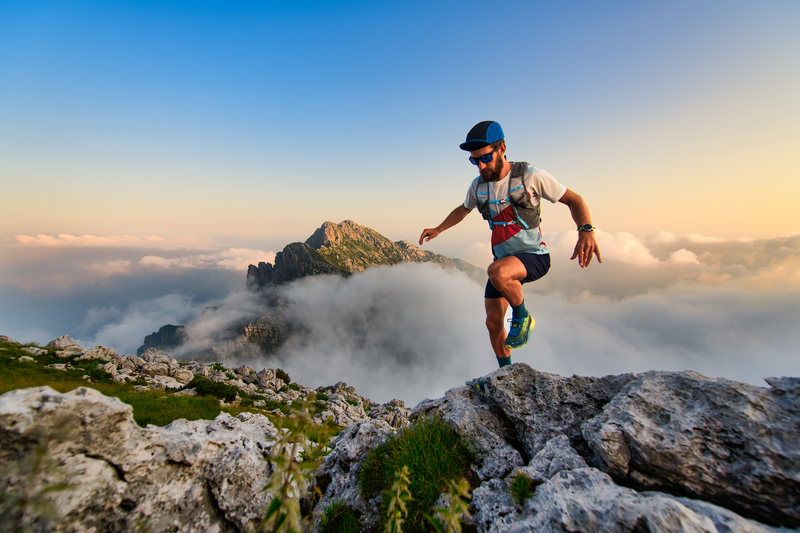
94% of researchers rate our articles as excellent or good
Learn more about the work of our research integrity team to safeguard the quality of each article we publish.
Find out more
ORIGINAL RESEARCH article
Front. Educ. , 07 April 2022
Sec. Assessment, Testing and Applied Measurement
Volume 7 - 2022 | https://doi.org/10.3389/feduc.2022.857451
Prior research has demonstrated links between academic achievement and family socioeconomic status SES, such that students from wealthier families generally exhibit higher academic performance. Recent work has also shown that students who attend schools with greater SES inequality and lower average family income also tend to have lower achievement test scores. Additional research has found that students from poorer nations have lower average achievement test scores. Despite the presence of this evidence supporting individual relationships between SES and achievement test performance, there is less extant information positing a mechanism for how these relationships at different levels interact with one another and are related to achievement test performance. The purpose of the current study was to propose and test two multilevel mediation models of the relationships between national funding for public services such as education, and economic inequality with shortages for educational resources and trained staff, as well as aggregated and individual family SES with academic achievement. Results demonstrated that a partially mediated model yielded good fit to the data with both direct and indirect relationships among national funding for public services and academic achievement, as mediated through school level resource shortages and family SES. Implications of these results are discussed.
Educational researchers have engaged in multiple investigations into social, scholastic, and familial antecedents to academic performance. This work has established clear relationships between scores on academic achievement tests and various demographic variables associated with individual students such as family socioeconomic status (SES) and parental level of education (Duncan and Brooks-Gunn, 2000; Sirin, 2005; Mayer, 2010; Tsai et al., 2017; Lee et al., 2019). In addition, investigators have found that school level effects are also associated with the academic performance of the children attending the schools, including the collective SES of students at the school (Rumberger and Palardy, 2005), as well as school level inequality with respect to family income and educational opportunities (Armor et al., 2018; Parker et al., 2018; Gubbels et al., 2020). Various hypotheses have been suggested to explain the link between SES and academic achievement, including potential relationships between family SES and factors such as environmental stimuli, school quality, nutrition, and stress levels (Muijs et al., 2004; Coulton et al., 2007). Similarly, educational researchers have posited that schools with relatively large collections of low SES students may themselves lack for resources, which is itself associated with lower educational performance (van Ewijk and Sleegers, 2010; Sullivan et al., 2013; Armor et al., 2018). Finally, there has been research suggesting that national educational policies regarding resource allocation, inequality, and school funding is also associated with academic performance (Carnoy and Marshall, 2005; Parker et al., 2018; Xie and Ma, 2019). The purpose of the current study was to link these various research strands by investigating models relating individual family SES, school level SES equity and resource availability, national level inequality and resource allocation to social services, and student level academic performance.
In the education research literature, family SES has typically been expressed in terms of the level of parent education, parental income, eligibility for free or reduced lunch, or household possessions and resources (Sirin, 2005; Eshetu, 2015). It is recognized as one of the most robust and consistent predictors of academic achievement that has been examined (Heppt et al., 2015; White et al., 2016). Although the relationship between family SES and student achievement sometimes varies depending on factors such as family composition (Eshetu, 2015), most research supports a strong and consistent relationship between SES and achievement across countries and cultures (Banerjee, 2016; Gabriel et al., 2016). For example, a meta-analysis examining 101,157 students from 6,871 schools, found medium to strong relations between family SES and student achievement (Sirin, 2005). Similarly, White et al. (2016) found that student SES explained 59% of the variation in math and language scores.
