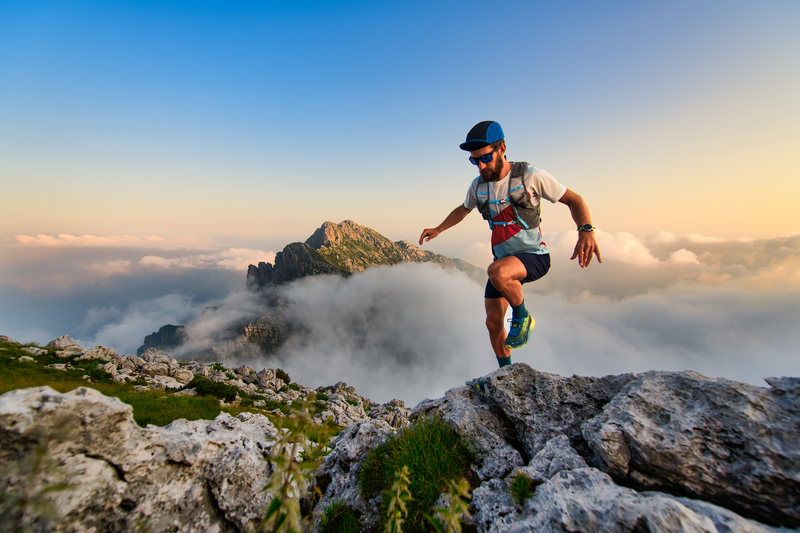
94% of researchers rate our articles as excellent or good
Learn more about the work of our research integrity team to safeguard the quality of each article we publish.
Find out more
ORIGINAL RESEARCH article
Front. Educ. , 17 February 2022
Sec. Educational Psychology
Volume 7 - 2022 | https://doi.org/10.3389/feduc.2022.743770
This article is part of the Research Topic Learning in times of COVID-19: Students’, Families’, and Educators’ Perspectives View all 41 articles
School closures associated with the COVID-19 pandemic very quickly led to many studies on distance education. Currently, there are only studies available that explored the importance of different features of distance education for student learning during school lockdowns in 2020 relying on a single perspective—student, parent, or teacher data. Thus, we present results from a multiple informant study in which we compared prediction models based on the different perspectives of relevant actors in the school system. Against the background of the context, input, process, and output model, we explored the impact of a broad range of features of distance education on central student learning outcomes using data from students (N = 315), parents (N = 518), and teachers (N = 499) in German and Austrian secondary schools. Although findings from relative weight analysis portray a relatively similar pattern of relevant predictors for students’ learning outcomes (i.e., self-rated achievement, learning effort, and intrinsic motivation) across the three respondent groups, some predictors largely differ between the groups. While students’ ability to self-organize emerged as the most significant predictor across all three informant groups, predictors, such as the lack of parental support during school closure, turned out to be relevant only from parents’ perspective. We discuss the implications of these findings for future educational practice and research.
The crisis caused by the COVID-19 virus in 2020 had far-reaching effects in nearly all social areas, including education. Indeed, schools were closed in the spring of 2020 in nearly all European countries (and beyond). Only a few countries, such as Sweden, decided to keep the schools open. In the context of this new and challenging situation of school lockdown, information on how this new situation was experienced by different actors was needed instantly to inform education policy and practice (see Helm et al. (2021) for a first review of approximately 100 surveys conducted in Germany, Austria, and Switzerland). Although studies that investigated the new situation with descriptive analysis have increased sharply since the first school lockdown, explanatory studies that investigated associations between different aspects of COVID-19 pandemic-related distance education are still rare and only just emerging (see Section 4 for a first elaboration). Moreover, to the best of our knowledge, we are not aware of studies that investigate students’ learning during school lockdown from multiple perspectives—that is, using multiple informant groups. Relying on the reports of only one informant group, such as either student reports, parent reports, or teacher reports, although common, runs the risk of biased estimates. Our review (Helm et al., 2021) indicates large differences between student, parent, and teacher ratings on various aspects of distance education. For instance, regarding the lack of technical equipment at home, the student and parent ratings range between 3% and 25% (depending on the survey), whereas teacher ratings range (depending on the survey) between 28% and 75%. Hence, the question arises as to which studies that explore student learning on the basis of different informants (students, parents, and teachers) yield converging findings.
Huber et al. (2020) were the first to launch a comprehensive survey www.schul-barometer.netthat included all three informant groups with around 25,000 respondents between late March 2020 and early April 2020. First results were published as an open access article on 21 April 2020 (Huber et al., 2020) and have been followed by further studies (e.g., Huber and Helm, 2020a). Using data from the international school-barometer survey, we performed a multiple informant study to answer two research questions that have been scarcely explored so far:
1) What are the relevant predictors of student learning during COVID-19 pandemic-related distance education in Germany and Austria in spring 2020?
2) To what extent do the results of Research Question 1 converge if the same analyses are based on data from different informant groups (i.e., students, parents and teachers)? That is, do different perspectives yield the same conclusions about the most relevant predictors of students’ learning during distance education in the spring of 2020?
We attempted to answer these questions against the background of a theoretical framework that is widely used in educational research—that is, the context, input, process, and output model (CIPO, e.g., Scheerens, 1990) and the offer-and-use model (Helmke, 2009). Both models are based on a system theory that describes student learning by a school-initiated transformation process of inputs (e.g., teacher knowledge) into outputs (e.g., student achievement). This process is embedded in a context that provides enabling or disabling conditions (e.g., the composition of the student body). The transformation process itself can be described by quality characteristics (e.g., opportunity to learn). Both the individual components and their relationship to each other must be thought of at different levels of the school system (e.g., school level, classroom level, and individual level). While the internationally widely used CIPO model views the transformation process as a black box, the offer-and-use model, which is widely used in the German tradition of educational effectiveness research, complements this view by describing the instructional process as a co-construction of teachers and learners from a more pedagogical-psychological perspective. Accordingly, teaching does not lead directly to effects. Rather, the transformation process is influenced by whether and how learners perceive the instruction offered and by the motivational, volitional, emotional, and social processes that occur on the student side (Fischer et al., 2011). From an empirical perspective, we base our study on existing educational research on the COVID-19 pandemic, drawn from both a systematic literature review on descriptive studies (Helm et al., 2021) and existing explanatory studies. Based on survey data from students, parents, and teachers, we used structural equation modeling techniques (latent correlations, latent regressions, and latent relative weight analyses) to examine the extent to which the same predictors of student learning in COVID-19 pandemic- related distance education emerged as particularly significant from the perspective of different groups of actors in the school system.
How does distance education in times of the COVID-19 pandemic work from a theoretical point of view? Which aspects of teaching and learning come more to the fore in distance education, which more to the background? In early COVID-19 pandemic-related educational research, many scholars attempted to answer these questions from a theoretical (e.g., Klieme, 2020; Köller et al., 2020; Meyer, 2020; Voss and Wittwer, 2020), quantitative (e.g., Grewenig et al., 2020; Huber and Helm, 2020a; Porsch and Porsch, 2020; Holzer et al., 2021; Steinmayr et al., 2021) and qualitative perspective (e.g., Frohn, 2020; Letzel et al., 2020). In line with some of these early research initiatives (e.g., Wildemann and Hosenfeld, 2020; Züchner and Jäkel, 2021) we adopted the CIPO/offer-and-use logic outlined above to delineate and identify relevant predictors of student learning during the pandemic. More specifically, we used models from homework research (e.g., Trautwein et al., 2006; Kohler, 2011). In line with the offer-and-use model, these models postulate that parental support and parental involvement in students’ learning at home, as well as home resources for learning in general (e.g., socioeconomic background, technical equipment at home), strongly influence the quality and success of home learning processes. A prominent model in the literature is the homework model developed by Trautwein et al. (2006). The homework model is based, among other things, on different motivational theories—especially expectancy-value theory (Wigfield and Eccles, 2000)—and common teaching-learning theories—especially the offer-and-use model (Helmke, 2009).1 While the offer-and-use model represents a comprehensive collection of factors whose impact on the learning process is empirically well established, Trautwein et al. (2006) focused more strongly on aspects that are relevant to homework practice, namely parental involvement, student motivation, quality of homework practice, and student homework behavior. More concretely, Trautwein et al. (2006) postulated that input factors, such as features of the learning environment, characteristics of teachers, students, and parents, parental learning support, etc., affect students’ motivation to learn. Student motivation, in turn, is hypothesized to be a necessary antecedent of students’ homework behavior, which is associated with student achievement.
Although the model focuses on the role of parents in students’ (external) learning, by nature, it makes no claims about distance education during the COVID-19 pandemic. Therefore, we draw attention below to existing assumptions and findings from the still-early research on distance education in the spring of 2020.
In the theoretical frameworks outlined above, the aspects listed below are assumed to be conducive to student outcomes (such as student achievement, student effort, and student motivation) in distance education during the school lockdown caused by COVID-19.
According to constructivist views, learning is always a self-regulated and self-organized process, regardless of whether students learn at school or at home. From a theoretical point of view, self-regulated learning skills can be defined as the ability to plan, monitor, and evaluate individual learning processes and adjust the learning process if necessary (Dignath and Veenman, 2021). Existing theories suggest that learners with high skills in self-regulation engage “actively and constructively in a process of meaning generation and that they adapt their thoughts, feelings, and actions as needed to affect their learning and motivation.” (Boekaerts and Corno, 2005, p. 201) Empirical findings about the significance of students’ self-regulation skills—particularly resource or time management—underpin their central role; especially in forms of digital learning (Broadbent and Poon, 2015). This is true for distance learning during school closures as well. Findings by Blume et al. (2021) reveal that students with higher self-regulation skills are more likely to learn independently and ask for assistance (from parents, peers, or teachers) fewer times. Furthermore, they are more likely to communicate their needs precisely and thus to seek help in a more effective way (Blume et al., 2021).
In the present study, we use the term self-organization to indicate that students’ self-regulation skills needed in distance learning go beyond planning, monitoring, evaluating and adjusting individual learning processes and include other aspects central to independent learning in distance education, such as structuring one’s daily routine, getting up early, shielding oneself from distracting activities, or maintaining an attitude conducive to learning.
Learning during school closures was associated with greater autonomy and increased student responsibility. With the closure of schools, many elements that structure learning were missing, challenging students’ self-organization skills. For instance, during school lockdown in spring 2020, the usual distribution of learning to the morning and leisure activities to the afternoon, the rhythms and structures of learning, for instance by schedules, by structuring lectures of teachers, and by common routines in schools, as well as the structuring of breaks and recreational phases, etc., were dropped. Moreover, important supportive elements of self-regulated and self-organized learning, such as target setting by the teachers, direct affective feedback (i.e., praise, admonition), content-related feedback, cooperative learning, and many more, were dropped too. Thus, distance learning increased demands upon students’ self-regulation (Blume et al., 2021). Consequently, there is no doubt that students’ self-organization and self-regulation skills are considered central for distance education. In line with this assumption, many studies confirmed the strong relationship between self-organization/self-regulation and student outcomes, such as student motivation, engagement, and rated achievement in distance learning (Huber and Helm, 2020b; Grewenig et al., 2020; Blume et al., 2021; Holzer et al., 2021; Korlat et al., 2021; Pelikan et al., 2021; Steinmayr et al., 2021).
The role of parents in children’s and adolescents’ learning at school has come into focus as a result of distance learning. But even before COVID-19 related distance learning, a research tradition was established in which the concept of parental involvement in children’s and adolescents’ learning processes at home was and is still being researched. Parental involvement refers to activities that parents set with the goal of supporting their children’s learning (e.g., Boonk et al., 2018). Based on self-determination theory (e.g., Deci and Ryan, 1985), two domains were distinguished: autonomy-supportive vs. controlling involvement (e.g., Pomerantz and Grolnick, 2017). For example, Moroni et al. (2015) showed that both quantitatively too high levels of involvement and controlling involvement are negatively associated with student achievement, whereas autonomy-supportive involvement has positive effects on achievement.
School closings due to the COVID-19 pandemic promoted parents to teachers—whether they liked it or not. From 1 day to the next, traditional instructional tasks, such as providing individual learning support, had to be taken over by parents. Therefore, the question arose quickly as to the extent to which parents are able to compensate for the loss of services usually delivered by schools and teachers. Early parent surveys (Porsch and Porsch, 2020) reported that in the most frequent cases (between 68% and 71%) parental learning support consisted of checking the correctness and completeness of the students’ assignments. Similarly, the majority of parents solved tasks together with their children. Another major task of parents was to motivate their children in home schooling, as 71% of parents reported in a survey by Wildemann and Hosenfeld (2020). Most often (50% and 46%, respectively), parents resorted to appealing for insight and understanding, or to spending leisure time together (e.g., playing, watching movies). In addition to these descriptive studies, several studies already exist that examine the role of parental involvement in distance learning using multivariate analyses. A recent German study by Sander et al. (2021) focusing on socioeconomic status (SES-)differences in involvement during school closures in spring 2020 found that higher-SES parents and non-German speaking parents paid more attention to the establishment of structures during distance learning (e.g., regular study times). Interestingly, they found that lower-SES parents and non-German speaking parents reported more process-focused learning support (e.g., help to apply meaningful learning methods). Similarly, a study from Portugal (Ribeiro et al., 2021) also found that lower-SES parents were more involved in terms of time in their children’s learning during school closures. Finally, Weber et al. (2021) examined whether social and ethnic disparities in the reading achievement of primary school students have widened during COVID-19-related school closures in spring 2020 and whether disparities were mediated by parental involvement in distance learning. Moreover, a series of mediation models did not provide any support for the hypothesis that parental involvement explained family background effects on reading achievement during the lockdown period.
