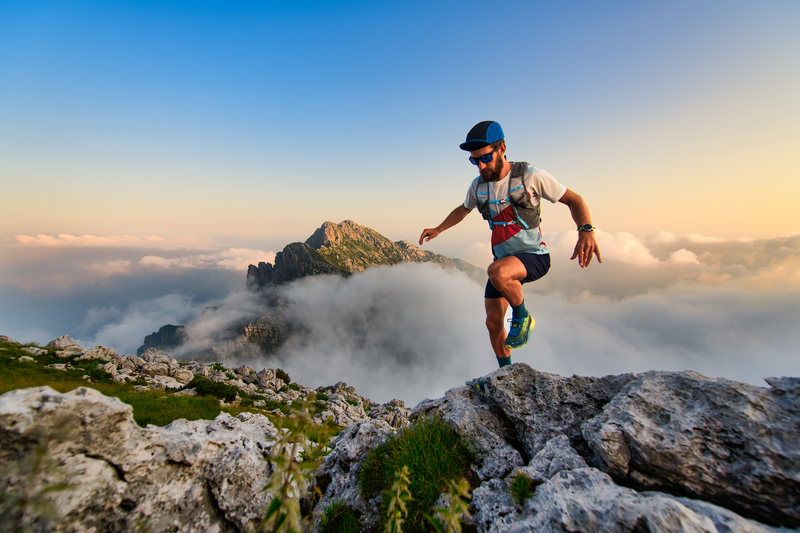
94% of researchers rate our articles as excellent or good
Learn more about the work of our research integrity team to safeguard the quality of each article we publish.
Find out more
ORIGINAL RESEARCH article
Front. Educ. , 10 January 2023
Sec. Digital Education
Volume 7 - 2022 | https://doi.org/10.3389/feduc.2022.1083651
This article is part of the Research Topic Educational Digital Transformation: New Technological Challenges for Competence Development View all 22 articles
This paper examines how factors associated with the digital divide such as ICT access, digital skills, and outcomes influenced synchronous online teaching in urban and rural schools in Kazakhstan during COVID-19 school closures. In addition to school location, this paper addresses how the speed and steadiness of the internet connection, and teacher characteristics such as age, qualification, and experience influenced teacher usage of synchronous teaching and learning mediums. Data in this paper consists of a nationally representative sample of nearly 4,000 teachers. This study found that the digital divide narrows when schools provide teachers with ICT access. While both, the speed of the internet and rural–urban residency have statistically significant effects on the use of ICT tools by teachers when considered separately, the interaction term between these two covariates was not statistically significant. Results indicated that age, experience, teacher workload and professional qualification were important determinants in teachers’ ability to engage in synchronous teaching.
Full and partial school closures due to COVID - 19 pandemic from March 2020 to January 2021 globally averaged 22 weeks, which is two-thirds of an academic year (UNESCO, 2021). This extraordinary global situation created a natural experiment allowing us to evaluate the resources available for remote learning and the digital divide that may exist for teachers and students. The digital divide embodies the inequality between those with technology access and digital skills and those without (Hartviksen et al., 2002; Van Dijk, 2006; Ragnedda and Kreitem, 2018). The current paper focuses on how the digital divide manifested itself during COVID – 19 induced remote learning and teaching in Kazakhstan, the largest country in Central Asia. Full and partial school closures in this country amounted to 32 weeks between March 2020 and January 2021 (UNESCO, 2021).
Research shows that the massive shift toward distance education could exacerbate existing educational inequalities (Hosszu and Rughinis, 2020; Quaicoe and Pata, 2020; Darmody et al., 2021; González-Betancor et al., 2021; León-Nabal et al., 2021). The challenges associated with technology and internet access shape how teachers and students engage in online learning. Lack of reliable data on who has access to online education and digital infrastructure as well as professional development for teachers pose an additional challenge for low- and middle-income countries (Crompton et al., 2021; Hennessy et al., 2022). These countries are novice to digital education and less aware of what technology can offer and achieve in learning and teaching.
In this context, the case of Kazakhstan is interesting for several reasons. Over the past decades, Kazakhstan has invested heavily in modernizing its education system (Ibrayeva, 2014) and digitalization (Digital Kazakhstan, 2018; Klymenko and Alpeissova, 2021). It has transitioned from lower-middle-income to upper-middle-income country status in less than two decades (World Bank, 2021). The geography of the education system consists of densely populated regions and regions with one of the lowest population densities in the world (Hambly, 2021). Although the government is racing to digitalize the country (Digital Kazakhstan, 2018), access to ICT remains uneven (UN Economic and Social Commission for Asia and the Pacific, 2020). Research has shown that issues associated with the digital divide, such as slow internet, and lack of proper equipment, were dominant challenges for parents in Kazakhstan during COVID-19 forced school closures (Bokayev et al., 2021a). A lack of effective interaction between educational stakeholders presented an additional challenge to address at least some of these issues promptly (Kovyazina et al., 2021; Bokayev et al., 2021b).
To better understand which factors characteristic of the digital divide conditioned the quality of online teaching in Kazakhstan, this paper seeks to examine how teachers during school closures in fall 2020 engaged in synchronous teaching using programs like Zoom, Microsoft Teams, Skype, Google Meet, and others. Online learning sets two basic formats, asynchronous and synchronous in what the difference refers to the time and place of educational activities. Asynchronous environments are characterized as being more individual-oriented, self-regulated, and less teacher-dependent, while synchronous online teaching means teachers use video-conferencing systems, including Zoom and Google Meets, to deliver lessons in real time (Fabriz et al., 2021; Wang and Wang, 2021; Stuart et al., 2022).
Among the major benefits of synchronous learning and teaching are the availability of verbal and non-verbal language, instant feedback and real-time interpersonal communication (Blau et al., 2017; Moorhouse and Wong, 2022). Given the interactivity factor of synchronous learning, we consider this mode essential for teachers to support students who are deprived of learning opportunities at school and might have difficulties with self-regulated learning while at home.
Literature finds that benefits of synchronous and asynchronous learning can be different for different age groups. Learning asynchronously might be more advantageous due to its flexibility, accessibility and learner-centredness and might be more suitable for university students because they are more likely to have better self-study skills and practice more self-regulation (Davies et al., 2020; Schreiber et al., 2022). For school-age children, which is the education level in the focus of this paper, synchronous learning is more beneficial because they are accustomed to learning with a teacher guiding and instructing them. Research also demonstrates that synchronous teaching has positive impacts on academic outcomes (Stuart et al., 2022), more feedback and greater support (Fabriz et al., 2021), and task motivation (Hrastinski et al., 2010).
This paper hence focuses on synchronous teaching and examines how it was associated with the digital divide. The paper examines this phenomenon through the focus of digital infrastructure such as the steadiness of the internet connection, and teacher characteristics such as age, qualification, and experience. The data analyzed in this paper comes from a survey of about 4,000 teachers, a nationally representative stratified random sample, conducted in the fall of 2020. Results of this research contribute to better understanding of factors critical to narrow the digital divide of access, skills and outcomes in the context of synchronous learning and teaching. As such, these results can inform better national and local-level decisions in supporting teachers and overcoming negative effect of digital divide in education.
