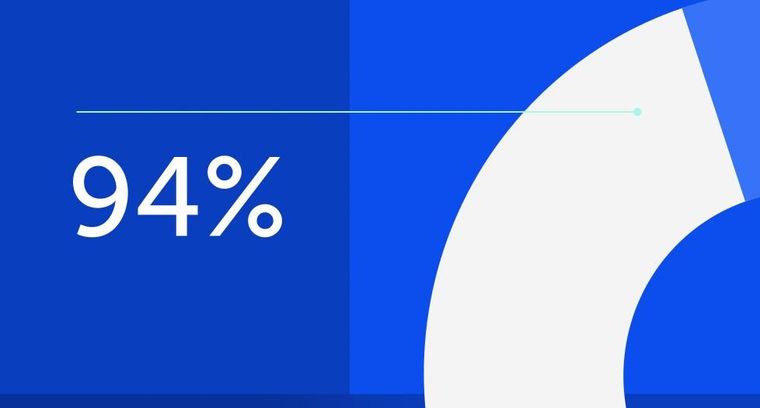
94% of researchers rate our articles as excellent or good
Learn more about the work of our research integrity team to safeguard the quality of each article we publish.
Find out more
ORIGINAL RESEARCH article
Front. Educ., 09 May 2022
Sec. Higher Education
Volume 7 - 2022 | https://doi.org/10.3389/feduc.2022.854436
The purpose of this study is to examine the influence of collegiate environments and experiences on students’ development of innovation capacities over four years of college. Drawing on an interdisciplinary theoretical framework and reliable innovation measures, students from nine postsecondary institutions in North America were surveyed at three time points: first-year fall, first-year spring, and fourth-year spring. Data were comprehensively analyzed using a growth mixture modeling approach. Results suggest that being a transfer student and having sustained engagement with experiences that connect in-class and out-of-class learning were associated with a robust innovation growth trajectory over-and-above known covariates, including personality traits. Implications for research, theory, and practice are considered.
An important current shaping postsecondary education over the last decade has been a focus on developing innovators – students and graduates able to effectively engage the process of generating and executing contextually beneficial new ideas (Wagner, 2012; Selznick and Mayhew, 2019). As institutions have increasingly recognized the benefits of developing students’ innovation capacities – those skills and abilities needed to effectively engage innovation (Selznick and Mayhew, 2018) – practices have moved beyond traditional associations with entrepreneurship education (Nabi et al., 2017) toward more inclusive presentations. This shift has resulted in novel learning experiences that can be woven throughout the curriculum (Swayne et al., 2021), considerations for institutional transformation (Hall and Lulich, 2021), and the establishment of durable innovation networks (e.g., Thompson, 2018). Indeed, many institutions and their stakeholders now consider innovation an area that can satisfy many while alienating few: it fulfills students’ demands for leveraging education for practical ends, achieves parents’ and employers’ demands for developing workforce-ready graduates, and addresses legislators’ demands for demonstrating 21st century value (e.g., Swayne et al., 2021).
Despite benefits associated with developing innovators (Wagner, 2012), and the expansion of resources associated with achieving this outcome (e.g., Wyllie, 2018), limited attention has been paid to examining the longitudinal development of students as innovators across four years of undergraduate learning. Previous efforts have considered the measurement of innovation capacities (Selznick and Mayhew, 2018); their development during the first year of undergraduate study (Selznick and Mayhew, 2019); the associations between specific collegiate interventions and innovation capacities (Mayhew et al., 2012, 2016, 2019, 2021); and the connection between students’ innovation intentions and integrative learning (Selznick et al., 2021a). These studies have been limited by their time horizon, with research designs unable to address the influence of a longer undergraduate career on the development of innovation capacities.
In this study we address this limitation and ask: What collegiate environments and experiences influence the development of students’ innovation capacities over four years of college? Utilizing growth mixture modeling (GMM; see Duncan et al., 1999) we identify latent classes within the data and test the extent to which development is related to pre-existing student characteristics and differential learning exposures.
This study is framed by two theoretical perspectives: Kegan’s (1994) lines of human development and ecological approaches to the study of college students. Kegan’s (1994) theory of human development maintains that personal growth occurs along three interrelated and interdependent dimensions of the self: intrapersonal, social, and cognitive. The learning that propels development along these lines can be either informative or transformative in nature. Transformative learning involves “the development of a capacity for abstract thinking so that one can ask more general, thematic questions about the facts” (Kegan, 2009, p. 42). While informative learning is vital, transformative learning can unlock the shifts in perception, thinking, and doing often associated with innovation capacities (see Wagner, 2012; Swayne et al., 2019). This framework informs the measurement of innovation capacities comprising intrapersonal (e.g., motivation), social (e.g., teamwork across difference), and cognitive (e.g., creativity) dimensions as well as directing our inquiry toward transformative learning experiences.
Our examination of these experiences and the environments in which they are embedded draws upon ecological systems theory, specifically Bronfenbrenner’s (1979; 1993) chronosystem and Renn and Arnold (2003) refinement of the concept. Ecological systems theory recognizes that students exist in a constant dynamic with actors, systems, policies, and historical legacies operating both within and beyond their immediate control. These systems and the individuals within them continuously vary over time, generating both organizational histories and trajectories of human development. These developmental trajectories differentiate according to an individual’s immutable features (e.g., personality, race), and how students interact with the environmental systems around them (e.g., peer cultures, college experiences, academic integration, institutional conditions). Thus, ecological systems theory is a valuable guide to the explanation and interpretation of differential developmental trajectories due to person-environment interactions.
While our study does not and cannot explicitly consider all aspects of the ecologies that motivate or inhibit students’ innovation capacity development, we are draw on these considerations when building our latent class analyses with respect to pre-college characteristics which may shape growth trajectories. We further draw on such approaches when modeling predictors of classification and growth (e.g., learning practices, academic pathways) associated with college-going.
We reviewed an interdisciplinary body of literature covering individual and institutional factors associated with innovation to specify our growth mixture model. While not exhaustive, each set of perspectives addresses concepts and variables previously incorporated in the study of developing innovators during college (e.g., Morris et al., 2013).
While innovation can be taught (Mars and Rhoades, 2012; Bock et al., 2020), the possession of innate personality characteristics has also been consistently associated with more powerful development of innovation skillsets (Pittaway and Cope, 2007; Brandstätter, 2011). Specifically, meta-analyses and studies utilizing the big-five personality inventory have reported possessing higher levels of openness to new experiences (Kerr et al., 2017), conscientiousness, proactivity (Newman et al., 2019), extraversion, and agreeableness (Leutner et al., 2014) to be associated with greater innovation. Hence, our measurement instrument and analytic model both included variables measuring personality traits.
