- 1School of Educational Technology, Faculty of Education, Beijing Normal University, Beijing, China
- 2College of Education Administration, Faculty of Education, Beijing Normal University, Beijing, China
Although massive open online courses (MOOCs) increase the number of choices in higher education and enhance learning, their low completion rate remains problematic. Previous studies have shown that learning engagement is a crucial factor influencing learning success and learner retention. However, few literature reviews on learning engagement in MOOCs have been conducted, and specific data analysis methods are lacking. Moreover, the internal and external factors that affect learning engagement have not been fully elucidated. Therefore, this systematic literature review summarized articles pertaining to learning engagement in MOOCs published from 2015 to 2022. Thirty articles met the inclusion and quality assurance criteria. We found that (1) learning engagement can be measured through analysis of log, text, image, interview, and survey data; (2) measures that have been used to analyze learning engagement include self-report (e.g., the Online Learning Engagement Scale, Online Student Engagement Questionnaire, and MOOC Engagement Scale) and automatic analysis methods [e.g., convolutional neural network (CNN), bidirectional encoder representations from transformers-CNN, K-means clustering, and semantic network analysis]; and (3) factors affecting learning engagement can be classified as internal (learning satisfaction, etc.) or external (curriculum design, etc.). Future research should obtain more diverse, multimodal data pertaining to social engagement. Second, researchers should employ automatic analysis methods to improve measurement accuracy. Finally, course instructors should provide technical support (“scaffolding”) for self-regulated learning to enhance student engagement with MOOCs.
Introduction
Massive open online courses (MOOCs) provide online learning opportunities for learners worldwide (Gallego-Romero et al., 2020), allowing them to learn anytime and anywhere (Shen et al., 2021). In addition to the high flexibility of learning whenever and wherever, MOOCs also enable the sharing and open access of high-quality, top university course resources (Atiaja and Proenza, 2016), which promotes educational equity.
During the coronavirus 2019 (COVID-19) pandemic, MOOCs provided higher education options and enhanced learning outcomes (Alamri, 2022), making it become an important means of education and training. However, the completion rate of MOOCs remains low (Reich and Ruipérez-Valiente, 2019; Kizilcec et al., 2020).
Bolliger et al. (2010) suggested that the low completion rates of MOOCs may be attributable to a lack of face-to-face interaction with others, leading to isolation and, potentially, failure to complete the course. Meanwhile, the cognitive effort and participation of students are crucial for MOOCs learning, for instance, the number of students' videos watched and posts are closely related to their MOOCs completion rate (Pursel et al., 2016). Compared with traditional learning, self-paced learning requires higher learning engagement such as a deeper understanding of knowledge and lasting positive emotion to achieve good results (Chaw and Tang, 2019). Such learning is characterized by the maintenance of attention, interest, passion, interactions, participation, and self-control during the learning process (Fisher et al., 2018), and relates to psychological engagement (Krause and Coates, 2008; Sun and Rueda, 2011). Previous studies showed that higher learning engagement is often associated with higher MOOC completion rates (Hone and El Said, 2016) and better academic achievement (De Barba et al., 2016). Assessing the learning engagement of students enrolled in MOOCs helps educators monitor the learning process, and can guide course instructors (Fisher et al., 2018); in this manner, the high dropout rate of MOOCs could be reduced.
Although learning engagement in MOOCs has received extensive attention from researchers, few reviews have focused on quantifying students' learning engagement, and an academic consensus has not been reached on the data of four sub-dimensions of learning engagement (Deng et al., 2019), nor on the optimal data collection (Khalil and Ebner, 2016; Chaw and Tang, 2019) and measurement methods (Atapattu et al., 2019; Zhou and Ye, 2020). Clarifying the data of four sub-dimensions of learning engagement in MOOCs is essential to effectively measure students' engagement in MOOCs, which provides a basis for instructors' perception of students' learning state. Research on analysis methods for learning engagement data may help us understand how to better monitor the engagement of students enrolled in MOOCs, and future researchers can also learn from it to select appropriate analysis methods. Finally, studies have mainly focused on internal factors that affect learning engagement (Veletsianos et al., 2015; Barak et al., 2016), although external factors also play a crucial role in learning engagement (Khalil and Ebner, 2016). Compared with internal factors, external factors are easier to improve, which can be an effective way for instructors to promote students' learning engagement.
This systematic literature review aimed to identify the various types of data and analysis methods associated with learning engagement, and to clarify the external and internal factors affecting learning engagement in MOOCs. The specific goals were to provide a reference for future research aiming to measure and analyze students' learning engagement in MOOCs.
Related works
MOOCs
MOOCs are open-access online learning platforms facilitating peer interaction and knowledge-sharing (Kop, 2011). In recent years, especially after the outbreak of COVID-19, MOOCs have become more popular worldwide (Liu et al., 2021). Many researchers believe that MOOCs are important for educating more people (Luik and Lepp, 2021). Moreover, they transcend geographic and social boundaries, granting access to educational resources to people all over the world (Hone and El Said, 2016). Compared with traditional classroom teaching, MOOCs have distinct advantages including “any-time” learning and the potential to enroll diverse groups of international learners (Lazarus and Suryasen, 2022).
However, MOOCs also have some limitations. For example, students may feel lonely when studying alone for protracted periods. Moreover, because of the minimal feedback provided during the MOOC learning process (Li and Moore, 2018) and the low quality of some MOOCs (Hone and El Said, 2016), high dropout rates and poor academic performance are becoming increasingly problematic. Jordan (2014) reported a completion rate for MOOCs of only 6.5%. To solve these problems, many researchers have performed studies, some of which found that learning engagement can have a positive impact on students' learning behavior and outcomes (Deng et al., 2020b). Students with high learning engagement, especially behavior engagement, tend to view more course resources, complete more assignments or quizzes (De Barba et al., 2016; Tseng et al., 2016), and interact with peers and inductors frequently. Therefore, they are more likely to complete a course and achieve better grades (Deng et al., 2020b).
Learning engagement in MOOCs
Learning engagement of students enrolled in MOOCs is essential to minimize dropout rates (Bezerra and Silva, 2017). Learning engagement is widely characterized in terms of the behavioral, cognitive, emotional, and social connections that MOOC participants make with the course content, instructor, and other learners (Deng et al., 2020a). Although some studies have classified learning engagement into behavioral, cognitive, and emotional engagement, this study argues that it is better to quantify learning engagement using a four-category approach that uses behavioral, cognitive, emotional, and social engagement. Because a MOOC is more like a diverse community than a traditional course, in which many learners engage in learning activities through interactions with course content, peers, or instructors, additional attention needs to be paid to learners' social engagement. Specifically, behavioral engagement refers to students' degree of involvement in educational activities (Jimerson et al., 2003), reflected in paying attention, asking questions, and participating in discussions during MOOCs (Jung and Lee, 2018). Behaviorally engaged individuals tend to comply with course requirements (Bingham and Okagaki, 2012), such as watching videos, completing assignments on time, and participating in extracurricular activities. Cognitive engagement refers to psychological investment in learning and relates to the use of self-directed strategies to improve one's understanding (Fredricks et al., 2004). Cognitive engagement is reflected in learners' efforts to acquire complex information or skills during the MOOC learning process (Jung and Lee, 2018). Emotional engagement refers to students' attitudes, interests, and values (Fredricks et al., 2004), and is reflected in the forging of emotional connections with institutions, instructors, peers, and the course content itself (Jimerson et al., 2003; Jung and Lee, 2018). Social engagement is reflected in student-student and student-teacher interactions; it is sometimes considered a subcategory of behavioral engagement, given that engagement may be viewed as a type of behavior. In many studies, however, social engagement is considered a fundamental component of students' perceptions and is measured separately from behavioral, cognitive, and emotional engagement (Deng et al., 2020a). A recent review of 102 empirical studies showed that engagement is among the major topics in the MOOC literature (Deng et al., 2019).
