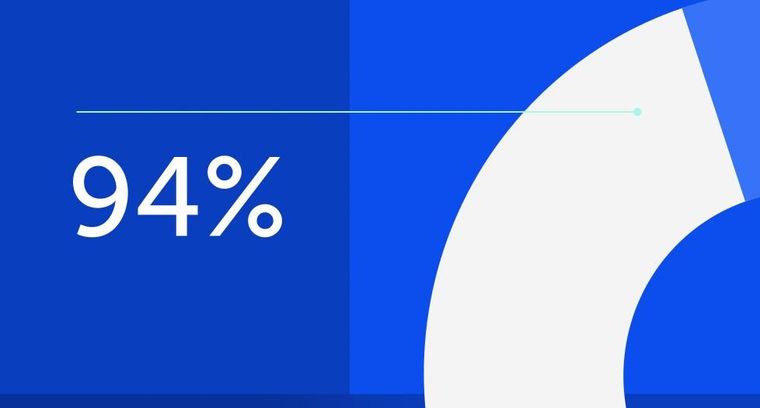
94% of researchers rate our articles as excellent or good
Learn more about the work of our research integrity team to safeguard the quality of each article we publish.
Find out more
ORIGINAL RESEARCH article
Front. Educ., 09 September 2021
Sec. Educational Psychology
Volume 6 - 2021 | https://doi.org/10.3389/feduc.2021.752199
This article is part of the Research Topic“Pathological”/ “Extreme”/ “Rational” Demand-Avoidance: Reviewing and Refining its Contested Terrain Through an Educational PerspectiveView all 4 articles
Students with School Refusal Behaviour (SRB) are a diverse group, often associated with negative academic repercussions (e.g., low academic performance, learning difficulties or academic demotivation). The aims of this research were: 1) to identify school refusal behaviour profiles based on low and high scores on the four functional conditions assessed by the School Refusal Assessment Scale-Revised (SRAS-R) and 2) to examine the relationship between SRB profiles and learning strategies. The SRAS-R and the Inventory of Learning and Study Strategies-High School version were administered to 1,261 students aged to 14 to 18 (M = 16.51; SD = 1.84). Four SRB profiles were obtained: SRB by positive reinforcement, Low SRB, SRB by negative reinforcement and Mixed SRB. School refusers belonging to the Mixed SRB and SRB by negative reinforcement profiles are characterised by low scores on learning strategies, except for the Anxiety dimension. The practical implications of these findings suggest that implementing study techniques and learning strategies programmes, in addition to courses on anxiety management and self-care will help students improve their learning paths and reduce anxiety-based school refusal.
According to the Sustainable Development Goals, in global terms, approximately 5.5 million primary school age students did not attend school in 2018 (United Nations, 2015). Sustainable Development Goal 4 is the education goal. It aims to ensure inclusive and quality education for all and to promote lifelong learning. To achieve this goal, it is necessary to prevent and assist school refusal behaviour. Previous research showed that students who refuse go to school leads to poorer academic performance and may have long-term consequences, such as school failure or early school dropout (Gubbels et al., 2019). On the contrary, the role of learning strategies in gaining academic success has been widely investigated showing that certain strategies are significant predictors of students’ academic success (Credé and Phillips, 2011; Richardson et al., 2012; Yip, 2013). Research on skills students with school attendance problems use to assist them in the learning process is limited and particularly interesting during adolescence. During this evolutionary stage the cognitive development allows self-regulation of their actions, which enables the planning, regulation and evaluation of the activities necessary to achieve meaningful learning and, therefore, better school performance (Steinberg, 2005). In order to know which learning strategies are the most common depending on the groups of adolescents who refuse go to school the present study was carried out.
School Refusal Behaviour (SRB) is a concept that involves child-motivated refusal or a student’s negative attitude regarding school attendance or the consistent difficulty in remaining in class for an entire day (Kearney and Fornander, 2016). This idea has been referred to as School Attendance Problems, or SAPs, which refer to the various categories of school absences or difficulties in attending or remaining in school (e.g., delays, occasional or continuous absences, unexcused consent).
Considering the assortment of each case, an analysis model has been developed including four functional conditions for the emergence and maintenance of SRB: 1) Avoidance of stimuli that provoke negative affectivity, 2) Escape from aversive social and/or evaluative situations, 3) Pursuit of attention from significant others, and 4) Pursuit of tangible reinforcement outside of the school (Kearney, 2002). Negative reinforcement (for example, overcoming stage fright when engaging in public speaking during a presentation in class) is preserved in the first two functional conditions, while the last two functional conditions are based on positive reinforcement (for example, staying at home using social media). These factors can be assessed by the School Refusal Assessment Scale (SRAS) (Kearney and Silverman, 1993) and its revised version (SRAS-R) (Kearney, 2002), which is the most widely used measure to recognize subgroups of students who refuse to attend school.
In line with the previous idea, several studies have attempted to establish profiles of students with SRB. According to the functional model of Kearney and Silverman (1993), four main groups have been distinguished: a Non-school Refusal group with low scores on the four cited factors of the SRAS-R (Gonzálvez et al., 2020), the SRB by negative reinforcement group, characterised by high scores on the first two functional conditions (Delgado et al., 2019), an SRB by positive reinforcement group having high scores on the last two factors of the instrument (Gonzálvez et al., 2018) and a Mixed SRB profile group, characterised by high scores on factors for both positive and negative reinforcement (Gonzálvez et al., 2020). Furthermore, other findings added an additional group that has high scores on all four functional conditions: the High SRB profile (Gonzálvez et al., 2019a). Despite identifying the profiles by cluster analysis (Gonzálvez et al., 2018; Gonzálvez et al., 2019a) or latent class analysis (Delgado et al., 2019; Schreiber, 2017; Su et al., 2019), recent investigation claims that latent profile analyses are a more accurate and sophisticated approach to identify profiles (Tein et al., 2013).