Considerable research suggests that gender plays an important role in predicting innovative and entrepreneurial outcomes due to systems of inequality and oppression that privilege patriarchal identities, values, and behaviors (Wilson et al., 2007). Cognitively, women and men may differ in how they perceive and process opportunities for innovation (DeTienne and Chandler, 2004, 2007) and women frequently exhibit less tolerance for risk (Shinnar et al., 2012), likely due to the disproportionate costs they may incur by failing. Regarding intrapersonal distinctions, women’s entrepreneurial self-concept and self-efficacy appear to be affected by subtle messaging in their environments, resulting in disparities that are evident from an early age and that reduce the propensity of women to actually engage in innovation, even when they possess the skill and opportunity to do so (Langowitz and Minniti, 2007; Wilson et al., 2007). As such, it is vital to include gender in any approach to modeling innovation trajectories through college (see also Huang-Saad and Celis, 2017).
In addition, structures of oppression provide opportunities to members of some racial and ethnic groups while denying them to others in the innovation space (Fairlie, 2005). Theoretical approaches have considered innovation among BIPOC students through sociocultural lenses (Walker, 2009; Gold, 2016; Wingfield and Taylor, 2016) as being responsive to contexts which are the result of historical exclusions from equitable access to capital, innovation power structures, and inclusive policy. Undergraduate studies have demonstrated that racially minoritized students engage in the entrepreneurial co-curriculum as frequently as their White peers (Huang-Saad and Celis, 2017), and that they are equally or more interested in becoming innovative entrepreneurs (Rodriguez et al., 2015; Gilmartin et al., 2019).
This study’s model also includes two important predictors associated with family: first generation college student status and family history of innovation. First, ample research (e.g., Pascarella et al., 2004; Tate et al., 2015; Carpenter and Peña, 2017) demonstrates the salience of including first-generation student status in empirical attempts to understand the relationship between collegiate learning and outcomes given possible structural, financial, and sociocultural constraints experienced by these student populations (Davis, 2010). Second, research emerging from entrepreneurship studies (e.g., Schmitt-Rodermund, 2004; Marques et al., 2018) suggest the importance of considering family history with innovation and/or entrepreneurship when examining students’ learning and development on these dimensions (Newman et al., 2019).
Finally, we included several predictors associated with students’ academic profiles to consider students’ emergent innovation capacites in the context of majors (Chopp et al., 2016), academic performance (Wagner, 2012), transfer status (Johnson, 2005), and international student presentations (see Moriano et al., 2012). Given previous approaches to this topic, this study was particularly attuned to the extent to which innovation capacity development was associated with major (e.g., business, engineering) and whether there could be a connection between transfer and innovation capacities along developmental dimensions (see Lukszo and Hayes, 2020).
In addition to summative examinations of how colleges can generate organizational identities that foster innovative campus climates (see Morris et al., 2017), multidisciplinary literature has considered the extent to which curricular and co-curricular experiences can promote undergraduate innovation. Studies of innovation-specific learning (Martín et al., 2017; McCarthy et al., 2018) and its possible associations with campus-wide entrepreneurship education (Nabi et al., 2017; Passaro et al., 2018) indicate that curricular experiences can support students’ innovation capacity development. Beneficial pedagogical elements include high-quality faculty teaching, active collaboration (Loes, 2019), experiential learning (Mason and Arshed, 2013), and opportunity identification (Corbett, 2005).
Studies of co-curricular experiences (e.g., Selznick et al., 2021a), have illuminated the benefits of integrative learning, or learning that connects student identities, classroom knowledge, and out-of-class experiences (e.g., across knowledge domains, social environments, etc.) in new ways. Additionally, engaging with student associations (Padilla-Angulo, 2019) and long-term career development (Lange et al., 2011) have been identified as positive contributors to innovation capacity development. As Walter and Lankes (2015) observe with respect to libraries: “Innovation may involve collaboration with student affairs professionals to consider the impact of co-curricular spaces on student learning” (p. 855). Motivated by this literature review, we incorporated aspects of students’ perceptions of their curricular (e.g., faculty challenge) and co-curricular (e.g., connecting experiences) experiences associated with innovation into our survey and subsequent quantitative model.
This study used quantitative data collected via an established survey of college students’ innovation capacities (Selznick and Mayhew, 2018). Using survey data collected at three different timepoints during college, longitudinal analyses were conducted to evaluate trends and predicators of students’ change in innovation capacities over time.
College students were sampled at 9 institutions, which were recruited to participate on the basis of their demonstrated interest in the project. The analytic sample included all students who responded to at least two of the three timepoints (N = 572) in order to protect the integrity of the longitudinal analysis. Descriptive statistics for all variables are reported in Table 1. Given the lack of a definable set of respondent characteristics associated with missingness, data were treated as missing at random (MAR). Full information maximum likelihood (FIML) estimation was then used to handle cases of missing data as it allows for utilization of all available data points, while maintaining the accuracy and integrity of model parameters (Wothke, 2000).
The survey was administered electronically at three timepoints. Students were initially surveyed as first-year college students in the fall of 2015 (Time 1). The second administration occurred in the spring of 2016 (Time 2). The third administration occurred in the spring of 2019 (Time 3).
The instrument employed was the Innovation Capacities Scale developed by Selznick and Mayhew (2018) to evaluate students’ innovation capacities as a higher education outcome. The internal structure of the scale and subscales demonstrated strong unidimensionality as evinced by Cronbach’s α values above 0.7 (Cronbach, 1951; DeVellis, 2016). The scale included 42 survey items capturing nine innovation capacities: intention to innovate (α = 0.78), self-concept (α = 0.80), creative cognition (α = 0.86), proactivity (α = 0.81), persuasive communication (α = 0.84), risk taking (α = 0.85), teamwork (α = 0.83), motivation (α = 0.72), and networking (α = 0.85). Using second-order confirmatory factor analysis (Rindskopf and Rose, 1988; Selznick and Mayhew, 2018) demonstrated the robust fit of their model, and established criterion validity with a sample of 1,379 first-year college students at six North American institutions. All nine innovation capacities were first-order factors comprising one higher-order factor: innovation capacity (α = 0.94). To account for the known influence of personality traits (Chell, 2008), the instrument also included the Ten Item Personality Inventory (TIPI; Gosling et al., 2003), a valid and reliable measure of the Big Five personality traits (Costa and McCrae, 1992).
The same framework and measures used by Selznick and Mayhew (2019) were applied in the current study. Specifically, innovation capacities factor scores were the outcome measure; composite scores for students’ various curricular and co-curricular experiences were used as environmental measures; survey items capturing student demographics and precollege characteristics were used as input measures; and student attributes related to the college environment were used as bridge measures.
The outcome measure was derived from that established by Selznick and Mayhew (2018). Specifically, factor scores computed from the second-order innovation capacities factor were used as the outcome of interest. Factor scores were computed via Mplus software employing the regression-based maximum a posteriori method (Muthén and Muthén, 1998/2017). Factor scores were converted to a 100-point scale, with 0 indicating the lowest possible innovation capacity and 100 indicating highest possible innovation capacity to assist result interpretation.
