- 1Department of Psychology, Kingston University, Kingston upon Thames, United Kingdom
- 2Institute of Psychiatry, Psychology and Neuroscience, King’s College London, London, United Kingdom
- 3Youthworks Consulting, East Molesey, United Kingdom
There is strong evidence showing that vulnerable children and adolescents, such as children who are carers for their family, in care themselves, or who have a physical disability or special educational needs, are at greater risk of mental health problems and poor social wellbeing. Recent research indicates this heterogenous but vulnerable group is also disadvantaged online and may be at greater risk of harm. This study aimed to examine participants’ vulnerability (vulnerable vs. non-vulnerable), psychological distress, parental e-safety support, and online lives, specifically their experiences of online risk. The roles of psychological distress and parental e-safety support were explored as possible mediators and moderators, respectively, for the relationship between vulnerability and online risk. Survey data was collected from 15,278 participants (11–17 years old; M = 12.60, SD = 1.44), 46.6% identified as females, 46.6% identified as males and 6% identified as “other”. Participants were recruited from schools/colleges in different parts of England, of whom 3,242 were categorised as vulnerable. Using Analysis of Variance (ANOVA) the results showed that, when compared to the non-vulnerable participants, the vulnerable groups (single or multiple vulnerabilities) experienced more psychological distress and online risks. They also received less e-safety support from parents or carers. Furthermore, mediation and moderated mediation analysis showed that the relationship between vulnerability and online risk was significantly and partially mediated by psychological distress. Parental e-safety support was found to moderate the relationship between psychological distress and online risk and between vulnerability and online risk. In conclusion, the results demonstrated that vulnerability offline is mirrored online. Psychological interventions and parental e-safety support are required to navigate the internet safely. Children’s services and practitioners should consider online risks in their assessments and interventions.
Introduction
As digital life has become more widespread and essential in recent decades, children, and adolescents have been spending ever more time online (Livingstone et al., 2017). This trend has been rapidly amplified by the COVID-19 pandemic as young people relied increasingly on the internet for education, entertainment, socializing, and solace (Eyimaya and Irmak, 2020; Parents Together, 2020). Although the internet has many positives for young people, such as social connectivity (Zilka, 2017), increased time spent online is associated with internet dependence, poor mental health, and increased exposure to online risks (Russ et al., 2009; Tonioni et al., 2012; Livingstone et al., 2017; McDool et al., 2020). Online risks have been identified based on their probability of harmful consequences (Livingstone, 2013). These risks include – but are not limited to – exposure to harmful content (e.g., self-harm, pro-anorexia, radicalisation) and risk of child sexual exploitation (Livingstone et al., 2011; Green et al., 2019).
There is evidence that some groups of young people are disproportionately susceptible to online risk exposure (Lau and Yuen, 2013; Notten and Nikken, 2014; El-Asam and Katz, 2018). It is concerning that recent research showed that vulnerable young people are more likely than non-vulnerable peers to spend extensive periods of time online (El-Asam and Katz, 2018) and to encounter such risks (d’Haenens et al., 2013; El-Asam and Katz, 2018). They were also more likely to share nude or explicit images, videos, or livestreams online (Katz and El-Asam, 2020).
Vulnerable children and young people are defined in this study as those with family/social challenges, physical disability, poor mental health, communication difficulties, or special educational needs. The number of children in this high-risk group is considerable. Of the 12 million children in England alone, approximately 400,000 children are in the social care system at any one time (Ofsted, 2020), and 78,000 of these are in care. Furthermore, 1.1 million have disabilities (Department for Education, 2020), and over 1.3 million have Special Educational Needs (Office for National Statistics, 2020). The Children’s Commissioner estimates that 2.3 million children are vulnerable due to family vulnerability and 1.6 million children are in families with complex needs (Children’s Commissioner, 2019), with a further 166,000 children and young people reported to be young carers (Office for National Statistics, 2011).
Whilst vulnerable children have been established as an at-risk population online (d’Haenens et al., 2013; El-Asam and Katz, 2018), the underlying mechanisms, protective factors or potential mediators of the relationship between vulnerability and risk are not fully understood. Hence, mental health and parental e-safety support are two key factors of interest in this study.
The link between mental health and online risk is well-established, though the relationship between the two appears complicated. Online risks have been identified as having a negative impact on young people’s mental health (Doyle et al., 2021), but are also more prevalent amongst those internet users with pre-existing mental health difficulties (Vandoninck et al., 2013). Research among adolescents has found that poorer psychological wellbeing, self-esteem, and self-control are related to the severity of problematic internet use (Mei et al., 2016). Earlier results, from a Europe-wide study, have shown that young people with psychological problems are more likely to encounter risks online and have a higher chance of being negatively impacted by the experience (Livingstone et al., 2011). There are several specific online risks which have been identified as being disproportionately experienced by those with mental health difficulties. For example, depression has been found to predict online sexual solicitation and online grooming (Whittle et al., 2013; Hornor, 2020), whilst sexting and accessing online adult sites has been associated with psychological difficulties (Livingstone and Smith, 2014). Online communities are often established amongst individuals with mental health difficulties who seek support from their peers. Pro-eating disorder communities are one example of online spaces where young people are exposed to high-risk content and interactions, such as the encouragement of disordered eating behaviours or promotion and glamorisation of thin ideals (Sowles et al., 2018).
Furthermore, resilience, which vulnerable children may lack, has been found to be a protective factor from harm experienced due to exposure to online risk (Vandoninck et al., 2013; Wisniewski et al., 2015; Vissenberg and d’Haenens, 2020). Lower levels of resilience have been associated with mental health difficulties and high levels of psychological distress (Wu et al., 2020) with resilience characteristics being associated with lower levels of anxiety and depression symptoms. As such, vulnerable children with poor psychological wellbeing might be at risk of greater harm from online risks.
There is rich evidence that vulnerable children and young people experience psycho-social disadvantages, such as a greater likelihood of mental health problems and poor social wellbeing (e.g., Emerson, 2003; Raphael et al., 2006; Taanila et al., 2009; Ryan and Tunnard, 2012; El-Asam et al., 2021a). Understanding the role psychosocial wellbeing plays in explaining online vulnerability is of particular urgency due to early evidence suggesting young people are increasingly isolated during pandemic-related restrictions and are at increased risk of harm online (UNICEF, 2020).
Along with positive mental health, online parental supervision is key in helping children avoid online risks while improving their resilience. Although parental restrictions and parental monitoring have been shown to be effective in mitigating against online risk (Livingstone and Helsper, 2008; Khurana et al., 2015) and building young people’s resilience (Green et al., 2019), online safety practices are falling behind (Phippen, 2009; Green et al., 2019). There is disconcerting evidence that current e-safety education or initiatives are neither evidence-based nor evaluated (Jones et al., 2013), are out of date and fail to fully include vulnerable children and young people (Annansingh and Veli, 2016; Badillo-Urquiola and Wisniewski, 2017; El-Asam and Katz, 2018). It is likely that this group may not benefit from the same e-safety guidance and parental support as their peers, due to a lack of adapted content and increased social or school exclusion (El-Asam et al., 2021a). Furthermore, results from the same study suggest that digital harms among these groups may be missed due to the prioritisation of need by professionals or carers, whereby behavioural or emotional needs are addressed first, or in instances where parents or carers underestimate the capacity of young people with learning difficulties to access the internet (El-Asam et al., 2021a). Although this may help explain their increased exposure to potentially high-risk online scenarios, little is known of the risks of cumulative disadvantage on online risk for children and young people.
