- 1Learning and Educational Technology Research Unit, Faculty of Education, University of Oulu, Oulu, Finland
- 2School of Computing, University of Eastern Finland, Joensuu, Finland
Emotions in collaborative learning both originate from and are externalized in students’ socio-emotional interactions, and individual group members evidently contribute to these interactions to varying degrees. Research indicates that socio-emotional interactions within a group are related with the occurrence of co- and socially shared regulation of learning, which poses a need to study individual contributions to these interactions via a person-centered approach. This study implements multimodal data (video and electrodermal activity) and sequence mining methods to explore how secondary school students’ (n = 54, 18 groups) participation in socio-emotional interactions evolved across a series of collaborative tasks. On this basis, it identifies subgroups of students with distinct longitudinal profiles. Furthermore, it investigates how students with different socio-emotional interaction profiles contributed to their groups’ regulation of learning. Three profiles were identified: negative, neutral, and diverse. Each profile represents a particular socio-emotional interaction pattern with unique characteristics regarding the emotional valence of participation and physiological emotional activation. The profiles relate to students’ contributions to group regulation of learning. Students with the diverse profile were more likely to contribute to regulation, whereas the neutral profile students were less likely to contribute. The results highlight the importance of person-centered methods to account for individual differences and participation dynamics in collaborative learning and consequently clarify how they relate to and influence group regulation of learning.
Introduction
In collaborative learning, emotions often originate from and are constructed and expressed in groups’ socio-emotional interactions (Järvenoja and Järvelä, 2013). Previous research evidence that socio-emotional interactions affect numerous factors in collaborative learning (Linnenbrink-Garcia et al., 2011; Mänty et al., 2020) and can either foster or inhibit the regulation of learning (Rogat and Adams-Wiggins, 2015; Järvelä et al., 2016), which involves the group members’ abilities to monitor, control, and redirect their cognition, motivation, emotions, and behavior (Hadwin et al., 2018). Previous research has mostly analyzed these phenomena at the group level and adopted a variable-centered approach to study the relations between variables (Reimann, 2009). However, findings have revealed individual differences among students in terms of how they experience emotions and engage in the regulation of learning (Ganotice et al., 2016; Li et al., 2020; Karamarkovich and Rutherford, 2021), which raises the question of whether the associations between socio-emotional interactions and regulation that were found by group-level analyses can be generalized to the different individuals in a group.
Variable-centered methods analyze general patterns by using data from all students. For instance, they may target the correlation between discrete learning processes and achievement (Rosato and Baer, 2012; Hickendorff et al., 2018). The aim of such methods is to derive a general pattern that is assumed to represent all students (Rosato and Baer, 2012). However, students—and humans in general—are heterogenous and present significant individual differences. Generalizations do not capture individual variations, and pooling students into a “central average” is reductive and hardly representative of the complex reality (Winne, 2017; Saqr and López-Pernas, 2021a,b). According to Winne (2017), means, samples, and populations poorly reflect individual students and cannot reliably forecast individual responses to interventions (Winne, 2017). In contrast, person-centered methods account for heterogeneity and individual differences by identifying “hidden patterns,” “latent classes,” and “profiles” or subgroups of individuals who are similar to each other, which are distinct from other subgroups (Rosato and Baer, 2012; Hickendorff et al., 2018). Person-centered methods commonly employ a clustering technique, such as latent class analysis, to discover classes of students’ engagement, self-regulation, and strategies, among other elements (Hickendorff et al., 2018). Previously, person-centered methods have been used to discover subgroups of students’ emotional profiles and cognitive differences (Quirk et al., 2013; Robinson et al., 2017), distinct profiles of motivation and engagement (Xie et al., 2020; Zhen et al., 2020), online strategies of learning programming, patterns of longitudinal strategies (López-Pernas and Saqr, 2021), and various profiles of students’ regulation of learning (Li et al., 2020; Malmberg et al., 2021). Such methods can clarify the dynamics of collaborative learning processes, such as the “when” and “to where” of the evolution of a group’s regulated learning process over time, by accounting for individual differences in students’ learning behaviors.
This study seeks to expand the previous research on emotions and regulation in collaborative learning by adopting a person-centered approach to distinguish students’ socio-emotional interaction profiles based on multimodal data, namely video observations and measurement of sympathetic arousal through electrodermal activity (EDA), and sequence mining methods. The study considers the temporal aspects of students’ socio-emotional interactions and their progression over time. The contribution to the literature is twofold: first, it combines a person-centered approach with the temporal aspect of socio-emotional interactions; second, it illuminates the association between profiles and regulation in collaborative learning.
Socio-Emotional Interactions and Regulation in Collaborative Learning
In collaborative learning, shared understandings, meanings, and solutions are constructed through students’ interactions (Roschelle and Teasley, 1995). Studies have demonstrated that this process of sharing and extending each other’s understandings is beneficial to enhance individual learning (Roscoe and Chi, 2008; Sinha et al., 2015). However, collaborative learning is not inherently successful; it requires students to engage in the regulation of learning by monitoring, controlling, and redirecting their group’s cognitive and socio-emotional processes (Barron, 2003; Hadwin et al., 2018). Co- and socially shared regulation refer to individuals’ actions toward regulating other group members and the group learning process (co-regulation) as well as group members’ shared reciprocal negotiations, whereby they collectively build on each other’s regulatory contributions through socially shared regulation to overcome socio-emotional and cognitive obstacles (Hadwin et al., 2018). Group members can also engage in a socio-emotional interaction as an operation to cultivate and maintain a favorable socio-emotional atmosphere (Törmänen et al., 2022). Socio-emotional interaction consists of purposeful interchanges between students to express and shape perceptions of emotions and the group’s socio-emotional atmosphere (Kreijns et al., 2003; Bakhtiar et al., 2018; Mänty et al., 2020). Positive socio-emotional interactions have been found to facilitate co- and socially shared regulation of learning (Rogat and Linnenbrink-Garcia, 2011; Lajoie et al., 2015; Rogat and Adams-Wiggins, 2015). Meanwhile, negative socio-emotional interactions hinder the collaborative learning process by affecting the quality of group learning activities (Rogat and Adams-Wiggins, 2015) and have been linked to negative emotional experiences of collaboration among group members (Mänty et al., 2020). Accordingly, socio-emotional interactions and group regulatory processes in combination form a basis for understanding how students engage in collaborative learning as well as how group members collectively construct and maintain favorable grounds for learning together (Järvenoja and Järvelä, 2013).
Person-centered research has evidenced that learning contexts involve a multiplicity of individual differences in students’ emotions (Ganotice et al., 2016; Robinson et al., 2017; Karamarkovich and Rutherford, 2021) and regulation behaviors (Ben-Eliyahu and Linnenbrink-Garcia, 2013; Li et al., 2020; Törmänen et al., 2021a). Karamarkovich and Rutherford (2021) have examined the emotional profiles of elementary mathematics students and identified two positive emotions profiles, one negative emotions profile, and a mixed emotions profile. Furthermore, Robinson et al. (2017) have discovered four affective profiles (positive, deactivating, negative, and moderate-low) among college students. In both studies, negative profile students were more disengaged and displayed lower levels of achievement compared to students with positive profiles. Interestingly, both studies describe a mixed profile of students who reported experiencing both positive and negative emotions during the learning process (Robinson et al., 2017; Karamarkovich and Rutherford, 2021). To study the interrelations between students’ affective states and regulation behaviors, Törmänen et al. (2021a) have investigated the co- and socially shared emotion regulation events of students during a collaborative task and found that regulation was more likely to be initiated by students who were in a negative de-activated state and thus had a personal need to restore their emotional grounds. In addition, regulation was more influential in changing a student’s affective state if the student was initiating the regulation in the group or was the target of co-regulation. Therefore, individual differences in students’ emotional states and profiles can impact how they contribute to the regulation of their group’s learning process.
Studying Regulation in Learning With Multimodal Process Data
In recent years, researchers in the field of regulation of learning have adopted a process-oriented perspective that considers regulation as a process evolving through changes, phases, and sequences instead of focusing solely on static, trait-like variables and their relations (Bannert et al., 2014; Molenaar and Järvelä, 2014). These studies have, for example, revealed a range of patterns and profiles for how students regulate their learning in collaboration (Malmberg et al., 2021) as well as different patterns in sequences of interactions as regulation unfolds over time (Mänty et al., 2022). This movement has been partly enabled by recent advancements in the collection of multimodal data. Such data derive from multiple channels, some of which extend beyond spoken or written language (e.g., physiological reactions, facial expressions, and video recordings, including gestures and tones of voice; Noroozi et al., 2020). Since the first implementation of these new opportunities for multimodal data, regulated learning research has focused on the group-level processes underway in collaborative learning in addition to individual learning settings (Järvenoja et al., 2018).
Combining multiple data channels allows researchers to notice invisible physiological markers of the regulated learning of groups. For example, Malmberg et al. (2019) have explored the connection between groups’ metacognitive monitoring and physiological synchrony (i.e., any interdependent or associated activity in the physiological processes of two or more individuals; Palumbo et al., 2017) in the context of collaborative learning. They located episodes of metacognitive monitoring in video data and then assessed the connection of physiological arousal to metacognitive monitoring and group members’ physiological synchrony. The results illustrate that metacognitive monitoring related to physiological arousal, and physiological synchrony occurred especially in situations in which the group struggled with the task. Likewise, by combining multimodal data channels, the present study seeks to uncover invisible markers of emotions, which may broaden knowledge of how emotional reactions accompany socio-emotional interactions and regulation as they occur (Malmberg et al., 2019; Törmänen et al., 2021a). An emerging body of research (e.g., Harley et al., 2015, 2019; Törmänen et al., 2021b) has investigated physiological arousal in connection with the activation dimension of emotions in the affective circumplex model (Russell and Barrett, 1999), which reflects the degree to which an emotion is physiologically arousing (Pekrun, 2006). Evidence suggests that high arousal of students in a learning situation relates to both a negative (Harley et al., 2019; Malmberg et al., 2019) and a positive affect (Törmänen et al., 2021b). Moreover, research findings have associated physiological synchrony with socio-emotional group processes, such as emotional engagement (Slovák et al., 2014), feelings of non-belonging to the group (Mønster et al., 2016), and the construction and maintenance of a common social and affective space (Cornejo et al., 2017). However, in group learning situations, individuals can respond differently to (emotional) stimuli (Gross and John, 2003; Lobczowski, 2020). There is a lack of research on the amount of diversity of patterns in students’ emotions or the physiological reactions and regulation connected to them in collaborative learning situations. By examining the diversity of students’ collaborative learning behaviors together with their physiological reactions, the present study expects to develop a more realistic and non-reductionist view of the diversity of student profiles.