Some studies have measured learning engagement in the context of MOOCs and suggested indicators to quantify the level thereof. Among the current MOOC learning engagement measurement methods, the self-report method is the most common. Many studies have used scales to quantify student engagement. For example, Deng et al. (2020a) used the MOOC Engagement Scale (MES) to measure students' behavioral, cognitive, emotional, and social engagement. Since MOOCs can provide rich data (log data, text data, etc.), there is an opportunity to quantify learning engagement. Many works used log files as their primary data source to explore engagement in MOOCs (Bonafini et al., 2017). Text data (i.e., discussion forum posts made by students) have been analyzed to measure learning engagement (Liu et al., 2022). With the development of multimedia technology, more data sources allowing for the measurement of learning engagement have become available, such as image data obtained during MOOCs. One study used facial analysis technology and machine learning algorithms to automatically measure student engagement (Batra et al., 2022). However, the advantages and characteristics of various algorithms for measuring and analyzing learning engagement have not been systematically reviewed.
Learning engagement in the context of MOOCs has received extensive attention, with many researchers reviewing the factors that affect it. Paton et al. (2018) found that well-designed assessment tasks, learner collaborations, and certification enhance learners' engagement and retention. However, almost all of the factors considered were external factors; internal factors such as self-regulation ability and prior knowledge were not analyzed. Meanwhile, Alemayehu and Chen (2021) explored the factors promoting and hindering learners' engagement from the perspectives of both instructors and students, but ignored the impact of external factors such as technical support. The influence of external factors on learning engagement should not be ignored because such factors can be modified to improve learning outcomes (Gallego-Romero et al., 2020).
To address the gaps in past research, this study investigated learning engagement data types and analysis methods, as well as the factors that promote engagement in MOOCs, by reviewing 30 empirical studies on learner engagement in MOOCs published between 2015 and 2022. The research questions were as follows:
RQ1: What data are analyzed to measure learning engagement in MOOCs?
RQ2: What analysis methods are used to quantify learning engagement in MOOCs?
RQ3: What factors influence learning engagement in MOOCs?
Materials and methods
To answer the above questions, a systematic review was conducted using a replicable search strategy. The Preferred Reporting Items for Systematic Reviews and Meta-Analyses 2020 (PRISMA 2020) statement guided this study (Page et al., 2021a,b). The PRISMA 2020 statement comprises:
• A 27-item checklist address the introduction, methods, results, and discussion sections of a systematic review report. This study strictly follows the above contents.
• A flow diagram depicts the flow of information through the different phases of a systematic review, it will be shown in detail in Section Article selection.
Search strategy
Five databases were searched for relevant studies: EBSCO ERIC, Elsevier ScienceDirect, Springer Link, Web of Science, and Wiley Online Library. Six keyword combinations were searched for in the title, keyword, and abstract fields, according to the search criteria of each individual database (Table 1). The last search was conducted on April 27, 2022.
Article selection
In total, 539 articles were retrieved from the five online databases. Figure 1 illustrates the articles selection process, the number of articles retained at each stage, and reasons for article exclusion. Thirty articles that met the selection criteria were included in the final analysis.
Data distribution
Figure 2 shows the distribution of the selected articles by year. We hope to summarize the research on the measurement and analysis of learning engagement in MOOCs in the past 10 years. But no articles published in 2013 and 2014 met the selection criteria. Thus 2015 is the starting year for article selection.
Previous studies distinguished among behavioral, cognitive, emotional, and social engagement (Deng et al., 2020a); Table 2 provides details of the articles reviewed herein. Several authors explicitly indicated the dimensions of learning engagement they discussed in their articles, which were directly followed for this study. If the researchers did not indicate the dimensions of learning engagement, the three reviewers divided them independently according to the definitions of the four dimensions (see Section Learning engagement in MOOCs). When the three reviewers had different opinions, a final agreement would be reached through negotiation.
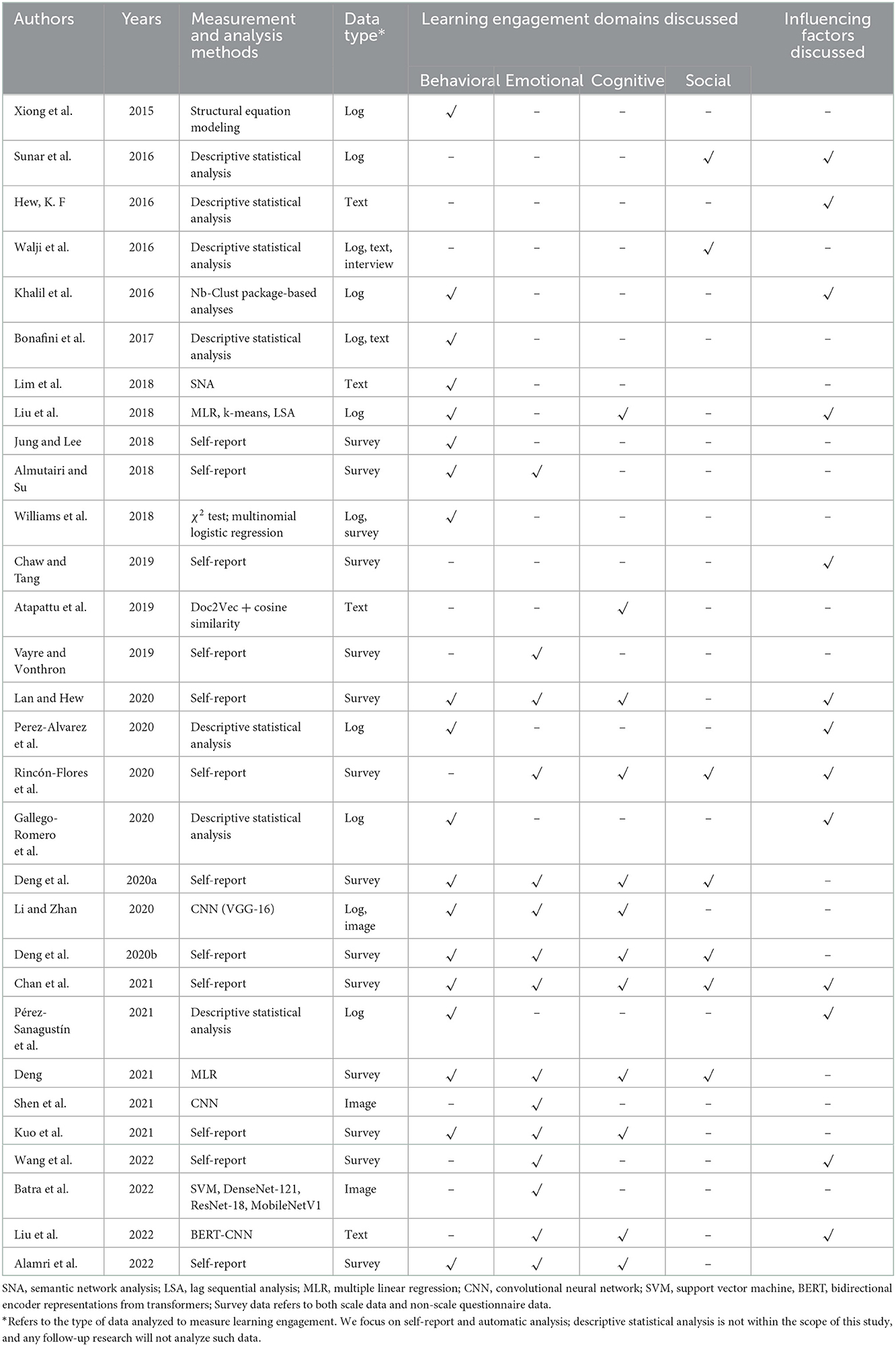
Table 2. Overview of the studies of learning engagement and influencing factors included in our review.
