- Department of Human Sciences and Crane Center for Early Childhood Research and Policy, The Ohio State University, Columbus, OH, United States
Introduction: Children who are absent from school, including preschool, do not make the same academic gains as their non-absent peers. However, we know little about what predicts absenteeism among preschool-attending children.
Methods: We used the Family and Child Experiences Study - 2009, a nationally representative sample of Head Start attendees (n = 2,842), to test the associations between a comprehensive set of child, family, and center factors, and children’s levels of absenteeism across the preschool year.
Results: Our findings highlight the multi-faceted nature of absenteeism. Family necessity, family routines, and center-level characteristics were all associated with absenteeism.
Discussion: Reducing preschool absenteeism requires a comprehensive approach as the factors that shape absences are varied. Our findings suggest that center-level strategies focused on outreach and classroom quality are important future directions.
Introduction
Preschool is an effective means to improving children’s early learning and development, especially for children from low-income homes (Phillips et al., 2017). Given the mounting evidence supporting the benefits of preschool, large investments are being made into these programs across the country (Duncan and Magnuson, 2013). Despite these potential benefits of preschool enrollment, there is growing evidence to suggest that children do not reap the maximum benefit if they are not regularly present in school (Connolly and Olson, 2012; Ansari and Purtell, 2018; Ehrlich et al., 2018; Fuhs et al., 2018; Rhoad-Drogalis and Justice, 2018; Ansari et al., 2021). However, there has been little work on understanding why children are absent in the earliest years of schooling.
To address this gap in scientific knowledge, we use a nationally representative sample of newly enrolled Head Start attendees to examine a comprehensive set of factors that we hypothesize will be associated with children’s preschool absences. Understanding why children are absent in Head Start is an important policy question because it is the largest federally funded preschool program in the U.S., serving over 1 million children from low-income homes in 2019 alone (Office of Head Start, Administration for Children and Families, 2016). Head Start was created in 1965 as part of President Johnson’s War on Poverty with the goal of minimizing the socioeconomic disparities in children’s achievement. Interestingly, Head Start was designed as a two-generation program, meaning that it targeted both children and their parents (Zigler and Styfco, 2000). Thus, understanding how the family and school systems shape absenteeism in Head Start is particularly important.
There is far less information available on the prevalence of absenteeism in preschool than formal schooling, but studies from Baltimore (Connolly and Olson, 2012) and Chicago (Ehrlich et al., 2018) suggest that absenteeism is especially high during the years leading up to kindergarten. For example, in Chicago, preschoolers were absent for roughly 10–13% of the school year (Ehrlich et al., 2018). These averages indicate that a large share of children were chronically absent, meaning they missed more than 10% of the school year. Results from preschool programs in Baltimore reveal even higher levels of chronic absenteeism, with almost 27% of children being chronically absent (Connolly and Olson, 2012). These high rates of absences is troublesome because: (a) children who are absent from preschool do not make the same academic gains as their classmates who are less frequently absent (Connolly and Olson, 2012; Ansari and Purtell, 2018; Ehrlich et al., 2018; Fuhs et al., 2018; Rhoad-Drogalis and Justice, 2018); and (b) the more often children are absent in the early years, the more likely they were to be absent later on (Connolly and Olson, 2012; Dubay and Hollar, 2016; Gottfried, 2017; Ansari and Pianta, 2019). But, overall, these findings are not entirely surprising; the K-12 literature has long highlighted the negative educational and financial implications of school absences (Gottfried, 2009, 2010, 2011; Ready, 2010; Gershenson et al., 2015; Gottfried and Hutt, 2019).
To understand the consequences of absenteeism, and eventually to develop effective solutions to reduce it, requires a deeper understanding of the barriers to school attendance and which children are more likely to miss time from school. And even though there has been recent advances in trying to understand “why” children are absent in K-12 (e.g., Gottfried, 2015, 2017; Morrissey et al., 2014; Gottfried and Gee, 2017), there has been little effort to understand these dynamics among preschool-aged children. This is an important gap in knowledge because preschool differs from formal schooling in many ways that may contribute to both the higher rates of absenteeism and the reasons why children are absent.
Primarily, preschool in the United States. is not mandated by law and because of its voluntary nature, some parents may view its role in promoting their children’s development differently than formal schooling. Indeed, while most parents believe that school attendance is important, there is variation in whether parents ascribe these beliefs in relation to preschool, or only when their children are older (Ehrlich et al., 2013). The potentially more varied beliefs about preschool may be one reason why there are higher levels of absences in preschool than later schooling (Dubay and Holla, 2015).
When studying absenteeism, children’s health is often considered as the primary contributor to absenteeism (Ehrlich et al., 2013; however, there are multiple pathways that may lead to children missing school, especially in early childhood when schooling is not mandatory. Two theoretical models help to navigate this complex process. First, Bronfenbrenner’s bioecological theory highlights the importance of multiple contexts in shaping children’s development (Bronfenbrenner and Morris, 2006). Two tenets of this theory are particularly relevant. First, the concept of the mesosystem, which focuses on interrelations across contexts, highlights the interrelatedness of family and school environments. Applied here, it may be that experiences in the home prevent (or promote) children’s preschool attendance. Second, this framework also emphasizes the role of the child in shaping their own home and school experiences. Thus, it may be that when a child (or parent) has positive experiences at preschool, they may be more motivated to go (or send their child), and in turn, reduce absenteeism.
The accommodations framework (Meyers and Jordan, 2006) also provides a useful lens through which to understand children’s school absences. This economic framework was initially developed to explain how families make choices about childcare for their children and highlights the complex web of factors that influence these choices. Many of these factors may also influence preschool attendance. For example, need and necessity are highlighted as key factors that shape parents’ decisions for childcare. When applied here, it may be that families with working parents have children with fewer absences because they need childcare so they can work; however, for families with mismatches between employment and preschool hours, absenteeism may be higher. This framework also highlights the importance of norms and values in parental decisions surrounding childcare. As discussed earlier, whether an individual parent values preschool in the same way society values later schooling is likely to have important implications for how likely they are to allow their child to miss extensive time from preschool.