Due to contact restrictions enacted as part of the pandemic containment measures, the use of digital media represents the only means of transmitting learning materials, learning tasks, and teacher-student communication, in addition to postal delivery or pick-up and drop-off of analog work packages. Hence, sufficient technical equipment for students at home is a necessary prerequisite and condition for initiating learning processes in distance education. Thus, it is not surprising that technical equipment represents one of the most frequently studied issues identified in online surveys conducted regarding COVID-19 pandemic-related distance education. These studies show that the use of digital tools is widespread. Across all surveys reviewed in Helm et al. (2021), an average of 70% of respondents indicated that e-mail was used most often as a means of communication in distance education. This was followed by learning platforms (45%) and the telephone/mobile phone (42%). Lastly, on average, across students, parents, and teachers, around 30% of the respondents stated that video chats and conferencing, messenger services, and the (school’s own) website were used as further communication media. As expected, most student surveys (e.g., Schwerzmann and Frenzel, 2020), parent surveys (e.g., Bezirkselternausschuss, 2020; Langmeyer et al., 2020; Thies and Klein, 2020), and teacher surveys (e.g., Eickelmann and Drossel, 2020; forsa, 2020) showed that digital tools were used much more often at the secondary school level than at the primary school level. Surveys conducted later during the pandemic yielded a higher proportion of respondents reporting that learning management systems, such as MS Teams or Moodle, were used. Hence, there is evidence that the use of these tools has increased over the duration of the pandemic (Helm et al., 2021; Helm and Postlbauer, 2021; Wößmann et al., 2021). Lastly, teacher surveys (e.g., Lorenz et al., 2020; Steiner et al., 2020; Tengler et al., 2020) showed that insufficient technical equipment caused challenges for distance education and thus might have been detrimental to students’ learning outcomes.
Another requirement of learning is an adequate study space (and the associated learning atmosphere). In school-based learning the learning space is provided by schools and thus usually does not vary between students. This is probably why the learning space was hardly perceived and researched as a relevant variable in the teaching-learning process before the pandemic (Talbert and Mor-Avi, 2019). reviewed existing studies in the field of Active Learning Classrooms, i.e., formal spaces in which learners convene for educational activities. The authors conclude that none of the studies encountered aimed to address the question of what specific architectural elements contribute the most to student outcomes. This research imperative will likely be resolved soon due to COVID-19-related distance learning. Many experts argued that in COVID-19-related distance learning students’ an appropriate study place at home is central for students’ learning. Particularly for students from socially disadvantaged backgrounds, it is questionable whether they have their own workplace/room for studying at home. With several children at home, it is also questionable whether a quiet and concentrated learning atmosphere is guaranteed. If this is not the case, an important prerequisite for a high share of learning time is missing.
Generic conceptual frameworks of instructional quality often demarcate three basic quality dimensions (Klieme et al., 2009; Fauth et al., 2014b; Praetorius and Charalambous, 2018) with several inherent facets. Firstly, classroom management comprises teacher actions that maximize students’ time on task and thus ensure an orderly learning environment free of disruption. High levels of discipline and attention arise, for instance, from teachers’ communication of clear rules and their monitoring of student activities. Secondly, the multifaceted construct of student learning support entails various approaches to meet students’ basic psychological needs and thus approaches to foster self-regulated learning. To support students’ experiences of competence, teachers provide differentiated and adaptive instruction, align the pacing of instruction to the learner group in attendance, and give constructive feedback. To enhance students’ experience of autonomy, teachers allow individual choices among (differentiated) tasks and create learning material that is of practical relevance and interest to students’ lives. To foster experiences of social relatedness, teachers demonstrate openness towards students’ opinions and contributions whilst encouraging the students to treat each other in a friendly, considerate, and helpful way. All these means aim to establish a warm and trusting learning climate. Thirdly, cognitive activation results from cognitively challenging tasks, questions, or even demanding problems. Furthermore, teachers who put students’ prior knowledge to the test, elicit and continually explore students’ lines of thinking, or stimulate discursive and co-constructive learning activities also take effective measures to foster students’ more extensive elaboration and understanding of the instructional content.
A vast and extensive number of studies (e.g., Baumert et al., 2010; Kunter, 2013; Fauth et al., 2014b; Förtsch et al., 2016; Schiepe-Tiska et al., 2016; Dorfner et al., 2018; Praetorius and Charalambous, 2018) document positive relationships between the three instructional quality attributes and student outcomes. In particular, classroom management has been found to be most strongly related to student achievement. For cognitive activation, the findings are somewhat more heterogeneous—presumably because cognitive activation in the sense of promoting students’ understanding is more likely to have an effect if it is operationalized as being domain-specific. Social learning support, on the other hand, is assumed to be less of a predictor of student achievement than that of student motivation (which in turn is associated with student achievement), what has been empirically confirmed (e.g., Klieme et al., 2009).
Klieme (2020), Voss and Wittwer (2020), and Meyer (2020) argued on the basis of theoretical considerations that the three constructs of instructional quality also represent important aspects of high-quality in distance education. Initial empirical work on distance education (Frohn, 2022; Helm et al., 2021; Jaekel et al., 2021; Steinmayr et al., 2021) supports these assumptions with both quantitative and qualitative data. For instance, Jaekel et al. (2021) found that teaching methods enabling social connectedness revealed positive associations with students’ and parents’ ratings of instructional quality and students’ learning experiences during distance learning. Finally, Steinmayr et al. (2021) found that distance teaching activities that corresponded with dimensions of teaching quality (e.g., feedback, teacher-child-communication) were comparatively strongly correlated with students’ motivation and learning progress during the school lockdown for both elementary and secondary school students.
In the present study we focus on predicting three central student outcomes in times of school closures: student achievement, student effort, and student motivation. Student achievement and student motivation are traditionally studied in school and teacher effectiveness research as central outcomes of educational processes (e.g., Fraser and Fisher, 1982; Kunter et al., 2005; Klieme et al., 2009). The reason is that cognitive (performance) and non-cognitive/affective (motivation) outcomes are inherent components of the concept of competence (“skill and will,” Weinert, 2002). In addition, we focus on student effort. In line with Skinner and Belmont (1993), we define student effort as the behavioral component of student engagement. That is engaged students exert intense effort and concentration in the implementation of learning task. Therefore, in the present study we focus on students’ learning time. We do so because the shift from face-to-face to distance learning greatly or completely reduced nominal instructional time and learning time. In distance learning students had to take much greater control and responsibility of their own learning time. Hence, student effort represents another central outcome in times of school closures. The three outcomes are mutually related to each other, which is why they are included in all models of the present study as either predictors or outcomes. Thus, reciprocal relations are hypothesized and (partially) empirically confirmed between 1) student achievement and student motivation (Shavelson et al., 1976; Schaffner et al., 2016; Hebbecker et al., 2019), 2) student achievement and student effort (Xu et al., 2018), and 3) student motivation and student effort (Skinner and Belmont, 1993). By analyzing these three outcomes, we focus on a complex but broad web of key variables that are aims of learning processes in traditional instruction and also in distance learning.
Although the first COVID-19 pandemic-induced lockdown in the spring of 2020 was only a year ago, there are already empirical studies that used advanced methods to predict key student outcomes. For example, Champeaux et al. (2020), Grewenig et al. (2020), Zaccoletti et al. (2020), Blume et al. (2021), Nusser et al. (2021), and Steinmayr et al. (2021) used ratings from parents to predict the learning outcomes of children during the lockdown. Dietrich et al. (2020), Huber and Helm C. (2020b), Grätz and Lipps (2021), Holzer et al. (2021), Pelikan et al. (2021) and Züchner and Jäkel (2021) used student data to explain self-reported ability to complete tasks during distance education. These studies identified several variables that significantly predicted the rated student outcomes of primary and/or secondary students during lockdown. In the following paragraphs, we summarize these existing findings as follows. First, we report only predictors of the three student outcomes analyzed in the present paper: student achievement, student effort, and student intrinsic motivation. Second, we initially sort them into predictors that are positively and negatively correlated to the outcomes and then, within these two categories, into individual and contextual characteristics.
Regarding student achievement (assessed by self-measures and external ratings), existing studies have identified the following positively correlated individual predictors: student age (Huber and Helm C. 2020b), students’ subject-specific (e.g., grade point average) and interdisciplinary skills (Züchner and Jäkel, 2021), students’ self-reported ability to use digital media before school closure (Züchner and Jäkel, 2021), and students’ self-organization skills (Huber and Helm C. 2020b). Further, students’ engagement (as reported by their parents, Steinmayr et al., 2021) and students’ self-reported invested learning time (Huber and Helm C. 2020b) positively predict learning success during COVID-19 pandemic-related distance education. Lastly, positive emotions (Huber and Helm C. 2020b) and leisure activities that promote learning, such as reading (Champeaux et al., 2020), are positively associated with student achievement during lockdown. Significantly positively correlated contextual predictors include features of the quality of distance education, such as teacher feedback on learning tasks (Huber and Helm C. 2020b; Steinmayr et al., 2021; Züchner and Jäkel, 2021) and student/parent-teacher communication (Steinmayr et al., 2021) as well as parental satisfaction with school support (Nusser et al., 2021). Negatively correlated individual predictors of learning success include the learning time invested by students at home (Züchner and Jäkel, 2021), the time spent at home in front of the screen, the time spent for extracurricular activities (Champeaux et al., 2020), and negative emotions experienced by students during the pandemic (Huber and Helm C. 2020b). The assessment of student solutions by teachers (Steinmayr et al., 2021) and regular family support for learning (Züchner and Jäkel, 2021) represent negatively correlated contextual predictors of self- and externally rated student achievement. The negative influence of invested learning time and family support is surprising. These unexpected findings are good examples of the challenging interpretation of cross-sectional results. Züchner and Jäkel (2021) argued that these findings might be interpreted in terms of reversed causality:
“Rather, the results of this sample indicate that those who spend more time on tasks and need regular parental support more often have difficulties coping with tasks—possibly family support and the amount of learning time is increased when task coping is less successful.” (Züchner and Jäkel, 2021).
In the study by Huber and Helm C. (2020b), the indicators of the quality of the teacher-student relationship and the quality of the family’s handling of the crisis also showed surprisingly weak negative effects on self-reported learning success. However, the corresponding bivariate correlations were positive. Hence, these findings should be interpreted with caution. Steinmayr and Christiansen (2020) also argued that the observed unexpected negative influence of parents’ assessed language competence of their children on perceived learning success in lockdown should be interpreted with caution, as no theory is able to plausibly explain this negative influence, and as bivariate correlations turn out positive.
When predicting students’ learning time during lockdown, the following variables have been identified as positively correlated individual predictors: age, gender, performance, diligence, emotions. Regarding students’ ages, the findings are heterogeneous. While Grewenig et al. (2020) pointed out that the reduction in learning time due to school closure was significantly less pronounced for younger students, Grätz and Lipps (2021) reported that this reduction was greater for secondary school students than for students older than 18 years. Grewenig et al. (2020) also reported that the reduction in learning time was significantly higher for boys than for girls. Students’ prior performance also matters: low achievers had a significantly greater reduction in learning time than high achievers. In addition to performance, students’ independence (Huber and Helm C. 2020b) and diligence (Grewenig et al., 2020) predict the amount of learning time invested during COVID-19 pandemic. Lastly, at the student level, positive and negative emotions (Huber and Helm C. 2020b) are important positive predictors of students’ effort. However, the positive influence of negative emotions should not be overinterpreted, as this effect is not observable in bivariate analyses and could therefore represent a methodological artifact (see Steinmayr et al., 2021). Positively correlated contextual predictors include school type (more learning time is invested by students from academic track, Grewenig et al., 2020), teaching quality characteristics such as the intensity of teacher support and task control by the teacher (Dietrich et al., 2020; Huber and Helm C. 2020b), learning support from classmates or friends (Dietrich et al., 2020), as well as home/family resources such as regular learning support, handling of the crisis, and technical equipment at home (Huber and Helm C. 2020b; Züchner and Jäkel, 2021). So far, no negatively correlated individual predictors have been identified in the previous studies available to us. By contrast, a few negatively correlated contextual predictors of learning time during COVID-19 pandemic-related school closures were observed. In particular, a home learning environment that is judged to be less conducive to learning (Dietrich et al., 2020) and errands for parents that keep students from learning (Huber and Helm C. 2020b) have a negative effect on the amount of time invested in learning in distance education.2 It should be pointed out that there are also studies (e.g., Nusser et al., 2021) that could not identify any significant predictors of students’ learning time during school closure. This indicates that predicting student learning time in distance education is likely to be difficult.
