- Center for Communication, Media and Information Technologies, Department of Electronic Systems, Aalborg University, Aalborg, Denmark
Faculty members are crucial to Blended Learning’s success in higher education. Despite substantial research into the elements that drive faculty adoption of BL, few have developed a model to explain how these factors combine and influence faculty intentions to teach in this mode. This study used data collected from 207 professors from 18 universities across Africa, the United States, Europe, and the Middle East to test and validate a Faculty Blended Learning Adoption Model which was derived from a Grounded Theory study. Four model constructs (institutional hygiene readiness, student BL disposition, faculty technology ready, and Pedagogy Technology Fit for BL) mediated by motivation were tested to predict faculty Blended Learning adoption using structural equation modeling. The results demonstrated an excellent model fit, with three of the six hypotheses in this study being supported. Faculty desire to utilize BL was found to be influenced by faculty technology readiness and task technology fit for BL, but not by institutional hygiene readiness or student BL disposition. This research presents a useful model for university administrators to use in their BL implementations. A thorough understanding of this model can assist decision-makers in identifying the factors that influence future faculty acceptance or resistance to blended learning, as well as helping them in enhancing acceptance and usage.
Introduction
The difficulty that faculty members have had in adopting Blended Learning (BL) in Higher Education Institutions (HEIs) has been thoroughly researched for many years (Callo and Yazon, 2020). In Ghana and elsewhere (Adarkwah, 2021), it is frequently asserted that BL has the ability to change academia and become the new normal for teaching and learning (Blieck et al., 2020). While this is so, faculty members are very slow in adopting BL implemented within the universities (Martin et al., 2019b). The key role faculty members play in the failure or successes in its implementation is well documented (Martin et al., 2019b). It is this concern over the failure of faculty members to adopt BL for teaching and learning that has generated the copious literature on the challenges of BL adoption (Aboagye et al., 2020). The ability to thrive in teaching in BL mode environment requires instructors to go through a learning curve, thus simply being a great teacher in a traditional face-to-face classroom is not enough (Albrahim, 2020). Only a few teacher education programs focus on the skills, methodologies, and techniques required for online teaching (Archambault and Larson, 2015; Rahmawati et al., 2021). As a result, many faculty members lack both theoretical and practical understanding of teaching and learning online (Adiyarta et al., 2018).
For this article, the journey to understand faculty BL adoption begun when the management of a public institution X in Ghana decided to update faculty teaching requirements to include having the competencies to teach in blended mode through acquiring BL certification at the university’s center for online learning and teaching. This led to an inquiry to investigate the barriers of faculty BL adoption after a management report detailed faculty reluctance to teach online despite considerable investment made in the acquisition of a learning management system (Moodle) and the retooling of the curriculum to accommodate BL. Additionally, the author wondered what students’ experience of BL was in the wake of the low faculty adoption, and thus conducted a GT using the experiences of the faculty members teaching BL to develop a faculty blended learning adoption model. Whiles most traditional HEI programs require an integration of technology into teaching and learning, there has been a less than commensurate effort on the part of management to ensure faculty members acquired the requisite competencies required for BL teaching and learning (Anthony Jnr, 2021). To this extent, there is minimal understanding of the elements that guide faculty preparation toward implementing a campus wide blended learning environment (Graham et al., 2019).
The purpose of this study is to test and validate the Faculty Blended Learning Adoption Model (FBLAM; Antwi-Boampong, 2020) and to explain the factors that motivate faculty toward adoption of BL. The constructs contained in this model were drawn from an exploratory Grounded Theory study Antwi-Boampong (2020) that modeled the lived experiences of faculty members’ BL adoption. According to the findings, teachers’ knowledge and abilities may be better understood, allowing for more targeted and customized professional development opportunities to better prepare educators for teaching in mixed contexts (Graham et al., 2019). The principal question this article asks is: what is the effect of the predictors of the constructs of FBLAM mediated by motivation on BL adoption within the HEI? The paper begins with an account of the antecedents of the FBLAM, then moves on to discuss the constructs empirically before discussing the formulation of the hypothesis. Thereafter, the methodology and the findings for the study are presented. We conclude by arguing for adopting the FBLAM as an empirically tested and validated model for BL adoption by HEIs.
Literature Review
To get a better understanding of the elements that impact faculty BL adoption, previous literature looked at BL research in the context of models that have shown promise in predicting faculty adoption.
Institutional Hygiene Readiness
A framework has been created to assist higher education institutions in making the move to improved blended learning. The suggested framework by Adekola et al. (2017) tackles the why (change agents), what (institutional concerns), how (organizational readiness), and who (stakeholders) of improved blended learning transitions. A successful institutional shift into improved blended learning necessitates the participation of all stakeholder groups (Adekola et al., 2017). Supportive factors, attitude, learning style, contentment, course management, and simplicity of use all positively impact learners’ and academic staffs’ perceptions of BL adoption. Similarly, studies show that faculty attitude toward BL adoption is influenced positively by strategy, structure, and support factors (Anthony et al., 2019). The findings support higher education institutions to plan and initiate BL policies. Anthony Jnr (2021) provides insights on BL from an institutional theory perspective. It was discovered that faculty members’ adoption of BL is strongly impacted by coercive, normative, and mimetic influences. In addition, the research highlighted institutional initiatives that have an impact on BL implementation. Institutional hygiene readiness represents the preparedness of the institution toward providing an environment that is conducive enough to motivate faculty to want to use BL for teaching and learning. This is confirmed in previous research (Machado, 2007; Wong et al., 2014; Rahmawati et al., 2021). The following primary hypotheses is tested in the current study, which is based on empirical data across a variety of jurisdictions.
Ha1 – IHR significantly influences BL adoption.
Ha2 – IHR significantly influences BL adoption mediated by motivation.