Learning strategies are the procedures for acquiring, organizing, and reconstructing information in the students’ cognitive structure to learn, plan, organize, execute, and evaluate learning activities (Kohler, 2008). Therefore, learning strategies refers to a set of skills that students use to assist them in the learning process. A lack of study habits and strategies may be a factor that encourages school failure (De la Fuente et al., 2008). It is a well-known fact that the use of appropriate learning strategies may help to improve academic results (Seli and Dembo, 2019).
In response to the need to assess skills that are essential for academic success, the Learning and Study Strategies Inventory-High School version (LASSI-HS) was designed (Weinstein, Schulte, and Palmer, 2002). This instrument is made up of 76 items divided in 10 subscales: Attitude (this subscale contains items addressing student attitudes and interests in achieving academic success), Motivation (this scale addresses the student’s self-discipline and disposition to make an effort to work hard), Time management (this scale examines the student’s use of time management principles and practices for academic tasks), Anxiety (this scale measures the degree to which the student worries about school and their academic performance), Concentration (this scale focuses on the student’s ability to direct and maintain their attention on academic tasks), Information processing (this scale measures how well the student can use imagery, verbal elaboration, comprehension monitoring and reasoning, to learn new information and skills); Selection of the main ideas (this scale considers the student’s ability to select important information for further study), Use of study aids (this scale examines the student’s willingness to create or use different academic resources when facing problems related to their performance), Self-assessment (this scale focuses on the student’s ability to review and prepare for classes and tests) and Test strategies (this subscale addresses the student’s use of both test preparation and test-taking strategies).
The conceptual framework of the Model of Strategic Learning underlies this instrument (Weinstein, Acee, and Jung, 2011). Three components (skill, will and self-regulation) are included in this model (Weinstein, Acee, and Jung, 2010). Skill refers to critical knowledge about and knowing how to use learning strategies and other thinking skills. Information Processing, Selecting Main Ideas, and Test Strategies are the LASSI-HS scales primarily related to the skill component. Will refers to the motivation and affective components of strategic learning that either contribute to or detract from academic success. Anxiety, Attitude, and Motivation are the LASSI-HS scales primarily related to the will component. Self-regulation is both the glue and the engine that helps students manage their strategic learning on both a global (using an instrumental approach to help seeking, and managing motivation for learning) and real time levels (focusing attention, maintaining concentration over time). Concentration, Self-Testing, Study Aids, and Time Management are the LASSI-HS scales primarily related to this category.
In this study the LASSI-HS was selected because is one of the primary instruments used to assess students’ strategic and self-regulated learning because targets not only students who want to improve their learning skills, but also students with a history of low academic achievement, students who are poorly motivated in school, as well as for making a successful transition into a college setting. The LASSI-HS provides important information for students, instructors, and researchers in an educational environment and offers a self-scored form or a computer-scored form (Weinstein and Palmer, 1990). Prior studies carried out with Spanish (Inglés et al., 2013), Norwegian (Samuelstuen, 2003), or North American (Olivárez and Tallent-Runnels, 1994) have shown the suitability and applicability of this instrument in adolescent population.
Previous studies have affirmed that a relationship exists between SRB and some of the dimensions evaluated by the LASSI-HS. Students with SRB are more likely to have emotional difficulties such as anxiety (Arribathi et al., 2021; Havik et al., 2015; Tekin et al., 2018), which is a normal human reaction to stressful situations (Fenton et al., 2020), and to real or imaginary threats, called phobias, caused by uncertainty (Namora, 2011); and depression (Nayak et al., 2018), which may be related to boredom, frustration, burnout apropos of school environment or academic performance (Fiorilli et al., 2017). A lack of motivation may also be a key cause of abandoning school (Kearney and Fornander, 2016), since studies have suggested that high achievers have high motivation, in other words, hard-working students who get better grades are highly motivated to go to school (Kurtz-Costes and Schneider, 1994; Meneguetti and De Beni, 2010). Many school refusers experience internalizing problems, which include social anxiety which is reflected by fear, fatigue and somatic complaints (e.g., stomach aches, nausea, tremors and headaches); and externalizing problems such as disobedience of family and teacher requests, aggression, temper tantrums (crying and screaming), running away from school or home and clinging (Kearney, 2001; Setzer and Salzhauer, 2001). SRB may disrupt the growth of positive self-perception and healthy social interactions of the refusers. This would ultimately lead to withdrawal from friends, rejection by peers and scholastic failure (Tekin et al., 2018; Rukmani and Hari, 2019).
All learning strategy dimensions evaluated by the LASSI-HS have never been analysed with regard to school refusal behavior. However, other studies with Spanish adolescents have examined the relationship between learning strategies and prosocial behaviour. Inglés et al. (2013) found that both boys and girls in secondary school having high levels of prosocial behaviour are related to high scores on different learning strategies. Thus, these students are more likely to have a positive attitude towards studying, higher academic motivation and better information processing, such as selection of the main ideas of a text, study aids, self-evaluation and test preparation. According to (Etxebarría et al., 2003), students with prosocial behaviour are characterized by more rational information processing, based on cooperative and significant learning. As (Duschesne et al., 2010) suggested, these teenagers are less likely to experience anxiety or be worried about school and school performance. These results confirm the relationship between emotional stability and prosocial behaviour.
