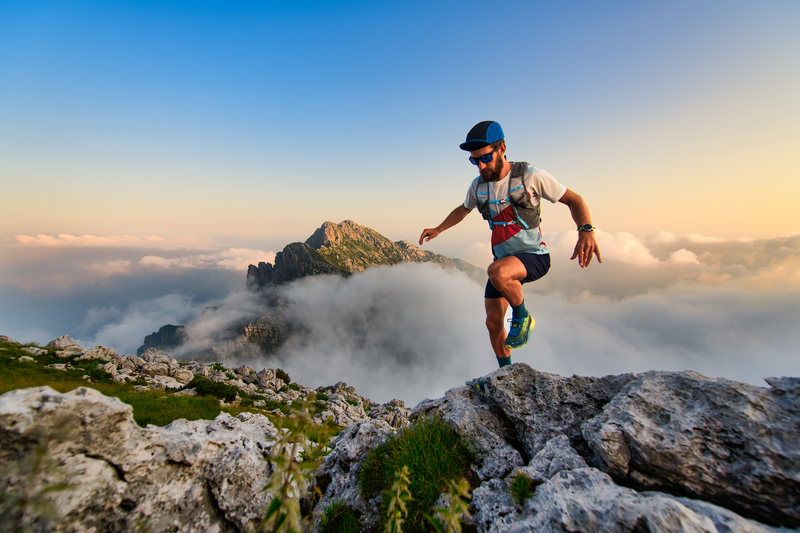
95% of researchers rate our articles as excellent or good
Learn more about the work of our research integrity team to safeguard the quality of each article we publish.
Find out more
ORIGINAL RESEARCH article
Front. Educ. , 21 November 2022
Sec. STEM Education
Volume 7 - 2022 | https://doi.org/10.3389/feduc.2022.1016415
Active learning pedagogies are shown to enhance the outcomes of students, particularly in disciplines known for high attrition rates. Despite the demonstrated benefits of active learning, didactic lecture continues to predominate in science, technology, engineering, and mathematics (STEM) courses. Change agents and professional development programs have historically placed emphasis on develop–disseminate efforts for the adoption of research-based instructional strategies (RBIS). With numerous reported barriers and motivators for trying out and adopting active learning, it is unclear to what extent these factors are associated with adoption of RBIS and the effectiveness of change strategies. We present the results of a large-scale, survey-based study of introductory chemistry, mathematics, and physics instructors and their courses in the United States. Herein, we evaluate the association of 17 malleable factors with the tryout and adoption of RBIS. Multilevel logistic regression analyses suggest that several contextual, personal, and teacher thinking factors are associated with different stages of RBIS adoption. These results are also compared with analogous results evaluating the association of these factors with instructors’ time spent lecturing. We offer actionable implications for change agents to provide targeted professional development programming and for institutional leaders to influence the adoption of active learning pedagogies in introductory STEM courses.
Research-based instructional strategies (RBIS; see Dean et al., 2012) and evidence-based instructional practices (EBIPs; see Stains and Vickrey, 2017) are similarly used labels for instructional practices with a basis in educational research, including active learning. Active learning in undergraduate science, technology, engineering, and mathematics (STEM) courses has been demonstrated to enhance student outcomes (Springer et al., 1999; Lorenzo et al., 2006; Haak et al., 2011; Ruiz-Primo et al., 2011; Freeman et al., 2014; Rahman and Lewis, 2019; Theobald et al., 2020). Compared to traditional lecture-based courses, active learning courses are associated with increased achievement across all STEM disciplines (Freeman et al., 2014). Notably, studies also show an increase in achievement outcomes for minoritized populations in active learning courses (Lorenzo et al., 2006; Kogan and Laursen, 2014; Synder et al., 2016; Ballen et al., 2017; Deri et al., 2018; Roberts et al., 2018; Stanich et al., 2018; Theobald et al., 2020). For college students enrolled at two-year institutions and community colleges, active learning has been shown to contribute to increased graduation rates (Riedl et al., 2021) and transfer rates (Wang et al., 2017). In this paper, we refer to these teaching practices as RBIS, which can include, but are not limited to, think-pair-share, small group work, peer instruction, peer-led team learning, flipped classroom, and just-in-time-teaching (for more comprehensive lists of RBIS, see: Henderson and Dancy, 2009; Borrego et al., 2013; Baker et al., 2014). These strategies have foundations in the education research literature, contrast with didactic lecture, and engage students in the learning process in lieu of passively listening to an instructor (Bonwell and Eison, 1991).
Although active learning pedagogies undoubtedly have established benefits in STEM, lecture-based pedagogical approaches remain dominant (Stains et al., 2018). The research literature suggests the prominence of lecture-oriented pedagogies may be a result of institutional failure to normalize use of RBIS (Henderson and Dancy, 2007; Shadle et al., 2017), failure to implement faculty incentives or rewards for using student-centered pedagogical techniques (Michael, 2007; Brownell and Tanner, 2012; Shadle et al., 2017), and failure to combat student resistance to instructional changes (Henderson and Dancy, 2007; Michael, 2007; Shadle et al., 2017), among the plethora of reasons.
In addition to these barriers, instructors have expressed feeling unprepared for changing the way they teach (Andrews and Lemons, 2015; Bathgate et al., 2019a). Foremost, instructors may have little or no knowledge about and awareness of alternatives to traditional lecturing, i.e., using RBIS (Hativa, 1995; Miller et al., 2000; Luft et al., 2004; Walczyk et al., 2007; Yarnall et al., 2007; Winter et al., 2012). Once aware, though, of RBIS and active learning strategies, instructors may lack opportunities to try out these new strategies (Handelsman et al., 2004; Ebert-May et al., 2011) or may be unconvinced that these strategies are more effective than lecturing (Miller et al., 2000; Yarnall et al., 2007; Winter et al., 2012).
While meta-analytic work by Freeman et al. (2014) and Theobald et al. (2020) have noted the importance of active learning in STEM education, parallel multidisciplinary studies of malleable factors (i.e., something that can be changed and altered) related to the adoption of such active learning pedagogies in postsecondary STEM courses are largely absent from and needed in the research literature (National Research Council (NRC), 2012; American Association for the Advancement of Science (AAAS), 2019). To date, only one study at such a level (Yik et al., 2022) details the association of malleable factors with the adoption of active learning in multiple STEM disciplines. In Yik et al. (2022), as with this study, we focus on introductory chemistry, mathematics, and physics, which are high-enrollment courses serving a large number of students [President’s Council of Advisors on Science and Technology (PCAST), 2012] and are barriers for students not finishing STEM degrees (Seymour and Hewitt, 1997; Koch, 2017; Seymour and Hunter, 2019). In Yik et al. (2022), we evaluated 17 malleable factors that have been reported in the research literature to be associated with percent lecturing (i.e., time not spent using active learning strategies) in gateway STEM courses. However, another measure of active learning is stage of adoption of RBIS. While knowing the amount of time lecturing allows for conclusions about the degree of student-centered learning, it does not provide information about an instructor’s awareness about RBIS, time spent learning about and readiness to tryout RBIS, and adoption of RBIS in their courses (Landrum et al., 2017); instructors’ needs are different based on their awareness, tryout, and adoption of RBIS (Viskupic et al., 2022). This study will add to the research literature by quantifying the association of malleable factors on the adoption of RBIS in introductory chemistry, mathematics, and physics courses. Evaluation of these malleable factors on RBIS adoption allows for comparisons with a previous variable, i.e., percent time lecturing (Yik et al., 2022), on the effects of different aspects of active learning, and thus, yields recommendations for the adoption of RBIS to promote active learning in introductory STEM courses.
In the context of introductory chemistry, mathematics, and physics courses, two research questions guide this study:
1. To what extent are malleable contextual, personal, and teacher thinking factors associated with the stages of RBIS adoption?
2. How do the association of malleable contextual, personal, and teacher thinking factors with the stages of RBIS adoption compare with percent lecturing?
Research on barriers and driving forces for the adoption of teaching strategies informed the selection of malleable factors that were previously modeled (see Yik et al., 2022) and are modeled in this study. Researchers (e.g., Gess-Newsome et al., 2003; Dancy and Henderson, 2010; Andrews and Lemons, 2015; Lund and Stains, 2015; Sturtevant and Wheeler, 2019) have described a number of contextual, personal, and belief factors that influence instructors’ pedagogical decisions. Through a comprehensive review of the literature, Woodbury and Gess-Newsome (2002) developed the Teacher-Centered Systemic Reform (TCSR) model to understand how classrooms change due to reform initiatives; this framework was later modified to better suit the higher education system (Gess-Newsome et al., 2003). The TCSR model focuses on teachers’ thinking and practices as the origin for change that happen within the classroom inside the context of a larger university system (Gess-Newsome et al., 2003). In a university context, the TCSR framework is comprised of three broad categories: contextual factors (e.g., institution type, discipline, and class size), personal factors (e.g., extent of teacher preparation and teaching-related professional development), and teacher thinking factors (e.g., knowledge about teaching and dissatisfaction with current teaching practices); these categories were used to situate the malleable factors modeled in our prior study (Yik et al., 2022). Our current study also aims to evaluate the association of these same malleable factors with the adoption of RBIS when institutional and disciplinary differences are accounted for. Non-malleable factors (e.g., race/ethnicity) are not included because they do not provide actionable implications. While this study focuses on introductory chemistry, mathematics, and physics courses, we include malleable factors that have been reported to be related to the adoption of teaching strategies in the broader STEM education literature. While there may be some disciplinary differences and STEM disciplines differ in the amount of research literature in this area, these malleable factors can be assumed to affect all STEM disciplines to an extent (Lund and Stains, 2015). A summary of the malleable factors, logic for inclusion in this study, and relevant literature citations are given in Table 1.
Table 1. Malleable factors included in this study situated within the TCSR framework and hypotheses about how factors impact the adoption of RBIS with relevant citations.
Dormant’s (2011) Chocolate Model of Change, also known as the CACAO model, has been used to conceptualize institutional change in STEM education (Marker et al., 2015; Landrum et al., 2017; Shadle et al., 2017; Earl et al., 2020; Pilgrim et al., 2020; Salomone et al., 2020; McAlpin et al., 2022; Viskupic et al., 2022). The CACAO model is organized around four dimensions: (1) Change, (2) Adopters, (3) Change Agents, and (4) Organization (Dormant, 2011). Change is a new idea, process, or system that you want a group of people to accept (e.g., adoption of RBIS). Adopters are the group of people that is targeted to adopt the change (e.g., instructors of introductory STEM courses). Change agents are the people and team trying to enact change (e.g., administrators, educational policy makers, educational researchers, instructional designers). The organization encompasses the change, adopters, and change agents. Organizational contexts and influences affect how change agents lead change initiatives in hopes that adopters accept or implement the change. In a higher education setting, departments, colleges/schools, and the institution all have influence on individuals’ beliefs and behaviors, which can influence social norms and thus change in an organization.
In operationalizing the dimensions of the CACAO model, we investigate change as the state of teaching transformations through the uptake of RBIS, adopters as the STEM instructors that amend their instruction to include using RBIS in their teaching practices, change agents as the individuals advocating for adoption of RBIS, and the organization as the members of higher education institutions. For adopters, Dormant (2011) outlines five stages of adoption: (1) awareness, (2) curiosity, (3) mental tryout, (4) hands-on tryout, and (5) adoption.
The TCSR framework and the CACAO model work in tandem to understand institutional change. The CACAO model is designed to aid organizational (i.e., institutional) change agents (i.e., change practitioners and leaders) to understand dimensions of change (Dormant, 2011); in this study, we focus on malleable factors that influence the dimensions of change. The TCSR framework focuses on teachers’ beliefs, which influence teacher practices, as the center for change that occurs within the institution (Gess-Newsome et al., 2003); contextual factors, personal factors, and teacher thinking factors are described as components in this larger institutional change context. Factors modeled in this study are situated within the components TCSR framework and influence the adopter’s (i.e., instructor’s) uptake of RBIS (i.e., the change) within the organization (i.e., institution). Evaluation of these malleable factors allows for insights and recommendations for change agents to provide opportunities that meet the needs of adopters in the different stages of adoption. Therefore, the TCSR and CACAO models are congruent and complementary, and together, situate our study.
Target courses are general chemistry, single-variable calculus, and quantitative-based introductory physics. Target institutions are two-year associate-degree granting institutions in the United States that offer all three of these target courses, and four-year bachelor’s and/or graduate degree-granting institutions that have conferred at least one bachelor’s degree in all three disciplines (i.e., chemistry, mathematics, and physics) between 2011 and 2016 as recorded by the National Center for Education Statistics’ Integrated Postsecondary Education Data System. Target participants are primary instructors for one of the three target courses that was not taught exclusively online in the 2017–2018 or 2018–2019 academic years in the United States.
A database of the target instructors was assembled through stratified consensus sampling centered around target institution type; the objective was to construct a representative sample of two-year institutions, four-year institutions, and universities to capture the different types of degree-granting institutions (i.e., associate, bachelor’s, and graduate, respectively). The database was constructed by the American Institute of Physics Statistical Research Center using publicly available online information and by contacting department chairs at the target institutions. The database contains 18,337 instructors that have met these criteria and is comprised of 8,933 instructors at two-year associate-degree granting institutions and 9,404 instructors at four-year bachelor’s and/or graduate degree-granting institutions.