Faculty Technology Readiness
This construct describes a set of implicit factors that are primarily related to the personal attributes of the faculty members needed as pre-requisite for BL delivery. These include the technological competences of faculty members which are requisites for instructional design and delivery of BL contents. According to Mercado (2008),Cutri and Mena (2020), and Legaspi et al. (2021) several colleges use a readiness technique to assess faculty technology readiness to teach online, however, the majority have not been properly investigated or experimentally evaluated. Only a few studies have investigated whether faculty members are ready to teach online. Junus et al. (2021) looked at how online instructors’ e-learning readiness was assessed before, during, and after the course was delivered. They discovered that online instructors had a pressing need for online support desk services. Faculty members do not feel well prepared to teach online (Martin et al., 2019a,c). However, identifying competences to equip faculty to teach online remains a priority, and by doing so, we will be able to provide recommendations on how to teach in BL mode. Callo and Yazon (2020) looked at teacher educators’ readiness and preparation for, as well as their perspectives of preservice teacher preparation. Martin et al. (2019b) define faculty readiness to teach online “as a state of faculty preparation for online Teaching.” In the context of this study, we are particularly interested in two elements of readiness: (1) faculty attitudes toward the relevance of online teaching, and (2) faculty views of their technological competence to teach online confidently. Faculty Technology-Readiness refers to an individual’s readiness to make use of new technology in the course of their work (Parasuraman, 2000; Cutri and Mena, 2020). Thus, the following hypotheses are proposed:
Ha3 – Faculty technology readiness significantly influences BL adoption.
Ha4 – Faculty technology readiness significantly influences BL adoption mediated by motivation.
Student’s Disposition to Accept Blended Learning
As opposed to teaching presence in non-BL situations, it is the student disposition that has a bigger effect over teaching presence in BL environments (Sangwan et al., 2021). Comparing web-based technologies to conventional classroom learning, despite their extensive use, web-based technologies still confront the difficulty of not being readily accepted when presented into a new application scenario (Adarkwah, 2021). Individual differences exist in students’ dispositions and preparedness to embrace and use web-based learning tools, as well (Geng et al., 2019). During the learning situations, students’ attitudes about technology-based applications reflect their level of technological preparedness (Legaspi et al., 2021). Cheon et al. (2012) discovered that college students’ attitudes about mobile learning had a favorable impact on their intention to use mobile learning. In the FBLAM setting, a good attitude about using online learning resources among students will encourage faculty to teach in BL mode and achieve the desired learning outcome (Antwi-Boampong, 2020; Al-Ayed and Al-Tit, 2021).
It has already been stated that the usage of learning technologies has a variety of effects on students’ learning outcomes, with some of these effects being produced by contextual and cognitive variables and others being driven by technological factors alone (Hong et al., 2014; Sangwan et al., 2021). In science education, it has been discovered that a BL atmosphere improves student attendance and learning pleasure (Tang, 2013). Students’ intellectual development can also be enhanced by utilizing online course materials (Teo et al., 2019). Students’ views and behaviors are influenced by a variety of factors, which is why it is important to investigate their preparedness for learning technologies as well as their impacts on their perceptions and behaviors. According to Parasuraman (2000) and Tubaishat and Lansari (2011), a measurement scale for technology readiness was developed and validated, and it consisted of 28 items that were divided into four categories: optimism, innovativeness, discomfort, and insecurity. Parasuraman (2000) found that the Technology Readiness Index (TRI) was effective in identifying individuals who were technologically ready. Each of these four categories reflects the individual’s attitude toward new technology in the context of the learning process in its whole (Parasuraman, 2000). The following hypotheses are proposed:
Ha5 – Student disposition to adopt BL significantly influences BL adoption.
Ha6 – Student disposition to adopt BL significantly influences BL adoption mediated by motivation.
Pedagogy-Technology-Fit
This construct is operationalized through dimensions of fit that consider: (1) the underpinning ontology of the domain, (2) the purpose of the task that the representation is meant to support, (3) how best to support the cognitive processes of the users of the representations, (4) users’ differing needs and preferences, and (5) the tool and environment in which the representations are constructed and manipulated (Masterman and Craft, 2013). To explore the extent to which it is possible to describe all the parts of a domain being described, as well as the connections between them, for problem-solving purposes, Masterman and Craft (2013) uses the phrase “ontology-fit.” Faculty technology readiness to teach online is defined as the level of faculty preparedness for online teaching because of technological advances. Specifically, in the context of this study, we will be focusing on two elements of preparedness: (1) faculty attitude on the importance of online teaching and (2) faculty competences and implicit ability to confidently teach online using technology. The hypothesized relationship of P-T-F is drawn from related literature and discussed below. The FBLAM proposes in line with similar studies (Okojie et al., 2006; Dennehy et al., 2016) that the P-T-F has a positive influence on faculty motivation to adopt BL (that is the better the pedagogy used for teaching fits or is compatible with the technology employed to deliver BL courses, the more positive the anticipation that faculty would teach in blended mode). In the context of BL, the anticipated benefits would include faculty being able to deliver courses to students more easily, quickly to improve their learning outcomes.
There have been several models in the literature developed to help organizations understand how individuals make use of technology (Susanto et al., 2020). A model for task–technology fit was created by Goodhue and Thompson (1995) to predict performance, with the idea of task–technology fit serving as a predictor. It has been studied what influence task–technology fit has on various domains by employing various elements of the Technology to Performance Chain (TPC). For the first time, McGill and Klobas (2009) used the technology to performance chain as a framework to investigate how task–technology fit affects the performance impacts of learning Management Systems (LMSs). Several findings (Fathema et al., 2015; Junus et al., 2021) offered significant evidence for the relevance of task–technology fit, which affected perceived impact on learning both directly and indirectly by the degree to which it was utilized. The role of pedagogy–technology fit in the BL domain has not yet been thoroughly studied (Dennehy et al., 2016). As a result, given the need for thorough study into the variables that affect faculty BL adoption, determining the relevance of the PTF might be a useful construct for determining the elements that drive faculty BL adoption. Based on the empirical findings the current study tests the following principal hypotheses:
Ha7 – PTF significantly influences BL adoption.
Ha8 – PTF significantly influences BL adoption mediated by motivation.
Teaching Motivation
According to the findings of faculty adoption research (Martin, 2010; Reeve, 2015; Garrote and Pettersson, 2016), motivation is a critical component in both online and in-class learning environments. Teaching motivation is the process through which goal-directed action is started and sustained, and it is represented in personal involvement as well as cognitive, emotional, and behavioral engagement in learning activities (Chen and Jang, 2010; Nikou and Economides, 2017). According to Hoffman (2013) both extrinsic and intrinsic variables are associated with faculty members’ desire to participate in online education in a favorable and statistically significant way. Some intrinsic variables include a faculty member’s opinions about the effectiveness of online education and their desire to broaden student access to higher education opportunities (Hartnett, 2016). A faculty member’s willingness to participate in online education was found to be the most important factor in their willingness to teach online courses (Sørebø et al., 2009). Intrinsic factors, including belief in the efficacy of online education and desire to increase student access to education, were found to have the strongest impact (Hoffman, 2013). Although much educational research (Pereira and Figueiredo, 2010; Gautreau, 2011; Maldonado et al., 2011) emphasizes on motivation, the effect of pedagogy-technology-fit, students BL disposition, institutional hygiene readiness and faculty technology readiness have not been explored in the blended learning setting (Antwi-Boampong, 2020).