The digital divide represents unequal access to the internet and digital affordances, occurring at individual, local, national, and international levels. It generally defines the gap “between those who do and those who do not have access to new forms of information technology” (Van Dijk, 2006, p. 222). In other words, the digital divide embodies the inequality between those with technology access and digital skills and those without. The focus in digital divide research has transitioned from inequalities of access to digital skills and usage, addressing the limitations of the past research that mainly referred to binary differences between haves and have-nots (van Deursen and van Dijk, 2014). For a long time, the digital divide was commonly seen as a technical issue rather than as an amalgam of deeper social problems (Light, 2001; Dijk et al., 2003). However, mere access to technology and infrastructure does not reflect the complex nature of the digital divide. Researchers (Hargittai, 2002; Van Dijk, 2006; Wei et al., 2011; Ragnedda and Kreitem, 2018) define three dimensions of digital inequality – digital access divide, digital capability divide, and digital outcome divide. This classification illustrates the multidimensional nature of the digital divide phenomenon that goes beyond mere technical metrics.
In the modern world, digital access, also known as material or physical access to ICT (Van Dijk, 2006), can be considered as one of the basic human rights because of the growing internet connectivity and ICT ownership. In addition to unequal access, digital skills and capabilities play an important role in determining to what extent people navigate the internet and technology confidently and safely. The biggest issue has become how well people are equipped with the skills necessary for the digital world. New terms have emerged to describe the skills focus, including “internet use,” “social media use,” “digital literacy and competencies,” to name the most ubiquitous (van Deursen and van Dijk, 2014).
Digital skills and access to ICT lead to the third aspect of the divide – the digital outcome. The digital outcome divide “underlines inequalities in the benefits gained from the different levels of access and usage of the Internet” (Ragnedda and Kreitem, 2018, p. 8). Individuals affected by this divide lack belief and self-efficacy, resulting in lower digital productivity (Mathrani et al., 2021). It leads to differences between people in learning outcomes and purposeful ICT usage (Wei et al., 2011; van Deursen and Helsper, 2015). Nevertheless, notes Scheerder et al. (2017), the digital divide research primarily focuses on access and skills divides, giving little attention to examining the benefits, outcomes, and effects of technology use (van de Werfhorst et al., 2020).
The three aspects of digital inequality - digital access, capability, and outcome divide - are relevant when assessing its effects on education. COVID-19 pandemic in 2020 when education systems in the world experienced a massive shift to remote and online education exacerbated the relevance of these three aspects of digital divide. To understand the effects of digital divide on teaching and learning, it is important to focus on not only the first crucial step, access to ICT, but also ICT literacy and outcomes measured as results of remote learning. This paper attempts to examine these three aspects of the digital divide in education by understanding what factors determine teacher use of synchronous programs like Zoom and others to support student learning and achievement.
The digital divide reflects broader contextual factors such as social, economic, cultural, and learning inequalities. As such, factors contributing to the digital divide are economic, social, ethnolinguistic, and infrastructural (Bagchi, 2005). Social groups in marginalized or minority positions are more likely to experience the negative effects of the digital divide. Inequalities in ICT access also manifest in urban and rural dimensions. Online education might increase the existing gaps between rural and urban populations whose access to online education significantly differs (Hosszu and Rughinis, 2020). It is true for Kazakhstan as well since the urban–rural divide remains unsolved across many areas of social life including digitalization (OECD, 2017). The overall share of internet and ICT access reported for cities in 2016 was 81.3 and 70.9% in the rural settlements of Kazakhstan. Despite these relatively high percentages of internet connectivity, access to high-speed internet remains uneven in favor of urban areas over rural locations (Digital Kazakhstan, 2018).
Other factors signaling the possible risk of experiencing negative effects of the digital divide are a person’s age and educational background (Ertl et al., 2020). Age is the most obvious factor that puts older people at a disadvantage in using digital technology. Starkey et al. (2017) refer to the generational gap between younger and older people that gives the space for a deficit model of ICT use in education. Indeed, teachers at younger ages show greater digital competencies over their more senior colleagues (Kale and Goh, 2014; Cantú-Ballesteros et al., 2017). Although experts note that the digital gap between young and older people does not seem to be closing in the near future (Enoch and Soker, 2006), professional development programs for teachers can offer a solution. Teachers who participate in professional development gain confidence in ICT usage (Pongsakdi et al., 2021), tend to use computers in class more often, focus more on teaching ICT skills and develop stronger ICT self-efficacy (Drossel and Eickelmann, 2017).
According to Teaching and Learning International Survey (TALIS, 2018), seven in 10 Kazakhstani teachers reported having “ICT for teaching” as part of their formal education or training, and almost all of them reported participation in professional development within the last year (OECD, 2019). At the same time, more teachers in Kazakhstan (30%) than in OECD countries (18%) reported a high need for professional development in ICT use (OECD, 2019). These contradictory results suggest the need for more effective pre-service and in-service teacher training in ICT usage for those who are digital novices in education in Kazakhstan to reduce digital inequality affecting education.
Education level is one more factor associated with the digital divide. People with higher levels of education are likely to be more competent at using computers and the internet. Research indicates that during the early years of digital expansion, people with college or higher degrees were 10 times more likely to have internet access at work than those with only a high school certificate (NTIA, 1998). While there is broad internet access today, individuals with university degrees appear to reap more ICT-related benefits than those who hold lower educational qualifications. Highly educated people seem to have better digital skills, which also empower them to be more productive while using ICTs (Correa, 2015; Azubuike et al., 2020). Teachers in Kazakhstan generally hold a bachelor’s degree and thus belong to those highly educated; teachers with a vocational or college education in pedagogy can still work in primary schools. At the same time, recent data shows that only about two-thirds of teachers have reported feeling prepared to use ICT in teaching after completing their formal studies in Kazakhstan (OECD, 2019).
Research before the COVID-19 pandemic showed that lack of teaching experience with ICT, absence of on-site support for teachers using technology, lack of help supervising children when using digital tools, insufficient technology training for students at school, limited availability of computers, the time required to integrate technology into the curriculum successfully, and inadequate financial incentives may all hinder teachers’ ability to effectively use ICT in their teaching (Mumtaz, 2000; Ekberg and Gao, 2018; Mynarˇíková and Novotný, 2021). With the pandemic-affected school closures, these obstacles did not vanish. The digital divide became an integral variable affecting the education process. The current article will proceed to examine how factors associated with the digital divide manifested in the use of synchronous online teaching mediums (e.g., Zoom) among teachers in Kazakhstan.
In this article, we use data from a large-scale online survey of mainstream schoolteachers conducted in Kazakhstan from October to November 2020 as part of the monitoring of distance learning in the country, commissioned by the Information Analytics Center (IAC) and approved by the Ministry of Education in Kazakhstan. IAC is a publicly-funded think tank tasked with conducting analytics and research to inform educational decision-making and policy.
The survey sampling design consisted of one-stage stratified sample with the proportional allocation of teachers to rural, urban and regional settings. Stratification was conducted according to Kazakhstan’s rural–urban area and official administrative division, resulting in 31 explicit strata. Four thousand teachers were allocated proportionally among explicit stratum with subsequent random sampling from the list of teacher population. The sampling frame used for selection was taken from the National Educational Database, which contains administrative data from all schools in the country. Before taking the survey, teachers invited to participate were provided with information on data use, anonymity, and confidentiality. Only those respondents who after viewing this information clicked “Yes” in the dialog window, answering a straightforward question about their willingness to participate in the survey, proceeded to answer other questions in the survey.
The survey questionnaire was specifically developed to examine how various socio-demographic characteristics of teachers are associated with the use of ICT, access to ICT, teaching in general and teaching to specific groups of students such as students with special educational needs, evaluation of the effectiveness of distance learning, and satisfaction with distance learning after switching to remote learning in Spring 2020 and continuing remote teaching in Fall 2020. To obtain additional variables on professional characteristics of teachers such as professional category, workload and experience, we combined survey data with data from the sampling frame taken in turn from National Education Data Base (NEDB).