Previous large-scale studies in postsecondary chemistry (Gibbons et al., 2018; Stains et al., 2018), mathematics (Johnson et al., 2018; Apkarian et al., 2019), and physics (Henderson and Dancy, 2009; Walter et al., 2016, 2021) informed the development of the survey instrument. The survey is comprised of five main elements: (1) course context, (2) instructional practices, (3) awareness and usage of active learning instructional techniques, (4) perceptions, beliefs, and attitudes related to students, learning, and departmental context, and (5) personal demographics and experience. Previous instruments and scales with reliability and validity evidence were used where applicable, e.g., mindset (Dweck et al., 1995) and the EBIP Adoption scale (Landrum et al., 2017).
Survey instrument data were collected by the American Institute of Physics Statistical Research Center between March–May 2019 with approval from the Western Michigan University Institutional Review Board (application no. 17-06-10); informed consent was obtained digitally. Survey respondents included 3,769 instructors (20.5% unit response rate) consisting of 1,244 chemistry, 1,349 mathematics, and 1,176 physics instructors; there were 1,099 instructors at two-year institutions and 2,670 instructors at four-year institutions. A total of 1,466 respondents were removed from this analysis due to incomplete responses for all the survey items used in the construction of the multilevel models. This resulted in the study sample of 2,303 respondents including 768 chemistry, 751 mathematics, and 784 physics instructors from 1,371 departments at 741 institutions; of these 2,303 instructors, 599 instructors are at two-year institutions and 1,704 instructors are at four-year institutions. Table 2 presents the institution type, discipline, and academic rank of the respondents included in this study; the group proportions of the respondents included in this study mirror that of the full survey sample as previously reported (Apkarian et al., 2021), and thus, can be considered as representative sample of the target population.
A full list of survey items and their coding used in this study can be found in our previous work (see Yik et al., 2022). The RBIS Adoption Scale (described below) resulted in the binary outcome variables used in the multilevel logistic regression models (described below). Factors used in this survey are described above in Table 1.
Respondents were classified by their discipline (reference: mathematics), highest degree offered by their department (reference: associate degree), and tenure status (reference: instructors with no opportunity to earn tenure). Respondents were asked about their class size; teaching load; role of student evaluations of teaching in decisions of review, promotion, or tenure; role of assessment of teaching performance in decisions of review, promotion, or tenure; growth mindset; and satisfaction with student learning. Ordinal scales for each of these variables are described in the Results (Table 3, below), with the exception of student evaluation of teaching (role of student evaluation of teaching in review, promotion, or tenure compared to other measures: 0 = not used, 1 = less weight, 2 = equal weight, 3 = more weight, 4 = only used; reference: not used). Class size is grand-median centered at 30–39 students. Growth mindset is the average of three items on a six-point Likert scale (Dweck et al., 1995); items were reverse-coded, and values were centered at the middle of the scale. Satisfaction of student learning is a single item on a five-point Likert scale (very satisfied to very satisfied) and values were centered at the middle of the scale.
Respondents were also asked about teaching experiences: decision making authority in their course (i.e., respondent has sole decision-making authority or is in collaboration with others to make decisions), previous RBIS use in courses when then were a student, participation in the scholarship of teaching and learning, enrollment in teaching-focused coursework, participation in teaching-related workshops, and in teaching-related new faculty experiences; these are all binary variables (yes/no; except decision making, reference: sole decision-making authority). Additionally, respondents were asked about their overall time spent lecturing which is defined as the overall percent of time during regular class meetings that students spend listening to the instructor lecture or solve problems.
The RBIS Adoption Scale is an adaption of the EBIP Adoption Scale (Landrum et al., 2017); the sole difference is in wording of the instrument where “EBIP” is replaced by “RBIS” (Table 4). This instrument contains six items to be used as a Guttman scale with ‘yes’/‘no’ responses. Guttman scales are unidimensional (i.e., is a measure of a single construct: degree of RBIS adoption), ordinal (i.e., items are ordered from the “least agreement” statement to the “most agreement” statement), and deterministic (i.e., results are analyzed based on the last statement the respondent agreed with; Guttman, 1944).
Guttman scales are self-scoring and is indicated when the response pattern changes from an agreement (‘yes’) to disagreement (‘no’). Deviations from this pattern, such as an agreement to a later item after a disagreement on an earlier item, indicate a lack of reliability of the scale and unidimensional measure of the construct. Four statistical measures characterize the quality of responses when using a Guttman scale: coefficient of reproducibility (CR), minimal marginal reproducibility (MMR), percent improvement (PI), and coefficient of scalability (CS; McIver and Carmines, 1981).
Together, the CR, MMR, PI, and CS aid in evaluating the reliability and unidimensionality of a Guttman scale (McIver and Carmines, 1981). First, the CR is a measure of the reliability of a Guttman scale and describes the proportion of differences between the observed and expected response pattern (Guttman, 1944). One issue with the CR is its sensitivity to extreme marginal distributions; in other words, if there are extreme patterns of responses or if respondents respond with extreme patterns, such as answering all scale items with the response ‘yes’ (Menzel, 1953; Guest, 2000). Second, the MMR reflects the reproducibility of the items based on the marginal distribution of the items; the value of MMR is the smallest value of CR that is possible given the observed proportion of agreement and disagreement responses for the items (Sudweeks, 2018). Third, PI is the difference between CR and MMR and reflects the improvement due to item ordering (McIver and Carmines, 1981). Finally, the CS is a measure of the predictability of the scale, or the proportion of responses that can be correctly predicted from the row and column marginals, and was introduced to combat artificially high CR (Menzel, 1953; Guest, 2000).
To demonstrate evidence of reliability and unidimensionality of the RBIS Adoption Scale, CR, MMR, PI, and CS values are calculated for the survey sample and for each discipline (i.e., chemistry, mathematics, and physics). For all data used in this study, every respondent provided full responses for the RBIS Adoption Scale (i.e., no items were left blank). Responses were ordered, and scale errors and marginal errors were calculated using the Goodenough–Edwards method (Goodenough, 1955; Edwards, 1957) to compute CR, PI, MMR, and CS values (Guest, 2000; Aiken and Groth-Marnat, 2006). For evidence of unidimensionality in a Guttman scale, CR > 0.90 and CS > 0.60 (Menzel, 1953; Guest, 2000; Aiken and Groth-Marnat, 2006; Abdi, 2010) are recommended standards along with lower MMR and larger PI values. The RBIS Adoption Scale demonstrates acceptable reliability for the study sample and three STEM disciplines that comprise the sample (Table 5).
Models were constructed using the melogit package in Stata version 17 (StataCorp, 2021) using mean and variance adaptive Gauss–Hermite quadrature (mvaghermite) integration with seven integration points. Predictor variables used in these models (see Table 1, above, and Table 3, below) have been previously reported (Yik et al., 2022) through a review of the research literature on malleable factors that affect the uptake of active learning strategies from a variety of STEM disciplines.
Multilevel models are advantageous when considering that participants are not independent from one another as they can be grouped by department or institutions, and thus, violate the assumption that observations are independent (Raudenbush and Bryk, 2002; Snijders and Bosker, 2012). Herein, multilevel regression models are used to explain two outcomes: RBIS tryout (i.e., Tryout Model) and RBIS adoption (i.e., Adoption Model). In the EBIP Adoption Scale by Landrum et al. (2017), each item is mapped onto one of the CACAO adoption stages (Dormant, 2011); in this study, we combine the mental tryout and hands-on tryout stages into single stage, tryout (see Table 4, above). Strategies suggested by the CACAO model for mental tryout include demonstrating examples of change and highlighting success, and strategies suggested for hands-on tryout include providing training, information, and resources (Dormant, 2011). We combine the two tryout stages because strategies are commonly employed together in teaching-related workshops and experiences (Henderson, 2008; Ebert-May et al., 2011; Baker et al., 2014), and we also combine the three distinct adoption stages because instructors in these later stages have been reported to share similar characteristics and teaching practices (Viskupic et al., 2022). The distribution of RBIS awareness, tryout, and adoption of the respondents in this study is given in Table 6.
Two multilevel logistic regression models are used to evaluate the association of the 17 malleable factors with RBIS tryout and RBIS adoption. Two models are used to distinguish between awareness and tryout (i.e., Tryout Model), and also, tryout and adoption (i.e., Adoption Model). We differentiate the two multilevel logistic regression models into what could be a single multinomial logistic regression model; however, this would result in a set of two different regression coefficients from a reference stage of adoption. A single multilevel ordinal logistic regression model could also be used to model the data, but this model assumes that the odds between each of the adoption stages are equivalent and proportional. Previous work has demonstrated that the needs of instructors are different depending on what stage of adoption they are at (Viskupic et al., 2022). For more parsimonious and interpretable regression coefficients from which we can provide recommendations to change agents, we therefore model two different outcomes (i.e., tryout and adoption) using two multilevel logistic regression models.
Two multilevel models are reported herein: the Tryout Model and Adoption Model. The Tryout Model includes sample of 1,079 respondents including 360 chemistry, 430 mathematics, and 289 physics instructors from 826 departments at 579 institutions; of these 1,079 instructors, 327 instructors are at two-year institutions and 752 instructors are at four-year institutions. The Adoption Model includes sample of 1,757 respondents including 591 chemistry, 501 mathematics, and 665 physics instructors from 1,118 departments at 663 institutions; of these 1,757 instructors, 419 instructors are at two-year institutions and 1,338 instructors are at four-year institutions.
The two multilevel models in this study are three-level models that are used to evaluate the association of malleable factors on the stages of RBIS adoption in introductory chemistry, mathematics, and physics. The intraclass correlation coefficient (ICC) is an index of the proportion of variance in the outcome variable that is explained by groups or clusters, and is the ratio of the between group variance and the total variance (Raudenbush and Bryk, 2002; Snijders and Bosker, 2012). Calculations for ICC values were performed as outlined in Liu (2015). The unconditional Tryout Model has an ICC of 0.11 for level 2 (department) and an ICC of 0.08 for level 3 (institution), meaning roughly, 11% of the variation in the outcome variable (i.e., stage of adoption: awareness or tryout) is accounted for by nesting instructors within departments and 8% of the variation in the outcome variable is accounted for by nesting departments within institutions. The unconditional Adoption Model has an ICC of 0.08 for level 2 and 0.04 for level 3. Small ICC values suggest that a two-level model would be appropriate; however, model misspecification results in less accurate fixed effect and standard error estimates, and inflation of lower-level variance estimates (Chen, 2012). Therefore, we specify the data using the more conceptually appropriate three-level model: instructors (level 1) are nested within departments (level 2) that are nested within institutions (level 3). Other studies (e.g., Porter and Umbach, 2001; Smart and Umbach, 2007; Yik et al., 2022) also use and advocate for these three-level models in similar contexts. Our previous work (Yik et al., 2022) describes the multilevel model used to evaluate the association of these same malleable factors with percent time lecturing.
We report the results of a national survey on the stages of RBIS adoption in introductory chemistry, mathematics, and physics in the United States. Data were collected and are modeled using three-level regression models based on the nested nature of the instructors (level 1) within departments (level 2) at institutions (level 3). 17 factors, comprised of 10 contextual, five personal, and two teacher thinking factors, are categorized using the TCSR model (Woodbury and Gess-Newsome, 2002; Gess-Newsome et al., 2003) in previous work (Yik et al., 2022). We report the results of two multilevel logistic regressions (i.e., RBIS Tryout and RBIS Adoption) using odd ratios (OR) which indicate the strength of the association between a predictor variable with the outcome variable (i.e., RBIS Tryout or Adoption) when all other predictor variables are accounted for in the model and held constant (Table 3).
Odds ratios are interpreted as the number of times higher when a variable is not zero when all other variables are held constant. For example, in the Tryout Model, academic discipline (i.e., chemistry, mathematics, physics) is evaluated using mathematics as the reference. When all other variables are held constant, the odds of RBIS tryout vs. RBIS awareness are not statistically different for instructors in chemistry departments (OR = 0.92, p > 0.05) than instructors in mathematics departments. However, the odds of RBIS tryout vs. RBIS awareness are 2.13 times higher for instructors in physics departments than for instructors in mathematics departments when all other variables are held constant. Odds ratios for the Adoption Model can be interpreted analogously where the odds ratio now represents the odds of RBIS adoption vs. RBIS tryout.
The Tryout Model and the Adoption Model both share some overlap in the statistically significant factors, but the models are also very different. Differences in the models further demonstrate (and corroborate the assumption) that two multilevel models better represent the data than a single multilevel ordered logistic regression model (i.e., proportional odds model) or multilevel multinomial logistic regression model. For the Tryout Model, statistically significant malleable factors include physics, class size, the interaction effect between class size and classroom setup, RBIS use as a student, teaching-related workshops, and satisfaction with student learning (see Table 3). For the Adoption Model, statistically significant malleable factors include physics, assessment of teaching performance, class size, classroom setup, RBIS use as a student, scholarship of teaching and learning, teaching-related new faculty experiences, growth mindset, and satisfaction with student learning (see Table 3).