Although it is widely accepted that family SES is associated with academic achievement, the exact mechanisms underlying this relationship are extremely complex and still not fully understood. SES is believed to be important because it provides students with access to important educational resources, such as books and computers, and with opportunities that stimulate learning and brain development (Broer et al., 2019). In addition, family SES is linked to the development of the social capital and knowledge of the education system, which facilitates success in school (Coleman, 1988). Lower family SES has also been linked to limited early learning experiences such as reading aloud, assistance with homework, or visiting educational museums or libraries (Davis-Kean, 2005). Furthermore, children growing up in poverty are more likely to live in single-parent homes, receive poor nutrition, and live in places that are crowded, unsafe, and noisy, all of which are known risk factors for educational success (Burkam and Lee, 2002; Coulton et al., 2007).
As noted above there has been an increased level of interest regarding the relationship between school level SES and academic performance of students within the schools. In these studies, school SES has typically been defined as the aggregated SES of the students at a particular school (e.g., Tsai et al., 2017). Research in this area has found that school level SES adds significantly and uniquely to the explanation of student achievement (Organisation for Economic Cooperation and Development [OECD], 2004; Sauter et al., 2012). For example, Perry and McConney (2010) studied 12,000 students from the Australian Programme for International Student Assessment (PISA) dataset and found significant relationships between school SES and student academic achievement even while controlling for individual SES. Marchant and Finch (2016) examined PISA data for 65 countries and found that school SES emerged as the strongest predictor of student achievement, accounting for more variation than family SES. In addition, researchers have found that differences in school resource allocation and SES equity within schools are also associated with differences in student academic achievement (Song et al., 2014; Parker et al., 2018).
These association between school SES and student achievement appears to emerge from differences in the availability of educational materials at the school, teacher quality, teacher turnover, and the teacher to student ratio (Wenglinsky, 1998; Ingersoll, 1999). Schools with low SES tend to offer fewer educational enrichment opportunities to their students, which in turn may lead to lower academic achievement (Duke, 2000). In addition, schools serving students with lower levels of SES may not receive the same levels of funding as do wealthier schools, leaving gaps in available resources, interventions, and additional programs (U.S. Department of Education, 2011). In addition, higher levels of SES inequality at the school level has been found to be associated with lower academic performance for students from lower SES families (e.g., Thomas et al., 2001; Alacaci and Erbas, 2010).
In comparison to the quantity of research examining relationships between academic achievement with individual level SES, school level SES, and school SES equity, there has been relatively less work devoted to relationships between nation level SES and individual student achievement. Work that has been done, however, suggests that SES inequality at the national level is negatively associated with student academic achievement (Micklewright and Sylke, 2007; Checchi et al., 2014; Parker et al., 2018). In addition, students from wealthier nations have been found to have higher levels of academic performance (Baker et al., 2002; Tucker Drob et al., 2014). Taken together, the evidence from these studies indicates that students’ academic performance is associated to some extent with a nation’s overall wealth and the equity with which that wealth is spread across the population. However, these earlier works do not fully explain how this relationship is transmitted from national level factors to student level performance. Thus, as explained in more detail below, the primary goal of this work is to investigate a possible mechanism for understanding the relationship between national, school, and student level factors, with specific attention on SES, economic equity, and resource allocation.
The primary goal of this study was to assess the plausibility of two models linking nation level funding for public services and economic inequality to school level educational resource and staff shortages, along with teachers’ opinions on educational equity, student level SES, and academic achievement. Given the literature cited above, there is evidence of a link between national, school, and student level variables with academic performance. However, there has not been a formalized model of how these variables are linked to one another. Thus, the current study was designed to test a model in which national level funding for public services and economic equality was related to educational resource and staff shortages in the schools, as well as teacher attitudes regarding educational equity, which were in turn related to student level SES and finally to performance on math and reading tests. These relationships were expressed in both a fully mediated model (Figure 1) and a partially mediated model (Figure 2), which were then compared to one another. The variable labels used in these figures are given in Table 1.
As described above, prior research has demonstrated that family SES is associated with students’ academic performance, as are the resources that schools can bring to the business of education. Furthermore, it has also been shown that greater levels of inequality with respect to SES is also associated with lower academic performance for lower SES students in those schools. Given that schools and students exist within the broader context of their nation states, it is also important to understand the extent to which national economic inequality and country level provision of resources to public services are associated with academic performance of students. Therefore, the goal of this study was to assess whether such national level effects are related to student level academic performance in the form of PISA reading and math test scores, as mediated through school level effects. Two models were considered, one in which the relationship between the national level variables and student achievement are fully mediated through school resources, and the second in which these relationships are partially mediated through school level effects.
The sample used in this study included a total of 612,004 15-year old students (50.3% female) from the 80 nations participating in the 2018 Programme for International Student Assessment (PISA, 2009). A total of 17,145 different schools were included in the sample, across the nations. School samples were selected to be representative within each country (OECD, 2019), and student participants within each school were chosen using KeyQuest software. Data analyses using the PISA student data in this study were weighted by the school and country weights. Only those nations (19) that administered all questionnaires (student, teacher, and school leader) were included in the data analyses.
Mathematics and reading achievement test scores served as the dependent variables in the statistical models that were fit to the data. The 2-h test contains a mixture of multiple-choice and open-ended items, and proficiency scale scores were created and met standards established through Item Response Theory. Family SES was measured by the PISA SES index (ESCS), which was derived by OECD (2010) from a factor analysis of variables that include parent education and occupation, home background, as well as possessions in the home (mean = 0, standard deviation = 1). The ESCS variable was measured at the student level, with higher scores indicating a higher family SES. The PISA dataset includes 10 plausible values for each of the dependent variables. Per guidelines provided by the OECD (2010), separate models were fit for each of the plausible values and then the parameter estimates were averaged, yielding the estimates reported in the results below. The standard errors were calculated so as to account for the within plausible value and between plausible value variability (OECD).