There is a need for a better understanding of the various factors that contribute to school non-attendance. Regarding the learning process, academic difficulties, dissatisfaction towards teachers’ methodology, or emotional distress arising from learning difficulties can contribute to the emergence of refusal to go to school (Filippello et al., 2019; Ingul et al., 2019), so it is of interest to know the ways in which students with school attendance problems learn, remember information, and study. Regarding the several variables assessed by the LASSI-HS, which may be highly relevant in order to better understand school refusal behaviour, this study attempts to determine the following:
1. To identify SRB profiles based on low and high scores on the four functional conditions assessed by the SRAS-R. According to prior research, it is expected that the most common profiles will be distinguished: Non-SRB, SRB by positive reinforcement, SRB by negative reinforcement and SRB by mixed reinforcements (Gonzálvez et al., 2018; Delgado et al., 2019).
2. To examine the relationship between SRB profiles and learning strategies. It is expected that students of the Mixed SRB and the SRB by negative reinforcement profiles will use fewer learning strategies than those of the Non-SRB or the SRB by positive reinforcement profiles, except on the Anxiety dimension, where these profiles will receive higher scores (Ollendick et al., 1990; Rubin and Coplan, 2004; Ingul and Nordahl, 2013).
A non-clinical sample was selected by random cluster sampling with the geographical areas of the Spanish provinces of Alicante and Murcia as the first level (central, north, south, east, and west), high schools as the second level randomly and proportionally selected in each geographic area with a total of 15 high schools from urban areas of Alicante and Murcia both public and private, and classrooms as the third level. The initial number of participants recruited was 1,334, of which 22 (1.6%) were excluded due to errors or omissions in their responses and 51 (3.8%) because their parents’ consent to participate in the study was not obtained. Consequently, the final sample consisted of 1,261 participants aged to 14 to 18 (M = 16.51; SD = 1.84). The majority of students came from urban areas (84.7%). Regarding the socioeconomic level of the families, 23% had a medium–low level, 64% a medium level, and 13% a medium–high level, determined from family income and parents’ education level.
The School Refusal Assessment Scale-Revised (SRAS-R, Kearney, 2002). The SRAS-R is a self-reporting measure that evaluates four functions of the SRB. It is formed by 24 items and a 7-point response scale (0 = Never; 6 = Always). In this specific study, the Spanish version of SRAS-R (Gonzálvez et al., 2016), made up of 18 items, was used. It measures the four functional conditions to maintain SRB: 1) Avoidance of school-related stimuli which provoke a sense of general Negative Affectivity (α=.64, “How often do you stay away from school since you will feel sad or depressed if you go?”), 2) Escape from aversive social and/or evaluative situations at school (α=.73, “How often do you stay away from places in school (e.g., hallways, places where specific groups of people are/where you would have to talk to somebody?”), 3) Pursuit of attention from significant others (α=.78, “How much would you rather be with your family than go to school?”) and 4) Pursuit of tangible reinforcement outside of the school setting (α=.56, “When you are not in school during the week (Monday to Friday), how often do you leave the house and do something enjoyable?”). The coefficients of internal consistency in this study were 0.72, 0.75, 0.71, and 0.69, respectively, for each of the four factors. Prior studies carried out with Spanish (Gonzálvez et al., 2021), German (Walter et al., 2017) and North American (Haight et al., 2011) have shown the suitability and applicability of this instrument in adolescent population.
Inventory of Learning and Study Strategies-High School version (LASSI-HS; Weinstein and Palmer, 1990). This instrument is the result of the adaptation of the original version of the LASSI (Weinstein, 1987) to the adolescent population. It is used to evaluate the required skills to achieve success in the academic field. It consists of 76 items assessed on a 5-point Likert-type scale (1 = Not at all like me; 5 = Very much like me) and assembled into 10 dimensions: Attitude (α=.74; “Do students only study for the courses they like?”; Motivation (α=.78, “Do they easily “give up” in difficult classes?”), Time management (α=.77, “Do students procrastinate about completing academic tasks?”), Anxiety (α=.82, “Do students worry so much when they are well-prepared?”), Concentration (α=.82, “Are students easily distracted?”), Information processing (α=.80, “Do students try to summarize or paraphrase their class reading assignments?”), Selection of the main ideas (α=.71, “Can students identify the key points in a lecture?”), Use of study aids (α=.68, “Do students avoid going for help?”, Self-assessment (α=.74 “Do they stop periodically while reading to review the content?”), and Test strategies (α=.81 “Do students know how to study for tests in different types of courses?”). The coefficients of internal consistency in this study were as follows: Attitude (α=.72), Motivation (α=.75), Time management (α=.71), Anxiety (α=.80), Concentration (α=.78), Information processing (α=.79), Selection of the main ideas (α=.73), Use of study aids (α=.76), Self-assessment (α=.79) and Test strategies (α=.79).
First, a meeting was arranged with the schools’ principals to introduce the study objectives and to request their collaboration. Written informed consent was delivered to the families, following their acceptance. Students participated voluntarily and completed the instruments during a school session lasting 45 min. At least one member of the research team was present during the sessions to resolve any doubts and to supervise the completion of the questionnaires. This study was carried out according to the ethical standards of the Declaration of Helsinki (World Medical Association, 1998) and the procedures were approved by the Ethics Committee of the University of Alicante (UA-2017-09-05).
To identify the different groups of students with SRB, the Latent Profile Analysis was employed. A series of Latent Profile Analyses were applied to determine the most adequate class solution. The Bayesian Information Criteria (BIC) and the Akaike Information Criterion (AIC) were used as goodness-of-fit measures The model with the lowest BIC and AIC values was considered appropriate. Furthermore, the Vuong-Lo-Mendell-Rubin likelihood-ratio test and the bootstrap likelihood-ratio test were also employed, taking into consideration that in both of them a p-value below 0.05 implies that the estimated k-class model is better than the (k-1)-class model, which is henceforth excluded, all for a model with at least k classes. Likewise, entropy was used as a criterion of the quality of class membership classification, with a score closer to one being desirable. The size of the classes should also be considered when determining the best model. With that in mind, classes should make up at least 1% of the sample.
