- Department of Biology and Chemistry, Institute of Chemistry Education, Justus Liebig University Giessen, Giessen, Germany
Drawing is a fundamental skill in science, technology, engineering, and mathematics (STEM) disciplines to express one’s reasoning and externalize mental models in problem-solving. Although research has highlighted the effectiveness of drawing as a learning strategy and the importance of drawing accuracy for learning success, little is known about learners’ actual drawing process. However, especially in organic chemistry, the investigation of drawing processes is of great importance as generating different representations, such as structural formulas, is inherent to problem-solving in this visual-laden discipline. Resonance structures, for example, are often used to estimate reactive sites in a molecule and to propose reaction pathways. However, this type of representation places a high cognitive demand on learners, which, besides conceptual difficulties, leads to drawing difficulties. To support learners in drawing and using resonance structures in problem-solving, it is necessary to characterize how they generate their drawings. To this end, a qualitative, exploratory study has been conducted to investigate undergraduate students’ (N = 20) drawing processes of resonance structures while solving an organic case comparison task. Using eye-tracking, the characteristics regarding the construction of productive and unproductive drawings became visible. Results indicate that unproductive drawings often stem from integrating and connecting unrelated information during the drawing process. Further, the results show that the productivity of a drawing depends on learners’ flexibility in information selection. Implications for supporting learners’ drawing process and using eye-tracking for characterizing drawing processes in other STEM disciplines are discussed.
Introduction
In science, technology, engineering, and mathematics (STEM) disciplines, scientists and learners rely heavily on external representations to make sense of scientific concepts and phenomena (Fiorella and Zhang, 2018; Ainsworth and Scheiter, 2021). As representations constitute a fundamental means for the construction and transmission of knowledge, students need to be proficient in analyzing and using given representations (Nitz et al., 2014) and be fluent in generating them (Ainsworth et al., 2011). In fact, by expressing one’s reasoning and externalizing mental models, drawings can help learning new concepts and support problem-solving (Cox, 1999; Ainsworth et al., 2011; Quillin and Thomas, 2015; Cooper et al., 2017; Wu and Rau, 2019). In recent years, much research has been conducted on drawing as a learning activity across different STEM contexts such as chemistry (Hellenbrand et al., 2019), physics (Maries and Singh, 2018), biology (Schmeck et al., 2014), and geography (Gobert and Clement, 1999) at school and college levels. Predominantly, it has been shown that prompting learners to visually depict content presented in text- or animation-based instructional materials is an effective learning strategy as students who draw not only build higher quality explanations and develop a more coherent mental model of a studied phenomenon, but also perform better in subsequent tasks and tests (Bobek and Tversky, 2016; Fiorella and Zhang, 2018; Cromley et al., 2019). Thus, drawing enhances learning outcomes related to retention, comprehension, and knowledge transfer (Van Meter and Garner, 2005; Leopold and Leutner, 2012; Schmeck et al., 2014; Fiorella and Zhang, 2018; Fiorella et al., 2020). However, drawing per se does not automatically enhance learning. By referring to the prognostic drawing principle, Schwamborn et al. (2010) point out that the quality of learners’ drawings is predictive of their learning outcomes, i.e., the more accurately and correctly learners draw, the better their performance. This finding has been replicated in various studies (Mason et al., 2013; Schmeck et al., 2014; Rellensmann et al., 2016; Fiorella and Zhang, 2018; Hellenbrand et al., 2019; Schmidgall et al., 2020; Stieff and DeSutter, 2020).
Despite focusing on the quality of final drawing products and their relation to learning outcomes, the actual drawing process leading to these products has not received much attention in research so far (Lobato et al., 2014). However, drawing constitutes a core scientific practice itself and, consequently, necessitates a profound understanding of how a drawing is sequentially generated and which factors influence its quality. In organic chemistry, for instance, drawing is fundamental to scientific thinking and model-based reasoning as diagrams and structural formulas have a high explanatory power and make imperceptible entities and processes visible (Goodwin, 2008; Cooper et al., 2017; Graulich and Bhattacharyya, 2017). Whether in the laboratory or the lecture, chemists sketch and manipulate molecular structures to explain findings and communicate chemical content (Kozma et al., 2000). Besides this displaying function, drawings of molecular structures are crucial problem-solving tools. Since much chemical information (e.g., connectivity, polarity) is embedded within molecular structures, drawing enables the expression of assumptions or predictions about the properties of molecules or possible reaction processes (Cartrette and Bodner, 2009; Cooper et al., 2017). Constructing resonance structures of organic molecules, for instance, serves as a mean to estimate reactive sites in a molecule by representing multiple variants of the electronic delocalization in a molecule which cannot be adequately represented by a single structure. By considering the hypothetical electronic distribution in a molecular structure (i.e., the contribution of each resonance structure to the dynamic electron density distribution of a molecule), resonance structures enable the prediction of reaction pathways (Richardson, 1986). Therefore, constructing appropriate resonance structures constitutes the first critical step in a chain of steps of inferences to derive structural properties and chemical reactivities (Cooper et al., 2012). Thus, not succeeding in this step hinders students from using the resonance structures adequately in subsequent problem-solving (Strickland et al., 2010; Carle and Flynn, 2020). To support students in the adequate use of the resonance concept and the respective drawing process of resonance structures, it is crucial to understand how students are sequentially generating their drawings and which drawing behavior characterizes the generation of productive, thus valid and significant resonance structures, and unproductive drawings, i.e., wrong or insignificant resonance structures.
A more profound, process-oriented characterization of drawing processes can be achieved by using eye-tracking. Without interfering with the construction process, recording eye movements quantitatively captures students’ visual processing of stimuli (e.g., by providing insights into learners’ attention distribution on structural features or their search behavior in terms of gaze patterns). This can help to draw conclusions about learners’ underlying cognitive processes and different strategic approaches when constructing representations such as molecular structures (Just and Carpenter, 1980; Cullipher et al., 2018). Therefore, using eye-tracking in the context of construction processes in STEM disciplines allows a deeper insight into students’ drawing processes and offers a new perspective on obstacles students encounter when generating representations such as chemical structures.
Prior research on students’ difficulties in the construction of organic molecular structures
Becoming proficient in using symbolic language in organic chemistry, such as drawing mechanisms or using the electron-pushing formalism, has been constantly shown to be difficult for students at various levels (Bodner and Domin, 2000; Graulich, 2015; Flynn and Featherstone, 2017; Dood and Watts, 2022). Several studies report that students depict mechanisms solely based on rote memorization, do not ascribe meaning to the electron-pushing formalism, place electron arrows as decoration instead, and exhibit difficulties in the construction of structural formulas (Bhattacharyya and Bodner, 2005; Cooper et al., 2010; Grove et al., 2012). Lewis structures are typically the first type of representations students encounter in organic chemistry to represent molecular structures as a variety of physical and chemical properties of molecular compounds can be inferred by constructing and inspecting Lewis structures (e.g., physical state, geometry, solubility) (Cooper et al., 2012; Tiettmeyer et al., 2017). In fact, drawing Lewis structures implies a high cognitive load on students, as students must consider various concepts (e.g., expanded octets, geometry) and sets of rules while coping with many exceptions to these very rules at the same time (Cooper et al., 2010; Kaufmann et al., 2017; Tiettmeyer et al., 2017; Karonen et al., 2021). Cooper et al. (2010) intensively investigated students’ ability to construct and manipulate Lewis structures across different learning levels. As a main finding, they demonstrated that students’ and even faculty members’ competence in constructing valid Lewis structures is deficient. Most students struggled with creating valid Lewis structures involving two or more carbon atoms (Cooper et al., 2010). Moreover, it became apparent that the success rate depended on how the formulas were presented to students. While students struggled with drawing a Lewis structure of methanol in the form of CH4O, more students could produce a correct structure if the functional group was depicted explicitly, i.e., as CH3OH (Cooper et al., 2010). Other common errors that students exhibit when drawing Lewis structures encompass the inability to determine the correct number of bonds, the arbitrary assignment of (formal) charges in ions, or the overreliance on rules such as the octet rule (Cooper et al., 2010; Kaufmann et al., 2017; Karonen et al., 2021). Concerning the latter, students were either likely to apply the octet rule when it was not possible to show atoms with full octets or tended to violate the octet rule, e.g., by exceeding the octet or depicting atoms different from carbon (e.g., nitrogen or oxygen atoms) as electron-deficient (Cooper et al., 2010). Some students in Cooper et al.’s (2010) study even invented their own rules and invalid strategies (e.g., reaching the highest symmetry). All this suggests that students tend to rely on memorized, salient cues while drawing, use rules mechanically, or approach drawing tasks by unsystematic trial-and-error strategies (Ahmad and Omar, 1992; Cooper et al., 2010; Kaufmann et al., 2017; Sandi-Urena et al., 2020).
These problems reported for the generation of single Lewis structures also apply to the construction of resonance structures. Resonance structures provide a more accurate way of describing molecules that single Lewis structures cannot accurately display due to the delocalization of π-electron pairs over several atoms. As such, molecules can be best described by drawing multiple structural formulas (e.g., Lewis structures or skeletal formulas) of the same molecule with a varying electron distribution. The combination of these drawings, depending on their contribution to the overall stability of the molecule, ultimately forms the resonance hybrid of the given molecule. Consequently, the properties and reactivities (e.g., charge density and product distribution) of molecules that exhibit resonance lie between the different canonical structures. Although students are expected to have a thorough understanding of this core chemical concept after introductory courses in organic chemistry and should be able to use it fluently to depict the electronic structure of compounds, research in chemistry education has demonstrated that the resonance concept puts a high cognitive load on students, even at the university level (Duis, 2011; Brandfonbrener et al., 2021). This leads to various misconceptions, such as considering resonance structures as equilibrium or electron reservoirs (Taber, 2002; Kim et al., 2019; Xue and Stains, 2020). As the application of this concept requires the integration of different concepts and prior knowledge (e.g., electronegativity, hybridization, electron-pushing formalism), a fragmented conceptualization of these relationships may hinder subsequent problem-solving (Betancourt-Pérez et al., 2010). Besides studies focusing on students’ conceptual understanding of resonance, little research has been conducted on how students draw resonance structures. For instance, Betancourt-Pérez et al. (2010), used different tasks to investigate students’ competence in the construction of resonance structures across different learning levels in organic chemistry by prompting their participants to (a) draw curved arrows to show the electron movement in resonance structures, (b) draw alternative structures for a given ion or molecule, (c) identify the most stable resonance structure, and (d) draw the resonance hybrid. Their results show that students perform poorly in drawing resonance structures and exhibit different errors in both the first and second semester. The most prevalent errors encompass, for example, the violation of the octet rule, e.g., by moving π-bonds toward atoms with a full octet, irrespective of the atom’s hybridization and number of bonds. Moreover, students tend to break σ-bonds between carbon and hydrogen atoms, put charges on atoms that are not charged or construct resonance structures with a different delocalized system, thus different connectivity, compared to the initial structure (Betancourt-Pérez et al., 2010). The authors concluded that students, especially at the beginning of their studies, do not pay much attention to details when drawing resonance structures (Betancourt-Pérez et al., 2010). In a recent study, Petterson et al. (2020) demonstrated that students struggled to identify the correct place to start the movement of electrons when deriving one resonance structure from another in the context of acid-base reaction mechanisms. Although these results may be explained as knowledge gaps related to the resonance concept, it remains unclear what actually characterizes students’ drawing process, i.e., what structural features learners perceptually pay attention to when translating one resonance structure into another, eventually leading to invalid structures and therefore causing wrong or erroneous inferences. Thus, students’ ability to decode and manipulate molecular structures requires a more profound analysis to determine the sources of students’ success or difficulties while drawing.
Theoretical framework
Representational competence
Learning chemistry includes learning how to effectively use representations such as chemical symbols, diagrams, or ball-and-stick-models to make “sense of the invisible and untouchable” (Kozma and Russell, 1997, p. 949) and, consequently, connect the molecular level to the corresponding macroscopic phenomenon (Johnstone, 1991). Often, multiple representations are combined to facilitate learning and problem solving, as they can either complement each other by offering different perspectives of the given phenomenon, constraint the interpretation of the provided material, or help in constructing a more profound understanding (e.g., by facilitating abstraction) (Ainsworth, 2006). Depending on the coding system of the representations in the working memory channel (i.e., symbolic or analogous), one can differentiate between multiple heterogeneous (i.e., a combination of symbolic and analogous representations) and homogeneous (i.e., either exclusively symbolic or exclusively analogous representations) representations (Ott et al., 2018; Malone et al., 2020). While a heterogeneous representational system in chemistry could be, for example, the combination of a drawn Lewis structure and a ball-and-stick model, a homogeneous system could be the combination of a text and a reaction equation or, more specifically, the depiction of multiple resonance structures. Irrespective of the combination, to profit from (multiple) representations and their synergies for knowledge acquisition and problem-solving, learners need to develop representational literacy (Lesh et al., 1987; Gilbert, 2005; Cooper et al., 2017). This means not only becoming proficient in the use of each representation, but also being able to interrelate corresponding information within and across these different representations and, thus, construct a coherent mental representation of the phenomenon (i.e., local and global coherence formation) (Seufert, 2003; Seufert and Brünken, 2006). Concerning the construction and use of resonance structures, for instance, the relationship between different structures, i.e., how one structure can be transformed into another, and their specific contribution to the overall electronic distribution in a molecule, is crucial.
Based on the comparison of expert and novice representational practices, Kozma and Russell (1997, 2005) defined representational competence by deriving a set of cognitive skills and practices that enable a person to successfully use representations to reason about, express ideas, and create meaning for scientific phenomena. In particular, representational competence encompasses the abilities to (1) use representations to describe observable chemical phenomena and their underlying molecular entities and processes, (2) select or generate a representation and explain its appropriateness for a particular purpose, (3) identify, describe and interpret features of a particular representation, (4) make connections across different related representations by mapping features of one representation onto those of another, and explain the relationship between them, (5) take the epistemological position that representations are modeling but are distinct from the phenomenon observed, (6) evaluate representations by describing limitations and affordances of different representations, and (7) use representations in social situations to support claims, draw inferences, and make predictions about chemical phenomena (Kozma and Russell, 1997, 2005). These skills follow a developmental trajectory, i.e., they develop in sophistication over time. Different proficiency levels can be achieved in varying contexts with different types of representations. For instance, a learner may master most of the skills listed above regarding a particular representation (e.g., Newman projections) but achieve only a low level of representational competence for other representations (e.g., reaction coordinate diagrams) (Kozma and Russell, 2005).
To transform resonance structures into one another, multiple representational skills play a role. First, this transformational process involves analyzing the given starting structure by decoding structural features which have the capacity to delocalize electrons. This skill necessitates a global, holistic view of the structure since the delocalization of electrons can be spread over multiple parts of a molecule and is not limited to a single structural feature. Following this selection process, one structure is translated into another by delocalizing π-electrons and constantly evaluating the hypothetical, resulting structure regarding plausibility and the overall electronic distribution. This can subsequently serve as a basis for predictions of reactions. It becomes evident that the translation in this specific homogeneous representational system does not only require the careful mapping of structural features but crucially depends on the ability to interpret a given structure and possible sources for resonance.