Three school level variables were taken from the PISA data, including measures of staff shortage (STAFFSHORT), educational resource shortage (EDUSHORT), and teacher attitudes toward educational equity (SCHEQUITY). The staff shortage items were completed by school leaders, and focused a lack of teaching personnel, poorly qualified teachers, lack of teaching assistants, and poorly qualified assistants. The resource shortage items were also completed by the school leader, and measured a lack of educational materials, poor quality materials, a lack of physical infrastructure, and poor quality infrastructure. Higher scores for both variables indicated more severe shortages. Finally, a measure of teacher attitudes toward educational equity was taken from the teacher questionnaire and included in the analyses, with higher scores reflecting school staff having a more positive attitude toward providing equitable educational opportunities to students.
At the national level, two variables were selected from the set used to create the fragile states index: Economic inequality and public service funding. These indices, as well as the fragile states index, are described in detail at www.fragilestatesindex.org. Higher values of the economic inequality index reflect a greater degree of unequal opportunities for individuals within a nation to benefit from growth in the economy. The public services variable reflects the extent to which the nation provides basic services (education, health, water, sanitation, transportation) to its citizens, and was scaled so that higher scores indicated a lower level of support for such public services.
In order to address the study goals outlined above, two 3-level mediation models were fit to the data using Mplus, version 8.4 (Muthén and Muthén, 2018), with sampling weights applied in all analyses. The first model appears in Figure 1, and reflects the fully mediated relationship between national levels of economic inequality and funding for public services, through school resources and equity, and student SES. In addition, the relationships between the school level variables and student achievement were fully mediated through SES. The second model (Figure 2) included the mediation effects described above as well as direct relationships between the national level and school level variables with student achievement. Level-1 of the model reflected student effects, level-2 reflected school effects, and level-3 reflected nation effects.
The full and partial mediation models were fit to the data using a Bayesian estimator based on the Monte Carlo Markov Chain (MCMC) approach. For each model, two chains were used, each of which was 20,000 replications long. The first 10,000 links in the chains served as the burn-in period, and the second 10,000 links were thinned with a 1/10 ratio; i.e., each 10th link was retained in order to form the posterior distributions for the model parameters. Model convergence was assessed using the potential scale reduction (PSR) statistic, with values less than 1.1 reflecting acceptable levels of convergence (Muthén and Asparouhov, 2012). In addition, trace plots for each parameter were examined to further investigate the issue of convergence. For both models, the PSR and trace plots revealed that estimation for all model parameters did converge. The mean of the posterior distribution for each parameter was used as the point estimate. The 2.5th and 97.5th percentiles of the posterior distribution were used to create the 95% credibility intervals for the parameter estimates. Those parameters for which the credibility did not include 0 were determined to be statistically significantly different from 0. Comparative model fit was assessed using the deviance information criterion (DIC), with the smaller value indicating better fit. Overall model fit was assessed using the posterior predictive p-value (PPP) and the 95% confidence interval for the difference between the observed and model predicted Chi-square values. PPP values greater than 0.05 were taken to indicate acceptable model fit (Kaplan, 2014), as did Chi-square difference confidence intervals that included 0 (Kaplan). Two aspects of model fit were considered: (1) Which model yielded the best fit as evidenced by having a lower DIC value and (2) Did the better fitting model yield acceptable fit to the data by having a PPP greater than 0.05 and a Chi-square difference confidence interval including 0.
Table 1 includes the means, standard deviations, minimum, and maximum values for the variables used in the analysis. Table 2 contains the Pearson correlation coefficients for the variables used in the full and partial mediation models. The largest correlations associated with the math and reading test scores were student SES (0.41/0.36), national economic inequality (−0.32/−0.23), and national lack of funding for public services (−0.33/−0.25). Thus, it appears that students coming from relatively better off families performed better on both tests, and those who lived in countries with greater economic inequality and lower funding of public services performed worse on both exams. In addition, students attending schools with more serious resource and staff shortages had lower SES values (−0.22 and −0.13), as did those who resided in countries with greater economic inequality (−0.30) and a more severe lack of funding for public services (−0.39). Finally, schools in nations with a higher lack of funding for public services scores also had higher shortages of educational resources (0.21).
Prior to investigating the relationships outlined in the study goals portion above, the intraclass correlations (ICCs) at each level of the data (school and country) were first examined. In order to do this, a null model was fit for reading and math, respectively, in which only the school and country identifiers were included along with the test scores. The variance estimates and ICC values appear in Table 3. The school level ICCs for math and reading were 0.43 and 0.35, respectively, whereas for the nation level they were 0.38 and 0.33. In other words, school and country each accounted for more than one third of the sample variation in test scores. Furthermore, the variance estimates for both outcomes at both levels were statistically significantly different from 0; i.e., the credibility intervals did not include 0. Thus, it can be concluded that the mean reading and math scores varied significantly across both schools and countries.
Table 3. Variance estimates, posterior standard deviations, 95% credibility intervals, and ICC/PCV values for null model and partial mediation models.