To answer the research question guiding this paper - how synchronous online teaching was associated with the urban or rural school location, the speed and steadiness of the internet connection, and teacher characteristics such as age, their qualification and experience – we used a 5-point Likert scale item with ordered categories (never, rarely, sometimes, often, and always) representing a frequency of video programs such as Zoom, Microsoft Teams, Skype, Google Meet used for synchronous teaching during Spring 2020 as the dependent variable. The distribution of answers for each category was 10.6% (never – one or two lessons), 11.3% (rarely – in some lessons), 26% (sometimes - in some lessons), 28.4% (often – in several lessons) and 23.3% (always – every lesson).
Regarding the digital divide, our main explanatory variables were the reported speed of internet connection, availability of home PC/laptop, availability of school PC/laptop and rural–urban place of living. The question on the internet consisted of five ordered categories with percentages of answers for “very high speed: fast download of video and online lessons: no lagging” – 17.6%, “high speed: with some lagging” – 29.1%, “average speed: need to wait” – 43.5%, “low-speed: almost impossible to watch” – 8.1% and “very-low speed: cannot open the video” – 1.6%. The categories were recorded in increasing rather than decreasing order, in the analysis we used the speed of the internet with five categories as a continuous variable. Although in some cases the use of Likert scale data in the parametric analysis is questionable (Lantz, 2013), there is a strand of research supporting the use of the categorical ordinal scales with at least five categories and a large enough sample size as continuous, interval in the parametric analysis (Hsu and Feldt, 1969; Carifio and Perla, 2007; Norman, 2010; Harpe, 2015).
Availability of school PC/laptop and home PC/laptop are represented as dichotomous items with Yes/No categories with the distribution of answers 36%/64 and 95.5%/4.5%, respectively. We expect a positive relationship between the reported speed of the internet and the use of video programs, faster internet connection leading to higher use of video programs. In line with this hypothesis, the availability of home and/or school computers should positively affect the use of ICT video tools for teaching as well.
Furthermore, we use rural or urban places of residence with 55% of respondents and 44.9% of respondents, respectively. To measure the difference between the rural and urban speed of internet connection, we construct an interaction between the two variables. If unequal access to the internet across rural and urban teachers holds, we expect a higher reported speed on the internet for urban teachers and as a result higher use of ICT video tools by urban teachers.
We include age and education to account for teachers’ socio-demographic characteristics. Education is represented by five categories starting with secondary (school level) and ending with a post-graduate degree (PhD). However, due to a significant imbalance between categories (Table 1), we recode education into a dichotomous variable with 1 for teachers with university degrees and 0 for teachers with secondary education and college degrees. The resulting distribution is 91.1% for the first group and 8.9% for the second. Age is a continuous variable with an unweighted sample mean of 40 and a standard deviation of 11. In line with Cantú Ballesteros et al. (2017), we expect that age is negatively associated with the use of online ICT video programs; older teachers use tools for synchronous teaching less than younger teachers. At the same time, education is expected to be positively related to the use of ICT programs; teachers with a university degree are more likely to use ICT tools than teachers who do not have a university degree.
The final set of independent variables consists of teachers’ professional qualifications and professional category achieved, teaching experience measured in years, and workload measured in teaching hours. In Kazakhstan, professional categories for teachers can be combined into four groups, from the lowest to the highest category. We code them from 1 to 4 with 4 representing teachers with the lowest category. Teacher’s categorization is specific to Kazakhstan’s educational context and reflects the teacher’s seniority, achievements, and professional development (Minister of Education and Science, Republic of Kazakhstan, order nr. 192, 2020). Descriptive statistics for continuous variables such as age, experience and workload are given in Table 2, whereas the distribution of teachers for categorical variables (education, professional category) is given in Table 1. We deleted the missing values by using the listwise deletion, thus the original dataset with 3,349 observations was reduced to 3,029 observations.
To avoid potential issues of multicollinearity due to the introduction of the interaction term into the analysis, we run the model using both, centered and non-centered data. For the centered case, we followed the centering strategy proposed by Kraemer and Blasey (2004). More specifically, all dichotomous variables were coded as +1/2 (+0.5) and − 1/2 (−0.5), whereas all continuous and ordinal were centered around median values. The categorical variable representing the teacher professional category was dummy coded with subsequent coding 1-1/m for 1 and − 1/m for 0. Regression results of the non-centered data are presented in Table 3. The regressions were calculated on the unweighted data, models with the weighted data produced non-convergent solutions.
Since the dependent variable is categorical ordinal, proportional odds ordinal logistic regression (McCullagh, 1980) was used in the analysis with subsequent Brant test to check whether the proportional odds assumption holds across the categories of the dependent variable (Brant, 1990; Long, 1997).
In case any of the regression coefficients are not equal across categories the proportional odds assumption is violated and it is more appropriate to use partial proportional odds models, generalized ordered or multinomial regression models (Fullerton, 2009). Further, to test ordinal regression goodness-of-fit we used McFadden, Nagelkerke and Cox-Snell pseudo-R square coefficients (McFadden, 1974; Cox and Snell, 1989; Nagelkerke, 1991) along with Pulkstein-Robinson deviance and chi-square tests, Hosmer Lemeshow, and Lipsitz likelihood ratio tests (Fagerland and Hosmer, 2017). In addition, we looked at Akaike Information Criterion (AIC) to compare different models (Akaike, 1972) and conducted likelihood ratio tests to see whether the addition of variables led to improvement in model fit (Vuong, 1989).
All data analysis in this paper was onducted in R statistical programming software (R Core Team, 2020). We used polr function from MASS package to run ordinal regression model analysis (Ripley et al., 2022), DescTools package for pseudo-R square coefficients (Signorell, 2021), generalhoslem for calculation of goodness-of-fit statistics (Jay, 2019), effects for calculation of predicted probabilities from ordinal regression models (Fox, 2022) and ggplot2 for the visualization of results (Wickham et al., 2022).
Overall, we consequently calculated three separate models. In the first model, we used the reported speed of the internet, geographic location, the interaction between them, and the availability of school and home PC/laptops. In the second model, we added age and education. The third model added experience, workload, and teachers’ professional category. The indicators of model fit AIC showed a gradual decrease from model 1 to model three from 8,989 to 8,910 (Table 4). The same positive increase can be pointed out across all pseudo-coefficients, with Nagelkerke showing an increase from 0.11 to 0.13 (Table 5). Furthermore, large p-values (p > 0.05) indicated a good fit for model 3 according to all four goodness-of-fit tests (Table 6). Finally, the likelihood ratio test showed a statistically significant difference of 0.0006 between model 1 and model 2 and between model 2 and model 3 (1.08e–14), meaning that the inclusion of socio-demographic characteristics and professional qualification variables led to the improvement in model fit. Regarding the proportional odds assumption Brant test showed large p values (> 0.05) for the omnibus test as well all variables in model 3 except the availability of Home PC/Lap meaning that the proportional odds assumption was satisfied, and the effects of the regression coefficients were the same across the categories of the dependent variable.
This section presents the results of three fitted models analyzing how access to ICT, socio-demographic and professional characteristics of teachers impacted teachers’ engagement in remote synchronous teaching (Table 4). We interpret a marginal change on one unit simultaneously, holding other continuous variables constant and categorical variables at their reference categories. Results are considered to be statistically significant based on the size of standard errors (s.e.) relative to the regression coefficient (β), if two standard errors do not include zero, then one can safely claim that a coefficient is statistically significant.
