- 1Institute for Secondary Education, University College of Teacher Education Vorarlberg, Feldkirch, Austria
- 2Department of Education Studies, University of Warwick, Coventry, United Kingdom
- 3Linz School of Education, Johannes Kepler University, Linz, Austria
Introduction: Across the globe, many national, state, and district level governments are increasingly seeking to bring about school “self improvement” via the fostering of change, which, at best, is based on or informed by research, evidence, and data. According to the conceptualization of research-informed education as inquiry cycle, it is reasoned that there is value in combining the approaches of data-based decision-making and evidence-informed education. The originality of this paper lies in challenging common claims that teachers’ engagement with research supports development processes at schools and pupil performance.
Methods: To put this assumption to test, a data-set based on 1,457 staff members from 73 English primary schools (school year 2014/2015) was (re-)analyzed in this paper. Not only survey information about trust among colleagues, organizational learning and the research use climate was used (cf. Brown et al., 2016), but also the results from the most recent school inspections and the results from standardized assessment at the end of primary school. Of particular interest was, as to whether the perceived research use climate mediates the association between organizational learning and trust at school on the one hand and the average pupil performance on the other, and whether schools that were rated as “outstanding,” “good,” or “requires improvement” in their most recent school inspection differ in that regard. Data was analyzed based on multi-level structural equation modelling.
Results: Our findings indicate that schools with a higher average value of trust among colleagues report more organizational and research informed activities, but also demonstrate better results in the average pupil performance assessment at the end of the school year. This was particularly true for schools rated as “good” in previous school inspections. In contrast, both “outstanding” schools and schools that “require improvement” appeared to engage more with research evidence, even though the former seemed not to profit from it.
Discussion: The conclusion is drawn that a comprehensive model of research-informed education can contribute to more conceptual clarity in future research, and based on that, to theoretical development.
Introduction
Across the globe, many national, state, and district level governments are increasingly seeking to bring about school “self improvement,” via the fostering of so-called “bottom-up” change (Brown et al., 2017; Brown, 2020; Malin et al., 2020): change undertaken by school staff to address their needs and which, when optimal, is based on, or informed by research, evidence, and data (Brown and Malin, 2022). The focus of this paper is on the English education system: one that previously has been characterized as hierachist (Coldwell, 2022), where rather autonomous local authorities, schools and professionals are seeking to “self-improve” while simultaneously situated within a system of strong central regulation and marketisation of state schooling which serves to influence behaviour (Helgøy et al., 2007). For example, the results in regularly administered standardized national tests or school inspection ratings are publicly accessible, and can be used by parents for their choice of school which, in turn affecting levels of school funding (cf., Coldwell, 2022).
Against this backdrop, this paper aims at investigating the link between the use of data feedback (e.g., school inspection results), the research-use climate at schools, which is framed by organizational conditions like trust among colleagues, or organizational learning in general. This is in turn expected to promote pupil performance in standardized assessments. In the following, research-informed education is introduced as umbrella term for the conceptual link between data-based decision-making and evidence-informed educational practice. As the data set analyzed here is based on a sample of educators at several English primary schools, the specific contextual conditions of the English education system are then introduced. This is followed by an overview of the state of research about the use of research by educational practitioners and schools, and of the specific effects of school inspections. Based on this, the research questions are specified, the methods are explained, and, in conclusion, the results are discussed with reference to the theoretical background of this paper.
Theoretical framework: Research-informed educational practice at English primary schools
As noted above, school “self improvement” is ideally achieved through teachers’ engagement with research, evidence, and data (Brown and Malin, 2022). Of course, how this engagement occurs, is as important as that it actually occurs in the first place. This is described in conceptual models of data-based decision-making and evidence-informed education. Even though the two approaches differ in their focus, they share the same assumptions about the sequence of phases nonetheless. The sequence is comparable to the steps of a research process (e.g., Teddlie and Tashakkori, 2006), but also to conceptions of learning as discovery or inquiry process (e.g., Bruner, 1961).
Conceptual models of data-based decision-making and evidence-informed education
Many commentators suggest that an “ideal sequence” of teachers’ and schools’ engagement with research, evidence or data is that described in conceptual frameworks, which model the process of evidence and data use as a complex, cognitive, knowledge-based problem-solving or inquiry cycle with consecutive phases, that are not ensued in a linear, but rather iterative fashion (Mark and Henry, 2004; Mandinach et al., 2008; Schildkamp and Kuiper, 2010; Coburn and Turner, 2011; Marsh, 2012; Schildkamp and Poortman, 2015; Schratz et al., 2018; Groß Ophoff and Cramer, 2022). This is outlined in Figure 1: In the course of the (1) conceptual phase, a question or problem is identified or a specific goal is set. Subsequently, data is collected and analyzed as part of the empirical phase (2). One point worthy of note here is, that only by way of systematic organization, processing, and analysis under consideration of theories, methods and context knowledge, can evidence evolve from (raw) data (Bromme et al., 2014; Groß Ophoff and Cramer, 2022). During the inferential phase (3), the available evidence allows focussing attention, provides new insights, challenges beliefs or reframes thinking, without immediate effect on decision-making. This was identified as conceptual research use by Weiss (1998). But evidence can also be used to identify or develop concrete measures to be taken (instrumental use), or even as justification or support of existing positions or established procedures (symbolic use). Based on the inferences drawn, possible change measures are identified, put to practice (4) and at best evaluated (5), too.
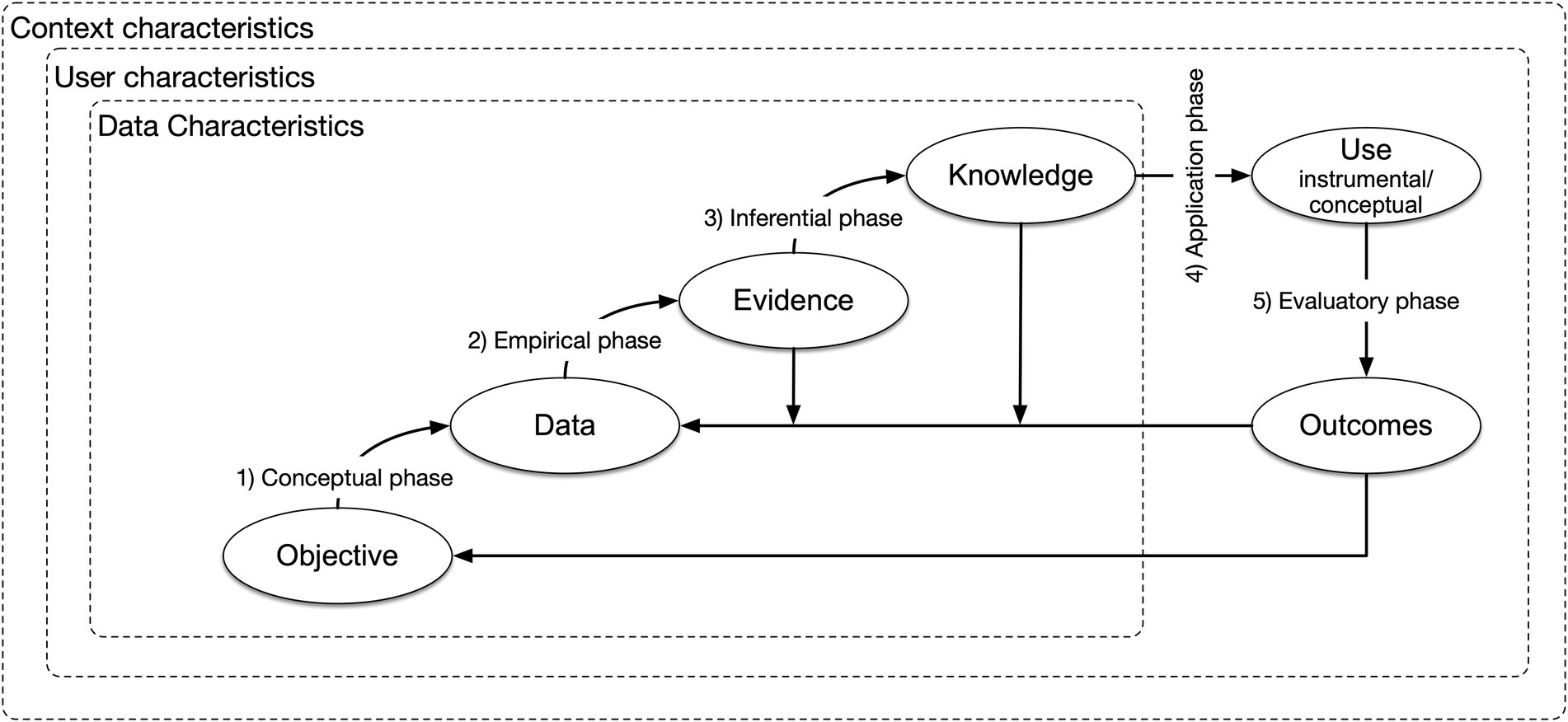
Figure 1. Conceptual framework of research informed-educational practice (adapted, a.o. Mark and Henry, 2004; Mandinach et al., 2008; Marsh, 2012; Schildkamp and Poortman, 2015; Schratz et al., 2018; Groß Ophoff and Cramer, 2022).
This inquiry cycle can be addressed on different levels of the educational system, that is, with regard to the characteristics of data or evidence (inner layer in Figure 1; e.g., graph types, cf. Merk et al., in press), of the users (middle layer in Figure 1; e.g., perceived usefulness, cf. Prenger and Schildkamp, 2018), or of the context (outer layer in Figure 1, e.g., trust among colleagues, cf. Brown et al., 2022). The current study mainly focusses on the latter, namely the schools’ culture of trust and organizational learning that can support (or hinder), how open to research schools are perceived by its staff.
Furthermore, the research process phases apply to both an engagement in research and an engagement with research (Borg, 2010). The former corresponds with being able to independently pass through the full research process, which requires advanced research-methodological competencies (Brown et al., 2017; Voss et al., 2020). The latter refers to the reflection on evidence for professionalization or development purposes, without the necessity to gather forms of evidence, such as research. The engagement with research is conceptually related to notions of data use and data-based decision-making, where approaches in this field increasingly take different kinds of data (e.g., from surveys, observations or conversations) into consideration that can be used for educational decisions and development processes (cf. Schildkamp et al., 2013; Mandinach and Gummer, 2016; Mandinach and Schildkamp, 2021; Groß Ophoff and Cramer, 2022). Accordingly, data available to schools can be further subdivided into internal school data (e.g., student feedback, collegial observations) and external sources (e.g., school inspection, regular mandatory pupil performance assessment, central exams), from more “generic” scientific research evidence (academic and professional literature, cf. Demski, 2017). Wiesner and Schreiner (2019) conceptualize the term “evidence” as a continuum that spans from evidence in the wider sense (e.g., school-internal data) to evidence in the stricter, that is, more scientific sense. The latter is derived as part of an engagement in research and serves as the foundation of theory development (Kiemer and Kollar, 2021; Renkl, 2022), but is usually viewed as rather abstract, impractical information by teachers (Harper et al., 2003; Hammersley, 2004; Zeuch et al., 2017). Less conclusive is the characterization of so-called referential data, e.g., from centrally purported school inspections or pupil performance assessments, as it can be characterized both as a) data and as b) evidence according to the above introduced problem-solving cycle (Groß Ophoff and Cramer, 2022). This ambiguity is used in the following to highlight two different lines of research in this field:
On the one hand (a), data feedback from school inspections or performance assessment might be perceived as “raw” data because it still requires sense-making (2: empirical phase) to being able to develop and implement of concrete instructional or school development measures. This perspective is typical for approaches in the field of data-based decision-making (Schildkamp and Kuiper, 2010; Mandinach, 2012; Schildkamp, 2019). Accordingly, Mandinach and Schildkamp (2021) describe the underlying conceptual models of data use as “theories of action” that conceptualize informed educational decision-making as a process of collecting and analysing different forms of data.
