hattie, J. (2009). Visible Learning: A Synthesis of Over 800 Meta-analyses Relating to Achievement. London: Routledge.
Helmke, A. (2012). Unterrichtsqualität und Lehrerprofessionalität. Diagnose, Evaluation und Verbesserung des Unterrichts. [Quality of Instruction and Teacher Professionalism – Diagnosis, Evaluation and Improvement of Teaching], 4. Seelze-Velber: Kallmeyer/Klett.
Herbert, B., and Schweig, J. (2021). Erfassung des Potenzials zur kognitiven Aktivierung über Unterrichtsmaterialien im Mathematikunterricht. Z. Erziehungswiss. 24, 955–983. doi: 10.1007/s11618-021-01020-9
Hess, M., and Lipowsky, F. (2020). “Zur (Un-)abhängigkeit von oberflächen- und tiefenmerkmalen im grundschulunterricht. fragen von lehrpersonen im öffentlichen unterricht und in schülerarbeitsphasen im vergleich,” in Empirische Forschung zur Unterrichtsqualität. Theoretische Grundfragen und quantitative Modellierungen. Beiheft der Zeitschrift für Pädagogik, 66, eds A. Praetorius, J. Grünkorn, and E. Klieme (Hrsg.) (Weinheim: Beltz), 117–131.
Hugener, I., Pauli, C., and Reusser, K. (2006). Dokumentation der Erhebungs- und Auswertungsinstrumente zur schweizerisch-deutschen Videostudie ,,Unterrichtsqualität, Lernverhalten und mathematisches Verständnis“. Videoanalysen (Materialien zur Bildungsforschung, 15. Frankfurt: GFPF.
Hugener, I., Pauli, C., and Reusser, K. (2007). “Inszenierungsmuster, kognitive aktivierung und leistung im mathematikunterricht. analysen aus der schweizerisch-deutschen videostudie,” in Professionell Lehren – Erfolgreich Lernen, eds D. Lemmermöhle, M. Rothgangel, S. Bögeholz, M. Hasselhorn, and R. Watermann (Hrsg.) (Münster: Waxmann), 109–121. doi: 10.25656/01:6779
Hugener, I., Pauli, C., Reusser, K., Lipowsky, F., Rakoczy, K., and Klieme, E. (2009). Teaching patterns and learning quality in Swiss and German mathematics lessons. Learn. Instr. 19, 66–78. doi: 10.1016/j.learninstruc.2008.02.001
Kleickmann, T. (2012). Kognitiv Aktivieren und Inhaltlich Strukturieren im Naturwissen¬Schaftlichen Sachunterricht. Kiel: IPN Leibniz-Institut für die Pädagogik der Naturwissenschaften an der Universität Kiel.
Kleickmann, T., Steffensky, M., and Praetorius, A.-K. (2020). Quality of teaching in science education: more than three basic dimensions? Z. Pädagog. 66, 37–55. doi: 10.3262/ZPB2001037
Kleinknecht, M. (2010). “Kognitive aktivierung und strukturierung durch aufgaben – analyse und weiterentwicklung der unterrichtlichen aufgabenkultur,” in Selbstbestimmung und Classroom-Management. Empirische Befunde und Entwicklungsstrategien zum guten Unterricht, eds T. Bohl, K. Kansteiner-Schänzlin, M. Kleinknecht, B. Kohler, and A. Nold (Hrsg.) (Bad Heilbrunn: Klinkhardt), 179–190.
Klieme, E. (2019). “Unterrichtsqualität,” in Handbuch Schulpädagogik, eds M. Harring, C. Rohlfs, and M. Gläser-Zikuda (Hrsg.) (Münster: Waxmann), 393–408.
Klieme, E., Lipowsky, F., Rakoczy, K., and Ratzka, N. (2006). “Qualitätsdimensionen und wirksamkeit von mathematikunterricht. theoretische grundlagen und ausgewählte ergebnisse des projekts ,,pythagoras“. [quality dimensions and effectiveness of mathematics instruction. theoretical background and selected findings of the pythagoras project],” in Untersuchungen zur Bildungsqualität von Schule. Abschlussbericht des DFG-Schwerpunktprogramms BIQUA, eds M. Prenzel and L. Allolio-Näcke (Hrsg.) (Münster: Waxmann), 127–146.
