- 1TUM School of Social Sciences and Technology, Department of Educational Sciences, Technical University of Munich, Munich, Germany
- 2Institute for Psychology, University of Freiburg, Freiburg, Germany
- 3Institute for Biology and Didactics, Freiburg University of Education, Freiburg, Germany
- 4School of Health Professions Education, Maastricht University, Maastricht, Netherlands
- 5School of Health Sciences, University of East Anglia, Norwich, United Kingdom
- 6Institute of Education, National Research University Higher School of Economics, Moscow, Russia
Video-based training offers teacher students approximations of practice for developing professional vision (PV; i.e., noticing and reasoning) of core teaching practices. While much video analysis research focuses on whole-classroom scenarios, for early PV training, it is unclear whether the focused instructional context of tutoring could be an appropriate and potentially supportive design element. The present study describes 42 biology teacher students’ performance on a tutoring video analysis task. With qualitative content analysis, we investigated how teacher students describe and interpret noticed tutoring events, with particular reference to research-informed PV indicators. With epistemic network analyses, we explored co-occurrences of PV indicators across teacher students’ six video analysis responses, contrasting low and high quality description and interpretation network models, respectively. We found that teacher students’ skills paralleled previous PV literature findings on novices (e.g., vague, general pedagogy descriptions). Yet, unexpectedly, some teacher students demonstrated aspects of higher sophistication (e.g., describing individual students, making multiple knowledge-based interpretations). Findings suggest tutoring is a powerful context for showing tutor-student interactions, making it suitable for initial teacher students’ PV training. Moreover, results offer hints about the range of teacher students’ PV mental models and highlight the need for more support in content-specific noticing and reasoning. Nevertheless, tutoring representations within PV video analysis training may offer teacher students support in student-centered attention and knowledge-oriented focus.
Introduction
A call for stronger emphasis on practice-based teacher education (Cohen and Ball, 1999; Grossman, 2018) aims to bridge the theory-practice divide (Broekkamp and van Hout-Wolters, 2007). For initial teacher students, with limited knowledge of pedagogy-in-action and few experiences teaching, the use of video-based analysis tasks offer a first step in the preparation of core teaching component implementation (Santagata and Angelici, 2010; Blomberg et al., 2013). In learning how to recognize and make sense of relevant practices from video examples, teacher students can begin to develop noticing skills and professional vision1 (PV; Goodwin, 1994; Sherin and van Es, 2009; Seidel and Stürmer, 2014).
The supportive structure within such approximations of practice (Grossman et al., 2009) can foster teacher students’ PV development. The video-based format offers a motivating learning context (Gaudin and Chaliès, 2015) with reduced complexity to facilitate processing (e.g., observing video vs. in-the-moment teaching). Moreover, the reduced authenticity provides teacher students with tasks to develop fundamental skills, building toward full practice performance (e.g., retrospectively noticing and interpreting observed events, training for spontaneous classroom attentional processing and decision-making; Kersting et al., 2010). With these supports, teacher students can begin to make connections between their theoretical and conceptual knowledge of teaching practices and the respective visual representations-in-action (Stürmer et al., 2013a), thus initiating PV for their future classrooms.
Much of the research on video-based training utilizes full classroom scenarios within the K-12 context. While these videos depict rich, authentic teaching, they may be overly complex for initial teacher students to analyze effectively (Derry et al., 2014). The potential load for novices’ mental processing in initial training (Mayer and Moreno, 2010) could be mitigated by emphasizing an instructional context that offers a distinct skill set also valuable to whole classroom teaching, such as tutoring (Lehman et al., 2012; van Merriënboer and Kirschner, 2018). With fewer students, tutoring has the potential to depict more student-centered instruction. This is important when fostering PV, as the classical models emphasize students’ learning processes (e.g., van Es and Sherin, 2002). Moreover, tutoring is an effective teaching method (Cohen et al., 1982; Graesser et al., 2011) wherein teacher students can apply general pedagogical and psychological knowledge (PPK) as well as pedagogical content knowledge (PCK) in their analysis. Limits in teacher students’ tutoring knowledge, such as giving long lecture-like content explanations before eliciting student’s prior knowledge or limited use of student interaction (Chi et al., 2001; Herppich et al., 2013), further demonstrate a need to target this context in teacher education. A video analysis of tutoring scenarios could facilitate initial teacher students’ development of tutoring PV skills as a transferable context to core components of student-centered instruction.
In the present study, we seek to gain a better understanding of initial teacher students’ strengths and deficits of tutoring PV from a video analysis task. By focusing on tutoring, we approach the central problem of teacher students’ difficulties in developing PV from complex classroom settings. Moreover, to better understand the complexity of PV and differentiate teacher students’ skills, we apply the novel approach of epistemic network analysis (ENA) to demonstrate the relationships between research-driven PV indicators across participants’ video analyses. Our description and exploration of initial teacher students’ PV performance on relevant tutoring practices offers a new stimulus to the field. Therein, we lay the groundwork for training development and future study of novice learning within similar contexts, and inspire further methodologies for instructional support.
In the following theoretical background, we elaborate on these points to provide a foundation of relevant research for the present study. First, we discuss the value of practice-based training in teacher education and specify video analysis as an example of such training. To follow, we discuss professional vision, and the PV skills that can be developed within video analysis training. We outline the two major components of PV (noticing and reasoning) and specify typical novice patterns of these subskills from content and quality perspectives. Next, we look at the evaluation of PV skills elicited from video analysis and the benefits to using ENA as a tool for depicting and clarifying the interconnected structure of PV skill components. Finally, we focus on the context of the present study: a video-based PV training developed for teacher students. We explain the use of scripted videos emphasizing tutoring (in biology) as a design aspect that could offer novice support in PV skill practice and development.
Practice-Based Teacher Education and Video Analysis
The everyday practices of teaching involve routines and activities taking place in a complex environment (Berliner, 2001). Many in-service teachers rely on a store of knowledge about teaching and learning, as well as their previous experiences, to continuously steer toward favorable decisions for student learning. But how can these automatic processes and routines of practice be translated for learning in teacher education?
Teacher students beginning to develop knowledge of general teaching and learning (i.e., PPK; Brophy, 2006; Voss et al., 2011), as well as subject-specific content instruction (i.e., PCK; Shulman, 1987; Darling-Hammond, 2006), lack the experience to fully apply this knowledge in practice. They need authentic application opportunities to gain experiential know-how. To realize the teacher education reform goal of increasing practice-based training, programs are increasingly focused on common and flexible core practices (Ball and Forzani, 2009; Windschitl et al., 2011; Kloser, 2014). This focus aims to balance maintaining field-like authenticity and complexity on the one hand, with suitability for novice initial mastery on the other hand (Grossman et al., 2009), offering opportunities for learning about teaching and student achievement with diverse methods in varied domains.
For initial teacher students, starting out with truly authentic practice experiences can be overwhelming and difficult to navigate. Alternatively, research suggests practices be taught in a gradual process of increasing complexity and authenticity (Grossman et al., 2009). Teacher students need opportunities to see practice-in-action representations (e.g., videos), learn how to “unpack” these practices into decompositions of their constituent parts (e.g., pre-training texts), and to “try on” a range of teacher moves, methods and procedures within approximations of practice (Grossman, 2018). Through these techniques, novices are thus supported in connecting theory and practice by accumulating increasingly authentic practical knowledge through the distribution of practice across a continuum of proximity (Grossman et al., 2009).
The use of video in educational training can offer a medium for learning and practicing core components of teaching. Video can promote effective learning, increase motivation, enhance noticing and reasoning, and elevate one’s practice in the classroom (e.g., Gaudin and Chaliès, 2015). However, it is not the video itself that supports effectiveness per se, but rather how it is used as a tool for reaching specific instructional goals (Brophy, 2004; Blomberg et al., 2013). The use of video for training knowledge application, analysis, and reflection has shown promise over the last 20 years (e.g., van Es and Sherin, 2002; Santagata et al., 2007). Beyond the practical advantages that (student) teachers can select particular sequences, pause, or view multiple times from various perspectives (Borko et al., 2008), video also allows learners the time to observe, decompose, and analyze events without the pressure of in-the-moment teaching (Sherin et al., 2009). Moreover, using video for analyses of teaching strategies and behaviors provides novices with approximations of practice to make connections between their knowledge and visual representations-in-action (Grossman, 2018).
Components of Teacher Students’ Professional Vision Skills
Through iterations and extensions of video analysis tasks, novices begin to cultivate their professional vision of the common patterns of practice they observe (Goodwin, 1994; Sherin and van Es, 2009; Seidel and Stürmer, 2014). From research in teacher expertise (Berliner, 2001), teacher PV denotes a range of particular characteristics expert educators embody, such as quickly and accurately recognizing meaningful patterns and automatically taking adaptable action. Educational researchers conceptualize PV to encompass two major components: (1) noticing, or the attention allocation toward particular observed patterns or practices; and (2) knowledge-based reasoning, representing how noticed events are interpreted in terms of one’s comprehension of the situation and associated knowledge (Borko et al., 2008; Sherin and van Es, 2009).
While some researchers delve deeper into particular noticed content (e.g., Star and Strickland, 2008), others focus on the overall PV structure. Seidel and Stürmer (2014), for example, decomposed knowledge-based reasoning into the subcomponents of describing, explaining and predicting, where observers describe what they noticed, and interpret these aspects by explaining their relevance to teaching-learning processes, and predicting potential learning consequences. Sherin and van Es (2002, 2009) differentiate PV into developmental stages of a “learning to notice framework,” comprising noticing, evaluation, and interpretation. Their research on teacher professional development video-clubs revealed a developmental shift in PV from simply describing noticed events at first, to incorporating positive or negative judgments, to finally using situational, theoretical, and practical knowledge as evidence to analyze the implications for student learning (van Es, 2011). Much of the research on teacher noticing has also emphasized the significance of content-specificity (Chan et al., 2021; Santagata et al., 2021). In the current study, we focus on teacher students’ written representations of PV’s main components: describing (i.e., noticing) and interpreting (i.e., knowledge-based reasoning). To further differentiate teacher students’ PV, we also look closer at important research-driven indicators of each overarching skill.
Describing depicts the events attended to, filtered through the observer’s professional knowledge of teaching and learning principles, relevant to the situation. What is noticed can depend on task aim, but commonly makes reference to the actors involved (e.g., teacher, students) and the topic of the event (e.g., subject-specific teaching move, classroom management strategy; Star and Strickland, 2008; van Es and Sherin, 2008). In terms of how these events are noticed, quality can differ according to event specificity and detail (Santagata et al., 2007). An observer is not likely to clearly recognize or specifically describe core practices they do not know about or understand. Thus, descriptions provide a window into the observer’s knowledge structures. A description with optimal PV “clearly differentiate[s] the relevant aspects of a noticed teaching and learning component” (Seidel and Stürmer, 2014, p. 7).
Looking into features of novice descriptions provides some insights on what they are likely to notice initially, and how they tend to describe it. In terms of the content, teacher students often focus on general, salient aspects of the whole class, and to superficial features of teacher pedagogy (Jacobs et al., 2010; van Es, 2011), rather than particular student actions related to content-specific issues. Moreover, they are more inclined to think of students as a uniform group (Jacobs et al., 2010) rather than a collection of diverse individuals with distinct needs for support. Concerning the way novice teachers describe the events they notice, observations usually remain limited to a vague, general level, lacking important details about the context (Jacobs et al., 2010; van Es, 2011), which would provide a more differentiated account of their observations.
Interpreting refers to justifications and/or analytical reasoning about a noticed teaching and learning event, with or without the use of knowledge-based evidence to support the claims. Skilled interpretations typically involve knowledge-based explaining or predicting (Seidel and Stürmer, 2014) with the use of evidence (Mason, 2002; Santagata and Angelici, 2010), but when less sophisticated, could simply make unjustified critiques (Sherin and van Es, 2009). Regarding the quality dimension, interpretations can range from unclear, illogical, or overly general associations, to argumentations connecting noticed events to multiple analytical points (e.g., cause-effect relationships; intention and rationale; inferences, implications) using evidence-based justifications from theoretical knowledge or video content references (Sherin and van Es, 2009; Kersting et al., 2010; König et al., 2014; Weger, 2019).
Taking a closer look at the features of novice interpretations, research indicates they are typically limited in explaining teacher actions and predicting student outcomes based on theoretical and/or material evidence. Often when teacher students attempt to interpret, they instead provide judgments that evaluate whether the noticed event was good or bad, without justification (e.g., evaluation: Sherin and van Es, 2002; van Es, 2011), or make oversimplifications about student comprehension (Jacobs et al., 2010). Additionally, the quality of their analytical argumentation usually demonstrates vague connections, at best (Jacobs et al., 2010).
While initial teacher students are likely to demonstrate novice-like PV skills, research provides some direction guiding the characteristics of higher quality PV skill, which outline the developmental trajectory their skills may take with continued practice (Jacobs et al., 2010; van Es, 2011). At the more distinguished end of the PV spectrum, experts display their describing and interpreting skills in qualitatively different ways, indicating a stronger focus on content- and situation-specific pedagogy (Jacobs et al., 2010), individual student understanding, and the provision of elaborative evidence based on video-specific associations and integrated understanding of teaching and learning principles (Jacobs et al., 2010; Santagata and Angelici, 2010; van Es, 2011). These component-level differences demonstrate the complexity involved in determining PV skill performance from video analysis.
Professional Vision and Epistemic Networking of Teacher Students’ Knowledge
Evaluating PV within a video analysis task involves recognizing what particular elements of an event are noticed and at what level of specificity they are described, as well as detecting the methods of reasoning, connection to evidence, and the elaboration of the argument. The individual what and how components (Berliner, 2001; van Es, 2011) identified within these skills can hint at particular areas of proficiency or deficit. However, a complex skill such as PV might also be more accurately modeled by exploring the interconnected structure of these components with one another.
For some theories in learning analytics (Shaffer, 2006), the idea of learning embodies “the development of an epistemic frame: a pattern of associations among knowledge, skills, habits of mind, and other cognitive elements” (Shaffer et al., 2016, p. 11). Learning is exemplified in how components of knowledge and skill that belong to a particular professional community are linked together within this epistemic structure. Developing epistemic frames involves skill building within a professional community’s “Big-D Discourse” (Gee, 1999, 2014), involving group-specific communication techniques, behaviors, values and beliefs, along with their associated tools. This broad conceptualization of professional Discourse, is not so unlike Goodwin’s (1994) original conception of professional vision, as “socially organized ways of seeing and understanding events” (p. 606) that are common and distinguishable for a particular group of professionals (Shaffer, 2018). Hence, analyzing (text) communication (i.e., “little-d discourse”; Gee, 1999, 2014) from individuals within a professional group (i.e., teacher students) in search of significant indicators of Discourse (i.e., PV skills) may provide information about the sophistication of their professional epistemic frames within a particular context (i.e., video analysis).
Research on discourse analysis demonstrates that frequent co-occurrences of ideas, themes, or concepts likely comprise cognitive associations (Lund and Burgess, 1996; Shaffer et al., 2016). This suggests that integrated knowledge structures can be modeled through epistemic networks depicting the connections between their constituent elements and the incidence rate of these links. Thus epistemic network analysis (ENA) is particularly valuable for modeling connectivity patterns between a group of related knowledge elements, when understanding the structure of the interrelationships among those elements provides more valuable information than the individual components alone (Shaffer et al., 2016). Within educational contexts, ENA has demonstrated increased visibility of complex relationship structures (e.g., Bauer et al., 2020). Not only does it allow for the exploration of individual networks, but also the capability of contrasting networks to visualize salient differences. Accordingly, for a video analysis task, exploring the interconnected structure of relationships between the various PV features of teacher students’ analyses may be beneficial for providing insight into the levels of integration within teacher students’ underlying PV mental models (Shaffer et al., 2016). Further, making comparisons between networks may reveal differences in teacher students’ depth of understanding across different levels of PV proficiency. Moreover, if similar components of PV are analyzed across different types of video analyses for PV training, PV epistemic networks could facilitate validity checks and comparisons between particular skills elicited from different learning materials, instructional contexts, or teaching scenarios tailored for specific groups or content areas.
Design Support: Scripted Videos of Tutoring Instruction
As evidenced by the wide range of PV skill components, video analysis offers the opportunity for (student) teachers to be immersed in a rich source of authentic, situated practice (Lave and Wenger, 1991; Goeze et al., 2014), and begin to develop links between these representations and professional knowledge (McDonald et al., 2013). This richness, however, can also challenge novices by overwhelming their mental processing capacities (Sweller, 1994; Mayer, 2001; Derry et al., 2014). For more efficient learning, teacher educators should consider facilitative design strategies associated with video implementation in pre-service teacher education (Seidel et al., 2013; Kang and van Es, 2019) and support beginners by preventing cognitive overload (Mayer and Moreno, 2010; Spanjers et al., 2010).