The FBLAM (Antwi-Boampong, 2020), as illustrated in Figure 1, which presents hypothesized relationships between pedagogy-technology-fit, students BL disposition, institutional hygiene readiness and faculty technology readiness in predicting faculty adoption is tested.
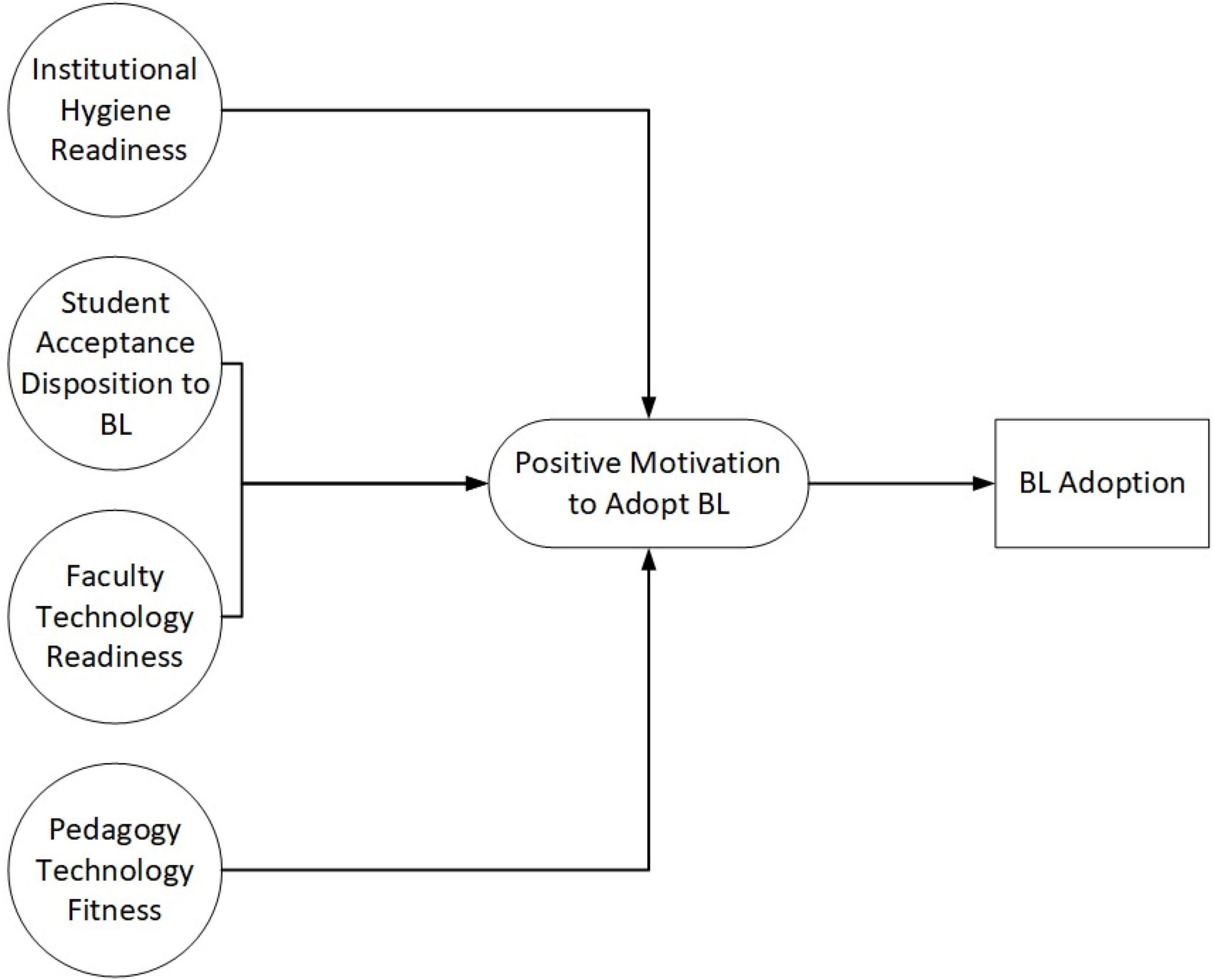
Figure 1. Faculty blended learning adoption model [adopted from Antwi-Boampong (2020)].
Table 1 presents the model constructs and descriptions that explains them.
Methodology
Design and Participants
The study used a correlational study design, which is consistent with previous studies undertaken to assess the BL adoption (Lin and Wang, 2012). MacDonald and Reid (2013) proposed a sample size determination-based chi-square for specified confidence interval at 1 degree of freedom on a population (N) of respondents. At a significance level of 5%, a closed ended questionnaire was administered to a sample of 207 from a population of 500 university lecturers and faculty administers and officers who have between 1 and 10 years’ experience in BL across 19 universities in six countries (Ghana, Namibia, Dubai, United States, Denmark, and Kuwait). Only lecturers and faculty members who been part and have experienced BL approach between 1 and 10 years met the selection criteria. The questionnaire was used to test the hypothesized model. Nearly all participants had taught courses in Science, Arts, Education, Engineering, Management, and other related courses. The mean length of teaching among participants was 8.5 years with a standard deviation of 0.7 years.
Data Analysis
Principal Component analysis and Structural equation modeling (SEM) approach were used as tools for data analysis using multivariate statistical approach of partial least squares. Sarstedt et al. (2014) confirmed that this technique is normally recommended when the aim is to confirm a theory from the constructs and the sample size is somewhat small. All constructs of the measurement model in Figure 1 as well as the mediation are based on the theoretical survey and can be categorized as reflective (Hair et al., 2012). For such measurement models, we use constructs validity (discriminate validity and convergent validity) and reliability analysis (Sarstedt et al., 2014). The reliability of internal consistency was analyzed by composite reliability and Cronbach’s Alpha, and both exceeded the threshold of 0.7 (Sarstedt et al., 2014). To establish how well the collected data measures the construct of the study, psychometric properties, including Principal Component Analysis (PCA), reliability and confirmatory factor analysis (CFA) tests were carried out, as proposed by Anderson and Gerbin (1998). Principal component analysis with Varimax rotation was also carried out to remove statements that did not significantly contribute to the constructs as such all constructs with underlying statements entered the PCA.