Klieme, E., and Rakoczy, K. (2003). Unterrichtsqualität aus schülerperspektive: kulturspezifische profile, regionale unterschiede und zusammenhänge mit effekten von unterricht,” in PISA 2000-Ein Differenzierter Blick auf die Länder der Bundesrepublik Deutschland, eds J. Baumert, C. Artelt, E. Klieme, M. Neubrand, M. Prenzel, U. Schiefele, et al. (Opladen: Leske & Budrich), 333–359. doi: 10.1007/978-3-322-97590-4_12
Klieme, E., Schümer, G., and Knoll, S. (2001). “Mathematikunterricht in der Sekundarstufe I: »Aufgabenkultur« und Unterrichtsgestaltung,” in TIMSS – Impulse für Schule und Unterricht. Forschungsbefunde, Reforminitiativen, Praxisberichte und Video-Dokumente, eds E. Klieme and J. Baumert (Hrsg.) (Bonn: Bundesministerium für Bildung und Forschung), 43–57.
Kobarg, M., and Seidel, T. (2003). “Prozessorientierte Lernbegleitung im Physikunterricht,” in Technischer Bericht zur Videostudie ,,Lehr-Lern-Prozesse im Physikunterricht, eds T. Seidel, M. Prenzel, R. Duit, and M. Lehrke (Hrsg.) (Kiel: Leibniz-Institut für die Pädagogik der Naturwissenschaften (IPN)), 151–200. doi: 10.25656/01:5490
Kunter, M., Brunner, M., Baumert, J., Klusmann, U., Krauss, S., Blum, W., et al. (2005). Der Mathematikunterricht der PISA-Schülerinnen und -Schüler: Schulformunterschiede in der Unterrichtsqualität. Z. Erziehungswiss. 8, 502–520. doi: 10.1007/s11618-005-0156-8
Kunter, M., and Trautwein, U. (2013). Psychologie Des Unterrichts. Paderborn: Schöningh. doi: 10.36198/9783838538952
Kunter, M., and Voss, T. (2013). “The model of instructional quality in COACTIV: A multicriteria analysis,” in Cognitive Activation in the Mathematics Classroom and Professional Competence of Teachers. Results from the COACTIV project, eds M. Kunter, J. Baumert, W. Blum, U. Klusmann, S. Krauss, and M. Neubrand (New York, NY: Springer), 97–124. doi: 10.1007/978-1-4614-5149-5_6
Kyndt, E., Raes, E., Lismont, B., Timmers, F., Cascallar, E., and Dochy, F. (2013). A meta-analysis of the effects of face-to-face cooperative learning. Do recent studies falsify or verify earlier findings? Educ. Res. Rev. 10, 133–149. doi: 10.1016/j.edurev.2013.02.002
Leuders, T., and Holzäpfel, L. (2011). Kognitive aktivierung im mathematikunterricht. Unterrichtswissenschaft 39, 213–230.
Lipowsky, F. (2020). “Unterricht,” in Pädagogische Psychologie, 3, eds E. Wild and J. Möller (Hrsg.) (Berlin: Springer), 70–118. doi: 10.1007/978-3-662-61403-7_4
Lipowsky, F., and Bleck, V. (2019). “Was wissen wir über guten Unterricht? – Ein Update,” in Unterrichtsqualität: Konzepte und Bilanzen gelingenden Lehrens und Lernens. Grundlagen der Qualität von Schule, 3, eds U. Steffens and R. Messner (Hrsg.) (Münster: Waxmann), 219–249.
Lipowsky, F., Rakoczy, K., Pauli, C., Drollinger-Vetter, B., Klieme, E., and Reusser, K. (2009). Quality of geometry instruction and its short-term impact on students’ understanding of the Pythagorean Theorem. Learn. Instr. 19, 527–537. doi: 10.1016/j.learninstruc.2008.11.001
Lotz, M. (2016). Kognitive Aktivierung im Leseunterricht der Grundschule. Eine Videostudie zur Gestaltung und Qualität von Leseübungen im ersten Schuljahr. Wiesbaden: VS Verlag für Sozialwissenschaften. doi: 10.1007/978-3-658-10436-8
Lotz, M., Lipowsky, F., and Faust, G. (2013). Dokumentation der Erhebungsinstrumente des Projekts “Persönlichkeits- und Lernentwicklung von Grundschulkindern” (PERLE). 3. Technischer Bericht zu den PERLE-Videostudien. (Materialien zur Bildungsforschung, 23. Frankfurt: GFPF.