One starting point for this endeavor may be a focus on tutoring. We define tutoring as an instructional context involving teaching individual students, or, as in the present study, teaching a small group of students. The advantage to this intimate setting offers teachers the opportunity to respond and adapt particularly well to students individually and to use more student-centered strategies, in contrast to teaching a whole class (Graesser et al., 2011). A focus on tutoring in PV training may reduce complexity for teacher students and facilitate learning. The 4C/ID-model for complex learning design (van Merriënboer and Kirschner, 2018) proposes that broader instruction categories retain a holistic view, while organizing task sequences from simple to complex (Reigeluth, 1999). This whole-task approach offers learners a fast-paced overview of the whole skill (primarily, at its most simple real-world form), which is then steadily broadened and deepened through further training iterations of increasing complexity (van Merriënboer and Kirschner, 2018).
Accordingly, if whole-class teaching is considered to be the whole task, a focus on tutoring skills can be seen as an example of emphasis manipulation, a sequencing method that emphasizes an integrated set of constituent skills within a focused context (i.e., tutoring skills), which are also transferable to the whole task (i.e., whole-classroom teaching) (van Merriënboer and Kirschner, 2018). Tutoring skills encompass student-centered behaviors and methods that take advantage of the small-group context. Emphasizing this skill set may better suit teacher students’ cognitive skill development of student-centered teaching strategies by helping them start to assimilate tutoring-specific knowledge into cognitive schemas for tutoring skill coordination (Janssen et al., 2015; van Merriënboer and Kirschner, 2018). The application of these skills can then extend beyond the tutoring context. Thus, when teacher students apply PV skills (i.e., noticing/describing and reasoning/interpreting) toward the targeted instructional context of tutoring, they are specifically developing tutoring PV skills, yet in a broader sense, these skills would also be relevant within whole-classroom teaching.
Video examples of tutoring offer greater potential to demonstrate a range of student-centered core teaching practices based on both general and content-specific pedagogical knowledge (e.g., Lu et al., 2007; Cade et al., 2008; Lehman et al., 2012). Tutoring skills with a more general focus (i.e., PPK) might involve eliciting students’ prior knowledge and uncovering potential comprehension problems (Chi, 1996); reacting to incorrect student utterances with directed questions or feedback (VanLehn et al., 2003); assessing students’ understanding by asking students to explain their thinking (Graesser and Person, 1994; Graesser et al., 2011); or keeping all students active in a positive social learning climate by balancing individualized attention with group focus (Kaufman and Holmes, 1996). Tutoring actions from a PCK perspective would focus on content-specific issues, for example, eliciting naïve preconceptions or misconceptions typical of the target subject matter and grade level (e.g., 8th grade biology, human circulatory system: blood flows back and forth from heart to body) so they can recognize and react to these faulty beliefs appropriately (Chi et al., 2001). Other moves may include offering scientific models as alternatives to students’ misconceptions (Kloser, 2014); evoking a cognitive conflict or “impasse” in students’ thinking to help them reevaluate their misconceptions (VanLehn et al., 2003); or check whether students made modifications to their misconceptions after instruction (Chi et al., 2004; Scharfenberg and Bogner, 2019). A wide range of student-centered strategies associated with PPK and PCK may be applicable to a tutoring context. However, research suggests designing video-based training for teacher students around only a few specific learning objectives (Kang and van Es, 2019). Thus, emphasizing a limited set of specific tutoring practices tied to PPK and PCK for observation and analysis may help teacher students begin to notice and make sense of important student-centered teaching moves.
Of course, PCK involves knowledge of teaching within a specific subject matter. While PV skills in mathematics PCK have been researched extensively (e.g., Santagata et al., 2021), less is known about teacher students’ PV skills in science. Moreover, research in science teaching, particularly in biology, already offers wide-ranging knowledge about PCK aspects (e.g., specified typical misconceptions), making it an ideal subject to investigate (Park and Chen, 2012; Schmelzing et al., 2013). Framing a video analysis task around biology tutoring offers an instructional setting that can model tutoring moves from content general PPK and content-specific PCK perspectives, to elicit teacher students’ PV skills for both. Since knowledge of subject-specific content plays an important role in PCK (Kleickmann et al., 2013; Großschedl et al., 2015), it would be important to match the content focus of a video analysis to the teacher students with prior content knowledge of the topic. Targeting biology teacher students for this training also allows for a direct comparison of their PV skills in PPK and PCK to distinguish where strengths and deficits may lie. While teacher students without biology-specific prior knowledge may still benefit from such a training, their lack of biological prior knowledge may render the training less effective, likely due to cognitive load-related problems understanding the content, rather than the tutoring actions associated with that content (Kalyuga, 2009; Renkl et al., 2009).
In addition to designing support with emphasis manipulation of targeted tutoring strategies, tasks using a video format offer a simplified medium for learning in contrast to genuine teaching. Typically, using videotaped teaching examples leverages the benefits of situated authenticity, particularly when selecting clips relevant to the learning goals (Sherin et al., 2009; Borko et al., 2014). For further simplification, scripted videos offer a range of complexity-reducing opportunities for the benefit of novice learning (e.g., condensing content, emphasizing particular target behavior, setting the intended scene, contrasting differentiated events) while still maintaining sufficient authenticity (Piwowar et al., 2018).
Thus, video analysis tasks utilizing scripted video representations of biology tutoring practice may offer support in training teacher students’ PV. Streamlined videos that highlight a range of content-general and content-specific tutoring strategies could help biology teacher students’ systematic professional observation of the target instruction. Further, they may facilitate deliberate connections to teacher students’ theoretical and conceptual knowledge to build increasingly complex mental models of core teaching practices (Mevorach and Strauss, 2012; Manrique and Abchi, 2015). To identify the learning prerequisites and needs of teacher students for PV training in this context, a first step would be the description and exploration of their PV skills in tutoring.
The Present Study
The present study describes teacher students’ professional vision skills elicited from a targeted video analysis task. This task is focused on aspects of the two subskills of PV, describing (noticing) and interpreting (reasoning about) relevant events within small-group tutoring scenarios in biology. From teacher students’ responses, we examine theoretically grounded indicators of PV content and quality for describing skills and interpreting skills, respectively. We also explore differences in teacher students’ PV skillsets by looking at relationship configurations among PV content elements and by contrasting these relationship networks between low and high quality descriptions and interpretations.
When looking deeper into features of novice descriptions of noticed events, research provides some guidance on what they are likely to notice (e.g., general aspects of teacher pedagogy and a focus on students as a group), and how they tend to describe it (e.g., making unspecific generalities and vague descriptions). To investigate the nature of teacher students’ descriptions of noticed tutoring events from the video analysis task, we consider the following set of description (D) research questions:
D-1 Do teacher students demonstrate typical novice PV patterns regarding the content they describe?
D-2 Do teacher students demonstrate typical novice PV patterns in terms of the quality of information they provide in their descriptions?
Moreover, we explore:
D-3 What are the relationship configuration structures among the content elements of teacher students’ descriptions at different levels of information quality, and how do they differ?
We estimate that teacher students will follow the typical novice patterns in the content (D-1) and information quality (D-2) of their descriptions of noticed tutoring events. However, we tentatively suggest that the tutoring video analysis context may offer support to novices in their descriptions. Next, we will explore the interrelated nature of teacher students’ content and quality within their descriptions and contrast low and high quality groups (D-3) to distinguish skill differences.
Taking a closer look at the features of novice interpretations of noticed events, research indicates that novices’ abilities are typically very limited in terms of the types of interpretations they make or the components of their arguments (e.g., some judgmental evaluations and few knowledge-based interpretations, such as explanations or predictions). They also demonstrate limits in the quality of their arguments (e.g., vague connections between analytical points). To further investigate the nature of teacher students’ interpretations of noticed tutoring events from the video analysis task, we ask the following set of interpretation (I) research questions:
I-1 Do teacher students demonstrate typical novice patterns regarding the components of their interpretations?
I-2 Do teacher students demonstrate typical novice patterns with respect to the quality of their analytical arguments?
In addition, we explore:
I-3 What are the relationship configuration structures among the components of teacher students’ interpretations at different levels of argumentation quality, and how do they differ?
We anticipate that teacher students will demonstrate the common novice patterns regarding the components (I-1) and argument quality (I-2) of their interpretation of tutoring events. However, we cautiously suggest that the tutoring video analysis context may offer support to novices in their interpretations. Next, we will elucidate PV skill differences between low and high levels of interpretation performance by exploring and contrasting the relationships among interpretive components and argumentation quality (I-3).
Our objective in describing and exploring initial teacher students’ tutoring PV performance is to present the commonalities and differences between the typical novice PV elicited from previous research and novice PV achieved from a new design perspective and instructional setting. Through this, we first hope to validate that initial PV skills can be observed within a video analysis training focused on tutoring, thus establishing a link to previous PV research. Secondly, we explore the potential of this new context by highlighting outcomes elicited from our instrument that seem ‘better-than-typical,’ relative to novice PV performance standards. With our identification of the range and composition of biology teacher students’ tutoring PV skills, we offer a baseline for future study and training development for novice skill building within similar PV training designs. Further, this initial application of ENA to the PV research community aims to offer a tangible display of this construct’s multifaceted nature across multiple levels of granularity, thus contributing relevant knowledge to the fields of teacher training and PV research.
Materials and Methods
Participants
Participants comprised 42 biology teacher students2 [78.6% female3, Mage = 23.26 years (SD = 3.99)] from two southern-German universities. Since video representations depicted instruction on the circulatory system, we focused our selection on biology teacher students to assure similarities in sample-wide content-specific prior knowledge. For increased ecological validity, students participated in the study within a biology teacher education seminar, where video analysis was a course objective.
Participants had attended an average of two to three semesters at the university level (0–4 semesters n = 27; 5–8 semesters n = 12; >8 semesters n = 3). Their average university coursework experience included five or more classes in biology content (76.2%), and three to eight generic pedagogical- (psychological) courses (71.5%). For biology-specific pedagogy and didactics, participants’ average experience was only one to four classes (83.3%), with 57.1% attending two or fewer, and only four participants with more than four. Additionally, they had an average experience of 5 to 8 weeks in a practical teaching internship, between 6 to 20 h of teaching in a classroom, and between 1 to 2 years of tutoring experience.
Study Design and Procedure
Study Context
Based on the framework for the teaching of professional practice (Grossman et al., 2009), this study was designed as a training that could be flexibly integrated and further adapted within teacher education courses. The framework’s three elements were incorporated to support preservice teachers’ acquisition of professional vision skills: representations: videos of biology tutoring scenarios; decompositions: introductory texts distinguishing specific core components of tutoring instruction; and approximations of practice: video analysis tasks wherein theoretical information (i.e., from the texts) is applied toward noticing and interpreting relevant events.
Procedure
For data collection (July, 2019), the study was digitally administered via secure online software. Participants were provided laptops and headphones and given anonymized login codes for condition randomization. After voluntary informed consent, participants began with all instructions and data collection, self-paced through the platform. The study was divided into three parts: (1) participant information and introductory text; (2) video analysis tasks; and (3) participants’ evaluation of the whole training.
First, we collected demographics and experience-related information (i.e., age, gender, semester of study, coursework, and experience in teaching and tutoring). To follow, participants were shown one of three randomly assigned introductory texts that provided theoretical input (i.e., decomposition of practice) for application in their analysis. The pre-training support (Mayer et al., 2002) from the texts included information regarding PPK or PCK components in tutoring, or important elements of systematic video observation. An even distribution of these texts helped us ensure a balanced distribution of knowledge for application at the sample level. Teacher students were instructed to read carefully (verified with a short quiz), so they could apply this knowledge toward professional observations and analyses of two video-based tutoring scenarios.
In the subsequent video analysis section, participants were first given contextual descriptions about the general setting and then instructed on the analysis task. They were to watch two video clips, one depicting the beginning of a tutoring lesson (elicitation phase) and one in the middle of a lesson (learning phase) (see section ‘‘Study Materials’’ for details). Upon starting the first video, participants should press the space bar (recording a timestamp) when they noticed something relevant to teaching and learning in tutoring. We indicated there was no upper limit, but they might see 5--10 events per video. To ensure similar viewing experiences for all participants, the video played continuously with no opportunity for pause or playback4. Following the first video, three of the participants’ noticed events (clips of 10 s.) were automatically selected at random for further review and comment. The PV prompt asked participants to write two to three sentences that (1) describe the tutor behavior or event they noticed, and (2) explain why it was relevant to the teaching-learning process for a tutoring situation. Participants completed this PV open response for the three events, then repeated this task for the subsequent learning phase video clip. The open response comments from these tasks provided the data for our investigation.
In the third study section, participants evaluated the whole training session with cognitive and motivational feedback measures. Upon completion, participants received a written debriefing and compensation (15€).
Study Materials
In the first study section, participants read one of three variations of an introductory text. Two texts focused on PPK or PCK, respectively, and juxtaposed strategies according to a student-centered tutoring style with strategies following an instructive style, less optimal for the tutoring context. A third text contrasted professional observational practices against common pitfalls of novice observation (for details, see study by Martin et al. (2021) on the comparison of these texts).
In the second part of the study, a sequence of two videos (i.e., elicitation phase and learning phase) were used for the targeted video analysis tasks. All videos portrayed a scene with one teacher/tutor and four eighth-grade biology students during an introductory lesson about the human circulatory system (see Figure 1). The videos were scripted and performed by actors. The scripts were developed by the research group based on authentic tasks and dialogs from a pilot video study. They were designed to exemplify an authentic context emphasizing tutoring skills, which exhibited a mixture of tutoring instructional strategies grounded in PPK and PCK (both student-centered and tutor-centered).
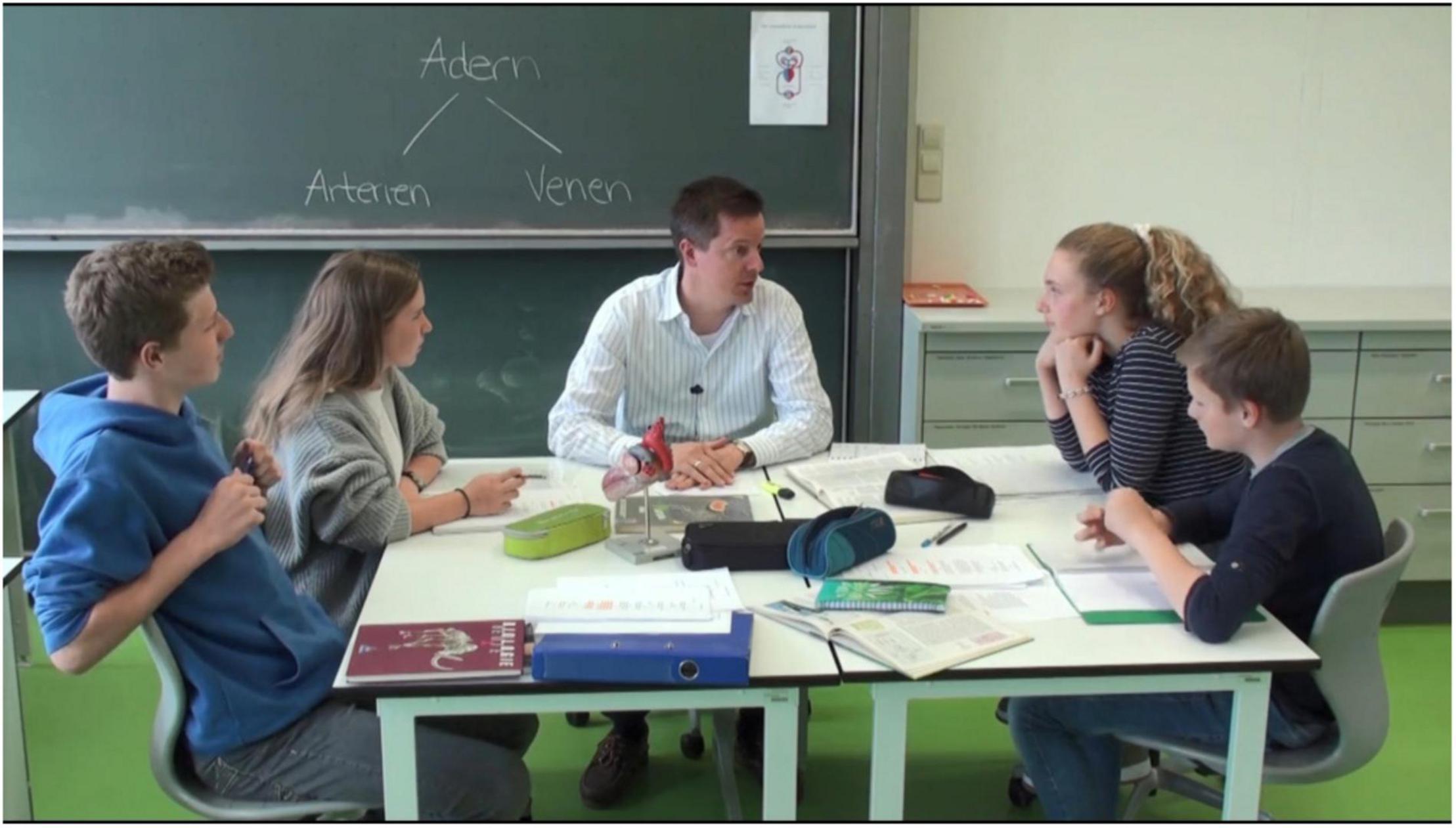
Figure 1. Tutoring videos. Screenshot from one of the videos, depicting the typical layout for all videos.