Aim and Research Questions
Previous research utilizing multimodal data in a collaborative learning context has mainly focused on shared group processes, such as physiological synchrony, that occur between group members. However, when integrated with person-centered methods, multimodal data can offer new possibilities for also studying the differences between individuals in a group. By conducting a person-centered analysis of multimodal data to explore how students participate in their group’s socio-emotional interactions across a collaborative learning period, the present research aims to identify distinct socio-emotional interaction profiles of students. Furthermore, it investigates how students with different socio-emotional interaction profiles contribute to their group’s regulation of learning. The study addresses two research questions:
1. Which longitudinal socio-emotional interaction profiles do students exhibit in collaborative learning, and what are their characteristics?
2. How do students with different socio-emotional interaction profiles contribute to group regulation of learning?
Materials and Methods
Participants and Context
The study was conducted in an actual science classroom at a Finnish secondary school. The research participants were 94 voluntary students—58 females and 36 males—who were around 13 years of age. The participants were from five classrooms of one secondary school. The data (Järvelä et al., 2021) were collected throughout a collaborative learning period consisting of four once-a-week 90-min science lessons during which the students were in small groups studying topics concerning light and sound. The science topic was derived from the national physics curriculum and students who did not agree to participate in the research studied the topic following the same pedagogical structure in a different classroom. The students’ last science grades from the preceding semester were collected and used to divide the students into heterogeneous groups based on their prior knowledge. The groups remained the same across the four sequential lessons. Each lesson followed a specific collaborative learning design, and the groups performed several collaborative learning tasks during the lessons. The collaborative learning design was based on the idea of a “flipped classroom.” That is, the students independently studied the upcoming topic in their science textbook prior to each lesson. At the beginning of the lesson, the teacher first introduced the new topic and ensured that each student had enough knowledge to engage in collaborative learning. Then, most of the lesson time was devoted to collaborative work in small groups. Each lesson ended with teacher-led discussions and conclusions and the provision of homework related to the topic of the next lesson (for more information about the collaborative learning design, see Järvenoja et al., 2020b). To ensure validity, the final analysis excluded five groups of four members, one dyad and six groups with poor-quality EDA data or many absences among group members. The study ultimately includes data from 54 students (31 females and 23 males) and 18 groups of three with a data set of 68 learning sessions (∼102 h of recorded video and EDA), as four sessions were missing due to absences.
Data Collection
Students’ collaborative learning interactions were videotaped with four Insta360 Pro 360°cameras, which were placed around the classroom, and audio was recorded with a separate table microphone for each small group. In addition, students’ sympathetic arousal was recorded with Shimmer 3 GSR + sensors (Realtime Technologies Ltd., Dublin, Ireland), which measured EDA at a sampling rate of 128 Hz. The measurement device included two gel electrodes that were placed at the thenar and hypothenar eminences on the palm of each student’s non-dominant hand (Dawson et al., 2007). The researchers observed the data collection from a separate room. To ensure the data quality, the data collection procedure was piloted during a 1-week pilot including one collaborative learning session for each group. The collected video and EDA data were used to detect students’ participation in socio-emotional interactions with different emotional valences as well as their underlying physiological emotional activation during the collaborative learning process. Furthermore, the video data were analyzed to observe the students’ contributions to the group-level regulation of learning.
Data Analysis
The data analysis began with a multistep procedure for coding the video data, which progressed from group-level socio-emotional interaction coding to a more detailed coding of individual students’ participation in interactions (Figure 1). The multistep video analysis covered students’ participation in socio-emotional interactions with different emotional valences. In addition, the EDA data were incorporated as an indicator of students’ emotional activation and integrated with the video analysis.
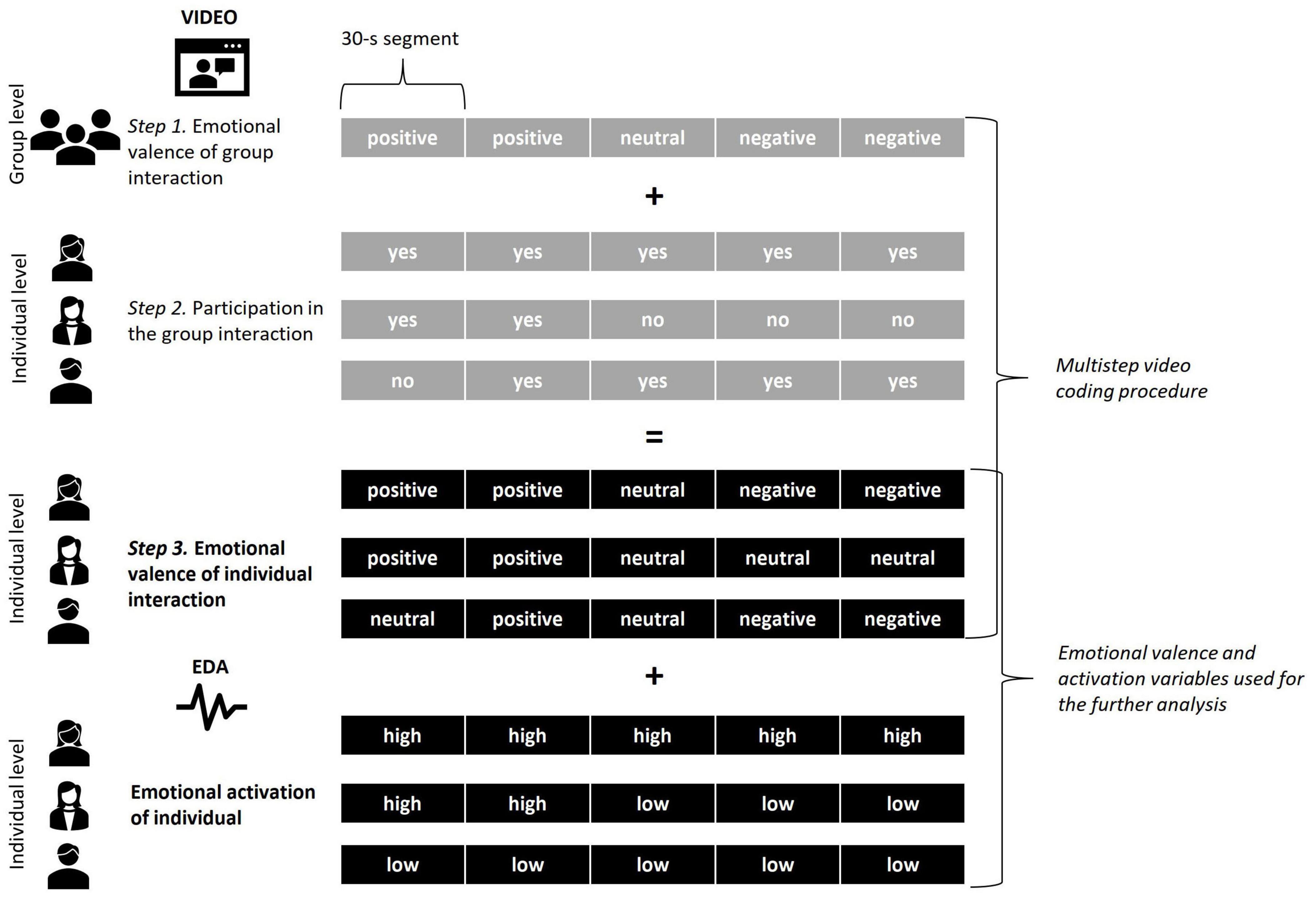
Figure 1. Multistep video-coding procedure starting with the coding of group-level socio-emotional interactions with different emotional valences (steps 1 and 2) followed by identifying each individual group member’s participation in those interactions with different valences (steps 3 and 4) and, finally, the combination of the video coding of students’ participation in socio-emotional interactions with students’ emotional activation as determined from the EDA data.
Students’ participation in socio-emotional interaction with different emotional valences was coded from the groups’ video data. The video-coding procedure started with coding the video data at the group level with a coding scheme based on the authors’ previous work (Törmänen et al., 2022). The video data of each group were processed with Observer XT software (Noldus Information Technology) and divided into 30-s segments. This duration was considered sufficient to properly capture interactions and conclude valid judgments and detailed observations of students’ collaborative behaviors (Porayska-Pomsta et al., 2013). Since the study focuses on collaborative working, the analysis excludes any other segments (e.g., teacher instructions). In total, 5,622 30-s segments (∼47 h of video data) were used for the video analysis.
In the first step, segments including group socio-emotional interaction (i.e., group members’ verbal or behavioral interactions related to group formation and group dynamics, including emotional expressions) were located. In practice, a socio-emotional interaction was coded when at least two group members expressed clear verbal or bodily cues to indicate affect or engaged in an emotionally charged interaction (see Table 1 for examples). Using Cohen’s kappa statistic, interrater reliability was assessed for 10% of the coded videos, and substantial agreement was indicated (κ = 0.77; Landis and Koch, 1977). The two coders discussed any unclear cases to reach a consensus.
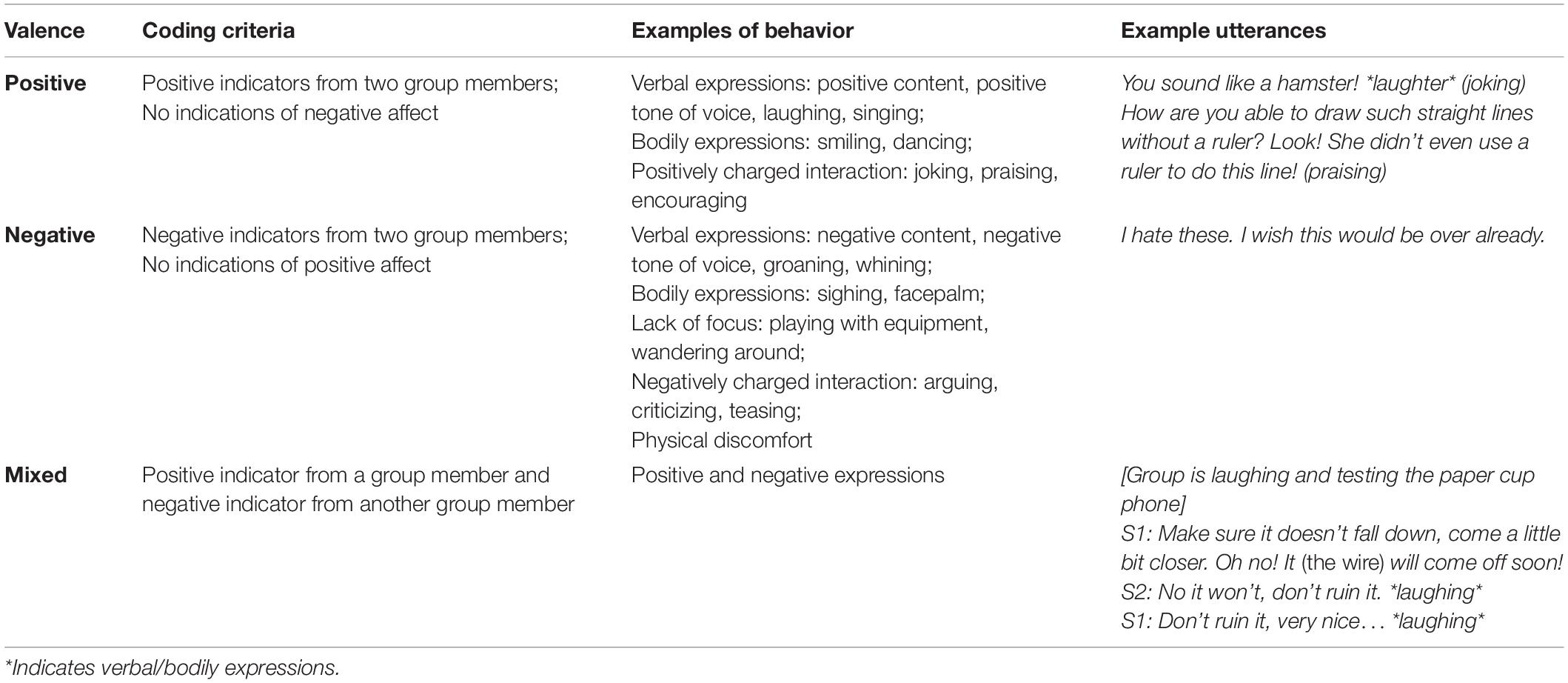
Table 1. Video coding examples of group socio-emotional interactions with different valences (Törmänen et al., 2022).