The model included twelve variables accounting for students’ demographic and pre-college characteristics. Specifically, these comprised measurements of the “big five” personality characteristics (extroversion, conscientiousness, neuroticism, openness, and agreeableness; Gosling et al., 2003), alongside their gender identity, their race/ethnicity, their high school grade point average (GPA), whether or not they were first generation students, whether or not they possessed a family member who started a new business or non-profit (family business), or possessed a family member who invented a new product, service, or process (family innovator). First generation status, family business, and family innovator were dummy coded.
Bridge measures are characteristics inherent to an individual, but that primarily exist within the college context (Astin, 1991). In the present model, these included students’ college major, whether or not they were an international student, and whether or not they had taken any courses in entrepreneurship, creativity, or innovation (innovation course taker), with these last two variables being dummy coded.
Drawing on measures initially developed as part of the Wabash National Survey (WNS; e.g., Pascarella et al., 2004, 2005), and continued in previous efforts in this line of research (e.g., Selznick, 2017), the model included eight composite environmental measures divided evenly between those measuring curricular and co-curricular experiences. These were gathered at Times 2 and 3. The curricular measures evaluated the degree to which faculty challenged them to think in new and original ways (faculty challenge, α = 0.83), the frequency and quality of their interactions with faculty members (faculty interaction, α = 0.80), how often they experienced assessments that required the development and defense of arguments (assessments: argument development, α = 0.80), and how frequently they experienced assessments that encouraged innovative problem solving (assessments: innovation problem solving, α = 0.71). The co-curricular measures evaluated how often participants were able to connect extracurricular experiences to in-class learning (connecting experiences, α = 0.88), how frequently they had social experiences that encouraged innovation (social experiences, α = 0.89), how encouraging of innovation they experienced the campus to be (campus encouragement, α = 0.87), and how deeply the campus supported their career development (career development support, α = 0.74).1 Each of these composite measures were standardized before entry.
This study used growth mixture modeling, or GMM (Muthén, 2004) within a structural equation modeling (SEM) framework in order to quantify longitudinal changes in students’ innovation capacities scores. All analyses were executed using Mplus (Muthén and Muthén, 1998/2017). Traditional growth models assume that the sample represents a single population that can be accurately characterized by one growth trajectory; GMM, however, relaxes that assumption and allows for the possibility that the sample includes multiple distinct subgroups, or “classes,” of individuals, with each subgroup characterized by its own separate change trajectory (Jung and Wickrama, 2008; Shiyko et al., 2012). It thus produces separate growth estimates for each subgroup inferred from the data, making it “naturally suited” for addressing person-centered research questions pertaining to longitudinal development (Diallo et al., 2017, p. 166).
In accordance with the methodological literature (e.g., Muthén, 2004; Grimm et al., 2017), our growth mixture modeling analysis included multiple steps: (1) identification of latent classes based on both statistical and theoretical considerations, (2) examination of developmental trajectories based on estimated growth parameters, and (3) prediction of class membership via incorporation of input and environmental measures.
The class enumeration process—or identification of the number of latent classes present in a dataset—is determined by a combination of factors including statistical fit indices, substantive theoretical justifications, and the interpretability of the latent classes (Muthén, 2004; Nylund et al., 2007). To begin the class enumeration process a one-class model was established as the baseline; this one-class model assumed that development was homogeneous across all students, and thus was fully captured by a single growth trajectory (Shiyko et al., 2012). Alternatively, models with n latent classes allow for the possibility that there are n distinct trajectories in students’ change in innovation capacities during college. If such a model offers an improvement over the baseline model, then it can be concluded that there are multiple (n) different developmental trajectories in students’ change in innovation capacities during college (Shiyko et al., 2012)—trajectories which are sufficiently distinct to warrant modeling separately.
Thus, using the innovation capacities outcome, models comprised of one, two, and three latent classes were estimated and evaluated. Comparative indicators of global model fit were used to guide the GMM class enumeration process. Those indicators included the Bayesian Information Criterion (BIC), sample-size Adjusted Bayesian Information Criterion (ABIC), and Akaike Information Criterion (AIC). In these indicators a lower value indicates a better fitting model. The Lo et al. (2001) Likelihood Ratio Test (LMR-LRT), Vuong, Lo, Mendell, and Rubin Adjusted Likelihood Ratio Test (VLMR), and Bootstrap Likelihood Ratio Test (BLRT) were also used to compare nested models. The LMR-LRT, VLMR, and BLRT are alternatives to the likelihood ratio test whereby a significant value suggests that an estimated model with K classes is superior to a model with K-1 classes.
Once the number of classes inferred from the data was established based on statistical fit, relevant theory, and model interpretability, growth trajectories for each identified subgroup were examined. In order to understand the trends of each identified subgroup, each class received its own unique estimate of the mean intercept and mean slope for innovation capacities. The mean intercept reflects students’ baseline innovation capacities at Time 1, and the mean slope reflects the magnitude and direction of change in students’ innovation capacities at Times 2 and 3. For both the intercept and slope, a significant value indicates that the estimate is significantly different from zero. Of particular interest in this study was the slope, as it serves as an indicator of whether students’ innovation capacities increased (or declined) in any significant ways during their time in college. The growth trajectory for each latent class was then modeled via a simple equation in which Outcome = Intercept + Slope(Time).
For this final model, entropy was also evaluated as a measure of classification quality, or how distinguishable the classes and their associated trajectories were from one another. Entropy values range from a low of 0 (i.e., not distinguishable) to a high of 1 (i.e., very distinguishable) (Grimm et al., 2017).
In order to evaluate which factors are most influential in promoting students’ development of innovation capacities in college, significant predictors of the growth trajectories were examined. Input, environmental, and bridge variables consistent with those presented by Selznick and Mayhew (2019) were incorporated into the GMM to evaluate which variables predicted students’ class membership. A manual three-step approach (Vermunt, 2010) was used. This approach did not treat students’ class membership as a perfectly reliable indicator; instead, it used the probabilities of class membership from the original unconditional GMM (step 1) to estimate the error in students’ classification (step 2), and then account for that error in the conditional predictor model (step 3) (Asparouhov and Muthén, 2014; Nylund-Gibson et al., 2019).
Consistent with the GMM framework, rather than assuming uniform influence across the sample, the influence of covariates was estimated separately for each latent class. The ability of covariates to predict class membership in GMMs was described via multinomial logistic regression parameters (Petras and Masyn, 2010). The resulting estimates were odds ratios, which indicated the likelihood of particular individuals having membership in one class compared to a reference class. To aid interpretation, odds ratios were also converted to probabilities.
This study has several important limitations. First, the institutional sample for this study is not representative of the full scope of postsecondary institutions. Subsequently, the students who self-selected into these institutions and into our sample via participation in the survey at multiple time points may not be fully representative of the diversity comprising postsecondary attendees. Resultantly, we make no claims of universal generalizability for our findings, instead recognizing that, despite the quality of our longitudinal data and rigor of our analysis, we can only forward suggestive, not definitive results.