Results
RQ1: What data are analyzed to measure learning engagement in MOOCs?
Of the 30 articles analyzed in this review, only 28 measured learning engagement and clearly delineated the measurement methods: one study did not report the learning engagement data types or analysis methods (Chaw and Tang, 2019), and another measured learning engagement based on a literature review (Hew, 2016). However, as both of those studies identified factors that influence learning engagement in MOOCs through empirical research, they were included in the final analysis (Table 2).
Behavioral engagement
To measure behavioral engagement in MOOCs, the reviewed studies mainly analyzed pre-class planning, course learning, and after-class activities. Sample items are shown in Table 3. In Tables 3–6, the sentences in the “Examples” column which are enclosed in quotation marks represent actual text extracted from the studies in the “References” column, or express similar meanings to those studies.
Emotional engagement
In some of the reviewed studies, the researchers stated that assessing students' overall attitudes toward in-class learning is necessary, while others aimed to closely examine students' views on curriculum content (i.e., knowledge, tasks, and assignments). Finally, some of the studies measured students' emotional experience during classes, rather than relying on self-report measures obtained thereafter (Table 4).
Cognitive engagement
Repeated learning according to the course plan was a focus of some of the studies measuring cognitive engagement. In addition, efforts that go beyond the course plan were regarded by some researchers as indicative of high-level cognitive engagement (Table 5).
Social engagement
There are two critical points to consider in the measurement of social engagement: the types of interactions that students have with others in or after classes pertaining to the knowledge acquired in MOOCs, and the associated emotional experience (Table 6).
Figure 3 shows the number of items used to assess the four dimensions of learning engagement. The total number of items exceeds 28 because many studies involved more than one of the various aspects of engagement (behavioral, cognitive, emotional, and social). Moreover, there were significantly more studies on behavioral, cognitive, and emotional engagement than social engagement; we explain the reasons for this in the Section Discussion.
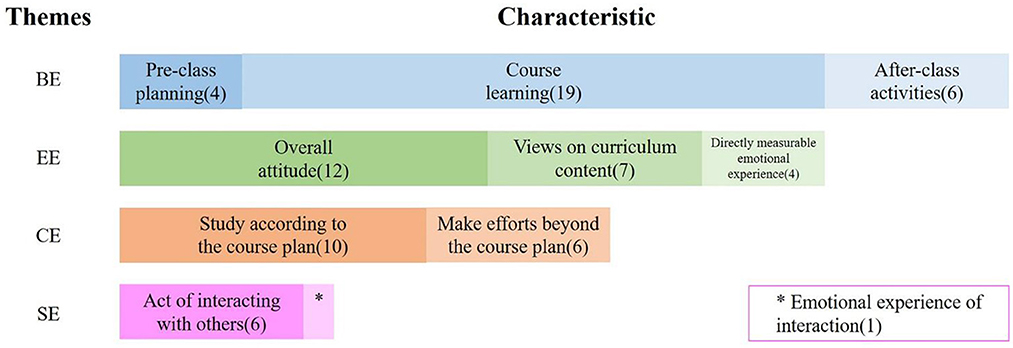
Figure 3. Numbers of items used to measure aspects of learning engagement in massive open online courses.
RQ2: What analysis methods are used to quantify learning engagement in MOOCs?
There are two main methods for measuring and analyzing learning engagement in MOOCs: self-report and automatic analysis (Tables 7, 8, respectively).
Self-report
The most commonly used method for measuring learning engagement in MOOCs is self-report, and the most widely used self-report scales are the Online Learning Engagement Scale (OLE), Online Student Engagement Questionnaire (OSE), and MOOC Engagement Scale (MES) (Table 9).
The strengths and limitations of the scales listed in Table 9 are presented in Table 10.
Automatic analysis
Automatic analysis of learning engagement involves many algorithms, such as K-means clustering, lag sequential analysis (LSA), semantic network analysis (SNA), support vector machine (SVM), convolutional neural network (CNN), bidirectional encoder representations from transformers (BERT)-CNN, etc. These algorithms can be applied for feature analysis, classification, calculation, and regression analysis of learning engagement (Table 11).
Feature analysis of learning engagement
Feature analysis of learning engagement involves exploring and analyzing the features of learning engagement, for example via LSA, SNA, and K-means clustering algorithms. Clustering allows us to understand the learning behavior of learners exhibiting different levels of engagement. Liu et al. (2018) applied K-means clustering to video recordings of MOOC events to categorize students according to learning engagement. To understand user engagement in MOOCs, this study employed LSA to identify the behavioral patterns of students who passed and failed their MOOCs. Khalil and Ebner (2016) used the Nb-Clust package to cluster university students into four categories: “dropouts,” “perfect students,” “gaming the system,” and “social.” They then made different recommendations for these various categories of students. Lim et al. (2018) measured associations between MOOC transcription and forum text data, and conducted a correlation analysis between the semantic network metrics and student performance to determine the impact of student engagement on course performance.