A multivariate analysis of variance (MANOVA) was performed to assess differences in the mean scores of learning strategies between the known SRB profiles. In addition, post hoc tests (Scheffé’s method) were performed and the effect size was determined using the d index. This index was evaluated in accordance with Cohen’s interpretation 1988) and has been interpreted as follows: values between 0.20 and 0.49 indicate a low effect size; those between 0.50 and 0.79 indicate a moderate effect size and values above 0.80 indicate a high effect size. Analyses were performed using the SPSS 24 statistical package and Mplus version 8.
The fit indices of the five assessed models including the AIC, the BIC and adjusted BIC, the Vuong-Lo-Mendell-Rubin likelihood ratio test, the Bootstrap likelihood ratio test, the entropy information and the size index for the number of classes that do not reach at least 1% of the sample are presented in Table 1. The lowest BIC and AIC scores were obtained by the six and seven class models. These classes were rejected, however, since the most restrictive criterion, the Vuong-Lo-Mendell-Rubin likelihood-ratio test, had a p > 0.05. The model made up of five classes, although the BIC and AIC had low scores and a p value <0 .05, was rejected since the size index was higher than 0. Combining all of the criteria, the fourth model was carefully chosen as the best fitting model with p < 0.05 for the Vuong-Lo-Mendell-Rubin likelihood-ratio test and the Bootstrap likelihood ratio test and having the lowest index in AIC and BIC, in contrast with the other models.
Figure 1 shows the four classes that were identified between 1,261 participants. Profile 1 (149 participants, 11.82% of the sample) is called SRB by Positive Reinforcement and is characterized by low scores on the first and second factor and high scores on the third and fourth factor of the SRAS-R); Profile 2 (808 participants, 64.08% of the sample) is called Low SRB, characterized by low scores on all four factors of the SRAS-R; Profile 3 (200 participants, 15.86% of the sample) is called SRB by Negative Reinforcement, with high scores on the first factor and a high tendency in the second factor of the SRAS-R); and Profile 4 (104 participants, 8.25% of the sample) is called Mixed SRB, with high scores on the first three factors of the SRAS-R.
The MANOVA results indicate that there are significant differences between all of the variables (Wilks’ lambda = 0.798, F(30,1257) = 9.79; p < 0.001, np2 = 0.07). The Low SRB profile obtained the highest means scores on all of the learning strategy dimensions except for Anxiety, Information processing and Study aids (see Table 2). It also had the lowest means on the Anxiety dimension. On the contrary, the Mixed SRB profile obtained the lowest mean scores on most of the dimensions, except for Anxiety, Use of study aids and Self-assessment strategies. Non-significant differences were found between the following learning strategy dimensions when comparing the scores on the different SRB profiles: Information processing, Use of study aids and Self-assessment, since the p value is greater than 0.5.
TABLE 2. Means and standard deviations obtained by the four profiles of school refusal behaviour in LASSI-HS dimensions.
Table 3 shows the post hoc tests that had statistically significant differences among the profiles. Large size differences were identified between the Low SRB and the Mixed SRB profiles on Attitude, Selection of the main Ideas and Test strategies. As for moderate effect size differences, these were found for the Attitude dimension between the SRB by positive reinforcement and Mixed SRB profiles, the Low SRB and the SRB by negative reinforcement profiles and the SRB by negative reinforcement and the Mixed SRB profiles. In terms of Time management and Concentration, moderate effect size differences also appeared between Low SRB and Mixed SRB. Finally, small size differences were found between SRB by positive reinforcement and Low SRB on the Anxiety dimension; and between SRB by positive reinforcement and Mixed SRB on the Selection of the main ideas dimension. Insignificant size differences also exist between Low SRB and SRB by negative reinforcement on Motivation, Time management, Anxiety, Concentration, Selection of the main ideas and Test strategies. Low SRB and Mixed SRB profiles present slight size differences in Motivation. As for SRB by negative reinforcement and Mixed SRB, minor size differences appear for Time Management and Concentration.
The aim of this study was twofold: first, to identify the SRB profiles based on a sample of 1,261 Spanish students; and second, to examine the relationship between the identified SRB profiles and learning strategies.
As stated in the first hypothesis, four SRB profiles (Non-SRB, SRB by positive reinforcement, SRB by negative reinforcement and SRB by mixed reinforcements) were expected to be found. According to our findings, this hypothesis has been partially confirmed. Three of the profiles (SRB by positive reinforcement, SRB by negative reinforcement and Mixed SRB) agreed with the initial hypothesis. Nevertheless, a profile that was not proposed in the first hypothesis was found, but it does not have high scores on all SRAS-R factors, although it has a negative tendency. This profile has been identified in previous research (Gonzálvez et al., 2018; Delgado et al., 2019; Gonzálvez et al., 2020), and it was called Low SRB, sharing some similarities with the Non-SRB profile. It is important to note that the Low SRB profile attained the highest representation between the identified profiles. More than 60% of the total sample, specifically 64.08%, belongs to the Low SRB profile. This may be explained by the characteristics of the participants since this study was made up of a community student sample, most of whom regularly attend school.