In the first model, there is a statistically significant effect of dummy coded rural–urban area, more specifically for urban teachers, who do not have an access to the school computers, the log odds of being in a higher category of ICT video tools use is 0.86 (s.e. = 0.08) higher than for rural teachers. With regards to the speed of the Internet, the effect for rural teachers is statistically significant, a category increase in the reported speed of the Internet leads to 0.34 (s.e. = 0.03) increase in the log odds of being in a higher reported category of ICT tools used for online teaching, holding everything else constant. However, the interaction of locality and speed of the Internet does not show a statistically significant effect, with the two standard errors being larger than the regression coefficient and covering 0 (β = −0.07, s.e. = 0.07). Moreover, while the availability of a home PC/laptop is not statistically significant, access to school PC/laptop for rural teachers positively affects the use of online video tools (β = 0.27, s.e. = 0.07).
In the second model, age indicates a statistically significant negative effect (β = −0.09, s.e. = 0.03), nonetheless, teachers’ education does not have a substantial impact on the use of ICT video tools for teaching (β = −0.05, s.e. = 0.11). However, one must keep in mind that the majority of teachers (90%) in the sample are with higher education degrees which could influence the estimates in the regression model. At the same time the type of locality (β = 0.87 - urban) and speed of the Internet (β= 0.33) continue to show statistical significance (s.e = 0.08 and s.e = 0.03), whereas interaction between them does not (β = −0.08, s.e. = 0.07).
The third model highlights the statistical effects of the group of variables related to professional characteristics. More specifically, more experienced, rural teachers with the highest professional category, without the access to school computers, tend to report a higher use of video tools for online lessons with the log odds coefficient of 0.02 (s.e. = 0.005). The same could be said about the workload where an increase in workload for a rural, teacher with the highest professional category and without the access to school PC, leads to 0.21 increase in the log odds of being in the group of the teachers with higher reported use of video tools as opposed to the group with lower reported use of video tools (s.e = 0.03). Furthermore, the results show statistically significant negative effects of dummy coded professional categories, the coefficients for categories 2, 3, and 4 (β = −0.34, β = −0.37, β = −0.52 respectively) are negative, meaning that rural teachers without the access to school computers with lower professional categories tend to report the lower frequency of use of video tools for online lessons as opposed to their colleagues with the highest category (reference category).
In other words, more senior teachers by the qualification category used ICT tools for synchronous teaching more than their junior colleagues in terms of the professional qualification category. At the same time, an increase in teachers’ workload and years of experience led to the increase in the use of ICT tools, whereas education, although highly imbalanced toward teachers with bachelor and master degrees, indicated no statistically significant effect. Surprisingly one of the main variables measuring digital divide – interaction between rural–urban locality and the speed of Internet did not show significance across all three models, while independently, both variables show statistically significant effects. In addition, the results indicated the importance of school PC/laptop availability for online synchronous teaching as opposed to home PC/laptop, which is not significant across all three models.
Table 6 gives percentage change between the minimum and maximum marginal predicted probabilities for statistically significant explanatory variables in model 3. More specifically, urban teachers have 11% more probability (0.28) of always using ICT video for synchronous class instruction each lesson than rural teachers (0.17). There is a 12% difference (0.21–0.33) between very low and very high reported speed of Internet with regards to teachers who often use ICT video tools for synchronous teaching. On average, the availability of school PC/laptops increases the use of ICT tools for synchronous online teaching by 2 (categories never, rarely, and often) to 5 (categories sometimes and always) percent. The above-mentioned effects are presented in Figures 1, 2.
Figure 1. Predicted probabilities of the effect of the Internet speed on the use of ICT video tools.
Figure 2. Predicted probabilities of the effect of rural -urban locality on the use of ICT video tools.
The non-centered solution (Table 3) did not substantially change the results of the modeling. The solution is almost similar to the model with centered predictors with the same statistical significance and direction of the regression coefficients for age, speed of Internet, school PC/lap, experience, and category. With almost everything being equal, we nonetheless stick to the output of the centered solution as it provides more stable estimates than the non-centered solution. More specifically the logit regression coefficients of rural–urban and teachers’ workload variables have larger standard errors (s.e. = 0.27 and s.e. = 0.12) in the non-centered as opposed to the centered solution (s.e. = 0.08 and s.e. = 0.03), although in both cases the regression coefficients indicate the statistical significance and the same direction of the effect.
The goal of this paper was to examine how three aspects of digital inequality - digital access, capability, and outcome divide - were influencing synchronous online teaching among mainstream schoolteachers in Kazakhstan during COVID - 19 school closures. The paper sought to investigate the effect of several factors. They were urban or rural location, the speed and steadiness of the internet connection, the availability of ICT at home and at school, and teacher characteristics such as age, qualification and work experience. This study reportedly found that socio-demographic differences exacerbated the digital divide in synchronous teaching over MS Teams, Zoom, and alike. Yet some of the results in this paper were unexpected.
In terms of access to digital technologies and the internet to engage in synchronous online teaching, the availability of school devices and the speed of the internet were found to be significant factors that predicted teachers` remote teaching experiences. In other words, teachers who had access to computers at schools and better internet were likely to use digital tools for synchronous online instruction more often when compared to those who did not have such physical access to ICT. This finding is in line with conclusions in literature that the digital divide due to the lack of ICT access remains a major problem in many parts of the world (Van Dijk, 2006; van Deursen and van Dijk, 2014; Pierce, 2018).
Interestingly, the availability of computers at school rather than at home predicted a higher engagement of teachers in synchronous online teaching. In Kazakhstan, even though schools were closed for students during the pandemic, teachers could access the school facilities and work from their classrooms following health safety measures. Another explanation for this finding might be that teachers working from home might not have been able to have a computer for their personal use only, needing to share their home devices with other family members. Thus, the availability of a computer that a teacher could use exclusively for their teaching, at school or provided by the school to be used at home, is an important factor in closing the digital divide.
In line with the previous studies (Bagchi, 2005; Hosszu and Rughinis, 2020), our results confirm the difference between rural and urban teachers in the use of ICT tools for synchronous teaching. Nonetheless, a surprising finding is the absence of statistical significance between the locality and speed of the internet. In Kazakhstan, internet coverage in rural settlements does not reach every household, but it does reach key institutions such as local municipal quarters, schools and hospitals (Aliyeva and Kovyazina, 2021). The result shows that the digital access divide may persist in the country, putting teachers in rural areas at a disadvantage.
Alternatively, these results can be related to the phenomena of social desirability bias (SDB) in survey responses (Edwards, 1957; Crowne and Marlowe, 1960; Phillips and Clancy, 1972) when respondents tend to give answers that show them in favorable light. Thus, teachers may not feel confident about judging the internet quality and restrained from criticizing it openly. This is particularly relevant for sensitive questions about attitude and behavior (Krumpal, 2013). Although to date there is only one attempt at measuring SDB in survey responses in the educational context of Kazakhstan (Nurumov et al., 2022), some surprising findings of OECD’s international large-scale surveys and assessments of TALIS and PISA (OECD, 2019, 2020a,b) may support the cautious interpretation of the results in this article regarding the quality of the internet connection keeping in mind potential presence of high SDB in responses.