Classification of learning engagement
Classification of learning engagement can be used to measure learners' engagement. This method usually divides learning engagement into several categories. For example, Shen et al. (2021) proposed a new facial expression recognition method based on a CNN using domain adaptation; their network can recognize the four most common facial expressions (understanding, neutral, disgust, and doubt). Then, they applied a formula to classify students according to learning engagement (high, moderate, or low). Their results showed the effectiveness of the proposed method for assessing learning engagement in real time, indicating that it could also be suitable for MOOCs. Batra et al. (2022) suggested that screenshots of videos can shed light on student engagement. They used the WACV dataset, which divides students into three categories: disengaged, partially engaged, and engaged. They used CNN and SVM methods, among others; deep learning algorithms including a densely connected convolutional network (DenseNet-121), residual network (ResNet-18), and MobileNetV1 were used for training the models and enhancing accuracy, with final classification accuracies of 78, 80, and 66%, respectively.
Quantification of learning engagement
Some researchers process data using algorithms that output specific values. This allows for more direct and objective quantification of learning engagement. For example, Liu et al. (2022) constructed a BERT-CNN model to process learners' forum text data; the model output scores for cognitive and emotional engagement. The results showed that the BERT-CNN outperformed other base models, and would be well-suited for processing MOOC text data. Atapattu et al. (2019) used a neural word-embedding (Doc2Vec) language model and cosine similarity to measure learners' cognitive engagement in MOOCs based on a dataset of online community posts and course materials. The results demonstrated that cognitive engagement was influenced by the nature of the MOOC task. Finally, Li and Zhan (2020) proposed a convolution neural network model (VGG-16) to analyze learning engagement using infrared images and log data. Strong agreement between the model results and a traditional online scale of student engagement was seen. However, their method requires temporal contiguity between the two data types.
Regression analysis of learning engagement
To explore the relationship between learning engagement and other factors, some studies conducted a regression analysis, which can be used to examine the relationship between dependent and independent variables. Liu et al. (2018) and Williams et al. (2018) both used multiple regression analysis to determine whether students' learning engagement is affected by discipline, sex, education level, age, and learner goals. Both studies found that discipline and age predicted engagement. Meanwhile, Deng (2021) applied multiple linear regression (MLR) analysis to explore the relationship between learner satisfaction with MOOCs and learning engagement based on data such as video view counts and the number and content of online posts. Behavioral, cognitive, and emotional engagement, but not social engagement, were significant predictors of satisfaction.
RQ3: What factors influence learning engagement in MOOCs?
Aiming to enhance learners' learning engagement in MOOCs, it is also crucial to explore the factors that influence learning engagement. By analyzing existing research, we identified internal and external factors affecting learning engagement (Table 13). Internal factors refer to the innate attributes of learners, these attributes are usually stable, and some of them will alter under the influence of external conditions, such as learners' emotions, attitudes, knowledge levels, and cognitive abilities. While external factors refer to the elements of the course that are not related to the attributes of the learners themselves, such as design, challenges, use of technology, etc.
Internal factors
After reviewing the literature, we identified five internal factors: (1) learning satisfaction, (2) perceived competence, autonomy, and sense of relevance (self-determination theory; SDT), (3) academic motivation and emotions, (4) academic achievement and prior knowledge, and (5) self-regulated learning (SRL).
Learning satisfaction
Chan et al. (2021) found that improving learning satisfaction was key to enhancing students' learning engagement in specific online learning courses. MOOC learning satisfaction is also affected by interactive and discussion-based activities, via the effects of such activities on learning engagement. For example, Dixson (2015) found that interactions and conversations with peers can help students fill gaps in their knowledge, promote satisfaction, and encourage greater participation in MOOCs.
Perceived competence, autonomy, and sense of relevance (SDT)
Lan and Hew (2020) found that all components of the SDT model had significant effects on behavioral, emotional, and cognitive engagement. Perceived ability had the most significant positive impact on all types of engagement, followed by perceived autonomy.
Academic motivation and emotion
Chaw and Tang (2019) revealed that negative and positive motivation promoted passive and active engagement in learners, respectively. In addition, Liu et al. (2022) found that both positive and “confusing” emotions correlated with higher levels of cognitive engagement; the opposite was seen for negative emotions.
In summary, each algorithm has its strengths and limitations (see Table 12), which should be used in specific contexts according to data type and purpose.
Academic achievement and prior knowledge
In addition to learning engagement, some studies used students' academic performance as an independent variable when exploring MOOC performance and engagement. For example, Pérez-Sanagustín et al. (2021) found that students with moderate grade point averages (GPAs) were more engaged with course curricula than those with relatively low or high GPAs.
Self-regulated learning
Some studies have shown that SRL directly impacts learners' activities in the context of MOOCs. For example, Pérez-Sanagustín et al. (2021) found that compared with a group without SRL scaffolding, a group with scaffolding was significantly more engaged, and showed more accurate and strategic learning. Pérez-Álvarez et al. (2020) found that learners' final outcomes were positively correlated with the use of self-reflection-based SRL strategies; such strategies allow learners to be more engaged with the curriculum.
External factors
External factors refer to elements of the curriculum such as design, challenges, use of technology, etc. We identified four external factors: (1) interaction with teachers, peers, and course content, (2) curriculum design and structure, (3) challenges, certificates, medals, etc., and (4) technical support.
Interaction with teachers, peers, and course content
Similar to the traditional classroom, interactions with teachers and peers promote engagement in MOOCs. Tseng (2021) found that teacher notes enhanced students' behavioral and cognitive engagement, while Wang et al. (2022) demonstrated that learner-content and learner-learner interactions predicted online learning engagement by enhancing enjoyment and reducing boredom.
Curriculum design and organizational structure
Gallego-Romero et al. (2020) listed some interventions that can improve learners' engagement in the curriculum: (1) providing step-by-step activities to simplify the learning process; (2) promoting a “growth” mindset among learners; (3) providing questions to be discussed in online forums and encouraging learners to contribute (Sunar et al., 2016); and (4) implementing innovative learning activities that extend beyond the MOOC itself (Hew, 2016).
Challenges, certificates, and medals
Khalil and Ebner (2016) reported that the use of grades, certificates, or badges encourages students to make progress and achieve better learning outcomes. Meanwhile, Rincón-Flores et al. (2020) found that the use of game-based challenges constituted an innovative strategy to evaluate the effectiveness of gamification as a teaching method for MOOCs.
Technical support (scaffolding)
Active learning in MOOCs can be promoted by the use of external tools. For example, Gallego-Romero et al. (2020) used the integrated development environment (IDE) to explore the impact on learners' engagement and behavior of third-party web-based code integrated into three MOOCs on Java programming: learners registered with the third-party “code board” were more engaged, spent more time writing code, and made more changes to the basic code.
Discussion
Addressing research questions
Measurement of learning engagement in MOOCs
Behavioral engagement is often regarded as analogous to learning engagement in studies measuring the latter (Williams et al., 2018; Gallego-Romero et al., 2020; Pérez-Sanagustín et al., 2021). Behavior is the most intuitive measure of the degree of learner engagement, and data thereon (such as the number of videos watched and exams taken) are very easy to obtain. This may explain why behavioral engagement is the most studied form of learning engagement. Many studies divided learning engagement into behavioral, emotional, and cognitive subtypes (Jung and Lee, 2018; Liu et al., 2018; Lan and Hew, 2020), social engagement is a less frequently used subtype of engagement. Since plenty of researchers have directly followed this way of defining the concept of learning engagement in their research, social engagement is the least frequently measured engagement dimension.
