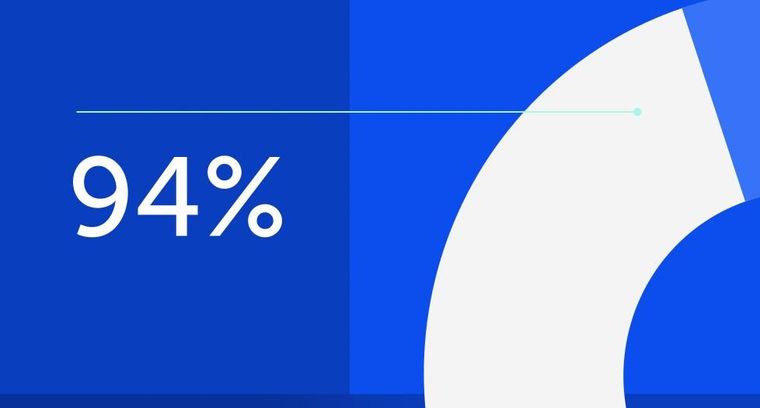
94% of researchers rate our articles as excellent or good
Learn more about the work of our research integrity team to safeguard the quality of each article we publish.
Find out more
METHODS article
Front. Educ., 15 July 2022
Sec. Educational Psychology
Volume 7 - 2022 | https://doi.org/10.3389/feduc.2022.867186
This article is part of the Research TopicMethodological and Empirical Advancements in Emotions and their Regulation in Various Collaborative Learning ContextsView all 6 articles
Collaborative learners share an experience when focusing on a task together and coevally influence each other’s emotions and motivations. Continuous emotional synchronization relates to how learners co-regulate their cognitive resources, especially regarding their joint attention and transactive discourse. “Being in-sync” then refers to multiple emotional and cognitive group states and processes, raising the question: to what extent and when is being in-sync beneficial and when is it not? In this article, we propose a framework of multi-modal learning analytics addressing synchronization of collaborative learners across emotional and cognitive dimensions and different modalities. To exemplify this framework and approach the question of how emotions and cognitions intertwine in collaborative learning, we present contrasting cases of learners in a tabletop environment that have or have not been instructed to coordinate their gaze. Qualitative analysis of multimodal data incorporating eye-tracking and electrodermal sensors shows that gaze instruction facilitated being emotionally, cognitively, and behaviorally “in-sync” during the peer collaboration. Identifying and analyzing moments of shared emotional shifts shows how learners are establishing shared understanding regarding both the learning task as well as the relationship among them when they are emotionally “in-sync.”
Co-present computer-supported collaborative learning (CSCL) scenarios often emphasize their capacity for “rich” interactions (Schmitt and Weinberger, 2018). Learners talk with each other and communicate with their bodies, pointing out objects, using tools, and taking control of the environment. Multimodal learning analytics (MMLA; Blikstein and Worsley, 2016; Di Mitri et al., 2018; Järvelä et al., 2020) broaden the perspective on co-present collaborative learning from phenomena of joint cognitive elaboration to shared emotional experiences of learning.
Emotions and especially the appraisal of learning experiences have been closely linked to motivation for learning (Järvenoja et al., 2018) as well as to information processing, e.g., as alarms for guiding attention (Pekrun and Linnenbrink-Garcia, 2012). Emotions are sometimes being treated as linked to cognition when recognized and labeled, which allows for retrospectively surveying participants’ emotions. Other perspectives regard emotions as quick responses to new information tied to physical states of arousal and, respectively, measured through bodily indicators, e.g., electrodermal activity (Järvenoja et al., 2018). Here we propose taking a perspective of emotions – particularly the convergence or divergence of emotions among learning partners – being functional markers of episodes of collaborative learning that call for further analysis of discourse and other modalities, such as shared gaze or joint attention. We assume that by combining and mutually informing the analyses of multiple modalities of collaborative learning processes, we can pinpoint episodes in which learners share experiences of emotional engagement in a learning task and then analyze how and why learners construct knowledge together. Earlier attempts at multimodal analysis have been promising. For instance, combining analysis of joint pupil dilation and attention through dual eye-tracking helped discover that collaborative learners who synchronously invested mental effort and who are working on the same area of interest on a concept map are more likely to perform well (Sharma et al., 2021).
How collaborative learners are synchronized in emotional, cognitive, and behavioral dimensions seems to be a central question with the consideration that working together is a “mostly synchronous interaction” (Pijeira-Díaz et al., 2019). Indeed, synchrony is associated with social affiliation and strengthening communication (Hove and Risen, 2009). Phenomena such as using the same gesture, looking at the same object, having the same facial expression in collaborative tasks have been observed and have been argued to serve mutual understanding and collaborative problem-solving (Louwerse et al., 2012). Here we suggest a multimodal approach to investigating the emotional, cognitive, and behavioral “synchrony” of two students learning together. In the following sections, we first review theories and studies on social-cognitive processes, emotion, and synchrony in collaborative learning. Then we introduce the framework for analyzing multimodal data, encompassing emotion, cognition, behavior, and their synchronizations in co-present collaborative learning. We then exemplify this multimodal approach with a contrasting case study, pinpointing how the multimodal learning analytics framework is applied to investigate the effect of joint attention instruction on dyads in a co-present CSCL scenario. We aim to see how the analyses of multiple modalities could inform each other and to what extent this method is sensitive to any impact of instructing collaborative learners to co-regulate sharing their gaze on the same areas of interest in a concept map environment. We conclude with a summary of the framework and propose a research track on collaborative learning synchrony.
Collaborative learning has typically been conceptualized to encompass social and cognitive processes that are reciprocally intertwined with each other and happening simultaneously. Participating in a collaborative task, learners are forming a social relation that can be instantaneous and dynamically evolving over a short period of learning together. This relation influences how learners build consensus and engage in a task together, both quantitatively in terms of their participation, which we regard as one of the prerequisites to learning collaboratively (see Weinberger and Fischer, 2006), as well as qualitatively regarding the value of their contributions toward solving the task. Other socio-cognitive prerequisites to learning together are some level of engagement and establishing joint attention on the task. The way learners establish and maintain joint attention serves indicating intention, building common ground, and helping to construct shared understanding (Dunham and Moore, 1995; Clark, 1996; Schneider et al., 2018). Building on these basic, required social cues that the learning partners mutually give and receive, schemata in long-term memory activate and facilitate cognitive processes (Schneider et al., 2021). The extent to which learners cue each other to constructively scrutinize and build on each other’s contributions explains some of the vast variation of learners’ cognitive processes working on the task in terms of content adequacy and argumentative quality as well as how learners influence each other’s conceptualization of the problem (Weinberger and Fischer, 2006).
Early collaborative learning studies (Miyake, 1986; Chi et al., 1994) have reasoned that peer collaboration provides learners with opportunities to adjust and adapt their cogitation based on the peer’s contributions. Social constructive activities prompt peer collaborators to elicit explanations from each other and modify each other’s suggestions for solving the task (Fischer et al., 2013). Such mutual scrutiny and critical negotiation may move learners beyond their respective individual capacity and thus make a strong case for scenarios of joint reasoning (Mercier and Sperber, 2017). Nevertheless, studies also show that these productive interactions need to be scaffolded (Weinberger et al., 2010). Collaboration scripts, for instance, that facilitate specific social processes and interaction patterns, such as reviewing each other’s contributions, show favorable effects on cognitive processes of learning. Learners’ social interactions are thus closely tied the cognitive process of learning (Hogenkamp et al., 2021). Therefore, analysis of collaborative learning benefits from a group level perspective, which can bring to light how learners synchronize in social interaction (see section “Synchrony of collaborative learners” below).
Learners jointly regulate the emotional quality of their social interactions. Each contribution to discourse is then loaded with an emotional value for a learner’s self-worth, striving for approval or fearing rejection through peers (Manstead and Fischer, 2001). Thus, learning partners possess interlocking, reciprocal roles of seeking and granting self-worth or not, which can create different dynamics among peers, e.g., an eye-for-an-eye-spiral toward mutual depreciation. These dynamics can be analyzed on both, the individual and group levels. For instance, learners’ emotional shifts in task work can be observed regarding individual emotional change and as an emotional change of the group.
In the following paragraphs, we will first briefly glance at some rudimentary functions of emotion from a psychological perspective. Based on this perspective, we will consider the role of emotion for individual learning, building on the control value theory of achievement emotions. Afterward, we illustrate how emotion works inter-individually with the help of social appraisal theory and emotion contagion theory. Lastly, we exemplify some studies and their findings on emotion in collaborative learning.
Emotion has become more pronounced in educational research as it is closely tied to other psychological processes, for instance, affection, cognition, behavior, and motivation (Pekrun et al., 2002; Pekrun and Linnenbrink-Garcia, 2012). Lane and Nadel (2002) have discussed several aspects of emotion, saying that (a) emotion builds upon one’s cognitive appraisals; (b) emotion is triggered by conscious behaviors; and (c) emotion imposes ramifications to cognitive processes, e.g., perception, attention, and memory. Therefore, emotion connects cognition and behavior and is central in the socio-cognitive processes of collaboration.
Emotion facilitates or hinders cognitive process of learning. Academic achievement-related emotion (Pekrun et al., 2002) supports responses by activating motivations, offering physiological energy, and guiding attention and intentions.
Conventional practice in emotional research is to distinguish various emotions according to the emotional valence. Emotions are categorized as having either positive valence, such as delight and pride, or negative valence, such as hopelessness and frustration (Pekrun and Linnenbrink-Garcia, 2012). Positive emotions typically result in high academic achievement but can also be detrimental to learning in some circumstances. Likewise, negative emotions may have differentiated effects on learning, depending on the environment and the learning task (Pekrun et al., 2002). In the context of learning, we define environment-related emotions as extrinsic emotions and learning task-related emotions as intrinsic emotions. The general postulate of extrinsic emotions is that these emotions introduce off-task cognitive processes in learning, thus reducing task-related cognitive activities, whereas intrinsic emotions are task-oriented and beneficial to learning.
In addition to these assumptions on emotion and learning, academic emotions can be distinguished on the activation dimension (Pekrun, 2006; Pekrun and Linnenbrink-Garcia, 2012). As a result, four categories of emotions are defined according to this valence-activation scheme: positive activating emotions, positive deactivating emotions, negative activating emotions, and negative deactivating emotions. Positive activating emotions are positive emotions that derive from emotional arousal (e.g., enjoyment and pride), whereas positive deactivating emotions are downward shifts from peaks of emotional arousal (e.g., relax and relief). Conversely, negative activating emotions are, for instance, anger and anxiety, and negative deactivating emotions are, for instance, hopelessness and boredom. Among these emotions, positive activating emotions benefit learning, and negative deactivating emotions are harmful.
In later work on academic emotion (Pekrun et al., 2011), nine emotions have been defined as achievement emotions in scenarios of learning, i.e., emotions that pertain to either learning activities or learning outcomes. These emotions are enjoyment, pride, relief, anger, anxiety, shame, hopelessness, and boredom. All these achievement emotions cast effects on learner’s appraisals, motivation, the learning strategies chosen in the learning task, and last but not least, the learning performance (see also Lane and Nadel, 2002). Moreover, depending on the length of the duration of these emotions, we can differentiate emotions as states during a learning situation or as event-specific traits, e.g., enjoying a moment in a task versus enjoying the interaction with peers (Pekrun et al., 2011).
The control value theory of achievement emotions portrayed above is about emotion at individual levels, explaining what emotional shifts an individual learner experiences and appraises. Nonetheless, in a collaborative learning setting, we also need to consider how emotion varies at the group level. We share a basic understanding of group emotion as follows: we first introduce social appraisal theory and emotion contagion theory. In addition, we look back at some studies on emotion in collaborative learning.
Social appraisal theory (Manstead and Fischer, 2001) is based on the assumptions that (a) appraisals are decisive to inner emotional experience; (b) albeit classic appraisals reveal how an individual values an event and relates it to one’s well-being, social appraisals affect social and cultural processes, meaning that thoughts or feelings of one or more other individuals add new appraisals to the existing ones; (c) appraisals are not constant over time, but vary depending on the perceived appraisals from others and appraised current occurrence, because people are curious and sensitive about other’s emotional reactions. It is speculative how learners’ emotions are dependent on control of achieving a task solution versus being dependent on the appraisals of others. But these assumptions explain why our experienced emotions differ from our anticipated emotions before receiving others’ appraisals. For instance, two learners are assigned to a collaborative learning task. One learner may think the learning task is interesting and is curious to learn more and engage in the task. The learning partner, however, may not take the task seriously. In such a constellation, these two learners will evaluate and adjust their individual appraisals based on the appraisals from their peers by either reducing or increasing their respective task engagement to arrive at comparable levels (Fischer et al., 2013).