Data Analysis
Introduction
The section presents and discusses the results in line with the hypothesized model. Six constructs with 26 items were used as independent variables to predict BL adoption mediated by motivation. Prior to that, descriptive statistics were used to measure the weight for each of the constructs based on the Likert scale. This was then followed by a path analysis (confirmatory factor analysis). The results from the path analysis were presented and passed through PCA to remove the items which could not load more than 0.5. Cronbach’s Alpha was used to further confirm the results and a re-specified model was developed and tested. Composite Reliability and Average Variance Extracted were used to further test the reliability and validity of the constructs, respectively. A path analysis was conducted for the re-specified model with model fitted test to demonstrate the rigorousness of the model. Finally, the results from the test were discussed and validated in the literature with the conclusions and implication of the study clearly elucidated.
Presentation of Results
Demographic Information
Table 2 illustrates the distribution of the institutions or universities of the respondents. Out of the total number of respondents, 37.7% represented respondents from the University of Namibia, 18.4% from the Ghana Communication Technology University and 9.7% represented The University of North Carolina at Chapel Hill. The Methodist University, University for Development Studies, and the University of Cape Coast had 4.3% responses. Aalborg University and University of Chicago both had 2.9 responses. All other universities had a total of 1.4%. The implication is that three times as many foreign universities participated in the survey as did local universities.
Table 3 below illustrates the teaching experience of the respondents from the surveyed universities. Out of the total number of respondents from the universities, 37.7% had 1–5 years of experience, 23.2% had taught between 6 and 10 years, 21.7% had 11–15 years teaching experience, 11.6% had taught from 16 to 20 years, whilst 5.8% had 20+ years teaching experience. The implication is that majority of the teachers had between 1 and 10 years teaching experience.
Table 4 shows a cross tabulation of the number of years’ respondents had in teaching courses using blended learning approach. The category of 1–5 years had majority of lecturers (74%) with BL experience who had taught between 1 and 20 years cumulatively. The other 26% of lecturers had BL experience between 6 and 20 years with same teaching experience. This finding suggests most lecturers acquired the BL experience in this recent past 5 years, indicating the level of adoption of BL in these universities studied in this last 5 years.
Descriptive Analysis
The weighted mean and standard deviation for Institutional Hygiene Readiness for BL adoption accounted for 2.36 and 1.13, respectively (with a 5-point Likert scale 1-Strongly Agree, 2-Agree, 3-Neutral, 4-Disagree, and 5-Strongly Disagree). The mean shows that averagely all respondents agreed that Institutional Hygiene Readiness contributes to BL adoption. The standard deviation shows the level of dispersion of respondents’ views with regards to Institutional Hygiene Readiness to the adoption of BL (see Table 5). The standard deviation of 1.13 shows a fair spread of the other views around the mean. The implication is that majority of the respondents agreed that Institutional Hygiene Readiness contributes significantly to BL adoption. Also, the weighted mean and standard deviation for Student Acceptance Disposition of BL adoption accounted for 2.16 and 0.92, respectively (see Table 5). The mean shows that averagely all respondents agreed that Student Acceptance Disposition of BL is a key construct which contributes to BL adoption. The standard deviation shows a good estimation of the mean indicating that very few people have diverse opinions of the contribution of the construct to BL adoption. Faculty Technology Readiness of BL adoption accounted for 2.27 and 0.99 on the weighted mean and standard deviation, respectively (see Table 5). The mean shows that averagely all respondents agreed that Faculty Technology Readiness contributes largely to BL adoption. The standard deviation shows a good estimation of the mean. The weighted mean and standard deviation for Pedagogy Technology Fit of BL adoption accounted for 2.18 and 0.99, respectively (see Table 5). The mean shows that averagely all respondents agreed that Pedagogy Technology Fit of BL contributes largely to BL adoption. The standard deviation shows a good estimation of the mean, depicting very few people had diverse opinions of the contributions of the Pedagogy Technology Fit to BL adoption. Motivation to Adoption Blended Learning of BL adoption accounted for 2.53 and 1.01, respectively, for mean and standard deviation (see Table 5). The mean shows that averagely all respondents agreed that Motivation to adopt BL significantly affects BL adoption.
Measurement of Constructs
The PCA was used to prune the variables in the hypothesized model to measurable constructs. This was to ensure that a rigorous process of measuring the model constructs was achieved. First a confirmatory factor analysis was conducted based on the hypothesized model. Then constructs with factor loadings less than 0.5 were removed, and further confirmed using Cronbach’s Alpha. Those constructs which passed the threshold of 0.7 were regrouped and a new model re-specified with the regrouped variables. The re-specified model was further tested using the path analysis and confirmed with the goodness of fit.
Hypothesized Model
Figure 2 shows the hypothesized model showing the constructs for the independent variables, mediating variables and the dependent variables. The results of the hypothesized model revealed that Institutional Hygiene and Readiness, Student Acceptance Disposition, Pedagogy Technology Fit could not predict or explain BL adoption directly (see Table 6) except Faculty Technology Readiness which predicted BL adoption (see Table 6). However, Institutional Hygiene and Readiness and Student Acceptance Disposition could not predict the mediating for (Motivation). But Faculty Technology Readiness and Pedagogy Technology could explain or predict the mediating factor (Motivation). As a result of these findings the study further conducted a PCA, Component Reliability and Average Variance Extracted test to ensure accuracy of the items measuring the constructs. A re-specified model was developed at the end of the test (see Table 7 and Figure 3).
Principal Component Analysis: KMO, Bartlett’s Test, Reliability and Validity
All constructs passed the Bartlett’s test of sphericity with an average 0.01 (α < 0.05) and Kaiser-Meyer-Olkin (KMO) average score of 0.762 was achieved which indicates suitability of the sample for factor analysis (see Table 7). As Hair et al. (2012) clarified, a factor loading scores higher than 0.5 for all items stated the satisfactory explanations of the item. Majority of the constructs (except two) had factor loadings greater 0.5. Constructs with factor loadings less than 0.5 were removed from the item list. The analysis reconfirmed twenty-six factors with a cumulative weighted average total variance explained of 67% (see Table 7). The twenty-six items were rotated in the Rotated Component Matrix and results highlighted (see Table 7). From the Table 7 below, results show a different grouping of items, measuring the construct and these new item groupings were further tested with Cronbach’s Alpha. The Cronbach’s Alpha showed an average Alpha value of 0.812 which is greater than the threshold of 0.7 as postulated by Hair et al. (2012), demonstrating the consistency in the responses elicited from the respondents.