In the second step, the emotional valence of interaction in each 30-s segment was coded according to four categories (positive, negative, mixed, neutral) based on group members’ emotional expressions. The academic emotions framework (Pekrun et al., 2002) and the affective circumplex model (Russell and Barrett, 1999) provided the theoretical foundation for differentiating between expressions of positive and negative affect. The video coding scheme for emotional valence was based on the authors’ previous work (Törmänen et al., 2021a,b). A socio-emotional interaction was coded as positive when at least two group members expressed clear signs of positive affect or made a positive comment and as negative in the opposite case. To account for possible mixed emotional states at both the individual (Karamarkovich and Rutherford, 2021) and group levels (Törmänen et al., 2021b), in cases where the valence was mixed within one group member (e.g., an individual displayed a negative verbal expression and a positive bodily expression) or two students’ expressions conflicted (i.e., positive vs. negative), the interaction was coded as mixed. If the group did not engage in socio-emotional interaction in the segment (i.e., the interaction included no emotional expression), then the interaction was coded as neutral. An interrater reliability analysis performed on 10% of the coded videos indicated substantial agreement (κ = 0.68; Landis and Koch, 1977). Table 1 presents examples of socio-emotional interaction and valence coding.
In the third step, the analysis shifted from the group level to the individual level, and each student’s participation in socio-emotional interaction in each 30-s segment was coded. Figure 1 illustrates how group-level video codes were translated to the individual level. If a student verbally participated in the interaction that occurred during the segment, they were coded as participating in the interaction.
In the fourth step, the video coding accounted for the valence of participation. For example, if a student verbally participated in the interaction with a positive valence, the valence of the participation was coded as positive. If the student did not participate in the socio-emotional interaction in the segment, the valence of the participation was coded as neutral—regardless of whether the valence of the group interaction in general was positive, negative, or mixed. Participation was also coded as neutral if the student participated in the group’s neutral interactions (i.e., those interactions not coded as socio-emotional). Again, Cohen’s kappa statistic was used to assess the interrater reliability of the coding of students’ participation for 10% of the coded videos, and substantial agreement was indicated (κ = 0.79; Landis and Koch, 1977).
The physiological component of emotions is closely linked to the activity of the autonomic nervous system (Kreibig, 2010). In this study, physiological emotional activation was measured with EDA, which is related to the function of sweat glands and is a sole measure of sympathetic arousal. Thus, it is closely connected to cognitive and emotional processing (Dawson et al., 2007; Braithwaite et al., 2013). EDA is divided into phasic short-term skin conductance response (SCR) and tonic skin conductance level (SCL) (Boucsein, 2012). EDA values can also increase without a specific external stimulus, and those fluctuations are called non-specific (NS)-SCRs (Dawson et al., 2007). In this study, the measurement of students’ emotional activation during each 30-s segment was based on the frequency of their NS-SCR peaks in EDA (Braithwaite et al., 2013). These peaks were selected because they strongly relate to emotional response and are more sensitive to variations in experimental conditions compared to the slowly changing SCL (Dawson et al., 2007; Christopoulos et al., 2016). In situations involving continuous stimuli (e.g., a collaborative learning situation), the frequency of NS-SCR peaks can be interpreted as an indicator of the current arousal state (Braithwaite et al., 2013). In this study, the EDA analysis started with the construction of a MySQL database to organize the data. The data were then down-sampled from 128 to 16 Hz to accelerate the analysis (Kelsey et al., 2018). First, the EDA data were visually inspected and any recordings with missing electrode contact were removed from the data set. Then, a Butterworth low-pass filter (frequency 1, order 5) was applied to remove small movement artifacts from the signal. The classical trough-to-peak method, which defines the SCR amplitude as the difference between the skin conductance value at the peak and at the preceding trough, was employed to identify NS-SCR peaks in each student’s signal (Dawson et al., 2007; Boucsein, 2012). The threshold was set to 0.05 μS, since it is the most used (Braithwaite et al., 2013) and thus, enabled the comparability of the peak detection results. Initially, different peak detection methods in Ledalab (continuous decomposition analysis and discrete decomposition analysis) were tested (Benedek and Kaernbach, 2010a,b). Eventually, the peaks were detected with the traditional trough-to-peak method because, even after several rounds of testing, decomposition-based methods seemed to provide unrealistically high frequency of peaks as a result. Subsequently, the frequency of NS-SCR peaks was calculated for each student for every 30-s segment of collaboration. The average number of peaks during one segment was then calculated individually for each student along with standard deviations. If the student’s number of peaks during the segment exceeded one standard deviation above their average, then the student was considered to be exhibiting high emotional activation. The limit values for high activation varied from five to nine peaks per 30 s. If the number of peaks was lower than the limit value, the activation was considered low.
Finally, group-level regulation of learning was coded from the video with a coding scheme deriving from the authors’ previous work (Törmänen et al., 2022). Regulation was defined as co- and socially shared activities addressing group members’ cognition, motivation, emotions, and behaviors (Hadwin et al., 2018). First, the coding identified regulation of learning in a segment when the group faced a cognitive, motivational, or emotional obstacle in their learning process, and an individual group member engaged in co-regulation, or group members together engaged in shared strategic negotiation (i.e., socially shared regulation) followed by a strategic change in action (for examples, see Table 2). However, when regulation was applied to maintain or strengthen the emotional state or motivation, no obstacle or change in action was needed (e.g., encouragement, social reinforcement; Järvenoja et al., 2019). Interrater reliability was assessed for 10% of the coded videos, and substantial agreement was indicated (κ = 0.79; Landis and Koch, 1977). Then, similarly to the coding of participation in socio-emotional interactions, individual students’ contributions to the regulation of learning were coded in each segment. A student was coded as contributing to the regulation of learning if they verbally contributed to the group’s regulatory interactions. However, if the student did not contribute, or there was no regulation occurring within the group, the student was coded as not contributing to regulation.
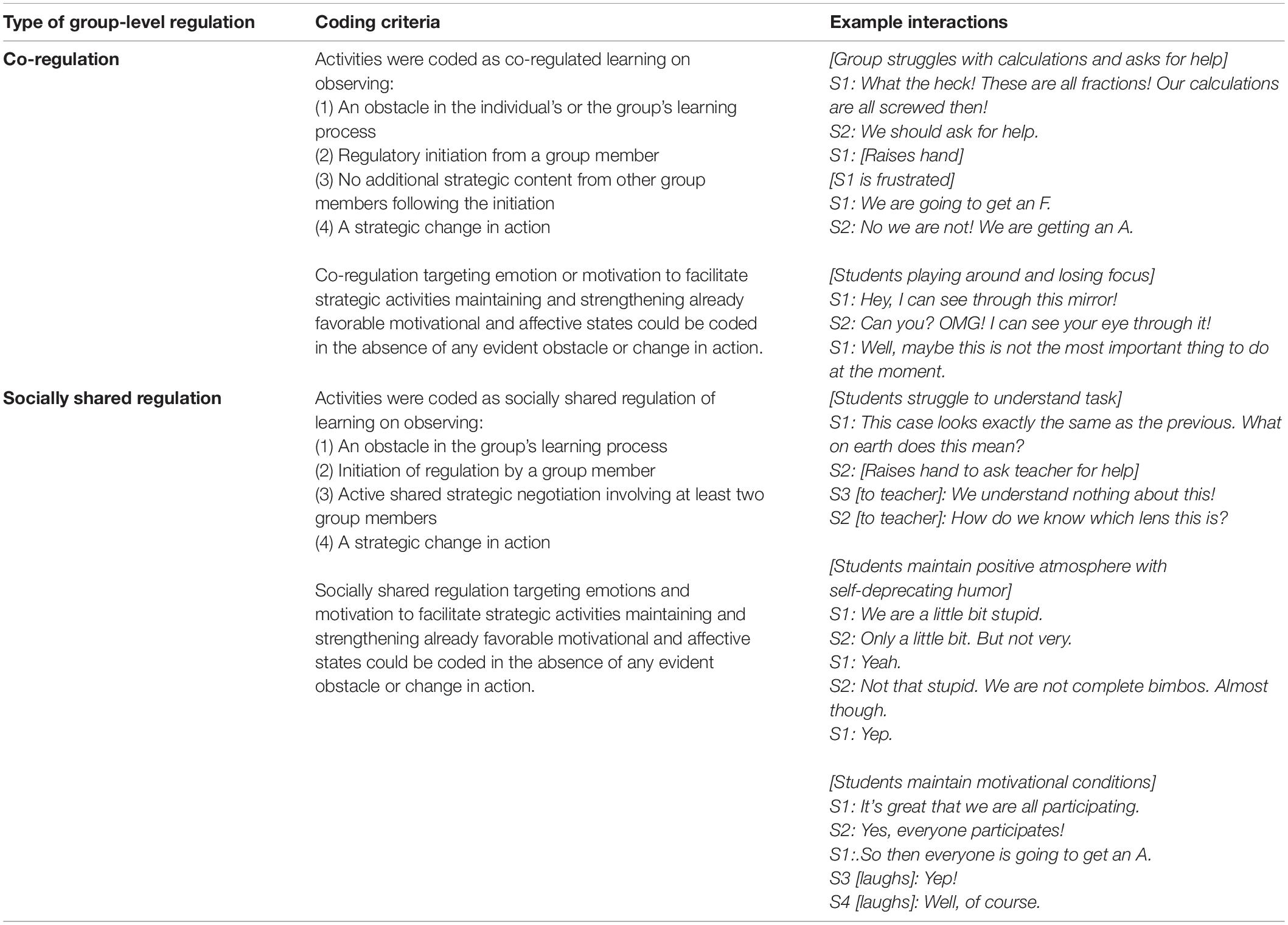
Table 2. Coding criteria and example interactions of co- and socially shared regulation of learning (Törmänen et al., 2022).