Supporting these theories, the K-12 literature suggests that the reasons underlying children’s school absences are complex and cut across different layers of the home and school systems in addition to the communities in which families reside (e.g., Baker et al., 2001; Epstein and Sheldon, 2002; Morrissey et al., 2014; Gottfried, 2015). Although a number of these features are likely to be important at the preschool level, it is important to consider the specific factors relevant to preschool absences, especially in the context of Head Start. In addition to serving children from lower-income families, Head Start has a longstanding focus on parent engagement which may shape absenteeism patterns in unique ways. Given that Head Start is the largest federal preschool program, understanding predictors of absenteeism in this context is critical.
We ground the factors we examine in these theories, the Head Start context, and their policy relevance. Understanding how various factors shape absenteeism provides information that can be used to improve attendance in the future. We focus on both family- and classroom factors, as both are shaped by policy and practice. Although many of these factors have not been examined in the context of Head Start, we rely on research on absenteeism in elementary school to describe them below.
Family circumstance/necessity
Although children’s health has been found to predict higher levels of absenteeism during the elementary and secondary school years (Allensworth and Easton, 2007; Ready, 2010; Childs and Lofton, 2021), other mechanisms, similar to those described in the accommodations framework have also been considered. For example, because parents sometimes consider preschool to be childcare, and less of an educational opportunity, how frequently their child attends may be driven by how much they need childcare and how frequently their need for care overlaps with the hours the program is open. Thus, family factors such as the other adults in the home and maternal employment may be associated with absenteeism.
Family stress and routines
. Other family factors, including the levels of stress and chaos within the home may make it more difficult for children to consistently attend preschool, as these challenges may make it difficult for families to get their child to school. Alternatively, these families may have a greater need for out of home care and thus, limit the number of times their child is absent. Family poverty is a related factor that has been documented as a consistent predictor of absenteeism, in part due to the reasons discussed above, but also because it is associated with poor neighborhood conditions and community violence, which make it more difficult for families to get to school (Chen et al., 2000; Allensworth and Easton, 2007; Gottfried, 2010; Ansari and Gottfried, 2020).
Children’s academic and social-behavioral skills
In addition to the above family processes, another important dimension emphasized by bioecological theory includes the attributes and skills of children (Bronfenbrenner and Morris, 2006). These skills and behaviors can either support or impede children’s experiences, including their school attendance. For example, children who demonstrate low skills or problematic behaviors may signal to their parents that they need more help, and thus, reduce the likelihood of absenteeism (i.e., compensatory effects). On the other hand, children who demonstrate more optimal skills and behaviors may encourage parents to continue to invest in their education and, consequently, parents may take extra efforts to make sure their children are not absent from school (i.e., enrichment effects). Although both possibilities have received theoretical support, the evidence in the K-12 literature with respect to absenteeism has been mixed (e.g., Gottfried and Gee, 2017; Ansari et al., 2020).
Center and classroom processes
Despite the challenges faced by low-income families, the center itself also has the potential to influence the frequency of absenteeism. For example, centers that make an effort to meet with families or provide transportation and medical care may increase the ability of families to attend preschool regularly (e.g., Gottfried, 2013, 2017). Similarly, centers that make efforts to increase parents’ beliefs in the importance of preschool for their children’s future are also likely to reduce absenteeism (Ehrlich et al., 2013). Children’s relationships with their teacher are also important to their overall schooling experience (Crosnoe et al., 2004). If a child has a close relationship with their teacher, and more generally, positive experiences within the classroom, they may be more likely to want to attend preschool. Likewise, if parents perceive the classroom as being a positive experience for their child, they may do more to ensure that their child is present as much as possible. These potential influences may be particularly relevant in preschool when parental beliefs are so variable.
Despite the clear rationale for hypothesizing that these cross-contextual factors would shape children’s preschool absences, most have not been examined empirically. To push the early childhood field forward we need to test these hypotheses, which requires theoretically grounded and advanced research methods. Thus, we sought to fill these gaps by examining the reasons underlying children’s preschool absences in a national sample of Head Start attendees. Because prior research has shown that one additional absence is not as detrimental for children’s academic achievement, but rather it is the accumulation of multiple days missed, we examine predictors of chronic absenteeism in addition to overall absences. Similar to other studies, we define chronic absenteeism as missing at least 10% of the school year (Balfanz and Byrnes, 2012). Taken together, this study can identify factors that can be targeted in the future to increase children’s preschool attendance, and ultimately, increase their kindergarten readiness.
Materials and methods
FACES 2009 followed a nationally representative sample of 3,349 3- and 4-year-old first time Head Start attendees across 486 classrooms (Moiduddin et al., 2012). For the purposes this investigation, we used data from the Head Start year (fall 2009 and spring 2010) and we excluded 444 children who did not have a valid longitudinal weight and 63 children who were in a home-based program, resulting in a final analytic sample of 2,842 children and families. On average, our final sample of children (50% female) were 3.84 years of age with the majority coming from ethnic minority households (36% Latine, 34% Black, 8% Asian/other). Table 1 presents full sample descriptives.
Missing data ranged from 0–17%, with an average of approximately 6% per indicator. In total, there were roughly 200 patterns of missing data. Approximately, 60% of children had complete case data. The most common pattern of missingness involved missing data on indicators of social support, employment, and absenteeism (7%). The next most common patterns involved missing data on classroom quality (5% of cases), maternal education (3% of cases), academic assessments (3% of cases), absenteeism (3% of cases), and maternal employment and coursework (3%). All other patterns of missing data represented less than 1–2% of cases.
Measures
Below, we describe our focal measures.
Absenteeism
During the spring parents were asked, “Approximately how many days has [CHILD] been absent since the beginning of the school year?” Responses were continuously measured and ranged from 0 to 20. Because not all parents reported on their children’s absences at the same time point (52% in March; 28% in April; and 20% in May), and because programs operated for a different number of days per week, we created an indicator of the proportion of days missed as a fraction of the days children were enrolled in school. To do so, we used parents date of assessment during the spring to gauge how long children were enrolled in school and divided the number of days children were absent by the number of months they were enrolled. This measured provided us with the number of days children were absent per month. Next, we multiplied the number of days children were absent per month by nine (the months of the school year). Finally, we divided this estimate by the number of days the program was in operation, which provided us with the proportion of the year children were absent. Chronic absenteeism was defined as missing 10% or more of the school year (Balfanz and Byrnes, 2012).