For each phase, participants were randomly assigned to watch a video from a pool of four videos (two pairs from two scripts). Participants were not all provided with the same videos so that the analysis of PV skills would not be tied to one particular set of videos depicting one set of events. Rather, they would capture noticing and interpreting skills across varying scenarios within a common context, thereby eliciting a more representative sample of the PV skills of the target population. All videos were long enough so that participants had plenty of events to notice and comment on. The elicitation phase (E-) videos (∼8 min) either depicted a scene where a tutor begins with a brainstorming activity, followed by a discussion of the students’ circulatory system knowledge (E-brainstorming script); or a scene where the tutor gives students the task of drawing their imagined cardiovascular system on a body outline worksheet, then discussing them (E-drawing script). The learning phase (L-) videos (∼6 min) showed either a scene where the tutor asks the students to compare a textbook diagram of the cardiovascular system with their own drawings, followed by a discussion (L-diagram script); or a scenario where the tutor role-plays a discussion as a content-naïve exchange student, followed by tutor-led content clarifications (L-role-play script). Scripts for each video were developed according to research-based guidelines and recommendations (e.g., expert selection of evidence-based practices for tutoring; balancing the distribution of events from PPK and PCK perspectives across all videos in script storyboard planning; Piwowar et al., 2018).
To ensure adequate authenticity of the videos, participants rated the authentic representation of each tutoring scenario with six items (α = 0.83; Piwowar et al., 2018) which rated the scene (e.g., “The video model was realistic”) and the actors (e.g., “The actor/actress performed his/her role as a teacher/tutor convincingly”), on a four-point scale [disagree (1), to fully agree (4)]. Overall, they deemed the videos sufficiently authentic, M = 2.99 (SD = 0.47), likewise comparable to scripted video ratings from Piwowar et al. (2018). Further, a parallel investigation of the same video materials found the two elicitation phase videos and the two learning phase videos to be of similar difficulty, with no significant differences for participants’ outcome measures (Martin et al., 2021).
Qualitative Content Analysis of Teacher Student Responses
Coding Scheme Development
The coding scheme consisted of analysis indicators including what the teacher students analyzed (content) and how they analyzed the video clips (quality). The content coding protocol was constructed through deductive and inductive iterations according to qualitative content analysis (Mayring, 2015). An initial deductive process extracted important noticing and interpreting PV indicators from the literature (Sherin and van Es, 2002, 2009; Santagata et al., 2007; Kersting, 2008; Sherin et al., 2008; Star and Strickland, 2008; Jacobs et al., 2010; van Es, 2011; Seidel and Stürmer, 2014). In a second iteration, the theoretically based indicators were inductively tested with a random 20% subsection of the open response data to narrow down codes and adapt guidelines. The quality coding protocol (based on Weger, 2019) was similarly developed.
Coding Scheme Indicators
For analysis, PV skills were divided into the subskills of (1) describing noticed tutoring events, actions, or moves (for D-1 to D-3); and (2) interpreting what was noticed (for I-1 to I-3). The descriptions of tutor actions were further broken down into content indicators (actor; topic; D-1) and quality indicators (quality of information; word count; D-2) (see Table 1).
The interpretations of teacher students’ noticed tutoring events, actions, or moves were further classified into content indicators (knowledge-based; uninformed; I-1) and quality indicators (quality of analytical argumentation; word count; I-2) (see Table 2).
Coding Procedure
The analysis aimed at understanding the content and quality of teacher students’ responses in order to evaluate their PV skills in describing and interpreting noticed tutor-specific events. To achieve this, each indicator was coded according to a coding protocol for each video clip response (6 responses per participant). The coding scheme outlined inclusion and exclusion criteria and guidelines for scoring. The first author and a second rater, trained in the study materials and coding protocols, independently coded the written responses for all indicators. Inter-rater reliability was assessed for each indicator and any disagreements were resolved through a coding manual review and consensus discussion.
For the content indicators, inter-rater reliability was assessed with Cohen’s Kappa (Cohen, 1960) after a randomized 20% of the data (n = 116 responses) had been coded. Consistency between raters was shown to be substantial or better (≥0.61; Landis and Koch, 1977) for seven indicators (ranging from κ = 0.66 to κ = 0.91). For two indicators, the Kappa statistic did not accurately capture the consistency of rater agreement due to prevalence (Di Eugenio and Glass, 2004). In these cases, we determined a prevalence index (PI)5, then calculated a prevalence-adjusted bias-adjusted kappa (PABAK) statistic (Byrt et al., 1993; Watson and Petrie, 2010) to correct for the unequal distribution of occurrences relative to probability (Tutor: κ = 0.28, PI = −0.94, PABAK = 0.91; T-S Interaction: κ = 0.45, PI = 0.71, PABAK = 0.72). Considering these adjusted values, we conclude there was substantial agreement between the two raters for all content indicators.
For the scaled quality indicators, intra-class correlation (ICC) was used to measure inter-rater reliability (Hallgren, 2012). ICC estimates were based on a mean-rating (k = 2), consistency, 2-way mixed-effects model. The average ICC for quality of information was 0.88, and for quality of analytical argumentation, 0.90. Thus, a high degree of reliability was found for both quality measures (Koo and Li, 2016). Table 3 provides example responses for these scaled quality indicators.
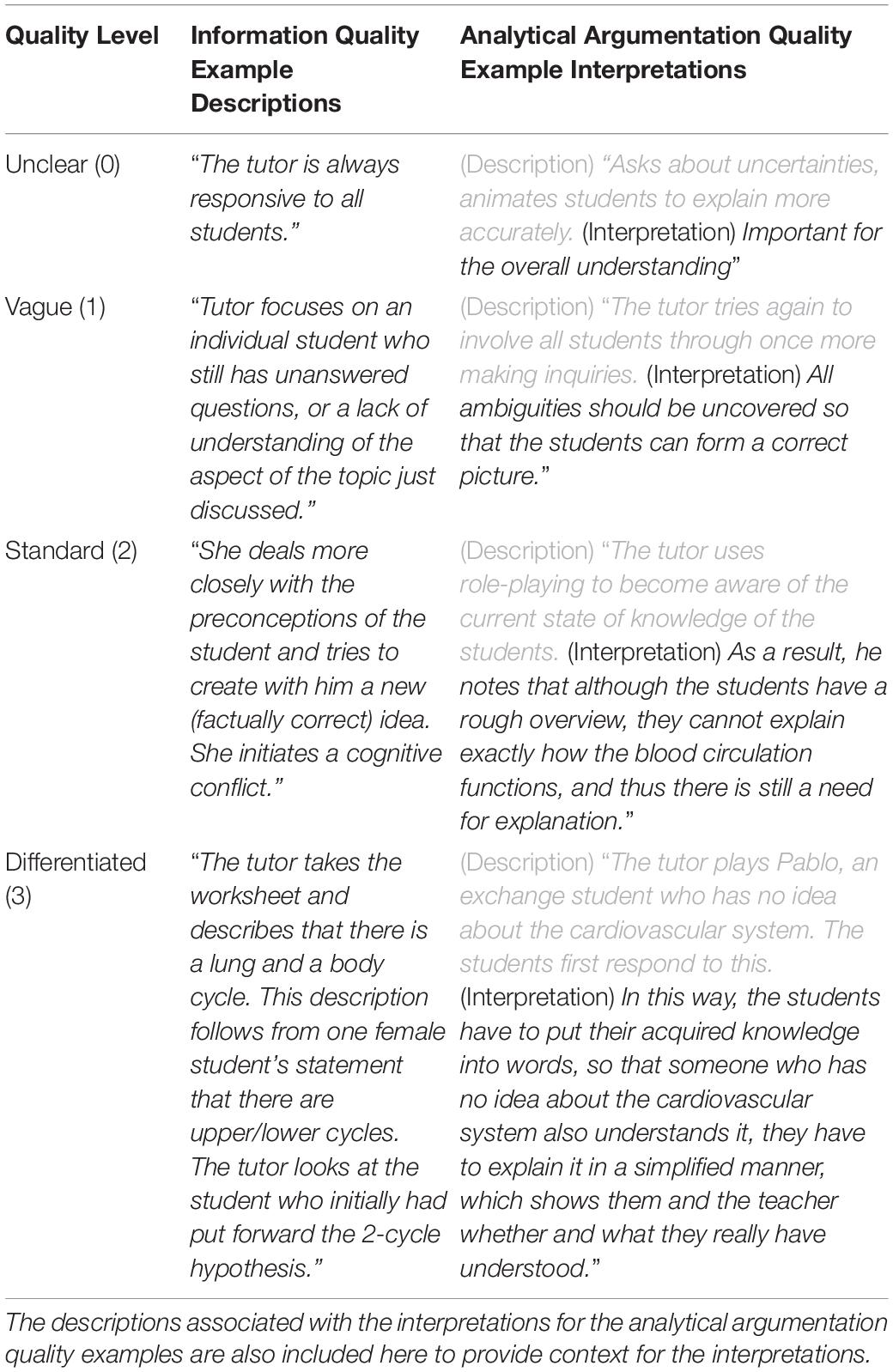
Table 3. Example responses at the four quality levels of information (describing) and analytical argumentation (interpreting).
Epistemic Network Analysis
We used ENA to facilitate the conceptualization of participants’ overall PV skill level. With this technique, we demonstrate the relationships that the PV indicators had between one another across participants’ video analysis responses for describing (D-3) and interpreting (I-3) tutoring events (Shaffer et al., 2016). This approach creates network models that illustrate connections between various components of a multifaceted (cognitive) system (e.g., elements of knowledge, and/or skill) (Shaffer, 2006; Shaffer et al., 2016). From coded data representing these network elements, ENA recognizes links, calculates the relationship intensity and the elemental structure of the associations between these features, and displays them graphically (Shaffer and Ruis, 2017; Bowman et al., 2021). In the context of educational research, ENA offers a novel means to depict complex relationships (e.g., Csanadi et al., 2018; Schnitzler et al., 2020).
We applied ENA to our coded data using the ENA1.7.0 Web Tool (Marquart et al., 2018), which uses three data levels to construct network models: nodes, stanzas and units. The smallest components of the network comprise nodes, which represent the codes of interest connected within the model (Shaffer and Ruis, 2017). Codes are assessed at the segment level, where each segment represents the smallest piece of text from a stanza (i.e., group of related segments), which will be assessed for co-occurrence of codes between other segments of the same stanza. For the describe network models, the codes making up the structure consist of the describe category content indicators: tutor move, group of students, individual student, PPK move, PCK move, and T-S interaction. For the interpret network models, the codes consist of the interpret category content indicators: explanation, prediction, assumption, and judgmental evaluation.
At the next level of abstraction, stanzas, represent the frame for assessing the co-occurrence of codes. They comprise a set of text segments that are meaningfully associated with each other to be analyzed at the individual network level. Since we wanted to examine the indicator connections between participants’ describing and interpreting skills, respectively, we define the stanzas for both network models as all responses associated with a single participant, where each segment of the stanza represents a single video analysis response. Thus, the co-occurrence of codes for each participant (across their 6 responses) represents conceptually associated sets that provide meaning for the interpretation of PV data interactions.
The third, meta-level of the network frame comprises the units, which arrange a series of stanzas into groups that sum up the associations between codes across all stanzas belonging to that particular unit group (see Shaffer et al., 2016, for details; Bowman et al., 2021). For both network models, unit groups were assembled according to the average quality of participants’ descriptions and interpretations, respectively. For the describe models, the network groups consist of all the responses associated with either unclear, vague, or standard/differentiated quality of information. For the interpret models, the network groups are defined as the respective responses within unclear, vague, or standard/differentiated quality of analytical argumentation.
Thus, with ENA we further explore PV network connectivity patterns between content indicators (i.e., elements that co-occur across teacher students’ responses), and contrast networks of different quality to elucidate pattern differences. For both PV skill categories, we will first present the ENA projection of the three quality group mean network models and report their goodness of fit (Pearson and Spearman correlations). The goodness of fit correlations represent the alignment of all projected points and the network mean locations, thus the networks and summary statistics are co-registered, reflecting similar information (Shaffer et al., 2016). Observing the location of the group mean networks offers information about the similarities or differences between the mean network groups in overall structure, based on their distance from one another in the space. Each group mean network placement represents the mean connection patterns for each constituent individual network, relative to all other networks. Thus, group mean networks that are closer together can be assumed to have more similar network structures and patterns compared to ones farther apart.
To facilitate further interpretation of the space, we will then contrast the visualizations for the low quality and high quality group mean networks. The group mean network visualizations can be interpreted such that thicker lines between nodes depict more frequent connections, and nodes positioned closer together have less variance between them (i.e., common co-occurrence patterns) compared to nodes positioned farther apart (Shaffer et al., 2016). We additionally compare the mean networks quantitatively with t-tests on the X- and Y-axis dimensions.
Results
Teacher Students’ Describing Skills in Tutoring Video Analysis
Content and Quality of Teacher Students’ Descriptions
The first set of research questions focused on teacher students’ describing skills. Results regarding the content they describe (D-1) indicated that almost all teacher students described a relevant tutor move [n = 232 responses overall; n = 36 participants (85.7%) for all six comments], and most were focused on general (psychological) pedagogy [i.e., PPK; n = 216 responses overall; n = 26 participants (61.9%) for all six comments]. In contrast, almost half of participants [n = 20 participants (47.6%)] did not make any reference to content-specific tutoring events (i.e., PCK; n = 34 responses overall). Additionally, more than two thirds of the comments referred to the students as a group [n = 168 responses overall; n = 30 participants (71.4%) for 4 of 6 comments or more], and about one third mentioned individual students [n = 81 responses overall; n = 31 participants (73.8%) in 1 to 3 of their comments].
For the quality indicators (D-2), results show that the majority of descriptions were low in the quality of information they described (M = 0.95, SD = 0.67; word count: 2–78 words, M = 15.41, SD = 9.36). The majority of descriptions fell into the unclear or vague categories [n = 201 responses; n = 28 participants (66.7%) for 4 of 6 comments or more]. Table 4 presents the extent each content and quality indicator was described across all teacher students’ responses (i.e., response level). Since each participant made six different responses, Figure 2 displays the distribution of the coded categories across all six responses at the participant-level.