To identify students with different socio-emotional interaction profiles, the study applied two levels of a clustering approach following the methods of López-Pernas and Saqr (2021). First, participation in socio-emotional interaction and emotional activation variables were clustered into four types of socio-emotional interaction states. Then, the clusters were used to construct sequences of socio-emotional interaction states. These sequences were again clustered into socio-emotional interaction profiles of individual students by employing mixture hidden Markov models (MHMMs). The two levels of the clustering process are presented in Figure 2, and the full details of the process are described below.
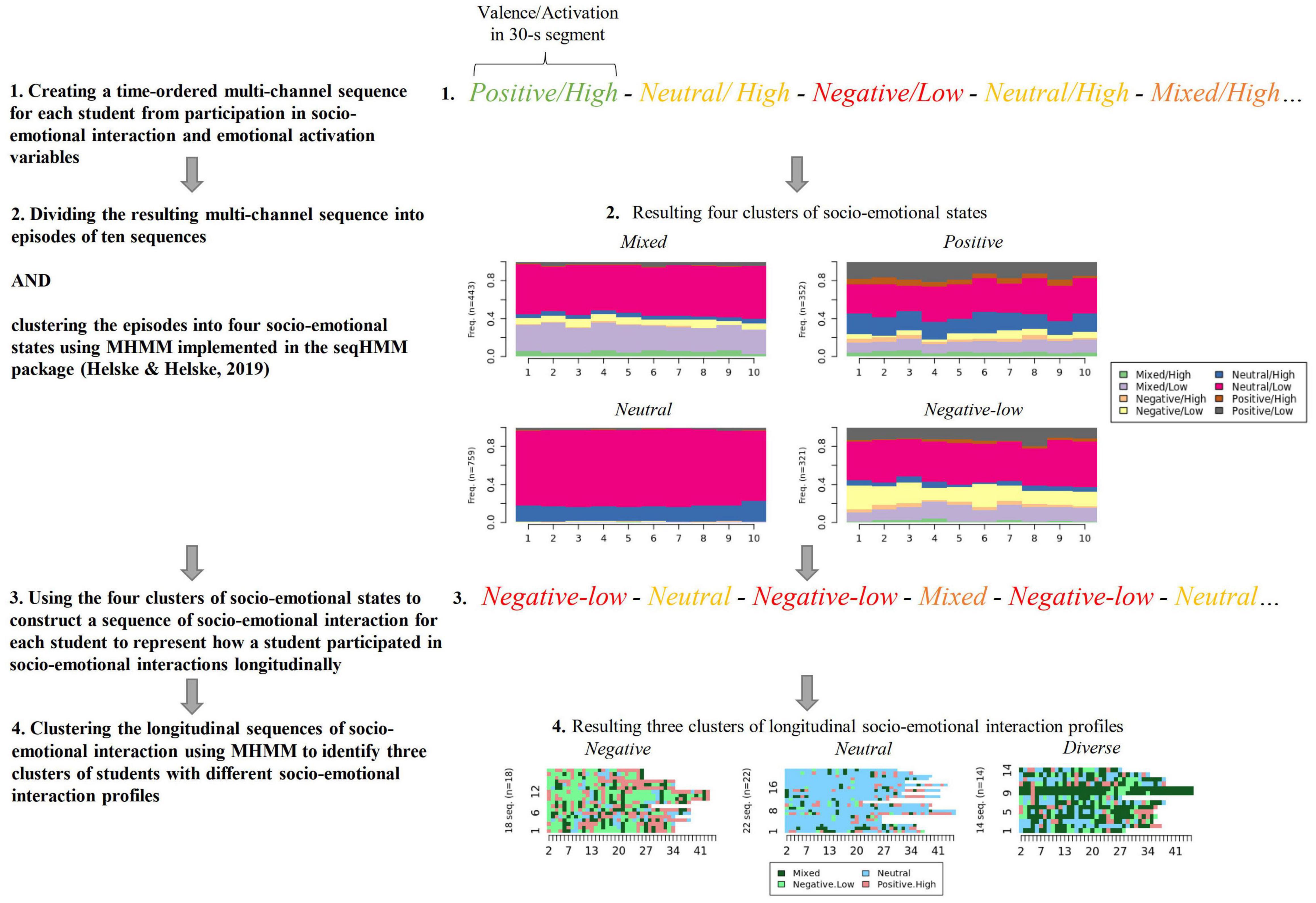
Figure 2. The two levels of the clustering approach (López-Pernas and Saqr, 2021) for identifying socio-emotional interaction profiles.
Participation in socio-emotional interaction and emotional activation variables were used to create time-ordered state sequence objects. Examples of the sequences created for (valence of) participation in socio-emotional interaction and emotional activation are as follows:
Then, the sequences were temporally aligned into a multi-channel sequence combining the two sequences, in which each sequence contained the two states. A multi-channel sequence could appear as follows:
The resulting multi-channel sequence was subsequently divided into equal episodes of 10 sequences. This decision was based on an analysis of the frequent subsequences, which found that 97% of all subsequence patterns were within a range of 10 sequences. The multi-channel object was clustered with an MHMM implemented in the seqHMM package (Helske and Helske, 2019). An MHMM can be perceived as a combination of a hidden Markov model (HMM) and latent class analysis. Because an MHMM extends an HMM by adding an additional layer of time-constant statuses, it is suitable for the clustering of longitudinal processes. Ten clustering models were estimated—each with unique cluster number—and the model with the lowest Bayesian information criterion (BIC) was selected. The model was estimated 1,000 times from random values to reach the global optimum (Helske et al., 2018). The work of Helske et al. (2018) fully details the foundations of the implemented methods, the mathematics, and the technique.
The resulting clusters of episodes of 10 sequences were labeled according to the frequent dominant pattern and the implication statistic. The implication statistic is a statistical means for calculating the dominant pattern of a sequence with a confidence interval. The four resulting clusters represent patterns of students’ socio-emotional interaction participation states (later referred to as socio-emotional states), which were designated as mixed, positive, neutral, and negative-low states. In the mixed state, students mainly participated in socio-emotional interactions with a mixed valence in either a low or high level of emotional activation. The positive state was dominated by participation in positive interactions together with either low or high emotional activation. The neutral state included predominantly neutral participation with low or high activation. Finally, the negative-low state encompassed mostly participation in negative interactions with a low level of activation. The four clusters were used to construct a sequence of socio-emotional interaction states for each student, which represents how that student experienced these socio-emotional states longitudinally across the collaborative learning period. One example of a sequence created for a student is as follows:
The resulting state sequence object, which contains all sequences of all students, was clustered through the same method of an MHMM implemented in the seqHMM package (Helske and Helske, 2019). The lowest BIC indicated three clusters of students’ socio-emotional interaction profiles. The clusters were plotted, labeled, and described.
To study the valence of students’ participation and emotional activation in the different profiles as well as the association between the profiles and students’ contributions to their group’s regulation of learning, Chi-squared tests of independence and multi-way contingency mosaic plots were used. Mosaic plots are suited to high-dimensional categorical data (i.e., when several categories are tested for association). A mosaic plot is similar to a Chi-squared test in that it visualizes where the cells are proportional to the observed vs. expected frequencies. The cells in a mosaic plot are signed as positive or negative concordant with the direction of the association. The height of each cell is proportional to the residual, and the width is proportional to the difference between the observed and expected frequencies (Meyer et al., 2006).
Results
Three Socio-Emotional Interaction Profile Types
The analysis discovered four socio-emotional interaction state types—mixed, positive, neutral, and negative-low—which were subsequently used to construct the longitudinal socio-emotional interaction profiles. Figure 3 demonstrates the characteristics of the states in terms of the valence of participation and emotional activation. The first state, mixed (n = 443), mainly includes the participation of students in socio-emotional interactions with a mixed valence and either a low or high level of emotional activation. The second state, positive (n = 352), is dominated by participation in positive interactions together with either low or high emotional activation. The third state, neutral (n = 759), contains mostly neutral participation with low or high activation. The fourth cluster, negative-low (n = 321), primarily reflects participation in negative interactions with a low level of activation.
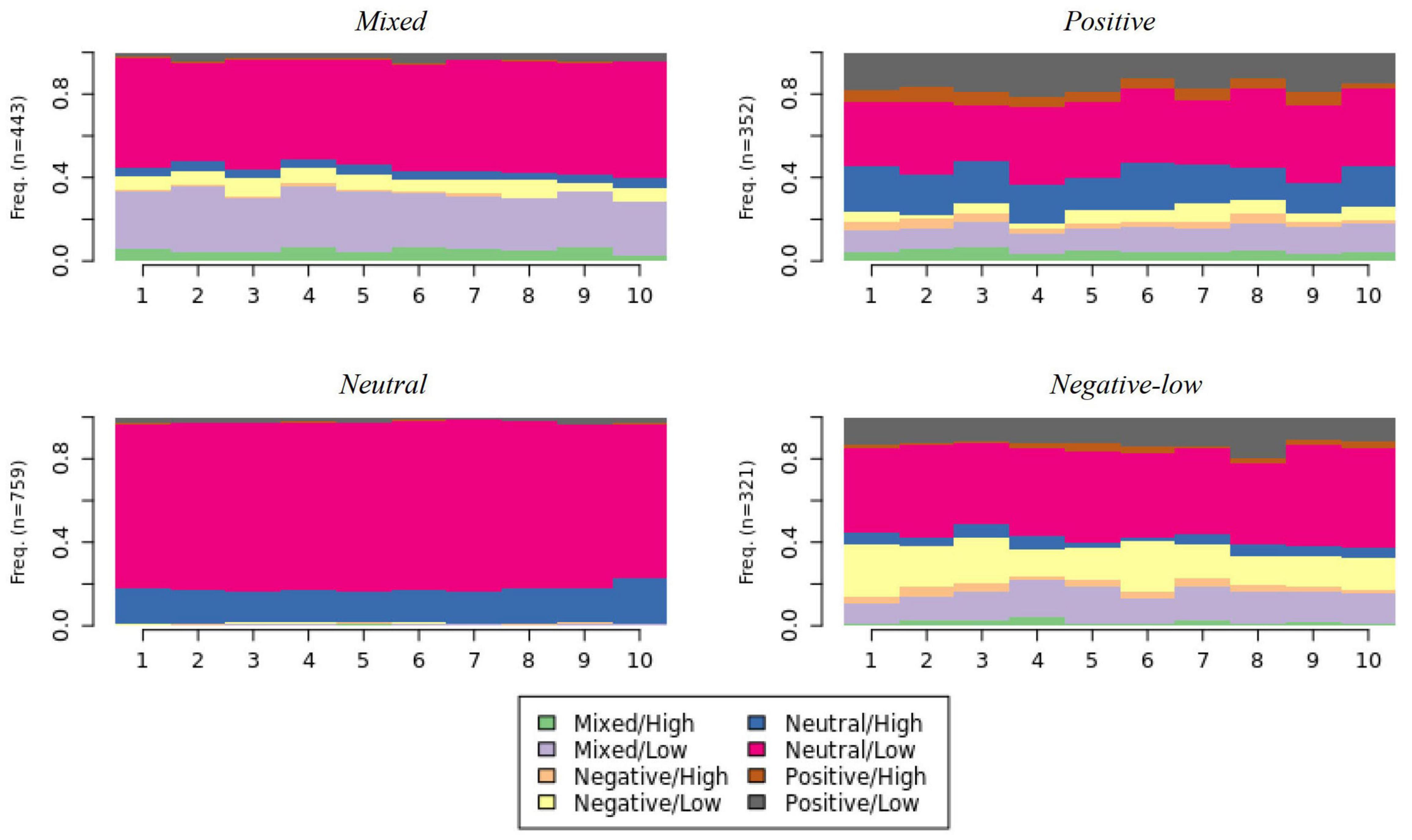
Figure 3. Index plots depicting the valence of participation and emotional activation characteristics for the four clusters of socio-emotional states.