“Overall, the regression results show that neither gender, type of school attended, nor reading skills measured in the previous year (start of school year 2018/2019; grade 7) have an impact on reported learning time during school closures. The educational background of the parents also has no influence on the mean reported learning time. This means that these characteristics hardly explain the differences in the average learning times of the students per week during the school closures. This is also confirmed by the low variance explanation of 6 percent (R2 = 0.06).” (Nusser et al., 2021, p. 40, p. 40).
Regarding the prediction of students’ intrinsic learning motivation during lockdown, the following positively correlated individual predictors proved significant: In line with self-determination theory, students’ individual experiences of autonomy and competence are positive predictors of learning motivation in distance education (Holzer et al., 2021). Furthermore, a number of students’ learning strategies (study goals and plans, meta-cognition, time management, Pelikan et al., 2021), as well as their learning engagement and diligence (Steinmayr et al., 2021), are positively correlated to students’ intrinsic motivation to learn. Lastly, students’ hours spent at home on extracurricular activities and learning are significant positive predictors of students’ emotional status (Champeaux et al., 2020). Positively correlated contextual predictors were identified in the study by Steinmayr et al. (2021). Teaching quality characteristics, such as student/parent-teacher communication, the frequency of learning tasks sent, and teacher feedback, represent significant predictors of students’ intrinsic motivation. Negatively correlated individual predictors of students’ learning motivation are students’ age (Huber and Helm C. 2020b; Zaccoletti et al., 2020), students’ negative emotionality (Steinmayr et al., 2021), students’ individual social inclusion and self-regulated learning (Holzer et al., 2021), and students’ procrastination (Pelikan et al., 2021). Negatively correlated contextual predictors are the grading of learning tasks by teachers (Steinmayr et al., 2021).
Having presented the state of research regarding predictors of student outcomes in COVID-19 pandemic-related distance education, we want to point out methodological issues that make it difficult to compare and contrast the findings of the different studies. First, these studies used different effect sizes (e.g., unstandardized vs. standardized coefficients). Second, some studies (e.g., Huber and Helm C. 2020b; Steinmayr et al., 2021; Züchner and Jäkel, 2021) reported possible suppression effects, which can lead to unexpected findings.3 Third, the studies used different informants; that is, they were either based on data from student surveys or from parent surveys. Given the different perspectives associated with different validities and biases, the question arises as to the extent to which the findings of these studies, which are based on different informant groups, are comparable or similar.
The previous framework outlined describes the relationship between context, input, process, and output variables. However, social and ecological theories (Bronfenbrenner, 1996; Bandura, 2001) postulate that these variables are perceived differently (and their interrelations are differently pronounced) depending on who is asked to provide information on these variables. For example, Mitchell et al. (2010, p. 272) argued that although actors in a school (e.g., students and teachers) “share a common objective experience, their differing roles within the school will likely lead to discrepant perceptions of the environment.” Different factors at multiple levels within the school influence each actor’s perception. There are a number of reasons given in the literature for differences in perceptions between students and teachers, all of which can be more or less attributed to the different positions of these actors in the school system (i.e., perspective validity and bias):
• Role-specific knowledge: Students, parents, and teachers each have role-specific knowledge and experience due to their specific positions, making them experts in their domains (Baumert et al., 2004; Wagner et al., 2010; Fauth et al., 2014a; Wettstein et al., 2018). For instance, teachers have pedagogical expertise that allows them—in comparison to students and parents—to better (or more adequately) assess certain aspects of instruction (e.g., achievement of instructional goals; instructional disruptions).
• Role-specific socially desirable response tendency: Depending on the position, different informants display different social response behaviors (What is expected of me in my position?). In the literature, this effect is called “wishful thinking” (Krammer et al., 2019), “impression management” (Wagner et al., 2010), “self-serving strategies” (Aldrup et al., 2018), “cheerleader effect” (Bingham et al., 1993), etc.
• Role-specific goal orientation: Informant-related differences in assessments can also result from different tasks and goals. For example, teachers’ tasks and goals are to teach material and foster students’ understanding, while students often aim to interact with other learners. This conflict of goals can lead to different perceptions of, for example, classroom disruptions (Wettstein et al., 2018).
• Role-specific situational framework of the rater: In class, the teacher is confronted with high social density, unstructured problems, dynamic situations, and multiple demands; and he/she must act under pressure. Students observe lessons from a largely comfortable situation and thus have an observational advantage over the teacher (Wettstein et al., 2018).
• Role-dependent difficulty of assessment: Some aspects are more difficult to assess, depending on the position of the rater (Brok et al., 2006; Wettstein et al., 2018; Krammer et al., 2019). For instance, some behaviors, such as clarity or strong control, “may also be easier to self-assess, raising the question of whether teachers and students base their responses on the same observational cues” (Krammer et al., 2019, p. 598).
• Role-dependent assessment focus: It is also conceivable that students, teachers, and parents each focus on different aspects when assessing certain dimensions (e.g., classroom structure and clarity), and thus each group of individuals “might add a particularly valuable perspective” (Kunter and Baumert, 2006; Aldrup et al., 2018, p. 1069).
• Role-specific variables influencing ratings: Studies (Desimone et al., 2010; Mitchell et al., 2010; Aldrup et al., 2018; Cipriano et al., 2019) show that ratings of different informants depend on characteristics of the individuals and respective contexts. For example, at the individual level, background variables such as students’ gender, age, socioeconomic status, ethnicity, motivation, prior achievement, and teacher popularity were shown to influence student ratings. For teachers, similar characteristics, such as gender, age, ethnicity, teaching experience, teacher self-efficacy and beliefs were related to teacher ratings. At the classroom level, high proportion of students with disruptive behavior problems, classroom climate, and teacher-student relationships were identified as predictors of student ratings. Finally, at the school level, staff and student turnover as well as student-teacher ratio were observed to influence assessments of school climate.
The manifold reasons for systematic differences between different informant groups outlined above are often cited as reasons for the lack of validity of self-assessment data. However, because survey data is often collected and used to inform decision-making, the data should be reliable and valid (Desimone et al., 2010). Hence, approaches that foster increased validity in surveys are needed. Multiple informant (report) studies or assessments (also called cross-informant studies/assessments) represent such an approach. In multiple informant studies the information of interest is collected from different perspectives—that is, groups of interest, such as students, parents, teachers, and school leaders. Multiple informant studies capture the unique perspectives of different informants on the same item (i.e., a question or statement). An underlying assumption is that different informants each have unique and valid perspectives (De Los Reyes et al., 2013); see the argument of the role-dependent assessment focus above.
Closely related to the validity problem of single informant studies is the common method bias/variance problem. Single-informant studies run into methodological problems such as common method bias (Podsakoff et al., 2003). If a single method (e.g., online questionnaires) and one source of information (e.g., students) are used, it is likely that the observed associations among the study variables are, to some degree, attributable to the single method used for all items. According to Podsakoff et al. (2003, p. 887), a major cause of common method bias is assessing study variables (i.e., predictors and criteria) from the same rater or source, and “one way of controlling for it (common method bias) is to collect the measures of these variables from different sources.” Thus, various biases (e.g., social desirability, lenient tendencies, implicit theories, dispositional, and transient mood states) can be avoided (Podsakoff et al., 2003, p. 887).
Regarding research related to COVID-19 and distance learning, existing studies run the risk of the problems listed above as they often examine very similar research questions but draw on single informant groups. For example, Champeaux et al. (2020), Zaccoletti et al. (2020), Blume et al. (2021), and Steinmayr et al. (2021) examined predictors of central student outcomes in distance education based on parent data, whereas Huber et al. (2020), Pelikan et al. (2021), Holzer et al. (2021), Züchner and Jäkel (2021) relied on student data. Further, central aspects of students’ learning processes during COVID-19 pandemic-related school lockdown, such as students’ learning progress and the instructional quality of distance education, are often assessed by teacher ratings only (i.e., Lorenz et al., 2020). Therefore, teachers’ data represent another important source for analyzing predictors of student learning success during COVID-19 pandemic. Given the role-specific influences discussed above, this raises the question of whether the use of the same questionnaire items yields the same findings regardless of the group inquired, or whether they are more likely to map perspective validities (e.g., Kunter et al., 2007). Hence, multiple informant studies on COVID-19 related distance learning are of particular interest. To date only a limited number of descriptive surveys have made use of multiple informants to increase validity (Bildungsdirektion, 2020; Huber et al., 2020; Letzel and Pozas, 2020; Schwab et al., 2020; Schwerzmann and Frenzel, 2020; Tengler et al., 2020; Trültzsch-Wijnen and Trültzsch-Wijnen, 2020; Garrote et al., 2021). However, to the best of our knowledge, there are no explanatory studies investigating student outcomes during lockdown that make use of multiple informants.
To sum up, our present study is motivated by the aim of showing that different informant sources may reveal different findings and lead to different conclusions. This is particularly important for COVID-19-related educational research as parents’ ratings are often used—which is rarely the case in “traditional” school and teaching effectiveness research. Hence, the validity of parents’ ratings is of high interest in COVID-19-related educational research. Therefore, our goal is to close this research gap by examining the impact of a broad range of features of distance education on central student learning outcomes using reports from multiple informants, that is, students, parents, and teachers from German and Austrian secondary schools.
The findings in the present paper are based on data from the second measurement occasion of the school-barometer survey (www.schul-barometer.net), which was conducted from 11 June 2020 to 22 July 2020 in Germany, Austria, and the German-speaking regions of Switzerland, including all stages of compulsory education (i.e., primary, lower secondary, and upper secondary school). We developed online questionnaires for students, parents, and teachers. We carefully recorded the same constructs with the same items in all questionnaire versions so that we could perform multiple informant analyses of predictors of student outcomes from all three perspectives.
As the response rate was low for the Swiss sample, as well as for the primary school type, we decided to exclude these samples and focus on secondary school data from Germany and Austria. In order to maintain a sufficient sample size for all three survey groups, we decided against conducting the analyses based on data from one country. However, pooling the data from Germany and Austria runs the risk of further increasing the heterogeneity in the data. Although the education systems and also the education policy responses to the COVID-19 pandemic are very similar in the two countries, they are not identical. Nevertheless, in distance learning aspects of different educational systems are assumed to be of less importance when predicting students’ learning outcomes because students do learn outside the school system in their homes and families which are assumed not to differ systematically between the two countries. Nevertheless, to exclude the influence of the country on the findings, we included country as a control variable in the subsequent analyses.
Table 1 provides information on the composition of the three assessed informant groups (students, parents and teachers). The ratio of the school type is quite similar across the parent and teacher sample (54% and 51%). Only in the student sample low secondary students are less strongly represented (38%).
For reasons of anonymity and ease of data collection, we refrained from collecting matched data. That is, we did not design the data collection process in a way that would have allowed links to be made between students, parents, and teachers; for instance, by means of a self-generated code. Thus, the analyzed samples are “separate samples of each group.”
Table 2 provides an overview of the constructs assessed in the school-barometer survey and analyzed in this paper. All of the items were self-developed but validated in previous research (Huber S. G. and Helm C., 2020). The response options for all the items ranged from 1 (strongly disagree) to 5 (strongly agree). Only student effort was assessed with an item that ranged from 1 to 40 h a week.
TABLE 2. Descriptive statistics and reliability information for the study variables in the three samples.
Information on the descriptive statistics (mean, standard deviation) and the reliability (Cronbach’s alpha) of the constructs are provided in Table 2 for the three different informant groups. In examining the mean values of the constructs, lack of technical equipment, lack of parental support, and student’s workplace at home showed comparatively low/high approval ratings in the student and/or parent sample, which may indicate bottom/ceiling effects. That is, many students and/or parents (but not as many teachers) reported that the lack of technical devices, the lack of parental learning support, and the quality of students’ workplace at home were not detrimental for distance education. These three predictors showed quite low standard deviations. Due to the low variance in these constructs, they are less likely predictive of student outcomes and less likely to be predicted by other variables themselves.