Whether learning engagement only subsumes behavioral, emotional, and cognitive engagement (Kuo et al., 2021), or should also include social engagement (Deng et al., 2020a), is debated. It has been suggested that peer learning should be classified into behavioral (Almutairi and White, 2018), cognitive (Liu et al., 2022), and social engagement subtypes (Walji et al., 2016; Deng, 2021). Attempts have been made to refine the concept of learning engagement by reference to specific categories (Vayre and Vonthron, 2019).
Figure 3 shows the main types of learning engagement identified in this literature review. Most researchers (Xiong et al., 2015; Khalil and Ebner, 2016; Lan and Hew, 2020) believe that measures of behavioral engagement should focus on students' behavior in the context of curriculum learning. However, learning plans devised by students before class, and efforts made to complete homework, notes, and after-class tests, have gradually emerged as more important indices of behavioral engagement (Deng et al., 2020a; Kuo et al., 2021). These findings can serve as a reference for researchers aiming to accurately quantify learning engagement (see Table 3).
Methods used for measuring and analyzing learning engagement in MOOCs
Two methods are used to measure learning engagement. Learning engagement in MOOCs is still mainly quantified via self-reported methods. It is typically measured using scales applied in traditional teaching; research has involved middle school and college students. Furthermore, some scales were not designed to address the widely recognized behavioral, cognitive, emotional, and social subtypes of engagement, and scales specifically focused on online learning or MOOCs have not been widely applied.
The second way to measure learning engagement is through automatic analysis. In terms of data, log, text, and image data are needed for automatic analysis. Log data can shed light on learning engagement if subjected to clustering analysis. For text data, SNA, BERT-CNN, Doc2Vec, and cosine similarity can be applied for data processing and analysis. Furthermore, using text data to train BERT-CNN models, semantic features can be identified to analyze learning engagement subtypes. Doc2Vec and cosine similarity are used to calculate semantic similarity, and the strength of correlations provides insight into the degree of learning engagement. As for image data, we can get information on students' emotional engagement through image emotion recognition.
In terms of algorithms applied, which can be divided into machine learning methods or deep learning methods. SVM is a typical machine learning classification algorithm that needs to extract features. Using SVM, researchers can analyze text, log or image data to acquire engagement classification. In addition, K-means is an excellent algorithm that can carry out cluster analysis on students' learning behavior to distinguish groups with different levels of learning engagement, which is convenient for instructors to carry out classified teaching later. Furthermore, CNN, DenseNet-121, ResNet-18, and MobileNetV1 deep learning algorithms have also been successfully applied to process image data. These methods are fast and highly accurate, but the interpretability of the output is low. In addition to these algorithms, MLR allows for determining the variables influencing learning engagement and the effect of it on other variables, such as learning satisfaction and academic achievement.
However, existing methods for analyzing learning engagement have several limitations, as follows: (1) poor ability to combine all types of data used for measuring and analyzing learning engagement; (2) self-report and automatic analysis methods are usually not applied in real-time; (3) the granularity of learning engagement assessments has not been optimized; and (4) primarily focused on behavioral and emotional engagement, with less attention paid to cognitive engagement. Further study needs to explore a comprehensive approach to analyze and measure multimodal data (such as text, log, image, and voice data) for a more precise evaluation of learning engagement. And researchers can also attempt to detect students' learning engagement in real-time through log data, video image data, etc., and give feedback to learners to help them learn. Furthermore, further studies on measuring learning engagement should go deeper. For example, researchers can break down negative emotions into anxiety, tension, depression, sadness, etc. In this way, emotional engagement can be precisely analyzed. Finally, researchers should pay more attention to mining students' cognitive engagement from existing data.
Factors affecting learning engagement in MOOCs
Factors affecting learning engagement in MOOCs can be classified as internal or external, as stated above. Regarding internal factors, this study demonstrated that students' learning satisfaction and motivation could affect learning engagement, consistent with Sahin and Shelley (2008); a high level of satisfaction can motivate students to persist with their studies and improves learning engagement. Learning satisfaction is an important indicator that can influence students' MOOCs learning. When students are more satisfied with the structural design, learning experience, and learning outcomes of the course, they are more likely to be spontaneously engaged in MOOCs learning. Also, we observed a correlation between negative emotions and cognitive engagement, consistent with Obergriesser and Stoeger (2020) reducing the former enhances the latter to some extent. Negative emotions often affect students' learning status, and when they are depressed, it is difficult for students to concentrate, let alone engage in high levels of cognitive activity. Finally, SRL is a vital concept when exploring the impact of curriculum design; this aligns with Littlejohn et al. (2016), who showed that learners with higher SRL proficiency tend to be more engaged in activities and materials related to their needs or interests. This is because learners with higher levels of SRL can often rationalize and manage their learning time and effort, develop a learning plan that suits their needs, and carry out learning activities accordingly. In this way, they tend to be more engaged in the course because they know exactly what they want to learn and how to achieve it through their learning.
Regarding external factors, we found that learner feedback and well-designed activities enhance engagement; this is consistent with Choy and Quek (2016), who found that student engagement depends on the discussions between lecturers and students, as well as the learning environment and course structure. On the one hand, a well-structured and logical design of course activities can attract students to participate in the course activities and make them receive a more systematic knowledge construction process, thus increasing their behavioral and cognitive engagement to a certain level; on the other hand, interaction and feedback with peers and instructors can allow students to view problems from different perspectives and gain a sense of recognition and satisfaction from interacting with others, thus increasing their social and emotional engagement. In addition to the non-directive incentives of well-designed activities and peer interaction, the direct incentives of challenges, certificates (Radford et al., 2014), and technical support (Bond et al., 2020) can also be a good way to enhance student engagement in courses. First, appropriate challenges can stimulate learners' interest in learning and attract them to invest more effort in the course. Second, learners' need for course certificates also motivates learners to engage in course activities. Finally, when learners encounter challenges or difficulties, practical technical support can serve as a valuable scaffolding to help learners apply what they have learned in practice and thus increase their learning engagement.
By reviewing the existing literature, we found that most of the existing studies explore the factors affecting students' learning engagement separately from both internal and external perspectives. There are relatively few studies that combine internal and external factors to analyze how to enhance learning engagement. As Bond et al. argue, the use of advanced technologies of the 21st century alone does not guarantee the desired learning outcomes (Bond et al., 2020), and it is necessary to ensure students' learning motivation and initiative while improving the technical means of MOOC platform development.