To examine how the digital capability divide influenced teacher engagement in synchronous online teaching and learning during Covid-19 school closures in Kazakhstan, this paper focused on teachers’ personal characteristics such as age and professional qualification and experience. Findings on the impact of teachers’ age on their synchronous online teaching practice were in line with those in other studies suggesting that age is the most common indicator resulting in unequal use of digital tools (Enoch and Soker, 2006; Ertl et al., 2020). Older teachers with higher professional rank in Kazakhstan engaged in synchronous online teaching more frequently than their younger counterparts. This suggests that senior teachers tend to adopt recent technologies and have necessary knowledge of using them in remote instruction. It confirms that teachers might be an exception to research findings that lower acceptance and awareness of new technology-led learning are most pronounced among older people compared to younger generations (Olphert and Damodaran, 2013; Wu et al., 2015; Vaportzis et al., 2017;). We initially expected that those teachers who are young in age yet have lower professional rank might be more exposed to a digital teaching environment and use synchronous modes of teaching more frequently than their older counterparts who might find online teaching demanding. Given the prevalence of the older age group among teachers who hold a higher professional rank in our study, suggesting the professional seniority of a teacher, it was a surprise that teachers with longer work experience reported higher engagement in synchronous online teaching than their younger and more recently employed colleagues. From the one hand these results confirmed that the professional rank of teachers in Kazakhstan is associated with their digital capability. From the other, teacher responses may be contaminated by socially desirable answers or other response styles. Given a large number of surveys and educational assessments in Kazakhstan, more research is needed in this direction.
The main conclusion emerging in this research about digital skills divide echoed those from other contexts that ICT skills and their use decrease with age and younger teachers apply ICT tools in their teaching more than their older and more experienced colleagues (Van Braak et al., 2004; Inan and Lowther, 2010; Pegler et al., 2010). At the same time, studies have shown that the likelihood of teachers using ICT in teaching increases if teachers are properly trained to do that (Drossel and Eickelmann, 2017; Pongsakdi et al., 2021). This paper did not examine the question of the teachers’ professional development in ICT in Kazakhstan. However, TALIS (OECD, 2019) findings on Kazakhstani teachers’ ICT training presents some inconclusive results. Even though seven in 10 Kazakhstani teachers reported having “ICT for teaching” as part of their formal education or training, many more Kazakhstani teachers than the OECD average reported a high need for training to use ICT. This suggests the need to assess the effectiveness of the measures taken to improve the digital capabilities of pre-service and in-service teachers in Kazakhstan. Also, ICT training for teachers in the pre-pandemic period appear to have no lasting effect when navigating the shift from traditional to distance teaching. For instance, Azhari and Fajri (2021) found that teachers’ knowledge and willingness to use ICTs before COVID-19 cannot be applied directly in the learning during the COVID-19 pandemic because they used to integrate ICT in typical classrooms. In other words, the experiences acquired through the ICT training courses are less likely to lead to the continued use of that digital knowledge and skills while implementing synchronous teaching during COVID-19.
A gap in digital access and capabilities leads to a digital outcome divide. The digital outcome divide “underlines inequalities in the benefits gained from the different levels of access and usage of the Internet” (Ragnedda and Kreitem, 2018, p. 8). It leads to different experiences in learning outcomes and purposeful ICT usage (Wei et al., 2011; van Deursen and Helsper, 2015). Furthermore, regarding teachers, inequality in access to digital technologies and the internet limits professional development, technical skills, and knowledge acquisition through the building of social networks (Valadez and Duran, 2007). Teachers’ ability to engage in synchronous online instruction using programs such as Zoom and Google Meet is an indicator of the divide in digital outcomes or lack of it thereof.
As data in this research shows, the most limiting factors for teachers in Kazakhstan to benefit from ICT access and usage in teaching are the urban–rural divide, availability of ICT at schools and teachers’ age. Thus, it can be argued that the most vulnerable to digital outcomes divide are rural, young and inexperienced teachers at schools that are not properly equipped with ICT technology. It is important to remember that limitations in digital access and capabilities for teachers are likely to affect the effectiveness of remote learning for students. While based on the data in this paper we cannot draw definite conclusions about how the digital outcomes divide experienced by teachers impacts students, research indicates that in settings with less digital access and capability among teachers, there is a bigger digital outcomes gap for students (Zhao et al., 2022). Thus, expanding digital access and capabilities among teachers would likely improve digital outcomes for students as well.
Kazakhstan was one of the countries that experienced extended school closures due to the COVID-19 pandemic in 2020. The whole education system switched to remote instruction regardless of the teacher preparation to teach online. An international review of remote learning and teaching during the COVID-19 pandemic found that synchronous teaching, which provides better opportunities for interaction, was more prevalent in the Global North countries (Lucas et al., 2020). This paper examined what factors of digital divide influenced synchronous teaching in Kazakhstan, an upper-middle-income country in Central Asia.
This study found that the digital access divide narrows when schools provide teachers with ICT, which they can use for teaching remotely. The difference in synchronous technology use between teachers in rural and urban areas was significant, suggesting the presence of the digital access divide. In terms of digital skills, the single most crucial factor for teachers’ capability to engage in synchronous teaching was age. Surprisingly, teachers with higher professional rank were keener to engage in synchronous teaching than their younger counterparts who belong to lower professional rank. In practical terms this means that investment in school ICT infrastructure development is worth wile to diminish digital divide in access. To reduce digital divide in skills, it is relevant to provide younger and less experienced teachers with ICT-related professional development which targets remote teaching specifically. These two steps will contribute to reducing divide in digital outcomes in teaching and learning.
To gain a more nuanced understanding of how teachers experience digital divide in access, skills and outcomes, it would be beneficial for future research to examine this question in the framework of qualitative research design. When extending the inquiry on this topic, including focus on asynchronous modes of teaching and learning would be important. This study was limited in its focus on synchronous teaching only. Research on blending both synchronous and asynchronous teaching modes might bring a more balanced perspective toward diverse remote teaching experiences in schools. Future research exploring both online teaching formats would be essential to mitigate the challenges associated with synchronous and asynchronous teaching and learning, allowing students to engage with what best suits their individual learning conditions and needs.
The raw data supporting the conclusions of this article will be made available by the authors, without undue reservation.
Ethical review and approval was not required for the study on human participants in accordance with the local legislation and institutional requirements. Written informed consent for participation was not required for this study in accordance with the national legislation and the institutional requirements.
AAm: conceptualization, investigation, and writing. KN: conceptualization, investigation, data curation, visualization, writing, and funding acquisition. RK: conceptualization, supervision, and writing – review and editing. AAk and AK: conceptualization, investigation and supervision. All authors contributed to the article and approved the submitted version.
This research was funded by the Science Committee of the Ministry of Education and Science of the Republic of Kazakhstan (Grant No. OR11465485).
This study would not be possible without the input into the study conceptualisation, methodology, and data collection by our colleagues at IAC.
The authors declare that the research was conducted in the absence of any commercial or financial relationships that could be construed as a potential conflict of interest.
All claims expressed in this article are solely those of the authors and do not necessarily represent those of their affiliated organizations, or those of the publisher, the editors and the reviewers. Any product that may be evaluated in this article, or claim that may be made by its manufacturer, is not guaranteed or endorsed by the publisher.
Akaike, H. (1972). “Information theory and an extension of the maximum likelihood principle,” in Selected papers of Hirotugu Akaike. eds. E. Parzen, K. Tanabe, and G. Kitagawa (New York: Springer Series in Statistics), 199–213.