Manstead and Fischer (2001, p. 10) pointed out the role of appraisals in a social context: “if the dyad or group shares a common fate, it seems likely that social interaction processes will result in an increased agreement in how to appraise stimuli, and a correspondingly greater degree of overlap with respect to emotional experience.” They explained that social appraisal affects shared social emotion and results in amplified emotions in public settings rather than in a private setting. Thus, given a high-stake task, two learners with diverging levels of engagement may be highly likely to converge and experience emotional synchrony.
Social appraisal theory provides one perspective on how emotional shifts depend on other people. The concept of emotion contagion provides an alternative perspective on how emotional congruence can be established over time (Parkinson and Simons, 2009). Interlocutors would mimic each other and reciprocally attain higher levels of emotional synchrony, which facilitates joint meaning-making and knowledge communication. Synthesizing both inborn emotional contagion and achievement emotions as a relation between the individual and the task, one could speculate how collaborators would seek out and establish positive emotions toward one another in relation to the task. In this perspective, collaborators would associate shared perspectives on the task as emotionally positive, sustaining self-worth through mutual recognition and agreement, and vice versa, disagreements as emotionally negative. These differently valent moments of shared emotions may come together and interact with joint or disjointed emotional shifts of activation, which we regard as “being emotionally in- or out-of-sync.”
Existing research on emotion in collaborative learning has two main branches, investigating (a) the effect of a specific emotional condition in collaboration and (b) emotion in the context of regulation responsible for maintaining motivation and engagement in collaboration (Järvenoja and Järvelä, 2009). For example, the differentiated effects of two prompts for inducing strategies and verbalization were that the latter improved quality of talk, but provoked negative emotions in fourth-grade learners (Schmitt and Weinberger, 2019). Another study showed how negative de-activating emotions initiated emotional regulation behavior at a group level, and activating emotions led learners to assist and maintain current regulatory activities. In contrast, positive and neutral de-activating emotions rendered learners to observers and sometimes to objects of others’ regulation (Törmänen et al., 2021).
As illustrated above, we are still left to speculate how emotions at the individual and the group level interplay with one another in the collaborative process, especially in terms of whether learners are in a shared emotional state in the collaborative task, as well as to whether a shared emotional state is beneficial to collaborative activities. Investigating such a dynamic can help us better understand the function of emotion in the knowledge co-construction process and its impact on the trajectory of collaboration. Delving into emotion synchrony promises answers to the questions above and involves multimodal observations to interpret collaborative learning mechanisms.
Synchronization in dyad communication has been conceptualized and operationalized in the forms of temporal and spatial behavior matching and is conducive to mutual understanding (Louwerse et al., 2012). Early studies of the emotional contagion paradigm have discussed mimicry in communication, evoking a boom of mirror neurons research. Some evidence has been collected showing that synchronization results from coordination, revealing that the degree of synchronization is associated positively with successful group work (Richardson and Dale, 2005; Elkins et al., 2009; Louwerse et al., 2012). While mechanisms and effects of being synchronized have been investigated in dyad communication, synchronization in collaborative learning is under-investigated concerning how synchronization can be used and encouraged to support effective collaborative learning.
Being synchronized can be observed only at the group level, where collaborative learners mutually influence each other. So far, several synchronized phenomena have been investigated independently, such as knowledge convergence, transactive dialog, and joint attention. Knowledge convergence is the commonly shared knowledge increment among all collaborators (Jeong and Chi, 2007; Weinberger et al., 2007), indicating the same knowledge acquisition from one group of learners in a specific shared time frame; in other words, it is the synchronization of knowledge acquisition among collaborators. Similarly, transactive dialog refers to the extent to which learners build arguments on the reasoning of their peers (Teasley, 1997); it means that learners are talking about the same thing in a shared time frame of collaboration and build on each other’s ideas. Hence, we can regard it as synchronization in discourse.
Additional insight into both, cognitive and emotional synchrony of collaborative learners can be expected from physiological measures that capture joint attention and physical arousal. Multiple levels of joint attention have been defined in joint attention research aligned with different levels of social attention including shared gaze, dyadic joint attention, and triadic joint attention (Oates and Grayson, 2004; Reddy, 2005; Striano and Stahl, 2005; Leekam and Ramsden, 2006; Okamoto-Barth and Tomonaga, 2006). Shared gaze is defined as the state of two individuals simply looking at the same third object (Butterworth, 2001; Okamoto-Barth and Tomonaga, 2006), constituting two circumstances: (a) two individuals do not know what the other is attending to, and (b) they both are aware that they are attending to the same target (Siposova and Carpenter, 2019). Dyadic joint attention is the state of two individuals orientating their visual attention toward each other, typically having eye contact (Leekam and Ramsden, 2006). And triadic joint attention is defined as one learner establishing eye contact with the peer and then cueing the peer to look at the same object (Oates and Grayson, 2004). Regardless of such intention-fueled, cognitively joint attention and further levels of dyadic and triadic joint attention states, we align with previous studies of collaborative learning that have investigated states of shared gaze as a behavioral measure of joint attention (also known as joint attention).
Physical arousal can be indicated by electrodermal activity (EDA) as the skin changes its electrical conductivity due to sweat gland activity. Some level of arousal could be regarded as a prerequisite to any learning process, especially with tasks that pose a challenge and are therefore emotionally loaded. Investigating the synchrony of physical arousal of two or more learners could then potentially highlight moments, in which learners would experience critical moments of working on the task together. Such moments would be marked by joint emotional shifts, which could then be analyzed for dynamics of positive or negative emotional valence of joint “breakthroughs” or “breakdowns.”
In the following section of the MMLA framework, we discuss how the different process dimensions and qualities of collaborative learning can be analyzed, using different data sources, tools, and approaches. We particularly focus on how gaze matching can be used as a variable in collaborative learning and how physiological data (especially EDA data) can be coupled to measure joint emotional shifts of learners. We use the expression “being in-sync” to describe all these synchronized processes and states of group members across three processes dimensions, i.e., emotion, cognition, and behavior, in collaboration.
As illustrated above, the phenomena of being “in-sync” relate to multiple dimensions and modalities of learning together. We gain understanding of the effects of emotions on collaborative learning processes by looking into instances of being “in-sync” and “out-of-sync.” Thanks to the recent development in multimodal learning analytics research, researchers are able to explore and interpret synchronization of multimodal signals in various contexts, which leads to a new approach of inspecting group interaction (Blikstein and Worsley, 2016; Di Mitri et al., 2018; Järvelä et al., 2020). Based on this notion, we present a multimodal learning analytics framework incorporating emotional, cognitive, and behavioral methods (see Figure 1) in the following sections.
Emotion interplays with multiple factors in the learning process, such as cognition, motivation, and behavior. One of the most common ways to measure emotions is by survey. Pekrun et al. (2011) have constructed a questionnaire for academic achievement emotions evaluation (AEQ). The AEQ contains the measurement of eight emotional states, namely enjoyment, hope, pride, anger, anxiety, shame, hopelessness, and boredom, in three different learning settings, in a class, during learning, and in a test. Such measurement is a robust and straightforward way of arriving at quantitative indices of learners’ emotions. To observe the group level phenomena of being “in-sync,” the variance of the rating scores on the same item of two or more group members can be aggregated and standardized. A low variation coefficient indicates high degrees of being emotionally “in-sync” and vice versa.
However, such measurement is scarce on details and variations of emotions and omits emotional fluctuations over time, thus providing emotional averages of the whole learning session. A temporal perspective on emotions seems necessary to distinguish traits and states of emotions (Pekrun et al., 2011). Moreover, there are obvious downsides to using aggregated, interindividual ways of measuring emotions. Aggregated and interindividual measurement cannot provide as much information as intraindividual analyses because (a) the assumption of homogeneity of the sample ignores the variance of collected data and idealizes the actual sample heterogeneity, and (b) data on learner characteristics varies in different time frames and enlarges the variations of variables (Molenaar and Campbell, 2009). Accounting for the trajectory of emotions across the time span of a collaborative learning experience, mixed methods such as utilizing observational and introspective, or qualitative and quantitative approaches combined with time series analysis seem to be adequate ways of measuring appraisal of emotions through self-reports.
Bodily expressions, such as gestures or mimics, can be used as indicators of learners’ emotions. To analyze young learners’ emotions in a co-present collaborative learning setting with tablets, for instance, a coding scheme has been developed that could reliably determine the positive and negative valence of children learning to reason with proportions based on their gestures only (Schmitt and Weinberger, 2019). Gestures like clapping hands, thumbs up, throwing hands up in the air, and clenching the fist are defined as indicators for positive emotions. Conversely, gestures such as threatening the iPad (the learning device), facing the palms upward, dismissive hand gestures, and face-palming reflect the negative emotions of learners. With this coding scheme, the evaluation of fluctuations of individual emotional states can be observed in fine-grained series and emotional “in-sync” states on the group level. One apparent shortcoming of this coding scheme is that it categorizes emotions only based on their valence rather than the types of emotions that learners experienced in the learning session and the degrees of activation. The impact of positive and negative emotions on collaborative learning cannot be determined based on coding valence alone.
Analyzing facial expressions is another approach to analyzing emotions (Ekman et al., 2002; Barrett et al., 2019), often utilizing tools that automatically detect human emotions in a video recording of facial close-ups. Ekman et al. (2002) have summarized the correspondence of facial expressions and 10 different emotions: amusement, anger, disgust, embarrassment, fear, happiness, pride, sadness, shame, and surprise. Facial expressions for amusement include tilting the head back, a Duchenne smile, separated lips, and a dropped jaw. Anger is shown by furrowed brows, wide eyes, and tightened lips. Disgust is expressed by narrowed eyes, wrinkled nose, parted lips, dropped jaw, and tongue showing. Signs of embarrassment are narrowed eyelids, controlled smile, and head turned away or down. Facial expressions such as eyebrows raised and pulled together, upper eyelid raised, lower eyelid tense, lips parted and stretched usually are found in the emotion of fear. Happiness is expressed through a Duchenne smile. People show pride through heads up and eyes down, while shame is usually associated with head and eyes down. Sadness is usually associated with physical actions such as brows knitted, eyes slightly tightened, lip corners depressed, lower lip raised. Finally, signals of surprise include raised eyebrows, raised upper eyelids, parted lips, and dropped jaw. Thus, categorizing emotions via facial expression allows for a differentiated analysis in comparison to the previously illustrated coding scheme of gestures.
Another method of coding emotion is to register facial expressions at defined points in time, allowing to use time series analysis (see Louwerse et al., 2012). Using the categories Ekman and associates (2002) defined, they have developed a coding scheme registering four main facial areas, namely, head, eyes, eyebrows, and mouth, and applied it to analyze 32 dialogs from four dyads. According to Louwerse et al. (2012), their coding process is “extremely time-intensive” because they coded the facial expression of each individual manually in 250 ms intervals. After the coding process, these data were aligned on a group level according to the timestamps and subjected to a time series analysis. In their case, cross recurrence quantification analysis (CRQA) was applied to match the data from each dyad and observe the occurrence of facial expression “in-sync.” This method is more robust than the “coding only” method since it takes the variance of the value at different time points into account. The time-series analysis also allows for conclusions on the overall degree of being “in-sync” across the whole collaborative session. Nonetheless, one limitation of the methods used in the study above is that the specific facial expression of being “in-sync” between the partners was omitted from the analysis. One needs to refer to the original time series data to find the correspondent value.
Physiological signals are found connected to cognitive processes and emotion which is explained by the function of the autonomous nervous system (ANS) preparing behavior responses (Kreibig, 2010; Critchley et al., 2013). In the past, various sorts of physiological data have been studied in different collaborative learning settings, for instance, electroencephalography (EEG), electrodermal activity (EDA), heart rate (HR), blood volume pressure (BVP), and skin temperature (TEMP; Blanchard et al., 2007; Sharma et al., 2019; Järvelä et al., 2020). Apart from EEG, all these physiological signals can be nowadays collected by wearable sensors such as patches, wristbands, and smartwatches.
To investigate synchronicity in collaborative learning, physiological data coupling aims to discover (dis)similarities between individual signals. In the past, indices were introduced for denoting physiological data differences within a dyad. Pijeira-Díaz et al. (2016) have done a systematic review on six physiological coupling indices for exploring physiological synchrony, namely signal matching (SM), instantaneous derivative matching (IDM), directional agreement (DA), Pearson’s correlation coefficient (PCC), Fisher’s z-transform (FZT), and weighted coherence (WC). The values of these indices indicate the extent to which collaborators are physiologically synchronized.