Pedagogical implications
This systematic literature review provides pedagogical implications from two perspectives to assist MOOC designers in designing and developing MOOCs activities and help instructors monitor students' learning process. From the perspective of course design, MOOC designers should pay more attention to students' learning satisfaction. For example, a link to a survey on learning satisfaction could be provided after each class to obtain real-time data related to the learning experience. This would enable instructors to promptly focus on students with low learning satisfaction and solicit suggestions for course improvement, thereby increasing student engagement and thus helping them to improve their academic performance. In addition, because it is challenging to change student characteristics significantly, MOOC designers can pay close attention to designing better instructional activities for the course. For example, it is possible to improve students' behavior and social engagement by setting more forum discussion tasks or using incentives such as medals, rankings, and certificates; it is possible to enhance students' emotional engagement by uploading vivid and interesting micro-videos; it is possible to promote cognitive engagement among students by assigning tasks such as note-taking and quizzes.
From the perspective of learning processing, designers and instructors can use algorithms to analyze students learning engagement so that they can identify whether there are some students out of good learning. For instance, (1) they can classify different students of learning engagement by k-means clustering to provide personalized instruction to students better; (2) they can directly quantify students' learning engagement to find individuals with low learning engagement (such as not completing course assignments, not participating in forum discussions and not watching course videos), giving supervision and warning; (3) they can improve their course content and activities according to overall students' learning engagement level. Moreover, during the learning process, instructors can post announcements and messages to remind students to take the course on time, helping facilitate students' behavioral engagement. At the same time, it is a good chance for them to interact with students in the discussion forum, which can enhance students' social engagement.
Conclusion
Thirty articles were included in our literature review, which explored learning engagement data and analysis methods, and summarized the internal and external factors influencing engagement in MOOCs. Four dimensions of learning engagement in MOOCs were identified. For example, behavioral engagement is reflected in observable actions such as after-class activities. Additionally, log, text, image, interview, and survey data can all be collected and subjected to self-report and automatic analysis methods (e.g., CNN, BERT-CNN, K-MEANS, SNA, etc.). This study also found that internal and external factors affect learning engagement, which could guide MOOC designers and teachers. Learning engagement is an excellent indicator of the learning condition. Based on this, designers and teachers can carry out more personalized learning support for different students and reflect their course design. However, this systematic literature review also had some limitations. First, the study selection criteria precluded the inclusion of literature published in certain languages, as well as conference papers. Moreover, we only searched five databases and thus may have missed some relevant articles. Therefore, future research should expand the search scope to obtain more exhaustive information.
Data availability statement
The original contributions presented in the study are included in the article/supplementary material, further inquiries can be directed to the corresponding author.
Author contributions
The idea for the article: RW. Literature search: RW and JC. Data coding: JC and YX. Drafted and revised the work: RW, YX, and JC. Final check: YL. All authors contributed to the article and approved the submitted version.
Funding
This paper was supported by the Beijing Natural Science Foundation (Grant No: 9222019).
Acknowledgments
We thank Michael Irvine, PhD, from Liwen Bianji (Edanz) (www.liwenbianji.cn) for editing the English text of a draft of this manuscript.
Conflict of interest
The authors declare that the research was conducted in the absence of any commercial or financial relationships that could be construed as a potential conflict of interest.
Publisher's note
All claims expressed in this article are solely those of the authors and do not necessarily represent those of their affiliated organizations, or those of the publisher, the editors and the reviewers. Any product that may be evaluated in this article, or claim that may be made by its manufacturer, is not guaranteed or endorsed by the publisher.
References
Alamri, M. M. (2022). Investigating students' adoption of MOOCs during COVID-19 pandemic: students' academic self-efficacy, learning engagement, and learning persistence. Sustainability 14, 714. doi: 10.3390/su14020714
Alemayehu, L., and Chen, H. L. (2021). Learner and instructor-related challenges for learners' engagement in MOOCs: a review of 2014–2020 publications in selected SSCI indexed journals. Interact. Learn. Environ. 636, 1–23. doi: 10.1080/10494820.2021.1920430
Almutairi, F., and White, S. (2018). How to measure student engagement in the context of blended MOOC. Interact. Technol. Smart Educ. 15, 262–278. doi: 10.1108/ITSE-07-2018-0046
Atapattu, T., Thilakaratne, M., Vivian, R., and Falkner, K. (2019). Detecting cognitive engagement using word embeddings within an online teacher professional development community. Comput. Educ. 140, 103594. doi: 10.1016/j.compedu.2019.05.020
Atiaja, L., and Proenza, R. (2016). The MOOCs: origin, characterization, principal problems and challenges in higher education. J. e-Learn. Knowl. Soc. 12, 171428. Retrieved from: https://www.learntechlib.org/p/171428/
Barak, M., Watted, A., and Haick, H. (2016). Motivation to learn in massive open online courses: examining aspects of language and social engagement. Comput. Educ. 94, 49–60. doi: 10.1016/j.compedu.2015.11.010
Batra, S., Wang, H., Nag, A., Brodeur, P., Checkley, M., Klinkert, A., et al. (2022). DMCNet: diversified model combination network for understanding engagement from video screengrabs. Syst. Soft Comput. 4, 200039. doi: 10.1016/j.sasc.2022.200039
Bezerra, L. N., and Silva, M. T. (2017). A review of literature on the reasons that cause the high dropout rates in MOOCs. Rev. Espacios 38, 1–23. Available online at: http://www.revistaespacios.com/a17v38n05/a17v38n05p11.pdf