Regarding the second hypothesis, it was expected that students belonging to the Mixed SRB or the SRB by negative reinforcement group use fewer learning strategies than the Non-SRB or the SRB by positive reinforcement profiles, except for the Anxiety dimension where these profiles obtain higher scores. Based on our findings, this hypothesis has been confirmed. Results indicated that adolescents belonging to Mixed SRB or SRB by negative reinforcement profiles use fewer learning strategies than adolescents belonging to the Non-SRB or SRB by positive reinforcement profiles. Specifically, students in the Mixed SRB profile received the lowest scores on most LASSI-HS dimensions, except for Anxiety, which was the second highest score below SRB by negative reinforcement. These results are in line with those obtained in other profile studies where youth who belong to the Mixed SRB or the SRB by negative reinforcement profiles revealed high levels in different types of anxiety: school anxiety (Gonzálvez et al., 2020), social anxiety (Gonzálvez et al., 2019b) and general anxiety (Bools et al., 1990; Dube and Orpinas, 2009). According to the results obtained by Broc-(Cavero, 2012) and (Berenhaum et al., 2003), the fewer the learning strategies implemented, the higher the failure rate, creating more anxiety and consequently, difficulty in implementing learning strategies.
Amongst the four latent groups found, the Low SRB profile received the highest scores on the evaluated LASSI-HS dimensions (Attitude, Motivation, Time management, Concentration, Selection of main ideas and Test strategies) and the lowest score on the Anxiety dimension. This learners’ profile was less likely to suffer from anxiety during the teaching-learning process. The present results showed the groups of high school students who belongs to the Low SRB and the SRB by Positive Reinforcement profiles did score higher than the other profiles in different areas of the strategic learning model. It obviously implies that the better learning and study strategies the high school students use, the less anxiety-based school refusal have. Along this line, the profiles of Mixed SRB and SRB by negative reinforcement are the most maladaptive ones, revealing greater difficulties in the learning strategy levels.
Large size differences were detected between the Low SRB and the Mixed SRB profiles on the LASSI-HS dimensions of Attitude, Selection of the main Ideas and Test strategies. This implies that groups with high scores on SRB for positive and negative reinforcement all at once are at a greater risk of not having a proper attitude and may be uninterested in the academic field, facing complications when distinguishing between important information for further study and less important and supporting details, and being unaware of how to handle test preparation and test-taking strategies, which may infer with their academic achievement and consequently, lead to school attendance problems.
In line with Oroujlou and Vahedi (2011), it has been assumed that wanting to study requires prior motivation, a positive attitude and knowledge of how to study, using appropriate learning strategies to achieve efficient results. It should be noted that the teaching-learning process should be more effective given the rapid changes in the educational system. Therefore, an active role is being taken by the learner, as compared to their previous passive role in the traditional education approach. Improving their creativity, thinking skills, learning strategies and developing teamwork skills will help students to increase their confidence for examinations and real-world problems (Saravanan et al., 2021). Previous research shows that students perform better on those skills that they value with a positive attitude (Liddell and Davidson, 2004) or when they have a special preparation in the methods of responding to exam questions (Biçak, 2013).
According to Garcia and Pintrich, 1992, being taught how to use learning strategies can impact student attention to specific stimuli, so that they will select, organize and acquire new information. The appropriate selection of study techniques is connected with student abilities, motivations, achievement expectations, etc. Pardo (2020) affirms that a combination of some of these is generally recommended since it offers further assurance of the learning consolidation and enables students to assess their form of study organization. According to (Arias Viteri and Macías Santos, 2019), participation by the entire school community is one of the key points for the development of the best academic and human potential of students, since teachers are not alone in helping students learn new study techniques, with peers also participating.
There are several limitations of this study that should be considered for upcoming research. A larger and more diverse sample should be used, considering other variables such as nationality, socioeconomic status, health, psychological data and differences between schools. In future surveys, different data collection sources (interviews, questionnaires, observations, personal information) may be included, taking into account family, teacher and classmate opinions. Finally, it would be interesting for future studies to analyse these variables in clinic samples. Despite these limitations, the results of this work are beneficial for future research and contribute to our knowledge of learning strategies and their relationship with SRB. According to our findings, a more cautious approach is necessary to reach firmer conclusions, since these items may be affected by other variables such as academic failure, the teachers’ classroom management, poor relationships with partners and educators or eventual dropout (Carroll, 2011; Havik et al., 2015).
In our current educational system, study techniques and learning strategies programmes are highly recommended in order to educate students so as to use them in their learning paths. The lack of these techniques leads to unsatisfactory results in the learning processes of some students (Bedolla, 2018). Courses on anxiety management and self-care programmes are also clearly needed to improve the studying process of students and to prevent school attendance problems. Anxiety during school years is one of the most common issues of emotional stress and high levels of anxiety reduce the efficiency of the learning process, diminishing the attention, concentration and retention of knowledge which may provoke a decline of the academic performance (Gutiérrez et al., 2019). In addition, create a warm, safe and supportive school environment where educational inclusive practices are implemented is essential for student well-being and achievement (Filippello et al., 2019; Bacon and Kearney, 2020).
The raw data supporting the conclusions of this article will be made available by the authors, without undue reservation.
The studies involving human participants were reviewed and approved by the Ethics Committee of the University of Alicante (UA-2017-09-05). Written informed consent to participate in this study was provided by the participants’ legal guardian/next of kin.
Conceptualization, MG-M, CG, and MV; methodology, RS and MA-F.; formal analysis, RS and JG-F; investigation, MG-M, CG and MV; writing—original draft preparation, MG-M and CG; writing-review and editing, CG, MV and MA-F; Supervision, JG-F; Funding, JG-F and CG. All the authors contributed equally to the research design, data analysis, and revision, and approved the final manuscript.
This research was funded by the Ministry of Science, Innovation and Universities and Fondos FEDER with the grant number RTI 2018-098197-B-I00 awarded to JG-F and the Project GV/2019/075 awarded to CG
The authors declare that the research was conducted in the absence of any commercial or financial relationships that could be construed as a potential conflict of interest.