Family circumstance/necessity
We included nine parent-reported measures of family circumstances that may influence children’s absenteeism. We included two measures of household composition: The number of adults and number of children in the household. We have three categories to describe parental marital status: Married, not married, and not a two-parent household (i.e., cohabitating). Mothers’ employment status was coded as full-time, part-time, or not employed. We also included an indicator for whether the mother was currently enrolled in classes. The family financial situation was captured by the income-to-poverty measure (1 = less than 50% of the Federal Poverty Line; 6 = above 200% of the Federal Poverty Line). We also included measures of other sources of childcare that children experienced before or after Head Start, which included: Relative or non-relative care in home, relative or non-relative care not in home, center-based care, or no other care. Each of the aforementioned indicators was measured at the beginning of the Head Start year.
Two aspects of social support were also included as part of families’ circumstances. These indicators were collected toward the end of the Head Start year. First, parents reported on six items that described how much social support they perceived having (α =0.86:1 = never true; 3 = always true). Sample items included “help watch child when parent runs errands” and “others will loan emergency cash.” Second, parents reported on how helpful they perceived the following sources to be in terms of helping with their children: child’s father, spouse, child’s grandparents, relatives, friends, Head Start, and other Head Start parents (1 = not very helpful; 2 = somewhat helpful; 3 = very helpful; 4 = not applicable). Due to the distribution of responses, we categorized responses into a dichotomous variable (0 = not very helpful, 1 = somewhat or very helpful) and included the not applicable response as a flag variable.
Family stress and routines
Parents also reported on several dimensions of family routines and stress, each of which was measured at the start of the Head Start year. First, family food insecurity was captured by a single item asking the frequency with which food runs out because of money (never true, sometimes true, often true). Adequacy of medical care was a sum of three items asking about whether the child had a doctor’s visit in the past year, a dental visit in the past year, and health insurance (Gershoff et al., 2007). Residential instability was the number of times the family moved in the past 12 months. Receipt of government benefits was the proportion of six benefits families received: TANF, unemployment insurance, Food Stamps, WIC, social security, and energy assistance. Mothers also reported on whether they received child support. Three items tapped into routines: The average number of hours the child slept, whether the child had at least 4 days a week that followed a regular sleep schedule, and the number of days per week the family ate dinner together. Two maternal health indicators were also included: mother’s depression, measured by 12 items from the CES-D (α = 0.86; Radloff, 1977), and whether the mother reported poor or fair health. An indicator for poor or fair child health was also included. Finally, mothers reported on their exposure to neighborhood violence using 4 items that captured whether parents saw violent or non-violent crimes in their neighborhood and whether they knew someone that was—or they themselves were—a victim of a violent crime. Responses were categorized into a 5-point scale capturing the severity of neighborhood violence (0 = witnessed no crimes; 5 = experienced a violent crime).
Children’s early academic and social-behavioral skills
Children’s early academic and social-behavioral skills were measured at the beginning of the Head Start year. First, children’s early academic skills were based on direct assessments of their language, literacy, and math skills. Language was captured by the Peabody Picture Vocabulary Test (Dunn and Dunn, 1997; α = 0.97), a measure of children’s receptive vocabulary. Literacy skills were captured through two subtests of the Woodcock-Johnson assessment, Letter-Word Identification (α = 0.85) and Spelling Word (α = 0.79; Woodcock et al., 2001). The two measures captured children’s ability to identify and write upper- or lower-case letters. Children’s math skills were also directly assessed with the Woodcock-Johnson Applied Problems subscale (α = 0.87; Woodcock et al., 2001). These measures were composited together to create an overall indicator of early academic achievement (α =0.74). Next, children’s behavior problems were reported on by teachers using 14 items from the Personal Maturity Scale (Entwisle et al., 1987) and the Behavior Problems Index (Peterson and Zill, 1986), which captured children’s aggressive hyperactive, and withdrawn behavior (α = 0.88). Finally, as part of the data collection, teachers also reported on children’s social skills (e.g., how often children followed directions, helped put things away, followed rules) using 12 items from the Personal Maturity Scale (Entwisle et al., 1987) and the Social Skills Rating System (Gresham and Elliott, 1990; α = 0.89).
Center and classroom processes
To capture center and classroom processes, we leveraged data from parents, teachers, and administrators. First, toward the end of the Head Start year, the child’s teacher reported on the frequency with which they performed home visits and their frequency of parent-teacher meetings. Next, at the beginning of the year, the center director reported on 15 different services provided to families (0 = no, 1 = yes), which were summed together (α = 0.72; e.g., medical care, dental care, transportation, and education or job training). All Head Start classrooms were also observed and rated on the CLASS in the spring (Pianta et al., 2008), which provides a measure of the quality of teacher-child interactions. The CLASS is based on a 7-point Likert scale (1–2 = low to 6–7 = high) and measures instructional, social–emotional, and organizational aspects of the classroom. Next, in the end of the year surveys, teachers reported on the number of children in their classroom who were chronically absent (1 = none, 4 = 5 or more) and the overall behavior of the classroom (1 = the group misbehaves very frequently and is almost always difficult to handle, 5 = the group behaves exceptionally well). Finally, in the end of year surveys, parents provided their perceptions of the center through seven items (1 = never, 4 = always), which were used to create two scales: parents’ feelings of welcomeness at the school (α = 0.74; e.g., teacher is supportive of parent, parent feels welcome by teacher) and children’s enjoyment of school (α = 0.64; e.g., child feels safe at school; child is happy at Head Start).
Covariates
In addition to the focal predictors discussed above, we also included a number of covariates that were collected at the start of the Head start year, namely: Child race/ethnicity, child gender, child disability status, mothers’ immigration status, whether the program was full day, whether the child was less than 1 year away from kindergarten, child and mother age, home language, and maternal education. Because of the large number of variables included, we examined all predictors for multi-collinearity issues and found none. Less than 1% of correlations among predictors were above 0.50.