While vulnerable children have received more attention from policy makers in recent years due to the regular collection of data by the Children’s Commissioner for England, what motivates and influences the digital lives of these children and young people is less well understood by frontline services who work with them (El-Asam et al., 2020, 2021b). When explaining why vulnerable young people are more exposed to risk than others one might consider the Social Compensation Hypothesis, which suggests that those who perceive their offline social networks to be lacking seek to compensate through their online activities, for example through their social interactions (e.g., Livingstone et al., 2005; Peter et al., 2005). Other research explains how young people experiencing attachment difficulties, mental health issues or loneliness may rely on the internet to fulfil aspects of their lives that are not met offline (Stamoulis and Farley, 2010; Palmer, 2015; Eichenberg et al., 2017; Efrati and Amichai-Hamburger, 2019). Vulnerable children and adolescents are likely to experience these psychosocial difficulties, which may explain their exposure to online risks as they attempt to reduce negative emotions and avoid real life problems by increasing their engagement online (Kardefelt-Winther, 2014). Consequently, vulnerable children might feel less restrained online and more likely to self-disclose; in a positive sense (increased confidence in sharing true thoughts/feelings) and in a negative sense (disregarding rules/safety and being more aggressive). This phenomenon is referred to as the Online Disinhibition Effect (Suler, 2004).
Current Research
Research on the online lives of vulnerable young people is scarce. An original study by El-Asam and Katz (2018) found that vulnerable young people spend more time online and encounter more online risks. This research does not adopt a specific theoretical framework, however, mental health and e-safety are key factors in our rationale. Research among children showed evidence of the protective effects of psychological wellbeing (Vandoninck et al., 2013; Wisniewski et al., 2015; Vissenberg and d’Haenens, 2020) and parental e-safety support (Livingstone and Helsper, 2008; Khurana et al., 2015) in keeping young people safe online. However, vulnerable young people are unlikely to benefit from these protective factors in the same way as their peers, due to the increased likelihood of mental health difficulties and poor social wellbeing (Emerson, 2003; Raphael et al., 2006; Taanila et al., 2009; Ryan and Tunnard, 2012; El-Asam et al., 2021a), and observations of poor, maladapted e-safety provision for this cohort (Annansingh and Veli, 2016; Badillo-Urquiola and Wisniewski, 2017; El-Asam and Katz, 2018).
The combination of a vulnerability condition, mental health and maladapted e-safety support is likely to put the vulnerable child more at risk of encountering or experiencing online risk. We argue that positive mental health and e-safety support play a significant role in protecting vulnerable children from such encounters while negative mental health might correlate with an increase in such encounters. We assume that vulnerable children have complex social and emotional needs, and the internet is a opportune place to compensate for such needs. They are also poorly monitored or supervised online and hence they may be disinhibited (less restrained) and as a result this is likely to motivate them to access high-risk online content (e.g., pro-anorexia, self-harm and extremist/radical content) and engage in high-risk behaviour (e.g., sexting and online aggression). In a sense, poor mental health and weak e-safety support from caring adults adds another layer of vulnerability to an already vulnerable group, while positive mental health and e-safety support act as protective factors from experiencing/encountering online risks.
This study aims to explore vulnerable children’s mental health (referred to as psychological distress: depression, anxiety, and self-esteem) and their online experiences (online risk encounters, parental e-safety support and perceived internet impact). More specifically we suggest the following hypotheses: (1) Vulnerable young people are more psychological distressed compared to their non-vulnerable peers; (2) Vulnerable young people are more disadvantaged online (experience more online risks, and receive less e-safety support) compared to their non-vulnerable peers; (3) Vulnerable children are more likely to experience online risks due to their higher psychological distress and poorer parental e-safety support compared to non-vulnerable children.
Materials and Methods
Participants
This study used non-probability opportunity sampling to gather data from 15,278 children and adolescents from 94 primary/secondary schools as well as colleges across different regions in England. Schools and colleges were approached to take part in “The Cybersurvey 2019”. Overall, 7,185 (46.6%) of the sample identified as males, 7,185 (46.6%) identified as females and 908 (6%) identified as “other”. Age ranged between 11 and 17 years (M = 12.60, SD = 1.44). Participants with self-reported, pre-existing social, physical, and educational related conditions or difficulties were categorised as vulnerable, this includes participants who: are carers for someone in their family; are residing in or leaving care; have a long-standing physical illness; cannot see well or at all; cannot hear well or at all; have speech difficulties; have learning difficulties; have Autism Spectrum Disorder. Vulnerability was computed and recoded into 0 = no vulnerability/control (n = 9,000), 1 = single vulnerability (n = 2,432), 2 = multiple vulnerabilities (i.e., two or more; n = 810). Participants who did not fall into those groups (e.g., had a mental health condition or skipped the question, n = 3,036) were excluded.
Design and Procedure
This study follows a cross-sectional design and utilised quantitative data (Cybersurvey-2019). The Cybersurvey is an annual data collection tool, adapted on a yearly basis to generate an understanding of children and young people’s experiences online (use, risks, safety, and impact). Researchers approached schools and colleges in different geographical locations across England via emails and phone calls. Schools and colleges were briefed about the study, including the aims, objectives, the ethical standards and adherence to GDPR. Following their written and verbal consents, each school/college nominated its representative who supported with collecting the data online (pre-designed online survey via an internet link).
Within each school/college participating young people were brought to the school’s IT-room or used tablets in class. After a register was taken, students were instructed to enter their unique school code in question 1 of the survey. This was based on school and location in England. This was designed to ensure participants’ anonymity and their right to withdraw data if needed. IP addresses were collected along with date and time of activity on the survey, to be able to report a concern about significant harm if disclosed in the survey. Upon completion, young people and schools were thanked and debriefed. Schools were encouraged to maintain contact with the researchers for dissemination purposes. Preliminary and anonymous survey results were posted online and shared with schools.
Measures
The survey was designed and constructed based on consultations, knowledge and key findings generated from previous research (e.g., Hinduja and Patchin, 2009, 2012; Livingstone et al., 2011; El-Asam and Katz, 2018). The survey benefited from a stringent validation process from educators, e-safety experts, psychologists and social workers. The survey went through rigorous language and academic checks to assess the suitability of the survey for young participants and its compatibility with the research aims and objectives (achieving face and content validity).
Internet Related Measures
All internet related measures were designed in line with research findings and new developments in children’s digital use and behaviour. Specifically, this study examined perceived internet impact, parental e-safety support and online risk encounters.
Perceived Internet Impact
This measure included 12 items to assess participants’ opinions on how the internet has impacted their lives. All items were scored on a 4-point scale (1 = never, 2 = hardly ever, 3 = sometimes, 4 = most of the time). Exploratory Factor Analysis (EFA) showed that the 12 items represent two factors, the sample size was deemed adequate [KMO = 0.851, χ2(66) = 47624.13, p < 0.001] and the model explained 61% of the variances. The first factor explained 31.6% of the variance and is referred to as “Perceived Positive Internet Impact” (six items, α = 0.82) illustrating social and psychological benefits (e.g., finding likeminded people to talk to, feeling supported and connected to people, escaping my issues). The second factor explained 29.7% of the variance and is referred to as “Perceived Negative Internet Impact” (six items, α = 0.80) which includes items that reflect the negative impact of the internet on the child’s life and environment (e.g., on relationships with family or friends, neglect of school or college work, anxiety or depression). For this study a mean score was computed for each of the factors.
Parental e-Safety Support
Using 13 items, this measure captured parents’/carers’ involvement in guiding their children to safely navigate the internet. All items were scored on a 4-point scale (1 = never, 2 = hardly ever, 3 = sometimes, 4 = most of the time). EFA showed that this scale has three underlying constructs, all of which explain 63% of the variances within the data [Sampling adequacy: KMO = 0.856, χ2(78) = 48895.01, p < 0.001]. The first factor “e-safety monitoring” included six items (α = 0.83) and reflected young people’s experience of their parents’ monitoring of their activity online (e.g., they check that games are rated OK for my age; they have set up controls to keep me safe). This factor explained 35.5% of the variance. The second factor included four items (α = 0.73) reflecting the child’s ability to “e-safety advice” from others (e.g., there are other people in my family I can turn to about online problems; I follow the advice from my parents or carers). This factor explained 16.7% of the variances. And the third factor included three items (α = 0.72) illustrating the child’s own “e-safety competence” (e.g., parents trust me to manage my online life; I can manage my online safety). This factor explained 10.7% of the variance. Together all 13 items generated a good level of internal consistency (α = 0.81). For this study an overall mean score was computed to reflect parental e-safety support.