All claims expressed in this article are solely those of the authors and do not necessarily represent those of their affiliated organizations, or those of the publisher, the editors, and the reviewers. Any product that may be evaluated in this article, or claim that may be made by its manufacturer, is not guaranteed or endorsed by the publisher.
Arias Viteri, I. G., and Macías-Santos, J. E. (2019). Técnicas de estudio en la calidad de rendimiento escolar. Diseño de aplicación móvil. Ecuador: Universidad de Guayaquil.
Arribathi, A. H., Suwarto Miftakhu Rosyad, A., Miftakhu Rosyad, A., Budiarto, M., Supriyanti, D., and Mulyati, fnm. (2021). An Analysis of Student Learning Anxiety during the COVID-19 Pandemic: A Study in Higher Education. J. Cont. Higher Edu., 1–14. doi:10.1080/07377363.2020.1847971
Bacon, V. R., and Kearney, C. A. (2020). School Climate and Student-Based Contextual Learning Factors as Predictors of School Absenteeism Severity at Multiple Levels via CHAID Analysis. Child. Youth Serv. Rev. 118, 105452–105459. doi:10.1016/j.childyouth.2020.105452
Berenbaum, H., Raghavan, C., Le, H.-N., Vernon, L. L., and Gomez, J. J. (2003). A Taxonomy of Emotional Disturbances. Clin. Psychol. Sci. Pract. 10 (2), 206–226. doi:10.1093/clipsy/bpg01110.1093/clipsy.bpg011
Biçak, B. (2013). Scale for Test Preparation and Test Taking Strategies. Educ. Sci. Theor. Pract. 13 (1), 279–289.
Bools, C., Foster, J., Brown, I., and Berg, I. (1990). The Identification of Psychiatric Disorders in Children Who Fail to Attend School: A Cluster Analysis of a Non-clinical Population. Psychol. Med. 20, 171–181. doi:10.1017/s0033291700013350
Broc Cavero, M. Á. (2012). Influencia relativa de variables metacognitivas y volitivas en el rendimiento académico de estudiantes de Bachillerato (LOE) = Relative influence of metacognitive and volitive variables in the academic achievement of baccalaureate students. Reop 23 (3), 63–80. doi:10.5944/reop.vol.23.num.3.2012.11462
Carroll, H. C. M. (2011). The Peer Relationships of Primary School Pupils with Poor Attendance Records. Educ. Stud. 37, 197–206. doi:10.1080/03055698.2010.510240
Credé, M., and Phillips, L. A. (2011). A Meta-Analytic Review of the Motivated Strategies for Learning Questionnaire. Learn. Individual Differences 21, 337–346. doi:10.1016/j.lindif.2011.03.002
De la Fuente, J., Pichardo, M., Justicia, F., and Berbén, A. (2008). Enfoques de aprendizaje, autorregulación y rendimiento académico en tres universidades europeas. Psicothema 20 (4), 705–711.
Delgado, B., Martinez-Monteagudo, M. C., Ruiz-Esteban, C., and Rubio, E. (2019). Latent Class Analysis of School Refusal Behavior and its Relationship with Cyberbullying during Adolescence. Front. Psychol. 10, 1916. doi:10.3389/fpsyg.2019.01916
Dube, S. R., and Orpinas, P. (2009). Understanding Excessive School Absenteeism as School Refusal Behavior. Child. Schools 31, 87–95. doi:10.1093/cs/31.2.87
Duchesne, S., Larose, S., Vitaro, F., and Tremblay, R. E. (2010). Trajectories of Anxiety in a Population Sample of Children: Clarifying the Role of Children's Behavioral Characteristics and Maternal Parenting. Dev. Psychopathol. 22 (2), 361–373. doi:10.1017/s0954579410000118
Etxebarria, I., Apodaca, P., Eceiza, A., Fuentes, M. J., and Ortiz, M. J. (2003). Diferencias de género en emociones y en conducta social en la edad escolar. Infancia y Aprendizaje 26 (2), 147–161. doi:10.1174/021037003321827759
Filippello, P., Buzzai, C., Costa, S., and Sorrenti, L. (2019). School Refusal and Absenteeism: Perception of Teacher Behaviors, Psychological Basic Needs, and Academic Achievement. Front. Psychol. 10, 1471. doi:10.3389/fpsyg.2019.0147146
Fiorilli, C., De Stasio, S., Di Chiacchio, C., Pepe, A., and Salmela-Aro, K. (2017). School Burnout, Depressive Symptoms and Engagement: Their Combined Effect on Student Achievement. Int. J. Educ. Res. 84, 1–12. doi:10.1016/j.ijer.2017.04.001
Garcia, T., and Pintrich, P. R. (1992). Critical Thinking and its Relationship to Motivation, Learning Strategies, and Classroom Experience. In Off. Educ. Res. Improvement.