Following the two levels of the clustering approach, the four clusters of socio-emotional states were used to construct a sequence of socio-emotional interactions for each student, which longitudinally represents how that student participated in socio-emotional interactions. These longitudinal socio-emotional interaction sequences were then clustered into three distinct socio-emotional profiles—negative, neutral, or diverse—each of which represents a subgroup of students (Figure 3). A Chi-squared test of independence indicated significant differences in students’ valence of participation [χ2 (6) = 2990.5, V = 0.46, p < 0.001] and emotional activation [(χ2 (2) = 40.0, V = 0.63, p < 0.001] between profiles (Figure 4). The mosaic plots in Figure 5 illustrate these differences.
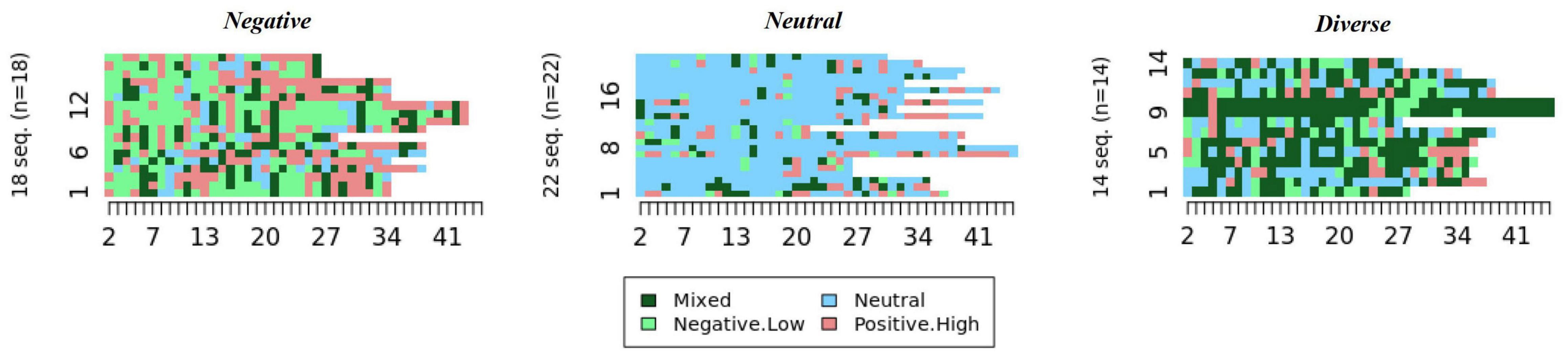
Figure 4. Index plot of the sequences of the three clusters of students with discrete socio-emotional interaction profiles; each colored block represents a socio-emotional state, and each line signifies a distinct student.
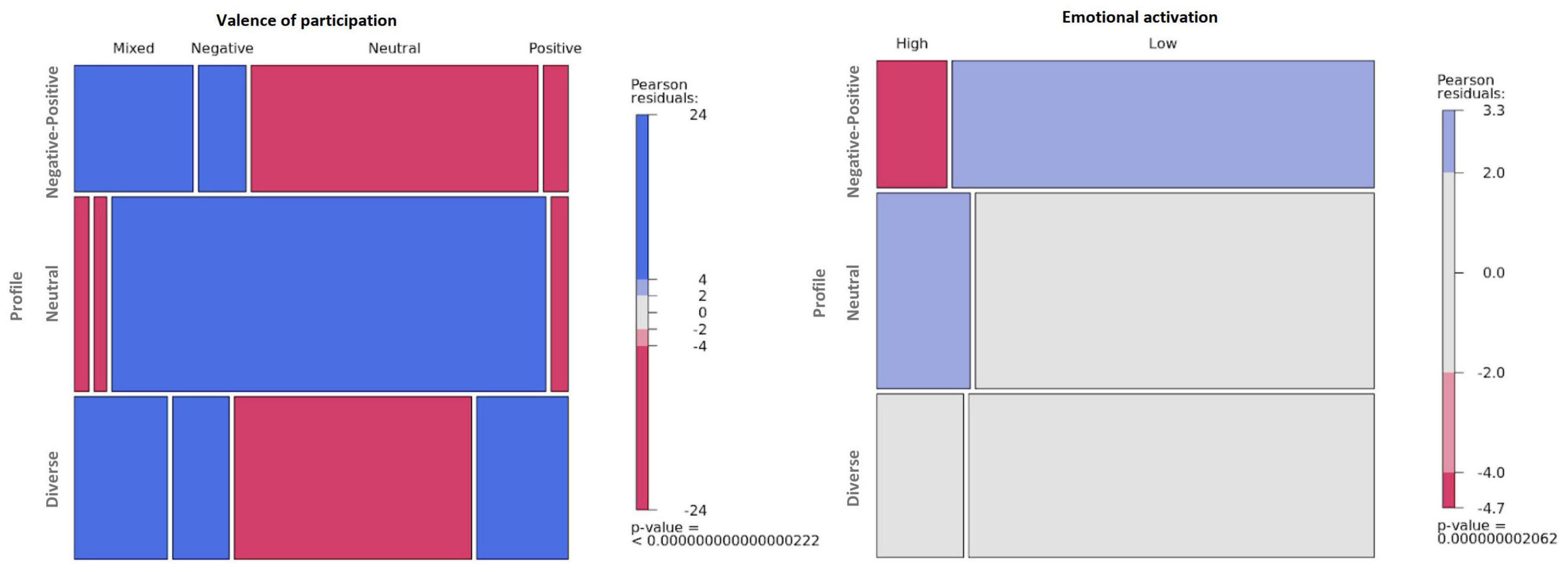
Figure 5. Mosaic plots visualizing the association of the valence of participation and the emotional activation between the three socio-emotional interaction profiles.
The first cluster (n = 18) is considered the negative profile since it includes students who participated more likely in negative and mixed interactions and less likely in neutral and positive interactions. However, by the end of the collaborative learning process, their socio-emotional state often changed to a positive one, which formed a pattern from a negative-low to positive state. In terms of emotional activation, the students in the negative profile were more likely to experience a state of low activation and less likely to be highly activated.
The second cluster (n = 22) was deemed the neutral profile since the students in the cluster were more likely to participate in neutral interactions throughout the collaborative learning process, and their behavior exhibited a pattern of neutral states normally following each other. The mosaic plots in Figure 4 confirm that the neutral profile students participated to a significantly lesser degree in their groups’ socio-emotional interactions (i.e., interactions with a positive, negative, or mixed valence). Thus, these students expressed their emotions in interactions at a lower frequency than that of students in the other two profiles. However, they were more likely to display high emotional activation.
The third cluster (n = 14) was defined as the diverse profile since the students in the cluster participated more likely in mixed, positive, and negative interactions and demonstrated diverse patterns in the various socio-emotional states that followed each other. Therefore, these students presented extensive variation in their socio-emotional interaction state during the collaborative learning process. Furthermore, they were equally likely to display high and low activation.
Associations Between the Socio-Emotional Interaction Profiles and Contribution to Regulation of Learning
A Chi-squared test of independence indicated significant differences in how students with different socio-emotional interaction profiles contributed to the regulation of their groups’ learning [χ2 (2) = 421.9, V = 0.63, p < 0.001]. As evident from the mosaic plot in Figure 6, students with the diverse profile were more likely to contribute to regulation, whereas students with the neutral profile were less likely to contribute. Students with the negative profile were as likely to contribute as they were to not contribute.