Bingham, G. E., and Okagaki, L. (2012). “Ethnicity and student engagement,” in Handbook of Research on Student Engagement (Boston: Springer), 65–95. doi: 10.1007/978-1-4614-2018-7_4
Bolliger, D. U., Supanakorn, S., and Boggs, C. (2010). Impact of podcasting on student motivation in the online learning environment. Comput. Educ. 55, 714–722. doi: 10.1016/j.compedu.2010.03.004
Bonafini, F. C., Chae, C., Park, E., and Jablokow, K. W. (2017). How much does student engagement with videos and forums in a MOOC affect their achievement? Online Learn. 21, 1270. doi: 10.24059/olj.v21i4.1270
Bond, M., Buntins, K., Bedenlier, S., Zawacki-Richter, O., and Kerres, M. (2020). Mapping research in student engagement and educational technology in higher education: a systematic evidence map. Int. J. Educ. Technol. Higher Educ. 17, 1–30. doi: 10.1186/s41239-019-0176-8
Chan, S., Lin, C., Chau, P., Takemura, N., and Fung, J. (2021). Evaluating online learning engagement of nursing students. Nurse Educ. Today 104, 104985. doi: 10.1016/j.nedt.2021.104985
Chaw, L. Y., and Tang, C. M. (2019). Driving high inclination to complete massive open online courses (MOOCs): motivation and engagement factors for learners. Elect. J. E-Learn. 17, 118–130. doi: 10.34190/JEL.17.2.05
Choy, J. L. F., and Quek, C. L. (2016). Modelling relationships between students' academic achievement and community of inquiry in an online learning environment for a blended course. Aust. J. Educ. Technol. 32, 2500. doi: 10.14742/ajet.2500
De Barba, P., Kennedy, G., and Ainley, M. (2016). The role of students' motivation and participation in predicting performance in a MOOC. J. Comput. Assist. Learn. 32, 218–231. doi: 10.1111/jcal.12130
Deng, R. (2021). Emotionally engaged learners are more satisfied with online courses. Sustainability 13, 11169. doi: 10.3390/su132011169
Deng, R., Benckendorff, P., and Gannaway, D. (2019). Progress and new directions for teaching and learning in MOOCs. Comput. Educ. 129, 48–60. doi: 10.1016/j.compedu.2018.10.019
Deng, R., Benckendorff, P., and Gannaway, D. (2020a). Learner engagement in MOOCs: scale development and validation. Br. J. Educ. Technol. 51, 245–262. doi: 10.1111/bjet.12810
Deng, R., Benckendorff, P., and Gannaway, D. (2020b). Linking learner factors, teaching context, and engagement patterns with MOOC learning outcomes. J. Comput. Assist. Learn. 36, 688–708. doi: 10.1111/jcal.12437
Dixson, M. D. (2015). Measuring student engagement in the online course: the online student engagement scale (OSE). Online Learn. 19, 561. doi: 10.24059/olj.v19i4.561
Fisher, R., Perényi, R., and Birdthistle, N. (2018). The positive relationship between flipped and blended learning and student engagement, performance and satisfaction. Act. Learn. Higher Educ. 22, 97–113. doi: 10.1177/1469787418801702
Fredricks, J. A., Blumenfeld, P. C., and Paris, A. H. (2004). School engagement: potential of the concept, state of the evidence. Rev. Educ. Res. 74, 59–109. doi: 10.3102/00346543074001059
Gallego-Romero, J. M., Alario-Hoyos, C., Estévez-Ayres, I., and Delgado Kloos, C. (2020). Analyzing learners' engagement and behavior in MOOCs on programming with the Codeboard IDE. Educ. Technol. Res. Dev. 68, 2505–2528. doi: 10.1007/s11423-020-09773-6
Hew, K. F. (2016). Promoting engagement in online courses: what strategies can we learn from three highly rated MOOCS. Br. J. Educ. Technol. 47, 320–341. doi: 10.1111/bjet.12235
Hone, K. S., and El Said, G. R. (2016). Exploring the factors affecting MOOC retention: a survey study. Comput. Educ. 98, 157–168. doi: 10.1016/j.compedu.2016.03.016
Jimerson, S. R., Campos, E., and Greif, J. L. (2003). Toward an understanding of definitions and measures of school engagement and related terms. Calif. School Psychol. 8, 7–27. doi: 10.1007/BF03340893
Jordan, K. (2014). Initial trends in enrolment and completion of massive open online courses. Int. Rev. Res. Open Dist. Learn. 15, 1651. doi: 10.19173/irrodl.v15i1.1651
Jung, Y., and Lee, J. (2018). Learning engagement and persistence in massive open online courses (MOOCS). Comput. Educ. 122, 9–22. doi: 10.1016/j.compedu.2018.02.013
Khalil, M., and Ebner, M. (2016). Clustering patterns of engagement in Massive Open Online Courses (MOOCs): the use of learning analytics to reveal student categories. J. Comput. Higher Educ. 29, 114–132. doi: 10.1007/s12528-016-9126-9
Kizilcec, R. F., Reich, J., Yeomans, M., Dann, C., Brunskill, E., Lopez, G., et al. (2020). Scaling up behavioral science interventions in online education. Proc. Natl. Acad. Sci. 117, 14900–14905. doi: 10.1073/pnas.1921417117
Kop, R. (2011). The challenges to connectivist learning on open online networks: learning experiences during a massive open online course. Int. Rev. Res. Open Dist. Learn. 12, 19. doi: 10.19173/irrodl.v12i3.882
Krause, K., and Coates, H. (2008). Students' engagement in first-year university. Assess. Eval. Higher Educ. 33, 493–505. doi: 10.1080/02602930701698892
Kuo, T. M., Tsai, C. C., and Wang, J. C. (2021). Linking web-based learning self-efficacy and learning engagement in MOOCs: the role of online academic hardiness. Internet Higher Educ. 51, 100819. doi: 10.1016/j.iheduc.2021.100819
Lan, M., and Hew, K. F. (2020). Examining learning engagement in MOOCs: a self-determination theoretical perspective using mixed method. Int. J. Educ. Technol. Higher Educ. 17, 1–24. doi: 10.1186/s41239-020-0179-5
Lazarus, F. C., and Suryasen, R. (2022). The quality of higher education through MOOC penetration and the role of academic libraries. Insights 35, 9. doi: 10.1629/uksg.577
Li, K., and Moore, D. R. (2018). Motivating students in massive open online courses (MOOCs) using the attention, relevance, confidence, satisfaction (ARCS) model. J. Format. Des. Learn. 2, 102–113. doi: 10.1007/s41686-018-0021-9
Li, Z., and Zhan, Z. (2020). Integrated infrared imaging techniques and multi-model information via convolution neural network for learning engagement evaluation. Infrared Phys. Technol. 109, 103430. doi: 10.1016/j.infrared.2020.103430
Lim, S., Tucker, C. S., Jablokow, K., and Pursel, B. (2018). A semantic network model for measuring engagement and performance in online learning platforms. Comput. Appl. Eng. Educ. 26, 1481–1492. doi: 10.1002/cae.22033
Littlejohn, A., Hood, N., Milligan, C., and Mustain, P. (2016). Learning in MOOCs: motivations and self-regulated learning in MOOCs. Internet Higher Educ. 29, 40–48. doi: 10.1016/j.iheduc.2015.12.003
Liu, C., Zou, D., Chen, X., Xie, H., and Chan, W. H. (2021). A bibliometric review on latent topics and trends of the empirical MOOC literature (2008–2019). Asia Pacif. Educ. Rev. 22, 515–534. doi: 10.1007/s12564-021-09692-y
Liu, M. C., Yu, C. H., Wu, J., Liu, A. C., and Chen, H. M. (2018). Applying learning analytics to deconstruct user engagement by using log data of MOOCs. J. Inform. Sci. Eng. 34, 1175–1186. Available online at: https://tpl.ncl.edu.tw/NclService/pdfdownload?filePath=lV8OirTfsslWcCxIpLbUfmhaNcSH2c_7jIYuWMRvIdhWGksqk5KLY2qwzv7Qnu00&imgType=Bn5sH4BGpJw=&key=ybfNQTSfQ9D4qNe3TvC_d9XlYqzRwG60eLoh1WmA1