On the other hand (b), referential data shows features of research evidence in the stricter sense, as data collection, analysis, and result processing are based on research-methodological and conceptual scientific knowledge. Therefore, data feedback itself can provide teachers and school with scientific knowledge that might be useful in supporting or even supplementing processes during the inferential phase (3), and even might indicate that there is a need for more knowledge (in the sense of informal learning, e.g., Evers et al., 2016; Mandinach and Gummer, 2016; cf. Brown et al., 2017). So departing from (a), the (3) inferential phase of the problem-solving cycle is more paramount in this case – even though research evidence can be, of course, useful throughout the whole process (Huguet et al., 2014). Whether the need for more information or a deeper understanding is recognized at all, and which information sources are chosen is meaningful for the depth, scope and direction of subsequent use processes (Brown and Rogers, 2015; Vanlommel et al., 2017; Dunn et al., 2019; Kiemer and Kollar, 2021). Such an engagement with research evidence requires some basic understanding of the underlying scientific concepts or, if necessary, the willingness to acquire the germane knowledge (Rickinson et al., 2020). However, conceptual models of data-based decision-making stay rather vague in that regard: For example Schildkamp et al. (2018), describe as late as for the application phase (4) of the Data Team Procedure (a school intervention based on the approach of data-based decision-making), that in order to gather ideas about possible measures, different sources can be used like “the knowledge of team members and colleagues in the school, networks such as the teacher’s union, practitioner journals, scientific literature, the internet and the experiences of other schools” (p. 38). In contrast, approaches in the field of evidence-informed teacher education (with focus on pre-service teachers, e.g., Kiemer and Kollar, 2021; Greisel et al., 2022) or research-informed educational practice (with focus on in-service teachers, e.g., Cain, 2015; Brown et al., 2016, 2017) concentrate on the process of an engagement with evidence (in the sense of acquiring scientific knowledge) and can therefore be described as taking in the perspective of a “theory of learning.” Accordingly, Kiemer and Kollar (2021) identify as grounds for evidence-informed education, that in case certain “educational problems come up repeatedly, […] teachers should be able to seek out, obtain and potentially apply what scientific research on teaching and learning has to offer to pave the way for competent action (p. 128), which is why it is “a core task for preservice teacher education to equip future teachers with the skills and abilities necessary to engage in competent, evidence informed teaching” (p. 129). With focus on practicing teachers, but still in the same line of reasoning, Brown et al. (2017) describe the process of research-informed educational practice as the use of “existing research evidence for designing and implementing actions to achieve change” (p. 158).
Despite the different foci of these approaches to data, evidence, or research use in education, Brown et al. (2017) point out that there is value in a comprehensive approach “to educational decision-making that critically appraises different forms of evidence before key improvement decisions are made” (Brown et al., 2017, p. 154) by combining “the best of two worlds” (Brown et al., 2017). Take this example: A certain school is rated “requires improvement” during school inspection particularly in the key judgment category quality of education (a.o. based on lesson observations, cf. Office for Standards in Education, 2022). According to the inspection report, progress in mathematics is inconsistent because there are missed opportunities for pupils to extend their mathematical knowledge and skills in other subjects. Based on the available recommendations, the school staff aims at providing more challenging and motivating learning activities in mathematics lessons (= data-based decision making). This is why the teachers involved search deliberately for relevant evidence (here for example: cognitive activation in mathematics, e.g., Neubrand et al., 2013), appraise and discuss the available information with their colleagues, but might also obtain advice by a school-based coordinator. The transition between the empirical (2) and the inferential phase (3) (= research-informed educational practice) is probably particularly sensitive to organizational conditions: If organizational learning is a matter of course and staff members trust each other, improvement measures are more easily implemented than in a school climate that is distrustful and adverse to change. Provided that supportive contextual conditions are given, staff at the exemplary school might not only draw the conclusion that there is a need to revise, extend or swap learning materials, but actually decide to put these changes into practice as conclusion of the (3) inferential phase. After the implementation of measures considered suitable (4, application phase), the school staff is interested in evaluating the impact of the concrete change measures (5), for example, based on the pupils’ performance in central assessment tests and exams or their learning progress in lessons. Another possibility here is to investigate, whether the collaboration between staff has considerably improved. If the results are not satisfactory or new questions emerge, this in turn can represent the starting point for another cycle of inquiry.
So as the meaning “research” envelopes the full inquiry cycle, and both data and evidence are part of that process (Groß Ophoff and Cramer, 2022), we propose to use the umbrella term “research-informed education,” where data-based decision-making (a) and evidence-informed teacher education/educational practice (b) are conceptualized as part of the same comprehensive process. To put this theoretical assumption to test, a data-set based on 1,457 staff members from 73 English primary schools (2014/2015) is (re-)analyzed in this paper. For this sample, not only survey information about trust among colleagues, organizational learning and the research use climate was available (originally used in Brown et al., 2016), but also the results from the most recent school inspections (before the school staff survey) and the results from standardized assessment at the end of primary school (after the survey). As such, the English educational system and its highly regulated, “hierachist” accountability regime (Coldwell, 2022) is explained in more detail below. It serves as the contextual framework of this study (see outer layer of conceptual model, Figure 1), where schools and teachers operate under so-called high-stakes conditions – even though rather the performance of pupils than organizational (learning) processes are affected by such conditions (e.g., Lorenz et al., 2016).
Characteristics of the English educational (accountability) system
The education system in England could, in modern times, be most accurately described as “self-improving” (Greany, 2017). Here accountability systems “combine quasi-market pressures – such as parental choice of school coupled with funding following the learner – with central regulation and control” (Greany and Earley, 2018). A key aspect of this system is the regular school inspections process undertaken by Ofsted (England’s school inspection agency). Ofsted inspections are highlighted by many school leaders as a key driver of their behaviour (Chapman, 2001; Greany, 2017). As a result of an inspection, for which there is typically less than 24 h’ notice, schools are placed into one of four hierarchical categories of grades. The top grade: “outstanding,” typically results in the school becoming more attractive for parents: thus more students apply, and more funding is directed toward the school. Conversely, schools with lower ratings like “requires improvement” find it more challenging to attract families and the funding attached to student applications. In addition, up until 2019, schools rated “outstanding” were exempt for immediate subsequent inspections, meaning that the pressures of accountability are subsequently considerably lessened (with the converse applying to those which require improvement). Given this, it is possible to suggest a theory of action for why inspections might drive school improvement, with school inspection serving the function of (i) gaining and reporting information about school’s educational quality, (ii) ensuring accountability with regard to educational standards, (iii) contributing to school development and improvement, and (iv) enforcing an adherence to educational standards and criteria (Landwehr, 2011; Hofer et al., 2020; Ali et al., 2021).
Another characteristic of the “rigorous system of quality control” (Baxter and Clarke, 2013, p. 714) in English education is the regular implementation of standardized assessment tests (SAT). For example, at the end of key stage 2 (KS2: sixth and final year in primary education) statutory external tests in English (reading, writing) and mathematics have to be carried out alongside regular teacher assessment (cf., Isaacs, 2010). The average student at the end of key stage 2 is expected to reach level 4. By way of context, in 2014, 78% of pupils, and in 2015, 80 percent achieved level 4 or above in reading, writing and mathematics combined (Department for Education, 2015). In a recent white paper, the UK Government (2022) announced the ambition (as one of the “levelling up missions”) for 90 percent of KS2 pupils to reach the expected standards by 2030. Overall, a number of different variables, student outcomes (e.g., KS2 SAT results), and inspection ratings are publicly available as government produced annual “league tables” of schools under https://www.compare-school-performance.service.gov.uk/, which enables schools to be ranked according to a number of different variables and student outcomes. As a result, it is acknowledged that England’s accountability framework both focuses the minds of – and places pressure – on educators to focus on very specific forms of school improvement. More specifically such improvement tends, in the main, on ensuring pupils achieve well in progress tests in key subject areas (e.g., English literacy and mathematics, Ehren, 2018).
Thus, at the centre of this contribution is the research-informed educational practice (RIEP) of a sample of primary schools, which is framed by two vital aspects of accountability. In order to being able to hypothesize possible effects, the next chapter gives an overview of the state of research on verifiable effects of research-informed education on teachers, schools and pupil learning.
Research use and school improvement – Is there a link?
Connected with the concept of research-informed education is the expectation that up-to-date (= evidence-informed), hence professional teachers are able to engage with the multitude of data available to them and maybe identify the results as occasion for (more) professional development or instructional and school development. This in turn is expected to improve the quality of teaching, and mediated by that support pupil performance (e.g., Davies, 1999; Slavin, 2002). In other words, research-informed education is about “making the study and improvement of teaching more systematic and “less happen-stance” and relying on evidence to solve local problems of practice” (Ermeling, 2010, p. 378). So, the question is whether this claim is valid. Below an overview of the current state of research is given.
Effects on schools and teachers
On school and teacher level (Outcomes, see Figure 1), the benefits thought to accrue from RIEP include improvements in pedagogic knowledge and skills, greater job satisfaction and greater teacher retention, and evidently support a changed perspective on problems, greater teacher confidence or self-efficacy and improved critical faculties, as well as the ability to make autonomous professional decisions (e.g., Lankshear and Knobel, 2004; Boelhauve, 2005; Bell et al., 2010; Mincu, 2014; Godfrey, 2016; König and Pflanzl, 2016). But instead of using data or evidence, research findings indicate that school leaders and practitioners reportedly prefer to rely on intuition during data-based decision-making (Vanlommel et al., 2017), which is prone to confirmation bias and mistakes (Fullan, 2007; Dunn et al., 2019). Moreover, even though teachers and school leaders report to read professional literature regularly (VanLeirsburg and Johns, 1994; Lankshear and Knobel, 2004; Broemmel et al., 2019), there appears to be a strong preference for practical or guidance journals with no or only limited evidence orientation (Hetmanek et al., 2015; Rochnia and Gräsel, submitted), This inclination appears to be rather stable, as it is already observed in initial teacher education (Muñoz and Valenzuela, 2020; Kiemer and Kollar, 2021). Furthermore, Coldwell (2022) draws the conclusion that research use among English teachers is rather low; a result that is comparable to reports from educational systems all over the world (Malin et al., 2020). Regarding the effects of school inspections, “the evidence base […] is scattered” (Malin et al., 2020, p. 4), but has increased in recent years (a.o., de Wolf and Janssens, 2007; Gärtner and Pant, 2011; Husfeldt, 2011; Penninckx and Vanhoof, 2015). In their recent systematic review, Hofer et al. (2020) identify positive inspections effects on school evaluation activities, probably due to the goal to prepare for future inspections. For example, English schools rated as “requires improvement” are faced with shorter inspection cycles (cf. Chapman, 2002). By contrast, negative and non-significant inspection effects were found in general for school or instructional processes (e.g., Gärtner et al., 2014; Ehren et al., 2016).
Effects on pupils
When it comes to pupils (Outcomes, see Figure 1), there is nascent, but still inconclusive evidence linking the use of data or evidence to learning or performance (see Figure 1; e.g., Mincu, 2014; Cain, 2015; Cordingley, 2015; Godfrey, 2016; Rose et al., 2017; Crain-Dorough and Elder, 2021). Extant studies have tended to be small scale and qualitative, so providing limited causal pathways linking research use by teachers and improved pupil performance. A similar picture emerges for data-based classroom and school development measures with findings suggesting their effect on pupil performance in the medium and long term is ambiguous (e.g., Hellrung and Hartig, 2013; Richter et al., 2014; Kemethofer et al., 2015; Lai and McNaughton, 2016; Van Geel et al., 2016). A different picture emerges for the effects of school inspections, as a specific example of quality control in education and the ensuing data feedback: According to Hofer et al. (2020), school inspections appear mainly to accomplish the enforcement of policy in schools. Even though they report twice as much non-significant effects identified compared to positive inspection effects, the latter most consistently emerged for pupil performance in standardized achievement tests – which critical voices might trace back to a narrowing of the curriculum and unwarranted teaching to the test (Au, 2007; Collins et al., 2010; Ehren and Shackleton, 2014).