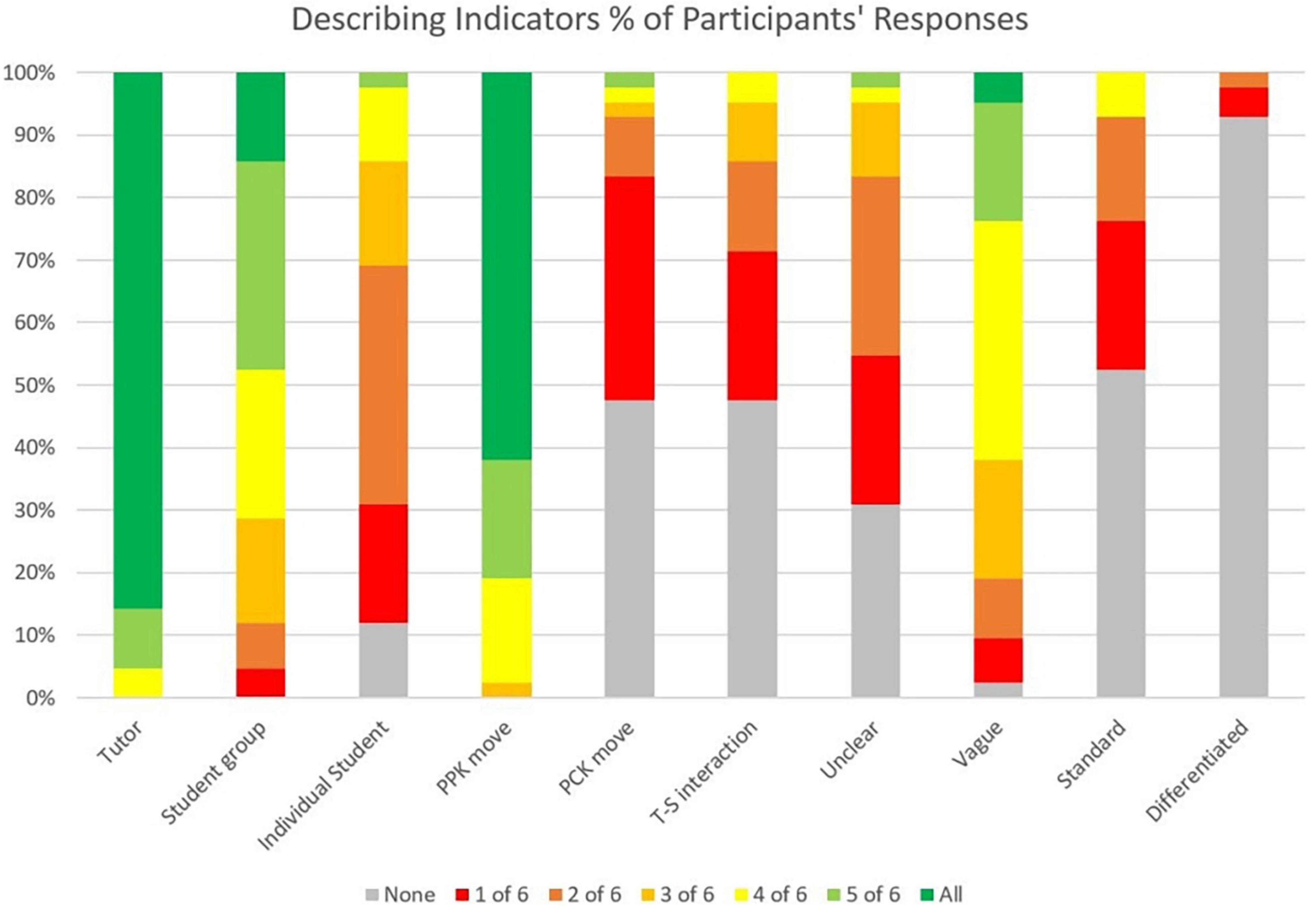
Figure 2. Proportion of describing indicators within participants’ responses. Teacher students’ made a total of six different video analyses. The Figure shows the proportion of each describing indicator present across all six descriptions of each participant. Response proportions are depicted with a red-yellow-green color scale to represent fewer to more presence of an indicator across a participants’ six responses. Indicator bars demonstrate the distribution of these proportions across all participants. Results based on 240 responses from 42 participants (6 responses per participant, 12 missing responses).
Epistemic Networks of Teacher Students’ Descriptions
Epistemic network analysis facilitated the visualizations of description content and quality connections across participants’ responses to explore and contrast relationship configurations and structures at a more sophisticated level (D-3). Figure 3 depicts the ENA projection space for the describe indicator networks distributed across their quality of information groups (model goodness of fit for X-Axis: Pearson r = 0.99, Spearman r = 0.98; for Y-Axis: Pearson r = 0.99, Spearman r = 0.97). The ENA space depicts the vague and standard/differentiated groups overlapping, indicating less variance between their structures, and the unclear group separated from the others, signifying greater variance between its structure and the structure of the other groups.
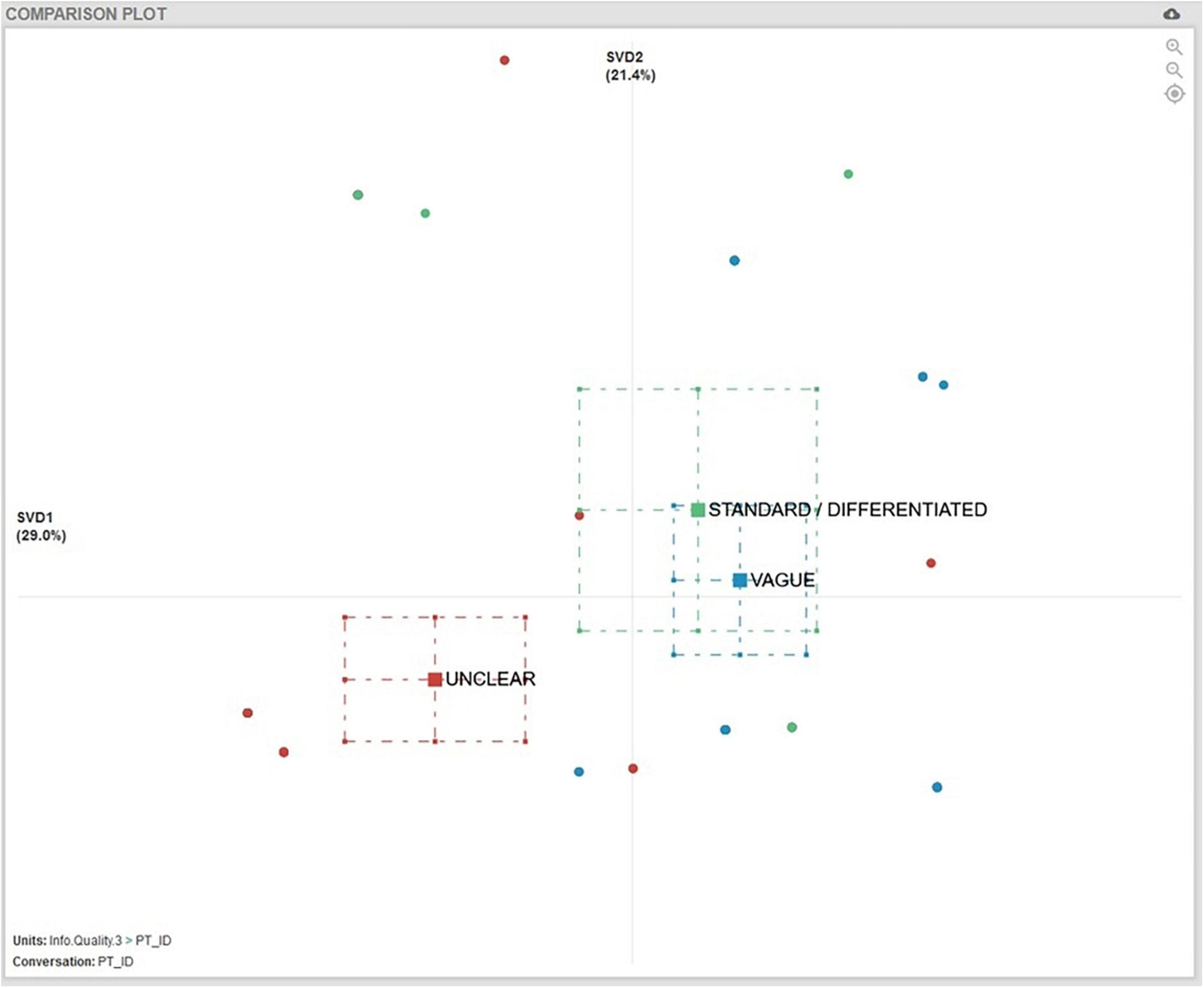
Figure 3. Position of describe mean networks on ENA projection space. This Figure shows the ENA space for the describe indicator quality networks and the three quality mean networks distributed on the space. Due to the lower frequency of their occurrence, the standard and differentiated quality indicators were combined into one group. However, small differences in group frequency do not distort the ENA models due to spherical normalization (Shaffer et al., 2016). Group network means, or group centroids, are depicted by the solid square points with their associated 95% confidence intervals shown with the dotted lines. SVD stands for singular value decomposition and SVD1 and SVD2 represent the two largest variances between models on the X- and Y-axis, respectively. Participants with at least one unclear, vague, or standard/differentiated description, respectively, formed the nested quality groups. While participants may belong to more than one quality group, the average of their six responses is represented by their placement on the projection space (colored dots). Thus, a participant’s network made up of mostly unclear responses, for example, would be placed closer to the unclear group centroid, compared to a participant’s network that includes only one unclear response. For the Describe mean networks, Unclear n = 29 participants; Vague n = 41 participants; and Standard/differentiated n = 20 participants.
More information about how the overall space (Figure 3) can be interpreted is provided by the positioning of the nodes in the mean networks (Figures 4A,B). Since network and summary statistics are co-registered on the space and nodes are fixed, the placement of the nodes reflects similar information about the space in general, and can be used to interpret the space overall (Shaffer et al., 2016). The nodes for the tutor, students as a group, and PPK tutor move are displayed near the center of the space, relatively close together. This indicates less variance between these codes, and their common connectivity across teacher student response networks within all three mean networks. The node representing connections with PCK tutor moves is located at the top of the space. Since the standard/differentiated mean network is closest to this node, this indicates that teacher students’ response networks from this group made more connections to PCK in contrast to response networks in the other mean networks. Moreover, the distance of this node from the others suggests increased variance between connections with this code and other describing indicators in teacher students’ response networks. The nodes for individual student and teacher-student dialogic interaction are located at the right-center of the space, showing that the vague and standard/differentiated mean networks (Figure 3, also on the right) and their respective teacher students’ response networks had more connections to these codes compared to the response networks comprising the unclear mean network. Moreover, the positioning of these nodes near each other points to similarity in their connectivity patterns in teacher student response networks.
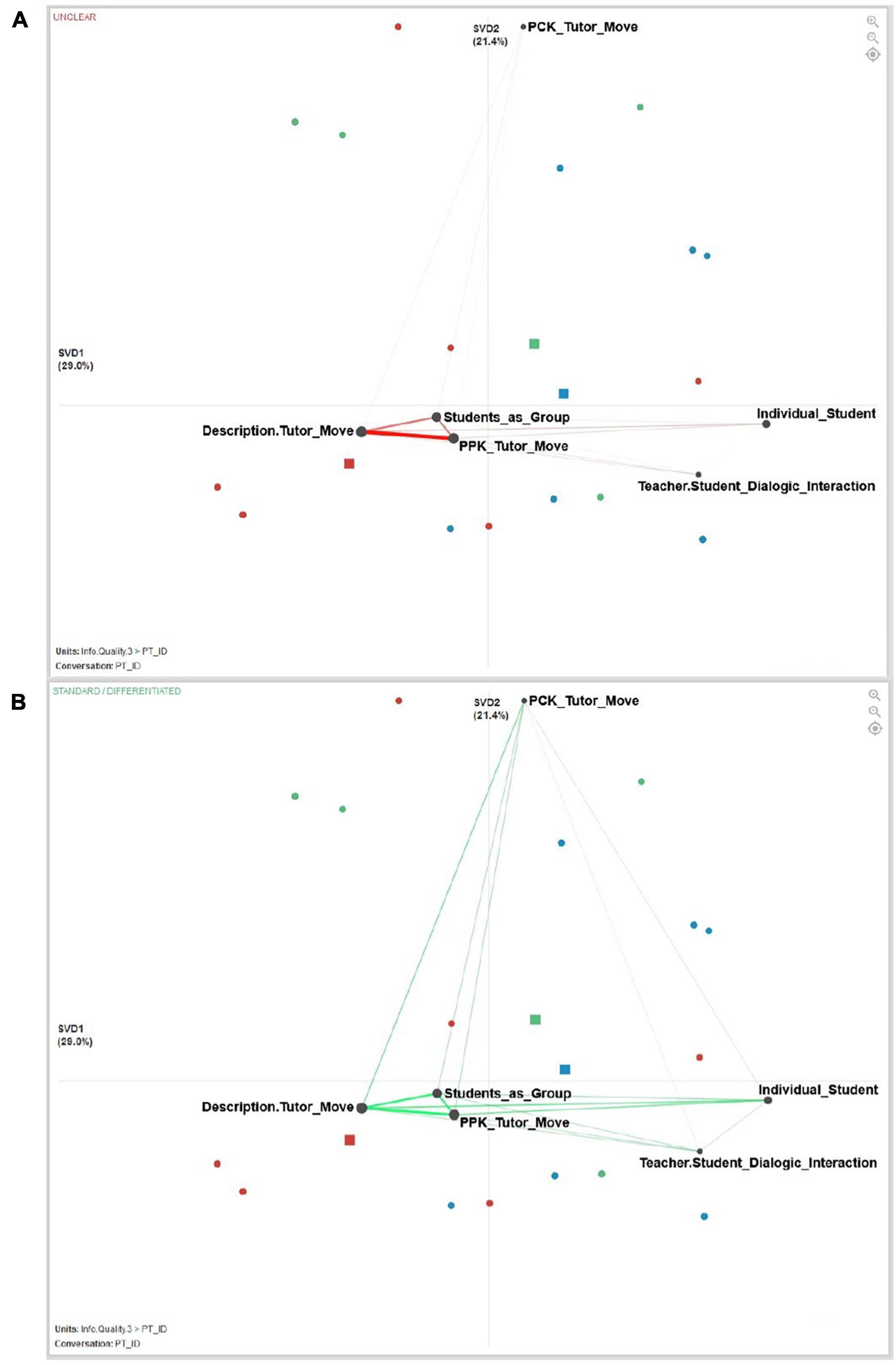
Figure 4. (A,B) Unclear and standard/differentiated describe mean networks. These Figures show the unclear quality group mean network for the describe indicators (A: top, red; n = 29 participants) and the standard/differentiated quality group mean network for the describe indicators (B: bottom, green; n = 20 participants). Nodes are depicted with black dots, while the colored dots represent the response networks for each participant. Network connections are represented with the colored lines between the nodes, where thicker lines represent more frequent connections. Only the unclear and standard/differentiated group mean networks are fully shown here to demonstrate node connection differences at the extremes, but the vague group mean network centroid (blue square) is still included in the space. As shown in Figure 3, the vague mean network mostly overlaps with the standard/differentiated mean network.
Some salient differences can be observed when comparing the unclear quality mean network (Figure 4A) with the standard/differentiated quality mean network (Figure 4B). Teacher students with lower quality response networks had most connections limited to descriptions of tutor moves concentrated on PPK. In contrast, teacher students with higher quality response networks showed many connections between all describing indicators, suggesting greater differentiation in their descriptions, and their attention to multiple relevant indicators at once.
A t-test comparing the mean positions of these networks on the X-Axis demonstrated a significant difference between the unclear network (N = 29; M = −0.93, SD = 1.12) and the standard/differentiated network (N = 20; M = 0.31, SD = 1.19), t(39.18) = 3.66, p < 0.001, with an effect size of d = 1.08. When contrasting the positioning of the mean networks and the nodes between the left and right sides of the space, this suggests that teacher students with unclear response networks had fewer connections to individual students and teacher-student interactions in their responses in contrast to teacher student response networks from the standard/differentiated group.
When comparing mean network positions along the Y-Axis, the t-test also revealed a significant difference between the unclear network (N = 29; M = 0.39, SD = 0.77) and the standard/differentiated network (N = 20; M = −0.41, SD = 1.22), t(29.45) = 2.60, p = 0.01, with an effect size of d = 0.82. Comparing the mean network and node positions between the top and bottom halves of the space, this difference suggests that teacher students’ response networks from the standard/differentiated network maintained more connections with PCK tutor moves than response networks from the unclear group.
Teacher Students’ Interpreting Skills in Tutoring Video Analysis
Content and Quality of Teacher Students’ Interpretations
The second set of research questions focused on teacher students’ interpreting skills. Results regarding the content of their interpretations (I-1) demonstrated that most teacher students’ comments provided some type of interpretation within their video analysis (no interpretation: n = 11 responses overall). At the participant level, all made at least one interpretation in four or more of their six responses. Surprisingly, most interpretations within the comments represented evidence-based explanations and/or predictions (n = 276 responses overall; all participants included at least 1 knowledge-based interpretation in 4 or more of their responses), while some inferences still lacked evidential support. These uninformed interpretations (n = 67 responses overall) included the pre-established indicator of judgmental evaluation, as well as an equally common, yet qualitatively different indicator we inductively uncovered, termed assumption (i.e., unjustified conjecture about tutor/student state of knowledge/learning). However, these uninformed interpretations were not pervasive through the whole sample. Specifically, nine participants (21.4%) did not include either uninformed interpretation in any of their responses.
In terms of the quality indicators for teacher students’ interpretations (I-2), descriptive analyses indicate that most teacher students’ analytical argumentation was of low quality (M = 0.74, SD = 0.73; word count: 2–76 words, M = 18.97, SD = 11.70). Most interpretations (n = 204 responses) were categorized under unclear or vague [n = 23 participants (54.8%) for 4 of 6 comments or more]. Table 5 displays the frequencies for each interpret indicator at the response level. Since teacher students provided six different responses in total, Figure 5 depicts the distribution of the coded categories across all six responses at the participant level.