Additionally, scholars (Bauer and Curran, 2003a,b; Bauer, 2007) have noted that GMM analyses can over-extract substantively meaningful classes and/or trajectories under some conditions. Furthermore, GMM’s can sometimes become stuck at local maxima, producing results that do not match the solution of the global maximum likelihood function (Hipp and Bauer, 2006). We thus urge a degree of caution and remind that the results of this study aim to provide a carefully conducted, if inherently probabilistic, empirical picture of latent classes, growth pathways, and predictors. Such findings are not meant to be end-point statements on these matters, but rather, evidence that is subject to further testing within collegiate contexts.
The class enumeration process included estimating and examining fit for the one-, two-, and three-class innovation capacities models. Evaluation of model fit criteria provided consistent evidence that the two-class model was a better fit than the one-class model, exhibiting lower AIC, BIC, and ABIC values and significant LMR and VLMR tests. While the AIC, BIC, and ABIC values were lower for the three-class model than the two-class model, the LMR and VLMR tests were non-significant, indicating that the three-class model did not provide a better fit to the data than the two-class model. Furthermore, the third class comprised only 1.5% of the sample, (i.e., less than 10 students). Any class consisting of such a small proportion of the sample is likely to be the result of sample-specific trends or overextraction, rather than representing meaningful differences in the larger population.
Statistical model fit indices used for comparison of the one-, two-, and three-class models are summarized in Table 2. Ultimately, evaluation of model fit criteria indicated that the two-class model fit the data best. In other words, there was sufficient heterogeneity in students’ growth trajectories for multiclass modeling and that heterogeneity was best captured by two separate latent classes. Accordingly, all subsequent analyses were conducted using the two-class GMM.
The 2-class model revealed distinct patterns of students’ change in innovation capacities over the three timepoints. Table 3 reports initial values (i.e., intercept) and average change over time (i.e., slope) by class. As depicted in Figure 1, the two classes included a no growth trajectory (class 1, 87% of the sample) and a high growth trajectory (class 2, 13% of the sample). Students in class 1 started their first year of college with moderate innovation capacities (intercept = 52.605, p < 0.001) and experienced no significant change in those capacities at subsequent timepoints (slope = − 0.592, p = 0.422). Contrarily, students in class 2 started their first year of college with moderate innovation capacities (i.e., though they were slightly higher than those of class 1; intercept = 75.090, p < 0.001) and experienced significant positive growth in those capacities during their time in college (i.e., slope = 7.449. p = 0.014). An entropy value of 0.790 suggests that the resulting two classes were sufficiently distinguishable from one another.
Figure 1. Growth trajectories of students’ innovation capacities over time. Innovation capacities = Intercept + (Slope * Time). Dotted trend line indicates non-significant slope (p > 0.05).
After establishing the growth trajectories for the 2-class GMM, the input, bridge, and environmental variables were added to the model to determine what characteristics or experiences predicted growth during college. The results, which were obtained using multinomial logistic regression, are reported in Table 4 with class 1 (the class with no significant growth) as the reference group and class 2 (the class demonstrating significant growth) as the focal group. Five significant predictors emerged: extroversion, conscientiousness, openness, transfer student status, and connecting experiences.
Input variables capturing students’ self-reported personality characteristics and transfer status significantly predicted growth in their innovation capacities. Students who reported higher extroversion had a 73.9% probability (odds ratio [OR] = 2.833; Probability [P] = 0.739; p-value [p] < 0.001) of belonging to the growth class. Students who reported higher conscientiousness had a 66.0% probability (OR = 1.938; P = 0.660; p = 0.008) of experiencing growth in innovation capacities. Students who reported higher openness had a 72.3% probability (OR = 2.604; P = 0.723; p < 0.001) of experiencing growth in innovation capacities. Additionally, students who reported that they transferred from another university had a 77.5% probability (OR = 3.448; P = 0.775; p = 0.008) of being in class 2.
One environmental/experiential variable significantly predicted class membership: connecting experiences. At time 3, students who reported having greater exposure to out-of-class experiences that had a positive influence on personal growth, attitudes, and values or that provided opportunities to translate knowledge and understanding from the classroom into action were significantly more likely to be in the growth class. Specifically, students who reported such connecting experiences had 90.2% probability (OR = 9.174; P = 0.902; p < 0.001) of developing their innovation capacities.
Results suggest that while several individual personality traits were significant predictors of innovation capacity development, the more powerful predictors of who developed as an innovator and who did not are what experiences students have in college. Specifically, high exposure to connecting experiences and being a transfer student were both statistically and practically significant predictors of belonging to the 13% of the sample that experienced a growth trajectory over four years of college. These findings potentially indicate pathways for stimulating the development of innovators and, ideally, coming to expand such development for an increasing number of undergraduate students.
The powerful association between connecting experiences and innovation capacity growth suggests that what happens outside of class – the myriad forms of intrapersonal, social, and cognitive development that are essential to providing a truly holistic postsecondary education – aren’t incidental or detrimental to student learning, but integral and central. In fact, it may be the case that time out of class is where the translation and application of new knowledge into contextually beneficial action must occur. This finding further supports the developmental benefits of applied learning, that is, approaches anchored in activity, collaboration, and integrating knowledge which may provide opportunities for transformation (Trolian and Jach, 2019). Moving forward, we suggest that traditional ‘sage-on-the-stage’ college learning may be limiting students’ innovation capacity development by systematically failing to ignite undergraduates’ active quests toward producing new ideas (Cavagnaro and Fasihuddin, 2016).
The finding regarding transfer student status predicting high development may indicate both individual and institutional lines of influence. Individually, the act of transferring may stimulate innovative coping (Anglin et al., 1995). Students who choose to transfer need to accept potential personal, social, and financial risks; be comfortable with quitting and starting anew; must possess intrinsic motivation and navigational capital to persist across institutional systems; and might need to be persuasive in their communication and proactive in establishing new social networks (Johnson, 2005). They may have also learned an important mindset for innovation – that there are what Dubner (2011) terms “upsides of quitting”. At the institutional level, this finding presents evidence that some students may transfer not because something is “wrong with them” but, instead, because the institution is not supporting their educational needs. Further, and in line with our previous findings (Selznick et al., 2021b), it is possible to create a campus climate and reputation for innovation. Therefore, students who transferred into the institutions in our sample may have done so because these institutions were known to provide supportive innovation environments.
While interpreting non-significant findings entails certain caveats (Field, 2017), no gender, race/ethnicity, major, or familial background predicted inclusion in the growth class. Such results in the presence of other significant predictors – most notably connecting experiences – indicate that such characteristics do not predetermine longitudinal innovation capacity development. This suggests that colleges can not only develop innovators, but can do so in ways that support diversity, equity, and inclusivity regarding who is deemed an innovator, in what contexts, and through what means (Hamilton, 2020).
Interpreting findings comprehensively through the lens of our theoretical framework, we contend that developing innovators is perhaps more ecological than idiosyncratic in nature (Wagner, 2012; Swayne et al., 2019). Specifically, college ecologies that prioritize the integration and sustained application of learning throughout the continuous experiences of class, co-curricular involvement, community engagement, friendships, and pre-college relationships appear to promote innovation; those which impose artificial discretization and do not encourage knowledge application seem less effective. Or, building on Kegan (1994), approaches that foster transformational learning contribute to multidimensional growth and innovative development, while approaches restricted to informational learning do not.