Online Risks
This measure reflects repetitive exposure to, or encounters with, potentially harmful online risks/scenarios. Overall, the 26 risks assessed reflect content-based risks (e.g., pro-anorexia and self-harm), contact-based risks (relationships), cyberscams and identity risks (e.g., credit card details stolen and identify thefts). Items were scored on a 3-point scale (1 = never, 2 = once/twice, 3 = often). Other risks were categorised as conduct-based risks which involve potentially problematic activities (age-related) such as sexting, gambling and meeting strangers. These were scored as 1 = yes (often/frequently), 0 = no (never/rarely). Although these categories (4Cs) were used thematically in previous research, to our knowledge such categories of risk were not statistically validated. Most previous studies have included fewer online risk types or scenarios.
Exploratory factor analysis was conducted to better understand the underlying structure of these items. The results showed that 26 risk items can be grouped under five factors, accounting for 61.3% of the variance [KMO = 0.938, χ2(325) = 49520.0, p < 0.001]. The first factor accounted for 30.6% of the variance and included 10 items (α = 0.90) which reflect “Content Risk” for example, content that promotes violence, hatred or racist views; pressures people to be very thin, talks about suicide; supports religious extremist views or terrorist acts. The second factor accounted for 11.2% of the variance and included six items (α = 0.75) which reflect “Relational Risk”, for example, has someone you met online tried to persuade you into some sexual activity you did not want? have you ever felt someone was trying to control or stalk you?.
The third factor is referred to as “Cyberscams” which included four items (α = 0.71) accounting for 7.9% of the variance. The items reflect potential or actual online scam encounters, for example, I’ve been tricked into buying fake goods; I’ve believed something that turned out to be a scam. The fourth factor accounted for 6.7% of the variance and included four items (α = 0.79) which enquire about children’s access to “Age Restricted Websites”. For example, accessing gambling websites or dating websites. The final model accounted for 4.9% of the variance and only included two items (α = 0.70) that reflect “Strangers’ Contact” i.e., I’ve added people as friends without knowing who they are, I have met strangers.
For this study the total score of all binary items in which the respondent selected ‘yes’ (often/frequently) and all other risks which were encountered “often” times (range: 0–26, and reliability of KT-20 = 0.88) was computed to reflect how many online risks participants have experienced.
Psychological Distress
We used the term psychological distress to reflect common psychological disturbances. Three scales were designed to reflect depression and anxiety related symptoms as well as difficulties in self-esteem. These scales were guided by DSM-5 Characteristics (American Psychiatric Association, 2013). Items were linguistically simplified for the use of child/adolescent sample and were scored on 4-point scale (1 = never, 2 = hardly ever, 3 = sometimes, 4 = most of the time). Confirmatory Factor Analysis (CFA) was conducted to understand how well items represent each of the constructs.
Depression was explored using 11 items which asked about feelings of sadness, negativity, tiredness, and psychosomatic problems (e.g., feeling sad and negative, inability to concentrate or sleep well, poor appetite and inability to make decisions). For depression symptoms, 11 items were shown to load sufficiently under a single construct. The model fit indexes suggest a good fit [χ2(33) = 1,540, p < 0.001; RMSEA = 0.060, GFI = 0.977, CFI = 0.939]. Items showed good internal reliability (α = 0.70).
Moreover, Generalised Anxiety was assessed using seven items reflecting feelings of anxiety and worrying thoughts (e.g., feeling nervous or anxious, I worry a lot, I get irritable and angry easily). CFA showed that the seven items with the anxiety scale highly load under a single factor with good model fit [χ2(12) = 73.73, p < 0.001; RMSEA = 0.019, GFI = 0.998, CFI = 0.998]. Internal consistency was at a good level (α = 0.84).
Self-Esteem was measured using four items (e.g., I’m proud of things I do, I feel there are some good things about me and overall, I’m happy with myself). CFA results suggest that the model has a good fit using 4 items [χ2(1) = 2.1, p = 0.148, RMSEA = 0.009, GFI = 1.00, CF = 1.00], with good level of internal consistency (α = 0.88).
For this study, the mean score was calculated for each of the three scales. Additionally, the mean score was computed for all items to represent Psychological Distress. Reverse coding was conducted for self-esteem items and others, so that higher mean scores reflect more psychological distress. Internal consistency for all items across the three scales was good (α = 0.88).
Social Support/Isolation
This measure included seven items reflecting communication and connection with others, social isolation and loneliness. Items reflect relationships and support from peers, friends, and school staff (e.g., having good friends, having adults they can trust at school, feeling alone and worrying about friendships). This measure was scored on a 4-point agreement scale (1 = strongly disagree, 2 = disagree, 3 = agree, 4 = strongly agree). EFA showed that all items loaded highly under a single factor, accounting for 63.7% of the variance [KMO = 0.838, χ2(21) = 21849.2, p < 0.001]. Furthermore, internal reliability showed good level of consistency between all items (α = 0.77). Negative items were reverse coded, and a mean score was computed to represent social support.
Data Analysis
Exploratory Factor Analysis as well as Confirmatory Factor Analysis were used through SPSS and AMOS respectively. EFA was used to examine the underlying structures/factors of sets of items constructed to assess perceived Internet impact, parental e-safety support, online risks, and social support/isolation. A list of all scales can be found in the Supplementary material.
Confirmatory factor analysis was used to assess if there is an association between observed items and their underlying latent factor or construct. CFA was used to assess how well items under depression, generalised anxiety and self-esteem correlate with their construct. CFA was deemed suitable as theses scales were designed based on existing research and diagnostic criteria [e.g., DSM-5 by the American Psychiatric Association (2013)]. Model Fitness was based on scores on the following indices: GFI (Goodness of Fit), CFI (Comparative Fit Index), RMSEA (Root-Mean-Square Error of Approximation) and Chi-Squared test (χ2) although the latter is sensitive to small and large sample sizes. Acceptable model fitness is based on the following values GFI/CFI > 0.90; RMSEA < 0.08 and a non-significant Chi-Squared test outcome (p > 0.05) (e.g., Hu and Bentler, 1999).
Descriptive statistics (Frequency, Mean and Standard Deviation) as well as Pearson’s r correlation coefficient were computed to understand the data and the relationships between all key variables. One-Way Analysis of Variance (ANOVA) was used to assess differences between vulnerable (offline vulnerability: one or multiple vulnerabilities) and non-vulnerable participants across all key variables.
Furthermore, Mediation Analysis (Model 4) and Moderated Mediation Analysis (Model 15) were conducted using SPSS macro-PROCESS by Hayes (2012). Model 4 assesses the extent to which psychological distress (M: mediator) mediates the relationship between vulnerability (X: predictor) and experiences of online risk (Y: outcome). Model 15 tests if the relationship between the predictor and the outcome and between the mediator and the outcome are moderated by parental e-safety support (W). Participants’ age and gender were used as Covariates in both models.
Results
General Statistics
Table 1 illustrates descriptive statistics and Pearson’s r correlation coefficients between key variables/measures. In general, the r coefficients showed significant correlations (p < 0.01) between psychological distress variables (depression, anxiety, self-esteem) and internet related experiences (parental e-safety support, positive and negative internet impact as well as online risk). It was evident that participants with higher depressive and anxiety symptoms and lower self-esteem, experienced less parental e-safety support and perceived the internet to have a negative impact on their lives. When examining online risk, participants with higher depressive (r = 0.274, p < 0.001) and anxiety (r = 0.230, p < 0.001) symptoms were shown to also have more encounters or experiences of online risk. Furthermore, those with more positive self-esteem had fewer encounters of online risk (r = −0.233, p < 0.001). Positive parental e-safety support is also associated with lower experiences of online risk (r = −0.268, p < 0.001).