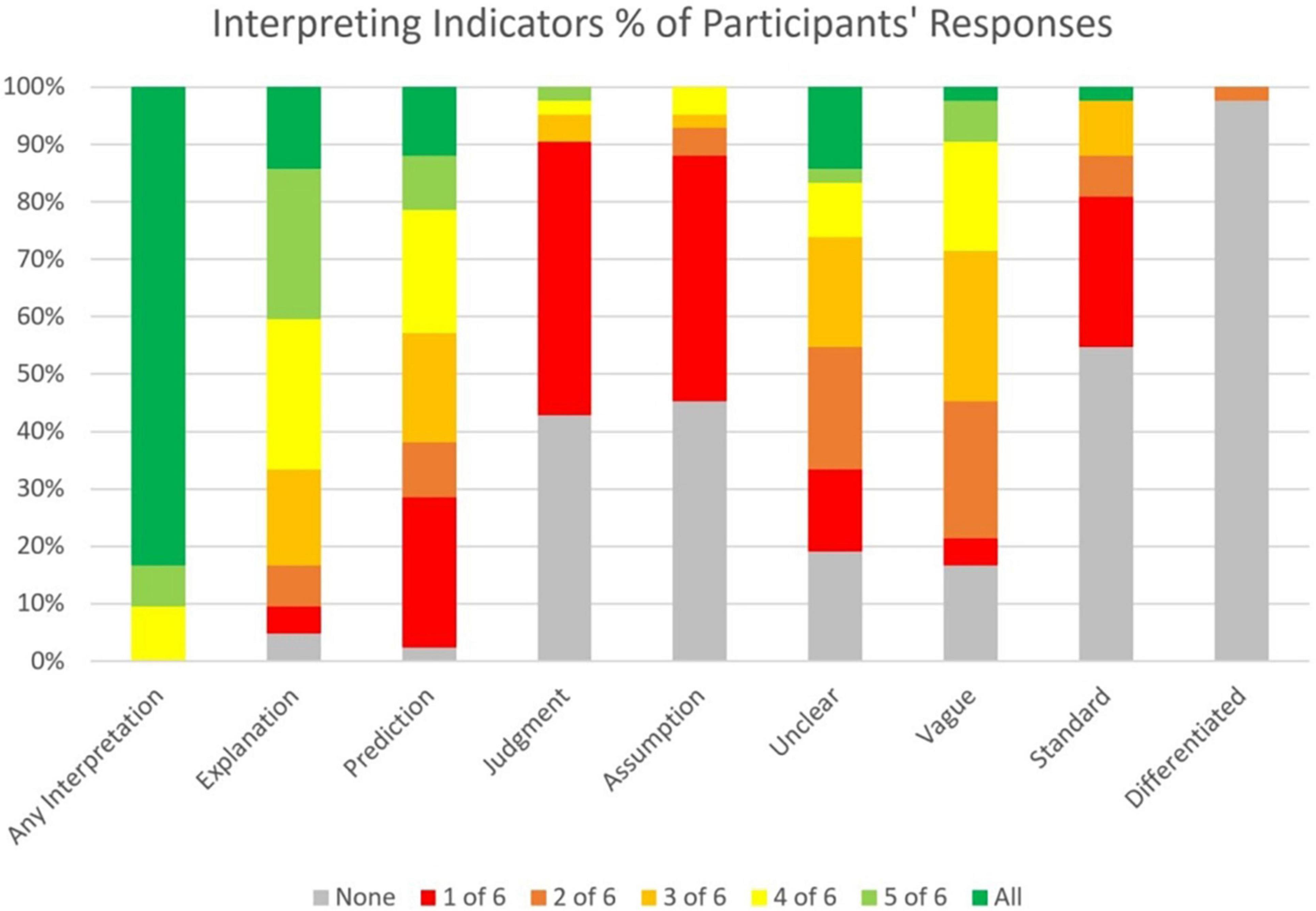
Figure 5. Proportion of interpreting indicators within participants’ responses. Teacher students’ made a total of six different video analyses. The Figure shows the proportion of each interpreting indicator present across all six interpretations of each participant. Response proportions are depicted with a red-yellow-green color scale to represent fewer to more presence of an indicator across participants’ six responses. Indicator bars demonstrate the distribution of these proportions across all participants. Results based on 240 responses from 42 participants (6 responses per participant, 12 missing responses).
Epistemic Networks of Teacher Students’ Interpretations
A closer exploration and comparison with ENA regarding the relationship configurations between interpreting content and quality indicators (I-3) demonstrated that the argumentation quality groups seemed to have moderately different structures, especially between the unclear and standard/differentiated groups (model goodness of fit for X-Axis: Pearson r = 0.93, Spearman r = 0.92; for Y-Axis: Pearson r = 0.98, Spearman r = 0.98). However, the confidence intervals of each network overlap, indicating less variance between their network structures.
Further analyzing the projection space (Figure 6), the unclear group is positioned on the left side of the space. Looking at the position of the nodes in the networks (Figures 7A,B), assumption and judgmental evaluation nodes are also on this side of the space, indicating that the teacher student response networks comprising the unclear group contain more connections to these uninformed indicators than those from the standard/differentiated group. The assumption and judgmental evaluation nodes are separated from one another in the top and bottom quadrants, indicating that participants often made connections to one or the other, but their co-occurrence was less common. In contrast, on the right side of the space, prediction and explanation nodes are positioned near each other, indicating less variance between them. This implies that teacher students’ response networks commonly had connections between these knowledge-based indicators, especially for the ones within the standard/differentiated group, also situated to the right of the projection space. The standard/differentiated group mean network’s position also indicates that teacher students’ response networks within this group contained fewer connections with judgment or assumption.
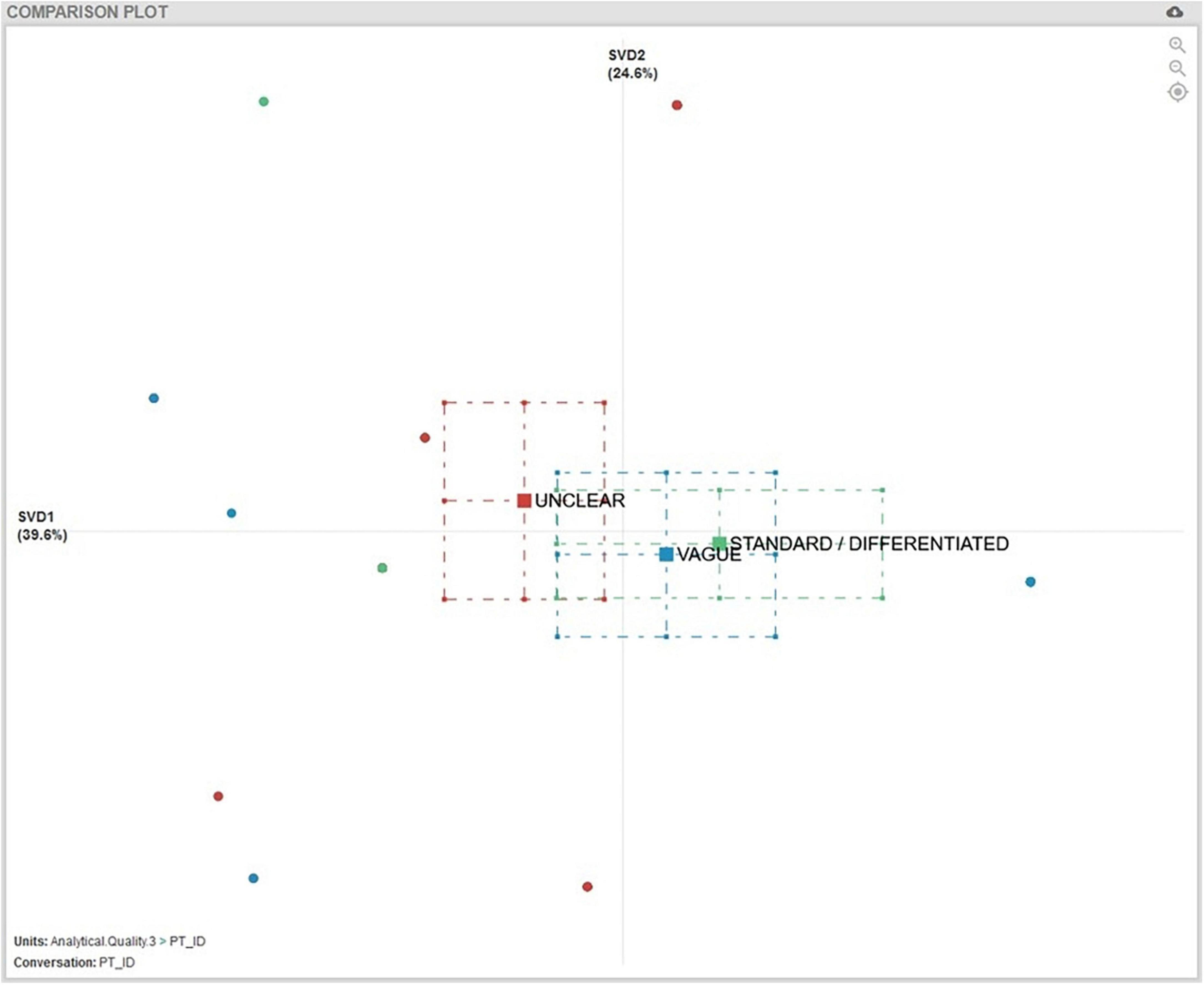
Figure 6. Position of interpret mean networks on ENA projection space. This Figure shows the ENA space for the interpret indicator quality networks and the three quality mean networks distributed on the space. Due to the lower frequency of their occurrence, the standard and differentiated quality indicators were combined into one group. Participants with at least one unclear, vague, or standard/differentiated interpretation, respectively, formed the nested quality groups. For the Interpret mean networks, Unclear n = 34 participants; Vague n = 35 participants; and Standard/differentiated n = 19 participants.
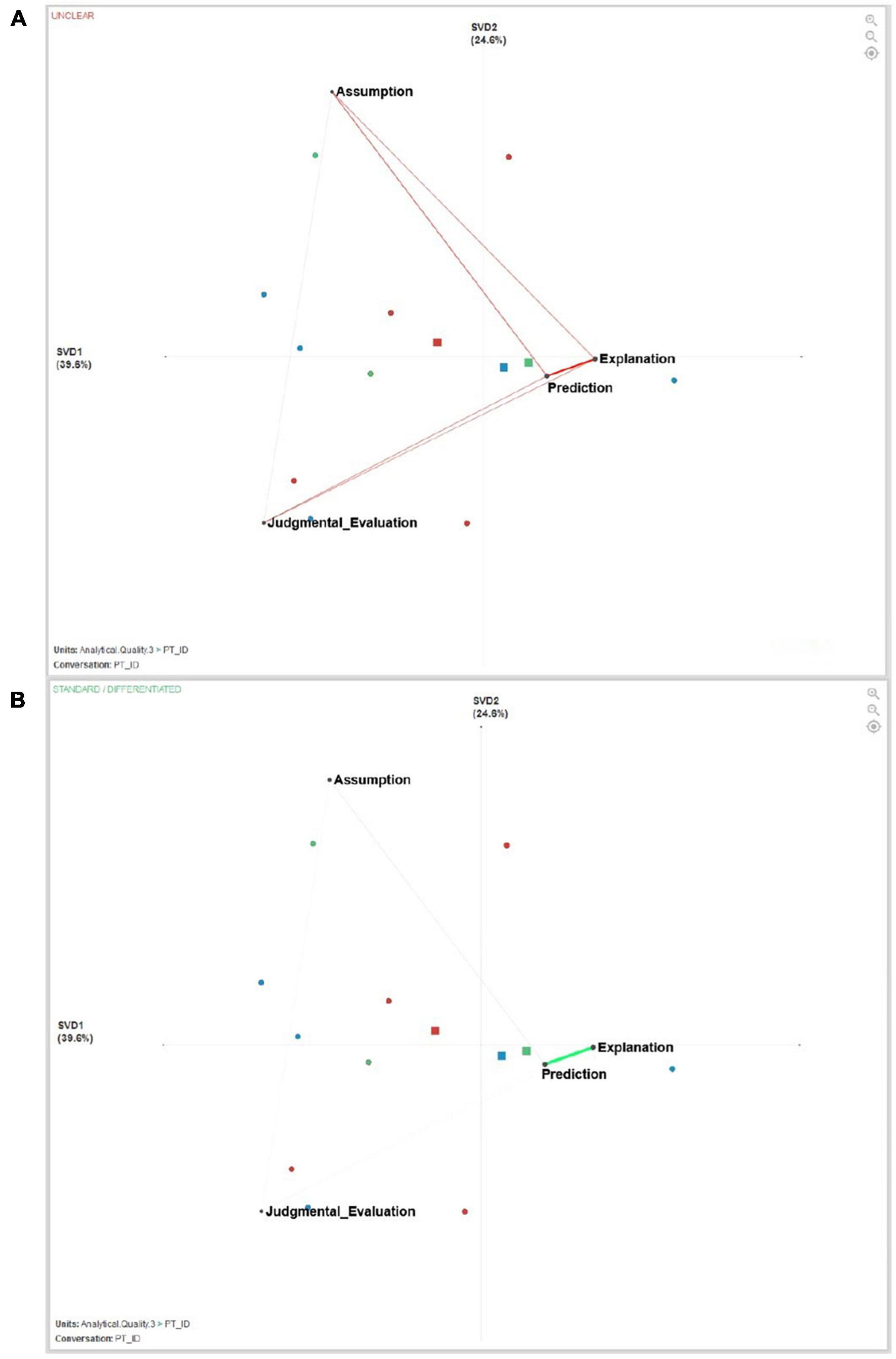
Figure 7. (A,B) Unclear and standard/differentiated interpret mean networks. These Figures show the unclear quality group mean network for the interpret indicators (A: top, red; n = 34 participants), and the standard/differentiated quality group mean network for the interpret indicators (B: bottom, green; n = 19 participants). Only the unclear and standard/differentiated mean networks are fully shown here to demonstrate differences at the extremes (the vague group mean centroid is still depicted here as the blue square). As shown in Figure 6, the vague mean network falls between the unclear and standard/differentiated mean networks.
Comparing the two networks demonstrates that most connections for the teacher students’ higher quality interpretation networks were between explanations and predictions (Figure 7B), whereas teacher students’ lower quality interpretation networks (unclear group) additionally included many connections with assumptions or judgmental evaluations (Figure 7A). A comparison of the mean positions between the unclear network (N = 34; M = −0.31, SD = 0.73) and standard/differentiated group (N = 19; M = 0.31, SD = 1.07) demonstrated a significant difference on the X-Axis t(27.45) = 2.24, p = 0.03, d = 0.72, but not on the Y-Axis. This indicates that the important distinction between teacher student quality interpretation networks involved making connections with uninformed interpretations (on the left) or not (on the right), but the type of uninformed indicator connection did not make a difference. These findings suggest that teacher students with more sophisticated PV interpretation skills typically refrained from making unjustified claims, whereas teacher students with less developed PV tended to reason about tutoring situations with a mixture of justified and baseless interpretations.
Discussion
Overview of Findings
We examined initial biology teacher students’ professional vision skills in tutoring (i.e., what and how they describe and interpret relevant events) elicited from a video-based training targeted toward novices. We observed how their skills compared with the typical novice performance, and explored the contrast of low and high quality PV skill network models.
Results regarding the features of teacher students’ describing skills on the video analysis task (D-1 to D-3) indicated support that teacher students demonstrated some typical novice patterns. Novice descriptions usually lack important details about the context, and rather remain vague and general (Jacobs et al., 2010; van Es, 2011). In this study, we also found the quality of information from most teacher students’ descriptions to be vague. In terms of the typical noticed content of novices, instead of describing content-specific student-related incidents, they generally tend to focus on salient and superficial pedagogical elements (Jacobs et al., 2010; van Es, 2011). Our results similarly reflected this pattern, with the majority of noticed events associated with PPK and almost half of participants never describing a content-specific event. Novices also typically make observations about students, not as diverse individuals, but rather as a collective group (Jacobs et al., 2010). The teacher students within our study mentioned the group of students more often, but also the majority of participants described an individual student within at least one of their noticed events. Our findings on the standard/differentiated mean describing network also suggest that some teacher students could notice and describe at higher levels (i.e., more attention to multiple relevant content areas, including individual students and PCK). Differences in low and high quality descriptions demonstrated limited versus diversified epistemic network connections.
Regarding teacher students’ interpretation skills (I-1 to I-3), results indicated some support that teacher students demonstrated typical novice patterns of interpretation. When novices interpret the events they notice, their typical patterns reflect their limits in conceptual knowledge and experience. To make sense of their observations, they characteristically demonstrate vague or oversimplified connections in their analytical arguments (Jacobs et al., 2010). Similarly, our findings indicated that teacher students’ quality of analytical argumentation remained low, with the majority of interpretations making unclear or vague connections between analytical points. In terms of novices’ typical interpretation composition patterns, they often have difficulty using theoretical knowledge or evidence from the video to justify their explanations or predictions (Santagata and Angelici, 2010; Stürmer et al., 2013b). Instead, teacher students often evaluate an event without justification by judging whether it was good or bad (e.g., Sherin and van Es, 2002; van Es, 2011), or overgeneralizing students’ learning that takes place (Jacobs et al., 2010). In our study, however, findings hinted that teacher students’ performance showed some advancements. In general, almost all teacher students’ video analyses contained at least one evidence-based interpretation in explaining and/or predicting noticed events. While over half of participants made some judgmental evaluations and/or unjustified assumptions, these uninformed interpretations were usually not prevalent across all participants’ responses and were typically mixed in with knowledge-based interpretations. With ENA, we found that differences in low and high quality interpretations could be clearly distinguished by the presence or absence of judgment and/or assumption in teacher students’ interpreting epistemic networks.