Mayring, P. (2015). Qualitative Inhaltsanalyse. Grundlagen und Techniken.12., überarbeitete Auflage. Weinheim: Beltz.
Meyer, O., Imhof, M., Coyle, D., and Banerjee, M. (2018). “Positive learning and pluriliteracies. growth in higher education and implications for course design, assessment and research,” in Positive Learning in the Age of Information. A Blessing or a Curse?, eds O. Zlatkin-Troitschanskaia, G. Wittum, and A. Dengel (Hrsg.) (Wiesbaden: Springer), 235–265. doi: 10.1007/978-3-658-19567-0_15
Minnameier, G., Hermkes, R., and Mach, H. (2015). Kognitive Aktivierung und Konstruktive Unterstützung als Prozessqualitäten des Lehrens und Lernens. Z. Pädagog. 61, 837–856. doi: 10.25656/01:15429
Newmann, F. M. (1988). Higher order thinking in the high school curriculum. NASSP Bull. 72, 58–64. doi: 10.1177/019263658807250812
Novak, J. D. (2002). Meaningful learning: the essential factor for conceptual change in limited or inappropriate propositional hierarchies leading to empowerment of learners. Sci. Educ. 86, 548–571. doi: 10.1002/sce.10032
Pauli, C., Drollinger-Vetter, B., Hugener, I., and Lipowsky, F. (2008). Kognitive aktivierung im mathematikunterricht. Z. Pädagog. Psychol. 22, 127–133. doi: 10.1024/1010-0652.22.2.127
Pauli, C., and Lipowsky, F. (2007). Mitmachen oder Zuhören? Mündliche Schülerinnen- und Schülerbeteiligung im Mathematikunterricht. Unterrichtswissenschaft 35, 101–124.
Pirner, M. (2007). Empirische Unterrichtsforschung zum bilingualen Religionsunterricht und Konsequenzen für den ,normalen‘ Religionsunterricht. Z. Religionspädagogik 6, 42–52.
Praetorius, A.-K., and Charalambous, C. Y. (2018). Classroom observation frameworks for studying instructional quality: looking back and looking forward. ZDM. 50, 535–553. doi: 10.1007/s11858-018-0946-0
Praetorius, A.-K., Rogh, W., and Kleickmann, T. (2020b). Blinde Flecken des Modells der drei Basisdimensionen von Unterrichtsqualität? Das Modell im Spiegel einer internationalen Synthese von Merkmalen der Unterrichtsqualität. Unterrichtswissenschaft 48, 303–318. doi: 10.1007/s42010-020-00072-w
Praetorius, A.-K., Klieme, E., Herbert, B., and Pinger, P. (2018). Generic dimensions of teaching quality: The German framework of Three Basic Dimensions. ZDM. 50, 407–426. doi: 10.1007/s11858-018-0918-4
Praetorius, A.-K., Herbert, B., Decristan, J., and Köhler, C. (2020a). “Lernergebnisse und unterrichtliche Wirkungen,” in Mathematikunterricht im internationalen Vergleich. Ergebnisse aus der TALIS-Videostudie Deutschland, eds J. Grünkorn, E. Klieme, A.-K. Praetorius, and P. Schreyer (Hrsg) (Frankfurt: Leibniz-Institut für Bildungsforschung und Bildungsinformation), 31–35.
Ranger, G. (2017). Kinder in kooperativen Lernphasen kognitiv aktivieren. Eine Videostudie zur Qualität der Peer-Interaktionen im naturwissenschaftlichen Unterricht. (Dissertation). Bad Heilbrunn: Verlag Julius Klinkhardt.