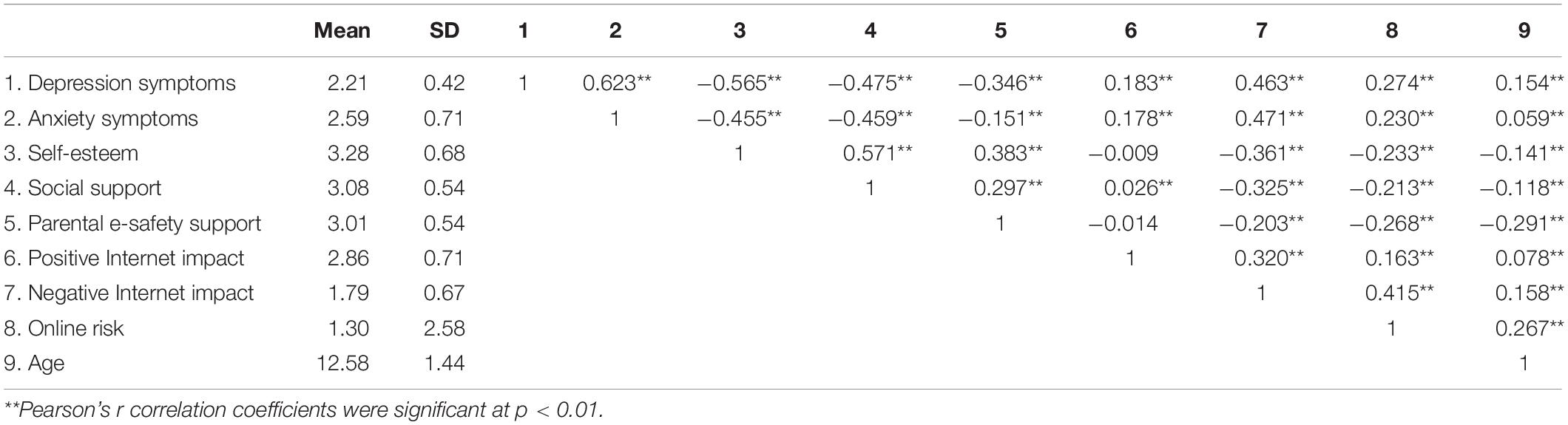
Table 1. Descriptive statistics and Pearson’s r correlations between psychological distress and internet related measures.
Vulnerability Group
One-Way Analysis of Variance (ANOVA) was used to examine group differences between vulnerable (offline vulnerability: one or multiple vulnerabilities) and non-vulnerable participants. The results showed that that offline vulnerability is mirrored in negative online experiences. Table 2 shows the mean scores for participants across the three groups. Vulnerable participants have significantly higher depressive symptoms [F(2, 11793) = 491.83, p < 0.001, ηp2 = 0.077], higher anxiety symptoms [F(2, 11791) = 522.76, p < 0.001, ηp2 = 0.081], poorer self-esteem [F(2, 11793) = 314.62, p < 0.001, ηp2 = 0.051] and less social support [F(2, 12023) = 436.33, p < 0.001, ηp2 = 0.068].
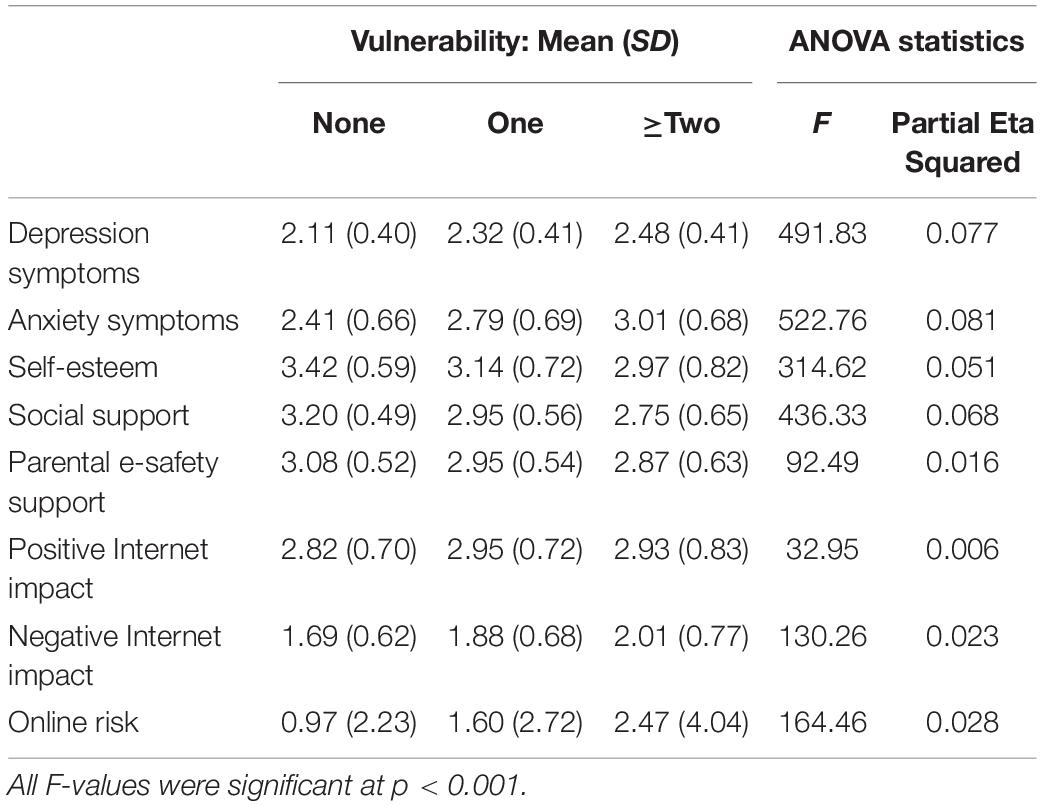
Table 2. One way analysis of variance and descriptive statistics illustrating group-based differences.
In terms of internet related behaviour, vulnerable children were more exposed to online risks, [F(2, 11595) = 164.46, p < 0.001, ηp2 = 0.028], experienced increased perceived negative internet impact [F(2, 10826) = 130.26, p < 0.001, ηp2 = 0.023], while receiving less parental e-safety support [F(2, 11347) = 92.49, p < 0.001, ηp2 = 0.016]. Furthermore, vulnerable participants perceived the internet to have more positive impact on their lives [F(2, 10820) = 32.95, p < 0.001, ηp2 = 0.006]. Post-hoc (Bonferroni) statistics showed significant difference (p < 0.01) between any two groups across all variables apart from perceived internet positive impact, where the two vulnerability groups were not significantly different from each other (p > 0.05).
Mediating Effect Analysis: Psychological Distress
Table 3 illustrates results from Mediation Analysis using Model 4 in SPSS macro-PROCESS by Hayes (2012). This model was adopted to test the mediating effect of psychological distress (M: Mediator) on the relationship between vulnerability (X: Predictor) and online risk (Y: Outcome) while controlling for age and gender as Covariates.
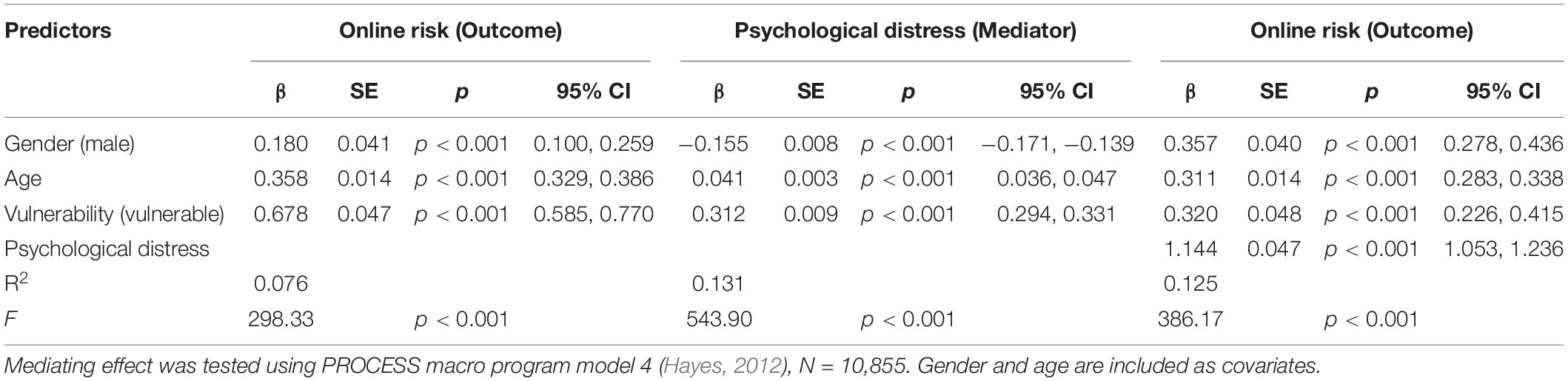
Table 3. Mediation analysis showing the direct and indirect (through mediator) effects of vulnerability on total online risk encounters.