Research questions
Overall, a causal link between inspections and school improvement cannot be clearly supported from the literature. Moreover, whether the available information about the scientific foundation of data feedback, or scientific evidence are considered by educational practitioners, is influenced by data or individual characteristics (a.o., Prenger and Schildkamp, 2018), In particular, the feeling of being controlled is reported to have detrimental effects on the development processes based on data (Kuper and Hartung, 2007; Maier, 2010; Groß Ophoff, 2013). But the focus of this paper lies particularly on the contextual conditions of research informed education. In that regard, innovative and data-based school culture, evidence-oriented leadership, communication, and collaboration (as indicators of trusting relationships, cf. Datnow and Hubbard, 2016), but also professional development measures are deemed supportive (Diemer and Kuper, 2011; Groß Ophoff, 2013; Vanhoof et al., 2014; Van Gasse et al., 2016; Van Geel et al., 2016; Wurster, 2016; Brown and Malin, 2017; Keuning et al., 2017; Schildkamp et al., 2018).
As stated above, there is – on one hand – a push by governments toward school and teacher engagement with research, evidence, and data in order to drive school “self improvement”; on the other hand, a lack of evidence suggesting that this actually has any materially positive outcomes for students. Given the hierarchist context within which particularly the English school system operates, and the role of inspection in reporting information about school’s educational quality by “signposting” which and how educational standards are to be achieved, in this paper we seek to ascertain a link between both data-based and evidence-informed school “self improvement” and student outcomes. For that purpose, data from a survey study of teachers and school leaders at English primary schools (Brown et al., 2016) is re-analyzed in combination with the schools’ previous Ofsted school inspection ratings and the KS 2 SAT results of pupils in the school year 2014/2015 (in both cases referential data, cf. Wiesner and Schreiner, 2019). In the survey study, school staff provided information about the research use climate (as an indicator of Evidence, see Figure 2) at their respective schools, which corresponds with the transition from the empirical to the inferential phase. In the study presented, characteristics like the perceived trust among colleagues and the organizational learning (both Context, see Figure 2) are particularly taken into consideration, because they are expected to support the research use climate at school (Evidence, see Figure 2). Mediated by ensuing change measures this is supposed to support pupil performance in standardized assessment tests like the KS2 SAT (Outcomes, see Figure 2). Furthermore, schools with other than “outstanding” inspection ratings might particularly perceive pressure to self-improve, for example based on or informed by research. And in that respect, the annual results in centralized assessment tests, but also the implementation of school inspection ratings (Data, see Figure 2) are both feedback sources that are strongly shaped by the specific conditions of the educational system. So, in the case of this study, the English system of quality control in education serves as an example of the more general contextual conditions, under which schools are supposed to improve based on, or informed by data, evidence, or research.
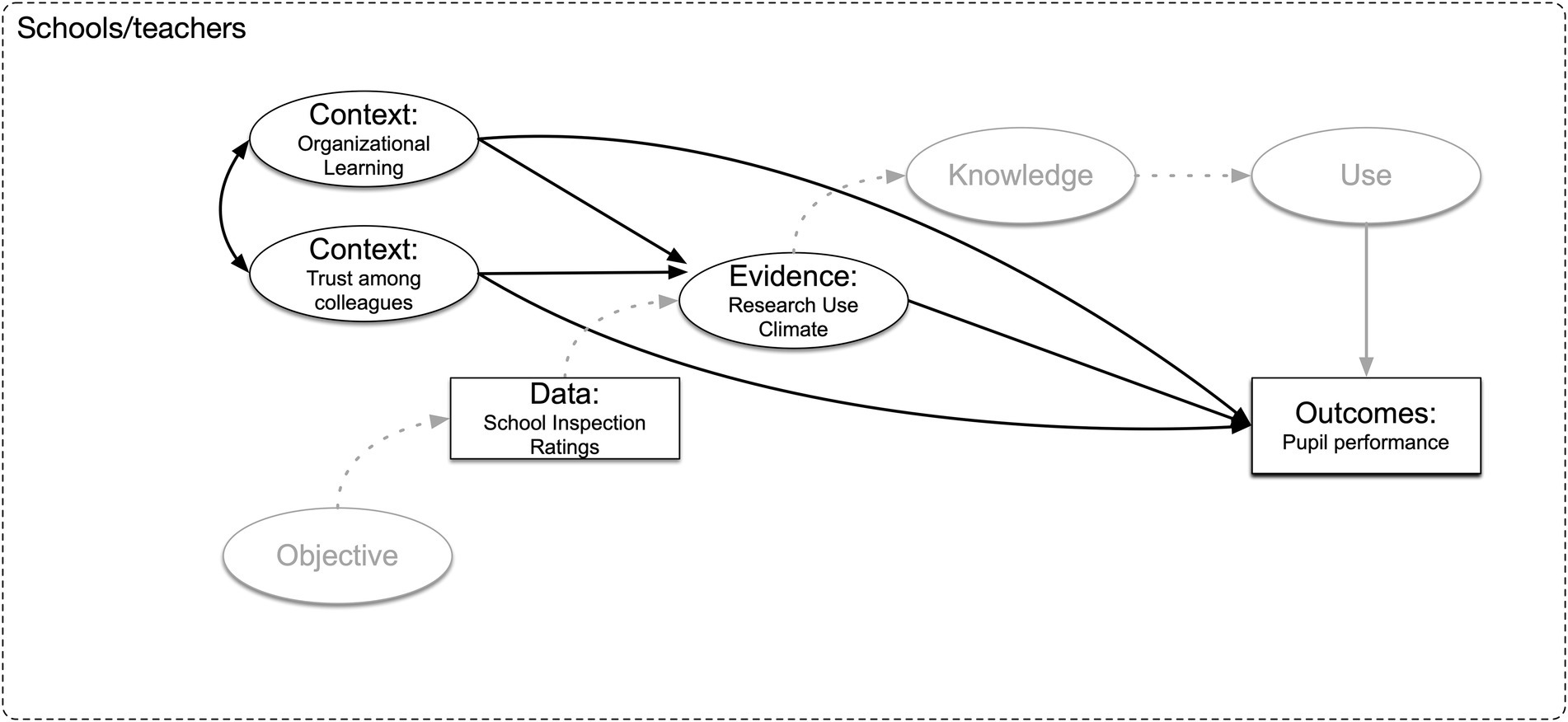
Figure 2. Conceptual framework of research informed-educational practice with the constructs included in the study presented in this paper.
Beyond providing insights into the links between data feedback (school inspection ratings and report) and evidence use at English schools, the specific contribution therefore lies in operationalizing the research use climate at schools as a possible mediating mechanism to support pupil performance on school organizational level. This points to the following research questions, that are ordered according to the steps of our analysis (see Chapter 4):
First of all, we assume that pupil performance (Outcome, see Figure 2) on the school level is positively influenced by the extent of research use at the same school (Evidence):
RQ1: What effect do teachers’ perceptions of the research use climate of their school have on the overall pupil performance in KS2 SATs?
Secondly, the research use climate (Evidence) is itself dependent on the trust and organizational climate within the school (Context, see Figure 2). Therefore, we investigate:
RQ2: What effect do teachers’ perceptions of the presence of in-school organizational learning and collegial trust have on the perceived research use climate at school, but also on the overall pupil performance in KS2 SATs?
Because of that, the research use climate (Evidence) represents a variable that supposedly mediates the relationship between trust/organizational climate (Context) and pupil performance (Outcomes). Such an effect should be reflected in statistically significant, indirect effects of trust/organizational climate on student achievement:
RQ3: Does the perceived research use climate actually mediate the association between organizational learning and trust at school and the overall school performance?
The feedback of school inspection ratings (Data) is expected to shape development activities on school level (Context, Evidence), both regarding their extent, but also the paths between the variables considered in RQ1-RQ3:
RQ4: Is there a difference in the research use climate between schools that were rated differently in school inspection? And how does this affect the association between trust among colleagues, organizational learning, research use, and performance in KS2 SAT?
Materials and methods
Sample
The sample for this study was gathered within a project that sought to investigate how schools can be supported in applying existing research findings to improve outcomes and narrow the gap in pupil outcomes. Funding for the project was granted by the Education Endowment Foundation in 2014. Schools were recruited by Brown and colleagues through use of Twitter, the direct contacts of the project team and via direct mail (e)mailing lists held by the UCL Institute of Education’s London Centre for Leadership in Learning. Schools were invited to sign up to the project straight away, to discuss the project and any queries directly with the project team or to attend one of two recruitment events held in June 2014. For the analysis presented below, a sample of 1,457 staff members from 73 primary schools was available. Approximately 20 teachers per school answered the survey. In terms of their characteristics, 70 percent of the study participants had, at that time, less than four years of experience working in their current position. Further, 81 percent were female; approximately 48 percent were serving as a subject leader (e.g., math lead or coordinator); and 18 percent held a formal and senior leadership position (e.g., headteacher). The majority of participating schools were judged as “good” in their most recent school inspection (67.1%), and a smaller amount were graded as “outstanding” (26.0%) or as “requir[ing] improvement” (4.1%), while for 2.7 percent no such information was available.
Data collection and operational variables
The survey data was collected during autumn of 2014 and included self-assessment scales (see below) and demographic background variables. Furthermore, social network data was collected, for which the results have been published elsewhere (Brown et al., 2016). In this paper, additional information on school inspection ratings and pupil performance in KS2 SATs for each participating school have been included in the analysis presented below.
School inspection rating (grouping variable)
School inspection results were documented for each of the schools investigated and were available before the study was carried out. However, it should be noted that the inspections were not carried out within the same time frame, but within a four-year interval for each school. The inspection results were used as control (grouping) variable in the analysis below. Schools are classified on a four-point grading scale used for inspection judgments as outstanding (grade 1), good (grade 2), requires improvement (grade 3), and inadequate (grade 4). To be judged, for example, as “outstanding,” schools must “must meet each and every good criterion” of overall school effectiveness (Office for Standards in Education, 2022), that is, with regard to the (former) four key judgment categories (i) achievement of pupils, (ii) the quality of teaching, (iii) the behaviour and safety of pupils, and (iv) leadership and management (Office for Standards in Education, 2022). None of the participating schools were rated as inadequate.
Trust among colleagues (predictor)
Instruments operationalizing trust in colleagues (Hoy and Tschannen-Moran, 2003; Finnigan and Daly, 2012) were adapted to the study sample and context. The final trust (TR) scale consisted of six items, on a five-point Likert type scale, which ranged from 1 (strongly disagree) to 5 (strongly agree), measuring teachers’ perceptions as to the levels of trust within their school. For example, by asking respondents to indicate the extent to which they were in agreement with statements such as “Staff in this school trust each other” (TR1, see Table 1). For this scale, Brown et al. (2016) identified a single factor solution on individual level, explaining 52.9 percent of the variance with Cronbach’s α of 0.82.
Organizational learning (predictor)
The organizational learning (OL) scale was drawn from a previously validated instrument (Garvin et al., 2008; Finnigan and Daly, 2012) and was again adapted to fit the study context. The OL scale is composed of six items on the same five-point Likert type scale, and measures schools’ capacity, cultures, learning environments as well as their structures, systems, and resources. A sample item is: “This school experiments with new ways of working.” (OL1, see Table 1). Based on exploratory factor analysis (EFA), on individual level a one-dimensional solution was identified (Brown et al., 2016) explaining 62.2 percent of the variance with Cronbach’s α of 0.88.
Research use climate (predictor, mediator)
The research use (RU) climate scale was adapted from a previous study (see Finnigan and Daly, 2012) and is composed of seven items on the same five-point Likert type scale. The construct measures participants’ perceptions as to whether school cultures are geared toward research use, both in terms of whether teachers felt encouraged to use research and evidence, and whether they perceived the improvement strategies of their schools to be grounded in research and evidence. For example, a sample item from the scale is “My school encourages me to use research findings to improve my practice.” (RU3, see Table 1). Based on EFA with only three of seven items, a single factor solution was identified by Brown et al. (2016) explaining 63.3 percent of the variance with Cronbach’s α of 0.71. In the current (re-)analysis, all seven items were included in the identification of the measurement model on both individual and school level.