A closer look at the group differences in the descriptive statistics offered the following insights: The effect size (Cohen’s d) of the group mean differences (of at least one of the three possible group comparisons) is relevant (i.e., d > 0.5) for all constructs, except for student effort. The reported average number of hours students invest does not significantly differ between the three informant groups. However, regarding all other constructs several different pictures emerged. In general, student ratings have the highest average approval rate, except regarding the two instructional features, learning support and structure, which were rated more positively by the teachers. While teachers tended to rate instructional features comparatively positively, parents tended to rate these features comparatively less positively. By contrast, students and parents rated home learning resources (parental support, workplace at home) comparatively positively, while teachers rated these resources comparatively less positively. Lastly, students rated their learning progress and learning motivation, as well as their self-organization skills, significantly better than parents and teachers did. The descriptive results also showed that most of the constructs assessed by teacher ratings have comparatively low standard deviations. Teachers seemed to agree more strongly regarding the evaluation of the constructs assessed.
Regarding the internal consistency of the scales, the low alpha values (below 0.6) of the constructs teachers’ support of cognitive activation, lack of parental support, and student’s workplace at home across all three informant groups were striking. The low values were primarily explained by the low number of items (two for each construct) that were available in the data for the operationalization of the respective construct. Across groups, the reliability values were lowest for the instructional features in the teacher sample. Beyond that, however, no further obvious deviations were discernible in the reliability values.
According to the research questions outlined in the introductory section, we aimed to identify the most relevant predictors of students’ learning during COVID-19 pandemic-related distance education. Moreover, we aimed to identify relevant differences in the importance of the predictors between the three respondent groups. To this end, the following statistical procedures were conducted separately for each informant group: students, parents, and teachers.
First, based on measurement error-adjusted constructs, we analyzed latent correlations to uncover bivariate relationships between various aspects of distance education and central student outcomes. Second, by means of latent regression analysis, we tested the extent to which the latent bivariate relations observed in analysis step (1) persisted when the influence of all predictors was modeled simultaneously. Lastly, based on latent bivariate correlations from analysis step (1), we performed a latent relative weight analysis (Tonidandel and LeBreton, 2011) to extend the findings from steps (1) and (2) regarding the following aspects:
• RWA assesses the direct and indirect effects of the predictors on the criterion variables and therefore provides information on the total effect of the predictor variables on the outcome variables.
• RWA illustrates the contribution of the respective predictor (i.e., aspect of distance education) in explaining the variance in the outcome variables (i.e., student achievement, student effort, and student motivation in distance education).
• In RWA, in contrast to regression analysis, high multicollinearity between constructs is not problematic (Stadler et al., 2017). Unlike in regression analysis, constructs do not need to be excluded if they are highly associated with other predictors.
To identify relevant RWA-differences between the respondent groups, we applied the following general principle: we only interpreted differences (∆) between two informant groups which were higher than 5%. Like the p value this number was arbitrarily chosen. However, Hattie (2009) ground-breaking work argues that an effect size (Cohen d value) of more than 0.40 is of relevant magnitude in educational science. This effect size equals an R2 difference of 4% and larger. Hence, we submit that a difference in R2 greater than 5% should work acceptably as a plausible threshold for relevant effects.
To conclude the analysis strategy, we would like to point out that a multi-group comparison would be the most effective way to analyze latent differences between two or more groups. However, we opted for relative weight analysis (RWA) as major analysis strategy as to our knowledge there is no software that does RWA for multiple groups simultaneously.
As described in the previous section, we estimated a latent correlation matrix for each survey group, i.e., students, parents and teachers (see Tables 4–6 in the text for the correlations of interest and Supplementary Tables SA7–SA9 for the full correlation matrix). In addition, we estimated 9 latent regression analyses (see Table 7); specifically, for each combination of outcome (i.e., student achievement, student effort, student motivation) and survey group (3 × 3 design). Each of the latent correlation matrices and latent regressions represent statistical models which we evaluated using common model fit indices (Little, 2013): the Bentlers comparative fit index (CFI ≥ 0.90), the Tucker-Lewis index (T LI ≥ 0.90), the root mean square error of approximation (RMSEA ≤ 0.08), and the standardized root mean square residual (SRMR ≤ 0.10). See Table 3 for the model fit evaluation.
Across all three datasets, the share of missing values was lower than 5% for the vast majority of items. The highest share of missing values for a single item was around 10% (students: 9%, parents: 12%, teachers: 12%). We used full maximum information likelihood (FIML) estimation, as employed in lavaan (Rosseel, 2012), to impute missing values. A central assumption of the FIML procedure is that conditioning on all variables in the analysis, the missing data of the analysis variables is missing at random; that is, the missing data is independent of the level of other variables in the analysis. Since our analyses did not include variables that are assumed to be related to missing data, such as objective student achievement and socioeconomic background, this assumption may not be entirely fulfilled. However, given the low share of missing values, we consider this a minor problem for the reliability of our findings.
Prior to the analyses of the associations between the study variables, we checked for measurement invariance of the assessed constructs between the three informant groups; that is, whether the items used in this study equally assessed the same construct in all three informant groups. Different types of measurement invariance have been distinguished in the literature. Most common are configural, metric, and scalar measurement. As we only investigated latent associations between the study variables in the present study, and because latent mean comparisons of the study variables are not of interest, the confirmation of configural and metric invariance is sufficient (Byrne et al., 1989; Temme and Hildebrandt, 2009). The confirmation of stronger types of measurement invariance, such as scalar invariance, is not needed but reported. The measurement invariance tables are provided in the Appendix. Metric invariance was assessed using the rule of thumb according to Chen (2007) and Cheung and Rensvold (2002). If the model fit does not drop too much; that is, as long as the CFI does not decrease by more than 0.005–0.010 units and the RMSEA does not increase by more than 0.015 units), metric measurement invariance can be assumed. The tables in the Appendix show that for all constructs except the constructs lack of technical equipment at home and teachers’ support of students’ learning, metric invariance is given. With regard to the construct teachers’ support of students’ learning, partial metric invariance is obtained if the loading of the item The completion of the learning/teaching tasks was monitored by the teachers during the school closure is allowed to vary across the three respondent groups.
All statistical analyses were conducted using the statistical software, R (R Core Team, 2014). The R package lavaan (Rosseel, 2012) was used to estimate the measurement models, latent correlations, and the latent regressions. To conduct RWA, a statistical code was written in R using (Johnson, 2000) formula.
In Table 3 we present indices for evaluating the model fit of a) the latent correlations of all study variables (shown in Tables 4–6; Supplementary Tables SA7–SA9) and b) the latent regression analysis for each of the three outcome variables and the three samples (shown in Table 7). The indices point to an acceptable model fit for all models estimated. That is, with exception of T LI the indices are above (CFI) or below (RMSEA, SRMR) the cut-off values suggested in the literature. According to the simulation study by Shi et al. (2019), CFI and T LI values can be underestimated when large models are estimated based on small samples. Hence, we argue that our estimated models are sufficiently well able to reproduce the data (i.e., the variance-covariance matrix) and thus are reasonably consistent with the data.
See Supplementary Tables SA7–SA9 for the full correlation matrices.
Table 4 (see the left part) shows that irrespective of the informant (i.e., students, parents or teachers) chosen, self- and externally assessed student achievement was (most) strongly related to students’ intrinsic motivation and self-organization skills. In addition, we observed strong correlations between student achievement and the lack of parental learning support, as well as quality features of distance education (teachers’ support of structure in online lessons and teachers’ maximization of learning time) in the parent dataset. The statistical tests of the differences between the correlations of two different samples (see the right part of Table 4) showed that a couple of associations were significantly higher in the teacher dataset than in the other two datasets (self-organization skills, lack of technical equipment at home, school type). By contrast, some associations were significantly lower in the teacher dataset than in the other two datasets (teachers’ support of individual learning and teachers’ support of structure in online lessons). Student effort was significantly more strongly associated with student achievement in the parent and teacher datasets than in the student dataset. Lastly, workplace at home was significantly less strongly associated with achievement in the parent dataset than in the other two datasets.
Table 5 (see the left part) shows that irrespective of the informant (i.e., students, parents or teachers) chosen, student effort was (most) strongly related to students’ self- and externally rated achievements and self-organization skills. In addition, negative correlations between student effort and the lack of technical equipment at home were observed in the teacher dataset. The statistical tests of the differences between the correlations of two different samples (see the right part of Table 5) showed that a few of associations were significantly higher in the teacher dataset than in the other two datasets (students’ intrinsic motivation, lack of technical equipment at home, teachers’ support of structure in online lessons, workplace at home). Students’ effort was more strongly linked to school type and teachers’ maximization of learning time in the teacher sample than in the parent sample. Furthermore, achievement was less strongly correlated with student effort when assessed by student data than by parent or teacher data. By contrast, students’ self- organization skills were less strongly associated with students when we used parent data instead of student and teacher data.
Table 6 (see the left part) shows that irrespective of the informant (i.e., students, parents or teachers) chosen, student intrinsic motivation was (most) strongly related to student achievement (see above) and to student self-organization skills. We observed a negative correlation between student motivation and a lack of parental support in the parent data. The statistical tests of the differences between the correlations of two different samples (see the right part of Table 6) showed that a couple of associations were significantly higher in the teacher dataset than in the other two datasets (student effort, lack of technical equipment at home). Features of instructional quality during distance education (teachers’ support of individual learning and teachers’ support of structure in online lessons) were less strongly correlated with student motivation in the teacher data than in the parent data. Workplace at home was less related to the outcome in the parent data than in both other datasets. Lastly, the lack of parental support during lockdown was most strongly related to student motivation in the parent dataset—and significantly higher than in the other two datasets.
The findings from bivariate latent correlation analyses indicate that only students’ self-organization skills were significantly related to all three outcomes across all three informant groups. However, regarding all other predictors of student learning during lockdown, the presented analyses revealed different patterns between the different informant groups. These differences underscore the need for cautious interpretation of findings based solely on one informant group, as such findings may be colored by a single perspective.
Latent correlation analyses show that many of the study variables were significantly related with each other. This raises the question of whether and to what extent the predictors of student outcomes are confounded, and thus lead to spurious relationships among the predictors and the outcome variables. Therefore, there is a risk of reporting spurious correlations; that is, two variables are associated but not causally related due to either coincidence or the presence of a certain third, unseen variable. To prevent this risk of misinterpretation, we performed multivariate regression analyses that controlled for other relevant variables by including them as explanatory variables. Table 7 shows the results of nine different regression models. For each of the three informant groups, the three student outcomes are predicted by all other study variables. However, some of the very high regression coefficients—particularly when student effort was regressed on the dimensions of instructional quality (teachers’ support of individual learning: β = −1.515, teachers’ support of structure in online lessons: β = 0.922 and teachers’ maximization of learning time: β = 0.812)—and some of their huge standard errors (S.E. = 1.076–2.453) point to issues of multicollinearity and are thus not trustworthy. Moreover, the high latent correlations observed in Supplementary Tables SA7–SA9 (e.g., between teachers’ support of individual learning and teachers’ maximization of learning time, r = 0.778) also point to multicollinearity problems. These issues call for other analytic approaches that are less sensitive to multicollinearity, such as relative weight analyses.
Table 7 provides information on the latent regressions predicting central student outcome variables in the three different samples.
Tables 8–10 provide information on the results of the relative weight analyses (RWA) for the three different informant samples. Each table shows the absolute and relative R2 values. Absolute R2 values refer to each predictor’s absolute contribution to the total explained variance in the outcome variable (left part of the tables). The tables also show the relative size of the absolute share when set in relation to the total explained variance (right part of the tables). As highlighted above, RWA values assess the direct and indirect effects of the predictors on the criterion variables and therefore provide information on the total effect of the predictor variables on the outcome variables. Following this interpretation, Tables 8–10 present the following findings:
Depending on the informant sample, the total variance in student achievement was explained to 55%–69% by the study variables. In examining all three informant samples, students’ intrinsic motivation emerged as the most important predictor of self-rated and externally rated student achievement during school-lockdown. Whereas 19% and 20% were explained from the student and teacher perspective, respectively, only 14% were explained from the parents’ point of view. From parents’ perspective, the lack of parental support during distance education was even more important (17%). Students’ self-organization skills ranked second among the most relevant conducive features of distance education during the pandemic lockdown (9%–18%). The dimensions of instructional quality of distance education, as well as student effort and the quality of the workplace at home, ranked in the middle when we averaged the values across informant groups. However, the scatter was high, ranging from 1% to 9% in the student sample, for instance. Technical equipment at home and school type proved to be the least relevant when predicting students’ self-rated and externally rated achievement during COVID-19 pandemic-related distance education (0%–4%).
Depending on the informant sample, 22%–34% of the total variance in student effort was explained by the study variables. By examining all three informant samples, students’ self-rated and externally rated achievement, as well as students’ self-organization skills, emerged as the most important predictors of the hours spent a week by students for learning and school issues during school closure. From the students’ and teachers’ perspectives, student self-organization skills seemed more relevant (8%–5%), whereas student achievement was more predictive if parent assessments were used (11%). Again, the dimensions of instructional quality of distance education ranked in the middle when we averaged the values across informant groups. However, the scatter was high, ranging from rather low values in the student and parent samples (i.e., 1%) to higher values in the teacher sample (i.e., 6%). Lack of parental support, technical equipment at home, and school type proved to be the least relevant when predicting students’ learning effort in hours per week during COVID-19 pandemic-related distance education (0%–2%).