We now turn to considerations of our findings for theory, research, and practice. Regarding theory, our findings are broadly in line with Kegan’s (1994) proposed mechanisms of college student learning. As he observes: “Educators seeking ‘self-direction’ from the adult students….are asking many of them to change the whole way they understand themselves, their world, and the relationship between the two” (Kegan, 1994, p. 275). This suggests higher education for innovation can come to be a place of un-learning (Battilana et al., 2019; Selznick and McCarthy, 2020) and that such applied (un)learning may overcome personality differentials and lead to more effective innovation capacity development.
Concerning ecological systems theory, our findings suggest that collegiate environments are perhaps more fluid than discrete in their manifestations and receptions by students. This consideration appears with respect to transfer students who leave one collegiate ecology in search of another; the importance of a sustained commitment to high-quality and intentional connecting experiences; the mechanisms that can support developing certain personality types as innovators; and even those climates that support developing innovators across genders, race/ethnicities, and family backgrounds. Recent work by Winks et al. (2020) further invites consideration of the importance of physical spaces and their associated cultures as key features of learning ecologies.
These findings suggest several new directions for research. First, future work should investigate connecting experiences to uncover where they are occurring in the ecology, amongst which student sub-cultures, and how precisely they stimulate innovation capacity development. Second, our findings should motivate more comprehensive studies of the distinctive postsecondary trajectories of transfer students (Lukszo and Hayes, 2020), including those who transfer from two- to four-year institutions and those who transfer among four-year institutions (Renn and Reason, 2013). Transfer students are at best under-studied and, at worst given the tuition-driven, marketized nature of postsecondary education (Taylor and Cantwell, 2019), blamed. Finally, and echoing our previous work (Mayhew et al., 2016), we suggest future researchers attempting to build comprehensive regression models consider the role of personality traits in influencing student behavior and outcomes.
Our considerations for practice concentrate on connecting experiences given their magnitude in our multinomial regression analysis. Guidance for contemporary employment of these practices is widespread, often focusing on forms of problem-based learning (Youngerman and Culver, 2019), collaborative learning (Loes, 2019), and/or transdisciplinary collaborations that bring students together and integrate their disciplinary insights to address complex societal issues (Heinrich et al., 2021). As indicated by ecological systems theory, the structured delivery of such experiences and subsequent student meaning-making must be an institutionally-supported priority, which empowers educators to establish integrative learning contexts (McCarthy et al., 2018; Barber, 2020). In short, if the pathway to developing innovators over-and-above personality traits or transferring lies in these experiences, they must be actively and intentionally promoted through close collaborations between academic, student life, and policy stakeholders.
While innovators may be born as a function of personality traits, findings from this study suggest they can also be developed through college experiences, regardless of family background, gender identification, or race/ethnicity. Given the complex challenges facing the 21st century, colleges must carefully consider how learning practices and environments proactively support students’ innovation capacity building – especially as this outcome is sought after by contemporary undergraduates and increasingly reflected in strategic plans. An emphasis on innovation, moreover, may have effects well beyond college and help ensure that postsecondary graduates confidently enter a world where fresh ideas and novel solutions are desperately needed.
The raw data supporting the conclusions of this article will be made available by the authors, without undue reservation.
The studies involving human participants were reviewed and approved by Institutional Review Board The Ohio State University. The patients/participants provided their written informed consent to participate in this study.
BS contributed to the introduction, literature review, findings, and discussion. MM contributed to the introduction, findings, and discussion. CW contributed to the methods, findings, and discussion. EM contributed to the introduction, literature review, and discussion. All authors contributed to the article and approved the submitted version.
We gratefully acknowledge the financial support of the Merrifield Family Foundation in conducting this research. This foundation provided funds for participant incentives. No grant number is available.
The authors declare that the research was conducted in the absence of any commercial or financial relationships that could be construed as a potential conflict of interest.
All claims expressed in this article are solely those of the authors and do not necessarily represent those of their affiliated organizations, or those of the publisher, the editors and the reviewers. Any product that may be evaluated in this article, or claim that may be made by its manufacturer, is not guaranteed or endorsed by the publisher.
Anglin, L. W., Davis, J. W., and Mooradian, P. W. (1995). Do transfer students graduate? A comparative study of transfer students and native university students. Commun. Coll. J. Res. Pract. 19, 321–330. doi: 10.1080/1066892950190404
Asparouhov, T., and Muthén, B. (2014). Auxiliary variables in mixture modeling: three-step approaches using Mplus. Struct. Equ. Modeling 21, 329–341. doi: 10.1080/10705511.2014.915181
Astin, A. W. (1991). Assessment for Excellence: The Philosophy and Practice of Assessment and Evaluation in Higher Education. New York, NY: Macmillan.
Barber, J. P. (2020). Facilitating the Integration of Learning: Five Research-Based Practices to Help College Students Connect Learning Across Disciplines and Lived Experience. Sterling, VA: Stylus.
Battilana, J., Bulter, B., Kimsey, M., Mair, J., Marquis, C., and Seelos, C. (2019). “Problem, person, and pathway: a framework for social innovators,” in Handbook of Inclusive Innovation, eds G. George, T. Baker, P. Tracey, and H. Joshi (Cheltenham: Edward Elgar), 61–74. doi: 10.4337/9781786436016.00012
Bauer, D. J. (2007). Observations on the use of growth mixture models in psychological research. Multivariate Behav. Res. 42, 757–786. doi: 10.1080/00273170701710338
Bauer, D. J., and Curran, P. J. (2003a). Distributional assumptions of growth mixture models: implications for overextraction of latent trajectory classes. Psychol. Methods 8, 338–363. doi: 10.1037/1082-989X.8.3.338
Bauer, D. J., and Curran, P. J. (2003b). Overextraction of latent trajectory classes: much ado about nothing? Reply to Rindskopf (2003), Muthén (2003), and Cudeck and Henly (2003). Psychol. Methods 8, 384–393. doi: 10.1037/1082-989x.8.3.384
Bock, C., Dilmetz, D., Selznick, B. S., Zhang, L., and Mayhew, M. J. (2020). How the university ecosystem shapes the innovation capacities of undergraduate students – evidence from Germany. Ind. Innov. 28, 307–342. doi: 10.1080/13662716.2020.1784710
Brandstätter, H. (2011). Personality aspects of entrepreneurship: a look at five meta-analyses. Pers. Individ. Dif. 51, 222–230. doi: 10.1016/j.paid.2010.07.007
Bronfenbrenner, U. (1979). The Ecology of Human Development: Experiments by Nature and Design. Cambridge, MA: Harvard University Press.
Bronfenbrenner, U. (1993). “Ecological models of human development,” in Readings on the Development of Children, 2nd Edn, eds M. Gauvain and Cole, M. (Amsterdam: Elsevier), 37–43.