Pupil performance (criterion)
The outcome variable in the path model analyzed below is average pupil performance operationalized by the percentage of students, who reached level 4 (L4, see Table 1) in the Key Stage 2 (KS2) Standard Assessment Test (SAT), i.e., the expected level to be achieved by the average 11 year old. This high-stakes test is carried out in the core subjects of English and Mathematics (since 2010 not anymore in Science, cf. Isaacs, 2010) in English state schools at the end of primary education (year 6) prior to the move to senior school (e.g., Tennent, 2021). The KS2 SAT results are published on school level for accountability and comparative purposes.1 The data set analyzed here contains KS2 SAT-results for the school year 2014/2015.
Analysis
In the present study, the specific characteristics of the school environment and its effects on a schools’ average pupil performance are of interest, which is why the appropriate level of analysis is the group level or school level. Hence, to investigate effects of the school context, the analytical approach of choice is a multilevel (or two-level) model. Accordingly, multilevel structural equation modelling was applied. All analyses were conducted in R (R Core Team, 2021) in combination with Mplus 8 (Muthén and Muthén, 2017).
As the available sample “only” comprises of 73 schools (of which only 3 schools with overall 25 teachers were judged as “requires improvement”), full multilevel latent covariate models (controlling for the measurement and sampling error = latent-latent) may not perform best. Instead, latent-manifest or manifest-latent models are superior with regard to estimation bias (Lüdtke et al., 2011; McNeish and Stapleton, 2016). This is why a sequence of different models [i.e., single level model, multilevel model (latent-latent), multilevel model (latent-manifest)] was performed to gain an understanding, whether different analytical approaches lead to differences in the coefficients of interest (i.e., the effects of trust and research use on school-average performance, see Supplementary Appendix A1). To account for the multilevel structure of the data (teachers clustered in schools; ICC of the items, see Table 1) we used TYPE = COMPLEX in all single level analyses and TYPE = TWOLEVEL in all multilevel analyses. The models were estimated by a robust maximum likelihood estimation (MLR). To determine the model fit, common cut-off criteria were used (Hu and Bentler, 1999) – Bentler’s comparative fit index (CFI ≥ 0.90), the Tucker-Lewis index (TLI ≥ 0.90), the root mean square error of approximation (RMSEA ≤0.08), and the standardized root mean square residual — at both the teacher and school levels (SRMR ≤0.08).
All of the variables used have missing values. Most of the items assessed contained between 9.6% (L4) and 53.2% (OL) missing values. To account for missing information, the Full Information Maximum Likelihood (FIML) method was used as implemented in Mplus.
Results
In the following, descriptive statistics and the model fit evaluation are reported. Subsequently, results related to the four research questions are presented.
Descriptive statistics
Table 2 contains the descriptive statistics, i.e., mean values, standard deviations, as well as reliability information and bivariate correlations. The mean values of the three scales all exceed the value of 4 and are thus quite high for a five-point Likert-scale, indicating ceiling effects. In line with the high mean scale values, the standard deviations are low, i.e., which means that there is high agreement among the teachers in general. Internal consistency (i.e., Cronbach’s alpha) is acceptable to good. The Level 4-value of 0.83 indicates further, that at the school investigated here, 83 percent of the students reached level 4 at the KS2 SAT on average.
On the right side of Table 2, correlation coefficients are reported (lower triangle = single level correlations, upper triangle = level 2 correlations). Depending on the analysis level, the associations between the study variables vary in size: In case of single-level analysis, the latent variables correlate only weakly compared to multilevel analysis, where the variables correlate moderately to strongly. In particular, RU and OL show a high correlation (r = 0.733). In order to avoid problems related to multicollinearity, in the final multigroup models (model 3–5, see Table 3) a joint factor representing RU and OL was specified, while in single level analyses (model 1 and 2), the originally assumed factor structure is kept (Supplementary Appendix A2 provides a test of the factor structure of RU and OL). The differences between the single-and multilevel model are illustrated in Figures 3, 4.
Model fit evaluation
In Table 4, the fit indices of the final analyses are reported. In Models 1 and 2, data is analysed on level-1 (teachers), while in Models 3 to 5 multilevel analysis was applied (Table 3, second row from left). Furthermore, Models 1, 3 and 4 analyze the full sample, whereas Models 2 and 5 distinguish between schools that were judged as “good” vs. “outstanding” in school inspection (third row from left, multigroup analysis).
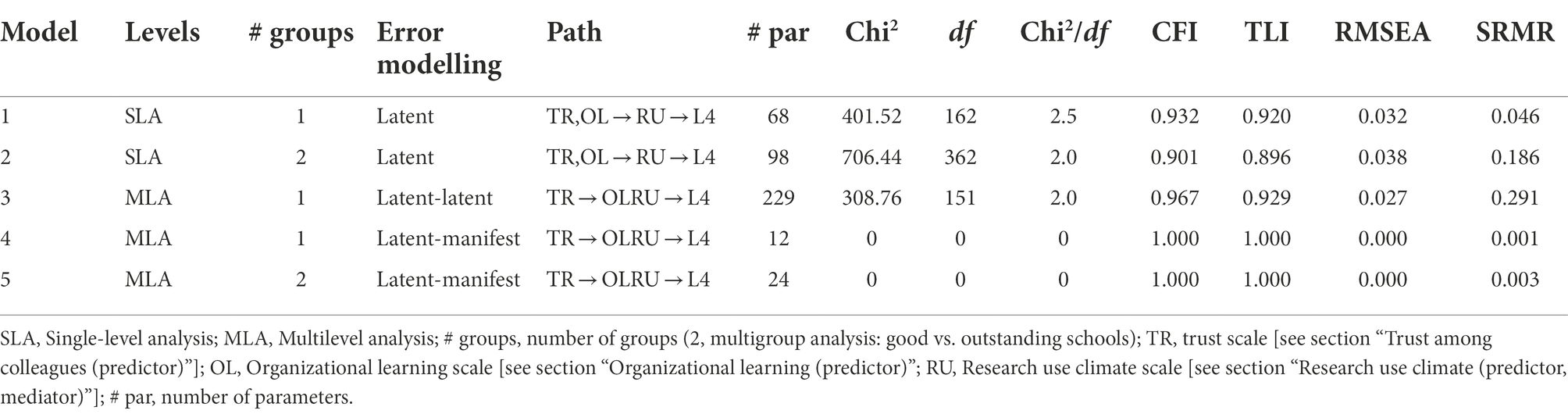
Table 4. Model fit indices for the structural models on single-level and multilevel for both single group and multigroup analysis and variants of error modelling.
According to Table 4, models 1 to 3 show acceptable to good fit, indicating that the proposed measurement models account reasonably for the observed data. In these cases, both measurement and sampling error are controlled. Deviating from this, in Models 4 and 5, residuals were modeled latent-manifest, which means that only sampling error is controlled. Because of that, both models are saturated and no model fit indices are provided.
Results for the research questions
RQ1: What effect do teachers’ perceptions of the research use climate of their school have on the overall pupil performance in KS2 SATs?
Teachers’ perception of the research use climate of their school is only significantly related to overall pupil performance in KS2 SATs at the school when the data is modeled at teacher level (single level analysis). However, the corresponding effect size is low (βRU → L4 = 0.130), but statistically significant (p = 0.05). That is, teacher’s individual perception of the research use climate is higher at schools with higher pupil performance. If the data is modeled at school level (multilevel analysis), the effect size is large (βOLRU → L4 = 0.507) but statistically insignificant (p = 0.965).
RQ2: What effect do teachers’ perceptions of the presence of in-school organizational learning and collegial trust have on the perceived research use climate at school, but also on the overall pupil performance in KS2 SATs?
As expected, teachers’ perceptions of the presence of in-school organizational learning and collegial trust significantly predict the perceived research use climate at school. The effect size of in-school organisational learning is βTR → RU = 0.137 (p = 0.006) when modeled at teacher level. The effect size of trust is βTR → RU = 0.290 (p < 0.001) when modeled at teacher level; and even higher when modeled at school level (latent-manifest: βTR → RUOL = 0.457, p = 0.060; latent-latent: βTR → RUOL = 0.651, p = 0.001). Contrary to our assumptions, neither teachers’ perceptions of the presence of in-school organizational learning nor collegial trust is significantly positively related to pupil performance in the five estimated models. Effect sizes range from βOL → L4 = −0.181 to.517. However, they do not reach statistical significance.
RQ3: Does the perceived research use climate actually mediate the association between organizational learning and trust at school and the school performance?
When modeled at teacher level, the indirect effects of organizational learning and trust at school on school performance via research use climate at school (= mediation) are weak (βOL_ind = 0.018, βTR_ind = 0.038) but statistically significant (95%-CIOL_ind: [0.006, 0.038], 95%-CITR_ind: [0.014, 0.079]). When modeled at the school level, the indirect effect of trust at school on school performance via the newly formed joint factor “research use climate and organizational learning at school” (= mediation) is comparably high in effect size (βTR_ind = 0.330), but – due to the small sample on school level – does not reach statistical significance.
RQ4: Is there a difference in the research use climate between schools that were rated differently in school inspection? And how does this affect the association between trust among colleagues, organizational learning, research use, and performance in KS2 SAT?
In preparation of the analyses related to RQ4, measurement invariance analyses were conducted to examine whether the instruments used to capture the study variables performed equally well in the three groups with different school inspection ratings (i.e., requires improvement, good, outstanding schools). The results show that only partial measurement invariance could be established (cf. Supplementary Appendix A3 for details).
Group comparison results (see Table 5) indicate that particularly schools, who received an school inspection rating as “good” (meaning that one key area was identified as “requires improvement,” cf. Office for Standards in Education, 2022) report a significantly lower mean value for research use climate at school (M = 4.37) compared to schools rated as “requires improvement” (M = 4.78) and “outstanding” (M = 4.72). The same is true for the other study variables trust and organisational learning. In other words, staff at “good” schools report slightly lower trust among colleagues and are a little less active in organizational learning and research use. Furthermore, there is also a considerable difference of 10 % between pupils’ performance in KS2 SATs in schools that were rated as “good” or as “requires improvement” and “outstanding” schools.
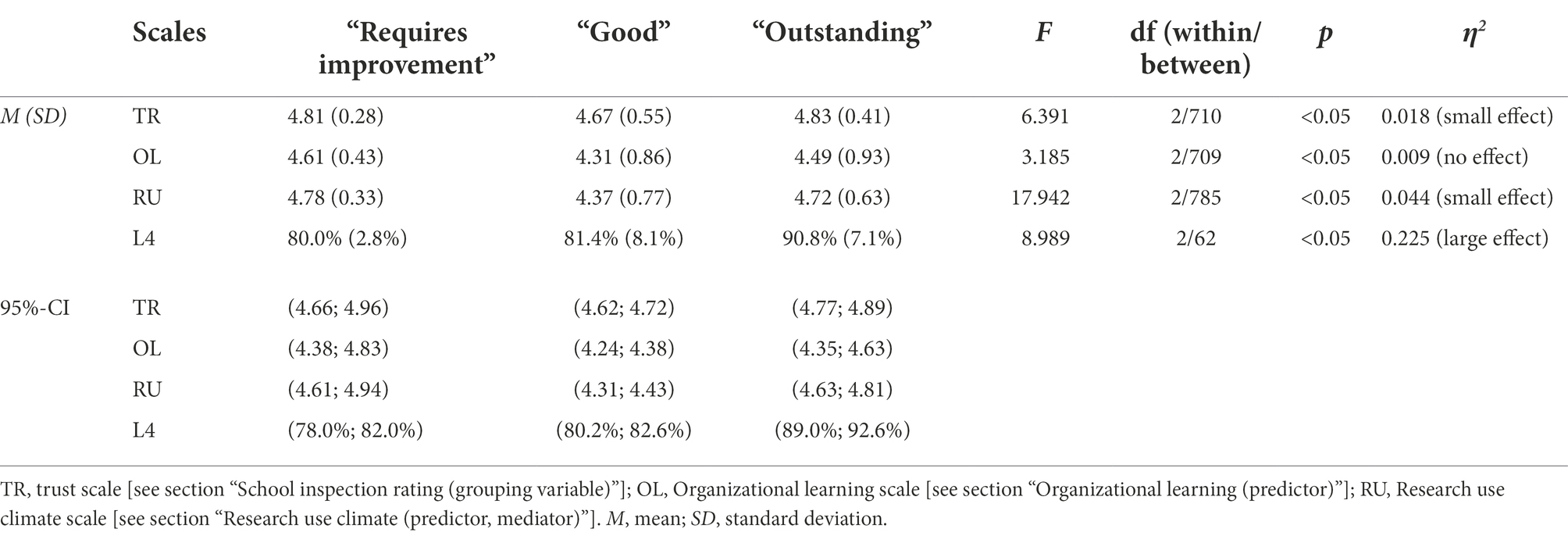
Table 5. Mean differences for trust, organizational learning, research use (individual level) and Level 4-results (school level).