In this regard, the ability to interpret representations further depends on different factors (Schönborn and Anderson, 2008, 2010). According to Schönborn and Anderson (2008, 2010), these factors consist of (1) the external features of the representation (Mode, M), (2) the use of underlying cognitive processes and skills to make sense of a representation (Reasoning, R), and (3) learners’ (prior) knowledge of relevant concepts (Conceptual, C). Moreover, these three main factors are intertwined, resulting in four additional factors influencing students’ ability to interpret representations. That is the R-C factor which encompasses students’ ability to employ appropriate conceptual knowledge necessary for interpreting the representation, the R-M factor that involves the deciphering and perception of the visual information embedded in the representation, and the C-M factor which describes the propositional scientific knowledge that is transmitted through the explicit features of a representation. This may concern, for example, the complexity and clarity of the representation. Finally, engaging all factors, the C-R-M factor embodies students’ ability to successfully interpret a representation by linking their conceptual knowledge to the representational features when decoding information communicated by the representation (Schönborn and Anderson, 2008, 2010). It becomes evident that the interpretation and subsequent construction of resonance structures require a sound conceptual understanding of resonance and highly depend on students’ ability to decipher and reason with relevant graphical features of a structural representation. Therefore, the perceptual mechanisms guiding the decoding of the representations and underlying the visuospatial operations when constructing resonance structures must be considered for a holistic characterization of students’ approaches when constructing resonance structures.
Mechanisms of visual selection
In order to make sense of the visual information representations convey while organizing and integrating it with prior knowledge and making it subsequently available for higher-order cognitive processes such as reasoning, the visual input has to be filtered to select relevant stimuli (Mayer, 2005; Anderson, 2013). Research on visual search differentiates between three competing mechanisms of visual selection driving the allocation of attention: a stimulus-driven, a goal-driven, and history-driven selection (Awh et al., 2012; Anderson, 2013; Theeuwes, 2019). The stimulus-driven selection is considered a bottom-up process that depends on factors external to the observer, such as the visual salience of the stimulus (e.g., heteroatoms in molecules) that is responsible for the attraction of attention (Theeuwes, 2019). In contrast to that, the goal-driven selection proceeds in a top–down process. Here, the visual search goals and, therefore, the intentional, deliberate control of an observer (e.g., the active search for a specific feature due to rules or prompts) influences the attention to features of a stimulus (Theeuwes, 2019). As a domain-specific prompt or problem task requires where to look or what to attend to, the degree of sophisticated domain knowledge may influence how attention is directed to a given visual input and how it is perceived. In constructing resonance structures, this could be embodied, for example, by carefully examining the fulfillment of the octet rule. The history-driven selection applies when previous experiences drive attentional selection. Thus, information selected in the past affects the way information is selected in subsequent situations. That may encompass, for instance, the probability that features having been repeatedly attended to in the past are more often selected and identified in a given situation (e.g., considering double bonds in the context of resonance structures as they have been often delocalized in previous exercises) (Theeuwes, 2019).
Research questions and hypotheses
Although research indicates that students encounter numerous difficulties when constructing resonance structures and that the construction of resonance structures crucially depends on students’ competence to deal with structural formulas in terms of decoding, selecting, and manipulating these representations (Kozma and Russell, 2005; Schönborn and Anderson, 2010), a profound analysis of their drawing processes is still lacking. Supporting students in drawing, therefore, requires a closer look at how students are sequentially generating their drawings. Recording and analyzing the eye-movement trajectories of students can help to characterize to what extent students use and perceive different drawing elements to generate resonance structures and to determine how different gaze patterns may relate to the productivity of the generated drawings. Specifically, by examining students’ drawing processes quantitatively and qualitatively, we seek to answer the following research questions (RQ) in this exploratory study:
1 What drawing elements do students connect when constructing productive or unproductive resonance structures (i.e., in terms of transitions and relations of the Areas of Interest)?
2 What structural features do students attend to in terms of attention distribution when translating one resonance structure into another and how is it related to students’ drawing moves?
3 How does a student’s approach to visual selection to construct resonance structures relate to the productivity of the drawings?
With regard to the first research question, we assume that students with unproductive drawings might exhibit a more varied search behavior, thus, cognitively connect more drawing elements in the construction process, including the use of and the transition between unrelated drawing or task elements. This hypothesis is supported by previous eye-tracking research across different STEM education disciplines as it has been repeatedly shown that successful problem-solvers fixate more on relevant aspects of a representation and generally show a more focused behavior, whereas unsuccessful problem-solvers exhibit a more distributed visual behavior including more fixations on irrelevant aspects of a given representation (Tang and Pienta, 2012; Hejnová and Kekule, 2018; Havelková and Gołębiowska, 2019). In accordance to that, it has been demonstrated that inexperienced individuals more often attend to salient information (i.e., stimulus-driven information selection) that may be irrelevant for task performance. Experienced individuals, on the other hand, efficiently attend to information knowing which information is important for task performance (goal-driven selection) (Jarodzka et al., 2010). Based on the consideration of visual search mechanisms, we assume for the second research question that the construction of unproductive drawings may stem from difficulties to select more specific task-relevant information, such as identifying interacting structural features in a resonance structure, and may result in an overreliance on single, salient structural features. Finally, we assume for the third research question that students who explicitly apply conceptual knowledge to the construction of resonance structures, such as the application of rules or the inference of implicit structural properties, more often construct productive drawings than students whose drawing approach is characterized by a mere rearrangement of surface structural features (Graulich et al., 2019).
Materials and methods
Context and participants
This research study was conducted at a German university in summer 2021. Students were recruited on a voluntary basis via e-mail and in-class announcements in the Organic Chemistry 1 (OC1) course and were given 20 euros as compensation for their time. A total of 21 students agreed to participate in this study. The participants’ ages ranged from 19 to 34; nine of them identified as male and twelve as female. One student was excluded from the analysis due to measurement errors, so the number of further analyzed participants was reduced to 20. All students were majoring in chemistry (i.e., they were chemistry, food chemistry, and chemistry teacher students) and were beginners in organic chemistry, i.e., all of them had taken OC1 as the first lecture in organic chemistry either in the second or fourth semester of their studies. Normally, this course consists of a weekly lecture (3 h) and weekly tutorial sections (1.5 h). Due to the pandemic situation, in summer 2021, a flipped format was adopted in which students watched tutorial videos and read material (e.g., book chapters) prior to solving content-related tasks and discussing questions in online tutorial sections. The OC1 course provides basic knowledge of organic chemistry, covers the reactivities of functional groups, deals with structure-property relationships and discusses typical reaction mechanisms (e.g., radical substitution, electrophilic addition, nucleophilic substitution, and carbonyl reactions). The study took place near the end of the course to assure that the students were familiar with the reactions used in this study, the resonance concept, and the construction of resonance structures.
All students who volunteered were informed about their rights and data handling beforehand; informed consent was obtained from all participants. Institutional Review Board approval was not required for this study. Nevertheless, the study followed ethical guidelines and it was clarified to the students that they had the opportunity to opt out at any time. All students gave their written consent for the collected data (i.e., their recordings and scans of their worksheets) being analyzed and published by the research team. In this study, participants were assigned pseudonyms and no identifying information was recorded or scanned to allow participants to re-identify. As the interviews were conducted in German, students’ interview excerpts were translated into English for this publication.
Data collection
Study design
The study followed a qualitative approach and used a semi-structured interview to explore both students’ reasoning with drawings and students’ drawing processes of structural formulas in organic chemistry (Figure 1). Before starting the interview, the interviewer explained the interview procedure and briefed the students that the topic of the study would be the resonance concept. In the first section, general questions about the resonance concept (e.g., “When does resonance occur?”) have been asked to refresh the students’ minds and to gather information on students’ abilities to draw resonance structures as well as to infer chemical information from the structural formulas (e.g., “What impact does resonance have on the energy of a structure?”). In the subsequent main section, the students were prompted to solve three organic case comparison tasks (Graulich and Schween, 2018) requiring resonance structures. We used case comparisons to elicit students’ drawing process as they necessitate drawing resonance structures in order to solve the given problems and estimate the differences between the two given reactions. All reactions were covered in the OC1 lecture. In the last section, problems with regard to the completion of the tasks as well as students’ needs for these types of tasks were addressed in a general reflection.
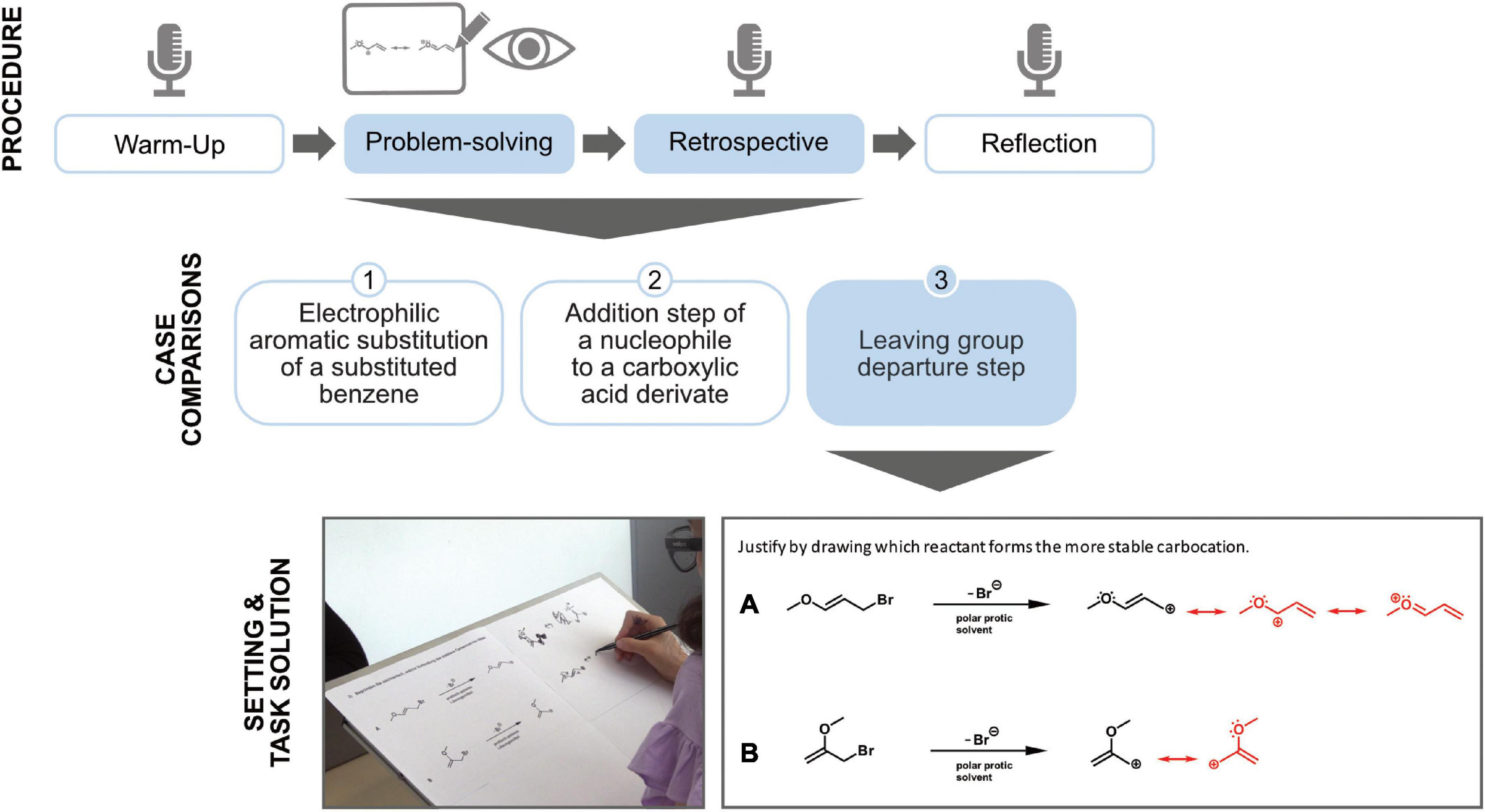
Figure 1. Study design and task solution for the utilized case comparison. Blue boxes indicate the results highlighted in this report.
The subsequent analysis focuses on the third case comparison task as it allows the direct comparison of students’ drawings. It describes the mechanistic leaving group departure step of a nucleophilic substitution reaction and asks the students to determine which of the two reactions would form the most stable product. As shown in Figure 1, bromide leaves as leaving group under the formation of a carbocation in both reactions. In each case, the substrate is a primary alkyl substrate which enables stabilization via resonance. Only the position of the methoxy group differentiates the substrates. To determine the most stable carbocation, one has to draw and evaluate the electron density distribution in the resonance structures of both products. While there are two (productive) resonance structures in B, there are three in A. It follows that the positive charge can be better distributed across the whole molecule in A. This is responsible for the lower potential energy and, consequently, for the higher stability of product A.
Qualitative interview
A qualitative semi-structured interview guided the investigation of students’ drawing and reasoning process. The interviews were conducted face-to-face between one participant and the interviewer, lasted between 83 and 140 min and were audio- and video-recorded. All materials were administered in a pencil-paper setting and the participants were encouraged to solve all the tasks freely, thus, they could write or draw as much as they considered necessary. To capture students’ eye movements in their natural problem-solving behavior, they were not disturbed or had to explain their approach during the completion of the case comparison tasks. However, they were allowed to think aloud if they wanted. After completing each case comparison task, a subsequent retrospective interview focused on students’ rationale for their drawings and their task specific problem-solving process. For instance, the students were asked to justify their final choice and to describe their drawing strategies (e.g., “How did you get to this structure?”).
Eye-tracking
A mobile eye-tracker (Tobii Pro glasses 3, 50 Hz) served as a tool to capture the participants’ eye-movements while drawing during the problem-solving process. To optimize the collection of the eye-tracking data, a drafting table was used and each task was presented on the upper left side of a 42.0 × 59.4 cm (DIN A2) sheet of paper (Figure 1). The eye-tracking glasses were calibrated and validated individually for each participant prior to solving the tasks. In case of nearsightedness and farsightedness, suitable corrective lenses were used. A vision test also validated their fit. All gaze samples ranged above 79% (average 91.7%).
Data analysis
Eye-tracking data
Data preparation
First, the gaze data of all participants’ recordings during the problem-solving phase, i.e., until the students gave their answers prior to subsequent possible refinements of the drawings, were manually mapped by a trained student research assistant using the software Tobii Pro Lab. The first author double-checked the mapped gaze points to check accuracy. Second, for every participant, the Times of Interest (TOI) were defined for each drawing event (i.e., the time sequence until completion of a single student-generated resonance structure). The duration of the overall drawing process for the task varied for each participant ranging from 1.02 to 12.25 min (average 4.06 min). The construction of the single resonance structures took the participants from 6 s to 5.26 min (average 51 s).
Third, the Areas of Interest (AOI) were defined for each drawing event (i.e., every constructed resonance structure until the final decision of the student) for each student. For the subsequent analysis, the AOIs were set on different grain sizes. An AOI was defined for every complete drawing and for smaller parts of the respective molecules, always maintaining the main features of the structures (i.e., the methoxy group, the double bond, and the positive charge) (Figure 2A). All eye-tracking analyses were conducted with the software Tobii Pro Lab and RStudio.