Depending on the informant sample, 69%–75% of the total variance of students’ intrinsic learning motivation was explained by the study variables. In considering all three informant samples, student self- organization skills emerged as the most important predictor of the students’ intrinsic learning motivation during school-lockdown. From students’ and parents’ perspectives, around 30% of variation in student motivation was explained (30% and 28% respectively); however, student self-organization skills were less relevant from the teacher perspective (22%). Self-rated and externally rated student achievement emerged as the second most important predictor, with similarly high explanatory contributions across samples (ranging between 15% and 20%). As with the prediction of student achievement and student learning time per week, the quality dimensions of distance education also ranked in the middle for the prediction of student intrinsic motivation. However, the RWA values were quite low, as was the scatter of the contributed shares for explaining the variation in the motivation variable (2%–7%), indicating a comparable relevance of the quality characteristics in all three informant groups. The rest of the study variables only contributed marginally to the prediction of students’ intrinsic learning motivation (e.g., student effort: 1%–2%, school type: 0%–2%).
In addition to identifying significant predictors of student outcomes in the time of COVID-19 pandemic, the goal of this study was to examine the extent to which different sources of information lead to the same findings. We investigated the difference reported in Tables 8–10 to obtain first indications.
Regarding the prediction of self-rated and externally rated student achievement, the main differences between the three informant groups are as follows: When we used students as informants, perceived teachers’ support of structure in online lessons was more relevant than when teachers were considered as informants (∆ = + 7% R2 contribution). When we used parents as informants, the predictors of lack of parental support (∆ = + 15–16% R2 contribution) and student effort (∆ = + 4 to 6 percent point R2 contribution) were of higher relevance than when using student or teacher reports. By contrast, students’ intrinsic motivation (∆ = −5–6% R2 contribution) was of lower relevance in the parent data than in the student and teacher data. Lastly, student self-organization skills (∆ = + 8–9% R2 contribution) were much more relevant if analyses were based on teacher data.
Regarding the prediction of student effort, the main differences between the three informant groups are as follows: Self-rated and externally rated student achievement yields a much higher R2 contribution in the parent data as opposed to the student and teacher data (∆ = + 7–9% R2 contribution). In addition, student self-organization skills were more relevant in the student data analysis than in the parent data analysis (∆ = + 7% R2 contribution).
Regarding the prediction of student intrinsic motivation, the main differences between the three informant groups are as follows: If the analysis was performed on the basis of the teacher data, students’ self-organization skills represented a less relevant predictor when compared to the analyses conducted on the basis of the student and parent data (∆ = −6–8% R2 contribution). As with the prediction of student self-rated and externally rated achievement, the lack of parental support was only relevant when we used parents as informants (∆ = + 6–7% R2 contribution). Lastly, the relevance of the predictor workplace at home differed between students’ and parents’ perspectives, with higher R2 contributions when assessed based on student reports (∆ = + 6% R2 contribution).
COVID-19 pandemic-related school closures opened a completely new, entirely unstudied field for educational research, which very quickly attracted media attention too. The sudden and high demand for information from different stakeholders has led to an abundance of descriptive survey studies being presented very quickly, while explanatory studies on the prediction of student outcomes during the pandemic are still rare. Moreover, most studies have been based on only one source of information (e.g., students, parents, or teachers). The question remains as to what extent the findings obtained in this way are subject to perspective bias.
The present study fills these research gaps by presenting initial findings on the prediction of students’ self- and externally-assessed learning success, learning engagement, and intrinsic motivation to learn during the COVID-19 pandemic based on student, parent, and teacher data.
Across all informant groups, students’ intrinsic motivation and self-organization skills emerged as the most important predictors of self-rated and externally rated student achievement during school lockdown, while instructional quality during distance education only contributed little to explaining rated learning success. These findings extend existing research that has previously identified leisure activities conducive to learning, such as reading (Champeaux et al., 2020), teaching activities such as feedback, student communication, and student engagement (Steinmayr et al., 2021), and self-reported ability to use digital media before school closure (Züchner and Jäkel, 2021).
Regarding student effort, students’ self-rated and externally rated achievement, as well as student self- organization skills, emerged as the most important predictors of students’ effort across all informant groups. Again, instructional quality during distance education contributed little to explaining students’ learning effort. The predictive power of rated student achievement is in line with the results from a study by Grewenig et al. (2020), who reported that high-achieving students invested more hours in school activities during COVID-19 pandemic. Although, prior research has identified several further predictive aspects of students’ effort, such as students’ learning environment at home, social support of classmates, teachers’ support intensity (Dietrich et al., 2020), students’ socioeconomic background, school type (Grätz and Lipps, 2021), and regular family learning support (Züchner and Jäkel, 2021), the present study adds new relevant predictors.
Students’ intrinsic motivation during COVID-19 pandemic-related distance education was most strongly determined by students’ self-organization skills and self-rated and externally rated student achievement across all informant groups. Again, instructional quality during distance education contributed little to explaining students’ intrinsic motivation during distance education. In line with the findings by Holzer et al. (2021), our results indicate that—as postulated by self-determination theory (Deci and Ryan, 1985)—students that perceive competence are more likely to report higher intrinsic motivation during distance education. Further, in line with previous research (Pelikan et al., 2021), our findings show that students with higher self-organization skills are more strongly intrinsically motivated during distance education.
With respect to our second research question regarding whether different informant groups yield different findings, our study did not reveal a clear picture. Following social cognitive theory, we expected that those aspects which are assumed to be more strongly in the focus of the respective group (according to their role) should have significantly higher importance for the prediction of the student outcomes. For example, instructional quality in distance learning represents aspects for which teachers are responsible and which are part of their daily work. Therefore, it can be assumed that these aspects are particularly in the focus of the teachers and that they are also given a higher importance in the context of instructional processes. Accordingly, these characteristics are expected to have a stronger predictive power for predicting outcomes in the teacher sample than in the student and parent sample. However, as the results from RWA analysis show, this assumption is not supported by the data. The R2 contributions of the instructional quality dimensions (sup, str, mlt, coa) do not vary significantly across the three samples. Only parental support (par) proves to be particularly predictive for all three outcomes in the parent sample, significantly more so than in the student and teacher sample. Considering all the findings, however, the assumption that some aspects can be assessed more “validly” (in the sense of a higher predictive validity) by one group than by other groups, is not confirmed in the present study.
In summary, the present study adds two aspects to the existing research:
1) Substantively, the findings point to the important role of learners’ self-organization skills in coping with COVID-19 pandemic-specific school closures.
2) Methodologically, the findings point to relevant but not coherent influence of the selected samples as sources of information.
This study has some theoretical limitations.
First, from a theoretical point of view we did not consider reciprocal effects between our study variables, particularly between the dependent and independent variables. For instance, in studies on self-regulated learning, intrinsic motivation leads to a higher level of implemented learning strategies; accordingly, assuming and modeling self-organization skills as predictors of motivation should be carefully reflected. Our assumption was that in the context of distance learning students with higher self-organization skills are less overwhelmed and experience competence more frequently than students with lower self-organization skills. Therefore, we assumed students’ self-organization skills being a central predictor of students’ motivation. However, with our study design we cannot examine (and exclude) reciprocal effects. Nevertheless, RWA does model all indirect associations of the specified predictors (Johnson, 2000). That is, our RWA models not only estimate the direct effects of students’ motivation and students’ self-organization skills on the outcomes but also the indirect effects of each predictor via all other predictors, i.e., of a) students’ motivation via students’ self- organization skills on the outcomes; and b) students’ self-organization skills via students’ motivation on the outcomes. Thus, the reciprocal effects of predictors are therefore taken into account in our relative weight analyses.
Second, it is critical that the domain specificity of learning is not considered in the present study. Especially against the background of initial findings showing that the extent of digitally supported instruction during COVID-19 pandemic clearly depends on the subject (Heller and Zügel, 2020), the question arises as to what extent the findings in this paper can claim validity for all or at least for the main subjects equally. Steinmayr et al. (2021) showed that domain-specific teacher variables did not add to the prediction of students’ motivation, competent and independent learning, and learning progress during the school lockdown. How- ever, the latter were not assessed domain-specifically. Hence, we argue that future research should conduct appropriate analyses with domain-specific measurement instruments to shed light on this question.
Third, regarding the type of school, while only secondary school students were used here, it is well known from other studies (e.g., Helm et al., 2021) that distance education during COVID-19 pandemic-related school closures was quite different at the primary level than at the secondary level. For example, a representative parent survey in Austria at the beginning of 2021 (Helm and Postlbauer, 2021) reported that primary students received only 45 min of online lessons per day, whereas students from upper secondary schools received.
4.2 h of online lessons per day. By contrast, in primary schools 78% of the parents’ report that learning assignments are provided in paper form, while in upper secondary schools only 4% report receiving paper pencil assignments, but 83% report receiving assignments via digital learning platforms. Further studies need to investigate these different learning environments and should elaborate on differences in the determinants of learning across school levels (see, e.g., Steinmayr et al. (2021) for such analyses).
In addition, this study has some methodological limitations.
First, our study represents a cross-sectional study; hence, no causal statements can be made. Nevertheless, cross-sectional studies can provide meaningful insights into the possible longitudinal relations of variables if statistical analyzes are rooted in solid theoretical assumptions about predictors and outcomes and if central control variables are modeled. In the present study, we claim both. However, since our theoretical model assumes reciprocal relationships between the characteristics (see earlier discussion in this section), future studies should be longitudinal (see, e.g., Schober et al., 2020).
Second, our sample represents an ad hoc sample that is not representative of the student, parent, and teacher body population but controlled against different characteristics of the respective population. Particularly, the small student sample probably only represents a certain part of the lower secondary students. Therefore, strictly speaking, the findings cannot be generalized beyond our sample. However, in COVID-19 pandemic-related educational research, representative samples are rare (about only one-fifth of the 97 studies identified in the review in Helm et al. (2021) was based on representative samples). As in other current surveys, it can be assumed for our sample that people from educationally disadvantaged backgrounds, in particular, are underrepresented in the data. This could lead to an underestimation of the dispersion in some survey variables and thus their correlation with other variables. It is therefore possible that the effects reported here are too conservative.
Third, measurement invariance analysis indicates that the weak measurement invariance (i.e., equal loadings) of the construct lack of technical equipment at home was not achieved. Hence, a prerequisite for the comparison of the findings between the three informant groups was violated. According to Meitinger et al. (2020) there are various reasons for the lack of measurement invariance. On the one hand, the content of the construct may differ across groups, or the respondents attribute different meanings with the items. On the other hand, measurement invariance might be a result of other sources of measurement error (e.g., method bias). In the present study a lack of metric invariance means that the factor loadings are not equal across groups, i.e., some items are stronger indicators of the latent factor in certain groups of respondents. For the construct “technical equipment at home” the stepwise release of the equality constraints of the factor loadings shows that almost all items and all groups contribute to the missing measurement invariance. This means that the three groups of respondents attribute significantly different meanings to the items, for instance:
• Item 2 “The computer/laptop/tablet in our household is/are up to date” had a comparable low loading in the student group.
• Item 3 “Due to poor internet connection, I often cannot access things I need for learning or participate in video conferencing” had a comparable low factor loading in the student group too but had a very high factor loading in the teacher group.
• Item 4 “I have enough opportunities to work on the computer/laptop/tablet for school.” had a comparable very high factor loading in the student group and an low factor loading in the parent group.
These differences show that while items 2 and 3 are good indicators of the construct in the parent and teacher group, they are not so in the student group. In contrast, item 4 seems to work well in the student group, but very bad in the parent group. One interpretation of these findings is that, when asked about the appropriateness of their technical equipment at home for distance learning, from a students’ perspective the number of opportunities to work on an electronical device is of higher importance then the quality of the internet connection and the whether the computer/laptop/tablet is up to date. For parents and teachers, it seems to be the other way around. It makes sense for teachers to think about internet connectivity first, as their focus is online teaching in distance learning. It also makes sense that the condition of the laptop is important for parents, since parents are probably especially needed by their children when there are technical problems with the user devices. These findings indicate that future studies aiming to examine students’ technical equipment for distance learning at home should first construct a measurement scale consisting of items that are interpreted in the same way by different respondent groups or should contain carefully designed bespoke instruments for different groups.