Our previous work (Yik et al., 2022) detailed the association of these malleable factors with percent lecturing. Stage of RBIS adoption and percent lecturing are two measures of active learning and comparison of these outcomes can provide further insight into the similarities and differences between these models. Table 7 presents the association of the stage of RBIS adoption with percent lecturing. A Kendall’s tau-b correlation was calculated to determine the relationship between the stage of RBIS adoption and percent lecturing among the 2,266 respondents that provided useable data for all survey items used in this study including the RBIS Adoption Scale and percent lecturing. There is a medium-sized, negative association between stage of RBIS adoption and percent lecturing (τb = −0.335, p < 0.001); increasing stage of RBIS adoption is associated with a decrease in percent lecturing.
Visualizations comparing the magnitude of the each of the instructor-level malleable factors between the Tryout Model and Percent Lecturing Model (Figure 1A) and the Adoption Model and Percent Lecturing Model (Figure 1B) are provided. In summary, nine malleable factors are statistically significantly associated with percent time lecturing: class size; classroom setup; the interaction effect between class size and classroom setup; decision making; RBIS use as a student; scholarship of teaching and learning; teaching-related workshops, new faculty experiences, and coursework; and growth mindset (Yik et al., 2022).
Figure 1. Association of instructor-level malleable factors between (A) RBIS Tryout and Percent Lecturing Models and (B) RBIS Adoption and Percent Lecturing Models. *p < 0.05, **p < 0.01, ***p < 0.001. Scaled magnitudes of odds ratios are shown on the left vertical axis (RBIS Tryout and Adoption) and scaled magnitudes of percentages are shown on the right vertical axis (Percent Lecturing). Additional levels for ordinal variables are not shown. The gray horizontal line indicates an odds ratio of one (RBIS Tryout and Adoption) and 0 % change (Percent Lecturing). Values above the horizontal line indicate of increased odds of (A) RBIS Tryout or (B) RBIS Adoption and decreased percent time lecturing; values below the horizontal line indicate of increased odds of (A) RBIS Awareness or (B) RBIS Tryout and increased percent time lecturing. Connecting lines indicate association between the same significant factor in both models, or between a factor that is significant in one model and not the other model.
In comparing the Tryout Model and Percent Lecturing Model (Figure 1A), there are fewer instructor-level malleable factors associated with RBIS tryout than percent lecturing. Similarly, across the models, experience with RBIS as a student and participation in teaching-related workshops are associated with RBIS tryout and a decrease in percent lecturing. Inversely, larger class sizes are associated with RBIS tryout, but an increase in percent lecturing. Additionally, the interaction effect of larger class sizes in rooms that allow for group work and dissatisfaction with student learning are associated with RBIS tryout but are not significantly associated with percent lecturing.
In comparing the Adoption Model and Percent Lecturing Model (Figure 1B), there are nearly an equal number of instructor-level malleable factors associated with RBIS tryout as percent lecturing, however, there are differences. Classroom allowing for group work is the factor most strongly associated with RBIS adoption and decrease in percent lecturing. Other strongly associated factors in both models include engagement in SOTL/DBER, experience with RBIS as a student, participation in teaching-related workshops and new faculty experiences, and holding a growth mindset. Similarly, larger class sizes are associated with adoption of RBIS and increase in percent lecturing. However, satisfaction with student learning is associated with RBIS adoption, but is not significantly associated with percent lecturing. Two factors, shared decision making and previous teaching-focused coursework, are associated with a decrease in percent lecturing, but are not significantly associated with RBIS adoption.
Multiple factors are associated with an increase in tryout and adoption of RBIS at the instructor level, when all other factors are held constant: assessment of teaching performance, class size, classroom setup, the interaction effect between class size and classroom setup, RBIS use as a student, participation in the scholarship of teaching and learning or discipline-based education research, teaching-related workshops, teaching-related new faculty experiences, holding a growth mindset, and satisfaction with student learning. Factors unique to either the Tryout Model or the Adoption Model allow for tangible recommendations for instructors in each group (i.e., those seeking to tryout an RBIS or those seeking to formally adopt an RBIS). Department-level factors (e.g., discipline and highest degree awarded) are unchangeable and thus lack formal implications in the context of this study; however, instructor-level factors can lead to tangible and practical implications for change efforts, and therefore, we will focus our discussion on these malleable instructor-level factors.
Instructors continually note that teaching large classes in large fixed-seating classrooms (i.e., auditorium-style lecture halls) make it difficult to promote student engagement and use RBIS (Henderson and Dancy, 2007; Hora, 2012; Lund and Stains, 2015; Sturtevant and Wheeler, 2019). Additionally, classrooms that can accommodate for group work, such as active learning classrooms or rooms with movable seats, desks, or tables, provide environments that are conducive to fostering student–student and student-instructor interactions (Beichner et al., 2007; Beichner, 2008; Cotner et al., 2013; Lund and Stains, 2015; Foote et al., 2016; Knaub et al., 2016). Our results indicate that instructors in the tryout and adoption stages are more likely to use RBIS as the class size becomes larger when compared to instructors in the awareness and tryout stages, respectively. However, instructors in the tryout stage are more likely than instructors in the awareness stage to implement RBIS regardless of the classroom space, but instructors in the adoption stage need (and potentially require or demand) classrooms that allow for group work. While class sizes have been reported to ease the facilitation of student engagement (Bressoud et al., 2015), our results indicate that instructors are less likely to use RBIS for smaller (< 30 students) class sizes.
Active learning, though, can occur in any classroom environment. Increasing odds of an instructor using RBIS when teaching larger courses suggest that uptake of RBIS is a means to make class sizes seem smaller by encouraging discussion and collaboration (Robert et al., 2016; Beane et al., 2020; Raker et al., 2021). For example, when large auditorium-style lecture halls are fitted with swivel chairs, students have been demonstrated to outperform their counterparts in a fixed-seat lecture hall (Ogilvie, 2008; Condon et al., 2016), which may be attributed to the room layout promoting discussion and collaboration. It has also been reported in the literature that various levels of student–student interactions can still be implemented with large class sizes in auditorium-style rooms (Lund et al., 2015).
Teaching large enrollment courses in classrooms that allow for group work is critical for instructors trying out RBIS. Classroom spaces designed to accommodate flexible teaching spaces can help aid in reducing barriers to implementing active learning (Ellis et al., 2016). These spaces may also help sustain the adoption of active learning practices due to the efforts it takes to learn about and support the implementation of these RBIS (Knaub et al., 2016). Instructors that have adopted RBIS may request to teach in such spaces because the space facilitates the use of active learning pedagogies, which can lead to further and sustained adoption (Foote et al., 2016).
Instructors’ perceived value of how their department or institution values the assessment of teaching performance is influential in their pedagogies. Incentives guide instructors’ professional decisions; for example, departments and institutions may require instructors to adopt RBIS in their teaching as a part of review, promotion, or tenure packages (Lund and Stains, 2015). Alternatively, if there are no structures in place to evaluate and reward instructors’ teaching, then there can be little external incentive to adopt RBIS in their classes (Hativa, 1995; Walczyk et al., 2007; Brownell and Tanner, 2012; Elrod and Kezar, 2017; Shadle et al., 2017; Johnson et al., 2018). Our results suggest that greater the perceived influence of assessment performance on instructors’ review, promotion, or tenure, the greater the odds of adoption of RBIS.
While instructors may be knowledgeable about and tryout RBIS, active learning strategies are most effective when instructors are committed to the pedagogy and are provided with ongoing support (Bressoud et al., 2015). For instructors to perceive emphasis on teaching, departmental (e.g., faculty and chairs) and institutional support (e.g., college, provost, dean) are needed for sustained change (Henderson and Dancy, 2007; Shadle et al., 2017; Carney et al., 2021; Dunnigan and Halcrow, 2021; Mingus and Koelling, 2021). Support of these endeavors can also come from other departments, centers for teaching and learning, and professional organizations (Mingus and Koelling, 2021). Departments and institutions can showcase value of teaching by rewarding instructors for their efforts in learning about, trying out, and adopting active learning strategies (Fairweather, 2008; Seymour et al., 2011; Wieman, 2015). Institutions can also support emphasis on adopting RBIS by providing travel support to external teaching-focused professional development programs and workshops (Reinholz and Apkarian, 2018; Carney et al., 2021), or small stipends or service credits to engage in institutional programs (Lotter et al., 2007; Foote et al., 2016; Herman et al., 2018; Reinholz and Apkarian, 2018).
The attitudes, beliefs, and intentions of instructors are partly a result of their own undergraduate and graduate education shaping the way that instructors currently enact pedagogies (Oleson and Hora, 2014; Lund and Stains, 2015; Fukawa-Connelly et al., 2016). Many instructors may have experienced more traditional, lecture-based instructional modalities as students, and thus, they may imitate these teaching styles in their own teaching practice (Adamson et al., 2003). However, instructors who experienced active learning a student have been reported to be more likely to implement active learning in their classes as an instructor (Lund and Stains, 2015; Yik et al., 2022). Our results support this idea; instructors who experienced RBIS as a student are more likely to tryout and adopt RBIS as an instructor. Experience with RBIS as a student is the highest influencing factor on RBIS tryout in our model; it is the among the highest for RBIS adoption, alongside class size and classroom effects.
The next generation of instructors, thus, will hopefully be greatly influenced by how we teach today. Our results point to the notion that “we teach the way we were taught” (Mazur, 2009). However, it is possible that instructors who use RBIS are more likely to recall the teaching strategies they experienced as a student, and those instructors who do not use RBIS are less likely to recall RBIS experiences when they were a student. Regardless, for lasting sustainable change in adopting active learning practices in introductory STEM courses, current instructors must adopt RBIS in their classes to influence the thinking of future instructors (i.e., current undergraduate and graduate students). Therefore, instructors must be incentivized to participate in teaching-related professional development and the scholarship of teaching and learning to assist in the adoption of RBIS.
Teaching-related workshops have many different forms from being more broad, such as how to manage a classroom and use the learning management system, to being more specific, such as on how to implement active learning strategies (e.g., Aebersold, 2019; Miller et al., 2021). Workshops have largely placed emphasis on develop–disseminate efforts to change individual instructor’s teaching practices (Beach et al., 2012; Borrego and Henderson, 2014), which stems from the belief that teaching is an individualistic effort (Tanner and Allen, 2006; Lane et al., 2019). Some studies suggest that these models for instructional change are generally ineffective (Henderson et al., 2011), and therefore, may not result in the desired widespread changes (Fairweather, 2008; Austin, 2011; Kezar, 2011; Froyd et al., 2017). However, our results demonstrate that participation in workshops aids instructors in trying out RBIS. Additionally, while participation in teaching-related new faculty experiences may not initially play a role in RBIS tryout, the effects of these experiences are prolonged by exhibiting a significant role in RBIS adoption.
Change can take place at many different levels, ranging from an individual instructor (Steinert et al., 2006), to departments [American Association of Colleges and Universities (AACU), 2014], and entire institutions (Elrod and Kezar, 2016). While change strategies may target a certain level, we recommend that change agents at all levels work together and support one another to achieve desired changes (American Association for the Advancement of Science (AAAS), 2019). Instructors should be active participants in their teaching roles and in learning about and adopting new teaching pedagogies (Fairweather, 2008; Smith et al., 2014; Quan et al., 2019). However, there can be a lack of incentives for instructors to participate in teaching-related professional development (Walczyk et al., 2007; Brownell and Tanner, 2012). External motivators to attend professional development events can greatly contribute to influencing instructors. Institutions and departments need to support, highly incentivize, and reward participation in these opportunities (Seymour et al., 2011; American Association for the Advancement of Science (AAAS), 2019; Bathgate et al., 2019b), and even stipends can act as a token of appreciation for instructors’ time and giving priority classroom preferences may sustain the adoption of RBIS (Soto and Marzocchi, 2021).
Continuing professional development is critical for the sustained implementation of active learning approaches (Speer and Wagner, 2009; Camburn, 2010; Quan et al., 2019; Pilgrim et al., 2021). Change in instructors’ beliefs and practices occur slowly (Derting et al., 2016). To facilitate successful change, interventions (e.g., teaching-related professional development) should last a semester or longer (Henderson et al., 2011). As time progresses, instructors may regress back to old teaching habits. It is necessary for instructors to continually engage with opportunities to learn about and demonstrate new teaching pedagogies (Brownell and Tanner, 2012). These opportunities should also be diverse across a continuum to assist instructors in their incremental growth in implementing active learning strategies (Soto and Marzocchi, 2021); our results show that instructors at the differing stages of adoption (i.e., awareness, tryout, and adoption) have different needs, and therefore, programs must be designed for instructors at various stages (Austin and Sorcinelli, 2013; Borda et al., 2020). Both teaching-related new faculty experiences and broader workshops are crucial for tryout and adoption of RBIS, and our results suggest that such programs should continue to be funded and operated.
Centers for teaching and learning serve as important resources for instructors to learn about, obtain advice on, and get help to properly implement RBIS. Additionally, these centers can support sustained adoption of RBIS through faculty learning communities (Cox, 2004; Pelletreau et al., 2018; Shadle et al., 2018; Dancy et al., 2019) and communities of practice (Henderson et al., 2017; Tomkin et al., 2019; Benabentos et al., 2021). One method is to incorporate faculty learning communities or communities of practice as a component of an extended (i.e., longer than one semester) new faculty experience (Beane-Katner, 2013). At institutions where centers for teaching and learning do not exist, instructor-organized communities may be effective at facilitating adoption of RBIS (Ma et al., 2019). Instructors can discuss teaching pedagogies through observation and feedback (Gormally et al., 2014; Smith et al., 2014) or successful adopters of RBIS may also engage with instructors trying out RBIS as form of peer-coaching (Desimone and Pak, 2017; Ma et al., 2018).