Carpenter, A. M., and Peña, E. V. (2017). Self-authorship among first-generation undergraduate students: a qualitative study of experiences and catalysts. J. Divers. High. Educ. 10, 86–100. doi: 10.1037/a0040026
Cavagnaro, L. B., and Fasihuddin, H. A. (2016). A moonshot approach to change in higher education: creativity, innovation, and the redesign of academia. Lib. Educ. 102, 8–17.
Chell, E. (2008). The Entrepreneurial Personality: A Social Construction, 2nd Edn. London: Routledge.
Chopp, R., Frost, S., and Weiss, D. H. (eds). (2016). Remaking College: Innovation and the Liberal Arts College. Baltimore, MD: The Johns Hopkins University Press.
Corbett, A. C. (2005). Experiential learning within the process of opportunity identification and exploitation. Entrep. Theory Pract. 29, 473–491. doi: 10.1111/j.1540-6520.2005.00094.x
Costa, P. T., and McCrae, R. R. (1992). NEO-PI-R Professional Manual. Odessa, FL: Psychological Assessment Resources.
Cronbach, L. (1951). Coefficient alpha and the internal structure of tests. Psychometrika 16, 297–334. doi: 10.1016/0020-7489(93)90092-9
Davis, J. (2010). The First-Generation Student Experience: Implications for Campus Practice, and Strategies for Improving Persistence and Success. Sterling, VA: Stylus.
DeTienne, D. R., and Chandler, G. N. (2004). Opportunity identification and its role in the entrepreneurial classroom: a pedagogical approach and empirical test. Acad. Manag. Learn. Educ. 3, 242–257. doi: 10.5465/amle.2004.14242103
DeTienne, D. R., and Chandler, G. N. (2007). The role of gender in opportunity identification. Entrep. Theory Pract. 31, 365–386. doi: 10.1111/j.1540-6520.2007.00178.x
Diallo, T. M. O., Morin, A. J. S., and Lu, H. (2017). Impact of total and partial inclusion or exclusion of active and inactive time invariant covariates in growth mixture models. Psychol. Methods 22, 166–190. doi: 10.1037/met0000084
Dubner, S. J. (2011). The Upside of Quitting (No. 42). [Audio podcast episode]. In Freakonomics. Available online at: https://freakonomics.com/podcast/new-freakonomics-radio-podcast-the-upside-of-quitting/ (accessed September 30, 2011).
Duncan, T. E., Duncan, S. C., Strycker, L. A., Li, F., and Alpert, A. (1999). An Introduction to Latent Variable Growth Curve Modeling: Concepts, Issues, and Application. Mahwah, NJ: Lawrence Erlbaum Associates.
Fairlie, R. W. (2005). Entrepreneurship among disadvantaged groups: an analysis of the dynamics of self-employment by gender, race and education. Handb. Entrep. 2, 437–478. doi: 10.1007/978-0-387-32313-8_15
Gilmartin, S. K., Thompson, M. E., Morton, E., Jin, Q., Chen, H. L., Colby, A., et al. (2019). Entrepreneurial intent of engineering and business undergraduate students. J. Eng. Educ. 108, 316–336. doi: 10.1002/jee.20283
Gold, S. J. (2016). A critical race theory approach to Black American entrepreneurship. Ethn. Racial Stud. 39, 1697–1718. doi: 10.1080/01419870.2016.1159708
Gosling, S. D., Rentfrow, P. J., and Swann, W. (2003). A very brief measure of the Big Five personality domains. J. Res. Pers. 37, 504–528. doi: 10.1016/s0092-6566(03)00046-1
Grimm, K. J., Ram, N., and Estabrook, R. (2017). Growth Modeling: Structural Equation and Multilevel Modeling Approaches. New York, NY: Guilford Press.
Hall, R., and Lulich, J. (2021). University strategic plans: what they say about innovation. Innov. High. Educ. 46, 261–284. doi: 10.1007/s10755-020-09535-5
Hamilton, A. (2020). It’s About Damn Time: How to Turn Being Underestimated into Your Greatest Advantage. New York, NY: Penguing Random House.
Heinrich, B., Ludwig, P., Lewis, E., McCarthy, S., Swayne, N., and Louson, E. (2021). “Testing assessments of integrated learning,” in Applying Design Thinking to the Measurement of Experiential Learning, eds A. Peck and D. M. DeSawal (Hershey, PA: IGI Global).
Hipp, J. R., and Bauer, D. J. (2006). Local solutions in the estimation of growth mixture models. Psychol. Methods 11, 36–53. doi: 10.1037/1082-989X.11.1.36
Huang-Saad, A., and Celis, S. (2017). How student characteristics shape engineering pathways to entrepreneurship education. Int. J. Eng. Educ. 33, 527–537.
Johnson, M. D. (2005). Academic performance of transfer versus “native” students in natural resources and sciences. Coll. Stud. J. 39, 570–579.
Jung, T., and Wickrama, K. A. S. (2008). An introduction to latent class growth analysis and growth mixture modeling. Soc. Pers. Psychol. Compass 2, 302–317. doi: 10.1111/j.1751-9004.2007.00054.x
Kegan, R. (1994). In Over Our Heads: The Mental Demands of Modern Life. Cambridge, MA: Harvard University Press.
Kegan, R. (2009). “What “form” transforms”? A constructive-developmental approach to transformative learning,” in Contemporary Theories of Learning: Learning Theorists…In Their Own Words, ed. K. Illeris (New York, NY: Routledge), 35–52.
Kerr, S. P., Kerr, W. R., and Xu, T. (2017). Personality Traits of Entrepreneurs: A Review of Recent Literature (No. 24097; NBER Working Paper Series). Available online at: https://www.nber.org/system/files/working_papers/w24097/w24097.pdf (accessed September 25, 2021).
Lange, J. E., Marram, E., Jawahar, A. S., Yong, W., and Bygrave, W. (2011). Does an entrepreneurship education have lasting value? A study of careers of 4,000 alumni. Front. Entrep. Res. 31:209–224.