Fourth, because we did not record information about which school the students, teachers, and parents belonged to (for reasons of anonymity), we also cannot account for the hierarchical structure in the data. This could lead to biased standard errors. However, intra-class correlation (ICC) values can be assumed to be low in the case of distance learning since classes have been disbanded and learning is more dependent on individual characteristics and home conditions. Findings from studies on distance learning point to rather low values below 10% (e.g., Jaekel et al., 2021). In addition, prior research from regular schooling shows that for motivational outcomes ICC values are low anyway (e.g., Kunter et al., 2005). Nevertheless, this lack of information is a key limitation that needs to be tackled in future research.
Fifth, for reasons of anonymity and ease of data collection, we refrained from collecting matched data. That is, we did not design the data collection process in a way that would have allowed links to be made between students, parents, and teachers. Therefore, a direct comparison of ratings of the same referent object from multiple perspectives is not possible in this study. This means, that the students who were assessed from the parents’ and teachers’ perspective (in the parent and teacher sample) do not necessarily coincide with the students of the student sample. Future studies should use matched samples to test the validity of the present findings.
Finally, it should be noted that student outcomes were assessed through self- and peer-ratings. This is especially critical for outcome learning success during lockdown. A meta-analysis by Hansford and Hattie (1982) concluded that self-ratings and performance measures are scarcely associated with each other or overlap only 4%–7%. It is therefore unclear to what extent the predictors identified here are also predictive of objectively assessed student performance measures.
Studies have repeatedly shown that children’s and adolescents’ abilities to self-organize and self-direct learning are predictive of the quality and outcomes of learning processes—not only in distance education (Boekaerts and Corno, 2005; Huber and Helm, 2020b; Steinmayr et al., 2021). Hence, in regular classes and in all different scenarios in which learning takes place without the supportive structures of school (e.g., lifelong learning, distance education due to natural disasters), it is particularly important to promote these skills intensively. For school practice, this could mean focusing more than before on types of learning environments that support self-directed learning and meta-learning, that is, learning how to regulate learning processes alone and with teachers. Findings from a student survey in Austria (Lenz and Helm, 2021) show that learners who were taught according to the concept of open and cooperative learning prior to COVID-19 pandemic are significantly more likely than students from traditional classes to report that they made higher progress during distance education. They also rated the quality of the teacher–student relationship during lockdown significantly higher than students from traditional classes. Huber (2021) discusses further implications for practice and policy in education, for example, the role of technology in promoting individualization and interactivity.
For researchers, the findings imply that both descriptive and explanatory analyses of distance education may arrive at partially different conclusion depending on which group of actors is used as the source of information. Only a few predictors, such as learners’ self-direction skills, were found to be highly significant in predicting learning outcomes across all informant groups. Future studies should therefore critically reflect on the validity of single informant studies against this background by discussing possible perspective validity and bias, as well as issues of reliability. As stated earlier, longitudinal studies with representative samples (taking demographic, socioeconomic and further relevant characteristics into account) are needed.
The raw data supporting the conclusion of this article will be made available by the authors, without undue reservation.
The studies involving human participants were reviewed and approved by the Teacher University Zug. Written informed consent from the participants’ legal guardian/next of kin was not required to participate in this study in accordance with the national legislation and the institutional requirements.
SH, CH, and Paula Günther, Nadine Schneider, Marius Schwander, Julia Schneider, and Jane Pruitt designed and carried out the study. CH performed the data analyses and was major contributor in writing the manuscript. All authors approved the submitted version.
This article was financially supported by the Open Access Publication Fund of the University of Teacher Education Zug.
The authors declare that the research was conducted in the absence of any commercial or financial relationships that could be construed as a potential conflict of interest.
All claims expressed in this article are solely those of the authors and do not necessarily represent those of their affiliated organizations, or those of the publisher, the editors and the reviewers. Any product that may be evaluated in this article, or claim that may be made by its manufacturer, is not guaranteed or endorsed by the publisher.
The Supplementary Material for this article can be found online at: https://www.frontiersin.org/articles/10.3389/feduc.2022.743770/full#supplementary-material
1Assumptions about multilevel logic and domain specificity are also incorporated into the model.
2Interestingly, Grewenig et al. (2020) found that mothers assessed the learning time of their children significantly lower than fathers.
3In a few studies (e.g., Huber and Helm, 2020b; Steinmayr et al., 2021; Züchner and Jäkel, 2021) unexpected effects were observed in multivariate procedures that are not observed in bivariate analyses.
Aldrup, K., Klusmann, U., Lüdtke, O., Göllner, R., and Trautwein, U. (2018). Social Support and Classroom Management Are Related to Secondary Students' General School Adjustment: A Multilevel Structural Equation Model Using Student and Teacher Ratings. J. Educ. Psychol. 110 (8), 1066–1083. doi:10.1037/edu0000256
Bandura, A. (2001). Social Cognitive Theory: An Agentic Perspective. Annu. Rev. Psychol. 52, 1–26. doi:10.1146/annurev.psych.52.1.1
Baumert, J., Kunter, M., Blum, W., Brunner, M., Voss, T., Jordan, A., et al. (2010). Teachers' Mathematical Knowledge, Cognitive Activation in the Classroom, and Student Progress. Am. Educ. Res. J. 47 (1), 133–180. doi:10.3102/0002831209345157
Baumert, J., Kunter, M., Brunner, M., Krauss, S., Blum, W., and Neubrand, M. (2004). “Mathematikunterricht aus Sicht der PISA-Schülerinnen und -Schüler und ihrer Lehrkräfte. [Mathematics education from the perspective of PISA students and their teachers,” in PISA 2003. Der Bildungsstand der Jugendlichen in Deutschland: [PISA 2003. The educational attainment of young people in Germany.]. Editors J. Baumert, W. Blum, R. Lehmann, D. Leutner, M. Neubrand, R. Pekrunet al. (Waxmann), 314–354.
Bezirkselternausschuss, M. (2020). Eltern-Umfrage zum Homeschooling: Ergebnisse und Schlussfolgerun- gen. [Parent survey on homeschooling. Results and conclusions]. Available at: https://bea-mitte.de/doc/umfrage/BEA_Mitte_Ergebnisse_Homeschooling-Umfrage.pdf.
Bildungsdirektion, N. (2020). Fernunterricht: fokusevaluation. [distance learning. Focus evaluation.]. Stans.
Bingham, R. D., Haubrich, P. A., and White, S. B. (1993). Explaining Teacher/Principal Differences in Evaluating Schools. J. Educ. Admin. 31 (1), 70–76. doi:10.1108/09578239310024728
Blume, F., Schmidt, A., Kramer, A. C., Schmiedek, F., and Neubauer, A. B. (2021). Homeschooling during the SARS-CoV-2 Pandemic: the Role of Students' Trait Self-Regulation and Task Attributes of Daily Learning Tasks for Students' Daily Self-Regulation. Z. Erziehungswiss. 24, 367–391. doi:10.1007/s11618-021-01011-w
Boekaerts, M., and Corno, L. (2005). Self-regulation in the Classroom: A Perspective on Assessment and Intervention. Appl. Psychol. 54 (2), 199–231. doi:10.1111/j.1464-0597.2005.00205.x
Boonk, L., Gijselaers, H. J. M., Ritzen, H., and Brand-Gruwel, S. (2018). A Review of the Relationship between Parental Involvement Indicators and Academic Achievement. Educ. Res. Rev. 24, 10–30. doi:10.1016/j.edurev.2018.02.001
Broadbent, J., and Poon, W. L. (2015). Self-regulated Learning Strategies & Academic Achievement in Online Higher Education Learning Environments: A Systematic Review. Internet Higher Education. 27, 1–13. doi:10.1016/j.iheduc.2015.04.007
Brok, P. d., Bergen, T., and Brekelmans, M. (2006). “Convergence and Divergence between Students' and Teachers' Perceptions of Instructional Behaviour in Dutch Secondary Education,” in Contemporary Approaches to Research on Learning Environments. Editors D. L. Fisher, and M. S. Khine (World Scientific), 125–160. doi:10.1142/9789812774651_0006
Bronfenbrenner, U. (1996). The Ecology of Human Development: Experiments by Nature and Design. 9th ed. Harvard Univ. Press.
Byrne, B. M., Shavelson, R. J., and Muthén, B. (1989). Testing for the Equivalence of Factor Covariance and Mean Structures: The Issue of Partial Measurement Invariance. Psychol. Bull. 105 (3), 456–466. doi:10.1037/0033-2909.105.3.456
Champeaux, H., Mangiavacchi, L., Marchetta, F., and Piccoli, L. (2020). Learning at home: Distance Learning Solutions and Child Development during the COVID-19 Lockdown: IZA Discussion Papers (No. 13819). Institute of Labor Economics IZA. Available at: https://ideas.repec.org/p/iza/izadps/dp13819.html.
Chen, F. F. (2007). Sensitivity of Goodness of Fit Indexes to Lack of Measurement Invariance. Struct. Equation Model. A Multidisciplinary J. 14 (3), 464–504. doi:10.1080/10705510701301834
Cheung, G. W., and Rensvold, R. B. (2002). Evaluating Goodness-Of-Fit Indexes for Testing Measurement Invariance. Struct. Equation Model. A Multidisciplinary J. 9 (2), 233–255. doi:10.1207/s15328007sem0902_5
Cipriano, C., Barnes, T. N., Pieloch, K. A., Rivers, S. E., and Brackett, M. (2019). A Multilevel Approach to Understanding Student and Teacher Perceptions of Classroom Support during Early Adolescence. Learn. Environ Res. 22 (2), 209–228. doi:10.1007/s10984-018-9274-0
De Los Reyes, A., Thomas, S. A., Goodman, K. L., and Kundey, S. M. (2013). Principles Underlying the Use of Multiple Informants' Reports. Annu. Rev. Clin. Psychol. 9, 123–149. doi:10.1146/annurev-clinpsy-050212-185617
Deci, E. L., and Ryan, R. M. (1985). Intrinsic Motivation and Self-Determination in Human Behavior. New York: Plenum Press.
Desimone, L. M., Smith, T. M., and Frisvold, D. E. (2010). Survey Measures of Classroom Instruction. Educ. Pol. 24 (2), 267–329. doi:10.1177/0895904808330173
Dietrich, H., Patzina, A., and Lerche, A. (2020). Social Inequality in the Homeschooling Efforts of German High School Students during a School Closing Period. Eur. Societies. 23, 1–22. doi:10.1080/14616696.2020.1826556
Dignath, C., and Veenman, M. V. J. (2021). The Role of Direct Strategy Instruction and Indirect Activation of Self-Regulated Learning-Evidence from Classroom Observation Studies. Educ. Psychol. Rev. 33 (2), 489–533. doi:10.1007/s10648-020-09534-0
Dorfner, T., Förtsch, C., and Neuhaus, B. J. (2018). Effects of Three Basic Dimensions of Instructional Quality on Students' Situational Interest in Sixth-Grade Biology Instruction. Learn. Instruction. 56, 42–53. doi:10.1016/j.learninstruc.2018.03.001
Eickelmann, B., and Drossel, K. (2020). SCHULE AUF DISTANZ: Perspektiven und Empfehlungen für den neuen Schulalltag. Eine repräsentative Befragung von Lehrkräften in Deutschland. [SCHOOL AT A DISTANCE. Perspectives and Recommendations for the New Everyday School Life. A Represen- tative Survey of Teachers in Germany.]. Düsseldorf: Vodafone Stiftung Deutschland. Available at: https://www.vodafone-stiftung.de/wp-content/uploads/2020/05/Vodafone-Stiftung- Deutschland_Studie_Schule_auf_Distanz.pdf.
Fauth, B., Decristan, J., Rieser, S., Klieme, E., and Büttner, G. (2014a). Grundschulunterricht aus Schüler-, Lehrer- und Beobachterperspektive: Zusammenhänge und Vorhersage von Lernerfolg*. Z. für Pädagogische Psychol. 28 (3), 127–137. doi:10.1024/1010-0652/a000129
Fauth, B., Decristan, J., Rieser, S., Klieme, E., and Büttner, G. (2014b). Student Ratings of Teaching Quality in Primary School: Dimensions and Prediction of Student Outcomes. Learn. Instruction. 29, 1–9. doi:10.1016/j.learninstruc.2013.07.001
Fischer, N., Holtappels, H. G., Klieme, E., Rauschenbach, T., Stecher, L., and Züchner, I. (2011). anztagsschule: Entwicklung, Qualität, Wirkungen: Längsschnittliche Befunde der Studie zur Entwick- lung von Ganztagsschulen (StEG). [All-day school: development, quality, effects. Longitudinal Findings of the Study on the Development of All-Day Schools (StEG).] (Weinheim: Beltz Juventa). Available at: http://www.socialnet.de/rezensionen/isbn.php?isbn=978-3-7799-2156-1.
forsa (2020). Das Deutsche Schulbarometer Spezial Corona-Krise. [The German School Barometer Spe- cial Corona Crisis.]. Berlin. Available at: https://deutsches-schulportal.de/unterricht/das-deutsche-schulbarometer-spezial- corona-krise/.