The DIC, PPP, and 95% confidence intervals for the difference between the observed and replicated Chi-square goodness of fit statistic values for the full and partial mediation models fit to the math test data appear in Table 4. These results reveal that the partial mediation model yielded better fit than the full mediation model based on its having a lower DIC value. In addition, because the PPP was larger than 0.05 and the 95% confidence intervals for the Chi-square difference included 0, we can conclude that the partial mediation model yields acceptable fit to the data for the math test. Given these results, the model coefficient estimates for the partial mediation were interpreted.
The proportion of variance in the math test scores accounted for by the partial mediation model was 0.26 (Table 3). In other words, considering all of the variables and paths across the data three levels, approximately 26% of student performance on the math test was accounted for by this model. In order to understand the impact of predictor variables at each level, we examined the proportion change in the variance (PCV; Merlo et al., 2006). The PCV represents the decline in random variance after the fixed effects were included in the model, with larger values indicating that the fixed effects accounted for more variance in the response variable. Based on the results in Table 3, it appears that at the student level, 3% of the variance in math test scores was accounted for by family SES. In contrast, at both the school and country levels, the fixed effects variables resulted in a decrease of more than 60% in the random effect variance. In other words, the included variables accounted for more than 60% of the variance that was associated with school or nation in the null model.
The fixed direct effects for the partial mediation for math appear in Table 5 and Figure 3. At the student level there was a statistically significant positive relationship between SES and performance on the math test. Thus, students coming from higher SES families exhibited higher performance on the math test. At the school level, there were statistically significant positive relationships between math scores and the school mean of student SES and school equity. Math test scores were significantly negatively related to shortages in educational resources and staff. In addition, there was a statistically significant negative relationship between school staff shortages and average SES at the school.
At the country level, there were statistically significant positive relationships between math test performance and both the country mean student SES and school equity score. There were significant negative direct effects between the math test score and education resource and staff shortages, economic inequality, a lack of public funding. There were also negative relationships between student mean SES and staff shortages and national economic inequality. There was also a statistically significant positive relationship between a lack of public funding and the school mean educational resource shortage; i.e., schools in nations that provided less funding to public institutions reported more severe educational resource shortages.
Based on these results, it can be concluded that nations with higher mean student SES and school equity scores had higher math test scores. In contrast, those nations with higher school means for educational resource and staff shortages, and those with greater economic inequality and lower funding for public services had lower mean math test scores. Nations which exhibited more mean school staff shortage scores, a greater lack of public funding, and higher economic inequality exhibited lower mean student SES. Finally, schools in nations exhibiting a lack of funding for public services had higher levels of educational resource shortages as well.
The indirect effects, posterior standard deviation, and 95% credibility intervals for the indirect effects for the math test appear in Table 6. There were statistically significant negative indirect effects to the math test score for education resource shortage through family SES, staff shortage through family SES, economic inequality through family SES, lack of funding for public resources through family SES, and lack of public funding through educational resource shortage and family SES. Thus, students attending schools with higher levels of educational resource and staff shortages had lower math test scores as carried through the SES of the students’ families. Likewise, examinees living in nations that had higher levels of economic inequality, and which provided less funding for public resources had lower math test scores, as carried through family SES. Finally, those living in nations that provided less funding for public resources had lower math test scores as carried through educational resource shortages in the schools and family SES.
Table 3 includes the total R2for the reading test, with a value of 0.25. Thus, the partial mediation model accounted for 25% of the variance in the reading test scores. The PCV values for the reading test also appear in Table 3. Based on these values, it appears that family SES resulted in a 0.02 decrease of variance in reading scores, the level-2 fixed effects resulted in a 0.68 decrease in reading test variance scores, and the level-3 fixed effects resulted in a 0.56 decrease in the country level variance.
As with the math test, full and partial mediation models were fit for the reading test scores, using the same set of independent variables at each level as with the reading test. And, as was true for the reading test, the partial mediation model provided the better fit, and indeed fit the data well, based on the values of PPP and the 95% confidence interval for the difference in observed and model implied Chi-square values (Table 4). Table 7 and Figure 4 include the direct effects for the reading test model. At the student level, there was a statistically significant positive relationship between family SES and reading score. In addition, there was a statistically significant positive relationship between the mean family SES and reading test score at the school level. The relationship between the school equity and reading test scores were also statistically significant and positive, whereas the relationships between reading achievement scores educational resource and staff shortages were significant and negative. Scores on the education resource and staff shortage variables were negatively associated with family SES at the school level.
At the country level, there was a statistically significant positive relationship between reading test scores and mean family SES and school equity scores. In contrast, there were significant negative relationships between reading test performance with the mean school staff shortage scores, national economic inequality, and a lack of funding for public services provided in the nation. There was a statistically significant positive relationship between a national lack of funding for public resources and the mean school level educational shortage score, and negative relationships between SES and school staff shortages, economic inequality, and a lack of public funding. With respect to the indirect effects for the reading test score (Table 6), there were significant negative mediated relationships for resource shortages, staff shortages, economic inequality, and a lack of funding for public services all through family SES. There was also a significant negative indirect relationship between a lack of funding for public resources and reading through the school education resource shortage scores and student SES.