Engagement in the scholarship of teaching and learning (SOTL) has been reported to be related with improvements in course transformations (Henderson et al., 2011) and it is believed that practicing the closely related field of discipline-based education research (DBER) yields similar results (Henderson et al., 2012). Work has shown that instructors engaged in SOTL employed instructional practices that were more student-focused (Pelletreau et al., 2018; Dancy et al., 2019; Tomkin et al., 2019; Benabentos et al., 2021; Yik et al., 2022). In our study, participation in SOTL or DBER was associated with the RBIS adoption, but not RBIS tryout. Engagement in SOTL is an approach to develop reflective educators (Henderson et al., 2011). By agreeing to the statement, “I have evidence that my teaching has improved since I started using RBIS” in the RBIS Adoption Scale (Table 2), instructors are testifying to engaging in SOTL in their teaching. This leads to the ambiguity whether adoption of RBIS leads to SOTL or engaging in SOTL leads to the adoption of RBIS; regardless, instructors are employing active learning strategies and are becoming reflective educators.
Instructors participating in SOTL or DBER can help advance the adoption of RBIS of other instructors. Our previous study (Yik et al., 2022) detailed the association of shared decision on instructional methods with decreased time lecturing, and thus, increased time spent on active learning. Other work (Lane et al., 2020) suggested that instructors predominantly talk to other instructors with similar teaching approaches, i.e., RBIS users talk with other RBIS users. One approach to guide instructors to higher stages of RBIS adoption is through co-teaching (e.g., Henderson et al., 2009) or course coordination (e.g., Apkarian and Kirin, 2017), which can involve instructors at earlier or mid-stages of RBIS adoption (i.e., awareness and tryout) with SOTL, and also support sustained change and continuous course improvement over an extended period of time (Marbach-Ad et al., 2007, 2014; Reinholz and Apkarian, 2018; Mingus and Koelling, 2021).
Instructors’ mindset has been shown to influence pedagogical decisions (Rattan et al., 2012; Aragón et al., 2018; Canning et al., 2019; Ferrare, 2019; Bathgate et al., 2019a; Richardson et al., 2020). Instructors holding a fixed mindset (i.e., student intelligence is fixed and cannot be changed; Dweck, 1999) adopt fewer active learning practices (Rattan et al., 2012; Aragón et al., 2018), and lecture more (Yik et al., 2022); correspondingly, instructors holding a growth mindset (i.e., student intelligence is malleable and can be improved with time and experience; Dweck, 1999) are more willing to consider (Johnson et al., 2018) and adopt more active learning practices (Aragón et al., 2018), and lecture less (Yik et al., 2022).
Our results show that mindset is not significantly associated with trying out RBIS but holding a growth mindset is significant in the adoption of RBIS; this finding mirrors previous findings that instructors’ growth mindset beliefs is associated with a decrease in time spent lecturing (Yik et al., 2022). Fixed mindset beliefs are associated with greater odds of RBIS tryout than adoption, which may explain why instructors leave the innovation-decision process when using RBIS after an initial implementation (Henderson et al., 2012). Alternatively, espousing a growth mindset may increase instructors’ persistence when adopting RBIS (Limeri et al., 2020).
Professional development workshops and experiences are possible avenues to promote instructors’ growth mindset (Pilgrim et al., 2021; Yik et al., 2022). However, instructors have differences in their levels of motivation to participate in teaching-related professional development (Woodbury and Gess-Newsome, 2002; Bouwma-Gearhart, 2012; McCourt et al., 2017). Interventions to promote growth mindset for students have been demonstrated to be effective, generalizable, and replicable (Dweck and Leggett, 1988; Dweck, 1999; Yeager et al., 2016; Bettinger et al., 2018; Yeager et al., 2019), and psychosocial interventions can be leveraged to motivate instructors to participate in professional development that can emphasize growth mindset beliefs (see Limeri et al., 2020). This is particularly imperative because instructors’ perceived mindsets have effects on students; students who perceive their instructors exhibiting growth mindsets are reported to have higher academic success, and positive motivational and psychological outcomes (Rattan et al., 2018; Fuesting et al., 2019; Lou and Noels, 2020; Muenks et al., 2020; LaCrosse et al., 2021), and this perception is strengthened with using active learning practices (Muenks et al., 2021). By holding a growth mindset, instructors are more likely to adopt RBIS and active learning, and resultingly, positively influence student outcomes.
The misalignment of teaching practices with instructional goals and student outcomes can result in instructors’ dissatisfaction with student learning or current instructional methods (Southerland et al., 2011a,b). The result of this disconnect between teacher thinking and practice can consequently lead to the adoption of new teaching strategies, such as RBIS (Feldman, 2000; Windschitl and Sahl, 2002; Gess-Newsome et al., 2003; Andrews and Lemons, 2015; Lund and Stains, 2015; Gibbons et al., 2018).
Our findings suggest that dissatisfaction with student learning is associated with the tryout of RBIS and the satisfaction with student learning is associated with the adoption of RBIS. Instructors’ dissatisfaction with student learning may stem from dissatisfaction with the current pedagogy, which can include current RBIS use, and result in a change of teaching practices, and therefore, the trying out using (different) RBIS (Gess-Newsome et al., 2003). It can be postulated that once instructors are satisfied with their students’ learning, it is due to the implemented pedagogies, and this satisfaction can lead to the sustained adoption of RBIS.
Change agents can leverage instructors’ dissatisfaction or satisfaction with students learning in change strategies. For example, dissatisfied instructors are more likely to tryout RBIS. These instructors would be prime targets to engage in teaching-related workshops to learn about RBIS, be shown evidence of success using RBIS, and be provided training and resources to implement RBIS (Dormant, 2011). Additionally, satisfied instructors are more likely to adopt RBIS. These instructors can not only benefit from learning about and experimenting with new RBIS, but more importantly, having evidence that teaching has improved since using RBIS (Landrum et al., 2017). Change agents can identify these instructors as participants to engage in the scholarship of teaching and learning; by engaging in SOTL, instructors will have evidence that shows supported student learning, and thus, instructor satisfaction.
The malleable factors associated with RBIS tryout and RBIS adoption are similar, but also different, to the malleable factors associated with percent lecturing. In all three regression models, only one factor has an association with higher odds of RBIS tryout and adoption and a decrease percent lecturing: experience in a course using RBIS as a student. Therefore, it is vital we ensure that current instructors adopt RBIS such that our current students (i.e., our future instructors) are more likely to implement and adopt RBIS and active learning strategies in the future.
Tryout and adoption of RBIS and percent lecturing are different measures of active learning. To such an end, the malleable factors used to consider change strategies differ depending on the desire to influence RBIS tryout, RBIS adoption, or percent lecturing. Regardless of the exact malleable factors chosen to inform change, any of the factors significantly associated with any of the three outcomes (i.e., RBIS tryout, RBIS adoption, and percent lecturing) have greater odds of increasing the uptake of active learning strategies.
Findings from this study are constrained by three noteworthy limitations. First, this study is limited to the disciplines of chemistry, mathematics, and physics, and is therefore limited in disciplinary scope; additionally, survey respondents were comprised of instructors from the introductory courses of these disciplines, and is therefore limited in course scope. Other survey-based studies illustrate differences between lower-and upper-level STEM courses (Benabentos et al., 2021) and observation-based studies report instructors are more likely to implement RBIS in introductory courses than in more advanced courses (Lund et al., 2015). Inclusion of additional STEM disciplines and courses would be in productive space for future studies.
Second, higher education is a complex system. The TCSR framework focuses on instructor beliefs and instructors as the primary change agents at an institution (Gess-Newsome et al., 2003). In our conceptualization of the TCSR framework, we situate barriers and motivations for instructional change into contextual factors, personal factors, and teacher thinking factors; however, it would be unfeasible to model every single possible factor in a regression model. For example, our study does not consider student-level factors. Some students are reported to find active learning classes to be disjointed with an overall feeling of frustration and confusion and many studies note student resistance as a barrier to implement active learning strategies (Deslauriers et al., 2019; Owens et al., 2020).
Lastly, plausible reliability threats may be due to the self-reported nature of our survey items. While we have provided evidence for the reliability of the instrument (i.e., RBIS Adoption Scale) used to obtain the outcome measure (i.e., stage of RBIS adoption), there may be reliability threats to survey items that correspond to respondents’ contextual, personal, and teacher thinking factors. Nonetheless, studies have demonstrated evidence to suggest that self-reported data regarding teaching practices align well with observational data (Durham et al., 2018; Gibbons et al., 2018), and data from observation-based studies would complement data from survey studies.
Findings from this study are constrained by three noteworthy limitations. First, this study is limited to the disciplines of chemistry, mathematics, and physics, and is therefore limited in disciplinary scope; additionally.
We advocate for the sustained adoption of research-based instructional strategies to promote use of active learning in introductory STEM courses. There are boundless paths instructors and change agents can take to that lead to a student-centered classroom which incorporates active learning strategies, and instructors should adopt RBIS that best fits the characteristics of their unique classroom contexts (Budd et al., 2013; Beane et al., 2020). In this journey on instructional change, instructors must be first aware of RBIS, tryout these strategies, and then adopt the pedagogies. The RBIS Adoption Scale is a quick, efficient, and reliable instrument to gage instructors’ stage of RBIS adoption; using this information, change agents can help facilitate instructors’ course transformation journeys. We offer recommendations for change agents to provide directed opportunities for instructors.
Recommendations for targeting instructors aware of RBIS and are working toward trying out RBIS:
• Identify instructors that are dissatisfied with their students’ learning
• Design workshops that provide training and resources to implement RBIS, and highlight the success stories and research-based data of those RBIS
• Encourage instructors that teach large class sizes to seek out classrooms that allow for group work and introduce RBIS that large class sizes appear smaller
Recommendations for targeting instructors trying out RBIS and are working toward adopting RBIS:
• Demonstrate the need to assess student learning with evidence when RBIS are implemented
• Engage instructors in SOTL so that instructors have the knowledge and resources to obtain evidence of enhanced student learning
• Encourage instructors to teach in classrooms that allow for group work, irrespective of class sizes
• Help foster growth mindset beliefs through interventions or other professional development programs
Recommendations for institutional leaders and policy makers:
• Value and reward teaching and instructors’ efforts in instructional change
• Build classroom spaces that allow for group work
• Encourage and incentivize the participation in teaching-related new faculty experiences and workshops
• Showcase benefits of RBIS and promote the uptake of RBIS use
The results from our national survey of introductory chemistry, mathematics, and physics instructors that evaluates the association of malleable factors with the adoption of active learning inform these recommendations for change agents, institutional leaders, and policy makers. Our goal is for these recommendations to inform instructor-focused change initiatives that result in meaningful and sustainable change around teaching practices.
The raw data supporting the conclusions of this article will be made available by the authors, without undue reservation.
The studies involving human participants were reviewed and approved by Western Michigan University. The patients/participants provided their written informed consent to participate in this study.
CH, MD, EJ, JR, and MS obtained financial support and conceived of the study. CH, MD, EJ, JR, MS, and NA designed the survey. NA cleaned and compiled the data. BY, JR, NA, and MS analyzed the data. BY carried out the formal analysis of the data and wrote the paper. All authors contributed to the article and approved the submitted version.
This material is based upon work supported by the National Science Foundation under grant nos. DUE 1726126 (JR), 2028134 (MS), 1726328 (CH), 1726042 (MD), and 1726281 (EJ).
The authors would like to thank Eun Sook Kim (University of South Florida) for aid in the development and interpretation of the multilevel models. We would like to thank all the instructors that participated in this study.
The authors declare that the research was conducted in the absence of any commercial or financial relationships that could be construed as a potential conflict of interest.
All claims expressed in this article are solely those of the authors and do not necessarily represent those of their affiliated organizations, or those of the publisher, the editors and the reviewers. Any product that may be evaluated in this article, or claim that may be made by its manufacturer, is not guaranteed or endorsed by the publisher.
Any opinions, findings, and conclusions or recommendations expressed in this material are those of the authors and do not necessarily reflect the views of the National Science Foundation.
Abdi, H. (2010). “Guttman scaling,” in Encyclopedia of research design. ed. N. J. Salkind (Thousand Oaks, CA: Sage), 559–560.
Adamson, S. L., Banks, D., Burtch, M., Cox, F. III, Judson, E., Turley, J. B., et al. (2003). Reformed undergraduate instruction and its subsequent impact on secondary school teaching practice and student achievement. J. Res. Sci. Teach. 40, 939–957. doi: 10.1002/tea.10117
Aebersold, A. (2019). The active learning institute: design and implementation of an intensive faculty development program. J. Cent. Teach. Learn. 11, 24–38.
Aiken, L. R., and Groth-Marnat, G. (2006). Psychological testing and assessment. Boston, MA: Pearson.
American Association for the Advancement of Science (AAAS) (2019). Levers for change: An assessment of progress on changing STEM instruction. Washington, DC.