Among these indices, SM is the absolute difference between normalized paired signals, indicating the overall similarity of two signals. A high SM value corresponds to low signal similarity and vice versa. IDM compares the similarity of slopes, i.e., the rate of physiological change, between two signals at certain moments, providing insights into whether two signals changed at a similar degree. A high IDM value indicates that the changes of two signals vary largely. DA shows the proportion of how much two signals share the same direction, i.e., how much the two signals are increasing or decreasing at the same time. A high DA implies two signals share a similar tendency. PCC represents the linear correlation between signals. Fisher’s z-transform (FZT) is not frequently used since it is converted from PCC, and its interpretation is not as intuitive as PCC. Therefore, PCC is typically chosen in most cases as synchrony index of physiological data (Pijeira-Díaz et al., 2016). WC is a unique index among all six indices because its value varies between 0 and 1, making it more suitable for analyzing the physiological activities with a specific frequency, such as heart rate variations, and breathing patterns (Henning et al., 2001).
In collaborative learning research, these indices have been implemented as indicators of physiological concordance among triads in a study on the relationship between physiological synchrony and peer monitoring in collaborative learning (Malmberg et al., 2019). However, only a vague connection between monitoring and physiological synchrony has been found.
Building on physiological data is not something new but has experienced a second wind in collaborative learning research using wearable biometric units. EDA is one of the common psychometrics related directly to physical arousals governed by the ANS. EDA signals can be collected relatively effortlessly in learning settings and its values can be interpreted intuitively. Therefore, EDA data coupling is more prevalent than coupling of other physiological signals.
In the history of EDA research, neurophysiologists have tried to connect EDA with an individual’s emotional state. Nevertheless, there is no solid empirical evidence so far showing that EDA can be used directly as an emotion indicator (Boucsein, 2012). According to review work by Kreibig (2010), the increase of electrodermal signals has been reported under different emotional states in previous studies. In other words, different emotions do not vary much regarding their EDA signal patterns, showing the impossibility of inferring specific emotions from the EDA signal’s general arousal, whereas the change of emotions – independent of the specific emotion – can be identified by EDA data analysis (Harrison et al., 2010; Critchley et al., 2013). Boucsein (2012) hence recommended a combination of subjective ratings and EDA measurement to evaluate emotions.
In collaborative learning research, EDA data can be used to indicate emotional state changes, and subjective rating data of emotion can specify this variation. As for observing emotional “in-sync” moments, a common approach is to compute the four synchrony indices mentioned above, which are SM, IDM, DA, and PCC (see Elkins et al. (2009) for computation of these indices).
In the previous exploration of EDA synchrony, Pijeira-Díaz et al. (2016) investigated how these four indices are associated with collaborative learning in a study with 16 triads. These triads were assigned to a breakfast design task for athletes in two conditions: with guided or unguided scripts. It turns out that IDM correlates with collaborative will and collaborative learning product, and DA correlates with dual learning gain. In a later study by Pijeira-Díaz et al. (2019), EDA data of 24 groups of triads were observed in a physics course. The results show that the arousal direction of these triads was not always aligned even though they have been in the same environment.
Building on Pijeira-Díaz et al.’s previous study (2016), Schneider and associates (2020) discussed again four of the six aforementioned synchrony indices, including SM, IDM, DA, and PCC. Forty-two dyads participated in their study and have worked collaboratively on a robot programming task. Four groups were allocated in conditions with and without information that encourages collaboration and in conditions with and without visualization of individual contributions. The study has found positive correlations between EDA synchrony and sustaining mutual understanding, dialog management, information pooling, reaching consensus, individual task orientation, overall collaboration, and learning.
In addition, recurrence quantification analysis (RQA) is another possibility to analyze synchronicity in physiological data. Dindar et al. (2019) have implemented such a method to couple the EDA signal of triads in their study. They were able to find a relation between two variables by observing a triad’s collaboration, indicating that the task type is a possible factor that affects the relation between physiological synchrony and shared monitoring since the significant relationship has been only found in one of two learning sessions (Dindar et al., 2019). From this finding, they conclude that this method can visualize the physiological signals in the collaborative processes with which critical moments in learner’s collaboration can be detected. Coupled physiological data allows for precisely identifying joint emotional changes. However, ambiguity still exists regarding the type of emotion that learners are jointly experiencing. In general, the EDA (or physiological signal) approach does not inform about the quality of the emotional states of learners, and therefore, it is barely possible to conclude on the specific emotion being “in-sync,” but instead highlights moments of joint emotional shifts. To further identify the exact type of emotion in such a moment of joint emotional shifts, additional subjective ratings of the emotion of specific moments a posteriori could be additionally collected.
Evaluating how much knowledge has been acquired by the learner in the learning session is still considered a central piece of evidence for learning. The most common practice in this area is by comparing the results of pre-and post-tests. Moreover, it is feasible to evaluate knowledge convergence within a group of learners, i.e., whether two or more learners have learned equivalent amounts of knowledge by analyzing the coefficient of variation on the group level or share knowledge on the same concepts, which could be regarded as two different measures of being cognitively “in-sync” (Weinberger et al., 2007). Nonetheless, the validity of pre-and post-tests and the notion of knowledge as cognitive residue have been heavily discussed, resulting in fewer studies evaluating learning only through the pre-and post-tests method (Nasir et al., 2022). The issues that come along with pre-and post-test measurement are, for instance, having test items of similar difficulty and discriminatory power in both tests. Furthermore, the learning effect from learners needs to be considered when using the same material in both tests. Besides, pre-and post-test results do not disclose details of learners’ knowledge-building process and how one learner’s contribution to discourse influences the peer’s learning trajectory, causing inequivalent or equivalent knowledge acquisition. Therefore, qualitative analysis (mostly discourse analysis) is applied in the majority of collaborative learning research as a supplement to analyzing learning with pre-and post-test.
Discourse analysis focuses on how and what collaborative learners discuss, building on the assumption that discourse is associated strongly with cognitive processes and reflects the knowledge-building process in collaboration (Chi, 1997; Leitão, 2000; Weinberger and Fischer, 2006). Discourse analysis allows comparing the cognitive level at which the respective collaborators are operating at any given moment. Weinberger and Fischer (2006) have developed a framework, exhibiting guidelines for analyzing epistemic and transactive dimensions of collaborative learning. The epistemic dimension discerns between on- and off-task discourse activities and identifies how knowledge is constructed by generating relations between concepts and task information in on-task talk. The sub-categories then depend on the respective concepts and tasks in question, but three facets need to be considered in problem-based learning: the problem space of the learning task, the conceptual space of the learning task, and the connection between the problem space and the conceptual space.
Transactivity implies how learners build up knowledge by referring to and building on their peers’ contributions and is an indicator of how information has been shared and processed within groups (Teasley, 1997; Weinberger and Fischer, 2006; Weinberger et al., 2007). According to Weinberger and Fischer (2006), there are five social modes that indicate the different levels of transactivity, namely externalization, elicitation, quick consensus building, integration-oriented consensus building, and conflict-oriented consensus building. Externalization is defined at the lowest level of transactivity, and conflict-oriented consensus building is at the highest level. The higher transactivity learners reached, the more elaborate arguments they used in the peer discussion, acquired more knowledge individually, shared more and more similar knowledge after the joint learning session (Weinberger and Fischer, 2006; Weinberger et al., 2007). An alternative to measuring transactivity by “shared thematic focus” on the epistemic dimension has shown correlation with analysis by social modes (Weinberger et al., 2013). Here, learners talking about the same concepts, the same problem case information, or both in direct response to one another would be showing high levels of transactivity. Recent efforts aim to conceptualize transactivity based on two qualities, the extent to which learners reference peer contributions and the extent to which learners build new ideas on top of peer contributions (Vogel and Weinberger, unpublished manuscript)1. In this framework, learners reciprocally deepen and widen the collaborative discussion scrutinizing the arguments of one another (Mercier and Sperber, 2017).
Similar to generating the time series of facial expressions mentioned in the previous section, time series analysis could also be applied in discourse analysis based on a specific sampling interval, e.g., each 10th sec. Currently, this method has not been widely applied as it poses a heavy workload to generate discourse time series manually and account for irregularity of progress in natural discourse. Still, this method could reveal discourse patterns of individual learners and group interaction by superimposing the time series of individual learners. Moreover, this method is a perfect fit for multimodal learning analytics where the multimodal data are usually presented in the forms of time series, which means we can align multimodal data and observe connections across multiple data sources, e.g., detecting fluctuation from one modality channel and relate this fluctuation to the data from other channels (Blikstein and Worsley, 2016; Di Mitri et al., 2018).
Using eye movement as an indicator for cognitive processes has been well established, for instance, in research on multimedia learning (Alemdag and Cagiltay, 2018). In collaborative learning research, eye-tracking technology could help to unveil how learners co-regulate their attention and information processing in collaboration through matching their eye movements.
Schneider and colleagues contributed to this specific research track with remote and co-present collaborative learning studies on joint attention (2014, 2018). Their earlier work has proposed an additional way of presenting and analyzing joint attention in collaborative learning (Schneider and Pea, 2014). They leveraged network analysis techniques to augment gaze synchronization visualization of 21 dyads, aiming at improving the conventional method of joint attention analysis, i.e., leaving out information on the visual target of joint attention. In their joint attention network, the nodes represent the screen region, the size of nodes indicates the number of fixations on a specific region, the edge between two nodes corresponds to the saccades between regions, and the width of the edge coincides with the number of saccades. With this new method, they were able to find correlations between numbers of joint attention instances and quality of collaboration, including reaching consensus, information pooling, and time management. In their later work (Schneider et al., 2018), 27 dyads were instructed to work with a tangible-user-interface (TUI) on a warehouse optimization task. Half of them have worked with 2D-TUI, and the rest has worked with 3D-TUI. As a result, a negative association was found between learning gains and the tendency of initiating and responding to joint attention.
Thus, the analysis of coupled gaze time series data allows us to infer whether two learners collaborate with a shared focus of attention. The typical method for coupling eye-tracking data is CRQA (Nüssli, 2011; Chanel et al., 2013; Schneider and Pea, 2014; Schneider et al., 2018). This method is built on the assumption that two dynamic systems’ trajectories “overlap” at the same moment in their respective phase space (Marwan et al., 2007). Recurrence is defined as a shared pattern of two dynamic systems. In the case of eye-tracking analysis, it refers to joint visual attention (Chanel et al., 2013; Schneider and Pea, 2014; Schneider et al., 2018). There are two prominent R packages for conducting cross recurrence quantification analysis, one is crqa package developed by Coco and Dale (2014), and the other is casnet developed by Hasselman (2020). Both packages can perform CRQA for both, discrete and continuous time series.
In many gaze coupling studies on collaborative learning, researchers prefer using an intuitive way to present gaze recurrence (see Schneider and Pea, 2014; Schneider et al., 2018). They chose to use recurrence plot (RP) instead of reporting and interpreting the output measures, e.g., recurrence rate (RR), determinism (DET), and laminarity (LAM). Based on the rudiment principles of categorical CRQA analysis (Hasselman, 2021), RP is a plot that reflects all recurrences of two complex systems, in this case, all instances that collaborative learners looked at the same target synchronously and asynchronously. RR indicates the proportion of recurrence points on the RP, e.g., the percentage of collaborators looking at the same target simultaneously and non-simultaneously. Recurrence points represent the recurrence of learners’ gaze, i.e., two learners looked at the same target at any moment in time, i.e., synchronously or asynchronously.
These recurrence points form lines on the RP, of which the diagonal lines indicate that one learner repeated looking at the same sequence of targets after the peer. The horizontal and vertical lines represent the events in which one learner looked at one target sometime after the peer looked at the same target. DET reveals the percentage of recurrence points falling on diagonal lines, indicating the recurrence pattern of a shared sequence, i.e., two learners look at different objects in the same sequence, and LAM stands for the recurrence points falling on vertical and horizontal lines, which implies the recurrence pattern of revisiting a specific object, e.g., a peer learner looking at the object a long time after a peers’ previous focus on the object. There are pros and cons to these different approaches to CRQA. RPs are intuitive ways of showing the recurrence pattern. However, they allow for qualitative, but not for quantitative comparisons of large data sets, e.g., comparing gaze pattern recurrence of 100 dyads. As conversely for the recurrence measures, they are not as intuitive as RR. Moreover, CRQA is new to many researchers, and it is challenging in terms of data interpretation and drawing conclusions from different measures correctly. Regardless of these mentioned disadvantages, there is obvious merit of working with quantified data: it allows researchers to compare large data sets through a quantitative process, formulating quantitative hypotheses and testing hypotheses via statistical tests, and finally, arrive at inferential results. Thus, the choice of CRQA should hinge heavily on the research question and the collected data.
Besides these common CRQA measures, i.e., RR, DET, and LAM, gaze matching (or any other time series coupling) can be also analyzed by the total number of recurrence points on the RP, the number of recurrence points on diagonal, horizontal, and vertical lines of the RP, as well as anisotropy and asymmetry of the RP, especially in the cases where RPs are not ideal to convey information on differences through visualization. These measures indicate specific features of RPs. Among those measures, the number of recurrence points, the number of recurrence points on the diagonal lines, and the number of recurrence points on vertical and horizontal lines are comparable to RR, DET, and LAM, but with absolute numbers instead of ratios.