Prior research has found evidence for an association between academic performance and student SES, availability and equitable distribution of school resources, and levels of national economic inequality (Rumberger and Palardy, 2005; Sirin, 2005; Coulton et al., 2007; Lee et al., 2019; Gubbels et al., 2020). The goal of this study was to investigate a possible mechanism for these relationships in the form of fully and partially 3-level mediated models. Whereas prior work has examined individual aspects of these relationships, there has been a paucity of published research including all of these factors together. Therefore, this study adds to the literature by including a model in which measures of national support for public services and economic inequality were associated with student academic achievement through the availability of economic resources and staff, as well as school staff attitudes toward educational equity, through family SES. The results presented above suggest that there exist relationships, both direct and indirect, between national, school, and student level variables and academic test performance. As anticipated given prior research (e.g., Tsai et al., 2017), students from lower SES families, and those attending schools with lower mean family SES had lower math and reading test scores. Likewise, students living in countries with higher rates of economic inequality and lower funding for public services also exhibited lower performance on the achievement tests, as reported in Parker et al. (2018). As noted, these results match earlier findings, particularly with regard to the student and school level effects.
A second set of results, which reflect new findings, demonstrated that students attending schools in which the administrative leadership reported greater shortages of educational materials and qualified teaching staff, performed worse on both math and reading achievement tests. In addition, a shortage of educational resources was also associated with a lack of public funding at the national level. In other words, school leaders working in nations that exhibited lower funding for public services, including education, reported more educational resource shortages. Schools in which administrators identified staff shortages also had lower mean student SES; i.e., schools lacking adequately trained teachers and assistants tended to serve more under resourced students. Finally, more positive attitudes toward educational equity among school staff were related to better achievement test performance by their students. This result held at the school level (i.e., test performance was higher in schools where teachers had more positive attitudes regarding educational equity) and at the national level (i.e., nations in which the average teacher attitude toward educational equity was more positive had higher mean test scores). This result is interesting in that it points to a relationship between teachers expressing strong support for educating all students equitably and student academic performance. Prior research (e.g., Song et al., 2014; Marchant and Finch, 2016) has focused primarily on measures of economic equity (which the current study does as well) rather than teacher attitudes.
In addition to assessing these direct effects, a primary goal of this study was to determine whether relationships between student academic performance and school and national level resource allocation and equity variables were mediated by one another, and by student SES. The results demonstrated that such mediation effects were indeed present. Specifically, there were negative indirect effects linking academic performance with school level resource and staff shortages, and national level economic inequality and lack of funding for public services through family SES. Thus, in addition to the direct relationships with academic test performance and these variables as described above, relationships involving them were also mediated through individual families’ economic well-being. Statistically significant negative indirect effects were also found for a lack of public funding through educational resource shortages and family SES to both math and reading test performance. In other words, there is evidence that a lack of funding for public services at the national level is related to greater educational resource shortages within schools, which in turn is associated with lower family SES for students attending those schools, and finally to lower test performance. This finding may provide some explanation of the previously identified link between national wealth and spending on public services and student academic performance (Checchi et al., 2014; Tucker Drob et al., 2014).
The results presented in this paper have several implications for educational research examining economic and resource antecedents to educational performance. First, it would appear that the well-established relationship between family SES and academic achievement has complex antecedents that should be considered by researchers and policy makers. These antecedents include the availability of educational resources, trained staff members, and staff attitudes toward equity within school. In turn, the availability of important resources was directly associated with public funding at the national level. In other words, decisions about funding public services that are made at the national level appear to be directly associated with the resources available to educate children, which in turn was associated with educational performance. A second implication of these results is that, in addition to the school average student SES, the availability of both a qualified teaching staff, and the necessary resources to provide educational services to children appear to be associated with academic performance. As described in the introduction, prior work (Sauter et al., 2012; Heppt et al., 2015; White et al., 2016) has focused on the school mean family SES and its relationship to academic achievement, showing that students who attend schools with lower average family SES tend to perform worse on academic tests. The current study adds to this literature by explicitly demonstrating that the availability of educational resources and trained teachers is also an important factor with regard to achievement test performance, in addition to the family SES for students in the school. Indeed, there is a link between school resources and family SES, such that individuals attending schools with fewer resources tend to come from families with lower SES, and in turn tend to perform worse on both reading and math tests. This complex web of relationships points to the need for policy makers to consider not only issues associated with individual families, but also with ensuring that the schools their children attend have adequate resources.
A third implication of this study comes through the demonstration that national level funding (or a lack thereof) for public services, as well as economic inequality at the national level appear to be associated with individual student achievement test performance. Individuals living in countries that provided less funding for services and where economic inequality was a greater problem performed worse on both reading and math tests, exclusive of their families’ SES or the resources available to the schools. Prior work has focused primarily on either school level effects (which the current study also did) or on indicators of national wealth such as gross domestic product. In the current study the overall mean family SES was incorporated by the multilevel model (see the SES to test relationships at the between countries level), but also included more explicit measures that reflected the national commitment to providing public services to citizens, as well as the degree of economic inequality present in the nation. The results presented here showed that in addition to the mean wealth of students’ families, it is also important to consider how much government support is provided and how unequal the economic landscape of a country actually is. Students living in nations with less public service funding and more economic inequality will tend to perform worse on academic achievement measures than do those in better resourced more equal nations, regardless of their own family’s economic circumstances.