American Association of Colleges and Universities (AACU) (2014). Achieving systemic change: A sourcebook for advancing and funding undergraduate STEM education. Washington, DC.
Anderson, R. D. (2002). Reforming science teaching: what research says about inquiry. J. Sci. Teach. Educ. 13, 1–12. doi: 10.1023/A:1015171124982
Andrews, T. C., and Lemons, P. P. (2015). It’s personal: biology instructors prioritize personal evidence over empirical evidence in teaching decisions. CBE life. Sci. Educ. 14:ar7. doi: 10.1187/cbe.14-05-0084
Apkarian, N., Henderson, C., Stains, M., Raker, J., Johnson, E., and Dancy, M. (2021). What really impacts the use of active learning in undergraduate STEM education? Results from a national survey of chemistry, mathematics, and physics instructors. PLoS One 16:e0247544. doi: 10.1371/journal.pone.0247544
Apkarian, N., and Kirin, D. (2017). Progress through calculus: Census survey technical report. Mathematical Association of America.
Apkarian, N., Smith, W. M., Vroom, K., Voigt, M., and Gehrtz, J., SEMINAL Project Team. (2019). X-PIPS-M survey suite. Available at: https://www.maa.org/sites/default/files/XPIPSM%20Summary%20Document.pdf
Aragón, O. R., Eddy, S. L., and Graham, M. J. (2018). Faculty beliefs about intelligence are related to the adoption of active-learning practices. CBE Life Sci. Educ. 17:ar47. doi: 10.1187/cbe.17-05-0084
Austin, A. E. (2011). Promoting evidence-based change in undergraduate science education. Washington DC: National Academy of Sciences.
Austin, A. E., and Sorcinelli, M. D. (2013). The future of faculty development: where are we going? New Dir. Teach. Learn. 2013, 85–97. doi: 10.1002/tl.20048
Baker, L. A., Chakraverty, D., Columbus, L., Feig, A. L., Jenks, W. S., Pilarz, M., et al. (2014). Cottrell scholars collaborative new faculty workshop: professional development for new chemistry faculty and initial assessment of its efficacy. J. Chem. Educ. 91, 1874–1881. doi: 10.1021/ed500547n
Ballen, C. J., Wieman, C., Salehi, S., Searle, J. B., and Zamudio, K. R. (2017). Enhancing diversity in undergraduate science: self-efficacy drives performance gains with active learning. CBE Life Sci. Educ 16:ar56. doi: 10.1187/cbe.16-12-0344
Bathgate, M. E., Aragón, O. R., Cavanagh, A. J., Frederick, J., and Graham, M. J. (2019a). Supports: A key factor in faculty implementation of evidence-based teaching. CBE Life Sci. Educ. 18:ar22. doi: 10.1187/cbe.17-12-0272
Bathgate, M. E., Aragón, O. R., Cavanagh, A. J., Waterhouse, J. K., Frederick, J., and Graham, M. J. (2019b). Perceived supports and evidence-based teaching in college STEM. Int. J. STEM Educ. 6:11. doi: 10.1186/s40594-019-0166-3
Bauer, C., Libby, R. D., Scharberg, M., and Reider, D. (2013). Transformative research-based pedagogy workshops for chemistry graduate students and postdocs. J. Coll. Sci. Teach. 043, 36–43. doi: 10.2505/4/jcst13_043_02_36
Bazett, T., and Clough, C. L. (2021). Course coordination as an avenue to departmental culture change. PRIMUS 31, 467–482. doi: 10.1080/10511970.2020.1793853
Beach, A. L., Henderson, C., and Finkelstein, N. (2012). Facilitating change in undergraduate STEM education. Change 44, 52–59. doi: 10.1080/00091383.2012.728955
Beane, R. J., Altermatt, E. R., Iverson, E. R., and Macdonald, R. H. (2020). Design and impact of the national workshop for early career geoscience faculty. J. Geosci. Educ. 68, 345–359. doi: 10.1080/10899995.2020.1722787
Beane-Katner, L. (2013). Developing the next generation of faculty: taking a learning community approach. J. Cent. Teach. Learn. 5, 91–106.
Beichner, R. J. (2008). The SCALE-UP project: A student-centered active learning environment for undergraduate programs. Washington DC: National Academy of Sciences.
Beichner, R. J., Saul, J. M., Abbott, D. S., Morse, J. J., Deardorff, D. L., Allain, R. J., et al. (2007). The student-centered activities for large enrollment undergraduate programs (SCALE-UP) project. College Park, MD: American Association of Physics Teachers.
Benabentos, R., Hazari, Z., Stanford, J. S., Potvin, G., Marsteller, P., Thompson, K. V., et al. (2021). Measuring the implementation of student-centered teaching strategies in lower-and upper-division STEM courses. J. Geosci. Educ. 69, 342–356. doi: 10.1080/10899995.2020.1768005
Bettinger, E., Ludvigsen, S., Rege, M., Solli, I. F., and Yeager, D. (2018). Increasing perseverance in math: evidence from a field experiment in Norway. J. Econ. Behav. Organ. 146, 1–15. doi: 10.1016/j.jebo.2017.11.032
Bonwell, C. C., and Eison, J. A. (1991). “Active learning: creating excitement in the classroom,” in ASHE-ERIC higher education report. (Washington DC: School of Education and Human Development, George Washington University).
Borda, E., Schumacher, E., Hanley, D., Geary, E., Warren, S., Ipsen, C., et al. (2020). Initial implementation of active learning strategies in large, lecture STEM courses: lessons learned from a multi-institutional, interdisciplinary STEM faculty development program. Int. J. STEM Educ. 7:4. doi: 10.1186/s40594-020-0203-2
Borrego, M., Cutler, S., Prince, M., Henderson, C., and Froyd, J. E. (2013). Fidelity of implementation of research-based instructional strategies (RBIS) in engineering science courses. J. Eng. Educ. 102, 394–425. doi: 10.1002/jee.20020
Borrego, M., and Henderson, C. (2014). Increasing the use of evidence-based teaching in stem higher education: A comparison of eight change strategies. J. Eng. Educ. 103, 220–252. doi: 10.1002/jee.20040
Bouwma-Gearhart, J. (2012). Research university STEM faculty members’ motivation to engage in teaching professional development: building the choir through an appeal to extrinsic motivation and ego. J. Sci. Educ. Tech. 21, 558–570. doi: 10.1007/s10956-011-9346-8
Bressoud, D., Mesa, V., and Rasmussen, C. (2015). Insights and recommendations from the maa national study of college calculus. MMA Press.
Bressoud, D., and Rasmussen, C. (2015). Seven characteristics of successful calculus programs. Not. Am. Math. Soc. 62, 144–146. doi: 10.1090/noti1209
Brownell, S. E., and Tanner, K. D. (2012). Barriers to faculty pedagogical change: lack of training, time, incentives, and tensions with professional identity? CBE Life Sci. Educ. 11, 339–346. doi: 10.1187/cbe.12-09-0163
Budd, D. A., van der Hoeven Kraft, K. J., McConnell, D. A., and Vislova, T. (2013). Characterizing teaching in introductory geology courses: measuring classroom practices. J. Geosci. Educ. 61, 461–475. doi: 10.5408/12-381.1
Burke, K. A., Greenbowe, T. J., and Gelder, J. I. (2004). The multi-initiative dissemination project workshops: who attends them and how effective are they? J. Chem. Educ. 81:897. doi: 10.1021/ed081p897
Camburn, E. M. (2010). Embedded teacher learning opportunities as a site for reflective practice: an exploratory study. Am. J. Educ. 116, 463–489. doi: 10.1086/653624
Canning, E. A., Muenks, K., Green, D. J., and Murphy, M. C. (2019). STEM faculty who believe ability is fixed have larger racial achievement gaps and inspire less student motivation in their classes. Sci. Adv. 5:eaau4734. doi: 10.1126/sciadv.aau4734
Carney, D., Sanders, M., Spiegel, S., Swanson, R., and Vasquez, A. (2021). Calculus II course redesign: supporting faculty in pedagogical change. PRIMUS 31, 643–657. doi: 10.1080/10511970.2020.1746450
Chen, Q. (2012). The impact of ignoring a level of nesting structure in multilevel mixture model: A Monte Carlo study. SAGE Open 2:21582440124425110. doi: 10.1177/2158244012442518
Clark, G., Blumhof, J., Gravestock, P., Healey, M., Jenkins, A., Honeybone, A., et al. (2002). Developing new lecturers: the case of a discipline-based workshop. Active Learn. High. Educ. 3, 128–144. doi: 10.1177/1469787402003002003
Condon, W., Iverson, E. R., Manduca, C. A., Rutz, C., Willett, G., Huber, M. T., et al. (2016). Faculty development and student learning assessing the connections. Bloomington, IN: Indiana University Press.
Cotner, S., Loper, J., Walker, J. D., and Brooks, D. C. (2013). It's not you, it's the room—are the high-tech, active learning classrooms worth it? J. Coll. Sci. Teach. 42, 82–88.
Cox, M. D. (2004). Introduction to faculty learning communities. New Dir. Teach. Learn. 2004, 5–23. doi: 10.1002/tl.129
Cox, B. E., McIntosh, K. L., Reason, R. D., and Terenzini, P. T. (2011). A culture of teaching: policy, perception, and practice in higher education. Res. High. Educ. 52, 808–829. doi: 10.1007/s11162-011-9223-6
Dancy, M., and Henderson, C. (2010). Pedagogical practices and instructional change of physics faculty. Am. J. Phys. 78, 1056–1063. doi: 10.1119/1.3446763
Dancy, M., Lau, A. C., Rundquist, A., and Henderson, C. (2019). Faculty online learning communities: A model for sustained teaching transformation. Phys. Rev. Phys. Educ. Res. 15:020147. doi: 10.1103/PhysRevPhysEducRes.15.020147
Dean, C. B., Hubbell, E. R., Pitler, H., and Stone, B. (2012). Classroom instruction that works: Research-based strategies for increasing student achievement. Alexandria, VA: Association for Supervision and Curriculum Development.
Denaro, K., Kranzfelder, P., Owens, M. T., Sato, B., Zuckerman, A. L., Hardesty, R. A., et al. (2022). Predicting implementation of active learning by tenure-track teaching faculty using robust cluster analysis. Int. J. STEM Educ. 9:49. doi: 10.1186/s40594-022-00365-9
Deri, M. A., Mills, P., and McGregor, D. (2018). Structure and evaluation of a flipped general chemistry course as a model for small and large gateway science courses at an urban public institution. J. Coll. Sci. Teach. 047, 68–77. doi: 10.2505/4/jcst18_047_03_68
Derting, T. L., Ebert-May, D., Henkel, T. P., Maher, J. M., Arnold, B., and Passmore, H. A. (2016). Assessing faculty professional development in STEM higher education: sustainability of outcomes. Sci. Adv. 2:e1501422. doi: 10.1126/sciadv.1501422
Desimone, L. M., and Pak, K. (2017). Instructional coaching as high-quality professional development. Theory Pract. 56, 3–12. doi: 10.1080/00405841.2016.1241947
Deslauriers, L., McCarty Logan, S., Miller, K., Callaghan, K., and Kestin, G. (2019). Measuring actual learning versus feeling of learning in response to being actively engaged in the classroom. Proc. Natl. Acad. Sci. U. S. A. 116, 19251–19257. doi: 10.1073/pnas.1821936116
Dunnigan, G., and Halcrow, C. (2021). If you don't build it, they will leave: reforming an applied calculus course by eliminating large lectures and incorporating active learning. PRIMUS 31, 413–433. doi: 10.1080/10511970.2020.1769234
Durham, M. F., Knight, J. K., Bremers, E. K., DeFreece, J. D., Paine, A. R., and Couch, B. A. (2018). Student, instructor, and observer agreement regarding frequencies of scientific teaching practices using the measurement instrument for scientific teaching-observable (MISTO). Int. J. STEM Educ. 5:31. doi: 10.1186/s40594-018-0128-1
Dweck, C. S. (1999). Self-theories: Their role in motivation, personality, and development. Philadelphia: Psychology Press.
Dweck, C. S., Chiu, C.-Y., and Hong, Y.-Y. (1995). Implicit theories and their role in judgments and reactions: A word from two perspectives. Psychol. Inq. 6, 267–285. doi: 10.1207/s15327965pli0604_1
Dweck, C. S., and Leggett, E. L. (1988). A social-cognitive approach to motivation and personality. Psychol. Rev. 95, 256–273. doi: 10.1037/0033-295X.95.2.256
Earl, B., Viskupic, K., Marker, A., Moll, A., Roark, T., Landrum, R. E., et al. (2020). “Driving change: using the CACAO framework in an institutional change project,” in Transforming institutions: Accelerating systemic change in higher education. eds. K. White, A. Beach, N. Finkelstein, C. Henderson, S. Simkins, and L. Slakey, et al. (Pressbooks), 19–32.
Ebert-May, D., Derting, T. L., Henkel, T. P., Middlemis Maher, J., Momsen, J. L., Arnold, B., et al. (2015). Breaking the cycle: future faculty begin teaching with learner-centered strategies after professional development. CBE Life Sci. Educ. 14:ar22. doi: 10.1187/cbe.14-12-0222
Ebert-May, D., Derting, T. L., Hodder, J., Momsen, J. L., Long, T. M., and Jardeleza, S. E. (2011). What we say is not what we do: effective evaluation of faculty professional development programs. Bioscience 61, 550–558. doi: 10.1525/bio.2011.61.7.9
Edwards, A. L. (1957). Techniques of attitude scale construction. Englewood Cliffs, NJ: Prentice-Hall.