Anisotropy is a ratio calculated by the difference between the number of recurrence points on horizontal and vertical lines and divided by the total numbers of recurrence points on horizontal and vertical lines, which reveals whether one learner tends to look at a certain target with a long period after the peer learner’s visit to that target, which is usually interpreted in combination with the asymmetry value. Asymmetry infers the ratio of recurrence points density on the upper triangle versus on the lower triangle of the recurrence plot, showing that one learner’s fixations on shared targets tend to happen earlier than the other learner’s fixations. These two measures emphasize the time lag (asynchrony) of the shared visual focus between two learners, including the case of two learners in triadic joint attention. Therefore, anisotropy and asymmetry measures can be the first indicator of grasping the recurrence points distribution on the plot and inferring the patterns of gaze leading and following of a dyad without referring to a RP.
Gaze coupling with the CRQA method has been intensively implemented in joint attention studies, however, one aspect should not be neglected: the inconsistency caused by the fine grain size of the eye-tracking data, and the nature of joint attention behavior, i.e., the coordination of focusing visual attention on the same target. Eye tracking data is usually collected with high sampling frequencies as eye movements are elusive (e.g., 60, 100, and even 120 Hz), meaning that the fixation data is recorded in milliseconds. Nevertheless, the establishment of joint attention happens usually with a lag of around 2 s (Richardson and Dale, 2005; Richardson et al., 2007), which appears to be inconsistent with the definition of joint attention of two persons looking at the same object simultaneously. Schneider and Pea (2017) and Schneider et al. (2018) have taken this aspect into account in their studies. They applied the 2 s lag in the CRQA analysis of their 2018 study which aided interpretation of the findings.
Gestures are relevant in co-present collaborations with respect to co-regulating the collaborative process, e.g., by providing gaze cues. Gestures can be classified into five categories: beat, deictic, iconic, metaphoric, and symbolic (Louwerse and Bangerter, 2010; Louwerse et al., 2012). According to Louwerse et al. (2012), beat gestures serve following the rhythm of a speech. Deictic gestures are for pointing to the object of interest. Iconic gestures are correspondent to what is said in the conversation, e.g., spreading the arms for indicating something large. Metaphoric gestures are for describing a mentioned concept, e.g., a movement of any object and distance by indicating a (smaller) here-to-there movement with the finger. Finally, symbolic gestures are for conveying “conventional markers,” e.g., thumbs up signaling “ok.”
Gesture use in learning can be traced back to the notion of embodied cognition (Glenberg, 1997, 2010) that the cognitive process is supported to a large extent by various bodily movements. Previous research on embodied cognition in learning has indicated facilitation effects on learning, from counting numbers with fingers in mathematics to pointing and tracing information in anatomy and geometry (Andres et al., 2007; Hu et al., 2015; Korbach et al., 2020). In previous studies, gesture data could be successfully collected either by sensors (e.g., Microsoft Kinect sensor) or by coding video data manually (Louwerse et al., 2012; Schneider and Blikstein, 2015). There are two approaches to analyze “in-sync” gestures: the first approach is through the qualitative comparison of two datasets of each individual, counting the frequency of gesture use in collaboration. The other is by applying the same analysis as the joint gaze pattern coupling. When each collaborative learner’s gesture is aligned with a timestamp, CRQA can be applied to the gesture time series generated from the gesture alignment.
Cross recurrence quantification analysis seems to be a promising method in the context of investigating dyads being “in-sync.” Nevertheless, some pitfalls of this method arise. First, CRQA output does not reflect the category (or the value) that recurred, meaning that we cannot infer the recurred patterns with the CRQA analysis only, i.e., based on the value of the recurrent point and its value change. Schneider and Pea (2014) also pointed out this issue and proposed a network presentation of recurrent gaze patterns (see the previous section). This downside of CRQA implies the necessity of combining descriptive statistics in the interpretation of CRQA data. Second, RR is ambiguous in gaze analysis. High RR values indicate a high recurrence of joint visual attention, but high RR can also be associated with two learners working together only on one or two tiny pieces of information in the whole session (Nüssli, 2011). This problem affords discourse analysis to cross-validate the gaze coupling result.
Participation has been regarded as a prerequisite in collaborative learning and is linked to other qualities of collaboration and learning (Lipponen et al., 2003; Weinberger and Fischer, 2006; Janssen et al., 2007). The measurement of participation can be relatively straightforward, building on a simple count of learners’ contributions. Two facets of participation need to be considered in the analysis, the quantity, and the homogeneity of participation (Weinberger and Fischer, 2006). The quantity of participation is indicated by each collaborator’s contribution, for instance, the number of utterances or the number of arguments brought up by each collaborator. The homogeneity of participation can be measured with the coefficient of variation of the participation measure. A large difference between participation indicates less homogeneity of the participation, which we would regard as being “out-of-sync” regarding participation, and vice versa. The homogeneity of participation can be evaluated for either a whole learning session or for multiple events selected in the learning session.
Taken together, the methods presented above utilize different data sources, such as surveys, EDA recordings, eye tracking, and video recordings, and relate to different dimensions of collaborative learning processes. Combining these methods for multimodal analysis of collaborative learning hinges heavily on the respective research questions of each study but typically includes both qualitative and quantitative methods to analyze “in-sync” and “out-of-sync” events. To provide an example of analyzing multimodal data and “in-sync” events, we present a case study of joint attention in co-present collaborative learning combining some of the methods presented above.
Joint attention is a fundamental ability, which develops at around 9 months of age (Tomasello, 1995). Parents direct their children’s attention toward objects of interest (and learning), and likewise, children call parents’ attention to objects that catch their interest. Joint attention is also a tool for daily communication, indicating our intention, building up common ground, and helping to construct shared understanding with our interlocutor (Dunham and Moore, 1995; Clark, 1996; Schneider et al., 2018).
Researchers found positive effects of joint attention in collaboration from multiple studies. In a study on remote collaborative learning supported with a gaze awareness tool, researchers have found that the number of shared gaze events was higher in the gaze awareness tool condition. Moreover, learners in this condition achieved high learning gain with high-quality collaboration (Schneider and Pea, 2017). Another study on gaze awareness facilitated through a conversational agent designed for remote collaboration has yielded similar results on learning performance and quality of collaboration (Hayashi, 2020). Not surprisingly, learners in co-present collaborative learning also benefit from looking at the same object as their peers. Evidence is accumulating on how shared gaze is advantageous in collaborative learning (Schneider and Blikstein, 2015; Schneider et al., 2018). However, in most co-present collaboration scenarios, learners have faced various challenges when trying to establish joint attention: learners seldom knew where and what needed to be looked at. As a result, they could not catch up with their peer in a learning task, they misinterpreted their peers’ contribution, and they faced phases of disorientation in collaboration. Despite establishing and maintaining joint attention being in our basic behavioral repertoire, these issues beg the question: to what extent can we facilitate collaborative learning by providing learners with gaze instructions? To answer this question, we build on the instructional approach of scripting CSCL, guiding learners to engage in specific collaborative activities.
Guiding learners stepwise through collaborative activities can enhance the co-regulation between learners and scaffold knowledge co-construction processes (Weinberger et al., 2005; Dillenbourg and Tchounikine, 2007; Vogel et al., 2017). Respectively, we assume that by providing learners with joint attention instructions, the frequency of joint visual attention events in face-to-face collaborative tasks rises and, in turn, affects being “in-sync” on other dimensions, i.e., emotion and cognition, too. Therefore, this contrasting case study investigates the following questions:
RQ1: How do joint attention instructions (with versus without) facilitate being “in-sync” on dimensions of emotion in terms of shared emotional states and shifts, cognition regarding transactivities in collaborative discourse, and behavior with respect to joint attention?
RQ2: How do the “in-sync” moments co-occur across the different dimensions of emotion, cognition, and behavior?
The following sections introduce the methods we used for multimodal data collection, followed by a description of how we analyzed the collected data, predicated on the proposed framework in the previous section. Afterward, we report the results of the data analysis and conclude on the findings of this case study.
This section introduces the procedure of data collection and analysis of this contrasting case study, investigating “being in-sync” in dyad collaboration. The goal of this small-scale study is to exemplify how data can be analyzed based on our theoretical and methodological framework, grasping phenomena of joint attention and its facilitation rather than arriving at generalizable conclusions on joint attention.
Four participants (1 male, 3 females) from Saarland University aged 25 to 39 were recruited in this study for course credit. Two dyads were randomly paired aligned with authentic scenarios of university education. Dyads were randomly assigned to a treatment without joint attention instruction (non-JA treatment) or a treatment with joint attention instruction (JA treatment), respectively.
Two separate laptops and one tabletop display were used for presenting the learning tasks. Two laptops were used for the individual learning task, and the tabletop display was dedicated to the collaborative learning task. I.e., the dyads worked together on a large, shared display. Considering the COVID-19 risk during the co-present collaboration, we deployed a transparent partition that allowed learners to stand next to and see each other while being physically divided. Besides, we implemented multiple devices to collect four different types of data in the study, including survey data, video recordings of collaborative learning sessions, eye-tracking data, and electrodermal activity (EDA) data. The two laptops collected survey data through Google Forms2. Two external cameras (Panasonic HC-W858) with their respective table microphones were positioned to catch each learner’s interactions in collaboration. For eye-tracking, we equipped each dyad with two mobile eye-trackers from Tobii AB (Tobii Glasses 2) with a 50 Hz to 100 Hz sampling rate. According to Tobii (2017), the average accuracy of the Tobii eye-tracker glasses is 0.62°, and the average precision (SD) is 0.27° under optimal conditions, i.e., ≤15°. Besides wearable eye-trackers, two wearable EDA recording units (Shimmer3 GSR+) were applied for EDA data collection.
Two learning tasks on “the impact of social media on our life” were designed for this study. An individual reading task provided background knowledge on the learning topic and constituted several research results on the different impacts of social media. The reading task introduced learners to the two most-discussed concepts influenced by social media in scientific research, including well-being (physical and psychological) and social relationships. Additionally, five short summaries of social media studies on different topics were presented in the reading task.
Subsequent to acquiring background knowledge on this topic through the reading task, learners were introduced to a concept map, which reflected the topic of the reading material (see Figure 2). The concept map comprised 22 concepts and 27 links connecting all concepts. To arrive at a joint conclusion, learners were asked to present their opinions about how social media impacts our lives and justify their opinions based on the individual reading and the concept map. Collaborative instructions were distributed to each learner before the start of collaboration which entailed two sections: a task scaffold and a concept map guide. The task scaffold detailed the goals of the collaboration, indicating learners their required steps in collaboration, including mutually presenting opinions and elaborating opinions based on the concept map information. The concept map guide provided a legend of the concept map structure, i.e., the hierarchy of central to outlying nodes and their relations so that learners could quickly read and understand the concept map information. For the dyad with the JA treatment, gaze instruction was added to the task scaffolds section. Gaze instruction reminded participants to bodily point out the very concept whenever they mentioned it and showed that concept to their collaborators.
The experiment lasted around 100 min for each dyad. Due to the COVID-19 restrictions, we have prepared one set of presentation slides containing text, audio, and video instructions for each case to assure a minimized contact between participants and experimenters. Participants could navigate themselves throughout the experiment by going through every presentation slide in front of them. This assured that the dyads received identical instructions, except for the experimental variation. All participants were allowed to ask questions at any time during the session. Study-related questions, e.g., the study’s research question, were answered only after the session. Besides, we also deployed an on-screen timer to inform participants of the remaining time on task.
Participants first read an introduction slide on the procedure and the multimedia content. Then, the participants were asked to read and sign the consent form, taking 5 min overall. Participants were brought to the pre-test and pre-survey stage of the experiment via an external link, assessing prior knowledge and collecting demographics. Pre-test and pre-survey lasted 15 min for each dyad. The pre-survey followed the pre-test, meaning that participants were allowed to access the pre-survey only after submitting their pre-test responses. After that, participants were brought back to the instruction slides and commenced with the individual tasks. Participants were given 10 min to read through the background material individually, and they were instructed to re-read the material when finishing early.
After the individual task, participants were guided to another room for the collaborative learning task. Before starting the collaborative task, participants were guided to wear devices, i.e., EDA recording units and mobile eye-tracking glasses, by following an instructional video with the assistance of the experimenters. The calibration and validation processes were included in the instructions on wearing the eye-trackers. We used one dot calibration in this study which was done by looking at a single dot from a calibration card. Then, collaborative task instructions were given to participants in audio format. Additional gaze instructions were covered in the JA case only. The collaborative scripts were presented on paper and distributed to each participant before the task started. Participants needed to count down together “3–2–1” before collaboration to mark the starting timestamp. The collaborative phase lasted for 20 min. Another validation process was introduced at the end of the collaborative task, supporting the areas of interest (AOIs) definition in the data analysis stage. Before participants went back to the previous room for the post-test and post-survey, they were assisted in taking off all the devices. Post-survey and post-test lasted 20 min. Like pre-test and pre-survey, another external link was given to participants. However, post-survey was precedent in this stage, and participants were asked to answer the survey questions before commencing with the post-test.