A Composite Reliability score of 0.85 was generated; indicating the internal consistency of six (6) constructs with twenty-six rotated items using Microsoft Excel to compute the CR score. The Average Variance Extracted score of 0.62 was also accounted, which was higher than 0.5 recommended by Hair et al. (2012) indicating convergence validity. Likewise, the value of AVE below 0.5, showed the degree to which the operationalization of a construct dissimilar, confirming the existence of discriminate validity. From the results obtained, these requirements were not violated as indicated by Hair et al. (2012).
Re-specified Model
The re-specified model (see Figure 3) revealed that Faculty Technology Readiness contributed 29% at a significant level of 0.05 on BL Adoption in the universities studied remained as the only construct that directly predicted BL Adoption without any mediation (see Table 8). Pedagogy Technology Fit could not predict BL Adoption but could predict BL adoption when mediated by Motivation for BL Adoption (see Table 8). Motivation for BL Adoption remained a direct predictor of BL Adoption as indicated earlier in the hypothesized model (see Table 8).
Generally, a goodness-of-fit test is a measure of how well observed data correspond to the fitted model. Kenny et al. (2015) noted that goodness-of-fit refers to how a hypothesized model reproduces the multivariate structure of a given set of the data. A goodness-of-fit index allows a researcher to claim that the model is a good one or that a mis-specified model is not necessarily a bad model.
The study examined the measurement model’s goodness-of-fit based on research objectives and hypothesized model (Little et al., 2002). The model was re-specified to reduce complexity and likelihood of unwanted interaction and effects between the variables (Taylor and Mackinnon, 2008).
The study reported multiple fit indices as appropriate (Cabrera-Nguyen, 2010) to be able to make a claim of its model fit. The fit indices demonstrate the level of rigorousness of the model after re-specification. Even though some constructs did not exceed the threshold mark, however, there was some level of improvement in the fit indices (see Table 9).
Baron and Kenny (1986) identified a direct effect (full mediation) and a case of both direct and indirect effects (partial mediation), which was confirmed by Zhao et al. (2010). In this study, both full and partial mediation were observed where Motivation to Adoption BL mediates between the independent constructs (Faculty Technology Readiness and Pedagogy Technology Fit) and BL Adoption. While a full mediation (direct effect) was observed between Faculty Technology Readiness and BL Adoption, a partial-mediated effect was observed between Pedagogy Technology Fit and BL Adoption. The study observes a total effect of 22% each for both Faculty Technology Readiness and Pedagogy Technology Fit on BL Adoption (see Table 10). The implication is that the factors (Faculty Technology Readiness and Pedagogy Technology Fit) that contribute to BL Adoption in the universities studied positively contribute 22% in total.
Discussion of Results
The aim of this study was to test and validate the FBLAM and to explain the constructs that influence faculty to adopt BL. Overall, the findings in this study show empirical support for the hypothesis that the four independent constructs can predict the effects on faculty motivation to adopt BL. As a result of these findings, the proposed model appears to be a good match in that it provides an acceptable description of the interactions among the elements that impacted faculty intentions to utilize BL for teaching and learning.
From the results of the hypothesized model, institutional hygiene readiness and student acceptance disposition to accept BL could not predict or contribute directly to faculty motivation. Whereas studies (Porter et al., 2014; Mestan, 2019) suggest that institutional readiness and students disposition to BL are relevant factors to successful adoption of BL what could account for these constructs not finding predictive support from the model could be the case that respondents are from institutions with mature implementation/growth stages of BL implementations (Graham et al., 2013). The result is that most respondents are confident in their abilities and understand the importance of BL learning in the process of facilitating the learning experience for their pupils. The institutional structures and processes for faculty members are being supported in this respect to establish the most effective and suitable approaches to integrate BL throughout the Universities.
However, in the re-specified model, faculty technology readiness and pedagogy-technology-fit had direct influence on faculty motivation to adopt BL. These are consistent with current research (Goodhue and Thompson, 1995; McGill and Klobas, 2009; Archibald et al., 2021). From the direct influences of faculty technology readiness and its positive effect on motivation to adopt BL, it is useful to infer that when faculty members have the implicit technology competences to design and use instructional technology, they have positive feelings to teach in BL mode. These feelings according to Teo (2011) reinforce faculty motivation to use technology. Pedagogic Technology Fit had a direct influence on motivation of faculty to adopt BL (i.e., β = 0.66, α-value = 0.01). This affirms the fact that the pedagogy used must have positive significance within the domain in which the instruction activities are conducted using technology as a medium to promote the teaching and learning (Lee et al., 2017). Consequently, there is a practical implication for HEIs to provide continuous training and development to equip faculty in their teaching experiences.
Poor pedagogy technology fit discourages some instructors from using the LMS (Martin et al., 2019a). When teachers perceive that the instructional method can adequately fit the medium (technology) being employed this can strengthen their intention to adopt the technology in this case (LMS) as the domain to teach in BL mode (Martin et al., 2019b). Similarly, motivation had a direct influence of faculty BL adoption. This is consistent with studies by Gautreau (2011) and Ibrahim and Nat (2019). As a result of the validation of the motivation as an essential mediating element for BL implementation, both the extrinsic and intrinsic motivational variables have a significant beneficial impact on instructors’ motivation to adopt BL in higher education institutions. Furthermore, it is critical for every higher education institution to assess their instructors’ motivation about any kind of technology before considering its ultimate adoption. This model can serve as a foundation in this regard, and higher education institutions are free to investigate and include any other factors that they believe would increase their instructors’ motivation in the BL setting (Ibrahim and Nat, 2019). Again, there is a policy implication for this finding. The finding affirms that higher education institutions readiness and support have provided diverse motivation to utilize technology to teach. Hence, institutions must enforce the policy to enhance the effective usage of the technology that can promote quality teaching and learning within the blended learning environment.
Conclusion
Results of our study indicate the effects of institutional hygiene preparedness, faculty technology readiness, student disposition to BL and pedagogic technology fit, which are mediated by motivation, on the faculty’s willingness to embrace BL. This work contributes to the body of knowledge about blended learning and the elements that influence it, which has not been adequately examined previously. By examining the effects of these separate variables on faculty adoption, our research provides empirical data and insights for educators to better understand faculty adoption of BL to improve teaching and learning, ultimately leading to higher learning outcomes. The findings of this investigation indicate that the suggested model provides a satisfactory match to the data set under consideration. Future study might examine if the model is invariant across different personal (e.g., gender, computer experience), organizational, and technical variables, among other things. It may be possible to discover the culture-invariant characteristics that impact teachers’ intentions to employ technology through comparative research across nations or cultures.