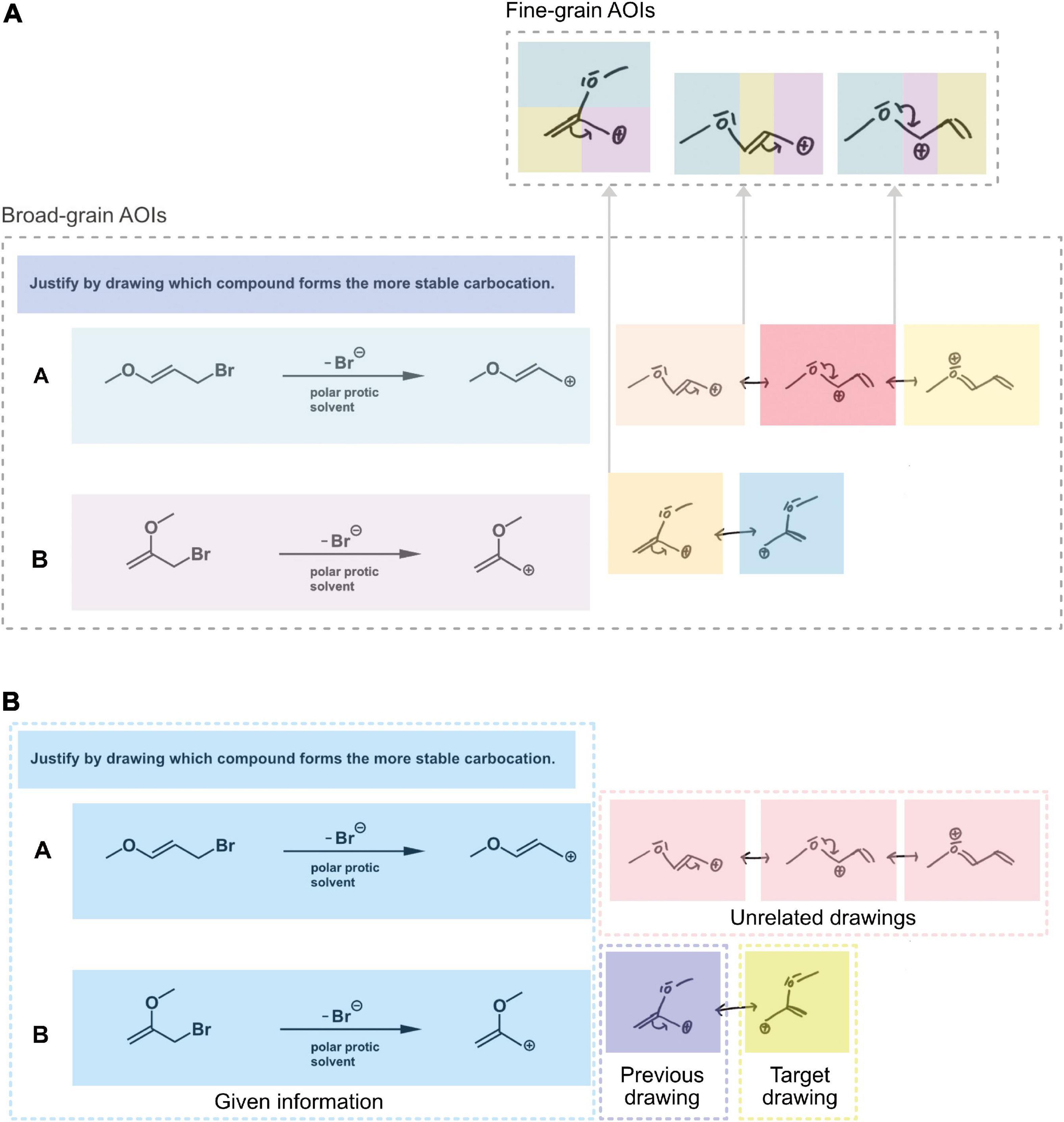
Figure 2. (A) Definition of the AOIs of the drawing elements at a broader and finer grain size exemplified by a student solution and (B) exemplary categorization of the AOIs in dependence of their function (target drawing, previous drawing, given information, unrelated drawings).
Furthermore, the students’ drawings were classified as productive, unproductive, and auxiliary drawings. While productive drawings encompass all correct resonance structures that help answer the given task, unproductive drawings are either incorrect resonance structures (e.g., violating the octet rule) or drawings that are technically correct, respecting the octet rule, but are, nevertheless, insignificant for this task due to the number of formal charges. The auxiliary drawings are either structures that the students copied from the given task or structures or texts that the students wrote for themselves and were, thus, not directly related to the construction of the resonance structures. They were thus not taken into consideration in the following analysis. Figure 3 provides an overview of the different drawing categories. Next, the data were analyzed in several steps using a combination of quantitative and qualitative approaches.
Examining the connection of drawing elements during the drawing process
To determine how students used the different drawing elements to construct subsequent resonance structures, the analysis of students’ gaze behavior was twofold, looking at the structures’ co-occurrence during the entire drawing process and the transitions between these structures (RQ 1). In doing so, we grouped all AOIs for each drawing event at a broader grain size into four categories to enable the comparison of the various construction processes of every student: target drawing (T), previous drawing (P), unrelated drawings (U), and given information (G) (cf. Figure 2B). While the target drawing is the resonance structure of interest, the previous drawing represents the drawing from which the target drawing results. The given information consists of all the structures and text available to the students in the task prompt. The transformation of one structure into another is carried out at the local structure, i.e., apart from the previous drawing, no other information is necessary in order to construct the target drawing. Therefore, we labeled all the structures and texts that the students might have noted but that are per se not necessary for constructing the target drawing (e.g., resonance structures of the product in A when the participant is constructing the resonance structures in B as well as additional explanatory text) as unrelated drawings. Although the given information is neither required for the construction of resonance structures and thus also represents unrelated information, we maintained this category to investigate whether there are tendencies of revisits as anchors when constructing a productive or unproductive drawing.
First, we conducted an Epistemic network analysis (ENA) to examine the co-occurrence of the various drawing elements during the construction of resonance structures and, thus, to explore different gaze patterns depending on the resulting productivity of the drawing. The ENA is an analytical method from the field of learning analytics, which has been used in numerous contexts, including eye-tracking analyses (Andrist et al., 2015; Shaffer et al., 2016; Bruckner et al., 2020). This method can be used to identify and quantify the structure of connections among coded data elements in any system by representing their associations in networks, i.e., the mere presence of isolated elements is not as important as their interrelations (Shaffer et al., 2016). Thus, the ENA allows the characterization of even complex and dynamic relationships (e.g., patterns of association within discourse or gaze behavior) by illustrating both the structure and the strength of connections in both single networks (e.g., in terms of their plotted point position) and network difference graphs which illustrate the differences between two networks by subtraction (Shaffer et al., 2016; Shaffer and Ruis, 2017). While the nodes in the network correspond to the analyzed codes and appear in every modeled network in exactly the same position, the edge width between the nodes reflects the relative frequency of co-occurrences between two codes. In order to model the connections, ENA uses a singular-value decomposition which performs dimensional reduction on a high-dimensional space, producing fewer dimensions that capture the maximum variance in the data (Shaffer et al., 2016).
In our analysis, we used the ENA Web Tool (version 1.7.0) to compare the weighted epistemic networks of the construction processes of productive and unproductive drawings on the basis of students’ collapsed AOI hit sequences during the construction of resonance structures. To this end, each drawing served as a unit, i.e., the piece of data for which the ENA constructs networks. Conversations indicate how to segment the data for analysis in terms of their relation to one another, i.e., units not in the same conversation are not related to one another in the network model. As we aimed at characterizing the drawing process of each resonance structure individually, again, we chose as conversation every drawing and chose the mode whole conversation so that the ENA modeled connections across the entire conversation. In the ENA, the nodes of the network represent the codes. In order to be able to compare the various drawing processes, our codes comprised the previously defined categories “given information,” “unrelated drawings,” “previous drawing,” and “target drawing” for every drawing event. Finally, as comparison served the productivity of the drawings, i.e., we differentiated between productive and unproductive drawings.
In a second step, the same AOI hit sequences used in the ENA beforehand were analyzed to determine the transitions between individual pairs of AOIs that the students made to construct resonance structures in order to reveal how students integrated the various drawing elements (i.e., the defined four drawing categories) when constructing structural formulas (Schmidt-Weigand et al., 2010; Johnson and Mayer, 2012). For that purpose, we used the GrpString R package (Tang et al., 2018) to calculate the transition matrix, the transition entropy and the total amount of transitions across the groups of unproductive and productive drawings, regardless of whether the drawings resulted from a previously productive or unproductive drawing as no discriminating differences could be found in a finer division. The transition entropy indicates the diversity of the transitions within a string or different string groups. While a higher entropy reflects more evenly distributed transitions, a lower entropy measure indicates a more biased distribution of transitions, mainly reflecting transitions between fewer AOIs (Tang et al., 2018). The transition matrices were also explored for statistical significance using the Mann-Whitney U test. A non-parametric test was chosen because a Shapiro-Wilk test (p < 0.05) indicated that not all of the data to be used for comparison are normally distributed (Shapiro and Wilk, 1965).
Analyzing the attention distribution while constructing resonance structures
To gain deeper insight into the translation process of single resonance structures, the analysis further concentrated on the connection of each drawing pair, i.e., the previous and target resonance structure (RQ 2). As the attention distribution may indicate the ascribed importance of a representation (Cullipher and VandenPlas, 2018), we examined the total fixation duration of the AOIs of the previous drawing to which students attended when constructing the target resonance structure. In doing this, we used the AOIs at the smaller grain size encompassing the different structural features of the respective previous resonance structure (cf. Figure 2A). With this data, we then calculated the ratio of attention distribution on each structural feature of the previous resonance structure. Again, we differentiated between productive and unproductive drawings for all drawings to examine possible differences in attention distribution.
Qualitative data
Determining the relationship between drawing moves and attention distribution while constructing resonance structures
To relate and compare the attention distribution to students’ drawing moves for possible relationships (RQ 2), students’ drawing moves made to get from one structure to the next one were inductively analyzed (i.e., the delocalization of electrons and change of the structural features). Different drawing moves could be identified which can be subsumed either as single drawing move (e.g., delocalizing one electron pair or charge) or as multiple drawing moves (e.g., delocalizing several electron pairs) (Figure 4).
The first author coded all drawings. Additionally, a trained student research assistant coded the entire data independently with the code book. A kappa coefficient κn of 0.87 (Brennan and Prediger, 1981) was calculated, showing high agreement and reliability for the coding rubrics (Kuckartz and Rädiker, 2019). Any ambiguities were discussed and resolved to reach a final agreement of 100%.
Characterizing students’ approaches of visual selection
All audio recordings of the interviews were transcribed verbatim and implemented into the coding software MAXQDA for subsequent qualitative content analysis (Saldaña, 2016). To examine students’ approaches of visual selection when constructing resonance structures and, consequently, triangulate the eye-tracking data findings, our qualitative analysis centered on how students verbally described their drawing process (RQ 3). The analysis was informed by the theory of visual selection described in section “Mechanisms of visual selection” (Theeuwes, 2019). However, we could not adopt the three different mechanisms of visual selection as codes due to different reasons. First, the problem-solving process in this study, as a whole, is clearly a top–down process because the participants knew that they had to use the resonance concept to solve the task and, consequently, to draw resonance structures by moving electrons within structural formulas. Therefore, the task demands by definition a goal-driven approach to construct the resonance structures. Second, it is not possible to properly distinguish between a stimulus-driven, history-driven, and goal-driven approach in many chemistry contexts, because reasoning and sense-making often depend on the interplay of deriving and perceiving explicit and implicit structural features and properties (Graulich et al., 2019). Students can exhibit both a bottom–up and top–down approach. Therefore, we adapted the aforementioned mechanisms into two categories which we applied as a lens to analyze students’ descriptions of their drawing approach: a knowledge-driven approach and a structure-driven approach. The code “structure-driven” was ascribed whenever the students described their drawing process by only referring to and mentioning the explicitly drawn structural features. Thus, it can be considered a bottom–up process resembling the stimulus-driven approach. The code “knowledge-driven” was given when the students explicitly used and verbalized their knowledge to construct the respective resonance structure. This may include different kinds of knowledge, e.g., experiences or concept knowledge such as the reference to (implicit) chemical concepts (e.g., stability), or rules (e.g., the octet rule). Therefore, this approach can be considered a top-down process that resembles the goal-driven and history-driven approach.
Moreover, we characterized the flexibility of students’ approaches. While a “centered” approach applies when students were focusing on one structural feature (e.g., the methoxy group of the molecule), students with a “variable” approach described their drawing process by taking into account the entire structure or at least several interacting structural sections of the starting molecule. Figure 5 provides the definitions of the resulting four codes and illustrates them by giving student examples for each coding category.
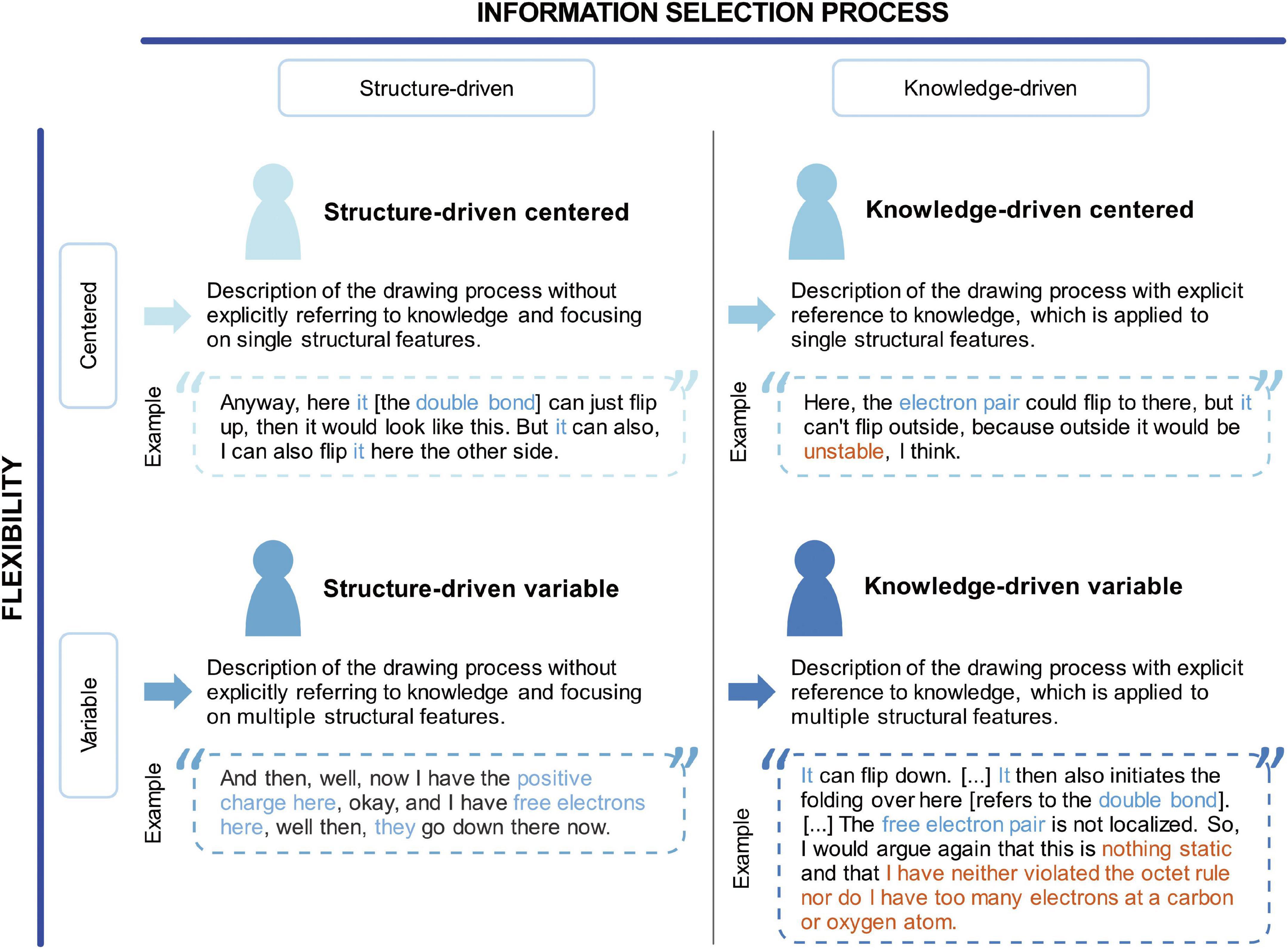
Figure 5. Coding scheme for students’ visual selection approaches with definitions and student examples. The highlighted blue text in the quotes indicates the structural features the students referred to, while the orange text highlights the verbalized knowledge (e.g., rules and chemical concepts).