Our overall findings indicated that teacher students generally performed similarly to the typical novice portrayal of PV (e.g., Santagata et al., 2007; Jacobs et al., 2010; van Es, 2011), linking previous PV findings to our instrument and validating its utility in PV training. Interestingly though, in some circumstances, teacher students seemed to demonstrate PV skills somewhat more professionally than previous studies have found (Chan et al., 2021). With these emerging distinctions, we cautiously infer that an emphasis on the tutoring instructional context might have offered some potential affordances for teacher students’ PV performance in some areas (i.e., student-centered focus; knowledge-based interpretations).
Beyond validating this targeted video analysis task as a means for initial training of teacher students’ PV skills, we further identified a specific range and composition of tutoring PV strengths and deficits particular to initial biology teacher students at a differentiating level of granularity, even for a rather homogenous population. Moreover, concerning the growing ethical considerations for data privacy, the development and use of scripted videos within our study (e.g., use of voluntary actors), addressed this limitation from authentic classroom examples. The ENA relationship configurations among key PV indicators demonstrated the multifaceted property of the professional vision construct and provided insight into the knowledge structures at work in video analysis processes. The initial indications from this descriptive and exploratory study offer direction for further empirical testing of this instructional setting for teacher student PV training. Moreover, this baseline might also serve as a starting point for further investigation into other promising instructional support techniques.
Teacher Students’ Describing Skills
Considering the first set of research questions on describing skills more specifically (D-1 and D2), our analysis revealed that teacher students had the most difficulty attending to biology-specific tutoring moves associated with PCK, such as noticing the tutor trying to work with a student on the specific naïve preconceptions they had about the circulatory system. Instead, answers would typically focus on vague descriptions of content-general tutoring moves toward the whole group: “The tutor gives information with a diagram and explains something to the students” (vague example).
However, many participants also attended to both the group and individual students, or specified their attention toward one particular student, hinting at increased sophistication in student-centered noticing compared to previous research (e.g., Santagata et al., 2007; Jacobs et al., 2010; van Es, 2011). The video context of small-group tutoring could have made the depiction of individual students more salient to teacher students, thus making them easier to notice at the individual level. Moreover, the emphasis on the tutoring context could have helped reduce teacher students’ cognitive load, thus enabling them to process more specific details of tutor-student exchanges (Mayer and Moreno, 2010). Perhaps teacher students would have had even more focus on the individual students, if the aim of the task was directed toward them more specifically. Further research within the context of the present study could investigate whether an emphasis shift from tutor to student could improve teacher students’ focus on individual students’ content-specific concerns.
With respect to the relationships between teacher students’ describing content and quality (D-3), with network analysis we could identify that higher quality descriptions attended to multiple facets of the noticed event: “The tutor takes the worksheet and describes that there is a lung and a body cycle. This description follows from one female student’s statement that there are upper/lower cycles. The tutor thereby looks at the student who initially had put forward the 2-cycle hypothesis” (differentiated example). Compared to the vague example mentioned earlier, this differentiated response demonstrates the use of elaborative, content-specific details to clearly depict what is taking place in the video during this event.
Getting an understanding of teacher students’ describing skills within a particular video analysis task affords researchers and teacher educators the opportunity to see novice teachers’ knowledge structures at work (Dreher and Kuntze, 2015; Dick, 2017). The deliberate attending toward particular events in a video example demonstrated teacher students’ awareness of the event and its importance to the analysis task goal. The description told us whether novices could make sense of what they observed in terms of what knowledge they could activate, and how they applied it toward identifying relevant situations (Star and Strickland, 2008; Stockero and van Zoest, 2013). Higher quality descriptions made more connections across multiple relevant indicators, likely demonstrating more sophisticated knowledge integration.
However, one area where most teacher students struggled was with PCK. When looking at our participants’ university coursework experience, in contrast to biology content and general (psychological) pedagogical classes, the majority of teacher students had little experience (and assumed prior knowledge) with regard to teaching biology. It is within these biology teaching and didactics courses that students build PCK knowledge (e.g., learn about common naïve preconceptions). Thus, it is likely that PCK tutoring moves in particular, were more difficult for our participants to notice, due to the sample-specific limits in overall PCK prior knowledge.
Still, the implications of the findings suggest a particular need in biology teacher education for support in developing PCK and having ample opportunity for domain-specific observation, analysis, and practice during their teacher training (e.g., Barnhart and van Es, 2015; Kang and Anderson, 2015). Exposure and application with multiple models through video analysis training might help students more clearly differentiate what information is relevant and what can be ignored (Haider and Frensch, 1996). In addition to PCK, like much of the research in teacher noticing, there is a need for more emphasis on individual students and types of teacher-student interactions involving subject-specific exchange (e.g., von Kotzebue et al., 2021).
Moreover, the more sophisticated teacher student PV networks offer a model PV for this population to strive toward. The interconnectedness of core practice elements within their networks seems to reflect more integrated PV mental models. For PV development toward this aim, building the knowledge and skills for noticing multiple important components at once may be supported with iterations of training that focus on one particular element at a time (i.e., emphasis manipulation at a lower level of granularity). One successful example can be seen with the Lesson Analysis Framework (Santagata et al., 2007), where teacher students watch a video clip three times, each with a different focus (e.g., lesson structure/goals, student thinking/learning, and teaching alternatives). A target video analysis task modeled after the present study could be flexibly extended in this way to focus the analysis on distinctive components with each viewing, or build in complexity by aiming toward noticing multiple foci simultaneously.
Teacher Students’ Interpreting Skills
Reflecting on the second set of research questions regarding PV interpreting skills, for our first research question on the content of teacher students’ interpretations (I-1), we observed that most teacher students’ video analyses provided some type of interpretation. Surprisingly, however, the majority of these were evidence-based. In contrast to previous literature indicating that novices tend to simply describe, or make attempts at interpretation with judgment (e.g., Sherin and van Es, 2009), the majority of study participants made interpretations with knowledge-based explanations, predictions, or both.
Teacher students’ somewhat advanced interpreting skills might have been partially influenced by the provision of the introductory texts as a pre-training (Mayer et al., 2002). The texts aimed to lend support by offering a focused, knowledge-based resource for direct application within the analysis task (see Martin et al., 2021). Moreover, the task design organized the text reading just before video viewing, perhaps contributing to recency recall (Zheng and Zhau, 2006). The emphasized instructional context of tutoring, may have also lent support for teacher students’ interpretations by depicting interactions with a smaller group of students. Perhaps this made video-based evidence more salient, in contrast to full classroom video examples. The combination of these design features might have made it easier for teacher students to make both theoretical and video-based connections to their observations. However, we estimate that this potential boost is limited, especially regarding the question of transfer over time. Nevertheless, these features deliver a nice hint on how teacher educators might instantly bring novices toward (at least) a slightly higher level of interpretation in PV training.
For further inquiry, we recommend investigation into particular aspects of video analysis task design that align with suggested principles of multimedia learning with video, such as visually signaling particular events, presenting videos in an event-segmented format, or analysis rehearsal techniques such as targeted self-reflection prompts (Mayer and Moreno, 2010; Derry et al., 2014; Martin et al., 2022). We suggest these design components could play a part in further supporting initial teacher students in their attempts at noticing and making sense of relevant events.
In terms of our second research question on participants demonstrating the typical novice patterns for interpretation quality (I-2), teacher students’ performance was low, as expected. This finding indicates that teacher students struggled to make (multiple) clear and logical deep connections between the events they noticed and their interpretations of them using video or text evidence to justify their claims. Even when they did, their arguments mostly remained vague, only somewhat linked to their observations. This suggests the pre-training texts could not fully make up the difference for teacher students’ prior knowledge deficits (especially for PCK), which likely limited their abilities to make theoretical connections beyond the surface level.
With reference to our third research question exploring and contrasting the relationships between interpretation content and quality (I-3), while most teacher students still struggled to make high quality arguments, our network analysis revealed that the differentiating components in interpretation quality were not that they did not use evidence at all, but rather that they sometimes used evidence while also making uninformed claims. These unjustified claims took the form of judgmental evaluations (e.g., “The tutor tries to explain it to the students by means of metaphors, but in my opinion uses too complicated and unmanageable examples, which could lead to the students being even more confused or uncertain than before”), and unexpectedly, a second type of uninformed interpretation which emerged from responses: assumptions about learning. These assumptions typically corresponded to Graesser et al.’s (2009) “illusions” of learning, for example, the illusions of knowledge mastery and transfer, wherein the tutor assumes the student completely understands his/her explanation about the content and thus can accurately transfer this knowledge (e.g., “The teacher responds to knowledge gaps he has identified. Students think about it again and deepen their knowledge and improve themselves”).
The network analysis further demonstrated that lower quality interpretations typically stated one type of uninformed interpretation, or the other, but rarely both. Perhaps this is the case because both interpretations are heuristic devices, used to quickly make sense of new situations based on (limited) experiential knowledge of similar situations or patterns (see value judgment; Scriven, 1972; and tacit assumption; Turner, 2012). Thus, if a teacher student had limited knowledge to draw from, the use of one heuristic would be enough to satisfy the task.
The results from our research questions on teacher students’ interpretations indicate the need for further training and support for teacher students in both professional observation practices, and knowledge building with regard to student understanding and evidence of learning. Judgmental reactions could be tempered with sensitivity training, according to Mason’s (2002) noticing strategies: remain neutral in observations; be sensitive to students’ subject-specific comprehension; and look for teachable moments that arise to “act freshly.” Additionally, a video-based reflection course could provide support for both shortcomings. One example comes from the Learning to Learn from Teaching course for preservice math teachers (van Es et al., 2017). Teacher students were first introduced to various noticing frameworks for math teaching, which were then applied in iterations of video-based analysis practice, emphasizing evidence artifacts to increase accuracy, elaboration, and integration.
Researchers and teacher educators alike can gain a deeper understanding of novice-level core teaching competencies by examining how well teacher students can apply, transfer, and reconstruct their knowledge (König et al., 2014) in their interpretations of varied examples of practice. Specifically, they can pinpoint what specific PPK and/or PCK is activated around noticed practices, and the content and quality of these mental representations demonstrated within their reasoning (Seidel and Stürmer, 2014; König et al., 2018). Interpretation sophistication can be observed from key PV indicators, such as knowledge-based explanations and predictions that use evidence from the video or their knowledge of teaching and learning principles to make clear and logical connections to what they noticed (i.e., PCK moves, individual students, teacher-student interactions). The depth of their interpretations can demonstrate where teacher students lie on the continuum of understanding, from general and simple to comprehensive and integrated (Kersting, 2008).
Limitations
One major goal in this study was to describe and examine a baseline measure of initial teacher students’ tutoring PV to validate this focused context as a population-appropriate medium for PV training. Results offered substantial evidence that the teacher students from our investigation seemed to demonstrate PV skills in noticing and interpreting similar to typical novice PV patterns. Further, findings hinted that teacher students sometimes performed at higher-than-typical levels, conceivably due to design elements tailored toward novice learning (i.e., emphasis on the tutoring context within scripted video representations; pre-training support from the introductory texts). However, we can only tentatively attribute these advances to elements of our study design due to the descriptive and exploratory nature of our investigations. Thus, we acknowledge the possibility that these outcomes could be the result of other factors. Perhaps after watching the full video, the feature of re-watching the noticed event clip before analysis helped teacher students to remember more specific details of the event, or make evidence-based interpretations based on their knowledge of the full-video context. Further research is necessary to substantiate any causal claims about the aspect(s) of the design that may have contributed to teacher students’ PV support.
Beyond limits to study design claims, the focus on tutoring, may have limited the scope of teacher students PV, with respect to this construct’s broader conceptualization in the field. The focus of teacher students’ analysis centered on the tutor and the particular pedagogy displayed in the videos. In much of the teacher noticing literature, however, the PV focus is typically on subject-matter student thinking (e.g., Chan et al., 2021; Santagata et al., 2021). While we agree that teacher students’ training of core practices should focus on student thinking and learning, we argue that for initial teacher students, a first step in this process is learning to notice particular (student-centered) teaching strategies in action and connecting these moves to one’s own conceptual pedagogical knowledge. With teacher students’ development of various mental models of practice, further training can build on this foundation to integrate the teacher-student dialectic and a student-centered focus. For example, a series of trainings could be implemented, with shifts in PV focus (Gopher, 2007), similar to the Learning and Teaching Geometry teacher professional development (Borko et al., 2011), which effectively trained multiple perspective noticing with a sequence of targeted video viewings and group discussions.
Finally, we acknowledge the role that participants’ prior knowledge may have played in their training performance and our limitation to disentangle it statistically. In terms of participants’ current study status, with 81% attending six semesters at university or less (and averaging only 2–3 semesters), we assumed participants’ were at the novice end of the teacher-education spectrum, thus appropriate candidates for our training. At the level of granularity that PV was analyzed for this study, it could be argued that in capturing the elements of participants’ tutoring PV Big-D Discourse (Gee, 1999, 2014), we were also measuring the sophistication of their applied knowledge. However, since we integrated a pre-training text-for-application into our design, our PV measure of knowledge likely represents a mixture of the knowledge from the text, along with participants’ relevant prior knowledge. Due to our more general and nominal-level measurements of participants’ prior knowledge and experience, we were limited in our ability to separate the differential impact these two knowledge variables might have played in participants’ PV performance.
To counter this, we randomly assigned the three pre-training texts to help mitigate any sample-wide discrepancies in participant’s prior knowledge. This ensured a balanced distribution of different types of knowledge for application in the video analyses at the sample level. While this may have diminished the overall impact of prior knowledge differences, we acknowledge that this may not have been the case for participants with very limited knowledge or coursework experiences (e.g., PCK, biology teaching and didactics). If just before analyzing a video, reading about particular tutoring strategies was a participants’ first introduction to the concept(s), the text support may not have been enough to mitigate their likely overwhelmed processing capacities to perform the task successfully (Cook, 2006; Kirschner et al., 2011). Thus, future studies should be more cognizant of the role prior knowledge plays in participants’ PV performance, especially for teacher students. Further, they should take intentional methodological steps to capture study-specific interval measures of prior knowledge and teaching experience so that their influence can be analyzed statistically.
Conclusion
In line with increasing teacher education reform efforts to integrate practice-based training and support (Ball and Forzani, 2009; McDonald et al., 2013), one promising approximation of practice is the analysis of video. In these trainings, teacher students apply their conceptual knowledge to notice and make sense of relevant events, building their professional vision (van Es and Sherin, 2008; Seidel and Stürmer, 2014). With growing evidence of the effectiveness of video analysis for PV training (Gaudin and Chaliès, 2015; Chan et al., 2021; Santagata et al., 2021), it was still unclear whether an emphasized instructional context, such as tutoring, could support initial teacher students in beginning their development of this complex skill.
Overall, the present study makes the following contribution to the literature:
First, our findings demonstrated that teacher students’ PV skills in describing and interpreting noticed tutoring events seemed to parallel typical novice PV performance. This suggests that using scripted video representations of tutoring scenarios within a video analysis task is an appropriate context for initial teacher student PV training of core instructional practices.
Secondly, design aspects potentially allow a head start for teacher students to demonstrate more sophisticated skills than expected in their video analysis. The emphasis on tutoring seemed to help teacher students focus more on individual students, while the short introductory texts might scaffold teacher students in their use of knowledge-based interpretations. While these indications are promising, further investigations are still needed for this new stimulus. Accordingly, we hope to inspire development and exploration into the potential affordances of (further) instructional design aspects that might offer support to initial teacher students in similar contexts.
Third, in recommending further support, our results demonstrate that aspects of PCK were much more demanding on teacher students than PPK. With this in mind, biology teacher educators need to be aware of teacher students’ limits in PCK knowledge. They can support teacher students’ skill development by offering numerous opportunities and diverse experiences with domain-specific models and teaching examples. Further, we suggest offering these experiences in combination with targeted theoretical explanations (e.g., in an introductory text) about the instructional methodology to observe and analyze from the perspective of both the teacher and student(s).
Fourth, ENA was a valuable analysis tool in this study to visualize, explore and contrast the complex, interconnected relationships of participants’ describing and interpreting PV skills in tutoring. Network depictions helped to uncover salient relationships, while network comparisons made notable differences more clearly visible in comparison to individual component frequency measures. With ENA, we were able to better understand the sophistication of participants’ professional epistemic frames (Shaffer et al., 2016) to see where further support is still needed in the development of their PV knowledge (e.g., noticing and interpreting content-specific tutoring events) and its integration into their mental models of tutoring (student-centered) instruction.