Langowitz, N., and Minniti, M. (2007). The entrepreneurial propensity of women. Entrep. Theory Pract. 31, 341–364. doi: 10.1111/j.1540-6520.2007.00177.x
Leutner, F., Ahmetoglu, G., Akhtar, R., and Chamorro-Premuzic, T. (2014). The relationship between the entrepreneurial personality and the Big Five personality traits. Pers. Individ. Dif. 63, 58–63. doi: 10.1016/j.paid.2014.01.042
Lo, Y., Mendell, N. R., and Rubin, D. B. (2001). Testing the number of components in a normal mixture. Biometrika 88, 767–778. doi: 10.1093/biomet/88.3.767
Loes, C. N. (2019). Applied learning through collaborative educational experiences. New Dir. High. Educ. 188, 13–21. doi: 10.1002/he.20341
Lukszo, C. M., and Hayes, H. (2020). Facilitating transfer student success: exploring sources of transfer student capital. Commun. Coll. Rev. 48, 31–54. doi: 10.1177/0091552119876017
Marques, C. S. E., Santos, G., Galvão, A., Mascarenhas, C., and Justino, E. (2018). Entrepreneurship education, gender and family background as antecedents on the entrepreneurial orientation of university students. Int. J. Innov. Sci. 10, 58–70. doi: 10.1108/IJIS-07-2017-0067
Mars, M. M., and Rhoades, G. (2012). Socially oriented student entrepreneurship: a study of student change agency in the academic capitalism context. J. High. Educ. 83, 435–459. doi: 10.1353/jhe.2012.0015
Martín, P. Potočnik, K., and Fras, A. B. (2017). Determinants of students’ innovation in higher education. Stud. High. Educ. 42, 1229–1243. doi: 10.1080/03075079.2015.1087993
Mason, C., and Arshed, N. (2013). Teaching entrepreneurship to university students through experiential learning: a case study. Ind. High. Educ. 27, 449–463. doi: 10.5367/ihe.2013.0180
Mayhew, M. J., Simonoff, J. S., Baumol, W. J., Wiesenfeld, B. M., and Klein, M. W. (2012). Exploring innovative entrepreneurship and its ties to higher educational experiences. Res. High. Educ. 53, 831–859. doi: 10.1007/s11162-012-9258-3
Mayhew, M. J., Simonoff, J. S., Baumol, W. J., Selznick, B., and Vassallo, S. (2016). Cultivating innovative entrepreneurs for the 21st century: a study of U.S. and German students. J. High. Educ. 87, 420–455. doi: 10.1353/jhe.2016.0014
Mayhew, M. J., Selznick, B. S., Zhang, L., Barnes, A. C., and Staples, B. A. (2019). Examining curricular approaches to developing undergraduates’ innovation capacities. J. High. Educ. 90, 563–584. doi: 10.1080/00221546.2018.1513307
Mayhew, M. J., Selznick, B. S., Zhang, L., Barnes, A., and Mangia, S. C. (2021). Teaching innovation capacities in undergraduate leadership courses: the influence of a short-term pedagogical intervention. J. High. Educ. 92, 877–896. doi: 10.1080/00221546.2021.1876480
McCarthy, S., Barnes, A., Holland, S. K., Lewis, E., Ludwig, P., and Swayne, N. (2018). “Making it: institutionalizing collaborative innovation in public higher education,” in Proceedings of the 4th International Conference on Higher Education Advances (HEAd’18), València, 1549–1557. doi: 10.4995/HEAd18.2018.8247
Moriano, J. A., Gorgievski, M., Laguna, M., Stephan, U., and Zarafshani, K. (2012). A cross-cultural approach to understanding entrepreneurial intention. J. Career Dev. 39, 162–185. doi: 10.1177/0894845310384481
Morris, M. H., Webb, J. W., Fu, J., and Singhal, S. (2013). A competency-based perspective on entrepreneurship education: conceptual and empirical insights. J. Small Bus. Manage. 51, 352–369. doi: 10.1111/jsbm.12023
Morris, M. H., Shirokova, G., and Tsukanova, T. (2017). Student entrepreneurship and the university ecosystem: a multi-country empirical exploration. Eur. J. Int. Manag. 11, 65–85. doi: 10.1504/EJIM.2017.081251
Muthén, B. (2004). “Latent variable analysis: growth mixture modeling and related techniques for longitudinal data,” in Handbook of Quantitative Methodology for the Social Sciences, ed. D. Kaplan (Newbury Park, CA: Sage Publications), 345–368.
Muthén, L. K., and Muthén, B. O. (1998/2017). Mplus User’s Guide, 8th Edn. Los Angeles, CA: Muthén & Muthén.
Nabi, G., Liñán, F., Fayolle, A., Krueger, N., and Walmsley, A. (2017). The impact of entrepreneurship education in higher education: a systematic review and research agenda. Acad. Manag. Learn. Educ. 16, 277–299. doi: 10.5465/amle.2015.0026
Newman, A., Obschonka, M., Schwarz, S., Cohen, M., and Nielsen, I. (2019). Entrepreneurial self-efficacy: a systematic review of the literature on its theoretical foundations, measurement, antecedents, and outcomes, and an agenda for future research. J. Vocat. Behav. 110, 403–419. doi: 10.1016/j.jvb.2018.05.012
Nylund, K. L., Asparouhov, T., and Muthén, B. (2007). Deciding on the number of classes in latent class analysis and growth mixture modeling: a Monte Carlo simulation study. Struct. Equ. Modeling 14, 535–569. doi: 10.1080/10705511.2014.882690
Nylund-Gibson, K., Grimm, R. P., and Masyn, K. E. (2019). Prediction from latent classes: a demonstration of different approaches to include distal outcomes in mixture models. Struct. Equ. Modeling 26, 967–985. doi: 10.1080/10705511.2019.1590146
Padilla-Angulo, L. (2019). Student associations and entrepreneurial intentions. Stud. High. Educ. 44, 45–58. doi: 10.1080/03075079.2017.1336215
Pascarella, E. T., Pierson, C. T., Wolniak, G. C., and Terenzini, P. T. (2004). First-generation college students: additional evidence on college experiences and outcomes. J. High. Educ. 75, 249–284. doi: 10.1080/00221546.2004.11772256
Pascarella, E. T., Wolniak, G. C., Seifert, T. A., Cruce, T. M., and Blaich, C. F. (2005). Liberal Arts Colleges and Liberal Arts Education: New Evidence on Impacts. San Francisco, CA: Jossey-Bass/ASHE.
Passaro, R., Quinto, I., and Thomas, A. (2018). The impact of higher education on entrepreneurial intention and human capital. J. Intellect. Cap. 19, 135–156. doi: 10.1108/JIC-04-2017-0056
Petras, H., and Masyn, K. (2010). “General growth mixture analysis with antecedents and consequences of change,” in Handbook of Quantitative Criminology, eds A. R. Piquero and D. Weisburd (New York, NY: Springer), 69–100. doi: 10.1007/978-0-387-77650-7_5
Pittaway, L., and Cope, J. (2007). Entrepreneurship education: a systematic review of the evidence. Int. Small Bus. J. 25, 479–510. doi: 10.1177/0266242607080656
Renn, K. A., and Arnold, K. D. (2003). Reconceptualizing research on college student peer culture. J. High. Educ. 74, 261–291. doi: 10.1353/jhe.2003.0025
Renn, K. A., and Reason, R. D. (2013). College Students in the United States: Characteristics, Experiences, and Outcomes. San Francisco, CA: Jossey-Bass.
Rindskopf, D., and Rose, T. (1988). Some theory and applications of confirmatory second-order factor analysis. Multivariate Behav. Res. 23, 51–67. doi: 10.1207/s15327906mbr2301_3
Rodriguez, J., Chen, H. L., Sheppard, S. D., Leifer, L., and Jin, Q. (2015). “Exploring the interest and intention of entrepreneurship in engineering alumni,” in Proceedings of the 122nd American Society for Engineering Education Annual Conference and Exposition, Seattle, WA, 26.738.1–26.738.22.