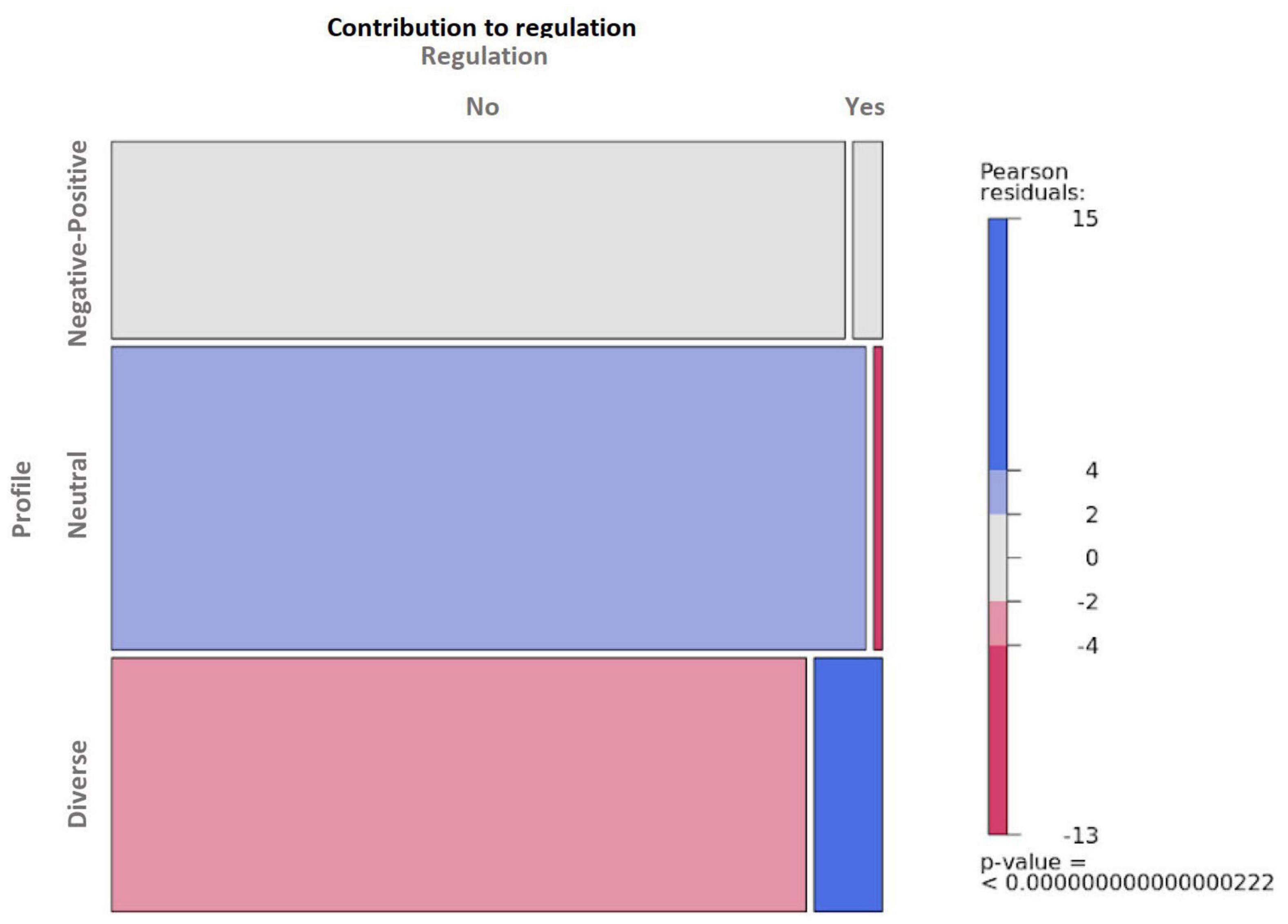
Figure 6. A mosaic plot visualizing the association of the contribution to regulation of learning and the three socio-emotional interaction profiles.
Discussion
This study has applied a person-centered approach to investigate how students participated in their groups’ socio-emotional interactions during a collaborative learning period. Accounting for individual differences and the diversity of socio-emotional interaction profiles was helpful to avoid aggregating distinct groups with contradictory characteristics into the same pool (e.g., individuals with predominately positive interactions and negative interactions), which would have resulted in a neutral average that represents neither the group in general nor any of its components. Therefore, the study has aimed to reveal heterogenous profiles of students and their relation to the regulation of learning. Each identified profile presents a distinct socio-emotional interaction pattern and may thus require, for example, a unique type of support in the learning process.
Through its analysis of the longitudinal profiles of socio-emotional interactions, the research offers results that extend previous findings highlighting differences in students’ emotional profiles in individual learning situations (Ganotice et al., 2016; Robinson et al., 2017; Karamarkovich and Rutherford, 2021) as well as in participation in interactions during a single collaborative learning event (Isohätälä et al., 2017; Törmänen et al., 2021a). Three longitudinal socio-emotional interaction profiles were identified: negative, neutral, and diverse. Students with the same profile demonstrated homogenous patterns in how they participated in socio-emotional interactions in their respective groups (i.e., how they expressed their emotions in collaborative interactions), and these patterns were distinct from those of the other profiles. Further investigation of the profiles evidenced that these socio-emotional interaction patterns were relatively stable throughout the collaborative learning period (e.g., across the lessons and a range of tasks). Students with the neutral profile participated mainly in neutral interactions, whereas students with the diverse profile exhibited considerable variation and participated in socio-emotional interactions with mixed, positive, and negative valences. Notably, students with the negative profile participated mainly in negative interactions, but the valence became positive toward the end of the collaborative learning period. In contrast to the prior research done in the individual learning settings (Robinson et al., 2017; Karamarkovich and Rutherford, 2021), this study did not identify a positive profile. This was surprising and might demonstrate the emotionally challenging nature of collaborative learning (Näykki et al., 2014; Järvenoja et al., 2019), where negative and mixed emotions might be particularly typical for the age group of this study (Riediger and Klipker, 2014; Somerville, 2016). Still, the socio-emotional interaction profiles specified in this study seem to align quite well with the individual emotional profiles outlined in previous studies (Robinson et al., 2017; Karamarkovich and Rutherford, 2021), which reinforces the notion that the emotions students experience in learning are reflected in their socio-emotional interaction behaviors (Bakhtiar et al., 2018; Mänty et al., 2020). However, to confirm this assertion, there is a need for additional research that relates these longitudinal socio-emotional interaction profiles to students’ self-reported emotions.
This study has determined that the socio-emotional interaction profiles were related to students’ contributions to the group regulation of learning. The identification of these profiles via a person-centered method is essential to understand the participation dynamics in collaboration as well as how they relate to or influence the regulation of learning of groups (Isohätälä et al., 2017). Students with the diverse profile were more likely to contribute to the regulation of learning, whereas the neutral profile students were less likely to contribute. The findings imply a reciprocal role of students’ emotions as both conditions and products of learning activities (Winne and Hadwin, 1998; Bakhtiar et al., 2018). Given that the regulation of learning was coded in relation to successfully overcoming obstacles that the group confronted, students with the diverse profile may have encountered recurrent obstacles in their group learning but were able to effectively engage in regulation (Hadwin et al., 2018). The diverse profile students participated in negative and mixed interactions, which may have grounded the obstacles in their learning process (Järvenoja et al., 2019); however, in turn, the obstacles might have provoked the students’ diverse emotional expressions and demand for the regulation of learning (Törmänen et al., 2021b). On the other hand, the diverse students participated more likely in positive interactions compared to the other profiles, which could have facilitated their successful regulatory contributions in the face of challenges (Lajoie et al., 2015; Järvelä et al., 2016).
Overall, based on their emotional reactivity and contribution to regulation, the diverse profile students might have been actively engaged in carrying out the collaborative tasks (Karamarkovich and Rutherford, 2021). However, this case may not be applicable to the negative and neutral profiles. Previous studies have found that students with negative and neutral emotional profiles were disengaged and had low levels of achievement (Robinson et al., 2017; Karamarkovich and Rutherford, 2021). In this study, the students with the negative profile seemed to be especially in need of regulation to restore more positive emotional grounds for learning. Despite this need, their negative and mixed interactions may have hindered their ability to engage in regulation (Bakhtiar et al., 2018). Regarding the neutral profile, those students were likely to display high activation even though they were less likely to express their emotions in group interactions. In addition to emotions, a high number of peaks in EDA—which was perceived as an indicator of emotional activation in this study—has been previously linked to adequate achievement (Pijeira-Díaz et al., 2018; Harley et al., 2019), which might reflect that the neutral profile students performed the tasks well without encountering considerable obstacles that would trigger emotional expressions. Alternatively, such students may be more likely to self-regulate their emotions and control their visible emotional expressions (Gross and Thompson, 2007). Regardless, further studies are needed to confirm the relation between the socio-emotional interaction profiles and the students’ engagement, task progress, and collaborative learning achievements.
This study capitalized on recent advances in sequence mining methods and MHMMs. First, a multi-channel sequence analysis was conducted to temporally align and examine multiple channels of data and how they unfolded together (Helske and Helske, 2019). Such methods fill a gap in multimodal data analysis, which requires the study of multiple data streams while preserving the temporal sequence of the data. Second, the MHMM allowed for the clustering of longitudinal profiles without losing the sequential time resolution of the data (López-Pernas and Saqr, 2021). Despite the many advantages of using multimodal data together with novel sequence mining methods (see Järvelä et al., 2022), the present study also has limitations that warrant discussion. For instance, the video data coding included only the variables of socio-emotional interaction participation and contribution to regulation, which were the specific variables of focus in this study. Research could further explain the differences in student behaviors between profiles by more thoroughly capturing, for example, the students’ progression in the tasks or the obstacles that prompted regulation, although more extensive video analysis would be required. The present study performed four rounds of detailed video coding, which, given the amount of coded video data, was already a labor-intensive endeavor. Thus, the decision was made to not proceed further with the in-depth qualitative video analysis in this study, which leaves the opportunity for future research to explore the important interplay between students’ emotional and cognitive learning processes in more detail. In terms of the EDA data analysis, the traditional trough-to-peak method used for the peak detection does not account for the superimposition of SCRs, and thus, may have caused an underestimation of the SCR amplitude (Benedek and Kaernbach, 2010a). However, to define the students’ emotional activation, instead of amplitude this study used the frequency of NS-SCRs, which is not that much influenced by the superimposition. The students’ emotional activation was divided into high and low states; this structure did not permit medium activation, which could be the optimal arousal for performance (Yerkes and Dodson, 1908), as a separate category. This decision was based on the affective circumplex model (Russell and Barrett, 1999) and the framework of academic emotions (Pekrun, 2006), which traditionally categorize emotions as either activating or de-activating. This study based this categorization on the average and standard deviation of students’ individual EDA peaks, which differentiated moments of high activation from those of low and medium activation. Thus, the low activation category also included moments of medium activation, and the results should be interpreted accordingly.
Conclusion
In conclusion, this study has identified three socio-emotional interaction profiles, each of which represents a distinct socio-emotional interaction pattern with particular characteristics of emotional valence, activation, and contributions to the regulation of learning. The results highlight a need for additional studies employing person-centered methods to identify these student subgroups and more clearly understand their individual needs regarding, for instance, regulation support. In this study, the negative profile students may have benefited from support targeting emotion regulation to secure more positive socio-emotional grounds for collaborative learning and, consequently, to support their shared cognitive learning processes (Barron, 2003; Isohätälä et al., 2018; Järvenoja et al., 2020a). Recent developments in multi-channel sequence analysis allow for the examination of factors that determine the category that describes a student. More importantly, novel methods can facilitate the prediction of future trajectories or longitudinal pathways of students (Helske and Helske, 2019). Such predictions could not only inform support in real time but also anticipate future actions and, hence, guide proper, proactive interventions.
Data Availability Statement
The datasets presented in this article are not readily available because the dataset includes personalized data from minor participants. The research permission approved by the ethics committee delimits the use of data to the research group members. The metadata for this study can be found in the Etsin, Finnish Fairdata services: https://etsin.fairdata.fi/dataset/2dc7f39a-c849-41a2-8094-59247161b767. Requests to access the datasets should be directed to SJ, c2FubmEuamFydmVsYUBvdWx1LmZp.
Ethics Statement
The studies involving human participants were reviewed and approved by the Ethics Committee of Human Sciences, University of Oulu, Finland. Written informed consent to participate in this study was provided by the participants’ legal guardian/next of kin.
Author Contributions
TT was primarily responsible for writing and editing the manuscript and contributed to data collection and video and EDA data analysis. MS was responsible for running the two levels of the clustering analysis. HJ, JM, and SJ were responsible for the research project, scientific design principles of the data collection and collaborative learning design and hold the main funding of the research. All authors contributed to writing and editing of the manuscript.