Aliyeva, S., and Kovyazina, K. (2021). The New Frontiers of Educational Inequality in Central Asia: From Measurement to Policy Change [Novyye Grani Obrazovatel’nogo Neravenstva v Stranakh Tsentral’noy Azii: Ustraneniye Problem s Izmeneniyem Politiki]. Available at: https://paperlab.Kz/research (Accessed May 16, 2021).
Azhari, B., and Fajri, I. (2021). Distance learning during the COVID-19 pandemic: school closure in Indonesia. Int. J. Math. Educ. Sci. Technol. 53, 1934–1954. doi: 10.1080/0020739X.2021.1875072
Azubuike, O., Adegboye, O., and Quadri, H. (2020). Who gets to learn in a pandemic? Exploring the digital divide in remote learning during the COVID-19 pandemic in Nigeria. Int. J. Educ. Res. Open 2:100022. doi: 10.1016/j.ijedro.2020.100022
Bagchi, K. (2005). Factors сontributing to global digital divide: some empirical results. J. Glob. Inf. Technol. Manag. 8, 47–65. doi: 10.1080/1097198X.2005.10856402
Blau, I., Weiser, O., and Eshet-Alkalai, Y. (2017). How do medium naturalness and personality traits shape academic achievement and perceived learning? An experimental study of face-to-face and synchronous e-learning. Research in Learning Technology, 25. doi: 10.25304/rlt.v25.1974
Bokayev, B., Torebekova, Z., Abdykalikova, M., and Davletbayeva, Z. (2021b). Exposing policy gaps: the experience of Kazakhstan in implementing distance learning during the COVID-19 pandemic. Transform. Gov. 15, 275–290. doi: 10.1108/TG-07-2020-0147
Bokayev, B., Torebekova, Z., Davletbayeva, Z., and Zhakypova, F. (2021a). Distance learning in Kazakhstan: estimating parents’ satisfaction of educational quality during the coronavirus. Technol. Pedagog. Educ. 30, 27–39. doi: 10.1080/1475939X.2020.1865192
Brant, R. (1990). Assessing proportionality in the proportional odds model for ordinal logistic regression. Biometrics 46, 1171–1178. doi: 10.2307/2532457
Carifio, J., and Perla, J. R. (2007). Ten common misunderstandings, misconceptions, persistent myths and urban legends about Likert scale and Likert response formats and their antidotes. J. Soc. Sci. 3, 106–116. doi: 10.3844/jssp.2007.106.116
Cantú-Ballesteros, L., Urías-Murrieta,, M.,, Figueroa-Rodríguez,, S.,, and Salazar-Lugo, G. M. (2017). Teacher‘s Digital Skills in Relation to Their Age, Gender, Time of Usage and Training with a Tablet. Journal of Education and Training. Studies 5. doi: 10.11114/jets.v5i5.2311
Correa, T. (2015). The power of youth: how the bottom-up technology transmission from children to parents is related to digital (in)equality. Int. J. Commun. 9, 1163–1186.
Crowne, D., and Marlowe, D. (1960). A new scale of social desirability independent of psychopathology. J. Consult. Psychol. 24, 349–354. doi: 10.1037/h0047358
Crompton, H., Burke, D., Jordan, K., and Wilson, S. W. G. (2021). Learning with technology during emergencies: A systematic review of K-12 education. British Journal of Educational Technology 52, 1554–1575. doi: 10.1111/bjet.13114
Darmody, M., Smyth, E., and Russell, H. (2021). Impacts of the COVID-19 control measures on widening educational inequalities. Young 29, 366–380. doi: 10.1177/11033088211027412
Davies, J. A., Davies, L. J., Conlon, B., Emerson, J., Hainsworth, H., and McDonough, H. G. (2020). Responding to COVID-19 in EAP contexts: A comparison of courses at four Sino-foreign universities. International Journal of TESOL Studies 2, 32–52.
Digital Kazakhstan (2018). Ministry of Digital Development, Innovations and Aerospace Industry of Kazakhstan. State Program of Digital Kazakhstan. Available at: https://digitalkz.kz/en/about-the-program/
Dijk, V., Jan, A. G. M., and Hacker, K. (2003). The digital divide as a complex and dynamic phenomenon. Inf. Soc. 19, 315–326. doi: 10.1080/01972240309487
Drossel, K., and Eickelmann, B. (2017). Teachers' participation in professional development concerning the implementation of new Technologies in Class: a latent class analysis of teachers and the relationship with the use of computers, ICT self-efficacy and emphasis on teaching ICT skills. Large Scale Assess. Educ. 5:9. doi: 10.1186/s40536-017-0053-7
Edwards, A. L. (1957). The Social Desirability Variable in Personality Assessment and Research, New York: Dryden.
Ekberg, S., and Gao, S. (2018). Understanding challenges of using ICT in secondary schools in Sweden from Teachers' perspective. Int. J. Inf. Learn. Technol. 35, 43–55. doi: 10.1186/s40536-017-0053-7
Enoch, Y., and Soker, Z. (2006). Age, Gender, Ethnicity and the Digital Divide: University Students’ Use of Web Based Instruction. Open Learning 21, 99–110. doi: 10.1080/02680510600713045
Ertl, B., Csanadi, A., and Tarnai, C. (2020). Getting closer to the digital divide: an analysis of impacts on digital competencies based on the German PIAAC sample. Int. J. Educ. Dev. 78:102259. doi: 10.1016/j.ijedudev.2020.102259
Fabriz, S., Mendzheritskaya, J., and Stehle, S. (2021). Impact of synchronous and asynchronous settings of online teaching and learning in higher education on Students' learning experience during COVID-19. Front. Psychol. 12:733554. doi: 10.3389/fpsyg.2021.733554
Fagerland, M. W., and Hosmer, D. W. (2017). How to test for goodness of fit in ordinal logistic regression models. Stata J. 17, 668–686. doi: 10.1177/1536867X1701700308
Fox, J. (2022). Package “effects”. Available at: https://cran.r-project.org/web/packages/effects/effects.pdf (Accessed August 18, 2021).
Fullerton, A. S. (2009). A conceptual framework for ordered logistic regression models. Sociol. Methods Res. 38, 306–347. doi: 10.1177/0049124109346162
González-Betancor, S., López-Puig, A., and Cardenal De La Nuez, M. (2021). Digital inequality at home. The school as compensatory agent. Comput. Educ. 168:104195. doi: 10.1016/j.compedu.2021.104195
Hambly, G. (2021). Kazakhstan. Available at: https://www.britannica.com/place/Kazakhstan#ref73555 (Accessed March 28, 2021).
Hargreaves, A. (2021). Austerity and inequality or prosperity for all? Educational policy directions beyond the pandemic. Educ. Res. Policy Prac. 20, 3–10. doi: 10.1007/s10671-020-09283-5
Harpe, S. E. (2015). How to analyze Likert and other rating scale data. Curr. Pharm. Teach. Learn. 7, 836–850. doi: 10.1016/j.cptl.2015.08.001
Hartviksen, G., Akselsen, S., and Eidsvik, A. K. (2002). MICTS: municipal ICT schools – a means for bridging the digital divide between rural and urban communities. Educ. Inf. Technol. 7, 93–109. doi: 10.1023/A:1020349509331
Hargittai, E. (2002). Second-Level Digital Divide: Differences in People’s Online Skills. First Monday 7.