Finally, we see our approximation of practice for novice learners as an example training methodology that could be flexibly integrated within teacher education seminars to initiate connections between conceptual understanding of core practice pedagogy and its representation-in-action, exemplified in tutoring scenarios. Moreover, this training could be adapted into a series of multiple practices, with the option of implementing or modifying various design features (e.g., focus shift or additional pre-training, metacognitive supports, manipulations to video presentation, extend analysis to classroom discussion, etc.) and systematically reducing them as needed to support teacher students’ learning and mastery (Blomberg et al., 2013; Roth McDuffie et al., 2014; Kang and van Es, 2019). The opportunities from this flexible training can help to mitigate the theory-practice divide (Cochran-Smith et al., 2015), supporting teacher students to initiate a foundation of practical knowledge and skills, to expand on and further integrate throughout their teacher training and practice.
Data Availability Statement
The datasets presented in this article are not readily available because as requested by the DFG, the data will be made available to other interested researchers upon request after completion of the project in 2022. Requests to access the datasets should be directed to MF, meg.farrell@tum.de.
Ethics Statement
The studies involving human participants were reviewed and approved by The Ethics Commission of the German Psychological Society (DGPs), and The Data Protection Office at the Technical University of Munich. The participants provided their written informed consent to participate in this study. Written informed consent was obtained from the individual(s), and minor(s)’ legal guardian/next of kin, for the publication of any potentially identifiable images or data included in this article.
Author Contributions
AR, WR, and TS contributed to the conceptualization of study ideas in terms of the formulation and evolution of overarching research goals and aims and were involved with study funding acquisition and project administration. MF, MM, AR, WR, KK, JM, and TS participated in the development and/or design of the study’s methodology. MF, MM, AR, WR, and TS contributed to the provision of study material resources. MF and MM conducted data collection, as well as database curation and management activities for study data. MF performed the formal analysis in the application of statistical, and other formal analysis/synthesis techniques of study data. MF wrote the initial manuscript draft, involving preparation, creation and presentation of original work, under the supervision of TS. MM, AR, WR, KK, JM, and TS participated in reviewing and editing the written manuscript, specifically in terms of offering critical content review and commentary. All authors contributed to manuscript revision, reading and approval of the submitted version.
Funding
This work was supported by the German Research Foundation (DFG) under Grant: SE 1397/12-1, number 393875851; and ENA analysis methods were funded in part by the National Science Foundation under Grant: DRL-1661036, DRL-1713110, and DRL-2100320, the Wisconsin Alumni Research Foundation, and the Office of the Vice Chancellor for Research and Graduate Education at the University of Wisconsin-Madison.
Author Disclaimer
The opinions, findings, and conclusion do not reflect the views of the funding agencies, cooperating institutions, or other individuals.
Conflict of Interest
The authors declare that the research was conducted in the absence of any commercial or financial relationships that could be construed as a potential conflict of interest.
Publisher’s Note
All claims expressed in this article are solely those of the authors and do not necessarily represent those of their affiliated organizations, or those of the publisher, the editors and the reviewers. Any product that may be evaluated in this article, or claim that may be made by its manufacturer, is not guaranteed or endorsed by the publisher.
Acknowledgments
Firstly, we thank the helpful and constructive comments from the Associate Editor, AT, and from the reviewers of this manuscript, which facilitated significant improvements to the text. We would also like to thank study participants for their efforts on our video analysis task. Additionally, we also thank Laura Pflieger for her diligent assistance in data coding, and Katharina Pichler for support in initial code development. Finally, we thank the Epistemic Analytics Lab at University of Wisconsin-Madison for ENA support.
Abbreviations
ENA, epistemic network analysis; PCK, pedagogical content knowledge; PPK, pedagogical and psychological knowledge; PV, professional vision; T-S interaction, teacher-student dialogic interaction; T&L, teaching and learning.
Footnotes
- ^ Within the literature of teacher training, the terms teacher noticing and professional vision are both used to denote quite similar, overlapping constructs. This paper is relevant to research under both labels. However, to maintain consistency, the term professional vision will be used throughout.
- ^ The sample of focus chosen for this paper’s finer-grained investigation is part of a larger project. See Martin et al. (2021) for further investigations.
- ^ A high proportion of women is typical for teacher training programs in Germany.
- ^ Due to the methodological restrictions for research, we had to limit participants’ video viewing options to maintain consistency of the training session for everyone. However, if this training were embedded within a teacher education seminar, we would encourage the instructor to omit these limitations, so that students could take advantage of the benefits of pausing and playback that the video medium offers. Accordingly, several participants expressed this wish within the overall feedback they gave us at the end of the study.
- ^ A prevalence index closer to | 1 | indicates a higher likelihood of prevalence present in the Kappa statistic.
References
Ball, D. L., and Forzani, F. M. (2009). The work of teaching and the challenge for teacher education. J. Teach. Educ. 60, 497–511. doi: 10.1177/0022487109348479
Barnhart, T., and van Es, E. (2015). Studying teacher noticing: examining the relationship among pre-service science teachers’ ability to attend, analyze and respond to student thinking. Teach. Teach. Educ. 45, 83–93. doi: 10.1016/j.tate.2014.09.005
Bauer, E., Fischer, F., Kiesewetter, J., Shaffer, D. W., Fischer, M. R., Zottmann, J. M., et al. (2020). Diagnostic activities and diagnostic practices in medical education and teacher education: an interdisciplinary comparison. Front. Psychol. 11:562665. doi: 10.3389/fpsyg.2020.562665
Berliner, D. C. (2001). Learning about learning from expert teachers. Int. J. Educ. Res. 35, 463–482. doi: 10.1016/S0883-0355(02)00004-6
Blomberg, G., Renkl, A., Gamoran-Sherin, M., Borko, H., and Seidel, T. (2013). Five research-based heuristics for using video in pre-service teacher education. J. Educ. Res. Online 5, 90–114.
Borko, H., Jacobs, J., Eiteljorg, E., and Pittman, M. E. (2008). Video as a tool for fostering productive discussions in mathematics professional development. Teach. Teach. Educ. 24, 417–436. doi: 10.1016/j.tate.2006.11.012
Borko, H., Jacobs, J., Seago, N., and Mangram, C. (2014). “Facilitating video-based professional development: planning and orchestrating productive discussions,” in Transforming Mathematics Instruction: Multiple Approaches and Practices, eds Y. Li, E. Silver, and S. Li (Cham: Springer), 259–281. doi: 10.1186/s40594-017-0086-z
Borko, H., Koellner, K., Jacobs, J., and Seago, N. (2011). Using video representations of teaching in practice-based professional development programs. ZDM Int. J. Math. Educ. 43, 175–187. doi: 10.1007/s11858-010-0302-5
Bowman, D., Swiecki, Z., Cai, Z., Wang, Y., Eagan, B., Linderoth, J., et al. (2021). “The mathematical foundations of epistemic network analysis,” in Advances in Quantitative Ethnography: Third International Conference, ICQE 2021, Virtual Event, eds B. Wasson and S. Zörgö (Cham: Springer), 91–105.
Broekkamp, H., and van Hout-Wolters, B. (2007). The gap between educational research and practice: a literature review, symposium, and questionnaire. Educ. Res. Eval. 13, 203–220. doi: 10.1080/13803610701626127
Brophy, J. (2006). “Observational research on generic aspects of classroom teaching,” in Handbook of Educational Psychology, 2nd Edn, eds P. A. Alexander, P. H. Winne, E. Anderman, and L. Corno (New York, NY: Routledge), 755–780.
Brophy, J. E. (ed.) (2004). Using Video in Teacher Education: Advances in Research on Teaching, Vol. 10. Bingley: Emerald Group Publishing Ltd.
Byrt, T., Bishop, J., and Carlin, J. B. (1993). Bias, prevalence and kappa. J. Clin. Epidemiol. 46, 423–429. doi: 10.1016/0895-4356(93)90018-v
Cade, W. L., Copeland, J. L., Person, N. K., and D’Mello, S. K. (2008). “Dialogue modes in expert tutoring,” in Lecture Notes in Computer Science: Intelligent Tutoring Systems: 9th International ITS Conference Proceedings, eds B. P. Woolf, E. Aïmeur, R. Nkambou, and S. Lajoie (Berlin: Springer), 470–479. doi: 10.1007/978-3-540-69132-7_50
Chan, K. K. H., Xu, L., Cooper, R., Berry, A., and van Driel, J. H. (2021). Teacher noticing in science education: Do you see what I see? Stud. Sci. Educ. 57, 1–44. doi: 10.1080/03057267.2020.1755803
Chi, M. T. (1996). Constructing self-explanations and scaffolded explanations in tutoring. Appl. Cogn. Psychol. 10, 33–49. doi: 10.1002/(sici)1099-0720(199611)10:7<33::aid-acp436>3.0.co;2-e
Chi, M. T. H., Siler, S. A., and Jeong, H. (2004). Can tutors monitor students’ understanding accurately? Cogn. Instr. 22, 363–387. doi: 10.1207/s1532690xci2203_4
Chi, M. T. H., Siler, S. A., Jeong, H., Yamauchi, T., and Hausmann, R. G. (2001). Learning from human tutoring. Cogn. Sci. 25, 471–533. doi: 10.1207/s15516709cog2504_1
Cochran-Smith, M., Villegas, A. M., Abrams, L., Chavez-Moreno, L., Mills, T., and Stern, R. (2015). Critiquing teacher preparation research: An overview of the field, part II. J. Teach. Educ. 66, 109–121. doi: 10.1177/0022487114558268
Cohen, D. K., and Ball, D. L. (1999). Instruction, Capacity, and Improvement. CPRE Research Report Series (RR-43). Consortium for Policy Research in Education. Washington, DC: Office of Educational Research and Improvement.
Cohen, J. (1960). A coefficient of agreement for nominal scales. Educ. Psychol. Meas. 20, 37–46. doi: 10.1177/001316446002000104
Cohen, P. A., Kulik, J. A., and Kulik, C.-L. C. (1982). Educational outcomes of tutoring: a meta-analysis of findings. Am. Educ. Res. J. 19, 237–248. doi: 10.3102/00028312019002237
Cook, M. P. (2006). Visual representations in science education: The influence of prior knowledge and cognitive load theory on instructional design principles. Sci. Educ. 90, 1073–1091. doi: 10.1002/sce.20164
Csanadi, A., Eagan, B., Kollar, I., Shaffer, D. W., and Fischer, F. (2018). When coding-and-counting is not enough: using epistemic network analysis (ENA) to analyze verbal data in CSCL research. Int. J. Comput. Support. Collab. Learn. 13, 419–438. doi: 10.1007/s11412-018-9292-z
Darling-Hammond, L. (2006). Constructing 21st-century teacher education. J. Teach. Educ. 57, 300–314. doi: 10.1177/0022487105285962
Derry, S. J., Sherin, M. G., and Sherin, B. L. (2014). “Multimedia learning with video,” in Cambridge Handbooks in Psychology: The Cambridge Handbook of Multimedia Learning, ed. R. E. Mayer (Cambridge: Cambridge University Press), 785–812. doi: 10.1017/CBO9781139547369.038
Di Eugenio, B., and Glass, M. (2004). Squibs and discussions. The kappa statistic: a second look. Comput. Linguist. 30, 95–101. doi: 10.1162/089120104773633402
Dick, L. K. (2017). “Investigating the relationship between professional noticing and specialized content knowledge,” in Research in Mathematics Education. Teacher Noticing: Bridging and Broadening Perspectives, Contexts, and Frameworks, eds E. O. Schack, M. H. Fisher, and J. A. Wilhelm (Cham: Springer), 339–358.
Dreher, A., and Kuntze, S. (2015). Teachers’ professional knowledge and noticing: the case of multiple representations in the mathematics classroom. Educ. Stud. Math. 88, 89–114. doi: 10.1007/s10649-014-9577-8
Gaudin, C., and Chaliès, S. (2015). Video viewing in teacher education and professional development: a literature review. Educ. Res. Rev. 16, 41–67. doi: 10.1016/j.edurev.2015.06.001
Gee, J. P. (1999). An Introduction to Discourse Analysis: Theory and Method. New York, NY: Routledge.
Gee, J. P. (2014). Unified Discourse Analysis: Language, Reality, Virtual Worlds, and Video Games. New York, NY: Routledge.
Goeze, A., Zottmann, J. M., Vogel, F., Fischer, F., and Schrader, J. (2014). Getting immersed in teacher and student perspectives? Facilitating analytical competence using video cases in teacher education. Instr. Sci. 42, 91–114. doi: 10.1007/s11251-013-9304-3
Gopher, D. (2007). “Emphasis change as a training protocol for high-demand tasks,” in Attention: From Theory to Practice, eds A. Kramer, D. Wiegmann, and A. Kirlik (Oxford: Oxford University Press), 209–224.
Graesser, A. C., D’Mello, S., and Cade, W. (2011). “Instruction based on tutoring,” in The Educational Psychology Handbook Series: Handbook of Research on Learning and Instruction, eds R. E. Mayer and P. A. Alexander (New York, NY: Routledge), 408–426.
Graesser, A. C., D’Mello, S., and Pearson, N. (2009). “Meta-knowledge in tutoring,” in The Educational Psychology Handbook Series: Handbook of Metacognition in Education, eds D. J. Hacker, J. Dunlosky, and A. C. Graesser (New York, NY: Routledge), 361–382.
Graesser, A. C., and Person, N. K. (1994). Question asking during tutoring. Am. Educ. Res. J. 31, 104–137. doi: 10.3102/00028312031001104
Grossman, P., Compton, C., Igra, D., Ronfeldt, M., Shahan, E., and Williamson, P. (2009). Teaching practice: a cross-professional perspective. Teach. Coll. Rec. 111, 2055–2100. doi: 10.1177/016146810911100905
Grossman, P. (ed.) (2018). Teaching Core Practices in Teacher Education. Cambridge, MA: Harvard Education Press.
Großschedl, J., Harms, U., Kleickmann, T., and Glowinski, I. (2015). Preservice biology teachers’ professional knowledge: Structure and learning opportunities. J. Sci. Teach. Educ. 26, 291–318. doi: 10.1007/s10972-015-9423-6
Haider, H., and Frensch, P. A. (1996). The role of information reduction in skill acquisition. Cogn. Psychol. 30, 304–337. doi: 10.1006/cogp.1996.0009
Hallgren, K. A. (2012). Computing inter-rater reliability for observational data: an overview and tutorial. Tutor. Quant. Methods Psychol. 8, 23–34. doi: 10.20982/tqmp.08.1.p023
Herppich, S., Wittwer, J., Nückles, M., and Renkl, A. (2013). Does it make a difference? Investigating the assessment accuracy of teacher tutors and student tutors. J. Exp. Educ. 81, 242–260. doi: 10.1080/00220973.2012.699900
Jacobs, V. R., Lamb, L. L. C., and Philipp, R. A. (2010). Professional noticing of children’s mathematical thinking. J. Res. Math. Educ. 41, 169–202. doi: 10.5951/jresematheduc.41.2.0169
Janssen, F., Grossman, P., and Westbroek, H. (2015). Facilitating decomposition and recomposition in practice-based teacher education: the power of modularity. Teach. Teach. Educ. 51, 137–146. doi: 10.1016/j.tate.2015.06.009
Kalyuga, S. (2009). Knowledge elaboration: a cognitive load perspective. Learn. Instr. 19, 402–410. doi: 10.1016/j.learninstruc.2009.02.003
Kang, H., and Anderson, C. W. (2015). Supporting preservice science teachers’ ability to attend and respond to student thinking by design. Sci. Educ. 99, 863–895. doi: 10.1002/sce.21182
Kang, H., and van Es, E. A. (2019). Articulating design principles for productive use of video in preservice education. J. Teach. Educ. 70, 237–250. doi: 10.1177/0022487118778549
Kaufman, D. M., and Holmes, D. B. (1996). Tutoring in problem-based learning: perceptions of teachers and students. Med. Educ. 30, 371–377. doi: 10.1111/j.1365-2923.1996.tb00850.x
Kersting, N. (2008). Using video clips of mathematics classroom instruction as item prompts to measure teachers’ knowledge of teaching Mathematics. Educ. Psychol. Meas. 68, 845–861. doi: 10.1177/0013164407313369
Kersting, N. B., Givvin, K. B., Sotelo, F. L., and Stigler, J. W. (2010). Teachers’ analyses of classroom video predict student learning of mathematics: further explorations of a novel measure of teacher knowledge. J. Teach. Educ. 61, 172–181. doi: 10.1177/0022487109347875
Kirschner, P. A., Ayres, P., and Chandler, P. (2011). Contemporary cognitive load theory research: the good, the bad and the ugly. Comput. Hum. Behav. 27, 99–105. doi: 10.1016/j.chb.2010.06.025
Kleickmann, T., Richter, D., Kunter, M., Elsner, J., Besser, M., Krauss, S., et al. (2013). Teachers’ content knowledge and pedagogical content knowledge: the role of structural differences in teacher education. J. Teach. Educ. 64, 90–106. doi: 10.1177/0022487112460398
Kloser, M. (2014). Identifying a core set of science teaching practices: a Delphi expert panel approach. J. Res. Sci. Teach. 51, 1185–1217. doi: 10.1002/tea.21171
König, J., Blömeke, S., Klein, P., Suhl, U., Busse, A., and Kaiser, G. (2014). Is teachers’ general pedagogical knowledge a premise for noticing and interpreting classroom situations? A video-based assessment approach. Teach. Teach. Educ. 38, 76–88. doi: 10.1016/j.tate.2013.11.004
König, J., Doll, J., Buchholtz, N., Förster, S., Kaspar, K., Rühl, A.-M., et al. (2018). General pedagogical knowledge versus pedagogical content knowledge? The structure of professional knowledge in pre-service teachers of German, English, and Mathematics at university. Z. Erziehungswiss. 21, 1–38. doi: 10.1007/s11618-017-0765-z
Koo, T. K., and Li, M. Y. (2016). A guideline of selecting and reporting intraclass correlation coefficients for reliability research. J. Chiropr. Med. 15, 155–163. doi: 10.1016/j.jcm.2016.02.012
Landis, J. R., and Koch, G. G. (1977). The measurement of observer agreement for categorical data. Biometrics 33, 159–174. doi: 10.2307/2529310
Lave, J., and Wenger, E. (1991). Situated Learning: Legitimate Peripheral Participation. Cambridge: Cambridge University Press.