Fourth, the indirect mediational chain of relationships for these national level variables, through school educational resource shortages and family SES to academic test performance provides support for a potential causal link between decisions made at the national level and individual student performance. We are not suggesting that the current study can provide definitive proof of such causal relationships. The manner in which the data were collected precludes our ability to make any such claims. However, the statistically significant indirect effects in the partial mediation model do provide intriguing evidence of the possibility of such causal links. Future research efforts building on the current work should be designed to examine the possibility of such causal relationships. Finally, the results of this study provide evidence for a relationship between teacher attitudes toward educational equity (i.e., the importance to consider and value cultures other than the dominant one in the educational process) and academic achievement. Again, causality cannot be assumed given the nature of the current study. However, these results do provide evidence of a link between teacher attitudes toward equity and student performance, and thus future work should investigate whether the association is merely correlational or whether some causal link is possible.
As with all research, there are limitations to the current work that need to be acknowledged, and that point to directions for future work. First, this study was limited by the nations for which there was PISA data available. Not all nations with economic inequality and public service funding data also had PISA student, teacher, and school leader data. Therefore, the findings described here can only be interpreted with respect to these participating nations. Because the sample of nations participating in PISA does change over time, future work should replicate the current study design in order to ascertain whether the results described here hold for another mix of nations.
A second limitation of this study is with respect to the measure of public funding for basic services. The index used here reflected funding for an array of public services, including not only education but also other services such as health care and sanitation. While the results of the current study supported a statistical link between such public funding and test scores from PISA, it is also true that this index reflects much more than just education spending. Therefore, future research should attempt to identify a measure for public funding of education for each nation and use that in an analysis similar to the one described here. It is important to note that such a measure of public funding for education would need to be comparable across nations (e.g., reflect funding for the same school levels and account for population size differences). One advantage of the index used in the current study is that it has been standardized to be comparable across nations.
As noted above, causality cannot be inferred from the results of this study. However, it would be possible, in theory, to conduct the data analysis using a method such as propensity score analysis or causal learning structures, in an attempt to tease out possible causal links. A difficulty with employing propensity score matching in the current context is that nations would need to be grouped in some fashion based on the variables for which causal investigation is desired (e.g., public funding of education) and the number of nations is relatively small. This twofold problem of arbitrary grouping and small samples could be avoided through the use of a model for continuous causal relationships, though the research into such approaches is in its nascent stage. In addition, synthetic matching represents another possible approach for causal modeling, but suffers from the same arbitrary grouping and sample size issues as does propensity score matching. Nonetheless, it would be certainly be profitable for researchers to consider alternative modeling strategies for this problem that might allow for investigation of causal relationships between the independent variables of interest, particularly public funding for education, and academic outcomes.
There is a strong body of evidence relating academic achievement to student SES, as well as to school level SES and inequalities therein. The current study expanded on this work in two primary ways. First, it established a possible model for this relationship by demonstrating both direct and indirect linkages between national level funding for public services and national economic inequality with the availability of sufficient educational resources and trained staff at the school level to student SES and finally to educational achievement. These relationships were found to be present after accounting for student and school level SES, meaning that they provide unique explanation of academic achievement beyond the well-established SES relationships described in the literature. In addition, this study demonstrated that student performance is associated with resource availability at both national and school levels, above and beyond simple family SES. Thus, educational achievement for individual students cannot be divorced from broader societal factors such as resource availability and inequality. Finally, there appears to be an association between teacher attitudes toward the importance of educational equity and student academic achievement. In short, the results of this study indicate that student academic performance is a multi-faceted phenomenon that is associated with factors beyond simply SES. Researchers and policy makers should, therefore, continue to broaden their view regarding antecedents to academic achievement. Efforts should be made to increase the number of trained teachers, improve access to appropriate educational resources, encourage teachers to take on a more multicultural lens in the classroom, and, where possible, to lobby governments for increased funding and greater economic equality in the general population. These efforts should also appeal to any national leader who is interested in improving student academic performance across their country.
Publicly available datasets were analyzed in this study. This data can be found here: https://www.oecd.org/pisa/data/.
HF conducted data analysis, drafted the methods, results, and discussion. MF conceptualized the research questions, drafted the introduction and discussion. Both authors edited the full manuscript.
The authors declare that the research was conducted in the absence of any commercial or financial relationships that could be construed as a potential conflict of interest.
All claims expressed in this article are solely those of the authors and do not necessarily represent those of their affiliated organizations, or those of the publisher, the editors and the reviewers. Any product that may be evaluated in this article, or claim that may be made by its manufacturer, is not guaranteed or endorsed by the publisher.
Alacaci, C., and Erbas, A. K. (2010). Unpacking the inequality among Turkish schools: findings from PISA 2006. Int. J. Educ. Dev. 30, 182–192. doi: 10.1016/j.ijedudev.2009.03.006
Armor, D. J., Marks, G. N., and Malatinszky, A. (2018). The impact of school SES on student achievement: evidence from U.S. statewide achievement data. Educ. Eval. Policy Anal. 40, 613–630. doi: 10.3102/0162373718787917
Baker, D. P., Goesling, B., and Letendre, G. K. (2002). Socioeconomic status, school quality, and national economic development: a cross-national analysis of the “Heyneman-Loxley Effect” on mathematics and science achievement. Comp. Educ. Rev. 46, 291–312. doi: 10.2307/3542092
Banerjee, P. A. (2016). A systematic review of factors linked to poor academic performance of disadvantaged students in science and maths in schools. Cogent Educ. 3, 1–17. doi: 10.1080/2331186X.2016.1178441
Broer, M., Bai, Y., and Fonseca, F. (2019). “A review of the literature on socioeconomic status and educational achievement,” in Socioeconomic Inequality and Educational Outcomes. IEA Research for Education (A Series of In-depth Analyses Based on Data of the International Association for the Evaluation of Educational Achievement (IEA)), Vol. 5, eds M. Broer, Y. Bai, and F. Fonesca (Cham: Springer).