The steps portrayed above assured multimodal data collection from two dyads in our collaborative learning setting. The following section illustrates our approach to analyzing and representing the synchronization of social-cognitive processes in our collaborative learning task.
We have analyzed four different modalities’ datasets: emotional items from the survey, EDA data from two pairs of participants, discourse data from two collaborative learning sessions, and eye-tracking data. This section lays out the methods of the case study predicating on the MMLA framework introduced above.
Emotion items from post-survey. Eleven items with five-point bipolar rating scales have been applied for subjective emotion rating. We have evaluated two types of emotions during the collaborative session. One is the academic achievement emotion, adapted from Pekrun et al.’s (2011) questionnaire of achievement emotion and transformed to four sets of bipolar adjective pairs. The other category is the general emotion. We have selected seven from 18 of the bipolar adjective pairs that Bradley and Lang (1994) have used in their study for emotion rating. The academic achievement adjective pairs encompassing bored and joyful, hopeful and hopeless, pride and shame, annoyed and pleased, whereas the general emotion, involving adjective pairs of happy and unhappy, relaxed and stimulated, calm and excited, sleepy and wideawake, dominant and submissive, guided and autonomous, and lastly, influenced and influential.
In order to compare how many similar emotional states have been shared within the two dyads in collaboration, we calculated differences in subject ratings for each case. The smaller the difference value, the more “in-sync” have been the emotional states between the two learners. For better visualization, each dyad’s calculated differences in subjective emotion ratings are presented respectively on the same radar graph. Accordingly, the small plane size on the graph indicates a high degree of emotional states “in-sync” and vice versa.
Electrodermal activity coupling on emotional change. Electrodermal activity data analysis constitutes two major steps, (1) pre-processing data, which entails mainly smoothing data, removing artifacts, as well as extracting the signal of skin conductance response (SCR) from the collected EDA and (2) coupling the dyad’s SCR signals.
We have used Ledalab (Benedek and Kaernbach, 2016), an add-on application for EDA data analysis of MATLAB, for this very purpose. We first used the adaptive smoothing function to smoothen our data and then applied the artifact correction function to remove the artifacts imposed on the signal. The artifacts are removed based on the two criteria introduced by Taylor et al. (2015), namely non-exponential decays of peaks and sudden changes in EDA related to motion. Finally, we have executed the continuous decomposition analysis to extract the SCR signal. Continuous decomposition analysis is an integrated tool in Ledalab that separates SCR and skin conductance level (SCL) signals from skin conductance data (Benedek and Kaernbach, 2010). After pre-processing, we coupled our SCR signals with IDM measures due to this index reflecting the change rate, i.e., the slope, of two signals at any instance (Pijeira-Díaz et al., 2016; Schneider et al., 2020), which we believe, should be the most relevant index to emotional change among the aforementioned indices, positing that emotion state change can be inferred from physiological signal changes as previously mentioned in our framework, i.e., changes of absolute slope values in EDA signal in our case. We followed the calculation formula for IDM from Elkins et al. (2009). The variation of IDM values from the two dyads was smoothened with a 10-s moving window.
Albeit IDM depicts the dyad EDA synchrony well, information on how these signals vary at individual levels would additionally help interpret the direction of the synchrony, i.e., whether the synchrony comes from a simultaneous onset or a simultaneous decrease of emotional excitement. We superimpose standardized individual SCR signals of the same-treatment dyads on the IDM plot (Figures 3A,B) to answer this question. The individual SCR signals were smoothened with the same moving window as the one used for smoothening the IDM index.
Figure 3. Plots of IDM index of the non-JA treatment (A) and JA treatment (B). Selected critical events in two collaborative learning sessions are marked in circles with numbers.
As said, EDA data does not picture all aspects of emotion due to its limitation of not reflecting exact emotional states but only indicating emotional changes. To focus on the emotional processes of these two learning sessions and for systematic sampling, we selected and analyzed unique moments of “in-sync” and “out-of-sync” with the largest absolute IDM index values, i.e., significant drops and peaks on the IDM index plot.
For the non-JA case, critical “in-sync” events could be identified from 4–5 min (event 1), 6–7 min (event 2), 10–11 min (event 3), and 12–14 min (event 4) plus critical “out-of-sync” events from 8.5–9.5 min (event 1′), 17–18 min (event 2′), as well as 18.5–19 min (event 3′).
As for the JA case, the critical “in-sync” events emerged from 5–6 min (event 1), 7.5–8.5 min (event 2), 13–14 min (event 3), 14–15 min (event 4), and 17–18 min (event 5). And the “out-of-sync” events happened from 6.5–7 min (event 1′), 9.5–10.5 min (event 2′), and 18–19 min (event 3′).
We investigated the synchrony directions of those moments by checking SCR signals from individuals. Furthermore, in the phase of discourse analysis, we selected corresponding discourse from these events to explore the accordance of EDA and collaborative discourse in the states of “in-sync” and “out-of-sync,” aiming to find patterns of being “in-sync” and “out-of-sync” across dimensions.
At this stage of analysis, we looked at collaborative discourse of the two dyads for details on their learning process. In this case study, we are interested in the transactivities of the dyads’ discourses. All discourse was segmented based on speaker turns. The five social modes (Weinberger and Fischer, 2006) have been adopted as our coding scheme for transactivities (also see section 1.4.2), including five categories, namely externalization (EX), elicitation (EL), quick consensus building (Q), integration-oriented consensus building (INT), and conflict-oriented consensus building (CON). Two coders have been trained for coding and reached high overall inter-rater reliabilities with Krippendorff’s α = 0.87 (Krippendorff, 2018).
In addition, we zoomed in onto the “in-sync” and “out-of-sync” events selected according to the IDM plot to further infer on the relation between emotion and cognition.
As for joint attention, we describe gaze “in-sync” without presenting a recurrence plot due to the insufficient number of gaze points collected from the areas of interest (AOIs) defined on the concept map. We had defined 22 AOIs on the concept map of the collaborative learning task, i.e., one AOI for each concept. The sizes of AOIs differ based on the size of the concept, with the width ranges between 206 and 360 pixels and height ranges between 68 to 128 pixels. We had an excess number of AOIs and as gaze data was collected via mobile eye-trackers in this study, increasing the possibility of learners not looking at concepts of the learning task.
We first mapped learner’s gaze to the 22 AOIs with the “assisted mapping” function of Tobii Lab pro application. Afterward, the mapped eye-tracking data time were aligned with timestamps and then downsized. Our eye-tracking output contains timestamps and fixation AOIs from collaborative sessions. Due to technical limitation, the start and end timestamps from each case requires further alignment. The reason for downsizing the data is because the original time series from the output was in a high sampling rate (51Hz) which cannot fit the CRQA function of the Casnet package due to the time series length limitation. We have decided to downsize data to 17Hz by resampling original time series. From the downsized time series data, we acquired CRQA measures with the rp_measures function of the Casnet package with the parameter settings for discrete categorical time series: DIM = 1, lag = 1, and RAD = 0.
We focused on three measures of the CRQA output that provide insights into the dynamic of joint visual attention in our collaborative learning session, namely the total number of recurrence points on the recurrence plot, the number of recurrence points on diagonal, horizontal, and vertical lines of the recurrence plot, anisotropy of the recurrence plot, and asymmetry of the plot.
We compared the difference between non-JA and JA dyads’ subjective ratings as presented in the radar graphs below (see Figure 4). The non-JA treatment dyad shared more similar academic and general emotional states than the JA treatment dyad since the plane size of the non-JA treatment is smaller than that of the JA treatment. Moreover, less variation of the item ratings was found in the non-JA treatment.
Figure 4. Radar graph of subjective emotional state rating differences within dyads. The small plane size formed on the radar graph indicates an emotional “in-sync” state.
According to Figures 3A,B, the overall IDM value from the JA dyad is lower than the non-JA dyad. According to the definition of IDM, a high IDM value indicates low signal synchronization. Hence in our case, the JA dyad was more synchronized in SCR change. Besides, more significant IDM value drops have been found in the JA case (n = 5), whereas only 4 significant IDM value drops have been found in the non-JA case. Albeit the different amount of significant “in-sync” instances, we found one EDA “in-sync” event from the non-JA dyad (annotated with number “4”) with a long duration (around 1 min according to the plot), and this is the most prolonged duration observed among all significant IDM value drops of the two case studies.
Furthermore, regarding the individual SCR in the marked events, we found that the directions of the SCR slopes from the non-JA case are consistent with the IDM index while less consistent in the JA case (consistency here means low IDM value should accord with the same direction of the SCR slopes and vice versa). For the non-JA case dyad, the individual SCR slopes were converged in the “in-sync” events and diverged in “out-of-sync” events. In event 2 and event 4, emotional excitements were occurring in both learner C1 and learner C2’s SCR signal. In event 1 and event 3, emotional excitements dwindled from the previous emotional excitements. Nevertheless, the SCR signals of the JA dyad look different. Among the “in-sync” events, only the SCR signal slopes from event 2 were convergent, showing coincident emotional excitements. Among the “out-of-sync” events, however, in event 1′ and event 2′, SCR signal slopes apparently converged, with a concurrent diminishing of emotional excitements in event 1′ and a concurrent occurring of emotional excitements in event 2′.
As for the results from discourse analysis, we have coded 460 discourse segments in total with 250 segments of the control dyad and 210 of the shared gaze dyad (Figures 5A,B). We first compared the transactivity dialog between the two dyads with Chi-squared test, and a significant difference has been found, χ2(4) = 20.57, p < 0.01, showing that regardless of the different numbers of segments, there is a significant difference regarding the distribution of discourse in five transactivity levels in both dyads.
Figure 5. Histograms of different categories of different social modes from control dyad (A) and experimental dyad (B)’s discourse.
Besides, we further investigated the discourse from selected critical events. Due to space limitations, here we only present event 1 and event 1′ of the two dyads. We use learner C to indicate the dyad in the non-JA, Control treatment and learner S for the JA treatment with instructions for Sharing gaze. Discourse from these four events is listed in Table 1.
Table 1. The collaborative discourse in critical EDA events of a non-JA dyad (noted with letter C) and JA dyad (noted with letter S).
Our discourse samples show some commonalities. Learner C1 is initiating discourse on each of the respective sub-topics by an exposition. Learner C1 is taking a critical stance reflecting the negative impact of social media. In response to learner C1’s expositions, learner C2 is transactively referring and adding to learner C1’s points but then turns to exhaust the position, using partly humorous counterexamples of productive use of social media. This strategy yields different results in the two discourse excerpts, however. In event 1, learner C1 and learner C2 were cognitively on the same page. They agreed on each other’s ideas to a large degree and actively added complementary arguments to each other’s contributions. Learner C2’s laughter could be regarded as a moment of shared positive emotion, followed by learner C1 giving learner C2 an affirmative response.
In event 1′, the initial disagreement is not resolved, and they are struggling to find points they both could agree on. Regarding the emotions within this event, the two learners’ giggling indicates some level of positive valence. However, the giggle initiated by learner C1 this time is evaluative of learner C2’s contribution and less favorable than the laugh in event 1. Learner C2 then giggles as well, followed by the talk on the sub-topic breaking down. Learner C1 is losing interest in the ongoing discussion and wonders about continuing talk for the remaining task time. Learner C2 then suggests discussing another concept from the map.
In spite of very similar surface features, learner C2’s struggle for acceptance of her counter-arguments on productive use of social media, while referencing learner C1’s positions, actively guiding the conversation, and reaching a common ground through humor, is differently successful and shows highly varied results on the emotional side of these interactions.
Again, some similarities can be identified between the two discourse samples. Most noticeably, learner S1 is leading the conversation in both excerpts to a large extent. However, in event 1, learner S1 frequently elicits information and invites participation of the learning partner, e.g., using “isn’t it?” and “is it that?”, aiming to establish common ground. Learner S2 keeps reacting positively to learner S1’s arguments. No conflict or disagreement between the two learners is found in this event. Learner S1 giggles in the discourse, indicating a positive emotion. Similar to the laughter in the non-JA treatment, learner S2 reacts positively to learner S1’s giggle. We infer that the two learners were in positive emotional states during this event.
Event 1′ is the opposite of event 1, where both learners showed uncertainty regarding the topic. Learner S2 asked learner S1 for agreement on the argument that social media and anxiety are correlated. However, learner S1 was confused by this statement because she did not see it on the concept map, so she asked learner S2 for clarification. In the same turn, learner S1 again came up with a question regarding the meaning of the word “alleviate.” No agreements were found, and the two learners experienced confusion coming along with negative emotions.