Ellis, J., Fosdick, B. K., and Rasmussen, C. (2016). Women 1.5 times more likely to leave STEM pipeline after calculus compared to men: lack of mathematical confidence a potential culprit. PLoS One 11:e0157447. doi: 10.1371/journal.pone.0157447
Elrod, S., and Kezar, A. (2016). Increasing student success in STEM: A guide to systemic institutional change. Washington, DC: Association of American Colleges & Universities.
Elrod, S., and Kezar, A. (2017). Increasing student success in STEM: summary of a guide to systemic institutional change. Change 49, 26–34. doi: 10.1080/00091383.2017.1357097
Emery, N., Maher, J. M., and Ebert-May, D. (2021). Environmental influences and individual characteristics that affect learner-centered teaching practices. PLoS One 16:e0250760. doi: 10.1371/journal.pone.0250760
Erdmann, R., Miller, K., and Stains, M. (2020). Exploring STEM postsecondary instructors’ accounts of instructional planning and revisions. Int. J. STEM Educ. 7:7. doi: 10.1186/s40594-020-00206-7
Fairweather, J. (2008). Linking evidence and promising practices in science, technology, engineering, and mathematics (STEM) undergraduate education.
Fairweather, J. S., and Rhoads, R. A. (1995). Teaching and the faculty role: enhancing the commitment to instruction in American colleges and universities. Educ. Eval. Policy Anal. 17, 179–194. doi: 10.3102/01623737017002179
Feldman, A. (2000). Decision making in the practical domain: A model of practical conceptual change. Sci. Educ. 84, 606–623. doi: 10.1002/1098-237X(200009)84:5<606::AID-SCE4>3.0.CO;2-R
Ferrare, J. J. (2019). A multi-institutional analysis of instructional beliefs and practices in gateway courses to the sciences. CBE Life Sci. Educ. 18:ar26. doi: 10.1187/cbe.17-12-0257
Foote, K., Knaub, A., Henderson, C., Dancy, M., and Beichner, R. J. (2016). Enabling and challenging factors in institutional reform: the case of SCALE-UP. Phys. Rev. Phys. Educ. Res. 12:010103. doi: 10.1103/PhysRevPhysEducRes.12.010103
Freeman, S., Eddy, S. L., McDonough, M., Smith, M. K., Okoroafor, N., Jordt, H., et al. (2014). Active learning increases student performance in science, engineering, and mathematics. Proc. Natl. Acad. Sci. U. S. A. 111, 8410–8415. doi: 10.1073/pnas.1319030111
Froyd, J. E., Henderson, C., Cole, R. S., Friedrichsen, D., Khatri, R., and Stanford, C. (2017). From dissemination to propagation: A new paradigm for education developers. Change 49, 35–42. doi: 10.1080/00091383.2017.1357098
Fuesting, M. A., Diekman, A. B., Boucher, K. L., Murphy, M. C., Manson, D. L., and Safer, B. L. (2019). Growing STEM: perceived faculty mindset as an indicator of communal affordances in STEM. J. Pers. Soc. Psychol. 117, 260–281. doi: 10.1037/pspa0000154
Fukawa-Connelly, T., Johnson, E., and Keller, R. (2016). Can math education research improve the teaching of abstract algebra? Not. Am. Math. Soc. 63, 276–281. doi: 10.1090/noti1339
Gess-Newsome, J., Southerland, S. A., Johnston, A., and Woodbury, S. (2003). Educational reform, personal practical theories, and dissatisfaction: the anatomy of change in college science teaching. Am. Educ. Res. J. 40, 731–767. doi: 10.3102/00028312040003731
Gibbons, R. E., Villafañe, S. M., Stains, M., Murphy, K. L., and Raker, J. R. (2018). Beliefs about learning and enacted instructional practices: an investigation in postsecondary chemistry education. J. Res. Sci. Teach. 55, 1111–1133. doi: 10.1002/tea.21444
Golnabi, A. H., Murray, E., and Su, H. (2021). How precalculus course coordination can impact students’ academic performance. J. High. Educ. Theory Pract. 21, 175–185. doi: 10.33423/jhetp.v21i5.4278
Goodenough, W. H. (1955). A technique for scale analysis. Educ. Psychol. Meas. 4, 179–190. doi: 10.1177/001316445500400301
Gormally, C., Evans, M., and Brickman, P. (2014). Feedback about teaching in higher ed: neglected opportunities to promote change. CBE Life Sci. Educ. 13, 187–199. doi: 10.1187/cbe.13-12-0235
Grubb, W. N. (2002). Honored but invisible: An inside look at teaching in community colleges. New York, NY: Routledge.
Guest, G. (2000). Using Guttman scaling to rank wealth: integrating quantitative and qualitative data. Field Methods 12, 346–357. doi: 10.1177/1525822X0001200406
Guttman, L. (1944). A basis for scaling qualitative data. Am. Sociol. Rev. 9, 139–150. doi: 10.2307/2086306
Haak, D. C., HilleRisLambers, J., Pitre, E., and Freeman, S. (2011). Increased structure and active learning reduce the achievement gap in introductory biology. Science 332, 1213–1216. doi: 10.1126/science.1204820
Handelsman, J., Ebert-May, D., Beichner, R., Bruns, P., Chang, A., DeHaan, R., et al. (2004). Scientific teaching. Science 304, 521–522. doi: 10.1126/science.1096022
Hativa, N. (1995). The department-wide approach to improving faculty instruction in higher education: A qualitative evaluation. Res. High. Educ. 36, 377–413. doi: 10.1007/BF02207904
Hattie, J., and Marsh, H. W. (1996). The relationship between research and teaching: A meta-analysis. Rev. Educ. Res. 66, 507–542. doi: 10.3102/00346543066004507
Henderson, C. (2008). Promoting instructional change in new faculty: an evaluation of the physics and astronomy new faculty workshop. Am. J. Phys. 76, 179–187. doi: 10.1119/1.2820393
Henderson, C., Beach, A., and Famiano, M. (2009). Promoting instructional change via co-teaching. Am. J. Phys. 77, 274–283. doi: 10.1119/1.3033744
Henderson, C., Beach, A., and Finkelstein, N. (2011). Facilitating change in undergraduate STEM instructional practices: an analytic review of the literature. J. Res. Sci. Teach. 48, 952–984. doi: 10.1002/tea.20439
Henderson, C., Connolly, M., Dolan, E. L., Finkelstein, N., Franklin, S., Malcom, S., et al. (2017). Towards the STEM DBER alliance: why we need a discipline-based STEM education research community. Int. J. STEM Educ. 4:14. doi: 10.1186/s40594-017-0076-1
Henderson, C., and Dancy, M. H. (2007). Barriers to the use of research-based instructional strategies: the influence of both individual and situational characteristics. Phys. Rev. Phys. Educ. Res. 3:020102. doi: 10.1103/PhysRevSTPER.3.020102
Henderson, C., and Dancy, M. H. (2009). Impact of physics education research on the teaching of introductory quantitative physics in the United States. Phys. Rev. Phys. Educ. Res. 5:020107. doi: 10.1103/PhysRevSTPER.5.020107
Henderson, C., Dancy, M., and Niewiadomska-Bugaj, M. (2012). Use of research-based instructional strategies in introductory physics: where do faculty leave the innovation-decision process? Phys. Rev. Phys. Educ. Res. 8:020104. doi: 10.1103/PhysRevSTPER.8.020104
Henderson, C., Turpen, C., Dancy, M., and Chapman, T. (2014). Assessment of teaching effectiveness: lack of alignment between instructors, institutions, and research recommendations. Phys. Rev. Phys. Educ. Res. 10:010106. doi: 10.1103/PhysRevSTPER.10.010106
Herman, G. L., Greene, J. C., Hahn, L. D., Mestre, J. P., Tomkin, J. H., and West, M. (2018). Changing the teaching culture in introductory STEM courses at a large research university. J. Coll. Sci. Teach. 47, 32–38.
Hora, M. T. (2012). Organizational factors and instructional decision-making: A cognitive perspective. Rev. High. Educ. 35, 207–235. doi: 10.1353/rhe.2012.0001
Hora, M. T., and Anderson, C. (2012). Perceived norms for interactive teaching and their relationship to instructional decision-making: A mixed methods study. High. Educ. 64, 573–592. doi: 10.1007/s10734-012-9513-8
Houseknecht, J. B., Bachinski, G. J., Miller, M. H., White, S. A., and Andrews, D. M. (2020). Effectiveness of the active learning in organic chemistry faculty development workshops. Chem. Educ. Res. Pract. 21, 387–398. doi: 10.1039/C9RP00137A
Indorf, J. L., Benabentos, R., Daubenmire, P., Murasko, D., Hazari, Z., Potvin, G., et al. (2021). Distinct factors predict use of active learning techniques by pre-tenure and tenured STEM faculty. J. Geosci. Educ. 69, 357–372. doi: 10.1080/10899995.2021.1927461
Johnson, E., Keller, R., and Fukawa-Connelly, T. (2018). Results from a survey of abstract algebra instructors across the United States: understanding the choice to (not) lecture. Int. J. Res. Undergrad. Math. Ed. 4, 254–285. doi: 10.1007/s40753-017-0058-1
Johnson, A. W., Su, M. P., Blackburn, M. W., and Finelli, C. J. (2021). Instructor use of a flexible classroom to facilitate active learning in undergraduate engineering courses. Eur. J. Eng. Educ. 46, 618–635. doi: 10.1080/03043797.2020.1865878
Kezar, A. (2011). What is the best way to achieve broader reach of improved practices in higher education? Innov. High. Educ. 36, 235–247. doi: 10.1007/s10755-011-9174-z
Knaub, A. V., Foote, K. T., Henderson, C., Dancy, M., and Beichner, R. J. (2016). Get a room: the role of classroom space in sustained implementation of studio style instruction. Int. J. STEM Educ. 3:8. doi: 10.1186/s40594-016-0042-3
Koch, A. K. (2017). It's about the gateway courses: defining and contextualizing the issue. New Dir. Higher Educ. 2017, 11–17. doi: 10.1002/he.20257
Kogan, M., and Laursen, S. L. (2014). Assessing long-term effects of inquiry-based learning: A case study from college mathematics. Innov. High. Educ. 39, 183–199. doi: 10.1007/s10755-013-9269-9
LaCrosse, J., Murphy, M. C., A, G. J., and Zirkel, S. (2021). The role of STEM professors’ mindset beliefs on students’ anticipated psychological experiences and course interest. J. Educ. Psychol. 113, 949–971. doi: 10.1037/edu0000620
Landis, C. R., Peace, G. E., Scharberg, M. A., Branz, S., Spencer, J. N., Ricci, R. W., et al. (1998). The new traditions consortium: shifting from a faculty-centered paradigm to a student-centered paradigm. J. Chem. Educ. 75:741. doi: 10.1021/ed075p741
Landrum, R. E., Viskupic, K., Shadle, S. E., and Bullock, D. (2017). Assessing the STEM landscape: the current instructional climate survey and the evidence-based instructional practices adoption scale. Int. J. STEM Educ. 4:25. doi: 10.1186/s40594-017-0092-1
Lane, A. K., McAlpin, J. D., Earl, B., Feola, S., Lewis, J. E., Mertens, K., et al. (2020). Innovative teaching knowledge stays with users. Proc. Natl. Acad. Sci. U. S. A. 117, 22665–22667. doi: 10.1073/pnas.2012372117
Lane, A. K., Skvoretz, J., Ziker, J. P., Couch, B. A., Earl, B., Lewis, J. E., et al. (2019). Investigating how faculty social networks and peer influence relate to knowledge and use of evidence-based teaching practices. Int. J. STEM Educ. 6:28. doi: 10.1186/s40594-019-0182-3
Limeri, L. B., Musgrove, M. M. C., Henry, M. A., and Schussler, E. E. (2020). Leveraging psychosocial interventions to motivate instructor participation in teaching professional development. CBE Life Sci. Educ 19:es10. doi: 10.1187/cbe.19-11-0236
Lindblom-Ylänne, S., Trigwell, K., Nevgi, A., and Ashwin, P. (2006). How approaches to teaching are affected by discipline and teaching context. Stud. High. Educ. 31, 285–298. doi: 10.1080/03075070600680539
Liu, X. (2015). Applied ordinal logistic regression using Stata: From single-level to multilevel modeling. Thousand Oaks, CA: Sage Publications.