Förtsch, C., Werner, S., von Kotzebue, L., and Neuhaus, B. J. (2016). Effects of Biology Teachers' Professional Knowledge and Cognitive Activation on Students' Achievement. Int. J. Sci. Education. 38 (17), 2642–2666. doi:10.1080/09500693.2016.1257170
Fraser, B. J., and Fisher, D. L. (1982). Predicting Students' Outcomes from Their Perceptions of Classroom Psychosocial Environment. Am. Educ. Res. J. 19 (4), 498–518. doi:10.3102/00028312019004498
Frohn, J. (2020). Bildungsbenachteiligung im Ausnahmezustand. [Educational disadvantage in a state of emergency.]. Z. für Schul- Professionsentwicklung. 6 (2), 59–83. doi:10.4119/pflb-3908
Frohn, J. (2022). Klassenführung, kognitive Aktivierung und konstruktive Unterstützung im Fernunter- richt: Ergebnisse einer Lehrkräftebefragung zur Unterrichtsgestaltung auf Distanz. Editors S. G. Huber, C. Helm, and N. Schneider [Classroom manage- ment, cognitive activation, and constructive support in distance learning. Results of a teacher survey on teaching at a distance.] CovER. Münster: Waxmann.
Garrote, A., Neuenschwander, M., Hofmann, J., Mayland, C., Niederbacher, E., Prieth, V., et al. (2021). Fernunterricht während der Coronavirus-Pandemie: Analyse von Herausforderungen und Gelingensbedingungen. [Distance learning during the coronavirus pandemic. Analysis of challenges and conditions for success. Fachhochschule Nordwestschweiz FHNW. doi:10.26041/fhnw-3707
Grätz, M., and Lipps, O. (2021). Large Loss in Studying Time during the Closure of Schools in Switzerland in 2020. Res. Soc. stratification mobility. 71, 100554. doi:10.1016/j.rssm.2020.100554
Grewenig, E., Lergetporer, P., Werner, K., Wößmann, L., and Zierow, L. (2020). COVID-19 and Educa- Tional Inequality: How School Closures Affect Low- and High-Achieving Students. Munich, IZA: Institute for Labor Economics.
Hansford, B. C., and Hattie, J. A. (1982). The Relationship Between Self and Achievement/Performance Measures. Rev. Educ. Res. 52 (1), 123–142. doi:10.3102/00346543052001123
Hebbecker, K., Förster, N., and Souvignier, E. (2019). Reciprocal Effects between reading Achievement and Intrinsic and Extrinsic reading Motivation. Scientific Stud. Reading. 23 (5), 419–436. doi:10.1080/10888438.2019.1598413
Heller, S., and Zügel, O. (2020). Schule zu Hause in Deutschland: Bestandsaufnahme im Corona-Lockdown aus Perspektive der Schüler/-innen und Eltern. [School at home in Germany. Taking stock in the corona lockdown from the perspective of students and parents.]. Zurich. Available at: https://www.telekom-stiftung.de/sites/default/files/files/media/publications/Ergebnisbericht-Homeschooling.pdf.
Helm, C., Huber, S., and Loisinger, T. (2021). Was wissen wir über schulische Lehr-Lern-Prozesse im Distanzunterricht während der Corona-Pandemie? - Evidenz aus Deutschland, Österreich und der Schweiz. Z. Erziehungswiss. 24, 237–311. doi:10.1007/s11618-021-01000-z
Helm, C., and Postlbauer, A. (2021). JKU bildungsbarometer #1. Schulen im 3. Lockdown: 1. Bericht zur repräsentativen elternumfrage in Österreich. Johannes Kepler Universität. Linz: Johannes Kepler University Linz.
Helmke, A. (2009). Unterrichtsqualität und Lehrerprofessionalität: Diagnose, Evaluation und Verbesserung des Unterrichts. [Teaching quality and teacher professionalism. Diagnosis, evaluation and improvement of teaching. Stuttgart: Klett Kallmeyer
Holzer, J., Korlat, S., Haider, C., Mayerhofer, M., Pelikan, E., Schober, B., et al. (2021). Adolescent Well-Being and Learning in Times of COVID-19-A Multi-Country Study of Basic Psychological Need Satisfaction, Learning Behavior, and the Mediating Roles of Positive Emotion and Intrinsic Motivation. PloS one. 16 (5), e0251352. doi:10.1371/journal.pone.0251352
Huber, S. G., and Helm, C. (2020a). COVID-19 and Schooling: Evaluation, Assessment and Accountability in Times of Crises-Reacting Quickly to Explore Key Issues for Policy, Practice and Research with the School Barometer. Educ. Assess. Eval. Account. 32, 1–34. doi:10.1007/s11092-020-09322-y
Huber, S. G., and Helm, C. (2020b). “Lernen in Zeiten der Corona-Pandemie,” in Langsam vermisse ich die Schule. Schule während und nach der Corona-Pandemie. [I’m Starting to Miss School. ” Schooling during and after the Corona Pandemic.]. Editors D. Fickermann, and B. Edelstein (Waxmann), 37–60. doi:10.31244/9783830992318.02
Huber, S. G., Günther, P. S., Schneider, N., Helm, C., Schwander, M., Schneider, J., et al. (2020). COVID-19 und aktuelle herausforderungen in schule und bildung. Münster: Waxmann.
Huber, S. G. (2021). Schooling and Education in Times of the COVID-19 Pandemic: Food for Thought and Reflection Derived from Results of the School Barometer in germany, austria and switzerland. Int. Stud. Educ. Adm. 49 (1), 6–17.
Jaekel, A.-K., Scheiter, K., and Göllner, R. (2021). Distance Teaching During the COVID-19 Crisis: Social Connectedness Matters Most for Teaching Quality and Students' Learning. AERA Open. 7, 233285842110520. doi:10.1177/23328584211052050
Johnson, J. W. (2000). A Heuristic Method for Estimating the Relative Weight of Predictor Variables in Multiple Regression. Multivariate Behav. Res. 35 (1), 1–19. doi:10.1207/S15327906MBR3501_1
Klieme, E. (2020). Guter Unterricht – auch und besonders unter Einschränkungen der Pandemie? [Teaching Quality – Also and especially under the Constraints of the Pandemic?]. In D. Fickermann, and B. Edelstein (Eds.),. [I’m Starting to Miss School . . .” Schooling during and after the Corona Pandemic.] (pp. 117–135). Waxmann
Klieme, E., Pauli, C., and Reusser, K. (2009). “The Pythagoras Study: Investigating Effects of Teaching and Learning in Swiss and German Mathematics Classrooms,” in The Power of Video Studies in Investigating Teaching and Learning in the Classroom. Editors T. Janik, and T. Seidel (Waxmann), 137–160. Available at: http://www.ciando.com/ebook/bid-40151.
Kohler, B. (2011). Hausaufgaben. Überblick über didaktische Überlegungen und empirische Untersuchungen. [Homework. Survey about Didactical Considerations and Empirical Research.]. DDS - Die Deutsche Schule. 103 (3), 203–218.
Köller, O., Fleckenstein, J., Guill, K., and Meyer, J. (2020). “Pädagogische und didaktische Anforderungen an die häusliche Aufgabenbearbeitung,” in Langsam vermisse ich die Schule. Schule während und nach der Corona-Pandemie. [I’m Starting to Miss School. ” Schooling during and after the Corona Pandemic.]. Editors D. Fickermann, and B. Edelstein (Waxmann), 163–174. doi:10.31244/9783830992318.10
Korlat, S., Kollmayer, M., Holzer, J., Lã¼ftenegger, M., Pelikan, E. R., Schober, B., et al. (2021). Gender Differences in Digital Learning During COVID-19: Competence Beliefs, Intrinsic Value, Learning Engagement, and Perceived Teacher Support. Front. Psychol. 12, 637776. doi:10.3389/fpsyg.2021.637776
Krammer, G., Pflanzl, B., and Mayr, J. (2019). Using Students' Feedback for Teacher Education: Measurement Invariance across Pre-service Teacher-Rated and Student-Rated Aspects of Quality of Teaching. Assess. Eval. Higher Education. 44 (4), 596–609. doi:10.1080/02602938.2018.1525338
Kunter, M., Baumert, J., and Köller, O. (2007). Effective Classroom Management and the Development of Subject-Related Interest. Learn. Instruction. 17 (5), 494–509. doi:10.1016/j.learninstruc.2007.09.002
Kunter, M., and Baumert, J. (2006). Who Is the Expert? Construct and Criteria Validity of Student and Teacher Ratings of Instruction. Learn. Environments Res. 9 (3), 231–251. doi:10.1007/s10984-006-9015-7
Kunter, M., Brunner, M., Baumert, J., Klusmann, U., Krauss, S., Blum, W., et al. (2005). Der Mathematikunterricht der PISA-Schülerinnen und -Schüler. Z. für Erziehungwissenschaft. 8 (4), 502–520. doi:10.1007/s11618-005-0156-8
Kunter, M. (2013). Cognitive Activation in the Mathematics Classroom and Professional Competence of Teachers: Results from the COACTIV Project, Vols. v. 8. New York: Springer.
Langmeyer, A., Guglhör-Rudan, A., Naab, T., Urlen, M., and Winklhofer, U. (2020). Kindsein in Zeiten von Corona: Erste Ergebnisse zum veränderten Alltag und zum Wohlbefinden von Kindern. [Being a child in times of Corona. First results on the changed everyday life and well-being of children]. Munich: Deutsches Jugendinstitut.
Lenz, S., and Helm, C. (2021). Do students from Open Learning Environments Perceive Advantages in Distance Learning during corona-related School Closures? Johannes Kepler Universität. Available at: https://edulead.net/schuba/factsheets/do-students-from-open-learning-environments-perceive-advantages-in-distance-learning-during-corona-related-school-closures/.
Letzel, V., and Pozas, M. (2020). “Inklusives Homeschooling aus Schüler/-innen-Eltern-und Lehrkräfteperspek- tive – Covid-19,” in [Inclusive Homeschooling from a Student-Parent and Teacher Perspective - Covid-19.]. Editor U. Trier.
Letzel, V., Pozas, M., and Schneider, C. (2020). Energetic Students, Stressed Parents, and Nervous Teachers: A Comprehensive Exploration of Inclusive Homeschooling during the COVID-19 Crisis. Open Education Stud. 2, 159–170. doi:10.1515/edu-2020-0122
Little, T. D. (2013). Longitudinal Structural Equation Modeling. New York, NY: Guilford Press. Available at: http://site.ebrary.com/lib/subhamburg/Doc?id=10664545.
Lorenz, R., Brüggemann, T., and McElvany, N. (2020). Unterricht während der Corona-Pandemie: Teil II Wohlbefinden der Lehrkräfte: Zweiter Ergebnisbericht der bundesweiten Lehrkräftebefragung. [Teaching during the Corona pandemic: Part II Teacher well-beingSecond report of results from the nationwide teacher survey.]. Dortmund: Institut für Schulentwicklungsforschung.
Meitinger, K., Davidov, E., Schmidt, P., and Braun, M. (2020). Measurement Invariance: Testing for It and Explaining Why It is Absent. Survey Research Methods, 14 (4), 345–349. doi:10.18148/srm/2020.v14i4.7655
Meyer, H. (2020). Didaktische Maßstäbe für Homeschooling in Corona-Zeiten. [Didactic benchmarks for homeschooling in Corona times.]. Cornelsen. Available at: https://www.cornelsen.de/magazin/beitraege/didaktische- massstaebe-homeschooling.
Mitchell, M. M., Bradshaw, C. P., and Leaf, P. J. (2010). Student and Teacher Perceptions of School Climate: a Multilevel Exploration of Patterns of Discrepancy. J. Sch. Health. 80 (6), 271–279. doi:10.1111/j.1746-1561.2010.00501.x
Moroni, S., Dumont, H., Trautwein, U., Niggli, A., and Baeriswyl, F. (2015). The Need to Distinguish between Quantity and Quality in Research on Parental Involvement: The Example of Parental Help with Homework. J. Educ. Res. 108 (5), 417–431. doi:10.1080/00220671.2014.901283
Nusser, L., Wolter, I., Attig, M., and Fackler, S. (2021). “Die Schulschließungen aus Sicht der Eltern. Ergebnisse des längsschnittlichen Nationalen Bildungspanels und seiner Covid-19-Zusatzbefragung,” in Schule während der Corona-Pandemie: Neue Ergebnisse und Überblick über ein dynamisches Forschungsfeld. [Schooling dur- ing the Corona Pandemic. New Results and an Overview of a Dynamic Field of Research.]. Editors D. Fickermann, and B. Edelstein (Waxmann Verlag GmbH), 33–50. doi:10.31244/9783830993315.02
Pelikan, E. R., Lüftenegger, M., Holzer, J., Korlat, S., Spiel, C., and Schober, B. (2021). Learning during COVID-19: the Role of Self-Regulated Learning, Motivation, and Procrastination for Perceived Competence. Z. Erziehungswiss. 24, 393–418. doi:10.1007/s11618-021-01002-x
Podsakoff, P. M., MacKenzie, S. B., Lee, J. Y., and Podsakoff, N. P. (2003). Common Method Biases in Behavioral Research: a Critical Review of the Literature and Recommended Remedies. J. Appl. Psychol. 88 (5), 879–903. doi:10.1037/0021-9010.88.5.879
Pomerantz, E. M., and Grolnick, W. S. (2017). “The Role of Parenting in Children’s Motivation and Competence: What Underlies Facilitative Parenting” in Handbook of Competence and Motivation. Editors A. J. Elliot, C. S. Dweck, and D. S. Yeager (Guilford Press), 566–585.