The first model (in Table 3) shows that vulnerability has a significant positive association with online risk (β = 0.678, SE = 0.047, p < 0.001), while the second model shows that vulnerability has a significant positive association with psychological distress (β = 0.312, SE = 0.009, p < 0.001). The third model included both vulnerability and psychological distress as predictors of online risk; the results show that psychological distress had a significant positive association with online risk (β = 1.144, SE = 0.047, p < 0.001). Moreover, vulnerability still maintained its significant positive association with online risk (β = 0.320, SE = 0.048, p < 0.001).
Finally, the upper and lower bounds of the bootstrapped 95% CI for the “Indirect Effect” of vulnerability on online risk (via psychological distress as mediator) did not include 0; this indicates that the mediating effect was significant (Indirect Effect = 0.357, SE = 0.020, 95% CI = 0.318, 0.397). In other words, psychological distress partially explains the relationship between vulnerability and experiences of online risk.
Following from the results above (Model 4, PROCESS), Moderated Mediation Analysis (Model 15, PROCESS) was used to assess if parental e-safety support (W) moderates the direct association between psychological distress (M) and vulnerability (X) on online risk (Y).
The results showed a significant index of moderated mediation (Index = −0.158, SE = 0.042, CI = −0.242, −0.076). This is further explained by the significant interaction effect (Table 4) between psychological distress and parental e-safety support (M*W) (β = −0.311, SE = 0.091, p < 0.001), and the significant interaction effect between vulnerability and parental e-safety support (X*W) in predicting/explaining exposure to online risks (β = −0.507, SE = 0.082, p < 0.001). In both interactions, it could be explained, although with small effect size, that parental e-safety support reduces the exposure to online risks when vulnerability and psychological distress are used as predictors of online risk.
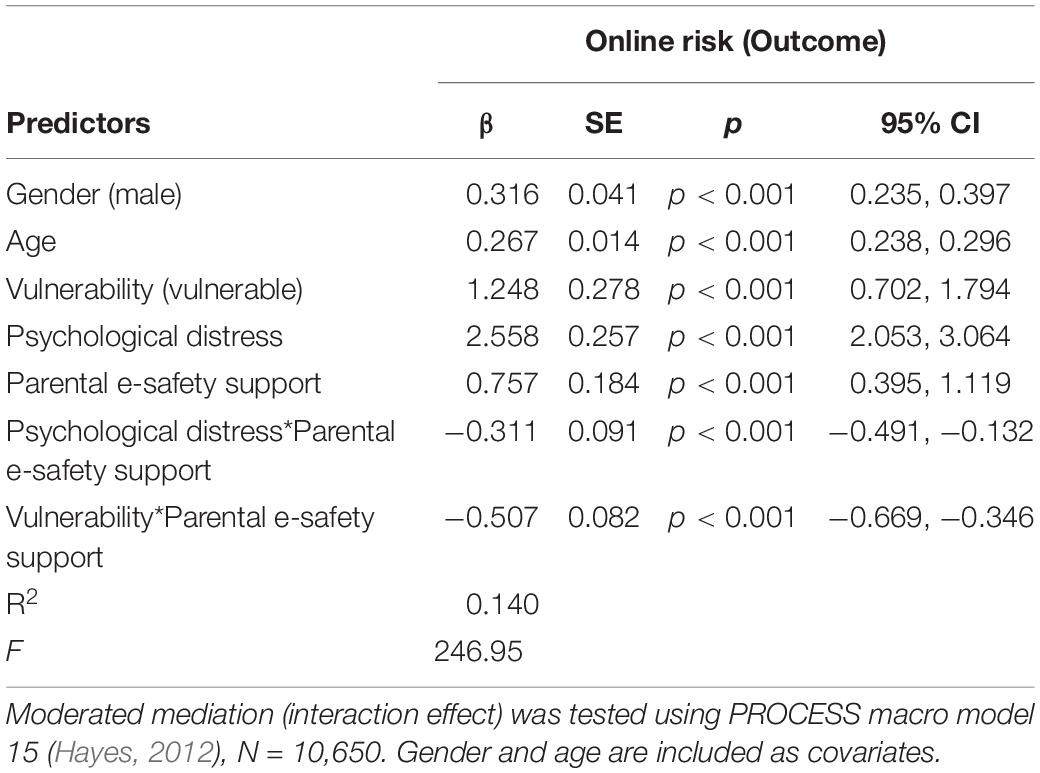
Table 4. Moderated mediational analysis examining the role of parental e-safety support as a moderator of the relationship between vulnerability and online risk, and between psychological distress and online risk.
Discussion
This study examined the psychological wellbeing (psychological distress) and the online lives of vulnerable young people compared to their non-vulnerable peers. It further examined the role of vulnerability in explaining exposure to online risks while evaluating the role of mental health as a potential mediator and parental e-safety support as a moderator.
The present results showed that vulnerable young people were deemed more disadvantaged across three domains. Firstly, at an indvidual level, they showed more psychological distress; secondly, in their online environment, they were more likely to experience online risk; and thirdly, from an e-safety perspective they were less likely to receive good parental e-safety support. Furthermore, psychological distress and poor parental e-safety support were found to partially explain exposure to online risks. These findings are discussed in the following sections according to the study hypotheses:
Psychological Distress
Corroborating previous research, vulnerable young people in our study had significantly poorer psychosocial wellbeing (Emerson, 2003; Raphael et al., 2006; Taanila et al., 2009; Ryan and Tunnard, 2012). These young people, especially those with multiple vulnerabilities, were found to have more depression and generalised anxiety symptoms, and were more socially isolated, perhaps as a result of their vulnerabilities or experiences of marginalisation. In line with the social compensation hypothesis, those who perceive their offline social networks to have undesirable characteristics, seek to compensate by developing more extensive online social networks (Valkenburg et al., 2005; Valkenburg and Peter, 2007). This might lead socially isolated young people to interact more with unknown users and create a large number of connections online, possibly taking risks to be accepted and to socially and emotionally compensate for what they are lacking offline.
Because sadness or depression is often disclosed or evident online, some vulnerable young people can be targeted by users who would harm them (May-Chahal et al., 2018). If they are anxious, being behind a screen can offer a disinhibiting effect (Suler, 2004), enabling them to disclose their fears and seek support, or act out more boldly. For those who are socially anxious, the anonymity and invisibility of being behind a screen can create a different dynamic if offline social situations seem threatening or stressful.
Disadvantaged Online
In line with previous research (d’Haenens et al., 2013; El-Asam and Katz, 2018), vulnerable young people in our study reported increased exposure to online risk. They also reported greater perceived negative internet impact, echoing previous findings (El-Asam et al., 2021a). Vulnerable young people in our sample were also found to have less e-safety support, confirming concerns in the literature (Badillo-Urquiola and Wisniewski, 2017; El-Asam et al., 2021a). These outcomes were found to increase with more reported vulnerabilities, highlighting the group’s cumulative and multi-faceted risk of experiencing harm online. This effect is not unique to experiencing harm online; there is evidence that people facing multiple disadvantages have poorer health and academic attainment (Prince et al., 2018) and have repeat contact with homelessness, substance misuse, and offending services (Bramley et al., 2015).