Analysis plan
Two sets of analyses were estimated using (StataCorp, 2011). First, we estimated OLS models to examine the associations between the predictors and the continuous measure of absenteeism. For these models, we provide effect sizes that correspond with how many standard deviations (SDs) our dependent variables change per SD increase in our continuous predictors. Given the categorical nature of some of our predictors (where SDs are not meaningful), for those variables (e.g., employment), we provide effect sizes that correspond with the unstandardized regression coefficient divided by the SD of the dependent variable. Second, we estimated logistic regression models to examine the predictors of the dichotomous chronic absenteeism variable. To gauge the meaningfulness of these associations we provide odds ratios which capture the differences in chronic absenteeism given a one-unit change in the predictor. To facilitate interpretation across variables, we also also provide a percent change in rates of chronic absenteeism given a one standard deviation change in all continuous variables. All models were clustered at the classroom level to account for dependence in child outcomes and weighted to be nationally representative. To account for missing data, we imputed 50 datasets using the chained equations method.
Additionally, because absenteeism is not distributed uniformly across schools and communities, we treat the above analytic framework as our primary specification and allow the variances in absenteeism to vary across different contexts. However, as an additional analysis, we also estimated additional models that implemented classroom fixed effects. In these models, we constrained the analysis to examining children within classrooms and, as such, we hold constant all classroom-level practices and processes. Thus, the classroom fixed effects models consider why some children are more (or less) likely to be absent than their classmates. Although not intended to be causal, classroom fixed effects provide a more rigorous estimation of how individual child and family factors are associated with absenteeism. We present both analytic specifications to provide a more balanced and nuanced portrait about absenteeism in Head Start. In doing so, it is important to note that classroom fixed effects models cannot be implemented with logistic regression and, consequently, when looking at chronic absenteeism as the outcome, we estimate a linear probability model. Coefficients for those models can be interpreted as the percentage change as a function of a one unit change in the predictor. Lastly, we estimated a robustness check using fractional response models for our OLS models due to the nature of our dependent variable.
Results
On average, children missed 5% of the school year and 12% were chronically absent.
Predictors of absenteeism
Our first model predicted the proportion of days a child was absent. Unstandardized and standardized coefficients are presented in the left two columns of Table 2. To begin, we found that very few family necessity and social support factors predicted children’s absences. However, the need for preschool (as captured by full-time employment), presence of siblings, and social support received, especially from other parents in the program, were linked with fewer school absences, with effect sizes ranging from roughly 5–15% of a SD. And even though children’s early academic and social-behavioral skills at the start of Head Start were not linked with absenteeism, family stress and routines did matter. More specifically, children whose families received greater government assistance were absent more often, whereas children who experienced more frequent family dinners had fewer absences. Not surprisingly, children in poor health missed a considerable more amount of school (ES = 33% of a SD) and so did children who lived in neighborhoods perceived by their mother to be violent.
Moving beyond the home context, we also found that a number of center and classroom characteristics were linked with preschool absences. For example, children who enjoyed school were less frequently absent and so were children who attended classrooms that provided higher quality services. In contrast, there was evidence of spillover effects, whereby children were more frequently absent when they were enrolled in classrooms with a higher proportion of absent peers. Effect sizes for these associations ranged from approximately 5–10% of a SD.
And although not a focal study objective, in terms of covariates, we found that Black and Latine children had fewer absences than White children. In contrast, children born to immigrant mothers had fewer absences than those whose mothers were born in the U.S. and children who attended a full-day program were also absent less frequently than children in part-day programs.
Predictors of chronic absenteeism
Our second model predicted whether children were chronically absent from Head Start. Unstandardized coefficients and odds ratios are provided in final two columns of Table 2. Overall, the patterns of results were similar to those documented above for absenteeism continuously measured, but there were a few notable differences.
When looking at family necessity, the three significant associations were the same as above: A greater number of children in the household, maternal full-time employment, and support from other Head Start parents were all predictive of a lower likelihood of chronic absences. In terms of family routines and stress, we again found that receipt of governmental benefits was associated with increased preschool absences; however, unlike our models predicting overall levels of absences, when predicting chronic absences, we found that the adequacy of medical care was linked with a lower likelihood of chronic absenteeism. Poor child health was again a sizeable predictor of chronic absenteeism, but unlike our first model predicting overall levels of absences, children’s sleep patterns was associated with chronic absenteeism. Specifically, children who had more hours of sleep per night were less likely to be chronically absent.
Like above, a similar pattern of center and classroom factors were also documented when examining how likely children were to be chronically absent, but many of the predictors were only marginally significant. And, in terms of covariates, the same patterns emerged. Black and Latine children (versus White children) were less likely to be chronically absent and children in full-day programs were less likely to be absent than those in half-day programs.
Classroom fixed effects
Our next set of analyses implemented classroom fixed effects (see Table 3). These results are presented in Table 3. Results from these analyses were generally similar to those reported above, but fewer stress and routine variables were associated with absenteeism when comparing children with their classmates. Importantly, however, even though fewer factors were significantly linked with within classroom absenteeism, the effect sizes for the focal associations were comparable across both specifications, suggesting that the reasons children were absent are comparable when making both within and between classroom comparisons.