Overall, while event 1 can be characterized by some level of equal back and forth between learner S1 and S2 with learner S2 indicating interest and supportively supplementing learner S1’s consideration, learner S2 does not manage to attract interest in her line of reasoning in event 1′ of the JA case.
Analyzing 11 more critical in and out-of-sync events like these, we found the “in-sync” events share the following similarities: (a) resolution of conflict, meaning that the learners agreed upon each other’s contribution and arrived at shared understanding; (b) light moments of shared mood, where learners were positive to laughter or had joint laughter. On the opposite, “out-of-sync” events share the following characteristics: (a) conflict and rejection, indicating that learners did not agree with each other and failed to reach a shared understanding; (b) confusion and misunderstanding, where learners came up with questions for clarification, or misinterpret peer collaborator’s argument.
The following table (Table 2) shows our result of the CRQA measurement. For the non-JA case, there are 30616 points on the recurrence plot, with 35.4% of those falling on the diagonal lines, 72.0% on vertical lines, and 58.5% on horizontal lines. There are 70622 recurrence points on the recurrence plot in the JA case, with 48.5% of those falling on the diagonal lines, 74.8% on vertical lines, and 74.5% on horizontal lines. There were other differences regarding the two plots’ anisotropy and asymmetry ratio measures. The anisotropy ratio of the non-JA dyad is −0.104, and the recurrence point asymmetry ratio is 0.181, whereas the anisotropy ratio of the JA dyad is −0.002, the recurrence point asymmetry ratio is 0.057.
Overall, more recurrence points can be found in the JA than the non-JA case. This implies that JA dyads had more instances looking at the same AOI than non-JA dyads. Fewer recurrence points fell on the diagonal lines in the non-JA case, meaning that the non-JA case dyad had less shared gaze pattern than the JA case dyad in the learning sessions. Similarly, fewer recurrence points were found falling on vertical and horizontal lines in the non-JA case, indicating that the non-JA case dyad had less shared AOIs than the JA case dyad in the collaborative session. The horizontal and vertical lines infer those events of one learner looking at one AOI for some time after the peer looked at the same AOI. The anisotropy value shows that the number of recurrence points on horizontal and vertical lines was distributed unequally between two dyads. There were more recurrence points on vertical lines than on the horizontal lines in both cases, indicating events in which learner C1 and S2 looked at the AOIs for a long period after their peers’ fixation happened more than the other way round. From the asymmetry value, we conclude that the density of recurrence points on the upper triangle of the plot is higher than the lower triangle of the plot for both cases, indicating learner C2 and learner S1 focused their visual attention on certain AOIs earlier than their peers. We interpret from asymmetry and anisotropy that learner C2 and learner S1 were leading gaze in the learning task, with learner C2 showing more gaze leading instances than learner S1.
The subjective emotional rating revealed that the two learners from the non-JA treatment dyad were more at similar levels of the different emotions during the collaboration than the JA treatment dyad. Additionally, the overall emotional states of the learners in the JA treatment were more varied, indicating the non-JA treatment dyad was more “in-sync” in terms of subjective-perceived emotional states of the whole session than the JA treatment dyad.
The IDM index plot, on the contrary, shows a different “in-sync” pattern. We found that more significant simultaneous emotion changes were detected in the JA dyad. Plus, the overall value (indicated by the curve’s height) of the IDM index line from the JA case is lower than the non-JA case, meaning that two learners in the JA dyad were more synchronized than the non-JA dyad regarding emotional changes. This finding seems to be incongruent with the finding from the subjective emotional rating. However, as mentioned before, EDA synchrony is not an indicator for emotional states but a gauge for emotional changes. Moreover, the subjective ratings are averaged scores of emotional states across the whole learning session. Hence, the ratings are not denotative of the emotion state at any specific moment in the collaborative task.
Our findings on the discrepancy of IDM index and dyad EDA signal slopes in the selected instances infer that synchrony indices are powerful tools for detecting critical moments of collaboration processes among peers. Nevertheless, the synchrony index does not seem sensitive enough to capture synchrony events accurately. It is plausible that the extreme SCR slope values cause the inconsistencies between IDM and SCR values in this case study (e.g., the slope of S2 approximating zero in JA dyad event 3). Thus, the IDM value makes sense mathematically but hardly connects to the real-world context since in these inconsistent “in-sync” events, regardless of the indication of “in-sync” by IDM index, lines of SCR signal of two individuals do not share a similar tendency. This finding is congruent with the findings from Schneider et al. (2020), showing only weak correlations between the IDM index and collaborative learning-related variables.
We can interpret these findings so that the emotional changes from the JA dyad were more “in-sync” than the non-JA dyad, yet a disparate emotional “in-sync” state was developed from these emotional change processes. Despite having more “in-sync” emotional shifts, JA treatment learners shared less similar emotional states than learners of the non-JA treatment. Furthermore, incongruences have been found between IDM value and peer SCR signals, which requires further data points and time series analysis of emotional states throughout the sessions of larger samples.
The discourse analysis showed that a large proportion of discourse segments in the two groups were categorized as externalization, quick consensus building, and conflict-oriented consensus building. Interestingly, we found that the discourse of the two groups differs significantly regarding the distribution of the five categories. For the non-JA case, a large proportion of discourse was classified as externalization and quick consensus building. However, the dyad rarely elicited information from one another. Concerning transactivities, the non-JA case learners were mainly at a low level, indicating that the non-JA case learners were less “in-sync” in terms of relating to one another in talking about a shared topic. The different social modes from the JA treatment dyad, on the other hand, were relatively equally distributed. The same number of segments have been found in the categories of externalization and conflict-oriented consensus building, as well as in the categories of elicitation and integration-oriented consensus building.
The emotional “in-sync” moments selected based on the IDM line plot were characterized by high involvement in discussion in both non-JA and JA cases. Learners constructed arguments on their viewpoints, they agreed and disagreed on what their peers had said, they elicited further explanations and elaborations from their peers, they supplemented their peers’ arguments, and they shared emotions with their peers. At the end of these events, they were able to arrive at a shared understanding. As for the “out-of-sync” events, learners in two dyads were interacting less favorably. We identified occurrences such as off-task discussion, decreased collaborative work, misunderstanding and confusion, disagreement and conflict, and negative emotional experience in these events.
It appears that the emotional “in-sync” and “out-of-sync” moments – as identified by EDA analysis – correspond with cognitive “in-sync” and “out-of-sync” moments – as identified by discourse analysis – implying that learners’ emotional fluctuations are prone to converge when they share the same focus and work collaboratively on the task. Similar findings were reported by Dindar et al. (2022), where shared physiological arousal events were mostly found in collaborative task-related events. Moreover, this result is congruent with Louwerse et al.’s (2012) findings that synchronization in face-to-face communication occurs across different modalities. Furthermore, this finding also substantiates that physiological synchrony is a sensible detector of critical peer collaboration events (Schneider et al., 2020).
In a nutshell, our findings imply that shifts in emotion and knowledge co-construction reciprocally influence each other during collaboration which underlines the need to analyze emotional events, as these emotional events involve emotion regulation that supports beneficial group interaction and pose positive effects to knowledge (Järvenoja and Järvelä, 2009). However, the findings from these events cannot answer the questions regarding the contrast of discourse segment distribution under five social modes. More observations and quantitative analysis are needed to answer this specific question.
Our results on joint attention show that the learners in the JA dyad had more instances of looking at the same thing, i.e., joint attention events in collaboration, indicating that the JA dyad did follow our gaze instruction and intentionally guided peer learners’ gaze to look at the same concept in discussion. Considering synchronization, we found that being “in-sync” in gaze seems to be in line with emotional “in-sync” events because the JA learners were found to be not only more synchronized in gaze, i.e., having a high percentage in shared gaze, but also more synchronized in emotional shifts. Moreover, the tendency to guide the gaze is congruent with how learners co-regulated their discourse. The gaze-leading learners in two cases, i.e., learner C2 and learner S1, were more in the role of guiding their peer learners throughout the collaborative discussion. Different from former studies with a 2-s lag tolerance for more recurrence points, a threshold of milliseconds was applied. As discussed above, a coarser grained approach may be better suited for identifying joint cognitive processes of learning.
To summarize results on RQ1, learners in the non-JA dyad shared more emotional states than the JA dyad, whereas learners in the JA dyad were more synchronized in experiencing emotional shifts than the non-JA dyad. Besides, learners in the JA dyad were “in-sync” at a higher level of transactivity than the non-JA learners. Additionally, learners in the JA dyad had more shared gaze moments than the non-JA dyad; learner C2 and learner S1 have led their partner’s gaze in the discussion more than the other way around. Taken together, the case studies would provide some first glance at how learners could be instructed for joint attention and how this would lead to synchronization on behavioral and emotional dimensions.
With respect to RQ2 on relations between being emotionally and cognitively “in-sync,” considering the discourse samples of critical moments of emotional shifts, we saw a connection between transactive discourse and being emotionally “in-sync.” As for being “in-sync” in emotional change and joint attention, a plausible link is suggested by the similar “in-sync” tendency found in JA and non-JA dyads. However, no salient association between the shared emotional state and joint attention has been found from the data collected. Finally, respecting “in-sync” in gaze and “in-sync” in discourse, our results show that the frequency of shared gaze events corresponds to convergent discourse inferred from the evidence that high degrees of synchronization in gaze and discourse were both observed in the JA dyad. This finding is congruent with the findings that the number of joint attention events positively affects collaboration quality, i.e., group coordination (Schneider et al., 2018; Hayashi, 2020). In sum, we can by no means say, that synchronization is a global phenomenon across different dimensions and modalities of learner interaction, but local co-occurrences of synchronicities can be observed. Detecting moments of being emotionally “in-sync” continues to be a promising approach toward identifying crucial moments of joint learning.
Additionally, the role of our learning task is not neglectable. Unlike the learning tasks on STEAM learning, i.e., learning tasks on Science, Technology, Engineering, the Arts and Mathematics, our task requires a combination of personal opinions and empirical study findings on a topic that people do share some common knowledge on. Further inspections are needed for STEAM learning tasks or other specific fields of study (cf. Dindar et al., 2019). We would expect a similar result but with a clearer pattern on multiple dimensions. Since these learning tasks confine the range of the discussion and simplify the trajectory of peer collaboration, we would expect to find more evident differences on learning outcomes between two dyads and more salient multimodal “in-sync” phenomena.
While serving as proof of concept for multimodal analysis of collaborative learners’ emotions, cognitions, and behaviors being “in-sync” or not, the case study showed some first indications for relations between these group characteristics. Still, scaling up this research would be necessary for inferring any systematic correlations between such “in-sync” events. Second, we have analyzed the emotional states of the whole collaborative session only instead of measuring the emotional states in each critical “in-sync” and “out-of-sync” event as identified by the EDA analysis. While allowing learners to collaborate without interruption, this creates a gap in interpreting the connection between being emotionally and cognitively “in-sync.” Potentially, further case studies could retrospectively interview participants on valence and type of emotion in such events. Third, due to our approach to joint attention analysis, we could not align the gaze analysis findings with the discourse data. Hence, we could not draw a clear picture of the interaction among shared gaze, physiological synchrony, and transactivity from our case study. In conclusion, analysis of one modality may sometimes not align with analyzing other modalities or even obstruct such analysis, e.g., analyzing facial expressions while wearing eye-trackers. In future studies, we aim to delve into the details of how different gaze instructions introduce impacts to collaborative learning regarding emotional and cognitive aspects with a larger sample size.
This paper proposes a MMLA framework that provides suggestions on analyzing phenomena of being “in-sync” in collaborative learning. We applied some of the suggested methods in a case study on joint attention in co-present, computer-supported collaborative learning.
Basic tenets of the MMLA framework are that there are some prerequisites to learning together, which partly root in physiological and behavioral processes. These include some level of physical arousal, joint attention, and participation in social interactions that constitute collaboration. Different from what emotional contagion would suggest, namely that synchronization develops autonomously in communication and collaboration, we emphasize the notion of analyzing the “in-sync” phenomena in collaborative learning across different dimensions. Additional facilitation of “in-sync” on one dimension would make any ripple effects on synchronization across dimensions additionally salient. Building on this, socio-emotional aspects of collaborative learning are intricate, and there is a need for further investigating its inner workings, i.e., the emotional “in-sync” patterns in peer interactions with the help of multimodal data. As suggested by Nasir et al. (2022), multimodal data can precisely detect learners’ productive learning behavior from non-productive behaviors with its rich information. However, the processes of collecting, analyzing, and interpreting multimodal data are challenging. We suggest first decomposing the multiple modalities into three dimensions: emotional, cognitive, and behavioral. Subsequently, respective methods can be applied to collect and analyze data depending on the research questions. The interpretation of the results requires the alignment of multimodal data. The alignment ensures that each instance discovered in a particular modality can be investigated for corresponding patterns of synchronicity in another data stream. Moreover, zooming in and out of the data may aid its interpretation, helping to contextualize and cross-validate the findings. Concentrating merely on fine-grained analysis and ignoring the overall tendencies of the data can be misleading.