Hennessy, S., D’Angelo, S., McIntyre, N., Koomar, S., Kreimeia, A., Cao, L., et al. (2022). Technology Use for Teacher Professional Development in Low- and Middle-Income Countries: A systematic review. Computers and Education Open 3. doi: 10.1016/j.caeo.2022.100080
Hosszu, A., and Rughinis, C. (2020). Digital divides in education. An analysis of the Romanian public discourse on distance and online education during the Covid-19 pandemic. Sociol. Românească 18, 11–39. doi: 10.33788/sr.18.2.1
Hrastinski, S. (2010). “How do e-learners participate in synchronous online discussions? Evolutionary and social psychological perspectives” in Evolutionary Psychology and Information Systems Research. ed. N. Kock (Boston, MA: Springer US), 119–147.
Hsu, T., and Feldt, S. L. (1969). The effect of limitations on the number of criterion score values on the significance level of the F-test. Am. Educ. Res. J. 6, 515–527. doi: 10.3102/00028312006004515
Inan, F. A., and Lowther, D. L. (2010). Factors affecting technology integration in K-12 classrooms: a path model. Educ. Technol. Res. Dev. 58, 137–154. doi: 10.1007/s11423-009-9132-y
Ibrayeva, L. (Ed.) (2014). “Education Reform and Internationalization: The Case of School Reform in Kazakhstan” in Educational Review. Vol. 74. ed. D. Bridges (Cambridge: Cambridge University Press). 158–159.
Jay, M. (2019). Package “generalhosem”. Available at: https://cran.r-project.org/web/packages/generalhoslem/generalhoslem.pdf (Accessed August 18, 2021).
Kale, U., and Goh, D. (2014). Teaching style, ICT experience and Teachers' attitudes toward teaching with web 2.0. Educ. Inf. Technol. 19, 41–60. doi: 10.1007/s10639-012-9210-3
Klymenko, E. Y., and Alpeissova, S. E. (2021). “The experience of Ukraine and Kazakhstan of digitalization education under quarantine conditions” in Advances in Digital Science. ICADS 2021 (Advances in Intelligent Systems and Computing). ed. T. Antipova (Cham: Springer), 1352.
Kovyazina, K., Boranbay, M., and Beisembayev, S. (2021). Distantsionnoye Obrazovaniye v Kazakhstane Glazami Pedagogov i Ekspertov: Vyzovy i Vozmozhnosti [Distance Education in Kazakhstan through the Eyes of Teachers and Experts: Challenges and Opportunities]. Paperlab. Available at: https://paperlab.kz/#rec163441660 (Accessed June 23, 2021).
Kraemer, H. C., and Blasey, C. M. (2004). Centring in regression analyses: a strategy to prevent errors in statistical inference. Int. J. Methods Psychiatr. Res. 13, 141–151. doi: 10.1002/mpr.170
Krumpal, I. (2013). Determinants of social desirability bias in sensitive surveys: a literature review. Qual. Quant. 47, 2025–2047. doi: 10.1007/s11135-011-9640-9
Lantz, B. (2013). Equidistance of Likert-type scales and validation of inferential methods using experiments and simulations. Electron. J. Bus. Res. Methods 11, 16–28.
León-Nabal, B., Zhang-Yu, C., and Lalueza, J. L. (2021). Uses of digital mediation in the school-families relationship during the COVID-19 pandemic. Front. Psychol. 12:687400. doi: 10.3389/fpsyg.2021.687400
Light, J. S. (2001). Rethinking the digital divide. Harv. Educ. Rev. 71, 709–734. doi: 10.17763/haer.71.4.342x36742j2w4q82
Long, S. J. (1997). “Regression models for categorical and limited dependent variables,” in Advanced Quantitative Techniques in the Social Sciences Number 7 (Thousand Oaks, CA: Sage Publications).
Lucas, M., Nelson, J., and Sims, D. (2020). Schools’ responses to Covid-19: Pupil engagement in remote learning. National foundation for Educational Research [NFER]. Available at: https://www.nfer.ac.uk/media/4073/schools_responses_to_covid_19_pupil_engagement_in_remote_learning.pdf
Mathrani, A., Sarvesh, T., and Umer, R. (2021). Digital divide framework: online learning in developing countries during the COVID-19 lockdown. Globalisation, Societies and Education 20, 625–640. doi: 10.1080/14767724.2021.1981253
McCullagh, P. (1980). Regression models for ordinal data. J. R. Stat. Soc. Ser. B (Methodological) 42, 109–127. doi: 10.1111/j.2517-6161.1980.tb01109.x
McFadden, D. (1974). “Conditional logit analysis of qualitative choice behavior” in Frontiers in Econometrics. ed. P. Zarembka (New York: Academic Press), 105–142.
Minister of Education and Science, Republic of Kazakhstan, order nr. 192. (2020). Rules for Assigning (Confirming) Qualification Categories to Teachers. Available at: https://adilet.zan.kz/eng/docs/V2000020618
Moorhouse, B. L., and Wong, K. M. (2022). Blending asynchronous and synchronous digital technologies and instructional approaches to facilitate remote learning. J. Comput. Educ. 9, 51–70. doi: 10.1007/s40692-021-00195-8
Mumtaz, S. (2000). Factors affecting teachers’ use of information and communications technology: a review of the literature. J. Inf. Technol. Teach. Educ. 9, 319–342. doi: 10.1080/14759390000200096
Mynarˇíková, L., and Novotný, L. (2021). The current challenges of further education in ICT with the example of the Czech Republic. Sustainability 13:4106. doi: 10.3390/su13084106
Nagelkerke, N. J. D. (1991). A note on a general definition of the coefficient of determination. Biometrika 78, 691–692. doi: 10.1093/biomet/78.3.691
Norman, G. (2010). Likert scales, levels of measurement and the “Laws” of statistics. Adv. Health Sci. Educ. 15, 625–632. doi: 10.1007/s10459-010-9222-y
NTIA (1998). Falling through the Net II: New Data on the Digital Divide. Available at: https://www.ntia.doc.gov/report/1998/falling-through-net-ii-new-data-digital-divide (Accessed December 3, 2021).
Nurumov, K., Hernández-Torrano, D., Ait Si Mhamed, A., and Ospanova, U. (2022). Measuring social desirability in collectivist countries: a psychometric study in a representative sample from Kazakhstan. Front. Psychol. 13:822931. doi: 10.3389/fpsyg.2022.822931
OECD (2017). Reforming Kazakhstan: Progress, Challenges and Opportunities. Available at: https://www.oecd.org/eurasia/countries/OECD-Eurasia-Reforming-Kazakhstan-EN.pdf
OECD (2019), TALIS 2018 Results (Volume I): Teachers and School Leaders as Lifelong Learners, TALIS, Paris: OECD Publishing.
OECD. (2020a). TALIS 2018 Results (Volume II): Teachers and School Leaders as Valued Professionals, TALIS, Paris: OECD Publishing.
OECD. (2020b). Regional Policies to Support Diversification and Productivity Growth in Kazakhstan. Available at: https://www.oecd.org/eurasia/competitiveness-programme/central-asia/Regional-Policies-to-Support-Diversification-and-Productivity-Growth-in-Kazakhstan-ENG.pdf (Accessed November 16, 2021).
Olphert, W., and Damodaran, L. (2013). Older people and digital disengagement: a fourth digital divide? Gerontology 59, 564–570. doi: 10.1159/000353630
Pegler, K., Kollewyn, J., and Crichton, S. (2010). Generational attitudes and teacher ICT use. J. Technol. Teach. Educ. 18, 443–458.