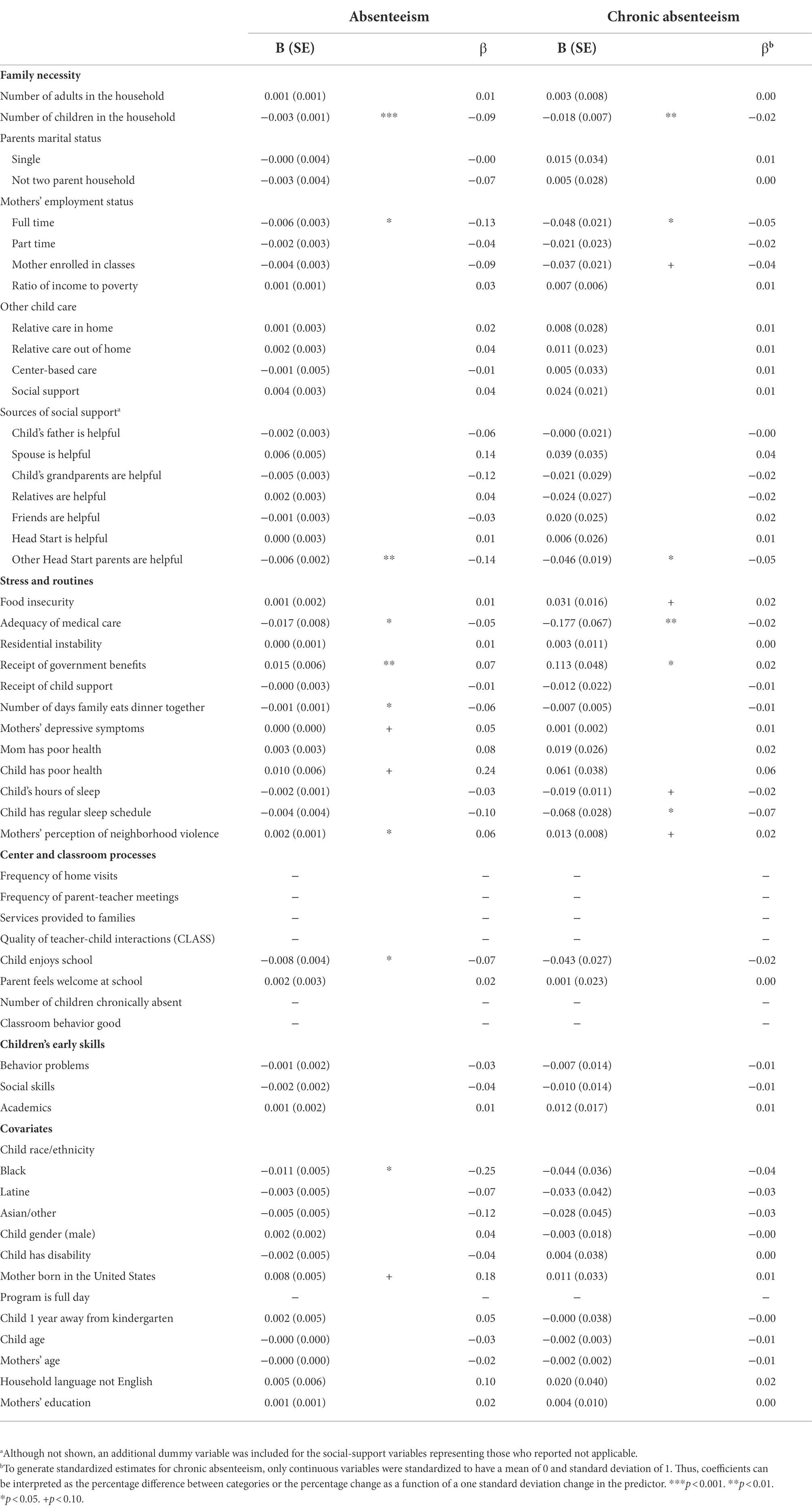
Table 3. Results from regression models predicting absenteeism and chronic absenteeism using classroom fixed effects.
Fractional response models
As a robustness check, we ran our OLS models using fractional response modeling. Because we had not included bounds for our imputations, 1–2% of cases had values that fell below 0%. To estimate fractional response models, we estimated models that: (a) excluded these 1–2% of cases and (b) recoded their values as 0. In both instances, our results were substantively similar to the results presented in Table 2 (results available from author upon request).
Discussion
Preschool absences are not uncommon. In fact, our results show that Head Start attendees miss approximately 5% of the school year on average and 12% of children are chronically absent. Because preschool attendance has been linked to improved academic achievement and later school attendance (Connolly and Olson, 2012; Ansari and Purtell, 2018; Ehrlich et al., 2018; Fuhs et al., 2018; Rhoad-Drogalis and Justice, 2018), it is critical to understand why children miss time from school. Resonating with both bioecological theory (Bronfenbrenner and Morris, 2006) and models of preschool selection (Meyers and Jordan, 2006), our results highlight the multifaceted nature of preschool absences, with multiple factors across contexts contributing to the likelihood that children would miss time from preschool.
We found a number of family factors that were associated with children’s absences. To start, children who had mothers that were employed full-time and children who were in a household with a greater number of children were both less likely to be absent and chronically absent. Families with multiple children and full-time employment may rely on preschool for childcare, and thus, be less likely to let their child miss significant time from school. The finding on number of children in the home is different from qualitative findings with elementary-aged children, where having a greater number of children in the household can make getting to school more challenging (Sugrue et al., 2016). Children whose families had more frequent routines were also absent less frequently than children whose families had fewer daily routines. Specifically, more family dinners were associated with fewer absences, and both the regularity and amount of children’s sleep were also associated with fewer absences. That families that are more regular in their routines at home would also be more routine in their children’s preschool experience is perhaps not surprising as more routines in the home are likely to mitigate stressors associated with absenteeism.
Similar to the existing literature on elementary school absences (e.g., Ready, 2010), we found that children’s health was strongly associated with absences and chronic absenteeism in preschool. On the contrary, neither mothers’ physical nor mental health played a role in their children’s absences, although it is plausible that these characteristics shaped absenteeism through their associations with family routines. Similar to prior research, a number of indicators of economic stressors were also associated with absenteeism and chronic absenteeism, including food insecurity, adequacy of medical care, and receipt of governmental assistance (Chang and Romero, 2008). These economic challenges are likely to be associated with day-to-day barriers to attendance, such as transportation, which is an important correlate of regular school attendance (Gottfried, 2017). Lastly, mothers who perceived their neighborhoods to be violent had children who were more frequently absent. It may be that this is operating as another marker of economic disadvantage, or it may be that living in dangerous neighborhoods poses a separate barrier to regular preschool attendance. Taken together, these findings suggest that social and economic disadvantages pose great challenges to high rates of attendance at Head Start. Programs focused on reducing absenteeism need to consider the complex circumstances families may be experiencing throughout the school year.