The first author coded the entire data set. During the data analysis, the authors regularly discussed and optimized the coding scheme to ensure that coding decisions faithfully represented the data. For interrater reliability, the second author coded a random sample of 20% of the data independently. A kappa coefficient κn of 0.94 (Brennan and Prediger, 1981) was calculated, indicating high agreement and reliability for the coding rubrics (Kuckartz and Rädiker, 2019). Any ambiguities were discussed and resolved. In the end, a 100% agreement between the two authors was reached.
Results and discussion
Of all 60 student-generated resonance structures, 41 drawings were productive, whereas 19 were unproductive, often resulting from previously productive drawings or building a sequence of unproductive resonance structures. Therefore, it is of interest to characterize what distinguishes the construction process of productive from unproductive drawings. Using a Mann–Whitney U test, we compared the construction of productive and unproductive drawings on the basis of different eye-tracking data (Table 1). Addressing our research questions, the subsequent sections present and discuss the main findings.
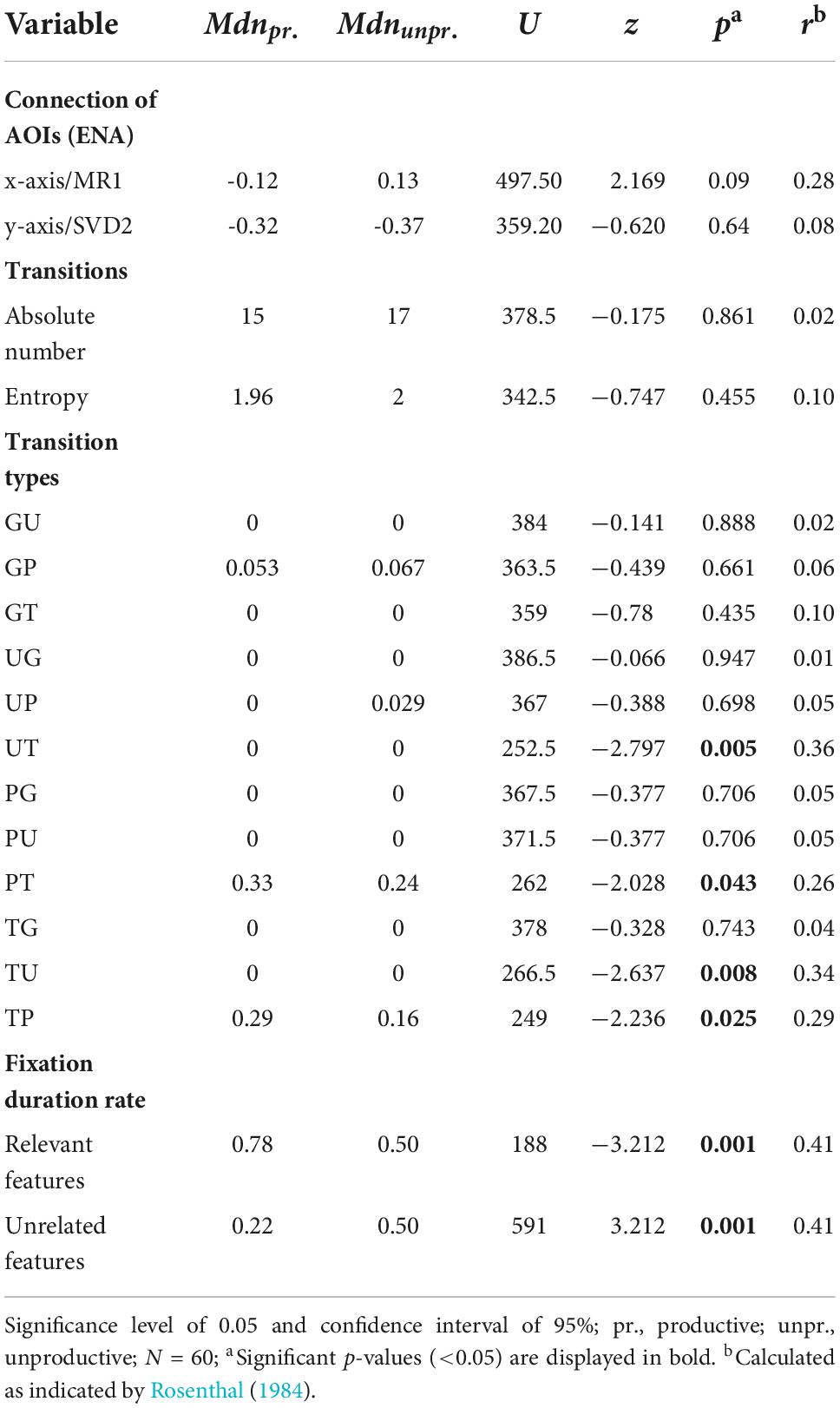
Table 1. Results of the Mann–Whitney U test for different eye-tracking data comparing the construction of productive and unproductive drawings.
RQ 1: What drawing elements do students connect when constructing productive or unproductive resonance structures?
On a global level, students exhibit a similar gaze behavior when using information from different drawing elements to construct productive or unproductive resonance structures. In fact, as the mean epistemic networks of productive and unproductive drawings illustrate (Figures 6A,B), the co-occurrences of the different drawing categories (i.e., target drawing, previous drawing, unrelated drawings, and given information) appear with a similar density and show, in general, no considerable differences concerning the type and frequency of connections.
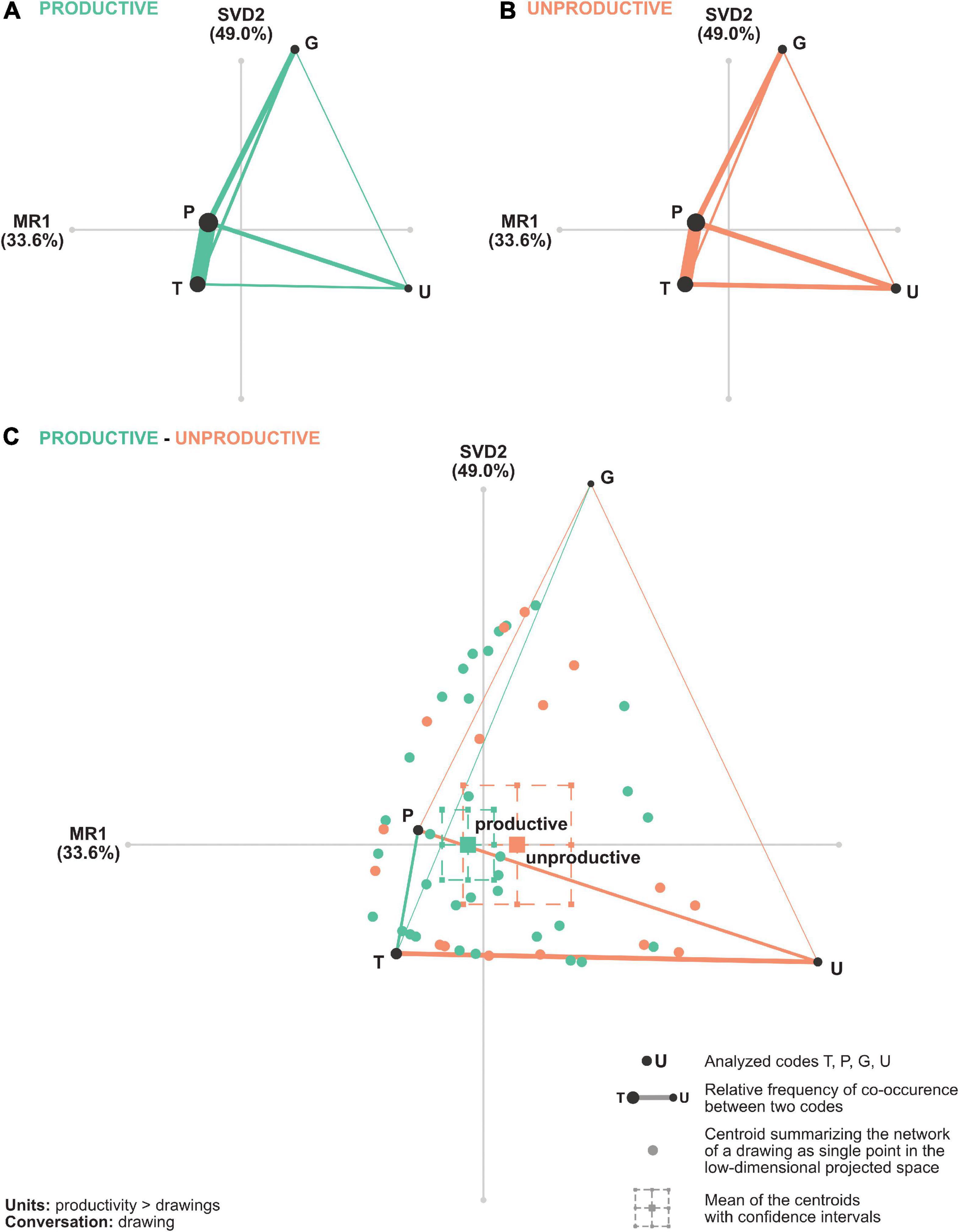
Figure 6. Epistemic network for (A) productive drawings, (B) unproductive drawings, and (C) the subtracted epistemic network of productive and unproductive drawings using the collapsed AOI hit sequences during the construction process of every drawing (N = 60). The drawing categories (T, target drawing; P, previous drawing; G, given information; U, unrelated drawings) serve as codes.
Consistent with this observation, a Mann–Whitney U test showed that neither along the x-axis (MR1) nor the y-axis (SVD2) productive drawings differ significantly from unproductive drawings (Table 1). From a qualitative perspective, the association between the previous drawing and the target drawing dominates in both groups. Comparing the different networks for productive and unproductive drawings, represented by the position of the respective network graph node (Figure 6C), no distinctive tendency concerning the patterns of co-occurrence of the drawing elements can be inferred. This result indicates that the construction of an (un-)productive resonance structure does, thus, not globally depend on the resulting network, i.e., the amount and density of associations of the different drawing elements. Hence, our hypothesis of unproductive drawings resulting from the connection of more drawing elements cannot be confirmed. However, the subtracted mean network (Figure 6C) shows that there exist differences concerning single connections. Students with productive drawings (green color) exhibit a stronger association of the previous and target drawing, whereas students with unproductive drawings (orange color) show more associations of the unrelated drawings with both the target drawing and the previous drawing. This indicates that using unrelated information seems to play a bigger role when constructing unproductive structural formulas. The subtracted mean network also illustrates that in both groups, the associations between the other drawing elements are altogether scarce and that the given information rarely co-occurs with the other drawing elements.
Although the ENA models the gaze-pattern networks of the different construction processes of (un-)productive drawings by depicting the weighted frequency of co-occurrences of the different drawing elements, it provides no insight into the direction of linkage, i.e., transitions, between those elements (e.g., in terms of backtracking). The ENA only depicts how often drawing elements co-occur altogether. However, transitions play a crucial role when processing information (Schmidt-Weigand et al., 2010). Thus, analyzing the linkage direction may also reveal differences regarding the direct connection of drawing elements, such as integrating information to construct productive and unproductive drawings.
A look at the different transitions during the drawing process of the resonance structures reveals that in general, in accordance with the ENA, the transition entropy for productive or unproductive resonance structures does not differ significantly (Table 1), thus, the gaze transition distribution is similar for both groups. Neither does the absolute number of transitions differ significantly. However, as listed in Table 1, there are significant differences between the types of transitions during the drawing process of a productive and unproductive drawing regarding the transitions to the target drawing.
Figure 7 depicts the subtracted transition matrix of productive and unproductive drawings with green cells indicating transitions more characteristic for productive drawings. In contrast, red cells show transitions rather occurring with unproductive drawings. According to Table 1, it becomes evident that the drawing process of productive resonance structures encompasses significantly more transitions between the previous drawing and the target drawing (p = 0.043, r = 0.26) and vice versa (p = 0.025, r = 0.29). In comparison, the drawing process of unproductive drawings comprises more transitions between the target drawing and unrelated drawings (p = 0.008, r = 0.34) and vice versa (p = 0.005, r = 0.36). Figure 7 shows as well that students with unproductive drawings connect the given information with the previous drawings more often than students who construct productive drawings. However, the difference of this transition type is statistically not significant. Given that transitions play a crucial role in the integration and processing of information (Schmidt-Weigand et al., 2010; Johnson and Mayer, 2012), an increased integration of unrelated information can impede the construction of valid resonance structures. In fact, integrating more information in the target drawing by focusing on diverse, less relevant, drawing elements, can indicate searching processes and uncertainty. That said, it is possible that learners try to align and transfer information from previous drawing processes or search for anchor points. This assumption is in line with previous research on problem-solving and the comprehension of visualizations (e.g., Gegenfurtner et al., 2011; Tang et al., 2014). For instance, Holmqvist et al. (2011) showed in the context of a mathematical problem-solving task that participants with low ability tended to scan all AOIs in the tasks while high-ability participants exhibited a more focused behavior. Existing research in STEM education also supports the finding that students’ drawing process of productive drawings is characterized by more direct transitions from previous to target drawing. Therefore, multiple studies show that high-performing participants (e.g., experts) more effectively process information, e.g., by transitioning between relevant parts of tasks (Baluyut and Holme, 2019; Connor et al., 2021), by efficiently attending to task-relevant features (Jarodzka et al., 2010; Tang and Pienta, 2012; Topczewski et al., 2016; Hejnová and Kekule, 2018; Havelková and Gołębiowska, 2019), or by exhibiting a more focused searching behavior when problem-solving (Holmqvist et al., 2011; Rodemer et al., 2020).