Funding
This research was funded by the Academy of Finland (grant nos. 297686, 259214, and 308809, 324381) and by the Finnish Cultural Foundation. It is connected to a strategic profiling funding in human sciences [Academy of Finland (grant no. 318930), https://www.oulu.fi/university/genz-profiling-project].
Conflict of Interest
The authors declare that the research was conducted in the absence of any commercial or financial relationships that could be construed as a potential conflict of interest.
Publisher’s Note
All claims expressed in this article are solely those of the authors and do not necessarily represent those of their affiliated organizations, or those of the publisher, the editors and the reviewers. Any product that may be evaluated in this article, or claim that may be made by its manufacturer, is not guaranteed or endorsed by the publisher.
Acknowledgments
Data collection was carried out with the support of LeaF Research Infrastructure (https://www.oulu.fi/leaf-eng/), University of Oulu, Finland.
References
Bakhtiar, A., Webster, E. A., and Hadwin, A. F. (2018). Regulation and socio-emotional interactions in a positive and a negative group climate. Metacogn. Learn. 13, 57–90. doi: 10.1007/s11409-017-9178-x
Bannert, M., Reimann, P., and Sonnenberg, C. (2014). Process mining techniques for analysing patterns and strategies in students’ self-regulated learning. Metacogn. Learn. 9, 161–185. doi: 10.1007/s11409-013-9107-6
Barron, B. (2003). When Smart Groups Fail. J. Learn. Sci. 12, 307–359. doi: 10.1207/S15327809JLS1203
Benedek, M., and Kaernbach, C. (2010a). A continuous measure of phasic electrodermal activity. J. Neurosci. Methods 190, 80–91. doi: 10.1016/j.jneumeth.2010.04.028
Benedek, M., and Kaernbach, C. (2010b). Decomposition of skin conductance data by means of nonnegative deconvolution. Psychophysiology 47, 647–658. doi: 10.1111/j.1469-8986.2009.00972.x
Ben-Eliyahu, A., and Linnenbrink-Garcia, L. (2013). Extending self-regulated learning to include self-regulated emotion strategies. Motiv. Emot. 37, 558–573. doi: 10.1007/s11031-012-9332-3
Boucsein, W. (2012). Electrodermal Activity, 2nd Edn. New York, NY: Springer, doi: 10.1007/978-1-4614-1126-0
Braithwaite, J., Watson, D., Robert, J., and Mickey, R. (2013). A Guide for Analysing Electrodermal Activity (EDA) & Skin Conductance Responses (SCRs) for Psychological Experiments. Psychophysiology 49, 1017–1034.
Christopoulos, G. I., Uy, M. A., and Yap, W. J. (2016). The Body and the Brain: measuring Skin Conductance Responses to Understand the Emotional Experience. Organ. Res. Methods 16, 1–27. doi: 10.1177/1094428116681073
Cornejo, C., Cuadros, Z., Morales, R., and Paredes, J. (2017). Interpersonal coordination: methods, achievements, and challenges. Front. Psychol. 8:1685. doi: 10.3389/fpsyg.2017.01685
Dawson, M. E., Schell, A. M., and Filion, D. M. (2007). “The Electrodermal System,” in Handbook of Psychophysiology, eds J. Cacioppo, L. G. Tassinary, and G. G. Berntson (Cambridge: Cambridge University Press), 159–181.
Ganotice, F. A., Datu, J. A. D., and King, R. B. (2016). Which emotional profiles exhibit the best learning outcomes? A person-centered analysis of students’ academic emotions. Sch. Psychol. Int. 37, 498–518. doi: 10.1177/0143034316660147
Gross, J. J., and John, O. P. (2003). Individual differences in two emotion regulation processes: implications for affect, relationships, and well-being. J. Pers. Soc. Psychol. 85, 348–362. doi: 10.1037/0022-3514.85.2.348
Gross, J. J., and Thompson, R. A. (2007). “Emotion regulation. Conceptual foundations,” in Handbook of Emotion Regulation, ed. J. J. Gross (New York, NY: The Guilford Press), 3–26.
Hadwin, A. F., Järvelä, S., and Miller, M. (2018). “Self-regulation, co-regulation and shared regulation in collaborative learning environments,” in Handbook of Self-Regulation of Learning and Performance, eds D. Schunk and J. Greene (New York, NY: Routledge), 83–106.
Harley, J. M., Bouchet, F., Hussain, M. S., Azevedo, R., and Calvo, R. (2015). A multi-componential analysis of emotions during complex learning with an intelligent multi-agent system. Comput. Hum. Behav. 48, 615–625. doi: 10.1016/j.chb.2015.02.013
Harley, J. M., Jarrell, A., and Lajoie, S. P. (2019). Emotion regulation tendencies, achievement emotions, and physiological arousal in a medical diagnostic reasoning simulation. Instr. Sci. 47, 151–180. doi: 10.1007/s11251-018-09480-z
Helske, S., and Helske, J. (2019). Mixture hidden Markov models for sequence data: the seqhmm package in R. J. Stat. Softw. 88, 1–32. doi: 10.18637/jss.v088.i03
Helske, S., Helske, J., and Eerola, M. (2018). “Combining Sequence Analysis and Hidden Markov Models in the Analysis of Complex Life Sequence Data,” in Sequence Analysis and Related Approaches. Life Course Research and Social Policies, eds G. Ritschard and M. Studer (Cham: Springer), 185–200. doi: 10.1007/978-3-319-95420-2_11
Hickendorff, M., Edelsbrunner, P. A., McMullen, J., Schneider, M., and Trezise, K. (2018). Informative tools for characterizing individual differences in learning: latent class, latent profile, and latent transition analysis. Learn. Individ. Differ. 66, 4–15. doi: 10.1016/j.lindif.2017.11.001
Isohätälä, J., Järvenoja, H., and Järvelä, S. (2017). Socially shared regulation of learning and participation in social interaction in collaborative learning. Int. J. Educ. Res. 81, 11–24. doi: 10.1016/j.ijer.2016.10.006
Isohätälä, J., Näykki, P., Järvelä, S., and Baker, M. J. (2018). Striking a balance: socio-emotional processes during argumentation in collaborative learning interaction. Learn. Cult. Soc. Interact. 16, 1–19. doi: 10.1016/j.lcsi.2017.09.003
Järvelä, S., Dindar, M., Sobocinski, M., and Nguyen, A. (2022). “Multimodal research for studying collaborative learning in higher education,” in Handbook for Digital Higher Education, eds R. Sharpe, S. Bennett, and T. Varga-Atkins (Cheltenham: Elgar).
Järvelä, S., Järvenoja, H., and Malmberg, J. (2021). Data from: CLEVER - Making Complex Learning Processes Visible for Enabling Regulation: Change human behavior for learning success (Version 1). Finland: University of Oulu, doi: 10.23729/fa61790c-1929-4408-9869-47d236255880
Järvelä, S., Järvenoja, H., Malmberg, J., Isohätälä, J., and Sobocinski, M. (2016). How do types of interaction and phases of self-regulated learning set a stage for collaborative engagement? Learn. Instr. 43, 39–51. doi: 10.1016/j.learninstruc.2016.01.005
Järvenoja, H., and Järvelä, S. (2013). “Regulating emotions together for motivated collaboration,” in Affective Learning Together. Social and emotional dimensions of collaborative learning, eds M. Baker, J. Andriessen, and S. Järvelä (New York, NY: Routledge), 162–181. doi: 10.4324/9780203069684
Järvenoja, H., Malmberg, J., Törmänen, T., Mänty, K., Haataja, E., Ahola, S., et al. (2020b). A collaborative learning design for promoting and analyzing adaptive motivation and emotion regulation in the science classroom. Front. Educ. 5:111. doi: 10.3389/FEDUC.2020.00111
Järvenoja, H., Järvelä, S., and Malmberg, J. (2020a). Supporting groups’ emotion and motivation regulation during collaborative learning. Learn. Instr. 70:101090. doi: 10.1016/j.learninstruc.2017.11.004
Järvenoja, H., Järvelä, S., Törmänen, T., Näykki, P., Malmberg, J., Kurki, K., et al. (2018). Capturing motivation and emotion regulation during a learning process. Front. Learn. Res. 6, 85–104. doi: 10.14786/flr.v6i3.369
Järvenoja, H., Näykki, P., and Törmänen, T. (2019). Emotional regulation in collaborative learning : when do higher education students activate group level regulation in the face of challenges? Stud. High. Educ. 44, 1747–1757. doi: 10.1080/03075079.2019.1665318
Karamarkovich, S. M., and Rutherford, T. (2021). Mixed feelings: profiles of emotions among elementary mathematics students and how they function within a control-value framework. Contemp. Educ. Psychol. 66:101996. doi: 10.1016/j.cedpsych.2021.101996
Kelsey, M., Akcakaya, M., Kleckner, I. R., Palumbo, R. V., Barrett, L. F., Quigley, K. S., et al. (2018). Applications of sparse recovery and dictionary learning to enhance analysis of ambulatory electrodermal activity data. Biomed. Signal Process. Control 40, 58–70. doi: 10.1016/j.bspc.2017.08.024
Kreibig, S. D. (2010). Autonomic nervous system activity in emotion: a review. Biol. Psychol. 84, 394–421. doi: 10.1016/j.biopsycho.2010.03.010
Kreijns, K., Kirschner, P. A., and Jochems, W. (2003). Identifying the pitfalls for social interaction in computer-supported collaborative learning environments: a review of the research. Comput. Hum. Behav. 19, 335–353. doi: 10.1016/S0747-5632(02)00057-2
Lajoie, S. P., Lee, L., Poitras, E., Bassiri, M., Kazemitabar, M., Cruz-panesso, I., et al. (2015). The role of regulation in medical student learning in small groups : regulating oneself and others’ learning and emotions. Comput. Hum. Behav. 52, 601–616. doi: 10.1016/j.chb.2014.11.073
Landis, J. R., and Koch, G. G. (1977). The Measurement of Observer Agreement for Categorical Data. Biometrics 33:159. doi: 10.2307/2529310
Li, S., Chen, G., Xing, W., Zheng, J., and Xie, C. (2020). Longitudinal clustering of students’ self-regulated learning behaviors in engineering design. Comput. Educ. 153:103899. doi: 10.1016/j.compedu.2020.103899
Linnenbrink-Garcia, L., Rogat, T. K., and Koskey, K. L. K. (2011). Affect and engagement during small group instruction. Contemp. Educ. Psychol. 36, 13–24. doi: 10.1016/j.cedpsych.2010.09.001
Lobczowski, N. G. (2020). Bridging gaps and moving forward : building a new model for socioemotional formation and regulation formation and regulation. Educ. Psychol. 55, 1–16. doi: 10.1080/00461520.2019.1670064
López-Pernas, S., and Saqr, M. (2021). Bringing synchrony and clarity to complex multi-channel data: a learning analytics study in programming education. IEEE Access 9, 1–1. doi: 10.1109/access.2021.3134844
Malmberg, J., Fincham, O., Pijeira-Díaz, H. J., Järvelä, S., and Gaševiæ, D. (2021). Revealing the hidden structure of physiological states during metacognitive monitoring in collaborative learning. J. Comput. Assist. Learn. 37, 861–874.