Regarding the association of the study variables, the multigroup comparison further shows, that only for schools rated as “good,” the research use climate is significantly predicted by trust (βTR → RU = 0.291, p < 0.001) and organisational learning (βOL → RU = 0.201, p = 0.008), while this is not true for schools rated “outstanding” (see Table 3).
Discussion
Even though it is unusual to start the discussion of a paper with its limitations, we would like to point out some, because they are of importance for the conclusions that can be drawn from this study: First of all, the data originates from the school year 2014/2015, and is therefore of some “age.” Between 2022 and 2014, certainly a lot of changes have taken place. The United Kingdom’s departure from the European Union in 2020 or the world-wide COVID-19-pandemic and the ensuing school closures are two examples that come to mind. English schools were affected by school closures during the pandemic, too, and – among others – the ramifications of cancelling traditional, centralised exams highlighted that the English market-and accountability oriented educational system is prone to crisis (Ziauddeen et al., 2020; McCluskey et al., 2021). But despite the continuing voices of criticism (Jones and Tymms, 2014; Perryman et al., 2018; Grayson, 2019; Coldwell, 2022), the overall educational governance strategy has remained the same (e.g., Office for Standards in Education, 2022; UK Government, 2022). Still, a new framework for school inspection has been introduced in 2019 and was updated just recently (major changes are, a.o., new school inspection labels, end of transition period for updating school curricula, new grade descriptors, etc., cf. Office for Standards in Education, 2022). Another limitation of the study presented here is, that even though the school inspection ratings existed before the study was carried out, the research design is not longitudinal, but correlational. In other words, causal interpretations are not eligible here. However, part of the re-analyzed data was originally used in the article by Brown et al. (2016), but particularly the school inspection ratings and the average pupil performance in the Key Stage 2 (KS2) Standard Assessment Test (SAT) were not included then. Therefore, the correlation with school organisational characteristics has not been analyzed until now. Another limitation is that even though the research use climate reported by school staff was included as a theoretically sound mediating variable (Vanhoof et al., 2014; Van Gasse et al., 2016; Keuning et al., 2017; van Geel et al., 2017; Schildkamp, 2019), we cannot know to what extent the schools in this sample engaged with the school inspection results, or more general, what kind of research was actually used, what (if any) improvement measures were implemented, and what other influencing factors were involved. Nor do we know how apt the teachers and school leaders in the current sample were in evidence-informed reasoning, or how they could have been scaffolded in that regard. This rather requires – at best–controlled before-after studies. Some examples for such study designs can be found in the field of evidence-informed initial teacher education, of which some are represented in this special issue (e.g., Futterleib et al., 2022; Grimminger-Seidensticker and Seyda, 2022; Lohse-Bossenz et al., 2022; Voss, 2022). Further limitations of this study are, that the sample size at level 2, i.e., the school level, was too low to estimate complex models like the multilevel latent covariate model and multilevel multiple group models. Furthermore, the schools included in this study might be more predisposed to research engagement than the majority of England’s primary schools, as they voluntarily applied for participation in a project that sought to investigate how schools can be supported RIEP.
We are aware, of course, that the average pupil performance in KS2-assessments is comparatively distal to collaborative research use processes among school staff, and the findings need to be interpreted with due caution because of that. Nonetheless, we insist that is an important contribution to this field to validate common claims, like for example, that teachers’ engagement with research (including centrally administered data and research evidence) facilitates professional and school development, and mediated by that, pupil performance (e.g., Davies, 1999; Slavin, 2002). Therein lies the originality of this paper. As this study was carried out in the field of educational practice, this corroborates the external validity of the findings presented here, too. Another strength is theoretical foundation of our approach (cf. Chapter 2) that combines the perspective of data-based decision making (as theory of action, cf. Mandinach and Schildkamp, 2021) and of evidence-informed education (as theory of learning, cf. Groß Ophoff and Cramer, 2022) – which was already proposed by Brown et al. (2017). For example, in this study the two forms of data feedback on school level were treated as (referential) data (in the tradition of data-based decision-making). According to the phases of the inquiry cycle, the school inspection results stand for evaluatory data at the conceptual phase (2), during which school staff ought to appraise the feedback (aka inspection report) under consideration of local school data with the medium-to long-term goal of “self-improvement” (see Figure 1). In turn, pupils’ performance in the KS2 assessments are located at the end of the inquiry cycle and therefore represent data that schools can use to evaluate (phase 5) the impact of hypothetical improvement measures. Both trust among colleagues and organisational learning were treated as school-contextual factors, that have been repeatedly identified as important for educational change in general (Louis, 2007; Ehren et al., 2020) and for the use of data or evidence in particular (Schildkamp et al., 2017; Gaussel et al., 2021; Brown et al., 2022). School staff’s reported research use climate was then modeled as an indicator of evidence-informed educational practice and was included in the analysis as mediator between the school organisational context and pupil performance at the end of the school year 2014/2015. So what is to be learned from the results?
According to the first research question (RQ1), an effect of teachers’ perceptions of the research use climate on the overall pupil performance in KS2 SATs was expected. But only a small effect, and only on teacher level could be identified. In other words, school staff who reported a higher research use climate worked at schools with higher pupil performance.
The second question (RQ2) aimed at investigating the effect of teachers’ perceptions of the presence of in-school organizational learning and collegial trust on the perceived research use climate at school, but also on the overall pupil performance. In line with the current state of research (see above), our findings indicate that both the perception of in-school organizational learning and collegial trust significantly predict the perceived research use climate at school, but not the pupil performance in central assessments. It should be noted, that on school level the latent constructs of research use and organizational learning could not be separated psychometrically as originally proposed by Brown et al. (2016). In deviation from the original analysis, we used a larger (available) school staff sample and all seven items assigned to research use, and we evaluated the measurement models not only on teacher, but on school level, too. Notwithstanding, this finding is theoretically plausible, as both constructs refer to collaborative learning processes required for the identification, application, and evaluation of school and instructional development measures (Brown et al., 2021).
The third research question (RQ3) pursued as to whether the perceived research use climate mediates the association between organizational learning and trust at school on the one hand and the average pupil performance on the other. This could be demonstrated both on teacher and school level, with the higher effect for the latter. This means that schools with a higher average value of trust among colleagues report more organizational and research informed activities and, mediated by that, demonstrated better results in the average pupil performance assessment at the end of the school year 2014/2015.
The fourth research question (RQ4) finally asked whether there is a difference between schools that were rated as “outstanding,” “good,” or “requires improvement” in the means of central variables like trust, organizational learning, research use climate, and average pupil performance, and the path model (see RQ3). And in fact, differences emerged here, too:
• “Good” schools demonstrated a significant lower percentage of students, who reached level 4 in the Key Stage 2 (KS2) Standard Assessment Test (SAT) compared to “outstanding” schools, and its staff reported slightly lower trust among colleagues, and to be slightly less active in organizational learning and research use.
• “Outstanding” schools showed considerably better results in KS2 SATs, that could not be predicted by the research-informed organizational learning processes at school or the trust among colleagues. Nonetheless, school staff reported a slightly more pronounced research use climate and trust among colleagues.
• School rated as “requires improvement” showed school organisational results comparable to outstanding schools, but were less successful in pupil performance assessment.
In particular, the more staff at “good” schools reported of organizational and research informed activities, the better was the average pupil performance in centralized assessments. In contrast, both outstanding schools and schools that require improvement appeared to engage more with research evidence, even though the former seemed not to profit (or need?) it. However, no conclusion can be made about schools that required improvement because of their too small proportion (3.9%) in this sample. In comparison, in the school year 2014/2015 19 percent of British primary schools received the same rating (Office for Standards in Education, 2015).
In sum, we could replicate the findings by Brown et al. (2016), according to which trust among colleagues is of vital importance in initiating research-informed educational practice. But our analysis goes beyond, as we could show that assumptions derived from the conceptual model of research-informed educational practice stood the test. School organizational conditions like trust among colleagues and organizational learning proved to be supportive for the research use climate on teacher level, and mediated by that, for the performance of pupils in assessment tests. Particularly interesting is, that on school level, organizational learning and research use climate could not be separated psychometrically, which supports the notion of research-informed education as a learning process that can (and should) take place on school level, too (cf. Argyris and Schön, 1978). Furthermore, it could be substantiated, that schools differ in the extent of research-informed organizational learning and in their “paths” to pupils’ performance depending on their school inspection ratings. This was particularly true for schools rated as “good” in school inspections. These schools are required to improve at least one key area of educational quality, but reported the lowest research use climate in this study. In our view, this finding is cause for optimism, as particularly such schools seem to benefit from an engagement with evidence and might be convinced to use it more, for example because of by professional development. In line with that, there is ample evidence on the importance that practitioners need to be convinced about the usefulness of data and evidence to engage with it in a meaningful way (e.g., Hellrung and Hartig, 2013; Prenger and Schildkamp, 2018; Rickinson et al., 2020).
So, what are the further implications of this study? First of all, our findings support the notion that the inquiry cycle of research-informed education (see Chapter 2) can and should combine the approaches to data-based decision-making and evidence-informed education (Brown et al., 2017). This is supported by the notion that “the strengths of each appear to mirror and compensate for the weaknesses of the other” (Brown et al., 2017, p. 156). For example, the authors identify as strength of data-based decision-making, that the school-specific vision and goals are considered for the problem identification (conceptual phase). Weaknesses are in turn, that ample data literacy is required for meaningful and in-depth data use processes. Data cannot provide educators with solutions that “work best.” Instead, a substantive content expertise is needed to be able to identify potential causes and solutions of a problem. In turn, the strength of evidence-informed educational practice lies in enabling schools to identify and understand the underlying mechanisms of effective approaches to improving teaching and learning, and provides (at best) instructions how research-informed approaches might be implemented to address a given problem. The pitfalls of this approach are, that it is challenging to recognize the (re-)sources that are adequate and relevant to the problem at hand. This particularly requires research literate teachers and school leaders. Continuing Brown et al.’s (2017) line of reasoning, the field of evidence-informed teacher education is another promising asset to the conceptualization of research-informed education. Research in this field focusses on the necessary learning processes and effective teaching strategies in higher education (error-based learning, e.g., Klein et al., 2017; case-based learning, e.g., Syring et al., 2015; inquiry learning, e.g., Wessels et al., 2019). Even though the strong focus on intervention studies in this field is a strength from a research-methodological perspective (internal validity), it is yet unresolved, whether the resulting insights can be applied to professional development and educational practice (external and consequential validity).