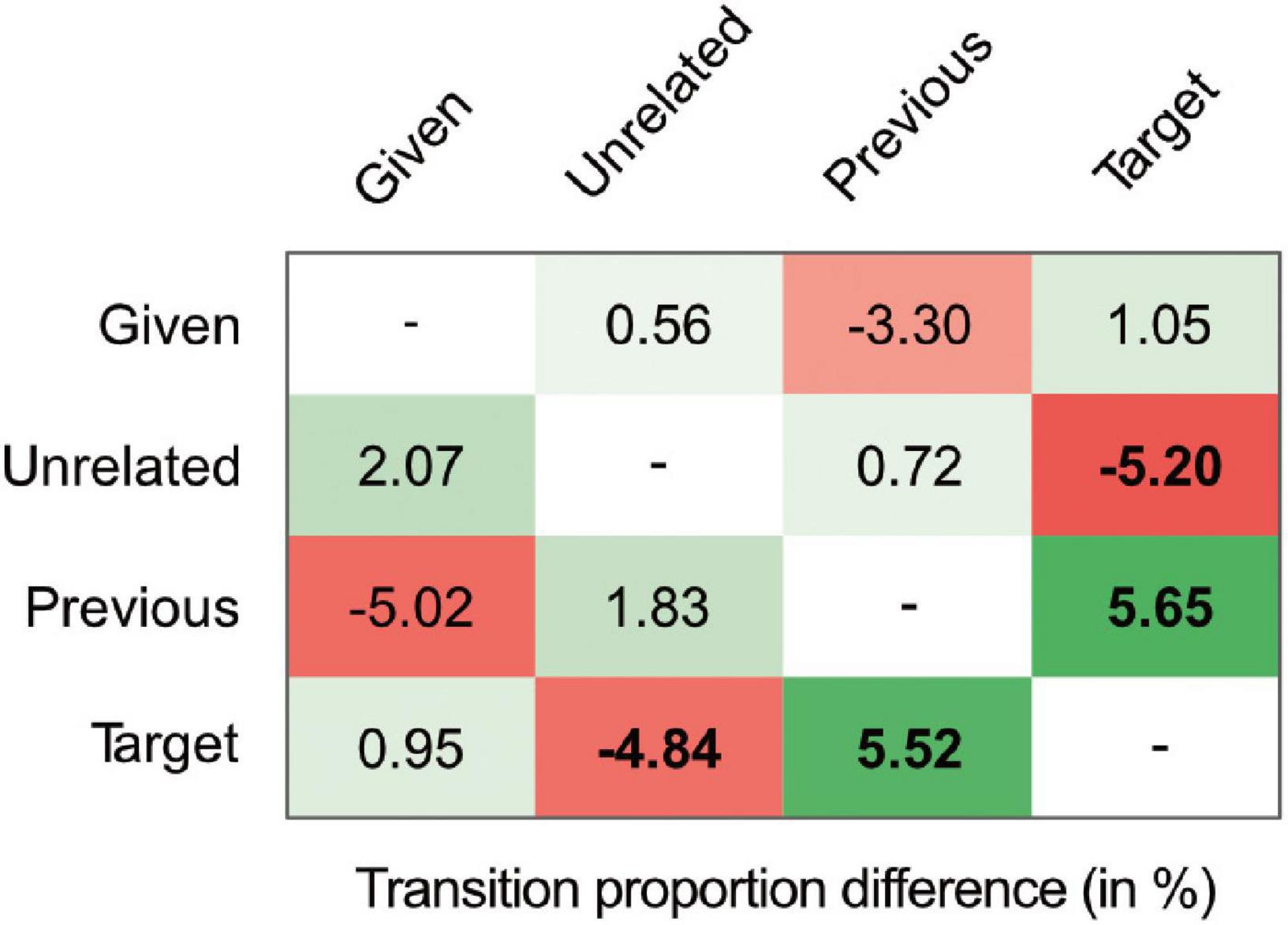
Figure 7. Subtracted transition matrix indicating characteristic transitions regarding the construction of productive (green cells) and unproductive drawings (red cells); significant differences in transition types are displayed in bold (p-value of < 0.05).
However, despite the group-specific differences in transitions, the connection between the previous drawing and the target drawing overall plays a major role in students’ construction process of resonance structures. This suggests that the students in this study already possess a distinct representational fluency concerning the direct translation from one representation into another. With regard to representational competence, as described by Kozma and Russell, students with productive and unproductive representations show similar abilities to identify and select structures necessary to transform a resonance structure into another (Lesh et al., 1987; Kozma and Russell, 1997, 2005).
RQ 2: What structural features do students attend to in terms of attention distribution when translating one resonance structure into another and how is it related to students’ drawing moves?
Since all students mainly connected the previous and the target drawing when constructing productive and unproductive resonance structures, it is of interest to investigate students’ attention distribution when translating one structure into another, and thus, to consider their fixation duration on the different parts of the starting molecule, the previous drawing, in order to characterize their decoding behavior (R-M factor) (Schönborn and Anderson, 2008, 2010).
Figure 8 illustrates the fixation duration rate for the construction of productive and unproductive resonance structures on the basis of productive, initial drawings (57 of 60 drawings). Due to their very specific and individual character, Figure 8 does not comprise the remaining three structures resulting from unproductive drawings. The frames of the respective pie charts indicate the adjacent parts of the molecular structure which are relevant for translating this structure into the corresponding productive structure. As Figure 8 illustrates, the fixation duration rate differs with regard to the constructed structures, showing an emerging attention distribution difference on different parts of the molecules when constructing productive or unproductive drawings. As indicated in Table 1, the fixation duration rate of relevant and unrelated structural features for productive and unproductive drawings differ significantly with medium effect size (relevant features: p = 0.001, r = 0.41; unrelated features: p = 0.001, r = 0.41). For the productive resonance structures, a slight trend emerges toward an increased attention to the relevant structural features for the resulting resonance structure. If we consider the productive subsequent resonance structures A1 and A2, students who construct these structural formulas on average focused more on the interrelated structural features necessary for the construction of the respective structure, i.e., on the positive charge and the double bond in the first structure (83% of the attention distribution), and the positive charge and the methoxy group in the second structure (80% of the overall attention distribution). To draw the productive structure A2* on the basis of the given, initial product in A, thus, skipping the second structure, students have to consider every part of the structural formula as electrons are delocalized throughout the whole molecule. However, it becomes apparent that students’ attention was guided by the double bond and the methoxy group (altogether 81%) and less by the positive charge (19%) that, however, represents a productive starting point for the drawing process.
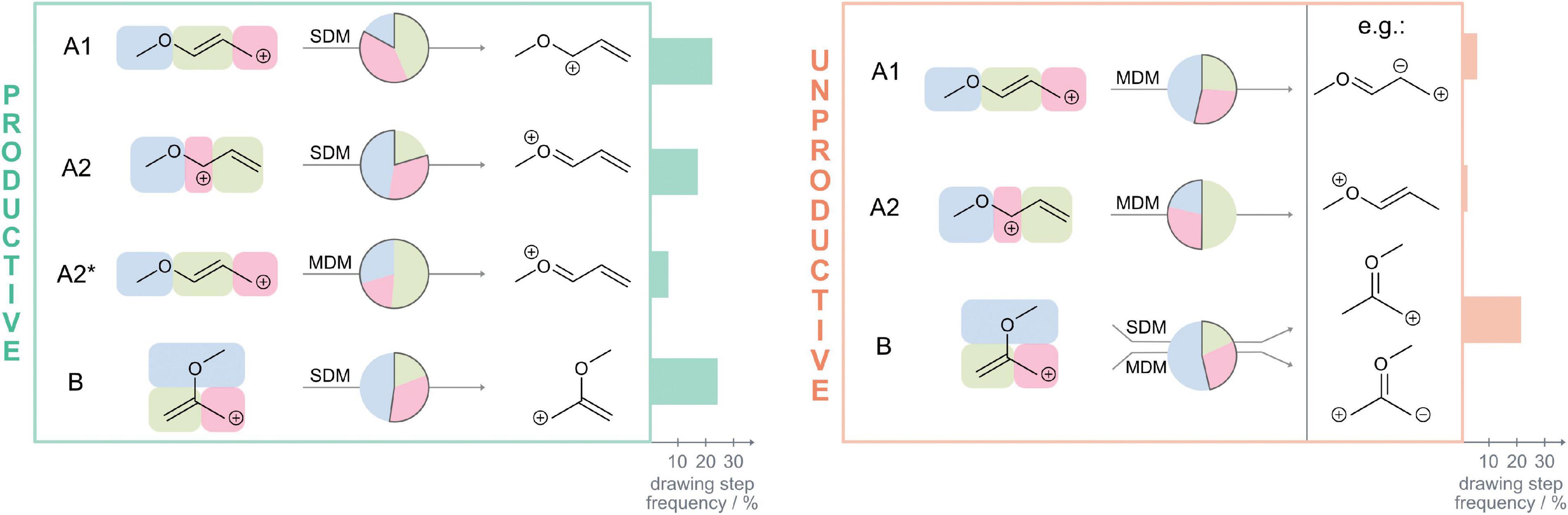
Figure 8. Relative drawing step frequency (N = 57) and fixation duration rates (pie charts) on the structural features of the initial structures (blue = methoxy group, green = double bond, pink = positive charge) when constructing a subsequent productive (green frame) or unproductive (orange frame) structure. The relevant, necessary structural features for the construction of the following structure are highlighted by framing. Drawing moves are indicated as single drawing move (SDM) and multiple drawing moves (MDM).
Figure 8 further illustrates that few students constructed unproductive drawings in reaction A, while most unproductive drawings resulted when constructing resonance structures in reaction B. In general, it can be derived from Figure 8 that unproductive drawings stem from a higher attention to unrelated structural features. For example, consider the attention distribution of the unproductive structure A1. In contrast to the productive structures A1 and A2, students shifted their attention more to the methoxy group (46%). In the unproductive structure A2, students payed their attention twice as often to the unrelated double bond (50%) whereas the structural features that are relevant for the construction of a productive structure (i.e., the methoxy group and the positive charge), were considered less (50%) compared to when students constructed a productive structure based on the same initial drawing. This trend of overly considering unrelated structural features while paying less attention to relevant, interacting structural features also applies to the unproductive resonance structures in B as students paid much attention to the methoxy group. However, here, the fixation durations rates do not differ as much from those that students exhibit when generating productive resonance structures in B. Regarding representational competence, it can be derived from these findings that although students are able to identify and connect relevant structures in order to construct subsequent resonance structures (Kozma and Russell, 2005), they differ in the decoding and interpretation of the representations by paying attention to different structural features. Consequently, they may perceive the external features of a structure differently (R-M factor), eventually resulting in unproductive drawings (Schönborn and Anderson, 2008, 2010).
Besides the attention distributions, Figure 8 depicts the drawing moves the students made to construct their drawings. In this task, productive drawings often stem from a single drawing move where electrons are delocalized toward an electron-deficient atom. As Figure 8 shows, students predominantly focus thereby on two adjacent, interrelated structural features, that is, the positive charge and the adjacent double bond or methoxy group. In contrast to that, students who perform multiple drawing moves construct either unproductive resonance structures, or draw a productive resonance structure but tend to skip a structure, which, however, would be necessary to answer the given task. Altogether, 13 of the 19 unproductive drawings are based on multiple drawing moves which may either involve arbitrarily moving charges, moving the lone pairs of the heteroatom throughout the whole molecule or moving electrons to an electron-rich atom (cf. Figure 4 for examples).
While there is a clear difference with respect to the fixation duration rate in the structures of reaction A, the gaze proportion on structures in reaction B does not differ much. Hence, our hypothesis for the second research question, that the construction of unproductive drawings results from difficulties in the selection of relevant information and, eventually, an overreliance on single, salient structural features, can only be partly confirmed. This lack of difference for reaction B may be due to different reasons. First, the different geometries of the molecules can be responsible for a different decoding behavior. While the molecules in reaction A are linear, the molecule in B is more branched, thus, spatially more complex. Following this, the decoding of the molecule and the identification of interacting structural features could have been cognitively more demanding and may have led to longer fixations of the methoxy group in order to decide upon its possible influence in the structure. In fact, a longer fixation on a structural feature correlates with longer mental processing of this information (Just and Carpenter, 1980), which may result from difficulties in interpretation or due to the perceived importance of the feature because of its salience (Cullipher et al., 2018). As students in both groups fixated much on the methoxy group, it may be that this structural feature was more difficult to interpret than the double bond, since a functional group containing an oxygen atom can have an electron-donating or electron-withdrawing function depending on its connectivity. Therefore, students may have tried to connect this feature with prior knowledge such as rules concerning its electronic effects (R-C factor) (Schönborn and Anderson, 2008, 2010). Our findings of students’ varying decoding and use of structural features when constructing resonance structures align with existing research on representational competence in chemistry. While it has been shown that students often exhibit difficulties regarding the comprehension and interpretation of representations (e.g., Keig and Rubba, 1993; Kozma and Russell, 1997; DeFever et al., 2015), more specifically, Olimpo et al. (2015) have shown that the translation of Newman projections and Dash-Wedge representations is easier for students when dealing with less complex molecules. Moreover, using eye-tracking, in Baluyut and Holme’s (2019) study it became evident that the visual complexity of particulate nature of matter diagrams impacted students’ viewing behavior as low-performing students fixated on more features of a task when working with such representations. Rodemer et al. (2020), on the other hand, showed that visual characteristics seem to influence students’ visual processing and problem-solving in organic chemistry, with more factors embedded in a case comparison leading to more transitions between representations.
Besides the higher spatial complexity, familiarity may also play a crucial role regarding students’ gaze behavior in case of the linear structures in reaction A. Both the allylic position of the carbocation and the linearity of the molecule are familiar to the students from the lecture. Therefore, it is possible that students felt more confident in decoding the structures and, consequently, could determine relevant, interacting structural features more easily, narrowing their gaze distribution on specific parts of the whole structure.
Finally, focusing on several, interacting structural features instead of statically attending to singular structural features is in accordance with the concept of resonance, requiring a global, dynamic view on the static structural formulas with keeping different structural features in mind in order to construct valid resonance structures (Nakhleh, 1992). Therefore, a predominant focus on singular (salient) structural features often leads to drawing mistakes (Cooper et al., 2010). Although familiarity and geometry may explain differences in the gaze distribution, fixations on structural features alone do not suffice to characterize productive and unproductive approaches when dealing with spatial complex molecules. For this reason, students’ explanations of their drawing approach need to be taken into account.
RQ 3: How does a student’s approach to visual selection to construct resonance structures relate to the productivity of the drawings?
Different visual selection approaches for the construction of resonance structures could be identified through students’ verbal explanations for their drawings, which differ regarding the information selection approach (knowledge-driven or structure-driven) and the flexibility of these approaches (centered or variable). Thus, four categories can be distinguished: structure-driven centered, structure-driven variable, knowledge-driven centered, and knowledge-driven variable (Figure 5). Figure 9 provides an overview of the absolute number of these approaches used for structure construction and the success rate for constructing a productive drawing. It can be derived from this table that about half of the drawings were constructed via a knowledge-driven variable approach, while only four drawings result from a structure-driven centered approach. Moreover, it can be seen that, contrary to our initial hypothesis, not a structure-driven or knowledge-driven approach, i.e., the explicit application of conceptual knowledge (Graulich et al., 2019), decides upon the productivity of the resulting drawing, but rather the flexibility of this approach, i.e., whether students focus on single structural features or take multiple structural features into account. At least 80% of the drawings with a variable approach are productive, whereas the amount of productive drawings reduces to under 30% if a centered approach has been used. In accordance with the results in the previous section, this shows that, besides being able to use resonance-related knowledge in the construction process (R-C factor), the success of constructing resonance structures heavily relies on students’ overall ability to decode the structural formulas (R-M factor) (Schönborn and Anderson, 2008, 2010). How these four approaches influence the construction of resonance structures and how they are related to the drawing moves, can be illustrated by taking a closer, exemplary look at descriptions of students’ drawing processes.