Lorenzo, M., Crouch, C. H., and Mazur, E. (2006). Reducing the gender gap in the physics classroom. Am. J. Phys. 74, 118–122. doi: 10.1119/1.2162549
Lotter, C., Harwood, W. S., and Bonner, J. J. (2007). The influence of core teaching conceptions on teachers' use of inquiry teaching practices. J. Res. Sci. Teach. 44, 1318–1347. doi: 10.1002/tea.20191
Lou, N. M., and Noels, K. A. (2020). Does my teacher believe i can improve?: the role of meta-lay theories in ESL learners’ mindsets and need satisfaction. Front. Psychol. 11:1417. doi: 10.3389/fpsyg.2020.01417
Luft, J. A., Kurdziel, J. P., Roehrig, G. H., and Turner, J. (2004). Growing a garden without water: graduate teaching assistants in introductory science laboratories at a doctoral/research university. J. Res. Sci. Teach. 41, 211–233. doi: 10.1002/tea.20004
Lund, T. J., Pilarz, M., Velasco, J. B., Chakraverty, D., Rosploch, K., Undersander, M., et al. (2015). The best of both worlds: building on the COPUS and RTOP observation protocols to easily and reliably measure various levels of reformed instructional practice. CBE Life Sci. Educ. 14:ar18. doi: 10.1187/cbe.14-10-0168
Lund, T. J., and Stains, M. (2015). The importance of context: an exploration of factors influencing the adoption of student-centered teaching among chemistry, biology, and physics faculty. Int. J. STEM Educ. 2:13. doi: 10.1186/s40594-015-0026-8
Ma, S., Herman, G. L., Tomkin, J. H., Mestre, J. P., and West, M. (2018). Spreading teaching innovations in social networks: the bridging role of mentors. J. STEM Educ. Res. 1, 60–84. doi: 10.1007/s41979-018-0002-6
Ma, S., Herman, G. L., West, M., Tomkin, J., and Mestre, J. (2019). Studying STEM faculty communities of practice through social network analysis. J. High. Educ. 90, 773–799. doi: 10.1080/00221546.2018.1557100
Manduca, C. A., Iverson, E. R., Luxenberg, M., Macdonald, R. H., McConnell, D. A., Mogk, D. W., et al. (2017). Improving undergraduate STEM education: the efficacy of discipline-based professional development. Sci. Adv. 3:e1600193. doi: 10.1126/sciadv.1600193
Manduca, C. A., Mogk, D. W., Tewksbury, B., Macdonald, R. H., Fox, S. P., Iverson, E. R., et al. (2010). On the cutting edge: teaching help for geoscience faculty. Science 327, 1095–1096. doi: 10.1126/science.1183028
Marbach-Ad, G., Briken, V., Frauwirth, K., Gao, L.-Y., Hutcheson, S. W., Joseph, S. W., et al. (2007). A faculty team works to create content linkages among various courses to increase meaningful learning of targeted concepts of microbiology. CBE Life Sci. Educ. 6, 155–162. doi: 10.1187/cbe.06-12-0212
Marbach-Ad, G., Schaefer Ziemer, K., Orgler, M., and Thompson, K. V. (2014). Science teaching beliefs and reported approaches within a research university: perspectives from faculty, graduate students, and undergraduates. Int. J. Teach. Learn. High. Educ. 26, 232–250.
Marker, A., Pyke, P., Ritter, S., Viskupic, K., Moll, A., Landrum, R. E., et al. (2015). “Applying the CACAO change model to promote systemic transformation in STEM,” in Transforming institutions: Undergraduate STEM education for the 21st centruy. eds. G. C. Weaver, W. D. Burgess, A. L. Childress, and L. Slakey (West Lafayette, IN: Purdue University Press), 176–188.
Matz, R. L., Fata-Hartley, C. L., Posey, L. A., Laverty, J. T., Underwood, S. M., Carmel, J. H., et al. (2018). Evaluating the extent of a large-scale transformation in gateway science courses. Sci. Adv. 4:eaau0554. doi: 10.1126/sciadv.aau0554
McAlpin, J. D., Ziker, J. P., Skvoretz, J., Couch, B. A., Earl, B., Feola, S., et al. (2022). Development of the cooperative adoption factors instrument to measure factors associated with instructional practice in the context of institutional change. Int. J. STEM Educ. 9:48. doi: 10.1186/s40594-022-00364-w
McCourt, J. S., Andrews, T. C., Knight, J. K., Merrill, J. E., Nehm, R. H., Pelletreau, K. N., et al. (2017). What motivates biology instructors to engage and persist in teaching professional development?. CBE Life Sci. Educ. 16:ar54. doi: 10.1187/cbe.16-08-0241
McIver, J. P., and Carmines, E. G. (1981). “An introduction to Guttman scaling,” in Unidimensional scaling (Thousand Oaks, CA: SAGE Publications, Inc.), 41–66.
Menzel, H. (1953). A new coefficient for scalogram analysis. Public Opin. Q. 17, 268–280. doi: 10.1086/266460
Michael, J. (2007). Faculty perceptions about barriers to active learning. Coll. Teach. 55, 42–47. doi: 10.3200/CTCH.55.2.42-47
Miller, E., Fowler, J., Johns, C., Johnson, J., Ramsey, B., and Snapp, B. (2021). Increasing active learning in large, tightly coordinated calculus courses. Primus 31, 371–392. doi: 10.1080/10511970.2020.1772923
Miller, J. W., Martineau, L. P., and Clark, R. C. (2000). Technology infusion and higher education: changing teaching and learning. Innov. High. Educ. 24, 227–241. doi: 10.1023/B:IHIE.0000047412.64840.1c
Mingus, T. T. Y., and Koelling, M. (2021). A collaborative approach to coordinating calculus 1 to improve student outcomes. PRIMUS 31, 393–412. doi: 10.1080/10511970.2020.1772919
Muenks, K., Canning, E. A., LaCrosse, J., Green, D. J., Zirkel, S., Garcia, J. A., et al. (2020). Does my professor think my ability can change? Students’ perceptions of their STEM professors’ mindset beliefs predict their psychological vulnerability, engagement, and performance in class. J. Exp. Psychol. Gen. 149, 2119–2144. doi: 10.1037/xge0000763
Muenks, K., Yan, V. X., Woodward, N. R., and Frey, S. E. (2021). Elaborative learning practices are associated with perceived faculty growth mindset in undergraduate science classrooms. Learn. Individ. Differ. 92:102088. doi: 10.1016/j.lindif.2021.102088
Murray, T. A., Higgins, P., Minderhout, V., and Loertscher, J. (2011). Sustaining the development and implementation of student-centered teaching nationally: the importance of a community of practice. Biochem. Mol. Biol. Educ. 39, 405–411. doi: 10.1002/bmb.20537
National Research Council (NRC). (2012). Discipline-based education research: Understanding and improving learning in undergraduate science and engineering. Washington, DC: The National Academics Press.
Ogilvie, C. A. (2008). Swivel seating in large lecture theaters and its impact on student discussions and learning. J. Coll. Sci. Teach. 37, 50–56.
Oleson, A., and Hora, M. T. (2014). Teaching the way they were taught? Revisiting the sources of teaching knowledge and the role of prior experience in shaping faculty teaching practices. High. Educ. 68, 29–45. doi: 10.1007/s10734-013-9678-9
Owens, D. C., Sadler, T. D., Barlow, A. T., and Smith-Walters, C. (2020). Student motivation from and resistance to active learning rooted in essential science practices. Res. Sci. Educ. 50, 253–277. doi: 10.1007/s11165-017-9688-1
Peace, G. E., Lewis, E. L., Burke, K. A., and Greenbowe, T. J. (2002). The multi-initiative dissemination project: active-learning strategies for college chemistry. J. Chem. Educ. 79:699. doi: 10.1021/ed079p699
Pelletreau, K. N., Knight, J. K., Lemons, P. P., McCourt, J. S., Merrill, J. E., Nehm, R. H., et al. (2018). A faculty professional development model that improves student learning, encourages active-learning instructional practices, and works for faculty at multiple institutions. CBE Life Sci. Educ. 17:es5. doi: 10.1187/cbe.17-12-0260
Pilgrim, M. E., Apkarian, N., Milbourne, H., and O’Sullivan, M. (2021). From rough waters to calm seas: the challenges and successes of building a graduate teaching assistant professional development program. PRIMUS 31, 594–607. doi: 10.1080/10511970.2020.1793851
Pilgrim, M. E., McDonald, K. K., Offerdahl, E. G., Ryker, K., Shadle, S., Stone-Johnstone, A., et al. (2020). “An exploratory study of what different theories can tell us about change,” in Transforming institutions: Accelerating systemic change in higher education. eds. K. White, A. Beach, N. Finkelstein, C. Henderson, S. Simkins, and L. Slakey, et al. ( Pressbooks), 97–108.
Porter, S. R., and Umbach, P. D. (2001). Analyzing faculty workload data using multilevel modeling. Res. High. Educ. 42, 171–196. doi: 10.1023/A:1026573503271
President’s Council of Advisors on Science and Technology (PCAST). (2012). Engage to excel: Producing one million additional college graduates with degrees in science, technology, engineering, and mathematics. Washington, DC: Office of Science and Technology.
Price, J., and Cotten, S. R. (2006). Teaching, research, and service: expectations of assistant professors. Am. Sociol. 37, 5–21. doi: 10.1007/s12108-006-1011-y
Prosser, M., and Trigwell, K. (1997). Relations between perceptions of the teaching environment and approaches to teaching. Br. J. Educ. Psychol. 67, 25–35. doi: 10.1111/j.2044-8279.1997.tb01224.x
Quan, G. M., Corbo, J. C., Finkelstein, N. D., Pawlak, A., Falkenberg, K., Geanious, C., et al. (2019). Designing for institutional transformation: six principles for department-level interventions. Phys. Rev. Phys. Educ. Res. 15:010141. doi: 10.1103/PhysRevPhysEducRes.15.010141
Rahman, M. T., and Lewis, S. E. (2019). Evaluating the evidence base for evidence-based instructional practices in chemistry through meta-analysis. J. Res. Sci. Teach. 57, 765–793. doi: 10.1002/tea.21610
Raker, J. R., Dood, A. J., Srinivasan, S., and Murphy, K. L. (2021). Pedagogies of engagement use in postsecondary chemistry education in the United States: results from a national survey. Chem. Educ. Res. Pract. 22, 30–42. doi: 10.1039/D0RP00125B
Rasmussen, C., Apkarian, N., Hagman, J. E., Johnson, E., Larsen, S., and Bressoud, D. (2019). Brief report: characteristics of precalculus through calculus 2 programs: insights from a national census survey. J. Res. Math. Educ. 50, 98–111. doi: 10.5951/jresematheduc.50.1.0098
Rasmussen, C., and Ellis, J. (2015). “Calculus coordination at PhD-granting universities: more than just using the same syllabus, textbook, and final exam,” in Insights and recommendations from the MAA national study of college calculus. eds. D. Bressoud, V. Mesa, and C. Rasmussen (Washington, DC: MMA Press), 111–120.
Rasmussen, C., Ellis, J., Zazkis, D., and Bressoud, D. (2014). “Features of successful calculus programs at five doctoral degree granting institutions,” In: Proceedings of the 38th conference of the International Group for the Psychology of mathematics education and the 36th conference of the north American chapter of the psychology of mathematics education. Vancouver, Canada.
Rattan, A., Good, C., and Dweck, C. S. (2012). It's ok — not everyone can be good at math: instructors with an entity theory comfort (and demotivate) students. J. Exp. Soc. Psychol. 48, 731–737. doi: 10.1016/j.jesp.2011.12.012
Rattan, A., Savani, K., Komarraju, M., Morrison, M. M., Boggs, C., and Ambady, N. (2018). Meta-lay theories of scientific potential drive underrepresented students’ sense of belonging to science, technology, engineering, and mathematics (STEM). J. Pers. Soc. Psychol. 115, 54–75. doi: 10.1037/pspi0000130
Raudenbush, S. W., and Bryk, A. S. (2002). Hierarchical linear models: Applications and data analysis methods. Thousand Oaks, CA: Sage.
Reinholz, D. L., and Apkarian, N. (2018). Four frames for systemic change in STEM departments. Int. J. STEM Educ. 5:3. doi: 10.1186/s40594-018-0103-x
Reinholz, D. L., Matz, R. L., Cole, R., and Apkarian, N. (2019). STEM is not a monolith: A preliminary analysis of variations in STEM disciplinary cultures and implications for change. CBE Life Sci. Educ. 18:mr4. doi: 10.1187/cbe.19-02-0038
Richardson, D. S., Bledsoe, R. S., and Cortez, Z. (2020). Mindset, motivation, and teaching practice: psychology applied to understanding teaching and learning in STEM disciplines. CBE Life Sci. Educ 19:ar46. doi: 10.1187/cbe.19-11-0238
Riedl, A., Yeung, F., and Burke, T. (2021). Implementation of a flipped active-learning approach in a community college general biology course improves student performance in subsequent biology courses and increases graduation rate. CBE Life Sci. Educ. 20:ar30. doi: 10.1187/cbe.20-07-0156
Riihimaki, C. A., and Viskupic, K. (2020). Motivators and inhibitors to change: why and how geoscience faculty modify their course content and teaching methods. J. Geosci. Educ. 68, 115–132. doi: 10.1080/10899995.2019.1628590
Robert, J., Lewis, S. E., Oueini, R., and Mapugay, A. (2016). Coordinated implementation and evaluation of flipped classes and peer-led team learning in general chemistry. J. Chem. Educ. 93, 1993–1998. doi: 10.1021/acs.jchemed.6b00395
Roberts, J. A., Olcott, A. N., McLean, N. M., Baker, G. S., and Möller, A. (2018). Demonstrating the impact of classroom transformation on the inequality in DFW rates (“D” or “F” grade or withdraw) for first-time freshmen, females, and underrepresented minorities through a decadal study of introductory geology courses. J. Geosci. Educ. 66, 304–318. doi: 10.1080/10899995.2018.1510235
Ruiz-Primo, M. A., Briggs, D., Iverson, H., Talbot, R., and Shepard, L. A. (2011). Impact of undergraduate science course innovations on learning. Science 331, 1269–1270. doi: 10.1126/science.1198976
Salomone, S., Dillon, H., Prestholdt, T., Peterson, V., James, C., and Anctil, E. (2020). “Making teaching matter more: reflect at the University of Portland,” in Transforming institutions: Accelerating systemic change in higher education. eds. K. White, A. Beach, N. Finkelstein, C. Henderson, S. Simkins, and L. Slakey, et al. (Pressbooks), 249–261.