Porsch, R., and Porsch, T. (2020). “Fernunterricht Als Ausnahmesituation,” in Langsam vermisse ich die Schule. Schule während und nach der Corona-Pandemie. [I’m Starting to Miss School. ” Schooling during and after the Corona Pandemic.]. Editors D. Fickermann, and B. Edel- stein (Waxmann), 61–78. doi:10.31244/9783830992318.03
Praetorius, A.-K., and Charalambous, C. Y. (2018). Classroom Observation Frameworks for Studying Instructional Quality: Looking Back and Looking Forward. ZDM Mathematics Education. 50 (3), 535–553. doi:10.1007/s11858-018-0946-0
R Core Team (2014). R: A Language and Environment for Statistical Computing. Vienna, Austria: R Foundation for Statistical Computing. Available at: http://www.R-project.org/.
Ribeiro, L. M., Cunha, R. S., Silva, M. C. A. e., Carvalho, M., and Vital, M. L. (2021). Parental Involvement during Pandemic Times: Challenges and Opportunities. Education Sci. 11 (6), 302. doi:10.3390/educsci11060302
Rosseel, Y. (2012). Lavaan: An R Package for Structural Equation Modeling. J. Stat. Softw. 48 (2), 1–36. doi:10.18637/jss.v048.i02
Sander, A., Schäfer, L., and van Ophuysen, S. (2021). Predictors of Process-Related and Structuring Parental Support during Distance Learning Due to Corona-pandemic. Z. Erziehwiss. 24, 1–24. doi:10.1007/s11618-021-01015-6
Schaffner, E., Philipp, M., and Schiefele, U. (2016). Reciprocal Effects between Intrinsic reading Motivation and reading Competence? A Cross-Lagged Panel Model for Academic Track and Nonacademic Track Students. J. Res. Reading. 39 (1), 19–36. doi:10.1111/1467-9817.12027
Scheerens, J. (1990). School Effectiveness Research and the Development of Process Indicators of School Functioning. Sch. Effectiveness Sch. Improvement. 1 (1), 61–80. doi:10.1080/0924345900010106
Schiepe-Tiska, A., Heine, J.-H., Lüdtke, O., Seidel, T., and Prenzel, M. (2016). Mehrdimensionale Bil- dungsziele im Mathematikunterricht und ihr Zusammenhang mit den Basisdimensionen der Unterricht- squalität: [Multi-Dimensional Educational Goals in Mathematics Classrooms and Their Relationship with Instructional Quality.]. Unterrichtswissenschaft. 44 (3), 211–225.
Schober, B., Lüftenegger, M., Spiel, C., Holzer, J., Ikanovic, S. K., Pelikan, E., et al. (2020). Lernen unter COVID-19-Bedingungen: Erste Ergebnisse Schüler*innen. [Learning under COVID-19 conditions. First results Students]. Vienna. Available at: https://lernencovid19.univie.ac.at/fileadmin/user_upload/p_lernencovid19/Zwischenergebnisse_Schueler_innen.pdf.
Schwab, S., Lindner, K.-T., and Kast, J. (2020). Inclusive home Learning – Quality of Experience of Students, Teachers and Parents and Current Implementation of home Schooling. Vienna.
Schwerzmann, M., and Frenzel, S. (2020). Umfrage zum Fernunterricht: Ergebnisse der Befragung im Juni 2020. [Distance Education Survey. Results of the survey in June 2020.]. Bildungs- und Kulturdeparte- ment. Lucerne.
Shavelson, R. J., Hubner, J. J., and Stanton, G. C. (1976). Self-Concept: Validation of Construct Interpretations. Rev. Educ. Res. 46 (3), 407–441. doi:10.3102/00346543046003407
Shi, D., Lee, T., and Maydeu-Olivares, A. (2019). Understanding the Model Size Effect on SEM Fit Indices. Educ. Psychol. Meas. 79 (2), 310–334. doi:10.1177/0013164418783530
Skinner, E. A., and Belmont, M. J. (1993). Motivation in the Classroom: Reciprocal Effects of Teacher Behavior and Student Engagement across the School Year. J. Educ. Psychol. 85 (4), 571–581. doi:10.1037/0022-0663.85.4.571
Stadler, M., Cooper-Thomas, H., and Greiff, S. (2017). A Primer on Relative Importance Analysis: Illustrations of its Utility for Psychological Research. Psychol. Test Assess. Model. 59, 381.
Steiner, M., Köpping, M., Leitner, A., and Pessl, G. (2020). COVID-19 LehrerInnenbefragung - Zwischen- ergebnisse: Was tun, damit aus der Gesundheitskrise nicht auch eine Bildungskrise wird? [COVID-19 Teacher survey - Interim results. What to Do to prevent the health crisis from becoming an educa- tion crisis as well?]. Available at: https://www.ihs.ac.at/de/publikationsportal/blog/beitraege/lehrerinnenbefragung- zwischenergebnisse/.
Steinmayr, R., and Christiansen, H. (2020). Vorabpressemitteilung: Studie Qualität von Homeschooling der TU Dortmund. Advance press release study Quality of Homeschooling by TU Dortmund University. Dortmund.
Steinmayr, R., Lazarides, R., Weidinger, A. F., and Christiansen, H. (2021). Teaching and Learning during the First COVID-19 School Lockdown: Realization and Associations with Parent-Perceived Students' Academic Outcomes. Z. für Pädagogische Psychol. 35, 85–106. doi:10.1024/1010-0652/a000306
Talbert, R., and Mor-Avi, A. (2019). A Space for Learning: An Analysis of Research on Active Learning Spaces. Heliyon. 5 (12), e02967. doi:10.1016/j.heliyon.2019.e02967
Temme, D., and Hildebrandt, L. (2009). Gruppenvergleiche bei hypothetischen Konstrukten - Die Prüfung der Übereinstimmung von Messmodellen mit der Strukturgleichungsmethodik. Schmalenbachs Z. Betriebswirtsch Forsch. 61 (2), 138–185. doi:10.1007/BF03372818
Tengler, K., Schrammel, N., and Brandhofer, G. (2020). Lernen trotz Corona: Chancen und Herausforderun- gen des distance learning an österreichischen Schulen. [Learning despite Corona. Opportunities and challenges of distance learning in Austrian schools.]. Medienimpulse. 58 (2), 1–37. doi:10.21243/mi-02-20-24
Thies, L., and Klein, Y. (2020). Unter Druck: Die Situation von Eltern und ihren schulpflichtigen Kindern während der Schulschließungen. [Under pressure. The situation of parents and their school-age children during school closures.]. Düsseldorf. Available at: https://www.vodafone-stiftung.de/wp-content/uploads/2020/04/Vodafone-Stiftung- Deutschland_Studie_Unter_Druck.pdf.
Tonidandel, S., and LeBreton, J. M. (2011). Relative Importance Analysis: A Useful Supplement to Regression Analysis. J. Bus Psychol. 26 (1), 1–9. doi:10.1007/s10869-010-9204-3
Trautwein, U., Lüdtke, O., Marsh, H. W., Köller, O., and Baumert, J. (2006). Tracking, Grading, and Student Motivation: Using Group Composition and Status to Predict Self-Concept and Interest in Ninth-Grade Mathematics. J. Educ. Psychol. 98 (4), 788–806. doi:10.1037/0022-0663.98.4.788
Trültzsch-Wijnen, C., and Trültzsch-Wijnen, S. (2020). REMOTE SCHOOLING during the COVID- 19 LOCKDOWN IN AUSTRIA (spring 2020): KiDiCoTi National Report. Salzburg.
Voss, T., and Wittwer, J. (2020). Unterricht in Zeiten von Corona: Ein Blick auf die Herausforderungen aus der Sicht von Unterrichts- und Instruktionsforschung. Unterrichtswiss. 48 (4), 601–627. doi:10.1007/s42010-020-00088-2
Wagner, S. M., Rau, C., and Lindemann, E. (2010). Multiple Informant Methodology: A Critical Review and Recommendations. Sociological Methods Res. 38 (4), 582–618. doi:10.1177/0049124110366231
Weber, C., Helm, C., and Kemethofer, D. (2021). “Bildungsungleichheiten durch Schulschließungen? Soziale und ethnische Disparitäten im Lesen innerhalb und zwischen Schulklassen,” in Schule und Schulpolitik während der Corona-Pandemie: Nichts gelernt? [Schools and School Politics During the Corona Pandemic: Nothing Learned?]. Editors D. Fickermann, B. Edelstein, J. Gerick, and K. Racherbäumer (Waxmann Verlag GmbH), 83–99.
Wettstein, A., Scherzinger, M., and Ramseier, E. (2018). Empirische Arbeit: Unterrichtsstörungen, Beziehung und Klassenführung aus Lehrer-, Schüler- und Beobachterperspektive. Psychol. Erziehung Unterricht. 65 (1), 58. doi:10.2378/peu2018.art04d
Wigfield, A., and Eccles, J. S. (2000). Expectancy-value Theory of Achievement Motivation. Contemp. Educ. Psychol. 25, 68–81. doi:10.1006/ceps.1999.1015
Wildemann, A., and Hosenfeld, I. (2020). Bundesweite Elternbefragung zu Homeschooling während der Covid 19 Pandemie: Erkenntnisse zur Umsetzung des Homeschoolings in Deutschland. Editor U Landau (Nationwide parent sur- vey on homeschooling during the Covid 19 pandemic. Findings on the implementation of homeschooling in Germany.]). Available at: http://www.zepf.eu/wp-content/uploads/2020/06/Bericht_HOMEschooling2020.pdf.
Wößmann, L., Freundl, V., Grewenig, E., Lergetporer, P., Werner, K., and Zierow, L. (2021). Bildung erneut im Lockdown: Wie verbrachten Schulkinder die Schulschließungen Anfang 2021?. [Education on lockdown again. How did school kids spend the early 2021 school closures?]. Munich.
Xu, J., Du, J., Wu, S., Ripple, H., and Cosgriff, A. (2018). Reciprocal Effects Among Parental Homework Support, Effort, and Achievement? an Empirical Investigation. Front. Psychol. 9, 2334. doi:10.3389/fpsyg.2018.02334
Zaccoletti, S., Camacho, A., Correia, N., Aguiar, C., Mason, L., Alves, R. A., et al. (2020). Parents' Perceptions of Student Academic Motivation During the COVID-19 Lockdown: A Cross-Country Comparison. Front. Psychol. 11, 592670. doi:10.3389/fpsyg.2020.592670
Keywords: COVID-19, student learning (at school), predictors and associations, structural equating modeling, multiple informant data, international study
Citation: Helm C and Huber SG (2022) Predictors of Central Student Learning Outcomes in Times of COVID-19: Students’, Parents’, and Teachers’ Perspectives During School Closure in 2020—A Multiple Informant Relative Weight Analysis. Front. Educ. 7:743770. doi: 10.3389/feduc.2022.743770
Received: 19 July 2021; Accepted: 07 January 2022;
Published: 17 February 2022.
Edited by:
Karin Gehrer, Leibniz Institute for Educational Trajectories (LG), GermanyReviewed by:
Jeffrey M. DeVries, Technical University Dortmund, GermanyCopyright © 2022 Helm and Huber. This is an open-access article distributed under the terms of the Creative Commons Attribution License (CC BY). The use, distribution or reproduction in other forums is permitted, provided the original author(s) and the copyright owner(s) are credited and that the original publication in this journal is cited, in accordance with accepted academic practice. No use, distribution or reproduction is permitted which does not comply with these terms.
*Correspondence: Stephan Gerhard Huber, c3RlcGhhbi5odWJlckBwaHpnLmNo
†Paula Günther, Nadine Schneider, Marius Schwander, Julia Schneider, and Jane Pruitt assisted in the data collection
Disclaimer: All claims expressed in this article are solely those of the authors and do not necessarily represent those of their affiliated organizations, or those of the publisher, the editors and the reviewers. Any product that may be evaluated in this article or claim that may be made by its manufacturer is not guaranteed or endorsed by the publisher.
Research integrity at Frontiers
Learn more about the work of our research integrity team to safeguard the quality of each article we publish.