Psychological Distress as a Mediator
Psychological distress in this study represents a combined score of young people’s self-reported depression, anxiety and self-esteem. Results showed that higher psychological distress and poorer social support are associated with greater exposure to online risk and perceived negative internet impact, highlighting the significance of psychosocial wellbeing in young people’s digital lives and adding to previous findings (Livingstone et al., 2011). Previous research has suggested that the relationship between online risk exposure and perceived negative internet impact may be adjusted by psychological wellbeing and resilience (Vandoninck et al., 2013; Wisniewski et al., 2015). This effect was found in the current study, with psychological distress partially mediating the relationship between offline vulnerability and poor internet experience i.e., encounters of online risk. In this sense, it is not only the individual’s “vulnerability condition” (e.g., being in care, being a carer, having special educational needs etc.) which increases their experiences of online risk and perceived negative internet impact. Instead, it is the poorer mental health and increased psychological distress considered as a by-product of these vulnerabilities, which could partially explain why these vulnerable young people are more at risk online. Indeed, being both vulnerable and having psychological distress increased the chances of experiencing online risk and perceived negative internet impact. Given the increased prevalence of poor psychosocial wellbeing in vulnerable groups, these findings raise significant concerns for their protection from harm online.
Parental e-Safety Support
Of significance, our findings demonstrate that parental e-safety support reduces the chances of experiencing negative internet impact, with this effect stronger among vulnerable young people. Yet, in our study, vulnerable young people were less likely to receive parental e-safety guidance, despite this being more beneficial in protecting them from harm online than their non-vulnerable peers. Furthermore, a stepped decrease in psychological wellbeing, parental e-safety support, social support and an increase in online risk and perceived negative internet impact was observed for young people with two or more offline vulnerabilities. This shows a relationship between multiple offline vulnerabilities and poor psychosocial wellbeing, echoing previous findings on cumulative disadvantage (Nurius et al., 2015), whilst demonstrating a novel, linear effect with poor internet experience and poor e-safety guidance.
Poorer parental e-safety support was also found to be associated with higher psychological distress (symptoms of depression, anxiety, and poor self-esteem), and increased exposure to online risks and perceived negative impact. In addition to highlighting the moderating role of parental e-safety guidance in protecting young people against risk online, supporting the importance of parental guidance described in the literature (Khurana et al., 2015; Schilder et al., 2016). These results indicate that mental health is associated with poorer online experiences and emphasise the central role of parental, school, and peer support. A focus on psychological resilience rather than rules alone is likely to improve learning around online safety and reduce risk-taking. For example, efforts to build resilience have been shown to have a positive impact both on risk-taking behaviours and on academic attainment (Public Health England, 2014).
Implications and Conclusion
Exposure to online risks is on the increase among all young people, and more specifically among vulnerable children who are at greater risk of poor mental health. Their psychological distress puts them at further disadvantage online, especially when combined with poor e-safety support. Practitioners working with vulnerable children should enhance their understanding of motivations (e.g., loneliness, attachment problems, social-compensations) behind exposure to online risk and be more informed about risks as a potential consequence of poor mental health combined with inadequate e-safety support.
Previous research highlighted a lack of awareness and training among practitioners who often do not consider the online lives of their vulnerable young people (El-Asam et al., 2020, 2021b). This lack of specialist training was also evident in a study of psychiatry trainees, only 9.7% of whom had received digital risk training (Aref-Adib et al., 2020). Practitioners working with vulnerable young people should make conscious efforts to empathetically explore their online lives. Training could be key, but there is also a lack of psychological tools assessing for online behaviour in general, and online risks specifically. This should motivate researchers to develop and validate new tools to assist practitioners when considering online behaviour and risk.
This is a significant study in that it generated a reasonably large sample of vulnerable young people increasing its potential generalisability; it touches on critical issues in their offline and online lives. It further enhances our understanding of the lives of disadvantaged children and young people and plugs important gaps in the literature.
Research Limitations and Recommendations
This study showed differences between vulnerable and non-vulnerable children in their online lives and psychological distress, particularly children with multiple vulnerabilities who were most disadvantaged online. Psychological distress and poor parental e-safety support were found to play a significant role too. However, it is important to acknowledge that the effect size and the regression coefficients were relatively small (although significant) hence such outcomes need to be treated with caution until further consolidated.
Online and internet-related variables were designed based on consultations with experts and previous research in online risks (e.g., Livingstone and Helsper, 2008; El-Asam and Katz, 2018), as well as e-safety and perceived internet impacts. As a result, this study utilised a large number of questions making such scales more comprehensive and unique. Although these scales have been tested through Exploratory and Factor Analysis, future research could seek to validate extracted factors using Confirmatory Factor Analysis. Additionally, due to the purpose of this study, all risks were combined in one outcome variable. It is advisable to explore each factor separately in relation to vulnerability and mental health.
Vulnerable groups were assumed homogeneous as different vulnerability groups were grouped together. Although they are similar in that they are “disadvantaged” and seem to have higher levels of “psychological distress” compared to their peers, more could be learnt about each vulnerability group’s unique needs to introduce more tailored interventions. Indeed, it could be that different vulnerability groups experience psychological distress differently, perhaps experiencing greater levels of either depression, anxiety, poor self-esteem, or loneliness and may as a result have varying experiences online. Exploring each vulnerable group further would offer greater insight into their needs, allowing for more nuanced recommendations.
This study looked at online risk as an outcome, unlike many other studies which often consider mental health or psychological distress as an outcome of experiences of risk online. The rationale behind our choice was that vulnerable children experiencing psychological distress might resort to the internet to socially compensate for their social isolation and cope with their mental health struggles; some are likely to search for potentially harmful online content. With increased presence online, they are also more likely to establish high-risk relationships leading to potential online victimisations (e.g., cyberbullying and aggression). The Social Compensation Hypothesis and the Online Disinhibition Effect were used in the rationale for this study to explain why vulnerable children are more likely to encounter online risks. However, they were not tested. Future research should seek to examine their role in explaining exposure to online risks among vulnerable children.
Finally, due to schools’ restrictions this data did not include socioeconomic variables such parents’ education, ethnicity, income, and geographical location. These variables would have improved the generalisability of the current findings.
Data availability statement
The raw data supporting the conclusions of this article will be made available by the authors, without undue reservation.
Ethics statement
The studies involving human participants were reviewed and approved by Kingston University London. Informed consent was provided by schools and participants. Participation was voluntary, anonymous and all participants had the right to withdraw from the study. Safeguarding measures were in place between schools and survey administrators.
Author contributions
AE-A contributed toward data collection, manuscript conceptualisation, and writing methods and results sections. AK contributed toward data collection and writing the discussion section. RL contributed toward writing the introduction and the discussion sections. All authors contributed to the article and approved the submitted version.
Funding
This project was funded by the Nurture Network (eNurture). eNurture is funded by UK Research and Innovation (UKRI) and their support is gratefully acknowledged (Grant reference: ES/S004467/1). The authors declare that this study received funding from Nurture Network (eNurture) funded by United Kingdom Research and Innovation (UKRI). The funder was not involved in the study design, collection, analysis, interpretation of data, the writing of this article or the decision to submit It for publication.
Acknowledgments
This manuscript was part of a project titled “Vulnerability, Online Lives and Mental Health: Towards a New Practice Model”.
Author disclaimer
Any views expressed here are those of the project investigators and do not necessarily represent the views of eNurture or UKRI.
Conflict of interest
AK is director of Youthworks Consulting Ltd, a partner in this grant.
The remaining authors declare that the research was conducted in the absence of any commercial or financial relationships that could be construed as a potential conflict of interest.
Publisher’s note
All claims expressed in this article are solely those of the authors and do not necessarily represent those of their affiliated organizations, or those of the publisher, the editors and the reviewers. Any product that may be evaluated in this article, or claim that may be made by its manufacturer, is not guaranteed or endorsed by the publisher.