Gonzálvez, C., Kearney, C. A., Jiménez-Ayala, C. E., Sanmartín, R., Vicent, M., Inglés, C. J., et al. (2018). Functional Profiles of School Refusal Behavior and Their Relationship with Depression, Anxiety, and Stress. Psychiatry Res. 269, 140–144. doi:10.1016/j.psychres.2018.08.069
Gonzálvez, C., Díaz-Herrero, Á., Sanmartín, R., Vicent, M., Fernández-Sogorb, A., and García-Fernández, J. M. (2020). Testing the Functional Profiles of School Refusal Behavior and Clarifying Their Relationship with School Anxiety. Front. Public Health 8, 598915. doi:10.3389/fpubh.2020.598915
Gonzálvez, C., Díaz-Herrero, Á., Sanmartín, R., Vicent, M., Pérez-Sánchez, A. M., and García-Fernández, J. M. (2019). Identifying Risk Profiles of School Refusal Behavior: Differences in Social Anxiety and Family Functioning Among Spanish Adolescents. Ijerph 16, 3731. doi:10.3390/ijerph16193731
Gonzálvez, C., Giménez, M., Vicent, M., Sanmartín, R., Quiles, M. J., and García-Fernández, J. M. (2021). School Refusal Behaviour Profiles and Academic Self-Attributions in Language and Literature. Sustainability 13 (13), 7512. doi:10.3390/su13137512
Gonzálvez, C., Inglés, C. J., Kearney, C. A., Sanmartín, R., Vicent, M., and García-Fernández, J. M. (2019a). Relationship between School Refusal Behavior and Social Functioning: a Cluster Analysis Approach. Ejep 12 (1), 17–29. doi:10.30552/ejep.v12i1.238
Gonzálvez, C., Inglés, C. J., Kearney, C. A., Vicent, M., Sanmartín, R., and García-Fernández, J. M. (2016). School Refusal Assessment Scale-Revised: Factorial Invariance and Latent Means Differences across Gender and Age in Spanish Children. Front. Psychol. 7, 2011. doi:10.1177/073428291771217310.3389/fpsyg.2016.02011
Gubbels, J., van der Put, C. E., and Assink, M. (2019). Risk Factors for School Absenteeism and Dropout: a Meta-Analytic Review. J. Youth Adolesc. 48 (9), 1637–1667. doi:10.1007/s10964-019-01072-5
Gutiérrez, S., Samaniego, I., and López, L. (2019). Efectos del programa mathfulness basado en técnicas cognitivo-conductuales sobre la atención, la ansiedad y el rendimiento en matemáticas, en un grupo de estudiantes de la Escuela primer ciclo Santa Librada. Conducta Científica 2 (1), 48–62.
Haight, C., Kearney, C. A., Hendron, M., and Schafer, R. (2011). Confirmatory Analyses of the School Refusal Assessment Scale-Revised: Replication and Extension to a Truancy Sample. J. Psychopathol. Behav. Assess. 33 (2), 196–204. doi:10.1007/s10862-011-9218-9
Havik, T., Bru, E., and Ertesvåg, S. K. (2015). Assessing Reasons for School Non-attendance. Scand. J. Educ. Res. 59 (3), 316–336. doi:10.1080/00313831.2014.904424
Havik, T., Bru, E., and Ertesvåg, S. K. (2015). School Factors Associated with School Refusal- and Truancy-Related Reasons for School Non-attendance. Soc. Psychol. Educ. 18, 221–240. doi:10.1007/s11218-015-9293-y
Inglés, C. J., Martínez-González, A. E., and García-Fernández, J. M. (2013). Conducta prosocial y estrategias de aprendizaje en una muestra de estudiantes españoles de Educación Secundaria Obligatoria. Ejep 6 (1), 33–53. doi:10.30552/ejep.v6i1.88
Ingul, J. M., and Nordahl, H. M. (2013). Anxiety as a Risk Factor for School Absenteeism: what Differentiates Anxious School Attenders from Non-attenders?. Ann. Gen. Psychiatry 12 (1), 25–29. doi:10.1186/1744-859x-12-25
Ingul, J. M., Havik, T., and Heyne, D. (2019). Emerging School Refusal: A School-Based Framework for Identifying Early Signs and Risk Factors. Cogn. Behav. Pract. 26 (1), 46–62. doi:10.1016/j.cbpra.2018.03.005
Kearney, C. A., and Fornander, M. J. (2016). “School Refusal Behavior and Absenteeism,” in Encyclopedia of Adolescence. Editor R. Levesque (Cham: Springer), 1–6. doi:10.1007/978-3-319-32132-5_236-2
Kearney, C. A. (2002). Identifying the Function of School Refusal Behavior: a Revision of the School Refusal Assessment Scale. J. Psychopathol. Behav. Assess. 24 (4), 235–245. doi:10.1023/A:1020774932043
Kearney, C. A. (2001). School Refusal Behavior in Youth: A Functional Approach to Assessment and Treatment. Washington, DC: American Psychological Association. doi:10.1037/10426-000
Kearney, C. A., and Silverman, W. K. (1993). Measuring the Function of School Refusal Behavior: the School Refusal Assessment Scale. J. Clin. Child Psychol. 22 (1), 85–96. doi:10.1207/s15374424jccp2201_9
Kohler, J. (2008). Estrategias de aprendizaje y rendimiento académico en estudiantes de psicología de 1ro al 4to ciclo de psicología de una universidad particular. Revista Cultura 22, 331–362.