Lehman, B., D’Mello, S., Cade, W., and Person, N. (2012). “How do they do it? Investigating dialogue moves within dialogue modes in expert human tutoring,” in Proceedings of the International Conference on Intelligent Tutoring Systems, ITS 2012. Lecture Notes in Computer Science, Vol. 7315, eds S. A. Cerri, W. J. Clancey, G. Papadourakis, and K. Panourgia (Berlin: Springer), 557–562. doi: 10.1007/978-3-642-30950-2_72
Lu, X., Di Eugenio, B., Kershaw, T. C., Ohlsson, S., and Corrigan-Halpern, A. (2007). “Expert vs. non-expert tutoring: Dialogue moves, interaction patterns and multi-utterance turns,” in Proceedings of the International Conference on Computational Linguistics and Intelligent Text Processing. CICLing 2007. Lecture Notes in Computer Science, Vol. 4394, ed. A. Gelbukh (Berlin: Springer), 456–467. doi: 10.1007/978-3-540-70939-8_40
Lund, K., and Burgess, C. (1996). Producing high-dimensional semantic spaces from lexical co-occurrence. Behav. Res. Methods Instrum. Comput. 28, 203–208. doi: 10.3758/BF03204766
Manrique, M. S., and Abchi, V. S. (2015). Teachers’ practices and mental models: Transformation through reflection on action. Aust. J. Teach. Educ. 40, 13–32. doi: 10.14221/ajte.2015v40n6.2
Marquart, C. L., Hinojosa, C., Swiecki, Z., Eagan, B., and Shaffer, D. W. (2018). Epistemic Network Analysis Tool (Version v. 1.7) [Computer software]. Available online at: https://www.epistemicnetwork.org/ (accessed October 22, 2020).
Martin, M., Farrell, M., Renkl, A., Rieß, W., Könings, K. D., van Merriënboer, J. J. G., et al. (2021). Knowing What Matters: Short Introductory Texts Support Pre-Service Teachers’ Professional Vision of Tutoring Interactions [Manuscript submitted for publication]. Freiburg: University of Freiburg.
Martin, M., Farrell, M., Renkl, A., Rieß, W., Könings, K. D., van Merriënboer, J. J. G., et al. (2022). Focused Self-Explanation Prompts and Segmenting Foster Pre-Service Teachers’ Professional Vision – But Only During Training. [Manuscript submitted for publication]. Freiburg: University of Freiburg.
Mason, J. (2002). Researching Your Own Practice: The Discipline of Noticing. New York, NY: Routledge.
Mayer, R. E., Mathias, A., and Wetzell, K. (2002). Fostering understanding of multimedia messages through pre-training: evidence for a two-stage theory of mental model construction. J. Exp. Psychol. Appl. 8, 147–154. doi: 10.1037/1076-898X.8.3.147
Mayer, R. E., and Moreno, R. E. (2010). “Techniques that reduce extraneous cognitive load and manage intrinsic cognitive load during multimedia learning,” in Cognitive Load Theory, eds J. L. Paas, R. Moreno, and R. Brünken (Cambridge: Cambridge University Press), 131–152. doi: 10.1017/cbo9780511844744.009
Mayring, P. (2015). “Qualitative content analysis: theoretical background and procedures,” in Advances in Mathematics Education: Approaches to Qualitative Research in Mathematics Education: Examples of Methodology and Methods, eds A. Bikner-Ahsbahs, C. Knipping, and N. C. Presmeg (Dordrecht: Springer), 365–380. doi: 10.1007/978-94-017-9181-6_13
McDonald, M., Kazemi, E., and Kavanagh, S. S. (2013). Core practices and pedagogies of teacher education: a call for a common language and collective activity. J. Teach. Educ. 64, 378–386. doi: 10.1177/0022487113493807
Mevorach, M., and Strauss, S. (2012). Teacher educators’ in-action mental models in different teaching situations. Teach. Teach. 18, 25–41. doi: 10.1080/13540602.2011.622551
Park, S., and Chen, Y. C. (2012). Mapping out the integration of the components of pedagogical content knowledge (PCK): Examples from high school biology classrooms. J. Res. Sci. Teach. 49, 922–941. doi: 10.1002/tea.21022
Piwowar, V., Barth, V. L., Ophardt, D., and Thiel, F. (2018). Evidence-based scripted videos on handling student misbehavior: the development and evaluation of video cases for teacher education. Prof. Dev. Educ. 44, 369–384. doi: 10.1080/19415257.2017.1316299
Reigeluth, C. M. (1999). “The elaboration theory: guidance for scope and sequence decisions,” in Instructional-Design Theories and Models: A New Paradigm of Instructional Theory, ed. C. M. Reigeluth (New York: Routledge), 425–453.
Renkl, A., Hilbert, T., and Schworm, S. (2009). Example-based learning in heuristic domains: a cognitive load theory account. Educ. Psychol. Rev. 21, 67–78. doi: 10.1007/s10648-008-9093-4
Roth McDuffie, A., Foote, M. Q., Bolson, C., Turner, E. E., Aguirre, J. M., Bartell, T. G., et al. (2014). Using video analysis to support prospective K-8 teachers’ noticing of students’ multiple mathematical knowledge bases. J. Math. Teach. Educ. 17, 245–270. doi: 10.1007/s10857-013-9257-0
Santagata, R., and Angelici, G. (2010). Studying the impact of the lesson analysis framework on preservice teachers’ abilities to reflect on videos of classroom teaching. J. Teach. Educ. 61, 339–349. doi: 10.1177/0022487110369555
Santagata, R., König, J., Scheiner, T., Nguyen, H., Adleff, A.-K., Yang, X., et al. (2021). Mathematics teacher learning to notice: a systematic review of studies of video-based programs. ZDM Int. J. Math. Educ. 53, 119–134. doi: 10.1007/s11858-020-01216-z
Santagata, R., Zannoni, C., and Stigler, J. W. (2007). The role of lesson analysis in pre-service teacher education: an empirical investigation of teacher learning from a virtual video-based field experience. J. Math. Teach. Educ. 10, 123–140. doi: 10.1007/s10857-007-9029-9
Scharfenberg, F. J., and Bogner, F. X. (2019). A role-play-based tutor training in preservice teacher education for developing procedural pedagogical content knowledge by optimizing tutor–student interactions in the context of an outreach lab. J. Sci. Teach. Educ. 30, 461–482. doi: 10.1080/1046560X.2019.1583034
Schmelzing, S., Van Driel, J. H., Jüttner, M., Brandenbusch, S., Sandmann, A., and Neuhaus, B. J. (2013). Development, evaluation, and validation of a paper-and-pencil test for measuring two components of biology teachers’ pedagogical content knowledge concerning the “cardiovascular system”. Int. J. Sci. Math. Educ. 11, 1369–1390. doi: 10.1007/s10763-012-9384-6
Schnitzler, K., Holzberger, D., and Seidel, T. (2020). Connecting judgment process and accuracy of student teachers: Differences in observation and student engagement cues to assess student characteristics. Front. Educ. 5:602470. doi: 10.3389/feduc.2020.602470
Scriven, M. (1972). “The exact role of value judgments in science,” in Proceedings of the 1972 Biennal Meeting Philosophy of Science Association, eds K. F. Schaffner and R. S. Cohen (Dordrecht: D. Reidel Publishing), 219–247. doi: 10.4103/0973-1229.40731
Seidel, T., Blomberg, G., and Renkl, A. (2013). Instructional strategies for using video in teacher education. Teach. Teach. Educ. 34, 56–65. doi: 10.1016/j.tate.2013.03.004
Seidel, T., and Stürmer, K. (2014). Modeling and measuring the structure of professional vision in preservice teachers. Am. Educ. Res. J. 51, 739–771. doi: 10.3102/0002831214531321
Shaffer, D. W. (2006). Epistemic frames for epistemic games. Comput. Educ. 46, 223–234. doi: 10.1016/j.compedu.2005.11.003
Shaffer, D. W. (2018). “Epistemic network analysis: Understanding learning by using big data for thick description,” in International Handbook of the Learning Sciences, eds F. Fischer, C. E. Hmelo-Silver, S. R. Goldman, and P. Reimann (New York, NY: Routledge), 520–531.
Shaffer, D. W., Collier, W., and Ruis, A. R. (2016). A tutorial on epistemic network analysis: analyzing the structure of connections in cognitive, social, and interaction data. J. Learn. Anal. 3, 9–45. doi: 10.18608/jla.2016.33.3
Shaffer, D. W., and Ruis, A. (2017). “Epistemic network analysis: a worked example of theory-based learning analytics,” in The Handbook of Learning Analytics, eds C. Lang, G. Siemens, A. Wise, and D. Gašević (Beaumont, AB: Society of Learning Analytics Research (SOLAR)), 175–187. doi: 10.18608/hla17.015
Sherin, M. G., Linsenmeier, K. A., and van Es, E. A. (2009). Selecting video clips to promote mathematics teachers’ discussion of student thinking. J. Teach. Educ. 60, 213–230. doi: 10.1177/0022487109336967
Sherin, M. G., Russ, R. S., Sherin, B. L., and Colestock, A. (2008). Professional vision in action: an exploratory study. Issues Teach. Educ. 17, 27–46.
Sherin, M. G., and van Es, E. (2002). “Using video to support teachers’ ability to interpret classroom interactions,” in Proceedings of the SITE 2002 - Society for Information Technology and Teacher Education International Conference, eds D. Willis, J. Price, and N. Davis (Waynesville, NC: Association for the Advancement of Computing in Education (AACE) Publishing), 2532–2536.
Sherin, M. G., and van Es, E. A. (2009). Effects of video club participation on teachers’ professional vision. J. Teach. Educ. 60, 20–37. doi: 10.1177/0022487108328155
Shulman, L. (1987). Knowledge and teaching: foundations of the new reform. Harv. Educ. Rev. 57, 1–23. doi: 10.4324/9781351233866-1
Spanjers, I. A. E., van Gog, T., and van Merriënboer, J. J. G. (2010). A theoretical analysis of how segmentation of dynamic visualizations optimizes students’ learning. Educ. Psychol. Rev. 22, 411–423. doi: 10.1007/s10648-010-9135-6
Star, J. R., and Strickland, S. K. (2008). Learning to observe: using video to improve preservice mathematics teachers’ ability to notice. J. Math. Teach. Educ. 11, 107–125. doi: 10.1007/s10857-007-9063-7
Stockero, S. L., and van Zoest, L. R. (2013). Characterizing pivotal teaching moments in beginning mathematics teachers’ practice. J. Math. Teach. Educ. 16, 125–147. doi: 10.1007/s10857-012-9222-3
Stürmer, K., Könings, K. D., and Seidel, T. (2013a). Declarative knowledge and professional vision in teacher education: effect of courses in teaching and learning. Br. J. Educ. Psychol. 83, 467–483. doi: 10.1111/j.2044-8279.2012.02075.x
Stürmer, K., Seidel, T., and Schäfer, S. (2013b). Changes in professional vision in the context of practice. Gruppendyn. Organisationsberat. 44, 339–355. doi: 10.1007/s11612-013-0216-0
Sweller, J. (1994). Cognitive load theory, learning difficulty, and instructional design. Learn. Instr. 4, 295–312. doi: 10.1016/0959-4752(94)90003-5
Turner, S. (2012). Making the tacit explicit. J. Theory Soc. Behav. 42, 385–402. doi: 10.1111/j.1468-5914.2012.00500.x
van Es, E. A. (2011). “A framework for learning to notice student thinking,” in Mathematics Teacher Noticing: Seeing Through Teachers’ Eyes, eds M. G. Sherin, V. R. Jacobs, and R. Philipp (New York, NY: Routledge), 164–181.
van Es, E. A., Cashen, M., Barnhart, T., and Auger, A. (2017). Learning to notice mathematics instruction: using video to develop preservice teachers’ vision of ambitious pedagogy. Cogn. Instr. 35, 165–187. doi: 10.1080/07370008.2017.1317125
van Es, E. A., and Sherin, M. G. (2002). Learning to notice: scaffolding new teachers’ interpretations of classroom interactions. J. Technol. Teach. Educ. 10, 571–596.
van Es, E. A., and Sherin, M. G. (2008). Mathematics teachers’ “learning to notice” in the context of a video club. Teach. Teach. Educ. 24, 244–276. doi: 10.1016/j.tate.2006.11.005
van Merriënboer, J. J. G., and Kirschner, P. A. (2018). Ten Steps to Complex Learning: A Systematic Approach to Four-Component Instructional Design, 3rd Edn. New York, NY: Routledge.
VanLehn, K., Siler, S., Murray, C., Yamauchi, T., and Baggett, W. B. (2003). Why do only some events cause learning during human tutoring? Cogn. Instr. 21, 209–249. doi: 10.1207/S1532690XCI2103_01
von Kotzebue, L., Förtsch, C., Förtsch, S., and Neuhaus, B. J. (2021). Dealing with student errors in whole-class discussions of biology lessons at German secondary schools. Int. J. Sci. Math. Educ. doi: 10.1007/s10763-021-10171-4 [Epub ahead of print].
Voss, T., Kunter, M., and Baumert, J. (2011). Assessing teacher candidates’ general pedagogical/psychological knowledge: test construction and validation. J. Educ. Psychol. 103, 952–969. doi: 10.1037/a0025125
Watson, P. F., and Petrie, A. (2010). Method agreement analysis: a review of correct methodology. Theriogenology 73, 1167–1179. doi: 10.1016/j.theriogenology.2010.01.003
Weger, D. (2019). Entwicklung Professioneller Unterrichtswahrnehmung für Mehrsprachig-Sprachbewusste Unterrichtsgestaltung [Development of Professional Teaching Perception in Translinguistic Lesson Planning]. Unpublished Ph.D. dissertation. Vienna: University of Vienna.
Windschitl, M., Thompson, J., and Braaten, M. (2011). Ambitious pedagogy by novice teachers: Who benefits from tool-supported collaborative inquiry into practice and why? Teach. Coll. Rec. 113, 1311–1360. doi: 10.1177/016146811111300702
Keywords: teacher education, teacher students, professional vision, teacher noticing, video analysis, tutoring, qualitative content analysis, epistemic network analysis (ENA)
Citation: Farrell M, Martin M, Renkl A, Rieß W, Könings KD, van Merriënboer JJG and Seidel T (2022) An Epistemic Network Approach to Teacher Students’ Professional Vision in Tutoring Video Analysis. Front. Educ. 7:805422. doi: 10.3389/feduc.2022.805422
Received: 30 October 2021; Accepted: 25 January 2022;
Published: 18 February 2022.
Edited by:
Auli Toom, University of Helsinki, FinlandReviewed by:
Ilona Södervik, University of Helsinki, FinlandLauri Heikonen, University of Helsinki, Finland
Copyright © 2022 Farrell, Martin, Renkl, Rieß, Könings, van Merriënboer and Seidel. This is an open-access article distributed under the terms of the Creative Commons Attribution License (CC BY). The use, distribution or reproduction in other forums is permitted, provided the original author(s) and the copyright owner(s) are credited and that the original publication in this journal is cited, in accordance with accepted academic practice. No use, distribution or reproduction is permitted which does not comply with these terms.
*Correspondence: Meg Farrell, meg.farrell@tum.de