Burkam, D. T., and Lee, V. E. (2002). Inequality at the Starting Gate: Social Background Differences in Achievement as Children Begin School. Washington, D.C: Economic Policy Institute.
Carnoy, M., and Marshall, J. (2005). Cuba’s academic performance in comparative perspective. Comp. Educ. Rev. 49, 230–261. doi: 10.1086/428721
Checchi, D., van de Werfhorst, H., Braga, M., and Meschi, E. (2014). “The policy response to educational inequalities,” in Changing Inequalities in Rich Countries: Analytical and Comparative Perspectives, eds W. Salverda, B. Nolan, D. Checchi, I. Marx, A. McKnight, I. Gyorgy Toth, et al. (Oxford: Oxford University Press).
Coleman, J. S. (1988). Social capital in the creation of human capital. Am. J. Sociol. 94, 95–120. doi: 10.1086/228943
Coulton, C. J., Crampton, D. S., Irwin, M., Spilsbury, J. C., and Korbin, J. E. (2007). How neighborhoods influence child maltreatment: a review of the literature and alternative pathways. Child Abuse Negl. 31, 1117–1142. doi: 10.1016/j.chiabu.2007.03.023
Davis-Kean, P. E. (2005). The influence of parent education and family income on child achievement: the indirect role of parental expectations and the home environment. J. Fam. Psychol. 19, 294–304. doi: 10.1037/0893-3200.19.2.294
Duke, N. K. (2000). 3.6 minutes per day: the scarcity of informational texts in first grade. Read. Res. Q. 35, 202–224. doi: 10.1598/rrq.35.2.1
Duncan, G. J., and Brooks-Gunn, J. (2000). Family poverty, welfare reform, and child development. Child Dev. 71, 188–196. doi: 10.1111/1467-8624.00133
Eshetu, A. A. (2015). Parental Socio-economic status as a determinant factor on academic performance of students in regional examination: case of Dessie town, Ethiopia. Int. J. Acad. Res. Educ. Rev. 3, 247–256.
Gabriel, M. N., Muasya, I., Mwangi, J., Mukhungulu, M. J., and Ewoi, L. (2016). The influence of parental socioeconomic status, involvement in learning activities and its influence on children’s academic performance in urban informal settlements in Westlands Division- Nairobi County. Int. J. Educ. Soc. Sci. 3, 54–65.
Gubbels, J., Swart, N. M., and Groen, M. A. (2020). Everything in moderation: ICT and reading performance of Dutch 15-year-olds. Large Scale Assess. Educ. 8:1. doi: 10.1186/s40536-020-0079-0
Heppt, B., Haag, N., Bohme, K., and Stanat, P. (2015). The role of academic-language features for reading comprehension of language-minority students and students from low-SES families. Read. Res. Q. 50, 61–82. doi: 10.1002/rrq.83
Ingersoll, R. (1999). The problem of underqualified teachers in American secondary schools. Educ. Res. 28, 26–37. doi: 10.3102/0013189X028002026
Lee, J., Zhang, Y., and Stankov, L. (2019). Predictive validity of SES measures for student achievement. Educ. Assess. 24, 305–326. doi: 10.3389/fpsyg.2020.557751
Marchant, G. J., and Finch, W. H. (2016). Student, school, and country: the relationship of SES and inequality to achievement. J. Glob. Res. Educ. Soc. Sci. 6, 187–196.
Mayer, S. E. (2010). The relationship between income inequality and inequality in schooling. Theory Res. Educ. 8, 5–20. doi: 10.1177/1477878509356346
Merlo, J., Chaix, B., Ohlsson, H., Beckman, A., Johnell, K., Hjerpe, P., et al. (2006). A brief conceptual tutorial of multilevel analysis in social epidemiology: using measures of clustering in multilevel logistic regression to investigate contextual phenomena. J. Epidemiol. Community Health 60, 290–297. doi: 10.1136/jech.2004.029454
Micklewright, J., and Sylke, S. (2007). Inequality of Learning in Industrialised Countries. Southampton Statistical Sciences Research Institute Applications & Policy Working Paper, A06/07. Southampton: University of Southampton.
Muijs, D., Harris, A., Chapman, C., Stoll, L., and Russ, J. (2004). Improving schools in socioeconomically disadvantaged areas – a review of research evidence. Sch. Eff. Sch. Improv. 15, 149–175. doi: 10.1076/sesi.15.2.149.30433
Muthén, B., and Asparouhov, T. (2012). Bayesian SEM: a more flexible representation of substantive theory. Psychol. Methods 17, 313–335. doi: 10.1037/a0026802
Muthén, L. K., and Muthén, B. O. (2018). Mplus User’s Guide, 8th Edn. Los Angeles, CA: Muthén & Muthén.