A promising approach that somewhat combines the theories of action and learning, is Beck and Nunnaley’s (2021) continuum of data literacy for teaching: Based on the works of Shulman (1987), Beck and Nunnaley distinguish four levels of expertise (novice users, developing users, developing expert users, expert users) for the use of data and apply them to the different phases of the inquiry cycle. For example, novice users may recognize that a problem exists, but are unable to identify relevant and appropriate data sources, and face difficulties in establishing connections between data and one’s own teaching methods or even in deriving improvement measures. With increasing expertise, for example developing users are already able to recognize the connection between a question and different data and evidence sources, but the effects of changes measures can still only be monitored superficially, etc. Even though the authors remain quite vague as to how particular levels of expertise can be reached, they see potential in practical training that is supported by (academic) mentors for pre-service teachers. For in-service teachers, they propose coaching (e.g., Huguet et al., 2014) or establishing professional learning communities (a.o. Brown, 2017; Brown et al., 2021) based on long-term and goal-oriented engagement with research. But obviously, the current conditions in the English educational-political context are not favorable in that regard: According to Coldwell (2022), educational governance “militates against widespread research evidence use” (Coldwell, 2022, p. 63) due to budget cuts, high accountability pressures, and the (unwarranted) politicization of research use. Related to that, unintended responses and side effects of school inspection and other data-based accountability measures have been a constant, still unresolved issue in this research field (a.o., Ehren and Visscher, 2006; Bellmann et al., 2016). This illustrates further, why it is instructive to take a closer look at educational systems that, in the case of England, represent a high-stakes quality control system, particularly as educational governance tends in general to oscillate between control and autonomy (e.g., Higham and Earley, 2013; Altrichter, 2019).
For future research, a comprehensive model of research-informed education is certainly useful, but necessitates the clarification, which kind of research (data, evidence, or a combination like in the current study) is of interest in the respective study, and related to that, which inquiry phases are to be investigated, what the target group of research users is (pre-service teachers, in-service teachers, or a combination), and on which level (school, staff like teachers or school leaders, pupils, etc.) development processes are expected. In future studies, both schools that “require improvement,” but also schools judged as “inadequate” need to be explicitly included, and at best oversampled, in order to gain more in-depth insights into the particular challenges for these schools (e.g., Keuning et al., 2017). But also schools rated as “good” are of interest, and so-called design-based approaches are auspicious in identifying enablers and barriers of self-improvement processes at schools (e.g., Mintrop et al., 2018; Mintrop and Zumpe, 2019). Another question for future research could be in particular, how trust as a crucial contextual condition of research-informed education can be fostered, for example by local educational leaders (e.g., meta-analysis for the economic sector: Legood et al., 2021), but also by central authorities (for the English educational system, e.g., Taysum, 2020). With regard to specifics of the study design presented here, further implications emerge: In particular, the lack of full measurement invariance for all three self-assessment scales (trust, organizational learning, and research use climate, see Data collection and operational variables and Supplementary Appendix A3) indicates that the items have different meanings across schools groups with different Ofsted ratings. This is an interesting finding, that calls for further in-depth inquiry. As mentioned above, the investigated path model relies on theoretically established (causal) assumptions, that cannot be conclusively tested based on the current cross-sectional data, but should be investigated in longitudinal studies. Furthermore, the path from organizational learning and communication processes is a long one, and other plausible mediators are not covered in our dataset, which is another mandate for future research.
Data availability statement
The data analyzed in this study is subject to the following licenses/restrictions: The data was kindly provided by Chris Brown, University of Warwick, UK. Requests to access these datasets should be directed to Chris.D.B.Brown@warwick.ac.uk.
Ethics statement
The studies involving human participants were reviewed and approved by UCL, Institute of Education. The patients/participants provided their written informed consent to participate in this study.
Author contributions
CB contributed to conception and design of the study. JG and CH organized the database and performed the statistical analysis. JG wrote the first draft of the manuscript. CB and CH wrote sections of the manuscript. All authors contributed to the manuscript revision, read, and approved the submitted version.
Funding
Funding for the project was granted by the Education Endowment Foundation in 2014. No extra funding was awarded for the current re-analysis of the data.
Conflict of interest
The authors declare that the research was conducted in the absence of any commercial or financial relationships that could be construed as a potential conflict of interest.
Publisher’s note
All claims expressed in this article are solely those of the authors and do not necessarily represent those of their affiliated organizations, or those of the publisher, the editors and the reviewers. Any product that may be evaluated in this article, or claim that may be made by its manufacturer, is not guaranteed or endorsed by the publisher.
Supplementary material
The Supplementary material for this article can be found online at: https://www.frontiersin.org/articles/10.3389/feduc.2022.1011241/full#supplementary-material
Footnotes
References
Ali, N. J. M., Kadir, S. A., Krauss, S. E., Basri, R., and Abdullah, A. (2021). A proposed framework of school inspection outcomes: understanding effects of school inspection and potential ways to facilitate school improvemessnt. Int. J. Acad. Res. Bus. Soc. Sci. 11, 418–435. doi: 10.6007/IJARBSS/v11-i2/8451
Altrichter, H. (2019). “School autonomy policies and the changing governance of schooling” in Geographies of Schooling (Cham: Springer), 55–73.
Argyris, C., and Schön, D. A. (1978). Organizational Learning: A Theory of Action Perspective. Boston, MA: Addison Wesley.
Au, W. (2007). High-stakes testing and curricular control: a qualitative metasynthesis. Educ. Res. 36, 258–267. doi: 10.3102/0013189X07306523
Baxter, J., and Clarke, J. (2013). Farewell to the tick box inspector? Ofsted and the changing regime of school inspection in England. Oxf. Rev. Educ. 39, 702–718. doi: 10.1080/03054985.2013.846852
Beck, J. S., and Nunnaley, D. (2021). A continuum of data literacy for teaching. Stud. Educ. Eval. 69:100871. doi: 10.1016/j.stueduc.2020.100871
Bell, M., Cordingley, P., Isham, C., and Davis, R. (2010). Report of professional practitioner use of research review: practitioner engagement in and/or with research. Available at: http://www.curee.co.uk/node/2303
Bellmann, J., Dužević, D., Schweizer, S., and Thiel, C. (2016). Nebenfolgen Neuer Steuerung und die Rekonstruktion ihrer Genese. Differente Orientierungsmuster schulischer Akteure im Umgang mit neuen Steuerungsinstrumenten. Z. Pädagog. 62, 381–402. doi: 10.25656/01:16764
Boelhauve, U. (2005). “Forschendes Lernen–Perspektiven für erziehungswissenschaftliche Praxisstudien” in Zentren Für Lehrerbildung–Neue Wege Im Bereich Der Praxisphasen (Paderborner Beiträge Zur Unterrichtsforschung), vol. 10 (Münster), 103–126.
Borg, S. (2010). Language teacher research engagement. Lang. Teach. 43, 391–429. doi: 10.1017/S0261444810000170
Broemmel, A. D., Evans, K. R., Lester, J. N., Rigell, A., and Lochmiller, C. R. (2019). Teacher Reading as professional development: insights from a National Survey. Read. Horiz. 58:2.
Bromme, R., Prenzel, M., and Jäger, M. (2014). Empirische Bildungsforschung und evidenzbasierte Bildungspolitik. Z. Erzieh. 17, 3–54. doi: 10.1007/s11618-014-0514-5
Brown, C. (2017). Research learning communities: how the RLC approach enables teachers to use research to improve their practice and the benefits for students that occur as a result. Res. All 1, 387–405. doi: 10.18546/RFA.01.2.14
Brown, C. (2020). The Networked School Leader: How to Improve Teaching and Student Outcomes Using Learning Networks. Bingley Emerald
Brown, C., Daly, A., and Liou, Y.-H. (2016). Improving trust, improving schools: findings from a social network analysis of 43 primary schools in England. J. Profess. Capit. Commun. 1, 69–91. doi: 10.1108/JPCC-09-2015-0004
Brown, C., MacGregor, S., Flood, J., and Malin, J. (2022). Facilitating research-informed educational practice for inclusion. Survey findings from 147 teachers and school leaders in England. Front. Educ. 7:890832. doi: 10.3389/feduc.2022.890832
Brown, C., and Malin, J. (2017). Five vital roles for school leaders in the pursuit of evidence of evidence-informed practice. Teach. Coll. Rec.
Brown, C., and Malin, J. R. (2022). The Emerald Handbook Of Evidence-Informed Practice in Education: Learning from International Contexts. Bingley Emerald Group Publishing
Brown, C., Poortman, C., Gray, H., Groß Ophoff, J., and Wharf, M. (2021). Facilitating collaborative reflective inquiry amongst teachers: what do we currently know? Int. J. Educ. Res. 105:101695. doi: 10.1016/j.ijer.2020.101695
Brown, C., and Rogers, S. (2015). Knowledge creation as an approach to facilitating evidence informed practice: examining ways to measure the success of using this method with early years practitioners in Camden (London). J. Educ. Chang. 16, 79–99. doi: 10.1007/s10833-014-9238-9
Brown, C., Schildkamp, K., and Hubers, M. D. (2017). Combining the best of two worlds: a conceptual proposal for evidence-informed school improvement. Educ. Res. 59, 154–172. doi: 10.1080/00131881.2017.1304327
Cain, T. (2015). Teachers’ engagement with research texts: beyond instrumental, conceptual or strategic use. J. Educ. Teach. 41, 478–492. doi: 10.1080/02607476.2015.1105536
Chapman, C. (2001). Changing classrooms through inspection. Sch. Leadersh. Manag. 21, 59–73. doi: 10.1080/13632430120033045
Chapman, C. (2002). Ofsted and school improvement: teachers’ perceptions of the inspection process in schools facing challenging circumstances. Sch. Leadersh. Manag. 22, 257–272. doi: 10.1080/1363243022000020390
Coburn, C. E., and Turner, E. O. (2011). Research on data use: a framework and analysis. Measurement 9, 173–206. doi: 10.1080/15366367.2011.626729
Coldwell, M. (2022). “Evidence-informed teaching in England” in The Emerald Handbook of Evidence-Informed Practice in Education. eds. C. Brown and J. R. Malin (Bingley: Emerald Publishing Limited), 59–68.
Collins, S., Reiss, M., and Stobart, G. (2010). What happens when high-stakes testing stops? Teachers’ perceptions of the impact of compulsory national testing in science of 11-year-olds in England and its abolition in Wales. Assess. Educat. 17, 273–286. doi: 10.1080/0969594X.2010.496205
Cordingley, P. (2015). The contribution of research to teachers’ professional learning and development. Oxf. Rev. Educ. 41, 234–252. doi: 10.1080/03054985.2015.1020105
Crain-Dorough, M., and Elder, A. C. (2021). Absorptive capacity as a means of understanding and addressing the disconnects between research and practice. Rev. Res. Educ. 45, 67–100. doi: 10.3102/0091732X21990614
Datnow, A., and Hubbard, L. (2016). Teacher capacity for and beliefs about data-driven decision making: a literature review of international research. J. Educ. Chang. 17, 7–28. doi: 10.1007/s10833-015-9264-2
Davies, P. (1999). What is evidence-based education? British Journal of Educational Studies, 47, 108–20.
de Wolf, I. F., and Janssens, F. J. G. (2007). Effects and side effects of inspections and accountability in education: an overview of empirical studies. Oxf. Rev. Educ. 33, 379–396. doi: 10.1080/03054980701366207
Demski, D. (2017). Evidenzbasierte Schulentwicklung: Empirische Analyse eines Steuerungsparadigmas. Wiesbaden Springer.
Department for Education. (2015). National curriculum assessments at key stage 2 in England, 2015 (revised) (National Statistics SFR 47/2015; p. 23). Department for Education. Available at: https://assets.publishing.service.gov.uk/government/uploads/system/uploads/attachment_data/file/483897/SFR47_2015_text.pdf
Diemer, T., and Kuper, H. (2011). Formen innerschulischer Steuerung mittels zentraler Lernstandserhebungen. Zeitsch. Pädag. 57, 554–571. doi: 10.25656/01:8746
Dunn, K. E., Skutnik, A., Patti, C., and Sohn, B. (2019). Disdain to acceptance: future teachers’ conceptual change related to data-driven decision making. Act. Teach. Educat. 41, 193–211. doi: 10.1080/01626620.2019.1582116
Ehren, M. C. (2018). Accountability and trust: two sides of the same coin? Invited talk as part of the University of Oxford’s: Intelligent accountability symposium II. Oxford.