Renkl, A. (1997). Lernen durch Lehren. Zentrale Wirkmechanismen beim kooperativen Lernen. Wiesbaden: DUV. doi: 10.1007/978-3-663-08696-3
Renkl, A. (2015). “Wissenserwerb,” in Pädagogische Psychologie, eds E. Wild and J. Möller (Hrsg.) (Berlin: Springer), 3–24. doi: 10.1007/978-3-642-41291-2_1
Reusser, K., and Pauli, C. (2010). “Unterrichtsgestaltung und Unterrichtsqualität. Ergebnisse einer internationalen und schweizerischen Videostudie zum Mathematikunterricht: Einleitung und Überblick,” in Unterrichtsgestaltung und Unterrichtsqualität. Ergebnisse einer internationalen und schweizerischen Videostudie zum Mathematikunterricht, eds K. Reusser, C. Pauli, and M. Waldis (Hrsg.) (Münster: Waxmann), 9–32.
Scheerens, J. (2013). The use of theory in school effectiveness research revisited. Sch. Eff. Sch. Improv. 24, 1–38. doi: 10.1080/09243453.2012.691100
Schroeder, N. L., Nesbit, J. C., Anguiano, C. J., and Adesope, O. O. (2018). Studying and constructing concept maps: a meta-analysis. Educ. Psychol. Rev. 30, 431–455. doi: 10.1007/s10648-017-9403-9
Seidel, T. (2014). “Performance assessment and professional development in university teaching,” in Incentives and Performance: Governance of Knowledge-Intensive Organizations, eds M. Osterloh, I. M. Welpe, J. Wollersheim, and S. Ringelhan (Hrsg.) (Heidelberg: Springer), 465–477. doi: 10.1007/978-3-319-09785-5_28
Seidel, T., Prenzel, M., Duit, R., and Lehrke, M. (2003). Technischer Bericht zur Videostudie ,,Lehr-Lern-Prozesse im Physikunterricht. Kiel: IPN.
Seidel, T., Prenzel, M., Rimmele, R., Dalehefte, I. M., Herweg, C., Kobarg, M., et al. (2006). Blicke auf den Physikunterricht. Ergebnisse der IPN Videostudie. Z. Pädagog. 52, 799–821. doi: 10.25656/01:4489
Stahns, R. (2013). Kognitive Aktivierung im Grammatikunterricht. Videoanalysen zum Deutschunterricht. Baltmannsweiler: Schneider.
van Boxtel, C., van der Linden, J., and Kanselaar, G. (2000). Collaborative learning tasks and the elaboration of conceptual knowledge’. Learn. Instr. 10, 311–330. doi: 10.1016/S0959-4752(00)00002-5
Vieluf, S., Praetorius, A., Rakoczy, K., Kleinknecht, M., and Pietsch, M. (2020). Angebots-Nutzungs-Modelle der Wirkweise des Unterrichts: Ein kritischer Vergleich verschiedener Modellvarianten. Z. Pädagog. 66, 63–80.
Keywords: classroom teaching research, basic dimensions of teaching quality, cognitive activity, cognitive activation, video analysis
Citation: Groß-Mlynek L, Graf T, Harring M, Gabriel-Busse K and Feldhoff T (2022) Cognitive Activation in a Close-Up View: Triggers of High Cognitive Activity in Students During Group Work Phases. Front. Educ. 7:873340. doi: 10.3389/feduc.2022.873340
Received: 10 February 2022; Accepted: 09 May 2022;
Published: 31 May 2022.
Edited by:
Stefinee Pinnegar, Brigham Young University, United StatesReviewed by:
Stefanie Findeisen, University of Konstanz, GermanyKadek Suranata, Ganesha University of Education, Indonesia
Copyright © 2022 Groß-Mlynek, Graf, Harring, Gabriel-Busse and Feldhoff. This is an open-access article distributed under the terms of the Creative Commons Attribution License (CC BY). The use, distribution or reproduction in other forums is permitted, provided the original author(s) and the copyright owner(s) are credited and that the original publication in this journal is cited, in accordance with accepted academic practice. No use, distribution or reproduction is permitted which does not comply with these terms.
*Correspondence: Lena Groß-Mlynek, grossl@uni-mainz.de
†These authors have contributed equally to this work and share first authorship