OECD (2010). PISA 2009 Results: Overcoming Social Background-Equity in Learning Opportunities and Outcomes, Vol. II. Paris: OECD Publishing. doi: 10.1787/9789264091504-en
OECD (2019). PISA 2018 Results: Learning to Learn – Student Engagement, Strategies, and Practices, Vol. III. Berlin: PISA, OECD Publishing.
Organisation for Economic Cooperation and Development [OECD] (2004). Equity and Quality in Education: Supporting Disadvantaged Students and Schools. Berin: OECD Publishing.
Parker, P. D., Marsh, H. W., Jerrim, J. P., Guo, J., and Dicke, T. (2018). Inequality and excellence in academic performance: evidence from 27 countries. Am. Educ. Res. J. 55, 836–858. doi: 10.3102/0002831218760213
Perry, L., and McConney, A. (2010). School socio-economic composition and student outcomes in Australia: implications for educational policy. Aust. J. Educ. 54, 72–85. doi: 10.1177/000494411005400106
Rumberger, R. W., and Palardy, G. J. (2005). Test scores, dropout rates, and transfer rates as alternative indicators of high school performance. Am. Educ. Res. J. 42, 3–42. doi: 10.3102/00028312042001003
Sauter, M. B., Allen, A. C., Nelson, L. A., and Hess, A. (2012). The Richest School Districts in AMERICA. 24/7 Wall Street. Available online at: http://247wallst.com/2012/06/06/americas-richest-school-districts/ (accessed March 14, 2022).
Sirin, S. R. (2005). Socioeconomic status and academic achievement: a meta-analytic review of research. Rev. Educ. Res. 75, 417–453. doi: 10.3102/00346543075003417
Song, S., Perry, L. B., and McConney, A. (2014). Explaining the achievement gap between Indigenous and non-Indigenous students: an analysis of PISA 2009 results for Australia and New Zealand. Educ. Res. Eval. 20, 178–198. doi: 10.1080/13803611.2014.892432
Sullivan, K., Perry, L. B., and McConney, A. (2013). How do school resources and academic performance differ across Australia’s rural, regional and metropolitan communities? Aust. Educ. Res. 40, 353–372. doi: 10.1007/s13384-013-0100-5
Thomas, V., Wang, Y., and Fan, X. (2001). Measuring Education Inequality: Gini Coefficients of Education. Policy Research Working Paper, No. 2525. Washington, DC: World Bank.
Tsai, S.-L., Smith, M. L., and Hauser, R. M. (2017). Families, schools, and student achievement inequality: a multilevel MIMIC model approach. Sociol. Educ. 90, 64–88. doi: 10.1177/0038040716683779
Tucker Drob, E. M., Cheung, A. K., and Briley, D. A. (2014). National GDP, science interest and science achievement: a person by nation interaction. Psychol. Sci. 25, 2047–2057. doi: 10.1177/0956797614548726
U.S. Department of Education (2011). The Potential Impact of Revising the Title I Comparability Requirement to Focus on School-Level Expenditures. Alexandria, VA: A policy brief from the U.S. Department of Education, Policy and Program Studies Service.
van Ewijk, R., and Sleegers, P. (2010). The effect of peer socioeconomic status on student achievement: a meta-analysis. Educ. Res. Rev. 5, 134–150. doi: 10.1016/j.edurev.2010.02.001
Wenglinsky, H. (1998). Finance equalization and within-school equity: the relationship between education spending and the social distribution of achievement. Educ. Eval. Policy Anal. 20, 269–283. doi: 10.3102/01623737020004269
White, G. W., Stepney, C. T., Hatchimonji, D. R., Moceri, D. C., Linsky, A. V., Reyes-Portillo, J. A., et al. (2016). The increasing impact of socioeconomics and race on standardized academic test scores across elementary, middle, and high school. Am. J. Orthopsychiatry 86, 10–23. doi: 10.1037/ort0000122
Keywords: PISA, multilevel model, SES, education funding, mediation model
Citation: Finch H and Finch MEH (2022) The Relationship of National, School, and Student Socioeconomic Status With Academic Achievement: A Model for Programme for International Student Assessment Reading and Mathematics Scores. Front. Educ. 7:857451. doi: 10.3389/feduc.2022.857451
Received: 18 January 2022; Accepted: 24 February 2022;
Published: 07 April 2022.
Edited by:
Sedat Sen, Harran University, TurkeyCopyright © 2022 Finch and Finch. This is an open-access article distributed under the terms of the Creative Commons Attribution License (CC BY). The use, distribution or reproduction in other forums is permitted, provided the original author(s) and the copyright owner(s) are credited and that the original publication in this journal is cited, in accordance with accepted academic practice. No use, distribution or reproduction is permitted which does not comply with these terms.
*Correspondence: Holmes Finch, d2hmaW5jaEBic3UuZWR1
Disclaimer: All claims expressed in this article are solely those of the authors and do not necessarily represent those of their affiliated organizations, or those of the publisher, the editors and the reviewers. Any product that may be evaluated in this article or claim that may be made by its manufacturer is not guaranteed or endorsed by the publisher.
Research integrity at Frontiers
Learn more about the work of our research integrity team to safeguard the quality of each article we publish.