Ehren, M. C., Honingh, M., Hooge, E., and O’Hara, J. (2016). Changing school board governance in primary education through school inspections. Educat. Manag. 44, 205–223. doi: 10.1177/1741143214549969
Ehren, M., Paterson, A., and Baxter, J. (2020). Accountability and trust: two sides of the same coin? J. Educ. Chang. 21, 183–213. doi: 10.1007/s10833-019-09352-4
Ehren, M. C., and Shackleton, N. (2014). Impact of School Inspections on Teaching and Learning in Primary and Secondary Education in the Netherlands, Netherlands, London: Institute of Education.
Ehren, M. C., and Visscher, A. J. (2006). Towards a theory on the impact of school inspections. Br. J. Educ. Stud. 54, 51–72. doi: 10.1111/j.1467-8527.2006.00333.x
Ermeling, B. A. (2010). Tracing the effects of teacher inquiry on classroom practice. Teach. Teach. Educ. 26, 377–388. doi: 10.1016/j.tate.2009.02.019
Evers, A. T., Kreijns, K., and Van der Heijden, B. I. (2016). The design and validation of an instrument to measure teachers’ professional development at work. Stud. Contin. Educ. 38, 162–178. doi: 10.1080/0158037X.2015.1055465
Finnigan, K. S., and Daly, A. J. (2012). Mind the gap: organizational learning and improvement in an underperforming urban system. Am. J. Educ. 119, 41–71. doi: 10.1086/667700
Futterleib, H., Thomm, E., and Bauer, J. (2022). The scientific impotence excuse in education – disentangling potency and pertinence assessments of educational research. Front. Educat. 7:1006766. doi: 10.3389/feduc.2022.1006766
Gärtner, H., and Pant, H. A. (2011). How valid are school inspections? Problems and strategies for validating processes and results. Stud. Educ. Eval. 37, 85–93. doi: 10.1016/j.stueduc.2011.04.008
Gärtner, H., Wurster, S., and Pant, H. A. (2014). The effect of school inspections on school improvement. Sch. Eff. Sch. Improv. 25, 489–508. doi: 10.1080/09243453.2013.811089
Garvin, D., Edmondson, A., and Gino, F. (2008). Is yours a learning organization? Harv. Bus. Rev. 86, 109–116.
Gaussel, M., MacGregor, S., Brown, C., and Piedfer-Quêney, L. (2021). Climates of trust, innovation, and research use in fostering evidence-informed practice in French schools. Int. J. Educ. Res. 109:101810. doi: 10.1016/j.ijer.2021.101810
Godfrey, D. (2016). Leadership of schools as research-led organisations in the English educational environment: cultivating a research-engaged school culture. Educat. Manag. Administr. Leader. 44, 301–321. doi: 10.1177/1741143213508294
Grayson, H. (2019). School accountability in England: a critique. Headteacher Update 2019, 24–25. doi: 10.12968/htup.2019.1.24
Greany, T. (2017). Karmel oration-leading schools and school systems in times of change: a paradox and a quest. Research Conference 2017-Leadership for Improving Learning: Lessons from Research, 15–20.
Greany, T., and Earley, P. (2018). The paradox of policy and the quest for successful leadership. Prof. Develop. Today 19, 6–12.
Greisel, M., Wekerle, C., Wilkes, T., Stark, R., and Kollar, I. (2022). Pre-service teachers’ evidence-informed reasoning: do attitudes, subjective norms, and self-efficacy facilitate the use of scientific theories to analyze teaching problems? Psychol. Learn. Teach. :147572572211139. doi: 10.1177/14757257221113942
Grimminger-Seidensticker, E., and Seyda, M. (2022). Enhancing attitudes and self-efficacy toward inclusive teaching in physical education pre-service teachers: results of a quasi-experimental study in physical education teacher education. Front. Educat. 7:909255. doi: 10.3389/feduc.2022.909255
Groß Ophoff, J., and Cramer, C. (2022). “The engagement of teachers and school leaders with data, evidence and research in Germany” in The Emerald International Handbook of Evidence-Informed Practice in Education. eds. C. Brown and J. R. Malin (Bingley: Emerald), 175–196.
Hammersley, M. (2004). “Some questions about evidence-based practice in education” in Evidence-Based Practice in Education. eds. G. Thomas and R. Pring, vol. 2004 (Berkshire: Open University Press), 133–149.
Harper, D., Mulvey, R., and Robinson, M. (2003). Beyond evidence based practice: rethinking the relationship between research, theory and practice. Appl. Psychol., 158–171. doi: 10.4135/9781446279151.n11
Helgøy, I., Homme, A., and Gewirtz, S. (2007). “Local autonomy or state control? Exploring the effects of new forms of regulation in education” in European Educational Research Journal, vol. 6 (London, England: SAGE Publications Sage UK), 198–202.
Hellrung, K., and Hartig, J. (2013). Understanding and using feedback–a review of empirical studies concerning feedback from external evaluations to teachers. Educ. Res. Rev. 9, 174–190. doi: 10.1016/j.edurev.2012.09.001
Hetmanek, A., Wecker, C., Kiesewetter, J., Trempler, K., Fischer, M. R., Gräsel, C., et al. (2015). Wozu nutzen Lehrkräfte welche Ressourcen? Eine Interviewstudie zur Schnittstelle zwischen bildungswissenschaftlicher Forschung und professionellem Handeln im
Higham, R., and Earley, P. (2013). School autonomy and government control: school leaders’ views on a changing policy landscape in England. Educat. Manag. Administr. Leader. 41, 701–717. doi: 10.1177/1741143213494191
Hofer, S. I., Holzberger, D., and Reiss, K. (2020). Evaluating school inspection effectiveness: a systematic research synthesis on 30 years of international research. Stud. Educ. Eval. 65:100864. doi: 10.1016/j.stueduc.2020.100864
Hoy, W. K., and Tschannen-Moran, M. (2003). “The conceptualization and measurement of faculty trust in schools: the omnibus T-scale” in Studies in Leading and Organizing Schools. eds. W. K. Hoy and C. G. Miskel (Greenwich, CT: Information Age Publishing), 181–208.
Hu, L., and Bentler, P. M. (1999). Cutoff criteria for fit indexes in covariance structure analysis: conventional criteria versus new alternatives. Struct. Equ. Model. Multidiscip. J. 6, 1–55. doi: 10.1080/10705519909540118
Huguet, A., Marsh, J. A., and Farrell, C. C. (2014). Building teachers’ data-use capacity: insights from strong and developing coaches. Educat. Pol. Anal. Arch. 22, 1–28. doi: 10.14507/epaa.v22n52.2014
Husfeldt, V. (2011). Wirkungen und Wirksamkeit der externen Schulevaluation. Z. Erzieh. 14, 259–282. doi: 10.1007/s11618-011-0204-5
Isaacs, T. (2010). Educational assessment in England. Assess. Educat. 17, 315–334. doi: 10.1080/0969594X.2010.491787
Jones, K., and Tymms, P. (2014). Ofsted’s role in promoting school improvement: the mechanisms of the school inspection system in England. Oxf. Rev. Educ. 40, 315–330. doi: 10.1080/03054985.2014.911726
Kemethofer, D., Zuber, J., Helm, C., Demski, D., and Rie, C. (2015). Effekte von Schulentwicklungsmaßnahmen auf Schüler/innenleistungen im Fach Mathematik. SWS-Rundschau 55, 26–47.
Keuning, T., Van Geel, M., and Visscher, A. (2017). Why a data-based decision-making intervention works in some schools and not in others. Learn. Disabil. Res. Pract. 32, 32–45. doi: 10.1111/ldrp.12124
Kiemer, K., and Kollar, I. (2021). Source selection and source use as a basis for evidence-informed teaching. Z. Pädagog. Psychol. 35, 127–141. doi: 10.1024/1010-0652/a000302
Klein, M., Wagner, K., Klopp, E., and Stark, R. (2017). Fostering of applicable educational knowledge in student teachers: effects of an error-based seminar concept and instructional support during testing on qualities of applicable knowledge. J. Educ. Res. Online 9, 88–104. doi: 10.25656/01:14898
König, J., and Pflanzl, B. (2016). Is teacher knowledge associated with performance? On the relationship between teachers' general pedagogical knowledge and instructional quality. Eur. J. Teach. Educ. 39, 419–436. doi: 10.1080/02619768.2016.1214128
Kuper, H., and Hartung, V. (2007). Überzeugungen zur Verwendung des Wissens aus Lernstandserhebungen. Eine professionstheoretische Analyse. Zeitschrift Für Erziehungswissenschaft 10, 214–229. doi: 10.1007/s11618-007-0028-5
Lai, M. K., and McNaughton, S. (2016). The impact of data use professional development on student achievement. Teach. Teach. Educ. 60, 434–443. doi: 10.1016/j.tate.2016.07.005
Landwehr, N. (2011). “Thesen zur Wirkung und Wirksamkeit der externen Schulevaluation [theses on the impact and effectiveness of the external evaluation of schools]” in Wirkungen und Wirksamkeit der externen Schulevaluation [Effects and Effectiveness of External School Evaluation]. eds. C. Quesel, N. Husfeldt, N. Landwehr, and P. Steiner (Bern, Switzerland: Hep der Bildungsverlag), 35–69.
Legood, A., van der Werff, L., Lee, A., and Den Hartog, D. (2021). A meta-analysis of the role of trust in the leadership-performance relationship. Eur. J. Work Organ. Psy. 30, 1–22. doi: 10.1080/1359432X.2020.1819241
Lohse-Bossenz, H., Bloss, C., and Doerfler, T. (2022). Constructing multi-theory vignettes to measure the application of knowledge in ambivalent educational situations. Front. Educat. 7
Lorenz, R., Eickelmann, B., and Bos, W. (2016). High-stakes testing and educational governance–insights and innovations in European school systems. Special Issue Editorial. J. Educat. Res. Online 8, 5–9. doi: 10.25656/01:12416
Louis, K. S. (2007). Trust and improvement in schools. Journal of Educational Change 8, 1–24. doi: 10.1007/s10833-006-9015-5
Lüdtke, O., Marsh, H. W., Robitzsch, A., and Trautwein, U. (2011). A 2 × 2 times taxonomy of multilevel latent contextual models: accuracy-bias trade-offs in full and partial error correction models. Psychol. Methods 16, 444–467. doi: 10.1037/a0024376
Maier, U. (2010). Accountability policies and teachers’ acceptance and usage of school performance feedback – a comparative study. Sch. Eff. Sch. Improv. 21, 145–165. doi: 10.1080/09243450903354913
Malin, J. R., Brown, C., Ion, G., van Ackeren, I., Bremm, N., Luzmore, R., et al. (2020). World-wide barriers and enablers to achieving evidence-informed practice in education: what can be learnt from Spain, England, the United States, and Germany? Humanit. Soc. Sci. Communicat. 7, 1–14. doi: 10.1057/s41599-020-00587-8
Mandinach, E. B. (2012). A perfect time for data use: using data-driven decision making to inform practice. Educ. Psychol. 47, 71–85. doi: 10.1080/00461520.2012.667064
Mandinach, E. B., and Gummer, E. S. (2016). What does it mean for teachers to be data literate: laying out the skills, knowledge, and dispositions. Teach. Teach. Educ. 60, 366–376. doi: 10.1016/j.tate.2016.07.011
Mandinach, E. B., Honey, M., Light, D., and Brunner, C. (2008). A conceptual framework for data-driven decision making. Data-Driven School Improvement: Linking Data and Learning, 13–31.