Nina, for instance, generated all her drawings for reaction A and B following a knowledge-driven centered approach (Figure 10), eventually resulting in unproductive structures, as the oxygen atom does not fulfill the octet rule, missing a positive charge on the oxygen atom in both cases. While expressing cluelessness about how to construct the resonance structures at first, Nina describes both her drawings by referring to experiences of delocalizing electrons of the oxygen atom as done in previous tasks. Therefore, the oxygen atom serves as an anchor guiding the drawing process and leading to multiple drawing moves, each starting from the oxygen atom and initiating the subsequent delocalization of the π-electrons of the double bond. As for the resonance structure in reaction A, she consequently misses a structure, which is, however, crucial for answering the task. Interestingly, while the initial positive charge remains unchanged in both structures, she creates a new, negative charge in each structure. The anchoring function of the oxygen atom at the extent of neglecting the other, more relevant structural features of the positive charge and double bond is also reflected in her attention distribution concerning the different structural features of the starting molecules in reaction A and B. Figure 10 shows that Nina spends about 70% of the fixation time on the methoxy group in reaction A. In reaction B, she almost exclusively (95%) focuses on the methoxy group, not paying attention to the double bond.

Figure 10. Nina’s drawing moves, fixation duration rates on the structural features and drawing description for her drawings in reaction A and B. The orange frame indicates the unproductivity of the resulting drawings.
A similar knowledge-driven centered approach can be seen in Catherine’s drawing process of the resonance structure in reaction A as she centers the drawing process description solely on the function of the oxygen atom (Figure 11). As Nina, Catherine explains her drawing approach by emphasizing that the oxygen atom must participate in generating resonance structures due to the lone pairs that could be delocalized. This utterance shows that Catherine employs an overgeneralized rule (McClary and Talanquer, 2011) guiding her drawing process and making the oxygen atom her starting point for the construction. Therefore, her prior knowledge, i.e., the R-C factor, mainly drives the interpretation of the structural formula and the constructing of the resulting resonance structure (Schönborn and Anderson, 2008). Similar to Nina, this results in multiple drawing moves involving the delocalization of electrons of the oxygen atom across the double bond to form another double bond. While the positive charge remains unchanged at the carbon atom, the oxygen atom does not carry a charge, thus, resulting in an unproductive drawing. However, Catherine’s fixation duration rate does not clearly reflect her drawing strategy (Figure 11). Despite spending much time on the methoxy group, Catherine also takes the double bond and positive charge into account, showing that she somewhat considered the whole molecule for the construction process.

Figure 11. Catherine’s drawing move, fixation duration rate on the structural features and drawing description for her drawing in reaction A. The orange frame indicates the unproductivity of the resulting drawing.
In contrast to these examples, Elizabeth has a structure-driven centered approach to the construction of the resonance structure in reaction B (Figure 12). Similar to the other students, she centers her drawing description only on the double bond as single structural feature after having tried to form a carbon–oxygen π-bond. Elizabeth’s approach illustrates a trial-and-error strategy (Ahmad and Omar, 1992), as she decides to delocalize the π-electrons of the double bond to the oxygen atom after struggling to move the electrons in the inverse direction. Although it becomes clear that she considers both structural features, as reflected in the fixation duration rates (Figure 12), she does not consider them in an interrelated manner but rather focuses on these structural features successively as singular entities. In Elizabeth’s case, this approach results in a single drawing move through which additional charges are generated, and the oxygen atoms clearly breaks the octet rule. Interestingly, neither in her description nor in the fixation duration rate, the positive charge gets much attention. Therefore, she does not see the positive charge as a prerequisite for resonance in this task, but rather approaches such drawing tasks by considering electron-rich features, as she stated: I guess lone pairs are also an indication for me that you can apply resonance, not only double bonds, but also lone pairs.

Figure 12. Elizabeth’s drawing move, fixation duration rate on the structural features and drawing description for her drawing in reaction B. The orange frame indicates the unproductivity of the resulting drawing.
So far, the centered approach shows that students justify their drawing processes by only referring to single structural features at the extent of neglecting other features within a molecule and considering single structural features as an anchor leading the entire drawing process. Many studies in STEM education research support our finding, that this anchoring function may be due to overgeneralized rules and heuristics, eventually causing algorithmic or arbitrary unproductive drawing steps and hindering the analysis of the given structure as a whole. In chemistry education, it has been shown that students are prone to focus on familiar and surface features, which eventually negatively impacts their problem solving (Kozma and Russell, 1997; Kraft et al., 2010; Anzovino and Bretz, 2015; Graulich and Bhattacharyya, 2017; Graulich et al., 2019) and supports their reliance on heuristics (McClary and Talanquer, 2011; Weinrich and Talanquer, 2015). Similarly, Inglis and Alcock (2012) have shown in the context of reading mathematical proofs that compared to mathematicians, undergraduate students spend more time focusing on surface features of an argument, i.e., they attend less to its logical structure.
In addition, these results may complement existing findings concerning the construction of structural formulas. As Ahmad and Omar (1992) and Cooper et al. (2010) stated, students often exhibit a trial-and-error approach or rely on memorized cues in drawing, which may result from the overreliance on singular structural features. Such a structural overreliance in the interpretation of structural representations (Schönborn and Anderson, 2008) may also offer a possible reason for the observed drawing difficulties of resonance structures reported by Betancourt-Pérez et al. (2010) and Petterson et al. (2020).
In contrast to the centered approach, the variable approach is characterized by a more holistic approach to the drawing process of different resonance structures. Students showing this approach take multiple, interrelated drawing features into account. For instance, consider Paula’s approach in reaction A (Figure 13).

Figure 13. Paula’s drawing moves, fixation duration rates on the structural features and drawing description for her drawings in reaction A. The green frame indicates the productivity of the resulting drawings.
Paula exhibits a structure-driven approach by merely referring to interacting structural features, i.e., the positive charge in both resonance structures and the electron-rich double bond or the lone pairs of the oxygen atom. However, in contrast to Elizabeth’s approach, she does not just focus on one feature, but sequentially considers smaller, interacting parts of the molecule which may aid in the delocalization of the positive charge. Therefore, as Figure 13 illustrates, this approach leads to single drawing moves delocalizing electron density toward an electron-deficient atom. This sequential approach is also reflected in Paula’s fixation duration rate, as she focuses most of the time on the positive charge and the double bond in the first resonance structure, and then, on the newly created positive charge and the methoxy group in the second structure.
Figure 9 illustrates that 35 of 60 drawings stem from a knowledge-driven variable approach, in which rules and concepts, such as stability or electronic effects, guided students’ drawing process. Phil’s sequential drawing process in reaction A and B exemplifies this approach. As Figure 14 depicts, each productive resonance structure results from a single drawing move, which shows the movement of electrons from an electron-rich source to an electron-deficient atom. In his verbalization of the drawing process, Phil repeatedly refers to the delocalization of the positive charge and stability as the driving force for the generation of each structure.
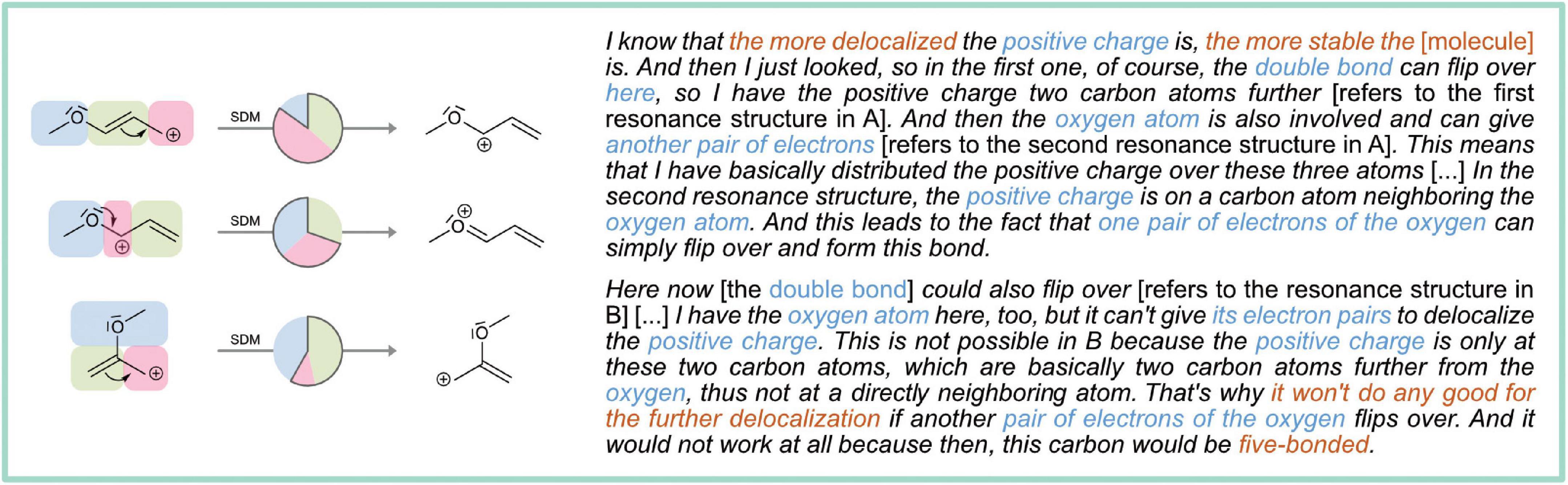
Figure 14. Phil’s drawing moves, fixation duration rates on the structural features and drawing description for his drawings in reaction A and B. The green frame indicates the productivity of the resulting drawings.
It can be inferred from Phil’s drawing approach that he centers his drawing moves around the positive charge. He takes a rather analytic, holistic approach by considering adjacent structural features and analyzing their contribution to the delocalization of the positive charge. Consider therefore the fixation duration rate during the construction process of the different resonance structures, exhibiting an attention distribution trend for the structures in reaction A (Figure 14). While Phil mainly fixates the double bond and the positive charge in the first resonance structure, his attention is drawn to the methoxy group and the positive charge in the second structure. In contrast to that, he centers his attention on the methoxy group and the double bond in the resonance structure in reaction B. Given that he verbalizes stability as the driving force for the construction of the structures, this gaze distribution may be a result of the analysis and weighing of the structural features and their influences on resonance. Therefore, Phil’s knowledge-driven variable approach shows that he reflects upon his drawing moves. This illustrates the need for the successful intertwined application of the different factors (R, C, M) in order to interpret a structural representation and subsequently construct another resonance structure (Schönborn and Anderson, 2008). This reflective drawing approach becomes even more apparent for Luke, another student who has a knowledge-driven variable approach. Concerning a resonance structure in A, he describes in detail the electronic effect of the oxygen atom and thereafter weighs the overall stability of the resulting resonance structure:
Then I thought, what does the oxygen atom do? The oxygen atom has a negative inductive effect, which is why the positive charge is intensified. But I thought that it can be neglected given the positive resonance effect, because the electron pair can be pushed toward the positive charge, and then, the positive charge would be here. I would say that is energetically not so favorable here with the positive charge on the oxygen, but it shows that the positive charge can be distributed relatively well.
Given that the gaze proportion of productive and unproductive drawings does not differ much in reaction B (cf. section “RQ 2: What structural features do students attend to in terms of attention distribution when translating one resonance structure into another and how is it related to students’ drawing moves?”), it may be inferred from these examples that students process the structural information differently by applying their related knowledge in different ways. While students with an unproductive drawing may tend to look at the given structure statically and apply thereon rules, for productive drawings, it may be the case that a higher gaze proportion indicates a more thorough weighing and reflection of its contribution to the drawing move. Hence, this inference strengthens Keig and Rubba’s (1993) finding that solving information-processing tasks (e.g., translations between structures) requires a thorough understanding (and thus application) of the underlying concept.
Altogether, it can be derived from these examples that students with a variable approach demonstrate more flexibility when constructing resonance structures by showing a more sequential, distributed attention to structural features, often resulting in productive single drawing moves. By being analytic in nature, they exhibit a more expert-like task approach (Stieff and Raje, 2008). By successively analyzing structural features and their relationship in terms of constructing a subsequent resonance structure, students consequently demonstrate both a local and global coherence formation (Seufert, 2003), altogether resulting in a holistic approach.
Conclusion and implications
This study is the first to explore in-depth students’ drawing processes in organic chemistry with the help of eye-tracking. It aimed at providing process-oriented insights into how students connect drawing-related information during the construction of resonance structures given students’ struggle with these representations. To this end, we analyzed in detail students’ transition patterns, the co-occurrence of fixated drawing elements during the construction process, students’ gaze proportion on structural features, and shed light on their visual selection approaches to navigating the drawing processes.
As the main results, we found that on a global level, students exhibit a similar gaze behavior concerning information retrieval and integration, irrespective of the productivity of their drawing, i.e., the same amount of information was used for the construction of resonance structures. However, the transition types distinguished a productive drawing from an unproductive drawing. While productive drawings result from more transitions between previous and target drawings, the construction of unproductive drawings is characterized by more transitions between target and unrelated drawings (RQ 1). Due to a predominant connection of previous and target drawings across all resonance structures, the analysis of the gaze proportion on structural features of the previous drawing revealed that productive structures are characterized by a higher sequential fixation on interrelated structural features. In contrast, a tendency of focusing single (unrelated) structural features emerged for unproductive drawings. However, this difference did not apply to resonance structures that were spatially more complex (i.e., a non-linear, branched molecule) (RQ 2). Finally, a qualitative look at students’ visual selection approaches, as reflected by students’ descriptions of drawing processes, showed that a variable approach underlies many productive drawings, i.e., an analytical approach, in which students attended to interrelated, relevant structural features. This is contrary to a centered approach, resulting in more unproductive drawings; here, students focused on singular structural features in a static manner (RQ 3). These findings bear different implications for both instruction and research.
Given that students with unproductive drawings often connect and focus on unrelated drawing elements, when, however, a holistic, analytical approach is required, instructional interventions should aim more at directing students’ attention, thus, supporting them to assess given information such as structural features, their relevance and their role within the construction of resonance structures (e.g., as possible starting points), therefore foster students’ ability to decode given representations (R-M factor) (Schönborn and Anderson, 2008, 2010). In this regard, possible instructional interventions could use process-oriented highlighting of interacting structural features, e.g., via tutorial videos (e.g., Rodemer et al., 2021). In this way, the learners’ attention could be better directed, as they can see and follow the actual drawing process instead of only seeing the static resonance structures as the final result on paper. As a possible intervention to externalize the viewing process, eye-movement-modeling examples could be used as worked examples (van Gog et al., 2009; Jarodzka et al., 2013). In addition, the results indicate that students’ conceptual knowledge of the resonance concept, specifically its flexible application, seems to be of great importance with respect to drawing productive resonance structures, thus, besides the R-M factor, emphasis should also be placed on the R-C factor (Schönborn and Anderson, 2008). Consequently, teaching algorithms how to draw such structures is not enough for building sustainable drawing skills. Instead, more effort should be put into addressing why certain drawing steps occur, e.g., what chemical concepts the drawing steps build upon. Creating such interventions would make students more reflective in assessing drawing moves. In turn, this could help students’ ability to flexibly decide when resonance applies and reduce their overreliance on heuristics and rules, such as searching for familiar or salient surface features.
As this study is explorative, more research in other contexts, with different task designs, and with more participants is needed to complement our findings and test whether our results can be confirmed. By finding little differences in eye movements while dealing with the more complex structure in reaction B, the construction of more complex structures deserves more attention. Due to their complexity and relevance, aromatic compounds are suitable for this purpose. However, more complex structures complicate the comparative analysis because, depending on the complexity, the number of differing drawing products increases. At the same time, the use of simpler aromatic compounds may lead students to proceed algorithmically. For instance, to investigate the differences in the information retrieval of drawing-related structural features, multiple choice tasks could be used, in which learners are asked to decide on a resulting structure based on an initial compound. As such, the comparative investigation of differing fixation distributions when choosing a productive or unproductive structure would be possible. Likewise, in such a setting, a stationary eye-tracker could be used, which provides more precise results regarding the fixation of single bonds and structural features.