More hearteningly, we found a number of center- and classroom-level features that were associated with fewer preschool absences. For example, children who attended centers that provided more services to families were less likely to be absent. This suggests that a continued focus on family outreach may benefit children by increasing attendance, in addition to its other positive impacts on families (e.g., Barnett et al., 2020). Children’s classroom experiences also played a role in the regularity of their attendance; specifically, children were less likely to be absent when their mothers’ perceived them as enjoying school and when they attended classrooms that were rated as higher quality. Thus, positive child experiences in the classroom is a potential pathway to reduced absenteeism. Interestingly, the other social support item that was associated with fewer school absences was parents’ perceptions of support from other Head Start parents, suggesting that facilitating relationships between parents is another important way that centers may be able to reduce absenteeism. Despite these promising avenues for reducing preschool absences, we also found that the concentration of absences within a classroom was associated with individual children’s absenteeism. Although speculative, it may be that a high concentration of absences in a classroom reflects a social norm, namely that preschool absences are okay (see also, Ehrlich et al., 2013; Gottfried et al., 2020).
Overall, our findings highlight the fact that no one mechanism stood out as the sole driver of absenteeism; but rather, there appeared to be many individual, family and center characteristics that shaped preschool absenteeism. This aligns with bioecological theory that suggests that multiple systems may shape absenteeism. Additionally, our findings provide support for multiple components of the accommodation’s framework. For example, children whose families likely had higher need for childcare, as evidenced by full-time employment, were less likely to be absent. But other factors mattered too, providing evidence for the framework’s assertion that parents’ decision-making around childcare, and in this case, attendance, is complex, and shaped by numerous factors.
Accordingly, there are many routes to reduce absenteeism in the future—and focusing on one factor alone is unlikely to make drastic reductions in absenteeism. A holistic approach that tackles both family- and classroom-level processes is necessary to improve children’s Head Start attendance. Having said that, there are successful models at other school levels that may be useful to future program development. One such successful elementary school model assigned monitors to engage with both families and school staff to increase attendance; this type of model may be particularly useful in Head Start, which already strives to increase parent-center communication, but has not yet been tested in the preschool years (Lehr et al., 2004). Other work has revealed a number of promising strategies to reduce preschool absences. First, in line with our findings, Katz et al. (2016) note that home-school connections are critical to facilitate school attendance. Even so, it is important to acknowledge that these positive relationships may not be enough to reduce the barriers present for some families. Thus, having other resources, such as information about transportation and medical care referrals, easily accessible to families is critical to reducing preschool absences. Additionally, Katz et al. (2016) find that staff members commonly feel that parents do not understand the importance of preschool for their children’s current and future learning. Finding successful ways to deliver this message to families requires continued attention, as parents’ beliefs about preschool are likely key to reducing absenteeism.
Despite the fact that our study represents one of the first efforts to understand why children miss time from preschool at the national level, our findings need to be interpreted in light of a few limitations. The primary limitation of our work is our reliance on parental report of children’s absences. Although the use of parent reports is common, administrative data that tracks children’s absences could increase precision when examining the predictors and outcomes of preschool absences. Nonetheless, FACES 2009 is one of only two national datasets with information on children’s preschool attendance (Mendez et al., 2016). Additionally, our data is limited to children attending Head Start and, thus, we cannot speak to the predictors of absenteeism in other types of preschool programs, which requires continued attention. Given that Head Start serves children from low-income families, our findings may be more generalizable to this population. However, given Head Start’s longstanding commitment to family and community engagement, our findings likely do not generalize beyond the program.
Given our large number of predictors, it is also important to note the potential for the Table 2 fallacy in our interpretation of our findings (Westreich and Greenland, 2013). Although the goal of this paper was to identify unique associations with children’s absenteeism, it is plausible that some of our predictors (e.g., indicators of financial instability) are mechanisms through which other predictors (e.g., employment status) are associated with children’s absenteeism. Understanding these pathways is an important direction for future research. It is also important to note that although we examined numerous predictors, there are still a number of potential factors not addressed that are key for future research. For example, more direct measures of transportation and logistical support are important to capture (Gottfried, 2017). Additionally, understanding parents’ perspectives regarding the importance of attendance in the preschool years may be key to understanding absenteeism patterns (Ehrlich et al., 2013). Lastly, understanding predictors of absenteeism within demographic groups may be critical to developing potent interventions. For example, although we found that Black and Latine children were less likely to be absent than their White peers, understanding factors that shape absenteeism within these groups may be necessary to improve attendance in the future.
Absenteeism, and particularly, chronic absenteeism is diminishing the potential benefits of preschool (Connolly and Olson, 2012; Ansari and Purtell, 2018; Ehrlich et al., 2018; Rhoad-Drogalis and Justice, 2018), especially for children who are from low-income families who: (a) are more likely to benefit from preschool (Weiland and Yoshikawa, 2013), but (b) are more likely to be absent than their higher-income peers (Morrissey et al., 2014). In this study, we find that there is no one underlying reason for absenteeism; rather, there are a number of factors that cut across contexts are contributing to the high levels of absences in the United States among a sample of preschoolers from low-income homes. As such, there is possible value of a package of efforts that target the different causes of absenteeism. Addressing these factors, and subsequently reducing absenteeism, is a critical pathway to increasing the school readiness of disadvantaged children.
Data availability statement
Publicly available datasets were analyzed in this study. This data can be found at: https://www.icpsr.umich.edu/web/ICPSR/series/236.
Ethics statement
The studies involving human participants were reviewed and approved by the Ohio State University IRB. Written informed consent to participate in this study was provided by the participants’ legal guardian/next of kin.
Author contributions
AA led analysis and preparation of methods and results. KP led preparation of introduction and discussion. AA and KP authors conceptualized the manuscript. All authors contributed to the article and approved the submitted version.
Conflict of interest
The authors declare that the research was conducted in the absence of any commercial or financial relationships that could be construed as a potential conflict of interest.
Publisher’s note
All claims expressed in this article are solely those of the authors and do not necessarily represent those of their affiliated organizations, or those of the publisher, the editors and the reviewers. Any product that may be evaluated in this article, or claim that may be made by its manufacturer, is not guaranteed or endorsed by the publisher.
References
Allensworth, E. M., and Easton, J. Q. (2007). What matters for staying on track and graduating in Chicago public high schools. Chicago, IL: Consortium on Chicago School Research.