Seymour, E., DeWelde, K., and Fry, C. (2011). Determining progress in improving undergraduate STEM education: the reformers’ tale. A White paper commissioned for the forum, “characterizing the impact and diffusion of engineering education innovations”. Available at: https://www.nae.edu/File.aspx?id=36664
Seymour, E., and Hewitt, N. M. (1997). Talking about leaving: Why undergraduates leave the sciences. Boulder, CO: Westview Press.
Seymour, E., and Hunter, A.-B. (2019). Talking about leaving revisited: Persistence, relocation, and loss in undergraduate STEM education. Cham, Switzerland: Springer International Publishing.
Shadle, S. E., Liu, Y., Lewis, J. E., and Minderhout, V. (2018). Building a community of transformation and a social network analysis of the POGIL project. Innov. High. Educ. 43, 475–490. doi: 10.1007/s10755-018-9444-0
Shadle, S. E., Marker, A., and Earl, B. (2017). Faculty drivers and barriers: laying the groundwork for undergraduate STEM education reform in academic departments. Int. J. STEM Educ. 4:8. doi: 10.1186/s40594-017-0062-7
Singer, E. R. (1996). Espoused teaching paradigms of college faculty. Res. High. Educ. 37, 659–679. doi: 10.1007/BF01792951
Smart, J. C., and Umbach, P. D. (2007). Faculty and academic environments: using holland's theory to explore differences in how faculty structure undergraduate courses. J. Coll. Stud. Dev. 48, 183–195. doi: 10.1353/csd.2007.0021
Smith, M. K., Vinson, E. L., Smith, J. A., Lewin, J. D., and Stetzer, M. R. (2014). A campus-wide study of STEM courses: new perspectives on teaching practices and perceptions. CBE Life Sci. Educ. 13, 624–635. doi: 10.1187/cbe.14-06-0108
Snijders, T. A. B., and Bosker, R. J. (2012). Multilevel analysis: An introduction to basic and advanced multilevel modeling. Thousand Oaks, CA: Sage Publishing.
Soto, R. C., and Marzocchi, A. S. (2021). Learning about active learning while actively learning: insights from faculty professional development. PRIMUS 31, 269–280. doi: 10.1080/10511970.2020.1746449
Southerland, S. A., Sowell, S., Blanchard, M., and Granger, E. M. (2011a). Exploring the construct of pedagogical discontentment: A tool to understand science teachers’ openness to reform. Res. Sci. Educ. 41, 299–317. doi: 10.1007/s11165-010-9166-5
Southerland, S. A., Sowell, S., and Enderle, P. (2011b). Science teachers’ pedagogical discontentment: its sources and potential for change. J. Sci. Teach. Educ. 22, 437–457. doi: 10.1007/s10972-011-9242-3
Speer, N. M., and Wagner, J. F. (2009). Knowledge needed by a teacher to provide analytic scaffolding during undergraduate mathematics classroom discussions. J. Res. Math. Educ. 40, 530–562. doi: 10.5951/jresematheduc.40.5.0530
Springer, L., Stanne, M. E., and Donovan, S. S. (1999). Effects of small-group learning on undergraduates in science, mathematics, engineering, and technology: A meta-analysis. Rev. Educ. Res. 69, 21–51. doi: 10.3102/00346543069001021
Srinivasan, S., Gibbons, R. E., Murphy, K. L., and Raker, J. (2018). Flipped classroom use in chemistry education: results from a survey of postsecondary faculty members. Chem. Educ. Res. Pract. 19, 1307–1318. doi: 10.1039/C8RP00094H
Stains, M., Harshman, J., Barker, M. K., Chasteen, S. V., Cole, R., DeChenne-Peters, S. E., et al. (2018). Anatomy of STEM teaching in North American universities. Science 359, 1468–1470. doi: 10.1126/science.aap8892
Stains, M., Pilarz, M., and Chakraverty, D. (2015). Short and long-term impacts of the Cottrell scholars collaborative new faculty workshop. J. Chem. Educ. 92, 1466–1476. doi: 10.1021/acs.jchemed.5b00324
Stains, M., and Vickrey, T. (2017). Fidelity of implementation: an overlooked yet critical construct to establish effectiveness of evidence-based instructional practices. CBE Life Sci. Educ. 16:rm1. doi: 10.1187/cbe.16-03-0113
Stanich, C. A., Pelch, M. A., Theobald, E. J., and Freeman, S. (2018). A new approach to supplementary instruction narrows achievement and affect gaps for underrepresented minorities, first-generation students, and women. Chem. Educ. Res. Pract. 19, 846–866. doi: 10.1039/C8RP00044A
Stegall, S. L., Grushow, A., Whitnell, R., and Hunnicutt, S. S. (2016). Evaluating the effectiveness of POGIL-PCL workshops. Chem. Educ. Res. Pract. 17, 407–416. doi: 10.1039/C5RP00225G
Steinert, Y., Mann, K., Centeno, A., Dolmans, D., Spencer, J., Gelula, M., et al. (2006). A systematic review of faculty development initiatives designed to improve teaching effectiveness in medical education: BEME guide no. 8. Med. Teach. 28, 497–526. doi: 10.1080/01421590600902976
Sturtevant, H., and Wheeler, L. (2019). The STEM faculty instructional barriers and identity survey (FIBIS): development and exploratory results. Int. J. STEM Educ. 6:35. doi: 10.1186/s40594-019-0185-0
Sudweeks, R. R. (2018). “Guttman scaling” in The SAGE encyclopedia of educational research, measurement, and evaluation. ed. B. B. Frey (Thousand Oaks, CA: SAGE Publications, Inc), 764–766.
Synder, J. J., Sloane, J. D., Dunk, R. D. P., and Wiles, J. R. (2016). Peer-led team learning helps minority students succeed. PLoS Biol. 14:e1002398. doi: 10.1371/journal.pbio.1002398
Talbert, R., and Mor-Avi, A. (2019). A space for learning: an analysis of research on active learning spaces. Heliyon 5:e02967. doi: 10.1016/j.heliyon.2019.e02967
Tharayil, S., Borrego, M., Prince, M., Nguyen, K. A., Shekhar, P., Finelli, C. J., et al. (2018). Strategies to mitigate student resistance to active learning. Int. J. STEM Educ. 5:7. doi: 10.1186/s40594-018-0102-y
Theobald, E. J., Hill, M. J., Tran, E., Agrawal, S., Arroyo, E. N., Behling, S., et al. (2020). Active learning narrows achievement gaps for underrepresented students in undergraduate science, technology, engineering, and math. Proc. Natl. Acad. Sci. U. S. A. 117, 6476–6483. doi: 10.1073/pnas.1916903117
Tomkin, J. H., Beilstein, S. O., Morphew, J. W., and Herman, G. L. (2019). Evidence that communities of practice are associated with active learning in large STEM lectures. Int. J. Stem Educ. 6:1. doi: 10.1186/s40594-018-0154-z
Villalobos, C., Kim, H. W., Huber, T. J., Knobel, R., Setayesh, S., Sasidharan, L., et al. (2021). Coordinating STEM core courses for student success. PRIMUS 31, 316–329. doi: 10.1080/10511970.2020.1793855
Viskupic, K., Earl, B., and Shadle, S. E. (2022). Adapting the CACAO model to support higher education STEM teaching reform. Int. J. STEM Educ. 9:6. doi: 10.1186/s40594-021-00325-9
Viskupic, K., Ryker, K., Teasdale, R., Manduca, C., Iverson, E., Farthing, D., et al. (2019). Classroom observations indicate the positive impacts of discipline-based professional development. J. STEM Educ. Res. 2, 201–228. doi: 10.1007/s41979-019-00015-w
Walczyk, J. J., Ramsey, L. L., and Zha, P. (2007). Obstacles to instructional innovation according to college science and mathematics faculty. J. Res. Sci. Teach. 44, 85–106. doi: 10.1002/tea.20119
Walter, E. M., Beach, A. L., Henderson, C., Williams, C. T., and Ceballos-Madrigal, I. (2021). Understanding conditions for teaching innovation in postsecondary education: development and validation of the survey of climate for instructional improvement (SCII). Int. J. Technol. Educ. 4, 166–199. doi: 10.46328/ijte.46
Walter, E. M., Henderson, C. R., Beach, A. L., and Williams, C. T. (2016). Introducing the postsecondary instructional practices survey (PIPS): A concise, interdisciplinary, and easy-to-score survey. CBE Life Sci. Educ. 15:ar53. doi: 10.1187/cbe.15-09-0193
Wang, X., Sun, N., Lee, S. Y., and Wagner, B. (2017). Does active learning contribute to transfer intent among 2-year college students beginning in STEM? J. High. Educ. 88, 593–618. doi: 10.1080/00221546.2016.1272090
Wieman, C. (2015). A better way to evaluate undergraduate teaching. Change 47, 6–15. doi: 10.1080/00091383.2015.996077
Windschitl, M., and Sahl, K. (2002). Tracing teachers’ use of technology in a laptop computer school: the interplay of teacher beliefs, social dynamics, and institutional culture. Am. Educ. Res. J. 39, 165–205. doi: 10.3102/00028312039001165
Winter, D., Lemons, P., Bookman, J., and Hoese, W. (2012). Novice instructors and student-centered instruction: identifying and addressing obstacles to learning in the college science. J. Scholarsh. Teach. Learn. 2, 14–42.
Wood, W., and Gentile, J. (2003). Meeting report: the first national academies summer institute for undergraduate education in biology. Cell Biol. Educ. 2, 207–209. doi: 10.1187/cbe.03-10-0042
Woodbury, S., and Gess-Newsome, J. (2002). Overcoming the paradox of change without difference: A model of change in the arena of fundamental school reform. Educ. Policy 16, 763–782. doi: 10.1177/089590402237312
Yarnall, L., Toyama, Y., Gong, B., Ayers, C., and Ostrander, J. (2007). Adapting scenario-based curriculum materials to community college technical courses. Community Coll. J. Res. Pract. 31, 583–601. doi: 10.1080/10668920701428881
Yeager, D. S., Hanselman, P., Walton, G. M., Murray, J. S., Crosnoe, R., Muller, C., et al. (2019). A national experiment reveals where a growth mindset improves achievement. Nature 573, 364–369. doi: 10.1038/s41586-019-1466-y
Yeager, D. S., Romero, C., Paunesku, D., Hulleman, C. S., Schneider, B., Hinojosa, C., et al. (2016). Using design thinking to improve psychological interventions: the case of the growth mindset during the transition to high school. J. Educ. Psychol. 108, 374–391. doi: 10.1037/edu0000098
Yik, B. J., Raker, J. R., Apkarian, N., Stains, M., Henderson, C., Dancy, M. H., et al. (2022). Evaluating the impact of malleable factors on percent time lecturing in gateway chemistry, mathematics, and physics courses. Int. J. STEM Educ. 9:15. doi: 10.1186/s40594-022-00333-3
Keywords: research-based instructional strategies, evidence-based instructional practices, institutional change, active learning, contextual factors, personal factors, beliefs about teaching
Citation: Yik BJ, Raker JR, Apkarian N, Stains M, Henderson C, Dancy MH and Johnson E (2022) Association of malleable factors with adoption of research-based instructional strategies in introductory chemistry, mathematics, and physics. Front. Educ. 7:1016415. doi: 10.3389/feduc.2022.1016415
Received: 10 August 2022; Accepted: 24 October 2022;
Published: 21 November 2022.
Edited by:
Muhammet Usak, Kazan Federal University, RussiaReviewed by:
Chia-Lin Tsai, University of Northern Colorado, United StatesCopyright © 2022 Yik, Raker, Apkarian, Stains, Henderson, Dancy and Johnson. This is an open-access article distributed under the terms of the Creative Commons Attribution License (CC BY). The use, distribution or reproduction in other forums is permitted, provided the original author(s) and the copyright owner(s) are credited and that the original publication in this journal is cited, in accordance with accepted academic practice. No use, distribution or reproduction is permitted which does not comply with these terms.
*Correspondence: Jeffrey R. Raker, anJha2VyQHVzZi5lZHU=
Disclaimer: All claims expressed in this article are solely those of the authors and do not necessarily represent those of their affiliated organizations, or those of the publisher, the editors and the reviewers. Any product that may be evaluated in this article or claim that may be made by its manufacturer is not guaranteed or endorsed by the publisher.
Research integrity at Frontiers
Learn more about the work of our research integrity team to safeguard the quality of each article we publish.