By inferring underlying processes for previously documented drawing difficulties, this study adds to existing research by focusing on the context of the resonance concept. Furthermore, given its process-oriented character, this study adds to existing STEM-related drawing research in general. Despite previous research on the effects of drawing activities and the drawing products’ quality on learning outcomes, few research focused on the construction process underlying learners’ drawing products so far (Lobato et al., 2014). However, a process-oriented perspective can provide additional diagnostic insights into representation-related difficulties as the drawing processes can reveal learners’ scientific thoughts and conceptions (Lobato et al., 2014) as well as their unconscious actions when generating representations. As this study exemplified, the use of eye-tracking showed that, besides conceptual knowledge, students’ ability to construct productive scientific representations crucially depends on their competence to decode and manipulate such representations. As such, this methodology could be also applied in other contexts and STEM disciplines to reveal cognitive processes underlying the generation of representations, e.g., what features learners (unconsciously) use and integrate to depict submicroscopic processes in biology or to construct diagrams in physics, and whether the use of specific features or drawing elements influences the overall drawing quality. Eye-tracking could also be used in other contexts in organic chemistry, such as observing learners’ stepwise construction of mechanisms and the use of the electron-pushing formalism (e.g., Bhattacharyya and Bodner, 2005; Grove et al., 2012; Flynn and Featherstone, 2017), or observing the order and linearity of the diagram construction depending on the learners’ expertise. Although our work yields first process-oriented insights, further research across different scientific disciplines is needed to provide a comprehensive picture of students’ drawing approaches and difficulties for scientific representations.
Limitations
This study was meant to be exploratory in nature and aimed at offering insights into students’ drawing processes in organic chemistry. Therefore, some limitations with respect to the analysis and results must be considered. First, the small number of students (N = 20) and the focus on one task might limit the generalizability of the findings. Additionally, students were required to construct only two additional resonance structures in the first, and one structure in the second reaction, both dealing with rather simple structural formulas. Unlike in the first reaction, the construction of resonance structures did not build upon each other in the second reaction. Given these constraints, it remains unclear to which extent our findings apply to drawing processes of more complex structures. Furthermore, case comparisons might have motivated students to include and compare information of both reactions in their drawing process, since the given structures only differ in their connectivity. Thus, this variable may also have influenced students’ drawing processes, specifically on the integration of unrelated information. Concerning the interviews, the participants were prompted to describe their drawing process. However, it is possible that they used knowledge that they did not verbalize and which, thus, remained implicit.
Finally, technical limitations with respect to the mobile eye-tracker must be considered. The eye-tracker exhibits technical measurement inaccuracies due to, among others, a variable field of view, movement of the head, and the slippage of the glasses as a result of a longer measurement period. These inaccuracies can affect the results and the data analysis (e.g., requiring the correction of systematic gaze point offset by adjusting the AOIs).
Data availability statement
The datasets presented in this article cannot be made openly available because of the privacy policy stated in the participants’ consent form. Requests to access the datasets should be directed to the corresponding author NG, Nicole.Graulich@didaktik.chemie.uni-giessen.de.
Ethics statement
Ethical review and approval was not required for the study on human participants in accordance with the local legislation and institutional requirements. The participants provided their written informed consent to participate in this study. Written informed consent was obtained from the individual(s) for the publication of any potentially identifiable images or data included in this article.
Author contributions
IB designed the study with the help of NG, collected the data, and wrote the manuscript. IB and AL analyzed the data. IB, AL, and NG reviewed, edited, and discussed the manuscript and the analyses. All authors contributed to the article and approved the submitted version.
Funding
This work was funded in part by the National Science Foundation (DRL-2100320, DRL-2201723, and DRL-2225240), the Wisconsin Alumni Research Foundation, and the Office of the Vice Chancellor for Research and Graduate Education at the University of Wisconsin-Madison. The opinions, findings, and conclusions do not reflect the views of the funding agencies, cooperating institutions, or other individuals.
Acknowledgments
This publication represents a component of the first author’s doctoral (Dr. rer. nat.) thesis in the Faculty of Biology and Chemistry at the Justus Liebig University Giessen, Germany. We thank all students who participated in the study as well as the members of the Graulich Research Group for fruitful discussions about the research. We are grateful to Ramon Buchczyk and Helena Rist for support in data analysis. In the study, we utilized the Epistemic network analysis (ENA) method.
Conflict of interest
The authors declare that the research was conducted in the absence of any commercial or financial relationships that could be construed as a potential conflict of interest.
Publisher’s note
All claims expressed in this article are solely those of the authors and do not necessarily represent those of their affiliated organizations, or those of the publisher, the editors and the reviewers. Any product that may be evaluated in this article, or claim that may be made by its manufacturer, is not guaranteed or endorsed by the publisher.
References
Ahmad, W.-Y., and Omar, S. (1992). Drawing Lewis structures: A step-by-step approach. J. Chem. Educ. 69, 791–792. doi: 10.1021/ed069p791
Ainsworth, S. (2006). DeFT: A conceptual framework for considering learning with multiple representations. Learn. Instr. 16, 183–198. doi: 10.1016/j.learninstruc.2006.03.001
Ainsworth, S., Prain, V., and Tytler, R. (2011). Drawing to learn in science. Science 333, 1096–1097. doi: 10.1126/science.1204153
Ainsworth, S. E., and Scheiter, K. (2021). Learning by drawing visual representations: Potential, purposes, and practical implications. Curr. Dir. Psychol. Sci. 30, 61–67. doi: 10.1177/0963721420979582
Anderson, B. A. (2013). A value-driven mechanism of attentional selection. J. Vis. 13, 1–16. doi: 10.1167/13.3.7
Andrist, S., Collier, W., Gleicher, M., Mutlu, B., and Shaffer, D. (2015). Look together: Analyzing gaze coordination with epistemic network analysis. Front. Psychol. 6:1016. doi: 10.3389/fpsyg.2015.01016
Anzovino, M. E., and Bretz, S. L. (2015). Organic chemistry students’ ideas about nucleophiles and electrophiles: The role of charges and mechanisms. Chem. Educ. Res. Pract. 16, 797–810. doi: 10.1039/C5RP00113G
Awh, E., Belopolsky, A. V., and Theeuwes, J. (2012). Top-down versus bottom-up attentional control: A failed theoretical dichotomy. Trends Cogn. Sci. 16, 437–443. doi: 10.1016/j.tics.2012.06.010
Baluyut, J. Y., and Holme, T. A. (2019). Eye tracking student strategies for solving stoichiometry problems involving particulate nature of matter diagrams. Chem. Teach. Int. 1, 1–13. doi: 10.1515/cti-2018-0003
Betancourt-Pérez, R., Olivera, L. J., and Rodríguez, J. E. (2010). Assessment of organic chemistry students’ knowledge of resonance-related structures. J. Chem. Educ. 87, 547–551. doi: 10.1021/ed800163g
Bhattacharyya, G., and Bodner, G. M. (2005). “It gets me to the product”: How students propose organic mechanisms. J. Chem. Educ. 82, 1402–1407. doi: 10.1021/ed082p1402
Bobek, E., and Tversky, B. (2016). Creating visual explanations improves learning. Cogn. Res. Princ. Implic. 1:27. doi: 10.1186/s41235-016-0031-6
Bodner, G. M., and Domin, D. S. (2000). Mental models: The role of representations in problem solving in chemistry. Univ. Chem. Educ. 4, 24–30.
Brandfonbrener, P. B., Watts, F. M., and Shultz, G. V. (2021). Organic chemistry students’ written descriptions and explanations of resonance and its influence on reactivity. J. Chem. Educ. 98, 3431–3441. doi: 10.1021/acs.jchemed.1c00660
Brennan, R. L., and Prediger, D. J. (1981). Coefficient kappa: Some uses, misuses, and alternatives. Educ. Psychol. Meas. 41, 687–699. doi: 10.1177/001316448104100307
Bruckner, S., Schneider, J., Zlatkin-Troitschanskaia, O., and Drachsler, H. (2020). Epistemic network analyses of economics students’ graph understanding: An eye-tracking study. Sensors 20, 1–33. doi: 10.3390/s20236908
Carle, M. S., and Flynn, A. B. (2020). Essential learning outcomes for delocalization (resonance) concepts: How are they taught, practiced, and assessed in organic chemistry? Chem. Educ. Res. Pract. 21, 622–637. doi: 10.1039/C9RP00203K
Cartrette, D. P., and Bodner, G. M. (2009). Non-mathematical problem solving in organic chemistry. J. Res. Sci. Teach. 47, 643–660. doi: 10.1002/tea.20306
Connor, M. C., Glass, B. H., Finkenstaedt-Quinn, S. A., and Shultz, G. V. (2021). Developing expertise in (1)H NMR spectral interpretation. J. Org. Chem. 86, 1385–1395. doi: 10.1021/acs.joc.0c01398
Cooper, M. M., Grove, N., Underwood, S. M., and Klymkowsky, M. W. (2010). Lost in Lewis structures: An investigation of student difficulties in developing representational competence. J. Chem. Educ. 87, 869–874. doi: 10.1021/ed900004y
Cooper, M. M., Stieff, M., and DeSutter, D. (2017). Sketching the invisible to predict the visible: From drawing to modeling in chemistry. Top. Cogn. Sci. 9, 902–920. doi: 10.1111/tops.12285
Cooper, M. M., Underwood, S. M., Hilley, C. Z., and Klymkowsky, M. W. (2012). Development and assessment of a molecular structure and properties learning progression. J. Chem. Educ. 89, 1351–1357. doi: 10.1021/ed300083a
Cox, R. (1999). Representation construction, externalised cognition and individual differences. Learn. Instr. 9, 343–363. doi: 10.1016/S0959-4752(98)00051-6
Cromley, J. G., Du, Y., and Dane, A. P. (2019). Drawing-to-learn: Does meta-analysis show differences between technology-based drawing and paper-and-pencil drawing? J. Sci. Educ. Technol. 29, 216–229. doi: 10.1007/s10956-019-09807-6
Cullipher, S., Hansen, S. J., and VandenPlas, J. R. (2018). “Eye tracking as a research tool: An introduction,” in Eye tracking for the chemistry education researcher, eds J. R. VandenPlas, S. J. R. Hansen, and S. Cullipher (Washington, DC: ACS Publications), 1–9. doi: 10.1021/bk-2018-1292.ch001
Cullipher, S., and VandenPlas, J. R. (2018). “Using fixations to measure attention,” in Eye tracking for the chemistry education researcher, eds J. R. VandenPlas, S. J. R. Hansen, and S. Cullipher (Washington, DC: ACS Publications), 53–72. doi: 10.1021/bk-2018-1292.ch004
DeFever, R. S., Bruce, H., and Bhattacharyya, G. (2015). Mental rolodexing: Senior chemistry majors’ understanding of chemical and physical properties. J. Chem. Educ. 92, 415–426. doi: 10.1021/ed500360g
Dood, A. J., and Watts, F. M. (2022). Mechanistic reasoning in organic chemistry: A scoping review of how students describe and explain mechanisms in the chemistry education research literature. J. Chem. Educ. 99, 2864–2876. doi: 10.1021/acs.jchemed.2c00313
Duis, J. M. (2011). Organic chemistry educators’ perspectives on fundamental concepts and misconceptions: An exploratory study. J. Chem. Educ. 88, 346–350. doi: 10.1021/ed1007266
Fiorella, L., Stull, A. T., Kuhlmann, S., and Mayer, R. E. (2020). Fostering generative learning from video lessons: Benefits of instructor-generated drawings and learner-generated explanations. J. Educ. Psychol. 112, 895–906. doi: 10.1037/edu0000408
Fiorella, L., and Zhang, Q. (2018). Drawing boundary conditions for learning by drawing. Educ. Psychol. Rev. 30, 1115–1137. doi: 10.1007/s10648-018-9444-8
Flynn, A. B., and Featherstone, R. B. (2017). Language of mechanisms: Exam analysis reveals students’ strengths, strategies, and errors when using the electron-pushing formalism (curved arrows) in new reactions. Chem. Educ. Res. Pract. 18, 64–77. doi: 10.1039/c6rp00126b
Gegenfurtner, A., Lehtinen, E., and Säljö, R. (2011). Expertise differences in the comprehension of visualizations: A meta-analysis of eye-tracking research in professional domains. Educ. Psychol. Rev. 23, 523–552. doi: 10.1007/s10648-011-9174-7
Gilbert, J. K. (2005). “Visualization: A metacognitive skill in science and science education,” in Visualization in Science Education, ed. J. K. Gilbert (New York, NY: Springer), 9–27.
Gobert, J. D., and Clement, J. J. (1999). Effects of student-generated diagrams versus student-generated summaries on conceptual understanding of causal and dynamic knowledge in plate tectonics. J. Res. Sci. Teach. 36, 39–53.