One substantial component in the multimodal analysis is leveraging time series analysis. It is exceptionally advantageous in providing reliable and valid information on individual and group interactions. It lends itself for coupling data from the same group of individuals and comparing data from various modalities via the shared time stamps, which allows us to observe the perturbations and select notable occurrences in any collaborative learning scenes. As a result, collaboration patterns can be easily spotted, involving more details on peer dynamics than interpreting static data only in collaborative learning research.
In addition to time series analysis, analyzing the patterns of “in-sync” and “out-of-sync” through data coupling seems fruitful for multimodal analysis of collaborative learning. These patterns are valuable indicators of whether learners are on the same page in a learning task and can be observed across various data modalities. What needs to be handled with care is that “in-sync” and “out-of-sync” states observed in one modality may not work the same or in the same direction in another modality. Being “in-sync” does not mean that the two individuals are in the same state but indicates a similar pattern shared between individuals. The general notion regarding being synchronized from previous research is that “in-sync” states are desirable in collaborative learning (Elkins et al., 2009; Louwerse et al., 2012; Pijeira-Díaz et al., 2016, 2019; Schneider et al., 2018, 2020). Nevertheless, at this point, we could not give a concrete conclusion on whether “in-sync” is absolutely advantageous or “out-of-sync” is entirely detrimental to collaboration. We assume that learners benefit from “in-sync” in collaboration, but they are also served by “out-of-sync” instances they need to struggle through to reach common ground again. Future research will show how such oscillating synchronization among collaborative learners can be modeled and investigate ways to facilitate such productive alignments.
The raw data supporting the conclusion of this article will be made available by the authors, without undue reservation.
Ethical review and approval was not required for the study on human participants in accordance with the local legislation and institutional requirements. The patients/participants provided their written informed consent to participate in this study.
LAL and AW contributed to the conception and design of the study. LAL performed the statistical analysis, interpreted the data for the work, and wrote the first draft of the manuscript. AW revised the work critically. Both authors contributed to manuscript revision, read, and approved the submitted version.
The authors declare that the research was conducted in the absence of any commercial or financial relationships that could be construed as a potential conflict of interest.
All claims expressed in this article are solely those of the authors and do not necessarily represent those of their affiliated organizations, or those of the publisher, the editors and the reviewers. Any product that may be evaluated in this article, or claim that may be made by its manufacturer, is not guaranteed or endorsed by the publisher.
The eye-tracking data analysis of this study was supported by Dr. Fred Hasselman from Radboud University, Netherland. We highly appreciate his valuable suggestions for our CRQA. Besides, we appreciate our colleague Allison Kolling for proofreading as well as all student assistances, who have helped us with data collection and data analysis for this work.
Alemdag, E., and Cagiltay, K. (2018). A systematic review of eye tracking research on multimedia learning. Comput. Educ. 125, 413–428. doi: 10.1016/j.compedu.2018.06.023
Andres, M., Seron, X., and Olivier, E. (2007). Contribution of hand motor circuits to counting. J. Cogn. Neurosci. 19, 563–576.
Barrett, L. F., Adolphs, R., Marsella, S., Martinez, A. M., and Pollak, S. D. (2019). Emotional expressions reconsidered: Challenges to inferring emotion from human facial movements. Psychol. Sci. Public Interest 20, 1–68. doi: 10.1177/1529100619832930
Benedek, M., and Kaernbach, C. (2010). A continuous measure of phasic electrodermal activity. J. Neurosci. Methods 190, 80–91. doi: 10.1016/j.jneumeth.2010.04.028
Benedek, M., and Kaernbach, C. (2016). Ledalab (Version V.3.4.9) [Computer Software]. Available online at: http://www.ledalab.de/ (accessed September 25, 2021).
Blanchard, E., Chalfoun, P., and Frasson, C. (2007). Towards Advanced Learner Modeling: Discussions on Quasi Real-Time Adaptation with Physiological Data. Manhattan, NY: IEEE, 809–813. doi: 10.1109/ICALT.2007.264
Blikstein, P., and Worsley, M. (2016). Multimodal learning analytics and education data mining: using computational technologies to measure complex learning tasks. J. Learn. Anal. 3, 220–238. doi: 10.18608/jla.2016.32.11
Boucsein, W. (2012). Electrodermal activity. Berlin: Springer Science & Business Media. doi: 10.1007/978-1-4614-1126-0
Bradley, M. M., and Lang, P. J. (1994). Measuring emotion: the self-assessment manikin and the semantic differential. J. Behav. Therapy Exp. Psychiatry 25, 49–59. doi: 10.1016/0005-7916(94)90063-9
Butterworth, G. (2001). “Joint visual attention in infancy,” in Blackwell Handbook of Infant Development, eds G. Bremner and A. Fogel (Hoboken, NJ: Blackwell Publishing), 213–240. doi: 10.1002/9780470996348.ch8
Chanel, G., Bétrancourt, M., Pun, T., Cereghetti, D., and Molinari, G. (2013). “Assessment of computer-supported collaborative processes using interpersonal physiological and eye-movement coupling,” in Proceedings of the 2013 Humaine Association Conference on Affective Computing and Intelligent Interaction, Manhattan, NY. doi: 10.1109/ACII.2013.26
Chi, M. T. (1997). Quantifying qualitative analyses of verbal data: a practical guide. J. Learn. Sci. 6, 271–315. doi: 10.1207/s15327809jls0603_1
Chi, M. T., De Leeuw, N., Chiu, M. H., and LaVancher, C. (1994). Eliciting self-explanations improves understanding. Cogn. Sci. 18, 439–477. doi: 10.1207/s15516709cog1803_3
Clark, H. H. (1996). Using language. Cambridge: Cambridge university press. doi: 10.1017/CBO9780511620539
Coco, M. I., and Dale, R. (2014). Cross-recurrence quantification analysis of categorical and continuous time series: an R package. Front. Psychol. 5:510. doi: 10.3389/fpsyg.2014.00510
Critchley, H. D., Eccles, J., and Garfinkel, S. N. (2013). Interaction between cognition, emotion, and the autonomic nervous system. Handb. Clin. Neurol. 117, 59–77. doi: 10.1016/B978-0-444-53491-0.00006-7
Di Mitri, D., Schneider, J., Specht, M., and Drachsler, H. (2018). From signals to knowledge: a conceptual model for multimodal learning analytics. J. Comput. Assist. Learn. 34, 338–349. doi: 10.1111/jcal.12288
Dillenbourg, P., and Tchounikine, P. (2007). Flexibility in macro-scripts for computer-supported collaborative learning. J. Comput. Assist. Learn. 23, 1–13. doi: 10.1111/j.1365-2729.2007.00191.x
Dindar, M., Alikhani, I., Malmberg, J., Järvelä, S., and Seppänen, T. (2019). Examining shared monitoring in collaborative learning: A case of a recurrence quantification analysis approach. Comput. Hum. Behav. 100, 335–344. doi: 10.1016/j.chb.2019.03.004
Dindar, M., Järvelä, S., Nguyen, A., Haataja, E., and Çini İricioğlu, A. (2022). Detecting shared physiological arousal events in collaborative problem solving. Contemp. Educ. Psychol. 69:102050. doi: 10.1016/j.cedpsych.2022.102050
Dunham, P. J., and Moore, C. (1995). “Current themes in research on joint attention,” in Joint attention: Its origins and role in development, eds C. Moore and P. J. Duham (Mahwah, NJ: Lawrence Erlbaum Associates), 15–28.
Ekman, P., Friesen, W. V., and Hager, J. C. (2002). Facial action coding system: the manual: on CD-ROM. Victoria: Research Nexus.
Elkins, A. N., Muth, E. R., Hoover, A. W., Walker, A. D., Carpenter, T. L., and Switzer, F. S. (2009). Physiological compliance and team performance. Appl. Ergon. 40, 997–1003. doi: 10.1016/j.apergo.2009.02.002
Fischer, F., Kollar, I., Stegmann, K., and Wecker, C. (2013). Toward a script theory of guidance in computer-supported collaborative learning. Educ. Psychol. 48, 56–66. doi: 10.1080/00461520.2012.748005
Glenberg, A. M. (1997). What memory is for. Behav. Brain Sci. 20, 1–19. doi: 10.1017/S0140525X97000010
Glenberg, A. M. (2010). Embodiment as a unifying perspective for psychology. Wiley Interdiscip. Rev. 1, 586–596. doi: 10.1002/wcs.55
Harrison, N. A., Gray, M. A., Gianaros, P. J., and Critchley, H. D. (2010). The embodiment of emotional feelings in the brain. J. Neurosci. 30, 12878–12884. doi: 10.1523/JNEUROSCI.1725-10.2010
Hasselman, F. (2020). casnet: A toolbox for studying complex adaptive systems and NETworks (0.1.6). Available online at: https://github.com/FredHasselman/casnet (accessed October 11, 2022).
Hasselman, F. (2021). Recurrence quantification analysis. casnet. Available online at: https://fredhasselman.com/casnet/articles/CRQA.html#computational-load-the-python-solution-pyrqa- (accessed December 10, 2021).
Hayashi, Y. (2020). Gaze awareness and metacognitive suggestions by a pedagogical conversational agent: an experimental investigation on interventions to support collaborative learning process and performance. Int. J. Comput. Support. Collaborat. Learn. 15, 469–498. doi: 10.1007/s11412-020-09333-3
Henning, R. A., Boucsein, W., and Gil, M. C. (2001). Social–physiological compliance as a determinant of team performance. Int. J. Psychophysiol. 40, 221–232. doi: 10.1016/S0167-8760(00)00190-2
Hogenkamp, L., van Dijk, A. M., and Eysink, T. H. (2021). Analyzing socially shared regulation of learning during cooperative learning and the role of equal contribution: a grounded theory approach. Educ. Sci. 11:512. doi: 10.3390/educsci11090512
Hove, M. J., and Risen, J. L. (2009). It’s all in the timing: interpersonal synchrony increases affiliation. Soc. Cogn. 27, 949–960. doi: 10.1521/soco.2009.27.6.949
Hu, F. T., Ginns, P., and Bobis, J. (2015). Getting the point: tracing worked examples enhances learning. Learn. Instr. 35, 85–93. doi: 10.1016/j.learninstruc.2014.10.002
Janssen, J., Erkens, G., Kanselaar, G., and Jaspers, J. (2007). Visualization of participation: Does it contribute to successful computer-supported collaborative learning? Comput. Educ. 49, 1037–1065. doi: 10.1016/j.compedu.2006.01.004
Järvelä, S., Gaševiæ, D., Seppänen, T., Pechenizkiy, M., and Kirschner, P. A. (2020). Bridging learning sciences, machine learning and affective computing for understanding cognition and affect in collaborative learning. Br. J. Educ. Technol. 51, 2391–2406. doi: 10.1111/bjet.12917
Järvenoja, H., and Järvelä, S. (2009). Emotion control in collaborative learning situations: Do students regulate emotions evoked by social challenges. Br. J. Educ. Psychol. 79, 463–481. doi: 10.1348/000709909X402811
Järvenoja, H., Järvelä, S., Törmänen, T., Näykki, P., Malmberg, J., Kurki, K., et al. (2018). Capturing motivation and emotion regulation during a learning process. Front. Learn. Res. 6:85–104. doi: 10.14786/flr.v6i3.369
Jeong, H., and Chi, M. T. (2007). Knowledge convergence and collaborative learning. Instr. Sci. 35, 287–315. doi: 10.1007/s11251-006-9008-z
Korbach, A., Ginns, P., Brünken, R., and Park, B. (2020). Should learners use their hands for learning? Results from an eye-tracking study. J. Comput. Assist. Learn. 36, 102–113. doi: 10.1111/jcal.12396
Kreibig, S. D. (2010). Autonomic nervous system activity in emotion: a review. Biol. Psychol. 84, 394–421. doi: 10.1016/j.biopsycho.2010.03.010
Krippendorff, K. (2018). Content analysis: An introduction to its methodology. Thousand Oaks, CA: Sage publications. doi: 10.4135/9781071878781
Lane, R. D., and Nadel, L. (eds) (2002). Cognitive neuroscience of emotion. Oxford: Oxford University Press, 3–4.