Schmitt-Rodermund, E. (2004). Pathways to successful entrepreneurship: Parenting, personality, early entrepreneurial competence, and interests. J. Vocat. Behav. 65, 498–518. doi: 10.1016/j.jvb.2003.10.007
Selznick, B. S. (2017). Higher Education for Undergraduate Innovation. New York, NY: New York University.
Selznick, B. S., and Mayhew, M. J. (2018). Measuring undergraduates’ innovation capacities. Res. High. Educ. 59, 744–764. doi: 10.1007/s11162-017-9486-7
Selznick, B. S., and Mayhew, M. J. (2019). Developing first-year students’ innovation capacities. Rev. High. Educ. 42, 1607–1634. doi: 10.1353/rhe.2019.0077
Selznick, B., and McCarthy, S. (2020). “Toward a theory of social innovation for higher education,” in Philosophy and Theory in Higher Education, Vol. 2, ed. A. J. Fulford (Bern: Peter Lang), 49–69. doi: 10.3726/PTIHE032020.0004
Selznick, B. S., Dahl, L. S., Youngerman, E., and Mayhew, M. J. (2021a). Equitably linking integrative learning and students’ innovation capacities. Innov. High. Educ. 47, 1–21. doi: 10.1007/s10755-021-09570-w
Selznick, B. S., Mayhew, M. J., Zhang, L., and McChesney, E. (2021b). Creating an organizational culture in support of innovation education: a Canadian case study. J. Coll. Stud. Dev. 62, 219–235. doi: 10.1353/csd.2021.0018
Shinnar, R. S., Giacomin, O., and Janssen, F. (2012). Entrepreneurial perceptions and intentions: the role of gender and culture. Entrep. Theory Pract. 36, 465–493. doi: 10.1111/j.1540-6520.2012.00509.x
Shiyko, M. P., Ram, N., and Grimm, K. J. (2012). “An overview of growth mixture modeling: a simple nonlinear application in OpenMx,” in Handbook of Structural Equation Modeling, ed. R. H. Hoyle (New York, NY: Guilford Press), 532–546.
Swayne, N., Lewis, E., McCarthy, S., Ludwig, P., and Selznick, B. (2021). “Identifying and cultivating a transdisciplinary faculty,” in Session Presented at the Annual Meeting of the Association of American Colleges and Universities (AAC&U), Seattle, WA.
Swayne, N., Selznick, B. S., McCarthy, S., and Fisher, K. A. (2019). Uncoupling innovation and entrepreneurship to improve undergraduate learning. J. Small Bus. Enterp. Dev. 26, 783–796. doi: 10.1108/JSBED-04-2019-0122
Tate, K. A., Caperton, W., Kaiser, D., Pruitt, N. T., White, H., and Hall, E. (2015). An exploration of first-generation college students’ career development beliefs and experiences. J. Career Dev. 42, 294–310. doi: 10.1177/0894845314565025
Taylor, B. J., and Cantwell, B. (2019). Unequal Higher Education: Wealth, Status, and Student Opportunity. New Brunswick, NJ: Rutgars University Press.
Thompson, M. (2018). Social capital, innovation and economic growth. J. Behav. Exp. Econ. 73, 46–52. doi: 10.1016/j.socec.2018.01.005
Trolian, T. L., and Jach, E. A. (eds) (2019). Applied Learning in Higher Education: Curricular and Co-curricular Experiences that Improve Student Learning. New Directions for Higher Education No. 188. San Francisco, CA: Jossey-Bass.
Vermunt, J. K. (2010). Latent class modeling with covariates: two improved three-step approaches. Polit. Anal. 18, 450–469. doi: 10.1093/pan/mpq025
Wagner, T. (2012). Creating Innovators: The Making of Young People Who Will Change the World. New York, NY: Scribner.
Walker, J. E. (2009). The history of Black business in America: Capitalism, race, entrepreneurship, 2nd Edn, Vol. 1. Chapel Hill, NC: UNC Press Books.
Wilson, F., Kickul, J., and Marlino, D. (2007). Gender, entrepreneurial self-efficacy, and entrepreneurial career intentions: implications for entrepreneurship education. Entrep. Theory Pract. 31, 387–406. doi: 10.1111/j.1540-6520.2007.00179.x
Wingfield, A. H., and Taylor, T. (2016). Race, gender, and class in entrepreneurship: intersectional counterframes and Black business owners. Ethn. Racial Stud. 39, 1676–1696. doi: 10.1080/01419870.2016.1178789
Winks, L., Green, N., and Dyer, S. (2020). Nurturing innovation and creativity in educational practice: principles for supporting faculty peer learning through campus design. High. Educ. 80, 119–135. doi: 10.1007/s10734-019-00468-3
Wothke, W. (2000). “Longitudinal and multigroup modeling with missing data,” in Modeling Longitudinal and Multilevel Data: Practical Issues, Applied Approaches, and Specific Examples, eds T. D. Little, K. U. Schnabel, and J. Baumert (Mahwah, NJ: Lawrence Erlbaum Associates Publishers), 219–240,269–281. doi: 10.1007/s11136-014-0746-0
Wyllie, J. (2018). College Have Spent Big Money on Innovation Centers. Do they Work? The Chronicle of Higher Education. Available online at: https://www.chronicle.com/article/Colleges-Have-Spent-Big-Money/242992 (accessed March 30, 2018).
Keywords: innovation, growth mixture modeling (GMM), longitudinal, student environment, human capital - skills, higher education
Citation: Selznick BS, Mayhew MJ, Winkler CE and McChesney ET (2022) Developing Innovators: A Longitudinal Analysis Over Four College Years. Front. Educ. 7:854436. doi: 10.3389/feduc.2022.854436
Received: 14 January 2022; Accepted: 11 March 2022;
Published: 09 May 2022.
Edited by:
Mona Hmoud AlSheikh, Imam Abdulrahman Bin Faisal University, Saudi ArabiaReviewed by:
Fulvia Pennoni, University of Milano-Bicocca, ItalyCopyright © 2022 Selznick, Mayhew, Winkler and McChesney. This is an open-access article distributed under the terms of the Creative Commons Attribution License (CC BY). The use, distribution or reproduction in other forums is permitted, provided the original author(s) and the copyright owner(s) are credited and that the original publication in this journal is cited, in accordance with accepted academic practice. No use, distribution or reproduction is permitted which does not comply with these terms.
*Correspondence: Benjamin S. Selznick, c2Vsem5pYnNAam11LmVkdQ==
Disclaimer: All claims expressed in this article are solely those of the authors and do not necessarily represent those of their affiliated organizations, or those of the publisher, the editors and the reviewers. Any product that may be evaluated in this article or claim that may be made by its manufacturer is not guaranteed or endorsed by the publisher.
Research integrity at Frontiers
Learn more about the work of our research integrity team to safeguard the quality of each article we publish.