Malmberg, J., Järvelä, S., Holappa, J., Haataja, E., Huang, X., and Siipo, A. (2019). Going beyond what is visible: what multichannel data can reveal about interaction in the context of collaborative learning? Comput. Hum. Behav. 96, 235–245. doi: 10.1016/j.chb.2018.06.030
Mänty, K., Järvenoja, H., and Törmänen, T. (2020). Socio-emotional interaction in collaborative learning : combining individual emotional experiences and group-level emotion regulation. Int. J. Educ. Res. 102:101589. doi: 10.1016/j.ijer.2020.101589
Mänty, K., Järvenoja, H., and Törmänen, T. (2022). The Sequential Composition of Collaborative Groups’ Emotion Regulation in Negative Socio-emotional Interactions. Eur. J. Psychol. Educ. 2022. doi: 10.1007/s10212-021-00589-3
Meyer, D., Zeileis, A., and Hornik, K. (2006). The strucplot framework: visualizing multi-way contingency tables with vcd. J. Stat. Softw. 17, 1–48. doi: 10.18637/jss.v017.i03
Molenaar, I., and Järvelä, S. (2014). Sequential and temporal characteristics of self and socially regulated learning. Metacogn. Learn. 9, 75–85. doi: 10.1007/s11409-014-9114-2
Mønster, D., Håkonsson, D. D., Eskildsen, J. K., and Wallot, S. (2016). Physiological evidence of interpersonal dynamics in a cooperative production task. Physiol. Behav. 156, 24–34. doi: 10.1016/j.physbeh.2016.01.004
Näykki, P. Järvelä, S., Kirschner, P. A.Järvenoja, H. (2014). Socio-emotional conflict in collaborative learning-A process-oriented case study in a higher education context. Int. J. Educ. Res. 68, 1–14. doi: 10.1016/j.ijer.2014.07.001
Noroozi, O., Pijeira-Díaz, H. J., Sobocinski, M., Dindar, M., Järvelä, S., and Kirschner, P. A. (2020). Multimodal data indicators for capturing cognitive, motivational, and emotional learning processes: a systematic literature review. Educ. Inf. Technol. 25, 5499–5547. doi: 10.1007/s10639-020-10229-w
Palumbo, R. V., Marraccini, M. E., Weyandt, L. L., Wilder-Smith, O., McGee, H. A., Liu, S., et al. (2017). Interpersonal Autonomic Physiology: a Systematic Review of the Literature. Personal. Soc. Psychol. Rev. 21, 99–141. doi: 10.1177/1088868316628405
Pekrun, R. (2006). The control-value theory of achievement emotions: assumptions, corollaries, and implications for educational research and practice. Educ. Psychol. Rev. 18, 315–341. doi: 10.1007/s10648-006-9029-9
Pekrun, R., Goetz, T., Titz, W., and Perry, R. P. (2002). Academic Emotions in Students’ Self-Regulated Learning and Achievement: a Program of Qualitative and Quantitative Research. Educ. Psychol. 37, 91–106. doi: 10.1207/S15326985EP3702
Pijeira-Díaz, H. J., Drachsler, H., Kirschner, P. A., and Järvelä, S. (2018). Profiling sympathetic arousal in a physics course: how active are students? J. Comput. Assist. Learn. 34, 397–408. doi: 10.1111/jcal.12271
Porayska-Pomsta, K., Mavrikis, M., and Mello, S. D. (2013). Knowledge Elicitation Methods for Affect Modelling in Education. Int. J. Artif. Intell. Educ. 22, 107–140.
Quirk, M., Nylund-Gibson, K., and Furlong, M. (2013). Exploring patterns of Latino/a children’s school readiness at kindergarten entry and their relations with Grade 2 achievement. Early Child. Res. Q. 28, 437–449. doi: 10.1016/j.ecresq.2012.11.002
Reimann, P. (2009). Time is precious: variable- and event-centred approaches to process analysis in CSCL research. Int. J. Comput. Collab. Learn. 4, 239–257. doi: 10.1007/s11412-009-9070-z
Riediger, M., and Klipker, K. (2014). “Emotion Regulation in Adolescence,” in Handbook of Emotion Regulation, ed. J. J. Gross (New York: Guilford Press), 187–202.
Robinson, K. A., Ranellucci, J., Lee, Y., Wormington, S. V., Roseth, C. J., and Linnenbrink-Garcia, L. (2017). Affective profiles and academic success in a college science course. Contemp. Educ. Psychol. 51, 209–221. doi: 10.1016/j.cedpsych.2017.08.004
Rogat, T. K., and Adams-Wiggins, K. R. (2015). Interrelation between regulatory and socioemotional processes within collaborative groups characterized by facilitative and directive other-regulation. Comput. Hum. Behav. 52, 589–600. doi: 10.1016/j.chb.2015.01.026
Rogat, T. K., and Linnenbrink-Garcia, L. (2011). Socially Shared Regulation in Collaborative Groups: an Analysis of the Interplay Between Quality of Social Regulation and Group Processes. Cogn. Instr. 29, 375–415. doi: 10.1080/07370008.2011.607930
Rosato, N. S., and Baer, J. C. (2012). Latent class analysis: a method for capturing heterogeneity. Soc. Work Res. 36, 61–69. doi: 10.1093/swr/svs006
Roschelle, J., and Teasley, S. D. (1995). “The construction of shared knowledge in collaborative problem solving,” in Computer Supported Collaborative Learning, ed. C. O’Malley (Berlin: Springer), 69–97.
Roscoe, R. D., and Chi, M. T. H. (2008). Tutor learning: the role of explaining and responding to questions. Instr. Sci. 36, 321–350. doi: 10.1007/s11251-007-9034-5
Russell, J. A., and Barrett, L. F. (1999). Core affect, prototypical emotional episodes, and other things called emotion: dissecting the elephant. J. Pers. Soc. Psychol. 76, 805–819. doi: 10.1037/0022-3514.76.5.805
Saqr, M., and López-Pernas, S. (2021a). “Idiographic Learning Analytics: A single student (N=1) approach using psychological networks,” in Companion Proceedings of the 11th International Conference on Learning Analytics & Knowledge [Irvine, CA: Society for Learning Analytics Research (SOLAR)], 456–463. Available online at: https://www.solaresearch.org/wp-content/uploads/2021/04/LAK21_CompanionProceedings.pdf
Saqr, M., and López-Pernas, S. (2021b). The longitudinal trajectories of online engagement over a full program. Comput. Educ. 175:104325. doi: 10.1016/j.compedu.2021.104325
Sinha, S., Rogat, T. K., Adams-Wiggins, K. R., and Hmelo-Silver, C. E. (2015). Collaborative group engagement in a computer-supported inquiry learning environment. Int. J. Comput. Collab. Learn. 10, 273–307. doi: 10.1007/s11412-015-9218-y
Slovák, P., Tennent, P., Reeves, S., and Fitzpatrick, G. (2014). Exploring skin conductance synchronisation in everyday interactions. Proc. 8th Nord. Conf. Hum. Comput. Interact. Fun Fast Found. Nord. 14, 511–520. doi: 10.1145/2639189.2639206
Somerville, L. H. (2016). “Emotional development in adolescence,” in Handbook of Emotions, eds L. F. Barrett, M. Lewis, and J. M. Haviland-Jones (New York: The Guilford Press), 350–368.
Törmänen, T., Järvenoja, H., and Mänty, K. (2021a). All for one and one for all – How are students’ affective states and group-level emotion regulation interconnected in collaborative learning? Int. J. Educ. Res. 109:101861. doi: 10.1016/j.ijer.2021.101861
Törmänen, T., Järvenoja, H., and Mänty, K. (2021b). Exploring groups’ affective states during collaborative learning: what triggers activating affect on a group level? Educ. Technol. Res. Dev. 69, 2523–2545. doi: 10.1007/s11423-021-10037-0
Törmänen, T., Järvenoja, H., Saqr, M., Malmberg, J., and Järvelä, S. (2022). Affective States and Regulation of Learning During Socio-emotional Interactions in Secondary School Collaborative Groups. Manuscript submitted for publication.
Winne, P. H. (2017). Leveraging Big Data to Help Each Learner and Accelerate Learning Science. Teach. Coll. Rec. 119, 1–24.
Winne, P. H., and Hadwin, A. F. (1998). “Studying as Self-Regulated Learning,” in Metacognition in Educational Theory and Practice, eds D. J. Hacker, J. Dunlosky, and A. Graesser (Hillsdale, NJ: Lawrence Erlbaum), 277–304. doi: 10.1016/j.chb.2007.09.009
Xie, K., Vongkulluksn, V. W., Lu, L., and Cheng, S. L. (2020). A person-centered approach to examining high-school students’ motivation, engagement and academic performance. Contemp. Educ. Psychol. 62:101877. doi: 10.1016/j.cedpsych.2020.101877
Yerkes, R. M., and Dodson, J. D. (1908). The Relation of Strength of Stimulus to Rapidity of Habit-Formation. J. Comp. Neurol. Psychol. 18, 459–482. doi: 10.1037/h0073415
Keywords: emotions, socio-emotional interaction, self-regulated learning, collaborative learning, person-centered approach, multimodal data
Citation: Törmänen T, Järvenoja H, Saqr M, Malmberg J and Järvelä S (2022) A Person-Centered Approach to Study Students’ Socio-Emotional Interaction Profiles and Regulation of Collaborative Learning. Front. Educ. 7:866612. doi: 10.3389/feduc.2022.866612
Received: 31 January 2022; Accepted: 24 May 2022;
Published: 13 June 2022.
Edited by:
Hugo F. Posada-Quintero, University of Connecticut, United StatesReviewed by:
Anna Van Der Want, Marnix Academie, NetherlandsJimmy Zambrano R., University of The Pacific, Ecuador
Tarique Hasan Khan, University of Connecticut, United States
Copyright © 2022 Törmänen, Järvenoja, Saqr, Malmberg and Järvelä. This is an open-access article distributed under the terms of the Creative Commons Attribution License (CC BY). The use, distribution or reproduction in other forums is permitted, provided the original author(s) and the copyright owner(s) are credited and that the original publication in this journal is cited, in accordance with accepted academic practice. No use, distribution or reproduction is permitted which does not comply with these terms.
*Correspondence: Tiina Törmänen, dGlpbmEudG9ybWFuZW5Ab3VsdS5maQ==