Recommendation
The literature gaps identified in this study suggests the lack of operationalized model for faculty BL adoption in the Ghanaian Higher Institution of Learning. It is recommended that the hypothesized and tested model extended to include other variables to explain other relevant issues mitigating the adoption and implementation of BL in higher institutions in Ghana. The findings present deep insights to guide policy implementation on BL adoption among the universities in Ghana and beyond. Further research work can investigate the factors that accounted for non-predictability of Institutional Hygiene Readiness among others to guide managers and administrators of higher institution of learning to make informed decisions.
Limitations of the Study
It became clear to us over the course of doing this research that the area of blended learning research is still in its early stages (Graham et al., 2019). There should be a greater emphasis on the creation of models and theories that may be used to guide practice in the future (Graham et al., 2014). Finding blended learning models that were supported by current measurement methodologies proved to be difficult, which was disappointing. In this case, it is possible that only instructors who were comfortable with technology responded to the online questionnaire, which might have resulted in a lack of inclusiveness among the teachers at each school, impairing the study’s ability to generalize its findings. Future studies should consider including pen and paper data collection into data collection strategy in addition to online data collection to make it more inclusive. Those who prefer to answer on paper or who have limited computer access will be able to take use of this alternative.
Data Availability Statement
The original contributions presented in the study are included in the article/supplementary material, further inquiries can be directed to the corresponding author.
Author Contributions
The author confirms being the sole contributor of this work and has approved it for publication.
Conflict of Interest
The author declares that the research was conducted in the absence of any commercial or financial relationships that could be construed as a potential conflict of interest.
Publisher’s Note
All claims expressed in this article are solely those of the authors and do not necessarily represent those of their affiliated organizations, or those of the publisher, the editors and the reviewers. Any product that may be evaluated in this article, or claim that may be made by its manufacturer, is not guaranteed or endorsed by the publisher.
References
Aboagye, E., Yawson, J. A., and Appiah, K. N. (2020). COVID-19 and E-Learning: the challenges of students in tertiary institutions. Soc. Educ. Res. 2, 109–115. doi: 10.37256/ser.122020422
Adarkwah, M. A. (2021). “I’m not against online teaching, but what about us?”: ICT in Ghana post Covid-19. Educ. Inf. Technol. 26, 1665–1685. doi: 10.1007/s10639-020-10331-z
Adekola, J., Dale, V. H. M., and Gardiner, K. (2017). Development of an institutional framework to guide transitions into enhanced blended learning in higher education. Res. Learn. Technol. 25, 1–16. doi: 10.25304/rlt.v25.1973
Adiyarta, K., Napitupulu, D., Rahim, R., Abdullah, D., and Setiawan, M. I. (2018). Analysis of e-learning implementation readiness based on integrated elr model. J. Phys. Conf. Ser. 1007:012041. doi: 10.1088/1742-6596/1007/1/012041
Al-Ayed, S. I., and Al-Tit, A. A. (2021). Factors affecting the adoption of blended learning strategy. Int. J. Data Netw. Sci. 5, 267–274. doi: 10.5267/j.ijdns.2021.6.007
Albrahim, F. A. (2020). Online teaching skills and competencies. Turkish Online J. Educ. Technol. 19, 9–20.
Anderson, J. C., and Gerbin, D. (1998). Structural equation modeling in practice: a review and recommended two-step approach. Psychol. Bull. 103, 411–423. doi: 10.1504/EJIM.2021.114662
Anthony Jnr, B. (2021). Institutional factors for faculty members’ implementation of blended learning in higher education. Educ. Train. 63, 701–719. doi: 10.1108/ET-06-2020-0179
Anthony, B., Kamaludin, A., Romli, A., Raffei, A. F. M., Eh Phon, D. N., Abdullah, A., et al. (2019). Exploring the role of blended learning for teaching and learning effectiveness in institutions of higher learning: an empirical investigation. Educ. Inf. Technol. 24, 3433–3466. doi: 10.1007/s10639-019-09941-z
Antwi-Boampong, A. (2020). Towards a faculty blended learning adoption model for higher education. Educ. Inf. Technol. 25, 1639–1662. doi: 10.1007/s10639-019-10019-z
Archambault, L., and Larson, J. (2015). Pioneering the digital age of instruction: learning from and about K-12 online teachers. J. Online Learn. Res. 1, 49–83.
Archibald, D. E., Graham, C. R., and Larsen, R. (2021). Validating a blended teaching readiness instrument for primary/secondary preservice teachers. Br. J. Educ. Technol. 52, 536–551. doi: 10.1111/bjet.13060
Baron, R. M., and Kenny, D. A. (1986). The moderator-mediator variable distinction in social psychological research. conceptual, strategic, and statistical considerations. J. Pers. Soc. Psychol. 51, 1173–1182. doi: 10.1037/0022-3514.51.6.1173
Blieck, Y., Zhu, C., Schildkamp, K., Struyven, K., Pynoo, B., and Cindy, L. (2020). A conceptual model for effective quality management of online and blended learning. Electr. J. Elearn. 18, 189–204. doi: 10.34190/EJEL.20.18.2.007
Byrne, B. M., and van de Vijver, F. J. R. (2010). Testing for measurement and structural equivalence in large-scale cross-cultural studies: addressing the issue of nonequivalence. Int. J. Test. 10, 107–132. doi: 10.1080/15305051003637306
Cabrera-Nguyen, P. (2010). Author guidelines for reporting scale development and validation results in the journal of the society for social work and research. J. Soc. Soc. Work Res. 1, 99–103. doi: 10.5243/jsswr.2010.8
Callo, E. C., and Yazon, A. D. (2020). Exploring the factors influencing the readiness of faculty and students on online teaching and learning as an alternative delivery mode for the new normal. Univ. J. Educ. Res. 8, 3509–3518. doi: 10.13189/ujer.2020.080826
Chen, K. C., and Jang, S. J. (2010). Motivation in online learning: testing a model of self-determination theory. Comput. Hum. Behav. 26, 741–752. doi: 10.1016/j.chb.2010.01.011
Cheon, J., Lee, S., Crooks, S. M., and Song, J. (2012). An investigation of mobile learning readiness in higher education based on the theory of planned behavior. Comput. Educ. 59, 1054–1064. doi: 10.1016/j.compedu.2012.04.015
Cutri, R. M., and Mena, J. (2020). A critical reconceptualization of faculty readiness for online teaching. Distance Educ. 41, 361–380. doi: 10.1080/01587919.2020.1763167
Dennehy, D., Kasraian, L., Raghallaigh, P. O., and Conboy, K. (2016). “Product market fit frameworks for lean product development,” in Proceedings of the R&D Management Conference, July, (Cambridge), 1–11.