Goodwin, W. M. (2008). Structural formulas and explanation in organic chemistry. Found. Chem. 10, 117–127. doi: 10.1007/s10698-007-9033-2
Graulich, N. (2015). The tip of the iceberg in organic chemistry classes: How do students deal with the invisible? Chem. Educ. Res. Pract. 16, 9–21. doi: 10.1039/C4RP00165F
Graulich, N., and Bhattacharyya, G. (2017). Investigating students’ similarity judgments in organic chemistry. Chem. Educ. Res. Pract. 18, 774–784. doi: 10.1039/C7RP00055C
Graulich, N., Hedtrich, S., and Harzenetter, R. (2019). Explicit versus implicit similarity–exploring relational conceptual understanding in organic chemistry. Chem. Educ. Res. Pract. 20, 924–936. doi: 10.1039/C9RP00054B
Graulich, N., and Schween, M. (2018). Concept-oriented task design: Making purposeful case comparisons in organic chemistry. J. Chem. Educ. 95, 376–383. doi: 10.1021/acs.jchemed.7b00672
Grove, N. P., Cooper, M. M., and Rush, K. M. (2012). Decorating with arrows: Toward the development of representational competence in organic chemistry. J. Chem. Educ. 89, 844–849. doi: 10.1021/ed2003934
Havelková, L., and Gołębiowska, I. M. (2019). What went wrong for bad solvers during thematic map analysis? Lessons learned from an eye-tracking study. ISPRS Int. J. Geo Inf. 9:9. doi: 10.3390/ijgi9010009
Hejnová, E., and Kekule, M. (2018). Observing students’ problem solving strategies in mechanics by eye-tracking method. Sci. Educ. 9, 102–116. doi: 10.14712/18047106.1018
Hellenbrand, J., Mayer, R. E., Opfermann, M., Schmeck, A., and Leutner, D. (2019). How generative drawing affects the learning process: An eye-tracking analysis. Appl. Cogn. Psychol. 33, 1147–1164. doi: 10.1002/acp.3559
Holmqvist, K., Andrà, C., Lindström, P., Arzarello, F., Ferrara, F., Robutti, O., et al. (2011). A method for quantifying focused versus overview behavior in AOI sequences. Behav. Res. Methods 43, 987–998. doi: 10.3758/s13428-011-0104-x
Inglis, M., and Alcock, L. (2012). Expert and novice approaches to reading mathematical proofs. J. Res. Math. Educ. 43, 358–390. doi: 10.5951/jresematheduc.43.4.0358
Jarodzka, H., Scheiter, K., Gerjets, P., and van Gog, T. (2010). In the eyes of the beholder: How experts and novices interpret dynamic stimuli. Learn. Instr. 20, 146–154. doi: 10.1016/j.learninstruc.2009.02.019
Jarodzka, H., van Gog, T., Dorr, M., Scheiter, K., and Gerjets, P. (2013). Learning to see: Guiding students’ attention via a model’s eye movements fosters learning. Learn. Instr. 25, 62–70. doi: 10.1016/j.learninstruc.2012.11.004
Johnson, C. I., and Mayer, R. E. (2012). An eye movement analysis of the spatial contiguity effect in multimedia learning. J. Exp. Psychol. Appl. 18, 178–191. doi: 10.1037/a0026923
Johnstone, A. H. (1991). Why is science difficult to learn? Things are seldom what they seem. J. Comput. Assist. Learn. 7, 75–83. doi: 10.1111/j.1365-2729.1991.tb00230.x
Just, M. A., and Carpenter, P. A. (1980). A theory of reading: From eye fixations to comprehension. Psychol. Rev. 87, 329–354. doi: 10.1037/0033-295X.87.4.329
Karonen, M., Murtonen, M., Södervik, I., Manninen, M., and Salomäki, M. (2021). Heuristics hindering the development of understanding of molecular structures in university level chemistry education: The Lewis structure as an example. Educ. Sci. 11:258. doi: 10.3390/educsci11060258
Kaufmann, I., Hamza, K. M., Rundgren, C.-J., and Eriksson, L. (2017). Developing an approach for teaching and learning about Lewis structures. Int. J. Sci. Educ. 39, 1601–1624. doi: 10.1080/09500693.2017.1342053
Keig, P. F., and Rubba, P. A. (1993). Translation of representations of the structure of matter and its relationship to reasoning, gender, spatial reasoning, and specific prior knowledge. J. Res. Sci. Teach. 30, 883–903. doi: 10.1002/tea.3660300807
Kim, T., Wright, L. K., and Miller, K. (2019). An examination of students’ perceptions of the Kekulé resonance representation using a perceptual learning theory lens. Chem. Educ. Res. Pract. 20, 659–666. doi: 10.1039/C9RP00009G
Kozma, R., Chin, E., Russell, J., and Marx, N. (2000). The roles of representations and tools in the chemistry laboratory and their implications for chemistry learning. J. Learn. Sci. 9, 105–143. doi: 10.1207/s15327809jls0902_1
Kozma, R., and Russell, J. (2005). “Students becoming chemists: Developing representational competence,” in Visualization in Science Education, ed. J. K. Gilbert (New York, NY: Springer), 121–145.
Kozma, R. B., and Russell, J. (1997). Multimedia and understanding: Expert and novice responses to different representations of chemical phenomena. J. Res. Sci. Teach. 34, 949–968.
Kraft, A., Strickland, A. M., and Bhattacharyya, G. (2010). Reasonable reasoning: Multi-variate problem-solving in organic chemistry. Chem. Educ. Res. Pract. 11, 281–292. doi: 10.1039/C0RP90003F
Kuckartz, U., and Rädiker, S. (2019). Analyzing Qualitative Data with MAXQDA. New York, NY:Springer.
Leopold, C., and Leutner, D. (2012). Science text comprehension: Drawing, main idea selection, and summarizing as learning strategies. Learn. Instr. 22, 16–26. doi: 10.1016/j.learninstruc.2011.05.005
Lesh, R., Post, T. R., and Behr, M. (1987). “Representations and translations among representations in mathematics learning and problem solving,” in Problems of Representations in the Teaching and Learning of Mathematics, ed. C. Janvier (Hillsdale, NJ: Lawrence Erlbaum), 33–40.
Lobato, J., Hohensee, C., and Diamond, J. M. (2014). What can we learn by comparing students’ diagram-construction processes with the mathematical conceptions inferred from their explanations with completed diagrams? Math. Educ. Res. J. 26, 607–634. doi: 10.1007/s13394-013-0106-3
Malone, S., Altmeyer, K., Vogel, M., and Brünken, R. (2020). Homogeneous and heterogeneous multiple representations in equation-solving problems: An eye-tracking study. J. Comput. Assist. Learn. 36, 781–798. doi: 10.1111/jcal.12426
Maries, A., and Singh, C. (2018). Do students benefit from drawing productive diagrams themselves while solving introductory physics problems? The case of two electrostatics problems. Eur. J. Phys. 39:015703. doi: 10.1088/1361-6404/aa9038
Mason, L., Lowe, R., and Tornatora, M. C. (2013). Self-generated drawings for supporting comprehension of a complex animation. Contemp. Educ. Psychol. 38, 211–224. doi: 10.1016/j.cedpsych.2013.04.001
Mayer, R. E. (2005). “Cognitive theory of multimedia learning,” in The Cambridge Handbook of Multimedia Learning, ed. R. E. Mayer (Cambridge, MA: Cambridge University Press), 31–48.
McClary, L., and Talanquer, V. (2011). Heuristic reasoning in chemistry: Making decisions about acid strength. Int. J. Sci. Educ. 33, 1433–1454. doi: 10.1080/09500693.2010.528463
Nakhleh, M. B. (1992). Why some students don’t learn chemistry: Chemical misconceptions. J. Chem. Educ. 69, 191–196. doi: 10.1021/ed069p191
Nitz, S., Ainsworth, S. E., Nerdel, C., and Prechtl, H. (2014). Do student perceptions of teaching predict the development of representational competence and biological knowledge? Learn. Instr. 31, 13–22. doi: 10.1016/j.learninstruc.2013.12.003
Olimpo, J. T., Kumi, B. C., Wroblewski, R., and Dixon, B. L. (2015). Examining the relationship between 2D diagrammatic conventions and students’ success on representational translation tasks in organic chemistry. Chem. Educ. Res. Pract. 16, 143–153. doi: 10.1039/C4RP00169A
Ott, N., Brünken, R., Vogel, M., and Malone, S. (2018). Multiple symbolic representations: The combination of formula and text supports problem solving in the mathematical field of propositional logic. Learn. Instr. 58, 88–105. doi: 10.1016/j.learninstruc.2018.04.010
Petterson, M. N., Watts, F. M., Snyder-White, E. P., Archer, S. R., Shultz, G. V., and Finkenstaedt-Quinn, S. A. (2020). Eliciting student thinking about acid–base reactions via app and paper–pencil based problem solving. Chem. Educ. Res. Pract. 21, 878–892. doi: 10.1039/C9RP00260J
Quillin, K., and Thomas, S. (2015). Drawing-to-learn: A framework for using drawings to promote model-based reasoning in biology. CBE Life Sci. Educ. 14:es2. doi: 10.1187/cbe.14-08-0128
Rellensmann, J., Schukajlow, S., and Leopold, C. (2016). Make a drawing. Effects of strategic knowledge, drawing accuracy, and type of drawing on students’ mathematical modelling performance. Educ. Stud. Math. 95, 53–78. doi: 10.1007/s10649-016-9736-1
Richardson, W. (1986). Teaching the concept of resonance with transparent overlays. J. Chem. Educ. 63, 518–519. doi: 10.1021/ed063p518
Rodemer, M., Eckhard, J., Graulich, N., and Bernholt, S. (2020). Decoding case comparisons in organic chemistry: Eye-tracking students’ visual behavior. J. Chem. Educ. 97, 3530–3539. doi: 10.1021/acs.jchemed.0c00418
Rodemer, M., Eckhard, J., Graulich, N., and Bernholt, S. (2021). Connecting explanations to representations: Benefits of highlighting techniques in tutorial videos on students’ learning in organic chemistry. Int. J. Sci. Educ. 43, 2707–2728. doi: 10.1080/09500693.2021.1985743
Rosenthal, R. (1984). Meta-Analytic Procedures for Social Research. Newbury Park, CA: Sage Publications.
Sandi-Urena, S., Cambronero, G. L., and Chaves, D. J. (2020). Conceptualisation of Lewis structures by chemistry majors. Chem. Teach. Int. 2, 1–9. doi: 10.1515/cti-2018-0019
Schmeck, A., Mayer, R. E., Opfermann, M., Pfeiffer, V., and Leutner, D. (2014). Drawing pictures during learning from scientific text: Testing the generative drawing effect and the prognostic drawing effect. Contemp. Educ. Psychol. 39, 275–286. doi: 10.1016/j.cedpsych.2014.07.003
Schmidgall, S. P., Scheiter, K., and Eitel, A. (2020). Can we further improve tablet-based drawing to enhance learning? An empirical test of two types of support. Instr. Sci. 48, 453–474. doi: 10.1007/s11251-020-09513-6
Schmidt-Weigand, F., Kohnert, A., and Glowalla, U. (2010). A closer look at split visual attention in system- and self-paced instruction in multimedia learning. Learn. Instr. 20, 100–110. doi: 10.1016/j.learninstruc.2009.02.011
Schönborn, K. J., and Anderson, T. R. (2008). A model of factors determining students’ ability to interpret external representations in biochemistry. Int. J. Sci. Educ. 31, 193–232. doi: 10.1080/09500690701670535
Schönborn, K. J., and Anderson, T. R. (2010). Bridging the educational research-teaching practice gap: Foundations for assessing and developing biochemistry students’ visual literacy. Biochem. Mol. Biol. Educ. 38, 347–354. doi: 10.1002/bmb.20436
Schwamborn, A., Mayer, R. E., Thillmann, H., Leopold, C., and Leutner, D. (2010). Drawing as a generative activity and drawing as a prognostic activity. J. Educ. Psychol. 102, 872–879. doi: 10.1037/a0019640
Seufert, T. (2003). Supporting coherence formation in learning from multiple representations. Learn. Instr. 13, 227–237. doi: 10.1016/S0959-4752(02)00022-1
Seufert, T., and Brünken, R. (2006). Cognitive load and the format of instructional aids for coherence formation. Appl. Cogn. Psychol. 20, 321–331. doi: 10.1002/acp.1248
Shaffer, D. W., Collier, W., and Ruis, A. R. (2016). A tutorial on epistemic network analysis: Analyzing the structure of connections in cognitive, social, and interaction data. J. Learn. Anal. 3, 9–45. doi: 10.18608/jla.2016.33.3
Shaffer, D. W., and Ruis, A. R. (2017). “Epistemic network analysis: A worked example of theory-based learning analytics,” in Handbook of Learning Analytics, eds C. Lang, G. Siemens, A. Wise, and D. Gašević (New York, NY: Society for Learning Analytics Research), 175–187. doi: 10.18608/hla17.015
Shapiro, S. S., and Wilk, M. B. (1965). An analysis of variance test for normality (complete samples). Biometrika 52, 591–611. doi: 10.2307/2333709
Stieff, M., and DeSutter, D. (2020). Sketching, not representational competence, predicts improved science learning. J. Res. Sci. Teach. 58, 128–156. doi: 10.1002/tea.21650
Stieff, M., and Raje, S. (2008). “Expertise and spatial reasoning in advanced scientific problem solving,” in International Perspectives in the Learning Sciences: Creating a learning world. Proceedings of the Eighth International Conference for the Learning Sciences ICLS, eds G. Kanselaar, V. Jonker, P. A. Kirschner, and F. J. Prins (Utrecht: The Netherlands: International Society of the Learning Sciences), 366–373.
Strickland, A. M., Kraft, A., and Bhattacharyya, G. (2010). What happens when representations fail to represent? Graduate students’ mental models of organic chemistry diagrams. Chem. Educ. Res. Pract. 11, 293–301. doi: 10.1039/C0RP90009E
Taber, K. S. (2002). Compounding quanta: Probing the frontiers of student understanding of molecular orbitals. Chem. Educ. Res. Pract. 3, 159–173. doi: 10.1039/B2RP90013K
Tang, H., Day, E. L., Atkinson, M. B., and Pienta, N. J. (2018). GrpString: An R package for analysis of groups of strings. R J. 10, 359–369. doi: 10.32614/RJ-2018-002
Tang, H., Kirk, J., and Pienta, N. J. (2014). Investigating the effect of complexity factors in stoichiometry problems using logistic regression and eye tracking. J. Chem. Educ. 91, 969–975. doi: 10.1021/ed4004113
Tang, H., and Pienta, N. (2012). Eye-tracking study of complexity in gas law problems. J. Chem. Educ. 89, 988–994. doi: 10.1021/ed200644k
Theeuwes, J. (2019). Goal-driven, stimulus-driven, and history-driven selection. Curr. Opin. Psychol. 29, 97–101. doi: 10.1016/j.copsyc.2018.12.024
Tiettmeyer, J. M., Coleman, A. F., Balok, R. S., Gampp, T. W., Duffy, P. L., Mazzarone, K. M., et al. (2017). Unraveling the complexities: An investigation of the factors that induce load in chemistry students constructing Lewis structures. J. Chem. Educ. 94, 282–288. doi: 10.1021/acs.jchemed.6b00363
Topczewski, J. J., Topczewski, A. M., Tang, H., Kendhammer, L. K., and Pienta, N. J. (2016). NMR spectra through the eyes of a student: Eye tracking applied to NMR items. J. Chem. Educ. 94, 29–37. doi: 10.1021/acs.jchemed.6b00528
van Gog, T., Jarodzka, H., Scheiter, K., Gerjets, P., and Paas, F. (2009). Attention guidance during example study via the model’s eye movements. Comput. Hum. Behav. 25, 785–791. doi: 10.1016/j.chb.2009.02.007
Van Meter, P., and Garner, J. (2005). The promise and practice of learner-generated drawing: Literature review and synthesis. Educ. Psychol. Rev. 17, 285–325. doi: 10.1007/s10648-005-8136-3
Weinrich, M., and Talanquer, V. (2015). Mapping students’ conceptual modes when thinking about chemical reactions used to make a desired product. Chem. Educ. Res. Pract. 16, 561–577. doi: 10.1039/C5RP00024F
Wu, S. P., and Rau, M. A. (2019). How students learn content in science, technology, engineering, and mathematics (STEM) through drawing activities. Educ. Psychol. Rev. 31, 87–120. doi: 10.1007/s10648-019-09467-3
Keywords: eye-tracking, organic chemistry, drawing, resonance, chemistry education research (CER), undergraduate, molecular structures
Citation: Braun I, Langner A and Graulich N (2022) Let’s draw molecules: Students’ sequential drawing processes of resonance structures in organic chemistry. Front. Educ. 7:1055280. doi: 10.3389/feduc.2022.1055280
Received: 27 September 2022; Accepted: 21 November 2022;
Published: 09 December 2022.
Edited by:
Pascal Klein, University of Göttingen, GermanyReviewed by:
Sandra Gudino Paredes, Monterrey Institute of Technology and Higher Education, MexicoDihua Xue, University of Minnesota Rochester, United States
Solaire Finkenstaedt-Quinn, University of Michigan, United States
Copyright © 2022 Braun, Langner and Graulich. This is an open-access article distributed under the terms of the Creative Commons Attribution License (CC BY). The use, distribution or reproduction in other forums is permitted, provided the original author(s) and the copyright owner(s) are credited and that the original publication in this journal is cited, in accordance with accepted academic practice. No use, distribution or reproduction is permitted which does not comply with these terms.
*Correspondence: Nicole Graulich, Nicole.Graulich@didaktik.chemie.uni-giessen.de