Liddell, M. J., and Davidson, S. K. (2004). Student Attitudes and Their Academic Performance: Is There Any Relationship?. Med. Teach. 26 (1), 52–56. doi:10.1080/01421590310001642993
Nayak, A., Sangoi, B., and Nachane, H. (2018). School Refusal Behavior in Indian Children: Analysis of Clinical Profile, Psychopathology and Development of a Best-Fit Risk Assessment Model. Indian J. Pediatr. 85, 1073–1078. doi:10.1007/s12098-018-2631-2
Olivárez, A., and Tallent-Runnels, M. K. (1994). Psychometric Properties of the Learning and Study Strategies Inventory-High School Version. J. Exp. Edu. 62 (3), 243–257. doi:10.1080/00220973.1994.9943843
Ollendick, T. H., Greene, R. W., Weist, M. D., and Oswald, D. P. (1990). The Predictive Validity of Teacher Nominations: A Five-Year Followup of At-Risk Youth. J. Abnorm. Child. Psychol. 18 (6), 699–713. doi:10.1007/bf01342755
Oroujlou, N., and Vahedi, M. (2011). Motivation, Attitude, and Language Learning. Proced. - Soc. Behav. Sci. 29, 994–1000. doi:10.1016/j.sbspro.2011.11.333
Richardson, M., Abraham, C., and Bond, R. (2012). Psychological Correlates of university Students' Academic Performance: A Systematic Review and Meta-Analysis. Psychol. Bull. 138, 353–387. doi:10.1037/a0026838
Rubin, K. H., and Coplan, R. J. (2004). Paying Attention to and Not Neglecting Social Withdrawal and Social Isolation. Merrill-Palmer Q. 50, 506–534. doi:10.1353/mpq.2004.0036
Rukmani, B., and Hari, A. (2019). Factors Associated with School Refusal Behaviour in Primary School Students. Mjssh 3 (1), 1–13. doi:10.33306/mjssh0210.33306/2590-3691/2019
Samuelstuen, M. S. (2003). Psychometric Properties and Item-Keying Direction Effects for the Learning and Study Strategies Inventory-High School Version with Norwegian Students. Educ. Psychol. Meas. 63 (3), 430–445. doi:10.1177/0013164403063003006
Saravanan, S., Mahesh, V., Kumar, D. G., and Kshatri, S. S. (2021). Improving Student's Learning with Efficient Learning Techniques: A Case Study of First Year Basic Electrical Engineering Course. Mater. Today Proc. doi:10.1016/j.matpr.2021.02.784
Schreiber, J. B. (2017). Latent Class Analysis: an Example for Reporting Results. Res. Soc. Adm Pharm 13 (6), 1196–1201. doi:10.1016/j.sapharm.2016.11.011
Seli, H., and Dembo, M. H. (2019). Motivation and Learning Strategies for College success: A Focus on Self-Regulated Learning. New York: Routledge. doi:10.4324/9781315724775
Setzer, N., and Salzhauer, A. (2001). Understanding School Refusal. New York: New York University Child Study Center.
Steinberg, L. (2005). Cognitive and Affective Development in Adolescence. Trends Cogn. Sci. 9, 69–74. doi:10.1016/j.tics.2004.12.005
Su, A., He, W., and Huang, T. (2019). Sociocultural Adaptation Profiles of Ethnic Minority Senior High School Students in mainland China: A Latent Class Analysis. Sustainability 11 (24), 6942. doi:10.3390/su11246942
Tein, J. Y., Coxe, S., and Cham, H. (2013). Statistical Power to Detect the Correct Number of Classes in Latent Profile Analysis. Struct. Equ. Model. 20, 640–657. doi:10.1080/10705511.2013.824781
Tekin, I., Erden, S., Şirin Ayva, A. B., and Büyüköksüz, E. (2018). The Predictors of School Refusal: Depression, Anxiety, Cognitive Distortion and Attachment. HumanSciences 15 (3), 1519–1529. doi:10.14687/jhs.v15i3.5084
United Nations (2015). Transforming Our World: The 2030 Agenda for Sustainable Development Resolution Adopted by the General Assembly on 25 September 2015. Available at: https://www.un.org/en/development/desa/population/migration/generalassembly/docs/globalcompact/A_RES_70_1_E.pdf.
Walter, D., von Bialy, J., von Wirth, E., and Doepfner, M. (2017). Psychometric Properties of the German School Refusal Assessment Scale-Revised. J. Psychoeducational Assess. 36, 644–648. doi:10.1177/0734282916689641
Weinstein, C. E., Acee, T. W., and Jung, J. (2011). Self-regulation and Learning Strategies, 2011. Wiley Online Library, 45–53. doi:10.1002/tl.443 Self-regulation and Learning Strategies New Dir. Teach. Learn.
Weinstein, C. E., Jung, J., and Acee, T. W. (2010). “Learning Strategies,” in International Encyclopedia of Education. Editors B. McGaw, P. L. Peterson, and E. Baker. 3rd ed. (New York: Elsevier), 323–329. doi:10.1016/b978-0-08-044894-7.00497-8
Weinstein, C. E. (1987). LASSI. (Learning and Study Strategies Inventory). Clearwater: H&H Publishing Company.
Keywords: school refusal behavior, learning strategies, SRAS-R, LASSI-HS, adolescence
Citation: Giménez-Miralles M, Gonzálvez C, Sanmartín R, Vicent M, Aparicio-Flores MdP and García-Fernández JM (2021) Classifying Students With School Refusal Behavior and Their Relationship to Learning Strategies. Front. Educ. 6:752199. doi: 10.3389/feduc.2021.752199
Received: 02 August 2021; Accepted: 30 August 2021;
Published: 09 September 2021.
Edited by:
Min-Hsien Lee, National Taiwan Normal University, TaiwanReviewed by:
Tzung-Jin Lin, National Taiwan Normal University, TaiwanCopyright © 2021 Giménez-Miralles, Gonzálvez, Sanmartín, Vicent, Aparicio-Flores and García-Fernández. This is an open-access article distributed under the terms of the Creative Commons Attribution License (CC BY). The use, distribution or reproduction in other forums is permitted, provided the original author(s) and the copyright owner(s) are credited and that the original publication in this journal is cited, in accordance with accepted academic practice. No use, distribution or reproduction is permitted which does not comply with these terms.
*Correspondence: Ricardo Sanmartín, cmljYXJkby5zYW5tYXJ0aW5AdWEuZXM=
Disclaimer: All claims expressed in this article are solely those of the authors and do not necessarily represent those of their affiliated organizations, or those of the publisher, the editors and the reviewers. Any product that may be evaluated in this article or claim that may be made by its manufacturer is not guaranteed or endorsed by the publisher.
Research integrity at Frontiers
Learn more about the work of our research integrity team to safeguard the quality of each article we publish.