Fathema, N., Shannon, D., and Ross, M. (2015). Expanding The Technology Acceptance Model (TAM) to examine faculty use of Learning Management Systems (LMSs) in higher education institutions. MERLOT J. Online Learn. Teach. 11, 210–232.
Garrote, R., and Pettersson, T. (2016). Lecturers’ attitudes about the use of learning management systems in engineering education: a Swedish case study. Australasian J. Educ. Technol. 23, 1–19. doi: 10.14742/ajet.1256
Gautreau, C. (2011). Motivational factors affecting the integration of a learning management system by faculty. J. Educ. Online 8, 1–25.
Geng, S., Law, K. M. Y., and Niu, B. (2019). Investigating self-directed learning and technology readiness in blending learning environment. Int. J. Educ. Technol. High. Educ. 16:17. doi: 10.1186/s41239-019-0147-0
Goodhue, D. L., and Thompson, R. L. (1995). Task-technology fit and individual performance. MIS Q. Manag. Inf. Syst. 19, 213–233. doi: 10.2307/249689
Graham, C. R., Borup, J., Pulham, E., and Larsen, R. (2019). K–12 Blended teaching readiness: model and instrument development. J. Res. Technol. Educ. 51, 239–258. doi: 10.1080/15391523.2019.1586601
Graham, C. R., Henrie, C. R., and Gibbons, A. S. (2014). “Developing models and theory for blended learning research”, in Blended Learning: Research Perspectives, Vol. 2, eds A. G. Picciano, C. D. Dziuban, and C. R. Graham (New York, NY: Routledge), 13–33.
Graham, C. R., Woodfield, W., and Harrison, J. B. (2013). A framework for institutional adoption and implementation of blended learning in higher education. Internet High. Educ. 18, 4–14. doi: 10.1016/j.iheduc.2012.09.003
Hair, J. F., Sarstedt, M., Ringle, C. M., and Mena, J. A. (2012). An assessment of the use of partial least squares structural equation modeling in marketing research. J. Acad. Mark. Sci. 40, 414–433. doi: 10.1007/s11747-011-0261-6
Hartnett, M. (2016). Motivation in Online Education. Berlin: Springer. doi: 10.1007/978-981-10-0700-2
Hoffman, M. S. (2013). An examination of motivating factors on faculty participation in online higher education (Doctoral dissertation). NDissertaition, Northeastern University, February. Boston, MA: Northeastern University, 146.
Hong, J. C., Hwang, M. Y., Liu, M. C., Ho, H. Y., and Chen, Y. L. (2014). Using a “prediction-observation-explanation” inquiry model to enhance student interest and intention to continue science learning predicted by their Internet cognitive failure. Comput. Educ. 72, 110–120. doi: 10.1016/j.compedu.2013.10.004
Hu, L. T., and Bentler, P. M. (1999). Cutoff criteria for fit indexes in covariance structure analysis: conventional criteria versus new alternatives. Struct. Equ. Model. 6, 1–55. doi: 10.1080/10705519909540118
Ibrahim, M. M., and Nat, M. (2019). Blended learning motivation model for instructors in higher education institutions. Int. J. Educ. Technol. High. Educ. 16:12. doi: 10.1186/s41239-019-0145-2
Junus, K., Santoso, H. B., Putra, P. O. H., Gandhi, A., and Siswantining, T. (2021). Lecturer readiness for online classes during the pandemic: a survey research. Educ. Sci. 11:139. doi: 10.3390/educsci11030139
Kenny, D. A., Kaniskan, B., and McCoach, D. B. (2015). The performance of RMSEA in models with small degrees of freedom. Sociol. Methods Res. 44, 486–507. doi: 10.1177/0049124114543236
Kline, R. B. (2013). Assessing statistical aspects of test fairness with structural equation modelling. Educ. Res. Eval. 19, 204–222. doi: 10.1080/13803611.2013.767624
Lee, J., Lim, C., and Kim, H. (2017). Development of an instructional design model for flipped learning in higher education. Educ. Technol. Res. Dev. 65, 427–453. doi: 10.1007/s11423-016-9502-1
Legaspi, M., Acsaran, A., Bahoy, D., Cabildo, P., and Molina, R. (2021). Readiness, challenges and coping strategies of selected faculty in an academic institution towards teaching in new normal. J. Xidian Univ. 15, 467–475. doi: 10.37896/jxu15.5/052
Lin, W. S., and Wang, C. H. (2012). Antecedences to continued intentions of adopting e-learning system in blended learning instruction: a contingency framework based on models of information system success and task-technology fit. Comput. Educ. 58, 88–99. doi: 10.1016/j.compedu.2011.07.008
Little, T. D., Cunningham, W. A., Shahar, G., and Widaman, K. F. (2002). To parcel or not to parcel: exploring the question, weighing the merits. Struct. Equ. Model. 9, 151–173. doi: 10.1207/S15328007SEM0902_1
MacDonald, C., and Reid, G. (2013). Instructional strategies of inclusive physical education teachers: development and validation of a self-determination scale. Eur. J. Adapted Phys. Activity 6, 43–56. doi: 10.5507/euj.2013.004
Machado, C. (2007). Developing an e-readiness model for higher education institutions: results of a focus group study. Br. J. Educ. Technol. 38, 72–82. doi: 10.1111/j.1467-8535.2006.00595.x
Mahmoud, A. B., and Khalifa, B. (2015). A confirmatory factor analysis for SERVPERF instrument based on a sample of students from Syrian universities. Educ. Train. 57, 343–359. doi: 10.1108/ET-04-2014-0038
Maldonado, U. P. T., Khan, G. F., Moon, J., and Rho, J. J. (2011). E-learning motivation and educational portal acceptance in developing countries. Online Inf. Rev. 35, 66–85. doi: 10.1108/14684521111113597
Martin, F., Budhrani, K., and Wang, C. (2019b). Examining faculty perception of their readiness to teach online. Online Learn. J. 23, 97–119. doi: 10.24059/olj.v23i3.1555
Martin, F., Budhrani, K., Kumar, S., and Ritzhaupt, A. (2019a). Award-winning faculty online teaching practices: roles and competencies. Online Learn. J. 23, 184–205. doi: 10.24059/olj.v23i1.1329
Martin, F., Wang, C., Jokiaho, A., May, B., and Grübmeyer, S. (2019c). Examining faculty readiness to teach online: a comparison of US and German educators. Eur. J. Open Distance Elearn. 22, 53–69. doi: 10.2478/eurodl-2019-0004
Martin, J. (2010). Applying the Motivation-Hygiene Theory As a Means of Measuring Learner Satisfaction With Blended Learning Courses in Higher Education a Aplicação Da Teoria Da Motivação-Higiene Como Meio De Medida Da Satisfação Dos Alunos Em Cursos De B-Learning No Ensino. Aveiro: Universidade de Aveiro.