Supplementary material
The Supplementary Material for this article can be found online at: https://www.frontiersin.org/articles/10.3389/feduc.2022.772051/full#supplementary-material
References
American Psychiatric Association (2013). Diagnostic and statistical manual of mental disorders, 5th Edn. Washington, DC: American Psychiatric Association. doi: 10.1176/appi.books.9780890425596
Annansingh, F., and Veli, T. (2016). An investigation into risks awareness and e-safety needs of children on the internet: a study of Devon. UK. Interact. Technol. Smart Educ. 13, 147–165. doi: 10.1108/ITSE-09-2015-0029
Aref-Adib, G., Landy, G., Eskinazi, M., Sommerlad, A., Morant, N., Johnson, S., et al. (2020). Assessing Digital Risk in Psychiatric Patients: mixed Methods Study of Psychiatry Trainees’ Experiences, Views, and Understanding. JMIR Ment. Health 7:7. doi: 10.2196/19008
Badillo-Urquiola, K., and Wisniewski, P. (2017). “For Those Who Need It Most: Helping “At-Risk” Youth Manage Online Risks,” in Extended Abstract presented at the Workshop on Equity and Inclusivity at the 2017 ACM Conference on Interaction Design and Children (IDC 2017) (Stanford, CA).
Bramley, G., Fitzpatrick, S., Edwards, J., Ford, D., Johnsen, S., Sosenko, F., et al. (2015). Hard Edges: Mapping severe and multiple disadvantage. London: Lankelly Chase Foundation.
Children’s Commissioner (2019). Childhood vulnerability in England 2019. Available online at: https://www.childrenscommissioner.gov.uk/report/childhood-vulnerability-in-england-2019/ (accessed date 4th July 2019).
Department for Education (2020). Special educational needs and disability: an analysis and summary of data sources. Available online at: https://assets.publishing.service.gov.uk/government/uploads/system/uploads/attachment_data/file/882802/Special_educational_needs_and_disability_-_an_analysis_and_summary_of_data_sources.pdf (accessed date June 2022).
d’Haenens, L., Vandoninck, S., and Donoso, V. (2013). How to cope and build online resilience?. London: EU Kids Online.
Doyle, C., Douglas, E., and O’Reilly, G. (2021). The outcomes of sexting for children and adolescents: a systematic review of the literature. J. Adolesc. 92, 86–113. doi: 10.1016/j.adolescence.2021.08.009
Efrati, Y., and Amichai-Hamburger, Y. (2019). The use of online pornography as compensation for loneliness and lack of social ties among Israeli adolescents. Psychol. Rep. 122, 1865–1882. doi: 10.1177/0033294118797580
Eichenberg, C., Schott, M., Decker, O., and Sindelar, B. (2017). Attachment style and internet addiction: an online survey. J. Med. Internet Res. 19:e170. doi: 10.2196/jmir.6694
El-Asam, A., Farooq, J., Lane, R., and Katz, A. (2021a). The digital lives of vulnerable young people: the role of vulnerability, e-safety and psychosocial wellbeing in explaining exposure to online risks. Manuscript unpublished.
El-Asam, A., Lane, R., Pearson, K., and Katz, A. (2021b). The “Glaring Gap”: practitioner experiences of integrating the digital lives of vulnerable young people into practice in England. Inform. Comm. Soc. 2021, 1–21. doi: 10.1080/1369118X.2021.1991976
El-Asam, A., and Katz, A. (2018). Vulnerable Young People and Their Experience of Online Risks. Hum. Comp. Interact. 33, 281–304. doi: 10.1080/07370024.2018.1437544
El-Asam, A., Katz, A., Street, C., Nazar, N. M., and Livanou, M. (2020). Children’s services for the digital age: a qualitative study into current procedures and online risks among service users. Child. Youth Serv. Rev. 122:105872. doi: 10.1016/j.childyouth.2020.105872
Emerson, E. (2003). Prevalence of psychiatric disorders in children and adolescents with and without intellectual disability. J. Intell. Disab. Res. 47, 51–58. doi: 10.1046/j.1365-2788.2003.00464.x
Eyimaya, A. O., and Irmak, A. Y. (2020). Relationship between parenting practices and children’s screen time during the COVID-19 Pandemic. J. Pediat. Nurs. 56, 24–29. doi: 10.1016/j.pedn.2020.10.002
Green, A., Wilkins, C., and Wyld, G. (2019). Keeping Children Safe Online. NCP, Nominent, Parentzone. Available online at: https://www.thinknpc.org/resource-hub/keeping-children-safe-online/ (accessed date 15 July 2019)
Hayes, A. F. (2012). PROCESS: a versatile computational tool for observed variable mediation, moderation, and conditional process modeling. Psychology 2012:2222661.
Hinduja, S., and Patchin, J. W. (2009). Bullying beyond the Schoolyard: Preventing and Responding to Cyberbullying. Thousand Oaks, CA: Sage Publications.
Hinduja, S., and Patchin, J. W. (2012). School climate 2.0: Preventing cyberbullying and sexting one classroom at a time. Thousand Oaks, CA: Sage. doi: 10.4135/9781506335438
Hornor, G. (2020). Online sexual solicitation of children and adolescents. J. Paediat. Health Care 34, 610–618. doi: 10.1016/j.pedhc.2020.05.008
Hu, L., and Bentler, P. M. (1999). Cutoff criteria for fit indexes in covariance structure analysis: conventional criteria versus new alternatives. Struct. Equ. Model. Multidiscip. J. 6, 1–55. doi: 10.1080/10705519909540118
Jones, L. M., Mitchell, K. J., and Walsh, W. A. (2013). Evaluation of internet child safety materials used by ICAC task forces in school and community settings. Final report. Washington, DC: US Department of Justice.
Kardefelt-Winther, D. (2014). A conceptual and methodological critique of internet addiction research: towards a model of compensatory internet use. Comp. Hum. Behav. 31, 351–354. doi: 10.1016/j.chb.2013.10.059
Katz, A., and El-Asam, A. (2020). Look at Me: Teens, Sexting and Risk. A report in The Cybersurvey series in partnership with Internet Matters. Available online at: https://www.internetmatters.org/about-us/sexting-report-look-at-me/ (accessed May 11, 2022).
Khurana, A., Bleakley, A., Jordan, A. B., and Romer, D. (2015). The protective effects of parental monitoring and internet restriction on adolescents’ risk of online harassment. J. Youth Adolesc. 44, 1039–1047. doi: 10.1007/s10964-014-0242-4
Lau, W. W. F., and Yuen, A. H. K. (2013). Adolescents’ risky online behavior: the influence on gender, religion, and parenting style. Comp. Hum. Behav. 29, 2690–2696. doi: 10.1016/j.chb.2013.07.005
Livingstone, S. (2013). Online risk, harm and vulnerability: reflections on the evidence base for child internet safety policy. ZER J. Comm. Stud. 18, 13–28. Available online at: http://eprints.lse.ac.uk/62278
Livingstone, S., Bober, M., and Helsper, E. (2005). Internet literacy among children and young people: Findings from the UK children go online project. London, UK: OFCOM/ESRC.
Livingstone, S., Davidson, J., Bryce, J., Batool, S., Haughton, C., and Nandi, A. (2017). Children’s online activities, risks and safety. A literature review by the UKCCIS Evidence Group. Available online at: https://assets.publishing.service.gov.uk/government/uploads/system/uploads/attachment_data/file/759005/Literature_Review_Final_October_2017.pdf (accessed date October 2017).
Livingstone, S., Haddon, L., Görzig, A., and Ólafsson, K. (2011). Risks and safety on the internet: the UK report. LSE. London: EU Kids Online.
Livingstone, S., and Helsper, E. J. (2008). Parental mediation of children’s internet use. J. Broadcast. Electr. Med. 52, 581–599. doi: 10.1080/08838150802437396
Livingstone, S., and Smith, P. K. (2014). Annual research review: harms experienced by child users of online and mobile technologies: the nature, prevalence and management of sexual and aggressive risks in the digital age. J. Child Psychol. Psychiatry 55, 635–654. doi: 10.1111/jcpp.12197
May-Chahal, C., Palmer, E., Dodds, S., and Milan, S. (2018). Rapid Evidence Assessment: Characteristics and vulnerabilities of victims of online-facilitated child sexual abuse and exploitation. Lancaster: Lancaster University.