Ansari, A., and Gottfried, M. A. (2020). Early childhood educational experiences and preschool absenteeism. Elem. Sch. J. 121, 34–51. doi: 10.1086/709832
Ansari, A., Hofkens, T. L., and Pianta, R. C. (2020). Absenteeism in the first decade of education forecasts civic engagement and educational and socioeconomic prospects in young adulthood. J. Youth Adolesc. 49, 1835–1848. doi: 10.1007/s10964-020-01272-4
Ansari, A., and Pianta, R. C. (2019). School absenteeism in the first decade of education and outcomes in adolescence. J. School Psychol. 76, 48–61. doi: 10.1016/j.jsp.2019.07.010
Ansari, A., Pianta, R. C., Whittaker, J. V., Vitiello, V. E., Ruzek, E. A., and Zhang, J. (2021). Does the timing of kindergarten absences matter for children’s early school success? School Psychol. 36, 131–141. doi: 10.1037/spq0000434
Ansari, A., and Purtell, K. M. (2018). Absenteeism in head start and children's academic learning. Child Dev. 89, 1088–1098. doi: 10.1111/cdev.12800
Baker, M. L., Sigmon, J. N., and Nugent, M. E. (2001). Truancy reduction: Keeping students in school. Rockville, MD: Juvenile Justice Bulletin.
Balfanz, R., and Byrnes, V. (2012). The importance of being in school: A report on absenteeism in the nation’s public schools. Baltimore: Johns Hopkins University Center for Social Organization of Schools.
Barnett, M. A., Paschall, K. W., Mastergeorge, A. M., Cutshaw, C. A., and Warren, S. M. (2020). Influences of parent engagement in early childhood education centers and the home on kindergarten school readiness. Early Child. Res. Q. 53, 260–273.
Bronfenbrenner, U., and Morris, P. A. (2006). “The bioecoloigical model of human development” in Handbook of child psychology: Theoretical models of human development. Vol. 1. eds. R. M. Lerner and W. Damon (New York, NY: Wiley). 793–828.
Chang, H. N., and Romero, M. (2008). Present, engaged, and accounted for: The critical importance of addressing chronic absence in the early grades. New York, NY: National Center for Children in Poverty. Available at: https://www.nccp.org/wp-content/uploads/2008/09/text_837.pdf
Chen, L., Jennison, B. L., Yang, W., and Omaye, S. T. (2000). Elementary school absenteeism and air pollution. Inhal. Toxicol. 12, 997–1016. doi: 10.1080/08958370050164626
Childs, J., and Lofton, R. (2021). Masking attendance: how education policy distracts from the wicked problem (s) of chronic absenteeism. Educ. Policy 35, 213–234. doi: 10.1177/0895904820986771
Connolly, F., and Olson, L. S. (2012). Early elementary performance and attendance in Baltimore city schools pre-kindergarten and kindergarten. Baltimore, MD: Baltimore Education Research Consortium.
Crosnoe, R., Johnson, M. K., and Elder, G. H. (2004). Intergenerational bonding in school: the behavioral and contextual correlates of student-teacher relationships. Sociol. Educ. 77, 60–81. doi: 10.1177/003804070407700103
Dubay, L., and Holla, N. (2015). Absenteeism in DC public schools early education program. Available online at: http://www.urban.org/sites/default/files/alfresco/publication-pdfs/2000082-Absenteeism-in-DC-Public-Schools-Early-Education-Program.pdf
Dubay, L, and Holla, N. (2016). Does attendance in early education predict attendance in elementary school? An analysis of DCPS’s early education program. Available online at: https://www.urban.org/research/publication/does-attendance-early-education-predict-attendance-elementary-school-analysis-dcpss-early-education-program/view/full_report
Duncan, G. J., and Magnuson, K. A. (2013). Investing in preschool programs. J. Econ. Perspect. 27, 109–132. doi: 10.1257/jep.27.2.109
Dunn, L. M., and Dunn, L.M. (1997). Peabody Picture and Vocabulary Test: Examiner’s Manual and Norms Booklet. 3rd edn. Circle Pines, MN: American Guidance Service.
Ehrlich, S. B., Gwynne, J. A., and Allensworth, E. M. (2018). Pre-kindergarten attendance matters: early chronic absence patterns and relationships to learning outcomes. Early Child. Res. Q. 44, 136–151. doi: 10.1016/j.ecresq.2018.02.012
Ehrlich, S. B., Gwynne, J. A., Pareja, A. S., and Allensworth, E. M. (2013). Preschool attendance in Chicago public schools: Relationships with learning outcomes and reasons for absences. Chicago, IL: University of Chicago Consortium on Chicago School Research.
Epstein, J. L., and Sheldon, S. B. (2002). Present and accounted for: improving student attendance through family and community involvement. J. Educ. Res. 95, 308–318. doi: 10.1080/00220670209596604
Entwisle, D. R., Alexander, K. L., Cadigan, D., and Pallas, A. M. (1987). Kindergarten experience: Cognitive effects or socialization? Am. Educ. Res. J. 24, 337–364. doi: 10.3102/00028312024003337
Fuhs, M. W., Nesbitt, K. T., and Jackson, H. (2018). Chronic absenteeism and preschool children’s executive functioning skills development. J. Educ. Stud. Placed Risk (JESPAR) 23, 39–52. doi: 10.1080/10824669.2018.1438201
Gershoff, E. T., Aber, J. L., Raver, C. C., and Lennon, M. C. (2007). Income is not enough: Incorporating material hardship into models of income associations with parenting and child development. Child Dev. 78, 70–95. doi: 10.1111/j.1467-8624.2007.00986.x
Gershenson, S., Jacknowitz, A., and Brannegan, A. (2015). Are student absences worth the worry in US primary schools?. Available online at: http://ftp.iza.org/dp9558.pdf
Gottfried, M. A. (2009). Excused versus unexcused: how student absences in elementary school affect academic achievement. Educ. Eval. Policy Anal. 31, 392–415. doi: 10.3102/0162373709342467
Gottfried, M. A. (2010). Evaluating the relationship between student attendance and achievement in urban elementary and middle schools an instrumental variables approach. Am. Educ. Res. J. 47, 434–465. doi: 10.3102/0002831209350494
Gottfried, M. A. (2011). The detrimental effects of missing school: evidence from urban siblings. Am. J. Educ. 117, 147–182. doi: 10.1086/657886
Gottfried, M. A. (2013). Quantifying the consequences of missing school: linking school nurses to student absences to standardized achievement. Teach. Coll. Rec. 115, 1–30. doi: 10.1177/016146811311500605
Gottfried, M. A. (2015). Can center-based childcare reduce the odds of early chronic absenteeism? Early Child. Res. Q. 32, 160–173. doi: 10.1016/j.ecresq.2015.04.002
Gottfried, M. A. (2017). Linking getting to school with going to school. Educ. Eval. Policy Anal. 39, 571–592. doi: 10.3102/0162373717699472
Gottfried, M. A., and Gee, K. A. (2017). Identifying the determinants of chronic absenteeism: A bioecological systems approach. Teach. Coll. Rec. 119, 1–34. doi: 10.1177/016146811711900704
Gottfried, M. A., and Hutt, E. L. (2019). Addressing absenteeism: Lessons for policy and practice. Stanford, CA: Policy Analysis for California Education, PACE.