Masterman, E., and Craft, B. (2013). Designing and evaluating representations to model pedagogy. Res. Learn. Technol. 21:20205. doi: 10.3402/rlt.v21i0.20205
McGill, T. J., and Klobas, J. E. (2009). A task-technology fit view of learning management system impact. Comput. Educ. 52, 496–508. doi: 10.1016/j.compedu.2008.10.002
Mercado, C. A. (2008). Readiness Assessment Tool for an E-Learning Environment Implementation: Special Issue of the International Journal of the Computer, the Internet and Management, 1–11. Available online at: https://www.academia.edu/3705074/Readiness_Assessment_Tool_for_an_eLearning_Environment_Implementation
Mestan, K. (2019). Create a fine blend: an examination of institutional transition to blended learning. Australasian J. Educ. Technol. 35, 70–84. doi: 10.14742/ajet.3216
Nikou, S. A., and Economides, A. A. (2017). Mobile-based assessment: integrating acceptance and motivational factors into a combined model of self-determination theory and technology acceptance. Comput. Hum. Behav. 68, 83–95. doi: 10.1016/j.chb.2016.11.020
Okojie, M. C., Olinzock, A. A., and Okojie-Boulder, T. C. (2006). The pedagogy of technology integration. J. Technol. Stud. 32, 66–71. doi: 10.21061/jots.v32i2.a.1
Parasuraman, A. (2000). Technology Readiness Index (Tri): a multiple-item scale to measure readiness to embrace new technologies. J. Serv. Res. 2, 307–320. doi: 10.1177/109467050024001
Pereira, I., and Figueiredo, A. D. (2010). “Promoting motivation and participation in higher education: a b-learning experience,” in Proceedings - Frontiers in Education Conference, FIE, (Arlington, VA) 1–6. doi: 10.1109/FIE.2010.5673204
Porter, W. W., Graham, C. R., Spring, K. A., and Welch, K. R. (2014). Blended learning in higher education: institutional adoption and implementation. Comput. Educ. 75, 185–195. doi: 10.1016/j.compedu.2014.02.011
Rahmawati, N. I., Fauziati, E., and Marmanto, S. (2021). Investigating the institutional blended learning adoption framework in higher education. Indonesian J. EFL Linguist. 6:101. doi: 10.21462/ijefl.v6i1.345
Reeve, J. (2015). “Extrinsic rewards and inner motivation,” in Handbook of Classroom Management, eds C. M. Evertson and C. S. Weinstein (Mahwah, NJ: Lawrence Erlbaum Associates Publishers), 645–664. doi: 10.4324/9780203874783.ch24
Sangwan, A., Sangwan, A., and Punia, P. (2021). Development and validation of an attitude scale towards online teaching and learning for higher education teachers. TechTrends 65, 187–195. doi: 10.1007/s11528-020-00561-w
Sarstedt, M., Ringle, C. M., and Hair, J. F. (2014). PLS-SEM: looking back and moving forward. Long Range Plan. 47, 132–137. doi: 10.1016/j.lrp.2014.02.008
Sørebø, Ø, Halvari, H., Gulli, V. F., and Kristiansen, R. (2009). The role of self-determination theory in explaining teachers’ motivation to continue to use e-learning technology. Comput. Educ. 53, 1177–1187. doi: 10.1016/j.compedu.2009.06.001
Susanto, R., Rachmadtullah, R., and Rachbini, W. (2020). Technological and pedagogical models: analysis of factors and measurement of learning outcomes in education. J. Ethnic Cult. Stud. 7, 1–14. doi: 10.29333/ejecs/311
Tang, C. M. (2013). Readiness for blended learning: understanding attitude of university students. Int. J. Cyber Soc. Educ. 6, 79–100. doi: 10.7903/ijcse.1086
Teo, T. (2011). Factors influencing teachers’ intention to use technology: model development and test. Comput. Educ. 57, 2432–2440. doi: 10.1016/j.compedu.2011.06.008
Teo, T., Doleck, T., Bazelais, P., and Lemay, D. J. (2019). Exploring the drivers of technology acceptance: a study of Nepali school students. Educ. Technol. Res. Dev. 67, 495–517. doi: 10.1007/s11423-019-09654-7
Tubaishat, A., and Lansari, A. (2011). Are students ready to adopt E-Learning? A preliminary E-readiness study of a University in the Gulf Region. Int. J. Inf. Commun. Technol. Res. 1, 210–215.
Whelan, R. (2008). Use of ICT in education in the South Pacific: findings of the Pacific eLearning observatory. Distance Educ. 29, 53–70. doi: 10.1080/01587910802004845
Wong, L., Tatnall, A., and Burgess, S. (2014). A framework for investigating blended learning effectiveness. Educ. Train. 56, 233–251. doi: 10.1108/ET-04-2013-0049
Keywords: blended learning, technology adoption, technology readiness, institutional readiness, motivation, pedagogy
Citation: Antwi-Boampong A (2022) Testing and Validating a Faculty Blended Learning Adoption Model. Front. Educ. 7:851921. doi: 10.3389/feduc.2022.851921
Received: 10 January 2022; Accepted: 21 February 2022;
Published: 06 April 2022.
Edited by:
Mark Bedoya Ulla, Walailak University, ThailandReviewed by:
Veronico N. Tarrayo, University of Santo Tomas, PhilippinesShubham Pathak, Walailak University, Thailand
Copyright © 2022 Antwi-Boampong. This is an open-access article distributed under the terms of the Creative Commons Attribution License (CC BY). The use, distribution or reproduction in other forums is permitted, provided the original author(s) and the copyright owner(s) are credited and that the original publication in this journal is cited, in accordance with accepted academic practice. No use, distribution or reproduction is permitted which does not comply with these terms.
*Correspondence: Ahmed Antwi-Boampong, aan@es.aau.dk