McDool, E., Powell, P., Roberts, J., and Taylor, K. (2020). The internet and children’s psychological wellbeing. J. Health Econ. 69:102274. doi: 10.1016/j.jhealeco.2019.102274
Mei, S., Yau, Y. H., Chai, J., Guo, J., and Potenza, M. N. (2016). Problematic Internet use, well-being, self-esteem and self-control: data from a high-school survey in China. Addict. Behav. 61, 74–79. doi: 10.1016/j.addbeh.2016.05.009
Notten, N., and Nikken, P. (2014). Boys and girls taking risks online: a gendered perspective on social context and adolescents’ risky online behavior. New Media Soc. 18, 966–988. doi: 10.1177/1461444814552379
Nurius, P. S., Prince, D. M., and Rocha, A. (2015). Cumulative disadvantage and youth well-being: a multi-domain examination with life course implications. Child Adolesc. Soc. Work J. 32, 567–576. doi: 10.1007/s10560-015-0396-2
Office for National Statistics (2011). Census: Aggregate data (England and Wales) [computer file]. UK Data Service Census Support. Available online at: http://infuse.mimas.ac.uk (accessed May 15, 2022).
Office for National Statistics (2020). Special educational needs in England. Available online at: https://explore-education-statistics.service.gov.uk/find-statistics/special-educational-needs-in-england (accessed date: 16 June 2022).
Ofsted (2020). Children’s Social Care in England 2019. Available online at:https://www.gov.uk/government/publications/childrens-social-care-data-in-england-2019/childrens-social-care-in-england-2019#:~:text=Out%20of%20the%2012%20million,social%20care%20services%20for%20children (accessed date 22 September 2020)
Palmer, T. (2015). Digital dangers: The impact of technology on the sexual abuse and exploitation of children and young people. Ilford: Barnado’s.
Parents Together (2020). Survey shows parents alarmed as kids’ screen time skyrockets during COVID-19 crisis. Available online at: https://parents-together.org/survey-shows-parents-alarmed-as-kids-screen-time-skyrockets-during-covid-19-crisis/?mod=article_inline (accessed date November 8, 2020).
Peter, J., Valkenburg, P., and Schouten, A. (2005). Developing a model of adolescent friendship formation on the internet. Cyberpsychol. Behav. 10, 423–430. cpb.2005.8.423 doi: 10.1089/cpb.2005.8.423
Phippen, A. (2009). E-safety Policy: A Survey of School e-Safety Policy and Practice in the South West of the UK. Exeter: South West Grid for Learning.
Prince, D. M., Rocha, A., and Nurius, P. S. (2018). Multiple disadvantage and discrimination: implications for adolescent health and education. Soc. Work Res. 42, 169–179. doi: 10.1093/swr/svy016
Public Health England (2014). Local action on health inequalities: Building children and young people’s resilience in schools. UCL Health Institute of Equity. Available online at: https://assets.publishing.service.gov.uk/government/uploads/system/uploads/attachment_data/file/355766/Review2_Resilience_in_schools_health_inequalities.pdf (accessed date September 2014).
Raphael, B., Stevens, G., and Pedersen, K. (2006). Vulnerability and mental health. Vulnerab. Child. Youth Stud. 1, 127–138. doi: 10.1080/17450120600863380
Russ, S. A., Larson, K., Franke, T. M., and Halfon, N. (2009). Associations between media use and health in US children. Acad. Pediat. 9, 300–306. doi: 10.1016/j.acap.2009.04.006
Ryan, M., and Tunnard, J. (2012). Evidence about the health and wellbeing needs of children and young people in contact with the youth justice system. London: Department of Health.
Schilder, J., Brusselaers, M., and Bogaerts, S. (2016). The Effectiveness of an Intervention to Promote Awareness and Reduce Online Risk Behavior in Early Adolescence. J. Youth Adolesc. 45, 286–300. doi: 10.1007/s10964-015-0401-2
Sowles, S. J., McLeary, M., Optican, A., Cahn, E., Krauss, M. J., Fitzsimmons-Craft, E. E., et al. (2018). A content analysis of an online pro-eating disorder community on Reddit. Body Image 24, 137–144. doi: 10.1016/j.bodyim.2018.01.001
Stamoulis, K., and Farley, F. (2010). Conceptual approaches to adolescent online risk-taking. Cyberpsychology 4, 966–988.
Suler, J. (2004). The online disinhibition effect. CyberPsychol. Behav. 7, 321–326. doi: 10.1089/1094931041291295
Taanila, A. M., Hurtig, T. M., Miettunen, J., Ebeling, H. E., and Moilanen, I. K. (2009). Association between ADHD symptoms and adolescents’ psychosocial well-being: a study of the Northern Finland Birth Cohort 1986. Internat. J. Circump. Health 68, 133–144. doi: 10.3402/ijch.v68i2.18324
Tonioni, F., D’Alessandris, L., Lai, C., Martinelli, D., Corvino, S., Vasale, M., et al. (2012). Internet addiction: hours spent online, behaviors and psychological symptoms. Gen. Hosp. Psychiatry 34, 80–87. doi: 10.1016/j.genhosppsych.2011.09.013
UNICEF (2020). Children at increased risk of harm online during global COVID-19 pandemic. New York, NY: UNICEF.
Valkenburg, P. M., and Peter, J. (2007). Who visits online dating sites? Exploring some characteristics of online daters. CyberPsychol. Behav. 10, 849–852. doi: 10.1089/cpb.2007.9941
Valkenburg, P. M., Schouten, A. P., and Peter, J. (2005). Adolescents’ identity experiments on the Internet. New Media Soc. 7, 383–402.
Vandoninck, S., d’Haenens, L., and Roe, K. (2013). Online risks: coping strategies of less resilient children and teenagers across Europe. J. Child. Media 7, 60–78.
Vissenberg, J., and d’Haenens, L. (2020). Protecting youths’ wellbeing online: studying the associations between opportunities, risks, and resilience. Media Comm. 8, 175–184. doi: 10.1186/s12913-016-1423-5
Whittle, H., Hamilton-Giachritsis, C., Beech, A., and Collings, G. (2013). A review of young people’s vulnerabilities to online grooming. Aggress. Viol. Behav. 18, 135–146. doi: 10.1016/j.avb.2012.11.008
Wisniewski, P., Jia, H., Wang, N., Zheng, S., Xu, H., Rosson, M. B., et al. (2015). “Resilience mitigates the negative effects of adolescent internet addiction and online risk exposure,” in Proceedings of the 33rd Annual ACM Conference on Human Factors in Computing Systems (New York, NY: Association for Computing Machinery), 4029–4038. doi: 10.1145/2702123.2702240
Wu, Y., Sang, Z., Zhang, X., and Margraf, J. (2020). The relationship between resilience and mental health in Chinese college students: a longitudinal cross-lagged analysis. Front. Psychol. 11:108. doi: 10.3389/fpsyg.2020.00108
Keywords: psychological distress, online risks, parental e-safety support, vulnerable children, online resilience, children and adolescents
Citation: El-Asam A, Lane R and Katz A (2022) Psychological Distress and Its Mediating Effect on Experiences of Online Risk: The Case for Vulnerable Young People. Front. Educ. 7:772051. doi: 10.3389/feduc.2022.772051
Received: 07 September 2021; Accepted: 22 June 2022;
Published: 10 August 2022.
Edited by:
Angela Mazzone, Dublin City University, IrelandReviewed by:
Rosa Goig Martínez, National University of Distance Education (UNED), SpainSebastian Wachs, University of Potsdam, Germany
Copyright © 2022 El-Asam, Lane and Katz. This is an open-access article distributed under the terms of the Creative Commons Attribution License (CC BY). The use, distribution or reproduction in other forums is permitted, provided the original author(s) and the copyright owner(s) are credited and that the original publication in this journal is cited, in accordance with accepted academic practice. No use, distribution or reproduction is permitted which does not comply with these terms.
*Correspondence: Aiman El-Asam, YS5lbGFzYW1Aa2luZ3N0b24uYWMudWs=