Gottfried, M. A., Kirksey, J. J., and Ozuna, C. S. (2020). Exploring the links between student and classmate chronic absenteeism. Teach. Coll. Rec. 122, 1–28. doi: 10.1177/016146812012201205
Gresham, F. M., and Elliott, S. N. (1990). The social skills rating system. Circle Pines, MN: American Guidance Service.
Katz, M., Johnson, M. C., and Adams, G. (2016). Improving prekindergarten attendance: School-level strategies for messaging, engaging parents, and responding to absences in four DC public schools. Washington, DC: Urban Institute.
Lehr, C. A., Sinclair, M. F., and Christenson, S. L. (2004). Addressing student engagement and truancy prevention during the elementary school years: a replication study of the check and connect model. J. Educ. Stud. Placed Risk 9, 279–301. doi: 10.1207/s15327671espr0903_4
Mendez, J., Crosby, D., and Helms, H. (2016). Using existing large-scale data to study early care and education among Hispanics: families’ utilization of early care and education. Available online at: http://www.childtrends.org/wp-content/uploads/2016/02/ECE-Series-Brief-No.-3.pdf
Meyers, M. K., and Jordan, L. P. (2006). Choice and accommodation in parental child care decisions. Community Dev. 37, 53–70. doi: 10.1080/15575330609490207
Moiduddin, E., Aikens, N., Tarullo, L. B., West, J., and Xue, Y. (2012). Child outcomes and classroom quality in FACES 2009. Washington, DC: Office of Planning, Research, and Evaluation, U.S. Department of Health and Human Services.
Morrissey, T. W., Hutchison, L., and Winsler, A. (2014). Family income, school attendance, and academic achievement in elementary school. Dev. Psychol. 50, 741–753. doi: 10.1037/a0033848
Office of Head Start, Administration for Children and Families. (2016). Head start program facts, Fiscal Year 2015. Available online at: https://eclkc.ohs.acf.hhs.gov/hslc/data/factsheets/2015-hs-program-factsheet.html
Peterson, J. L., and Zill, N. (1986). Marital disruption, parent-child relationships, and behavior problems in children. J. Marriage Fam. 295–307. doi: 10.2307/352397
Phillips, D., Lipsey, M. W., Dodge, K. A., Haskins, R., Bassok, D., Burchinal, M. R., et al. (2017). Puzzling it out: The current state of scientific knowledge on pre-kindergarten effects. A consensus statement. Brookings Institution and the Center for Child and Family Policy.
Pianta, R. C., La Paro, K.M., and Hamre, B. K. (2008). Classroom assessment scoring system (CLASS). Baltimore: Paul H. Brookes Publishing Company.
Radloff, L. S. (1977). The CES-D scale: A self-report depression scale for research in the general population. Appl. Psychol. Meas. 1, 385–401. doi: 10.1177/014662167700100306
Ready, D. D. (2010). Socioeconomic disadvantage, school attendance, and early cognitive development: the differential effects of school exposure. Sociol. Educ. 83, 271–286. doi: 10.1177/0038040710383520
Rhoad-Drogalis, A., and Justice, L. M. (2018). Absenteeism in Appalachian preschool classrooms and children’s academic achievement. J. Appl. Dev. Psychol. 58, 1–8. doi: 10.1016/j.appdev.2018.07.004
Sugrue, E., Zuel, T., and Laliberte, T. (2016). The Ecological Context of Chronic School Absenteeism in the Elementary Grades. Child. Sch. 38, 137–145. doi: 10.1093/cs/cdw020
Weiland, C., and Yoshikawa, H. (2013). Impacts of a prekindergarten program on children’s mathematics, language, literacy, executive function, and emotional skills. Child Dev. 84, 2112–2130. doi: 10.1111/cdev.12099
Westreich, D., and Greenland, S. (2013). The table 2 fallacy: presenting and interpreting confounder and modifier coefficients. Am. J. Epidemiol. 177, 292–298. doi: 10.1093/aje/kws412
Woodcock, R. W., McGrew, K. S., and Mather, N. (2001). Woodcock-Johnson III tests of achievement. Itasca, IL: Riverside Publishing.
Keywords: absenteeism, preschool, Head Start, families, FACES 2009
Citation: Purtell KM and Ansari A (2022) Why are children absent from preschool? A nationally representative analysis of Head Start programs. Front. Educ. 7:1031379. doi: 10.3389/feduc.2022.1031379
Edited by:
Gil Keppens, Vrije University Brussel, BelgiumReviewed by:
Markus Klein, University of Strathclyde, United KingdomLilly Augustine, Jönköping University, Sweden
Copyright © 2022 Purtell and Ansari. This is an open-access article distributed under the terms of the Creative Commons Attribution License (CC BY). The use, distribution or reproduction in other forums is permitted, provided the original author(s) and the copyright owner(s) are credited and that the original publication in this journal is cited, in accordance with accepted academic practice. No use, distribution or reproduction is permitted which does not comply with these terms.
*Correspondence: Kelly M. Purtell, UHVydGVsbC4xNUBvc3UuZWR1