Phillips, L. D., and Clancy, J. K. (1972). Some effects of “social desirability” in survey studies. Am. J. Sociol. 77, 921–940. doi: 10.1086/225231
Pongsakdi, N., Kortelainen, A., and Veermans, M. (2021). The impact of digital pedagogy training on in-service teachers’ attitudes towards digital technologies. Educ. Inf. Technol. 26, 5041–5054. doi: 10.1007/s10639-021-10439-w
Quaicoe, J. S., and Pata, K. (2020). Teachers’ digital literacy and digital activity as digital divide components among basic schools in Ghana. Educ. Inf. Technol. 25, 4077–4095. doi: 10.1007/s10639-020-10158-8
R Core Team (2020). R: A Language and Environment for Statistical Computing, Vienna, Austria: R Foundation for Statistical Computing.
Ragnedda, M., and Kreitem, H. (2018). The three levels of digital divide in east EU countries. J. Russ. Med. J. Stud. 5, 5–26. doi: 10.30547/worldofmedia.4.2018.1
Ripley, B., Venables, B., Bates, D. M., Hornik, K., Gebhardt, A., and Firth, D. (2022). Package “MASS”. Available at: https://cran.r-project.org/web/packages/MASS/MASS.pdf (Accessed August 10, 2021).
Scheerder, A., van Deursen, A., and van Dijk, J. (2017). Determinants of Internet skills, use and outcomes: A systematic review of the second- and third-level digital divide. Telematics and informatics 34, 1607–1624. doi: 10.1016/j.tele.2017.07.007
Schreiber, W. B. (2022). Teaching in a pandemic: Adapting preparations for asynchronous remote learning using three evidence-based practices. Scholarship of Teaching and Learning in Psychology 8, 106–112. doi: 10.1037/stl0000208
Signorell, A. (2021). Package “Desc Tools”. Available at: https://cran.r-project.org/web/packages/desctools/desc tools.pdf (Accessed August 16, 2021).
Starkey, L., Sylvester, A., and Johnstone, D. (2017). Negotiating digital divides: perspectives from the New Zealand schooling system. J. Res. Technol. Educ. 49, 31–42. doi: 10.1080/15391523.2017.1292161
Stuart, J., O’Donnell, A. W., Scott, R., O’Donnell, K., Lund, R., and Barber, B. (2022). Asynchronous and synchronous remote teaching and academic outcomes during COVID-19. Distance Education 43, 408–425. doi: 10.1080/01587919.2022.2088477
TALIS (2018). Country Note: Results from TALIS 2018: Kazakhstan. Available at: https://www.oecd.org/countries/kazakhstan/TALIS2018_CN_KAZ.pdf (Accessed June 25, 2021).
UN Economic and Social Commission for Asia and the Pacific. (2020). An in-depth National Study on ICT Infrastructure Deployment with Road Transport and Energy Infrastructure in Kazakhstan. Part 1. Available at: https://unescap.org/resources/kazakhstan-study-ict-deployment-along-transport-and-energy-part-1# (Accessed August 9, 2021).
UNESCO. (2021). UNESCO Figures Show Two Thirds of an Academic Year Lost on Average Worldwide Due to Covid-19 School Closures. Available at: https://en.unesco.org/news/unesco-figures-show-two-thirds-academic-year-lost-average-worldwide-due-covid-19-school (Accessed March 8, 2021).
Valadez, J., and Duran, R. (2007). Redefining the digital divide: beyond access to computers and the internet. High Sch. J. 90, 31–44. doi: 10.1353/hsj.2007.0013
van Braak, J., Tondeur, J., and Valcke, M. (2004). Explaining different types of computer use among primary school teachers. Eur. J. Psychol. Educ. 19, 407–422. doi: 10.1007/BF03173218
van Deursen, A. J., and Helsper, E. J. (2015). “The third level digital divide: who benefits most from being online?” in Communication and Information Technologies Annual. vol. 9 eds. L. Robinson, S. R. Cotten, and J. Schulz (Studies in Media and Communications, Emerald), 29–52.
van Deursen, A. J., and van Dijk, J. A. (2014). The digital divide shifts to differences in usage. New Media Soc. 16, 507–526. doi: 10.1177/1461444813487959
Van Dijk, J. A. G. M. (2006). Digital divide: research, achievements and shortcomings. Poetics 34, 221–235. doi: 10.1016/j.poetic.2006.05.004
van de Werfhorst, H. G., Kessenich, E., and Geven, S. (2020). The Digital Divide in Online Education. Inequality in Digital Preparedness of Students and Schools before the Start of the COVID-19 Pandemic. SocArXiv, 1–31 doi: 10.31235/osf.io/58d6p
Vaportzis, E., Clausen, M. G., and Gow, A. J. (2017). Older adults perceptions of technology and barriers to interacting with tablet computers: a focus group study. Front. Psychol. 8:1687. doi: 10.3389/fpsyg.2017.01687
Vuong, Q. H. (1989). Likelihood ration tests for models selection and non-nested hypotheses. Econometrica 57, 307–333. doi: 10.2307/1912557
Wang, J., and Wang, Y. (2021). Compare Synchronous and Asynchronous Online Instruction for Science Teacher Preparation. Journal of Science Teacher Education 32, 265–285. doi: 10.1080/1046560X.2020.1817652
Wei, K.-K., Teo, H.-H., Chan, H. C., and Tan, B. C. Y. (2011). Conceptualizing and testing a social cognitive model of the digital divide. Inf. Syst. Res. 22, 170–187. doi: 10.1287/isre.1090.0273
Wickham, H., Chang, W., Henry, L., Pedersen, T. L., Takahashi, K., Wilke, C., et al. (2022). Package “ggplot2”. Available at: https://cran.r-project.org/web/packages/ggplot2/ggplot2.pdf (Accessed November 10, 2022).
World Bank (2021). Kazakhstan at-a-glance. Available at: https://www.worldbank.org/en/country/kazakhstan (Accessed December 15, 2020).
Wu, Y., Damnée, S., Kerhervé, H., Ware, C., and Rigaud, A. (2015). Bridging the digital divide in older adults: A study from an initiative to inform older adults about new technologies. Clinical Interventions in Aging 10, 193–201. doi: 10.2147/CIA.S72399
Keywords: teachers, online teaching, digital divide, COVID-19, online survey, Kazakhstan
Citation: Amirova A, Nurumov K, Kasa R, Akhmetzhanova A and Kuzekova A (2023) The impact of the digital divide on synchronous online teaching in Kazakhstan during COVID-19 school closures. Front. Educ. 7:1083651. doi: 10.3389/feduc.2022.1083651
Received: 29 October 2022; Accepted: 28 November 2022;
Published: 10 January 2023.
Edited by:
Antonio Palacios-Rodríguez, Sevilla University, SpainReviewed by:
Noraffandy Yahaya, Universiti Teknologi Malaysia, MalaysiaCopyright © 2023 Amirova, Nurumov, Kasa, Akhmetzhanova and Kuzekova. This is an open-access article distributed under the terms of the Creative Commons Attribution License (CC BY). The use, distribution or reproduction in other forums is permitted, provided the original author(s) and the copyright owner(s) are credited and that the original publication in this journal is cited, in accordance with accepted academic practice. No use, distribution or reproduction is permitted which does not comply with these terms.
*Correspondence: Aida Amirova, YWlkYS5hbWlyb3ZhQG51LmVkdS5reg==
Disclaimer: All claims expressed in this article are solely those of the authors and do not necessarily represent those of their affiliated organizations, or those of the publisher, the editors and the reviewers. Any product that may be evaluated in this article or claim that may be made by its manufacturer is not guaranteed or endorsed by the publisher.
Research integrity at Frontiers
Learn more about the work of our research integrity team to safeguard the quality of each article we publish.