Mandinach, E. B., and Schildkamp, K. (2021). Misconceptions about data-based decision making in education: an exploration of the literature. Stud. Educ. Eval. 69:100842. doi: 10.1016/j.stueduc.2020.100842
Mark, M. M., and Henry, G. T. (2004). The mechanisms and outcomes of evaluation influence. Evaluation 10, 35–57. doi: 10.1177/1356389004042326
Marsh, J. A. (2012). Interventions promoting educators’ use of data: research insights and gaps. Teach. Coll. Rec. 114, 1–48. doi: 10.1177/016146811211401106
McCluskey, G., Fry, D., Hamilton, S., King, A., Laurie, M., McAra, L., et al. (2021). School closures, exam cancellations and isolation: the impact of Covid-19 on young people’s mental health. Emot. Behav. Diffic. 26, 46–59. doi: 10.1080/13632752.2021.1903182
McNeish, D., and Stapleton, L. M. (2016). Modeling clustered data with very few clusters. Multivar. Behav. Res. 51, 495–518. doi: 10.1080/00273171.2016.1167008
Merk, S., Groß Ophoff, J., and Kelava, A. (in press). Rich Data – Poor in-Formation? Teacher’s Perceptions of Mean Differences in Graphical Feedback from State-Wide Tests Learning and Instruction.
Mincu, M. (2014). Inquiry Paper 6: Teacher Quality and School Improvement – What is the Role of Research?. Available at: https://www.bera.ac.uk/wp-content/uploads/2014/02/BERA-RSA-Interim-Report.pdf
Mintrop, R., and Zumpe, E. (2019). Solving real-life problems of practice and education leaders’ school improvement mind-set. Am. J. Educ. 125, 295–344. doi: 10.1086/702733
Mintrop, R., Zumpe, E., and Baral, M. (2018). Design-Based School Improvement and Research for Education Leaders. The SAGE Handbook of School Organization. London: Sage Publications.
Muñoz, C., and Valenzuela, J. (2020). Demotivation in academic reading during teacher training. J. Res. Read. 43, 41–56. doi: 10.1111/1467-9817.12288
Neubrand, M., Jordan, A., Krauss, S., Blum, W., and Löwen, K. (2013). “Task analysis in COACTIV: examining the potential for cognitive activation in German mathematics classrooms” in Cognitive Activation in the Mathematics Classroom and Professional Competence of Teachers: Results from the COACTIV Project. eds. M. Kunter, J. Baumert, W. Blum, U. Klusmann, S. Krauss, and M. Neubrand (New York: Springer US), 125–144.
Office for Standards in Education. (2022). School Inspection Handbook. Available at: https://www.gov.uk/government/publications/school-inspection-handbook-eif/school-inspection-handbook#reaching-a-judgement-of-outstanding
Office for Standards in Education (2015). The Annual Report of Her Majesty’s Chief Inspector of Education, Children’s Services and Skills 2014/15, London: HM Government.
Penninckx, M., and Vanhoof, J. (2015). Insights gained by schools and emotional consequences of school inspections. A review of evidence. Sch. Leadersh. Manag. 35, 477–501. doi: 10.1080/13632434.2015.1107036
Perryman, J., Maguire, M., Braun, A., and Ball, S. (2018). Surveillance, Governmentality and moving the goalposts: the influence of Ofsted on the work of schools in a post-panoptic era. Br. J. Educ. Stud. 66, 145–163. doi: 10.1080/00071005.2017.1372560
Prenger, R., and Schildkamp, K. (2018). Data-based decision making for teacher and student learning: a psychological perspective on the role of the teacher. Educ. Psychol. 38, 734–752. doi: 10.1080/01443410.2018.1426834
R Core Team. (2021). R: A Language and Environment for Statistical Computing. Vienna R Foundation for Statistical Computing.
Renkl, A. (2022). Meta-analyses as a privileged information source for informing teachers’ practice? Z. Pädagog. Psychol. 36, 217–231. doi: 10.1024/1010-0652/a000345
Richter, D., Böhme, K., Becker, M., Pant, H. A., and Stanat, P. (2014). Überzeugungen von Lehrkräften zu den Funktionen von Vergleichsarbeiten. Zusammenhänge zu Veränderungen im Unterricht und den Kompetenzen von Schülerinnen und Schülern. Z. Pädagog. Psychol. 60, 225–244. doi: 10.3262/ZP1402225
Rickinson, M., Sharples, J., and Lovell, O. (2020). “Towards a better understanding of quality of evidence use” in Getting Evidence into Education: Evaluating the Routes to Policy and Practice. ed. S. Gorard (Abingdon: Routledge), 219–233.
Rochnia, M., and Gräsel, C. (submitted). Can the utility value of educational sciences be induced based on a reflection example or empirical findings—Or just somehow? 7.
Rose, J., Thomas, S., Zhang, L., Edwards, A., Augero, A., and Rooney, P. (2017). Research learning communities evaluation report and executive summary. Available at: https://educationendowmentfoundation.org.uk/public/files/Projects/Evaluation_Reports/Research_Learning_Communities.pdf
Schildkamp, K. (2019). Data-based decision-making for school improvement: research insights and gaps. Educ. Res. 61, 257–273. doi: 10.1080/00131881.2019.1625716
Schildkamp, K., Handelzalts, A., Poortman, C. L., Leusink, H., Meerdink, M., Smit, M., et al. (2018). The Data Team Procedure: A Systematic Approach to School Improvement, Cham, Switzerland: Springer.
Schildkamp, K., and Kuiper, W. (2010). Data-informed curriculum reform: which data, what purposes, and promoting and hindering factors. Teach. Teach. Educ. 26, 482–496. doi: 10.1016/j.tate.2009.06.007
Schildkamp, K., Lai, M. K., and Earl, L. (2013). Data-Based Decision Making In Education. Challenges and Opportunities. Dordrecht: Springer
Schildkamp, K., and Poortman, C. (2015). Factors influencing the functioning of data teams. Teach. Coll. Rec. 117, 1–42. doi: 10.1177/016146811511700403
Schildkamp, K., Poortman, C., Luyten, H., and Ebbeler, J. (2017). Factors promoting and hindering data-based decision making in schools. Sch. Eff. Sch. Improv. 28, 242–258. doi: 10.1080/09243453.2016.1256901
Schratz, M., Wiesner, C., Rößler, L., Schildkamp, K., George, A. C., Hofbauer, C., et al. (2018). Möglichkeiten und Grenzen evidenzorientierter Schulentwicklung. Nationaler Bildungsbericht Österreich 2, 403–454. doi: 10.17888/nbb2018-2-10
Shulman, L. S. (1987). Knowledge and teaching: foundations of the new reform. Harv. Educ. Rev. 57, 1–23. doi: 10.17763/haer.57.1.j463w79r56455411
Slavin, R. E. (2002). Evidence-Based Education Policies: Transforming Educational Practice and Research. Educational Researcher 31, 15–21. doi: 10.3102/0013189x031007015
Syring, M., Kleinknecht, M., Bohl, T., Kuntze, S., Rehm, M., and Schneider, J. (2015). How problem-based or direct instructional case-based learning environments influence secondary school pre-service teachers’ cognitive load, motivation and emotions: a quasi-experimental intervention study in teacher education. J. Educ. Hum. Dev. 4, 115–129. doi: 10.15640/jehd.v4n4a14
Taysum, A. (2020). An innovative strategy to re-build trust in educational governance systems after 40 years of English destabilising rapid reforms. J. Educat. Administr. History 52, 145–161. doi: 10.1080/00220620.2019.1605341
Teddlie, C., and Tashakkori, A. (2006). A general typology of research designs featuring mixed methods. Res. Sch. 13, 12–28.
Tennent, W. (2021). The assessment of reading comprehension in English primary schools: investigating the validity of the key stage 2 reading standard assessment test (SAT). Education 3–13 49, 481–494. doi: 10.1080/03004279.2020.1735472
UK Government (2022). Levelling up the United Kingdom. Executive Summary. Available at: https://assets.publishing.service.gov.uk/government/uploads/system/uploads/attachment_data/file/1095544/Executive_Summary.pdf
Van Gasse, R., Vanlommel, K., Vanhoof, J., and Van Petegem, P. (2016). Teacher collaboration on the use of pupil learning outcome data: a rich environment for professional learning? Teach. Teach. Educ. 60, 387–397. doi: 10.1016/j.tate.2016.07.004
Van Geel, M., Keuning, T., Visscher, A. J., and Fox, J.-P. (2016). Assessing the effects of a school-wide data-based decision-making intervention on student achievement growth in primary schools. Am. Educ. Res. J. 53, 360–394. doi: 10.3102/0002831216637346
van Geel, M., Keuning, T., Visscher, A., and Fox, J.-P. (2017). Changes in educators’ data literacy during a data-based decision making intervention. Teach. Teach. Educ. 64, 187–198. doi: 10.1016/j.tate.2017.02.015
Vanhoof, J., Vanlommel, K., Thijs, S., and Vanderlocht, H. (2014). Data use by Flemish school principals: impact of attitude, self-efficacy and external expectations. Educ. Stud. 40, 48–62. doi: 10.1080/03055698.2013.830245
Vanlommel, K., Van Gasse, R., Vanhoof, J., and Van Petegem, P. (2017). Teachers’ decision-making: data based or intuition driven? Int. J. Educ. Res. 83, 75–83. doi: 10.1016/j.ijer.2017.02.013
Voss, T. (2022). Not useful to inform teaching practice? Student teachers hold skeptical beliefs about evidence from education science. Front. Educat. 7:976791. doi: 10.3389/feduc.2022.976791
Voss, T., Zeeb, H., Dehmel, A., and Fauth, B. (2020). “Forschungsmethoden in der Lehrerinnen-und Lehrerbildung” in Handbuch Lehrerbildung. eds. C. Cramer, J. König, M. Rothland, and S. Blömeke (Klinkhardt/UTB), 667–672.
Weiss, C. H. (1998). “Improving the use of evaluations: whose job is it anyway?” in Advances in Educational Productivity. Evaluation Research for Educational Productivity. eds. A. J. Reynolds and H. J. Walberg, vol. 7 (Greewich, CT: JAI Press Inc.), 263–276.
Wessels, I., Gess, C., and Deicke, W. (2019). “Competence development through inquiry-based learning” in Inquiry-Based Learning–Undergraduate Research, ed. H. A. Mieg (Berlin: Springer), 59–69.
Wiesner, C., and Schreiner, C. (2019). “Implementation, Transfer, Progression und Transformation: Vom Wandel von Routinen zur Entwicklung von Identität. Von Interventionen zu Innovationen, die bewegen. Bausteine für ein Modell zur Schulentwicklung durch Evidenz (en)” in Praxistransfer Schul-und Unterrichtsentwicklung. eds. C. Schreiner, C. Wiesner, S. Breit, P. Dobbelstein, M. Heinrich, and U. Steffens (Münster: Waxmann), 79–140.
Wurster, S. (2016). Evaluationsgeschützte Schul-und Unterrichtsentwicklung: Eine multiperspektivische Analyse von Vergleichsarbeiten, zentralen Abschlussprüfungen, Schulinspekion und interner Evaluation. Berlin Humboldt-Universität zu Berlin.
Zeuch, N., Förster, N., and Souvignier, E. (2017). Assessing teachers’ competencies to read and interpret graphs from learning progress assessment: results from tests and interviews. Learn. Disabil. Res. Pract. 32, 61–70. doi: 10.1111/ldrp.12126
Keywords: research-informed practice, evidence-informed teacher education, data-based decision-making, organisational learning, trust, school inspection, english education system, pupil performance assessment
Citation: Groß Ophoff J, Brown C and Helm C (2023) Do pupils at research-informed schools actually perform better? Findings from a study at English schools. Front. Educ. 7:1011241. doi: 10.3389/feduc.2022.1011241
Edited by:
Ingo Kollar, University of Augsburg, GermanyReviewed by:
Elisabeth Mayweg-Paus, Humboldt University of Berlin, GermanyRobin Stark, Saarland University, Germany
Copyright © 2023 Groß Ophoff, Brown and Helm. This is an open-access article distributed under the terms of the Creative Commons Attribution License (CC BY). The use, distribution or reproduction in other forums is permitted, provided the original author(s) and the copyright owner(s) are credited and that the original publication in this journal is cited, in accordance with accepted academic practice. No use, distribution or reproduction is permitted which does not comply with these terms.
*Correspondence: Jana Groß Ophoff, jana.grossophoff@ph-vorarlberg.ac.at