Liu, S., Liu, S., Liu, Z., Peng, X., and Yang, Z. (2022). Automated detection of emotional and cognitive engagement in MOOC discussions to predict learning achievement. Comput. Educ. 181, 104461. doi: 10.1016/j.compedu.2022.104461
Luik, P., and Lepp, M. (2021). Are highly motivated learners more likely to complete a computer programming MOOC? Int. Rev. Res. Open Dist. Learn. 22, 41–58. doi: 10.19173/irrodl.v22i1.4978
Obergriesser, S., and Stoeger, H. (2020). Students' emotions of enjoyment and boredom and their use of cognitive learning strategies: how do they affect one another? Learn. Instruct. 66, 101285. doi: 10.1016/j.learninstruc.2019.101285
Page, M. J., McKenzie, J. E., Bossuyt, P. M., Boutron, I., Hoffmann, T. C., Mulrow, C. D., et al. (2021b). The PRISMA 2020 statement: an updated guideline for reporting systematic reviews. Int. J. Surg. 88, 105906. doi: 10.1016/j.ijsu.2021.105906
Page, M. J., Moher, D., Bossuyt, P. M., Boutron, I., Hoffmann, T. C., Mulrow, C. D., et al. (2021a). PRISMA 2020 explanation and elaboration: updated guidance and exemplars for reporting systematic reviews. BMJ 372, n160. doi: 10.1136/bmj.n160
Paton, R. M., Fluck, A. E., and Scanlan, J. D. (2018). Engagement and retention in VET MOOCs and online courses: a systematic review of literature from 2013 to 2017. Comput. Educ. 125, 191–201. doi: 10.1016/j.compedu.2018.06.013
Pérez-Álvarez, R. A., Maldonado-Mahauad, J., Sharma, K., Sapunar-Opazo, D., and Pérez-Sanagustín, M. (2020). Characterizing learners' engagement in MOOCs: an observational case study using the NoteMyProgress tool for supporting self-regulation. IEEE Trans. Learn. Technol. 13, 676–688. doi: 10.1109/TLT.2020.3003220
Pérez-Sanagustín, M., Sapunar-Opazo, D., Pérez-Álvarez, R., Hilliger, I., Bey, A., Maldonado-Mahauad, J., et al. (2021). A MOOC-based flipped experience: scaffolding SRL strategies improves learners' time management and engagement. Comput. Appl. Eng. Educ. 29, 750–768. doi: 10.1002/cae.22337
Pursel, Zhang, L., Jablokow, K., Choi, G., and Velegol, D. (2016). Understanding MOOC students: motivations and behaviours indicative of MOOC completion. J. Comput. Assist. Learn. 32, 202–217. doi: 10.1111/jcal.12131
Radford, A. W., Robles, J., Cataylo, S., Horn, L., Thornton, J., and Whitfield, K. E. (2014). The employer potential of MOOCs: a mixed-methods study of human resource professionals' thinking on MOOCs. Int. Rev. Res. Open Dist. Learn. 15, 1842. doi: 10.19173/irrodl.v15i5.1842
Reich, J., and Ruipérez-Valiente, J. A. (2019). The MOOC pivot. Science 363, 130–131. doi: 10.1126/science.aav7958
Rincón-Flores, E. G., Mena, J., and Montoya, M. S. R. (2020). Gamification: a new key for enhancing engagement in MOOCs on energy? Int. J. Interact. Des. Manuf. 14, 1379–1393. doi: 10.1007/s12008-020-00701-9
Sahin, I., and Shelley, M. (2008). Considering students' perceptions: the distance education student satisfaction model. Educ. Technol. Soc. 11, 216–223. Available online at: https://www.jstor.org/stable/10.2307/jeductechsoci.11.3.216
Shen, J., Yang, H., Li, J., and Cheng, Z. (2021). Assessing learning engagement based on facial expression recognition in MOOC's scenario. Multimedia Syst. 28, 469–478. doi: 10.1007/s00530-021-00854-x
Sun, J. C. Y., and Rueda, R. (2011). Situational interest, computer self-efficacy and self-regulation: their impact on student engagement in distance education. Br. J. Educ. Technol. 43, 191–204. doi: 10.1111/j.1467-8535.2010.01157.x
Sunar, A. S., White, S., Abdullah, N. A., and Davis, H. C. (2016). How learners' interactions sustain engagement: a MOOC case study. IEEE Trans. Learn. Technol. 10, 475–487. doi: 10.1109/TLT.2016.2633268
Tseng, S. F., Tsao, Y. W., Yu, L. C., Chan, C. L., and Lai, K. R. (2016). Who will pass? Analyzing learner behaviors in MOOCs. Res. Pract. Technol. Enhanc. Learn. 11, 1–11. doi: 10.1186/s41039-016-0033-5
Tseng, S. S. (2021). The influence of teacher annotations on student learning engagement and video watching behaviors. Int. J. Educ. Technol. Higher Educ. 18, 1–17. doi: 10.1186/s41239-021-00242-5
Vayre, E., and Vonthron, A. M. (2019). Relational and psychological factors affecting exam participation and student achievement in online college courses. Internet Higher Educ. 43, 100671. doi: 10.1016/j.iheduc.2018.07.001
Veletsianos, G., Collier, A., and Schneider, E. (2015). Digging deeper into learners' experiences in MOOCs: participation in social networks outside of MOOCs, notetaking and contexts surrounding content consumption. Br. J. Educ. Technol. 46, 570–587. doi: 10.1111/bjet.12297
Walji, S., Deacon, A., Small, J., and Czerniewicz, L. (2016). Learning through engagement: MOOCs as an emergent form of provision. Dist. Educ. 37, 208–223. doi: 10.1080/01587919.2016.1184400
Wang, Y., Cao, Y., Gong, S., Wang, Z., Li, N., and Ai, L. (2022). Interaction and learning engagement in online learning: the mediating roles of online learning self-efficacy and academic emotions. Learn. Individ. Differ. 94, 102128. doi: 10.1016/j.lindif.2022.102128
Williams, K. M., Stafford, R. E., Corliss, S. B., and Reilly, E. D. (2018). Examining student characteristics, goals, and engagement in massive open online courses. Comput. Educ. 126, 433–442. doi: 10.1016/j.compedu.2018.08.014
Xiong, Y., Li, H., Kornhaber, M. L., Suen, H. K., and Goins, D. D. (2015). Examining the relations among student motivation, engagement, and retention in a MOOC: a structural equation modeling approach. Global Educ. Rev. 2, 23–33. Available online at: https://files.eric.ed.gov/fulltext/EJ1074099.pdf
Keywords: learning engagement, MOOCs, measurement methods, analysis methods, influencing factors
Citation: Wang R, Cao J, Xu Y and Li Y (2022) Learning engagement in massive open online courses: A systematic review. Front. Educ. 7:1074435. doi: 10.3389/feduc.2022.1074435
Received: 19 October 2022; Accepted: 02 December 2022;
Published: 20 December 2022.
Edited by:
Yung-Wei Hao, National Taiwan Normal University, TaiwanReviewed by:
Anita Lee-Post, University of Kentucky, United StatesKarla Andrea Lobos, University of Concepcion, Chile
Copyright © 2022 Wang, Cao, Xu and Li. This is an open-access article distributed under the terms of the Creative Commons Attribution License (CC BY). The use, distribution or reproduction in other forums is permitted, provided the original author(s) and the copyright owner(s) are credited and that the original publication in this journal is cited, in accordance with accepted academic practice. No use, distribution or reproduction is permitted which does not comply with these terms.
*Correspondence: Yanyan Li, bGl5eUBibnUuZWR1LmNu