Leekam, S. R., and Ramsden, C. A. (2006). Dyadic orienting and joint attention in preschool children with autism. J. Autism Dev. Disord. 36:185. doi: 10.1007/s10803-005-0054-1
Leitão, S. (2000). The potential of argument in knowledge building. Hum. Dev. 43, 332–360. doi: 10.1159/000022695
Lipponen, L., Rahikainen, M., Lallimo, J., and Hakkarainen, K. (2003). Patterns of participation and discourse in elementary students’ computer-supported collaborative learning. Learn. Instr. 13, 487–509. doi: 10.1016/S0959-4752(02)00042-7
Louwerse, M. M., and Bangerter, A. (2010). Effects of ambiguous gestures and language on the time course of reference resolution. Cogn. Sci. 34, 1517–1529. doi: 10.1111/j.1551-6709.2010.01135.x
Louwerse, M. M., Dale, R., Bard, E. G., and Jeuniaux, P. (2012). Behavior matching in multimodal communication is synchronized. Cogn. Sci. 36, 1404–1426. doi: 10.1111/j.1551-6709.2012.01269.x
Malmberg, J., Haataja, E., Seppänen, T., and Järvelä, S. (2019). Are we together or not? The temporal interplay of monitoring, physiological arousal and physiological synchrony during a collaborative exam. Int. J. Comput. Support. Collaborat. Learn. 14, 467–490. doi: 10.1007/s11412-019-09311-4
Manstead, A. S., and Fischer, A. H. (2001). “Social appraisal. The social world as object of and influence on appraisal processes,” in Appraisal processes in emotion: Theory, methods, research, eds K. R. Scherer, A. Schorr, and T. Johnstone (Oxford: Oxford University Press).
Marwan, N., Romano, M. C., Thiel, M., and Kurths, J. (2007). Recurrence plots for the analysis of complex systems. Phys. Rep. 438, 237–329. doi: 10.1016/j.physrep.2006.11.001
Mercier, H., and Sperber, D. (2017). The Enigma of Reason. Cambridge, MA: Harvard University Press. doi: 10.4159/9780674977860
Miyake, N. (1986). Constructive interaction and the iterative process of understanding. Cogn. Sci. 10, 151–177.
Molenaar, P. C., and Campbell, C. G. (2009). The new person-specific paradigm in psychology. Curr. Dir. Psychol. Sci. 18, 112–117. doi: 10.1111/j.1467-8721.2009.01619.x
Nasir, J., Kothiyal, A., Bruno, B., and Dillenbourg, P. (2022). Many are the ways to learn identifying multimodal behavioral profiles of collaborative learning in constructivist activities. Int. J. Comput. Support. Collaborat. Learn. 16, 485–523. doi: 10.1007/s11412-021-09358-2
Nüssli, M. A. (2011). Dual eye-tracking methods for the study of remote collaborative problem solving. Ph. D. thesis. Lausanne: École Polytechnique Fédérale de Lausanne.
Oates, J. E., and Grayson, A. E. (2004). Cognitive and language development in children. New York, NY: Open University Press.
Okamoto-Barth, S., and Tomonaga, M. (2006). “Development of joint attention in infant chimpanzees,” in Cognitive development in chimpanzees, eds T. Matsuzawa, M. Tomonaga, and M. Tanaka (Tokyo: Springer), 155–171. doi: 10.1007/4-431-30248-4_10
Parkinson, B., and Simons, G. (2009). Affecting others: social appraisal and emotion contagion in everyday decision making. Pers. Soc. Psychol. Bull. 35, 1071–1084.
Pekrun, R. (2006). The control-value theory of achievement emotions: assumptions, corollaries, and implications for educational research and practice. Educ. Psychol. Rev. 18, 315–341.
Pekrun, R., Goetz, T., Frenzel, A. C., Barchfeld, P., and Perry, R. P. (2011). Measuring emotions in students’ learning and performance: The Achievement Emotions Questionnaire (AEQ). Contemp. Educ. Psychol. 36, 36–48. doi: 10.1016/j.cedpsych.2010.10.002
Pekrun, R., Goetz, T., Titz, W., and Perry, R. P. (2002). Academic emotions in students’ self-regulated learning and achievement: A program of qualitative and quantitative research. Educ. Psychol. 37, 91–105. doi: 10.1207/S15326985EP3702_4
Pekrun, R., and Linnenbrink-Garcia, L. (2012). “Academic emotions and student engagement,” in Handbook of research on student engagement, eds S. L. Christenson, A. L. Reschly, and C. Wylie (Boston, MA: Springer). doi: 10.1007/978-1-4614-2018-7_12
Pijeira-Díaz, H. J., Drachsler, H., Järvelä, S., and Kirschner, P. A. (2016). “Investigating collaborative learning success with physiological coupling indices based on electrodermal activity,” in Proceedings of the sixth international conference on learning analytics & knowledge, Edinburgh. doi: 10.1145/2883851.2883897
Pijeira-Díaz, H. J., Drachsler, H., Järvelä, S., and Kirschner, P. A. (2019). Sympathetic arousal commonalities and arousal contagion during collaborative learning: How attuned are triad members? Comput. Hum. Behav. 92, 188–197. doi: 10.1016/j.chb.2018.11.008
Reddy, V. (2005). “Before the ‘third element’: understanding attention to self,” in Joint attention: Communication and other minds, eds N. Eilan, C. Hoerl, T. McCormack, and J. Roessler (Oxford: Oxford University Press). doi: 10.1093/acprof:oso/9780199245635.003.0005
Richardson, D. C., and Dale, R. (2005). Looking to understand: The coupling between speakers’ and listeners’ eye movements and its relationship to discourse comprehension. Cogn. Sci. 29, 1045–1060. doi: 10.1207/s15516709cog0000_29
Richardson, D. C., Dale, R., and Kirkham, N. Z. (2007). The art of conversation is coordination. Psychol. Sci. 18, 407–413. doi: 10.1111/j.1467-9280.2007.01914.x
Schmitt, L. J., and Weinberger, A. (2018). “Computer-supported collaborative learning: Mediated and co-present forms of learning together,” in Second Handbook of Information Techonology in Primary and Secondary Education, eds J. Voogt, G. Knezek, R. Christensen, and K.-W. Lai (Berlin: Springer), 217–231. doi: 10.1007/978-3-319-71054-9_15
Schmitt, L. J., and Weinberger, A. (2019). Fourth graders’ dyadic learning on multi-touch interfaces—versatile effects of verbalization prompts. Educ. Technol. Res. Dev. 67, 519–539. doi: 10.1007/s11423-018-9619-5
Schneider, B., and Blikstein, P. (2015). Unraveling students’ interaction around a tangible interface using multimodal learning analytics. J. Educ. Data Min. 7, 89–116.
Schneider, B., Dich, Y., and Radu, I. (2020). Unpacking the relationship between existing and new measures of physiological synchrony and collaborative learning: A mixed methods study. Int. J. Comput. Support. Collaborat. Learn. 15, 89–113. doi: 10.1007/s11412-020-09318-2
Schneider, B., and Pea, R. (2014). Toward collaboration sensing. Int. J. Comput. Support. Collaborat. Learn. 9, 371–395. doi: 10.1007/s11412-014-9202-y
Schneider, B., and Pea, R. (2017). “Real-time mutual gaze perception enhances collaborative learning and collaboration quality,” in Educational media and technology yearbook eds M. Orey and R. Branch (Cham: Springer), 99–125. doi: 10.1007/978-3-319-45001-8_7
Schneider, B., Sharma, K., Cuendet, S., Zufferey, G., Dillenbourg, P., and Pea, R. (2018). Leveraging mobile eye-trackers to capture joint visual attention in co-located collaborative learning groups. Int. J. Comput. Support. Collaborat. Learn. 13, 241–261. doi: 10.1007/s11412-018-9281-2
Schneider, S., Beege, M., Nebel, S., Schnaubert, L., and Rey, G. D. (2021). The Cognitive-Affective-Social Theory of Learning in digital Environments (CASTLE). Educ. Psychol. Rev. 34, 1–38. doi: 10.1007/s10648-021-09626-5
Sharma, K., Olsen, J. K., Verma, H., Caballero, D., and Jermann, P. (2021). “Challenging joint visual attention as a proxy for collaborative performance,” in Proceedings of the 14th International Conference on Computer-Supported Collaborative Learning - CSCL 2021, eds C. E. Hmelo-Silver, B. De Wever, and J. Oshima (Bochum: International Society of the Learning Sciences), 91–98.
Sharma, K., Pappas, I., Papavlasopoulou, S., and Giannakos, M. (2019). “Towards automatic and pervasive physiological sensing of collaborative learning,” Proceedings of the 13th International Conference on Computer Supported Collaborative Learning (CSCL), Lyon.
Siposova, B., and Carpenter, M. (2019). A new look at joint attention and common knowledge. Cognition 189, 260–274. doi: 10.1016/j.cognition.2019.03.019
Striano, T., and Stahl, D. (2005). Sensitivity to triadic attention in early infancy. Dev. Sci. 8, 333–343. doi: 10.1111/j.1467-7687.2005.00421.x
Taylor, S., Jaques, N., Chen, W., Fedor, S., Sano, A., and Picard, R. (2015). “Automatic identification of artifacts in electrodermal activity data,” in Proceedings of the 2015 37th Annual International Conference of the IEEE Engineering in Medicine and Biology Society (EMBC), Manhattan, NY. doi: 10.1109/EMBC.2015.7318762
Teasley, S. D. (1997). “Talking about reasoning: How important is the peer in peer collaboration?,” in Discourse, tools and reasoning, eds L. B. Resnick, R. Saljo, C. Pontecorvo, and R. Burge (Berlin: Springer). doi: 10.1007/978-3-662-03362-3_16
Tobii, A. B. (2017). Eye tracker data quality report: Accuracy, precision and detected gaze under optimal conditions-controlled environment. Sweden: Tobii Technology.
Tomasello, M. (1995). “Joint attention as social cognition,” in Joint attention: Its origins and role in development, eds C. Moore and P. J. Dunham (Mahwah, NJ: Lawrence Erlbaum Associates, Inc).
Törmänen, T., Järvenoja, H., and Mänty, K. (2021). All for one and one for all–How are students’ affective states and group-level emotion regulation interconnected in collaborative learning? Int. J. Educ. Res. 109:101861. doi: 10.1016/j.ijer.2021.101861
Vogel, F., Wecker, C., Kollar, I., and Fischer, F. (2017). Socio-cognitive scaffolding with computer-supported collaboration scripts: A meta-analysis. Educ. Psychol. Rev. 29, 477–511. doi: 10.1007/s10648-016-9361-7
Weinberger, A., Ertl, B., Fischer, F., and Mandl, H. (2005). Epistemic and social scripts in computer–supported collaborative learning. Instr. Sci. 33, 1–30. doi: 10.1007/s11251-004-2322-4
Weinberger, A., and Fischer, F. (2006). A framework to analyze argumentative knowledge construction in computer-supported collaborative learning. Comput. Educ. 46, 71–95. doi: 10.1016/j.compedu.2005.04.003
Weinberger, A., Marttunen, M., Laurinen, L., and Stegmann, K. (2013). Inducing socio-cognitive conflict in Finnish and German groups of online learners by CSCL script. Int. J. Comput. Support. Collaborat. Learn. 8, 333–349. doi: 10.1007/s11412-013-9173-4
Weinberger, A., Stegmann, K., and Fischer, F. (2007). Knowledge convergence in collaborative learning: Concepts and assessment. Learn. Instr. 17, 416–426. doi: 10.1016/j.learninstruc.2007.03.007
Keywords: co-present, CSCL, emotion, joint attention, synchronization, multi-modal learning analytics
Citation: Aoyama Lawrence L and Weinberger A (2022) Being in-sync: A multimodal framework on the emotional and cognitive synchronization of collaborative learners. Front. Educ. 7:867186. doi: 10.3389/feduc.2022.867186
Received: 31 January 2022; Accepted: 29 June 2022;
Published: 15 July 2022.
Edited by:
Hanna Järvenoja, University of Oulu, FinlandReviewed by:
Anna Shvarts, Utrecht University, NetherlandsCopyright © 2022 Aoyama Lawrence and Weinberger. This is an open-access article distributed under the terms of the Creative Commons Attribution License (CC BY). The use, distribution or reproduction in other forums is permitted, provided the original author(s) and the copyright owner(s) are credited and that the original publication in this journal is cited, in accordance with accepted academic practice. No use, distribution or reproduction is permitted which does not comply with these terms.
*Correspondence: Lena Aoyama Lawrence, bC5hb3lhbWEubGF3cmVuY2VAZ21haWwuY29t; Armin Weinberger, YS53ZWluYmVyZ2VyQGVkdXRlY2gudW5pLXNhYXJsYW5kLmRl
Disclaimer: All claims expressed in this article are solely those of the authors and do not necessarily represent those of their affiliated organizations, or those of the publisher, the editors and the reviewers. Any product that may be evaluated in this article or claim that may be made by its manufacturer is not guaranteed or endorsed by the publisher.
Research integrity at Frontiers
Learn more about the work of our research integrity team to safeguard the quality of each article we publish.