- 1Center for Advanced Research in Education (FB0003), Institute of Education, Universidad de Chile, Santiago, Chile
- 2Center for Mathematical Modeling (AFB170001), Universidad de Chile, Santiago, Chile
- 3Department of Mathematical Engineering, Universidad de Chile, Santiago, Chile
- 4Departamento de Evaluación, Medición y Registro Educacional, Universidad de Chile, Santiago, Chile
- 5CLLE (Cognition, Langues, Langage, Ergonomie), UT2J CNRS, University of Toulouse, Toulouse, France
Middle bias has been reported for responses to multiple-choice test items used in educational assessment. It has been claimed that this response bias probably occurs because test developers tend to place correct responses among middle options, tests thus presenting a middle-biased distribution of answer keys. However, this response bias could be driven by strong distractors being more frequently located among middle options. In this study, the frequency of responses to a Chilean national examination used to rank students wanting to access higher education was used to categorize distractors based on attractiveness level. The distribution of different distractor types (best distractor, non-functioning distractors…) was analyzed across 110 tests of 80 five-option items administered to assess several disciplines in five consecutive years. Results showed that the strongest distractors were more frequently found among middle options, most commonly at option C. In contrast, the weakest distractors were more frequently found at the last option (E). This pattern did not substantially vary across disciplines or years. Supplementary analyses revealed that a similar position bias for distractors could be observed in tests administered in countries other than Chile. Thus, the location of different types of distractors might provide an alternative explanation for the middle bias reported in literature for tests’ responses. Implications for test developers, test takers, and researchers in the field are discussed.
Introduction
Multiple-choice tests are widely used in educational assessment, students’ performance on these tests being sometimes highly consequential (Gierl et al., 2017). Thus, it may become critical that tests do provide valid and reliable learning measures (Haladyna and Downing, 2004). Even if item-writing guidelines have been advanced in literature to help test developers design better multiple-choice instruments (Haladyna and Downing, 1989a; Haladyna et al., 2002; Haladyna and Rodriguez, 2013), item-writing flaws are still commonly found, impacting tests’ psychometric properties, students’ scores, and even pass-fail outcomes (Downing, 2005; Tarrant and Ware, 2008; Ali and Ruit, 2015).
One rather common test construction flaw is that the placement of correct responses (also called answer keys) across a test is middle-biased, key position providing an unwanted strategic clue to examinees (Metfessel and Sax, 1958; Haladyna and Downing, 1989b; Attali and Bar-Hillel, 2003). Empirical results have confirmed that students do consider option position when taking a test (Carnegie, 2017) and that students’ responses themselves present a middle-bias pattern, which can lead to less discriminative items with high accuracy rates when middle-keyed (Attali and Bar-Hillel, 2003). One recent explanation for students’ response bias lies in the test developers’ own middle bias when positioning answer keys (Bar-Hillel, 2015).
However, it might be distractors, not keys, what really drives middle-biased responses among students. If the strongest distractors were to be more frequently positioned as middle options, examinees would consequently select middle options more frequently than edge options when responding inaccurately (Gustav, 1963). This would be consistent with the fact that the reported students’ response bias is sometimes more robust for incorrect responses than for correct ones (see, for example, Attali and Bar-Hillel, 2003).
Literature has shown that strong distractors’ position impacts item difficulty (Friel and Johnstone, 1979; Ambu Saidi and Khamis, 2000; Kiat et al., 2018; Shin et al., 2019). However, the distribution of strong distractors across a test has not been addressed. In a systematic research synthesis examining test developers’ practice regarding options placement (Authors, 2021, under review), more than 50 relevant studies were identified, none of them considering strong distractors’ arrangement. Neither did any of these studies focus on weak distractors’ placement. Interestingly, however, one study noticed that most of non-selected distractors from a sample of 151 five-option items were located as last option (Siddiqui, 2018). Since an unbalanced distribution of strong/weak distractors may provide students with valuable information to reject some options when strategically solving items, studying the overall arrangement of distractors might prove to be enlightening.
This study was conceived to examine the distribution of different types of distractors in multiple-choice tests. Previous studies have shown that many tests present either a middle-keying bias (Attali and Bar-Hillel, 2003) or an overbalanced distribution of answer keys (Bar-Hillel and Attali, 2002), suggesting that test developers rarely randomize options order during test assembly. We thus expected results to provide new insights into both test development and item creation processes. Our study was guided by the working hypothesis that when test developers design items, they tend to generate distractors following a plausibility order, which ultimately correlates with distractors’ placement within the options list, with strong distractors being positioned before weak ones.
Materials and Methods
Data Collection
All of the examinees’ responses to Chilean national examination PSU (Prueba de Selección Universitaria) from 2016, 2017, 2018, 2019, and 2020 were gathered. PSU is a paper-and-pencil, high-stakes, standardized examination which students must take to enter most universities in Chile. The assessment comprises two mandatory exams that all students must take (one in mathematics and one in language) and several other optional exams belonging to different domains that students voluntarily take depending on the program they apply to (such as chemistry, history, or physics). Observed tests were from four domains: language, mathematics, science, and history. Final data set included 8,800 multiple-choice items from 110 eighty-item tests.
Individual responses per item ranged from 1,567 to 66,821, totaling 318,859,763 single-item responses. All items had five options and were designed and field-tested by DEMRE (Departamento de Evaluación, Medición y Registro Educacional), the Chilean state institution in charge of developing and administering national university admission exams. All participants signed a written informed consent stating that their responses could be used for research purposes.
A second data set, obtained from a previous systematic research synthesis (Authors, 2021, under review), was also used. Data consisted of 421 items (108 five-option and 313 four-option items) from 13 item sets (four for five-option and nine for four-option items), obtained from 11 studies. Studies were identified during the selection process of the systematic research synthesis and were included because they provided not only answer keys’ distribution but also test-takers responses to multiple-choice items for each option position separately, making it possible to identify the different types of distractors. Items from this second data set were from tests used in countries other than Chile.
Data Analysis
The first set of analyses consisted of examining the distribution of the two most classically studied distractor types: best distractor and non-functioning distractors. The best distractor (also called the most attractive distractor) for each item was defined as the erroneous response most frequently selected by examinees, following previous studies (e.g., Shin et al., 2019). A non-functioning distractor was defined as an erroneous response selected by less than five percent of examinees, as standardly defined in most previous studies (e.g., Tarrant et al., 2009). It is worth mentioning that an item can have various non-functioning distractors but no more than one best distractor. Occasionally, items had no best distractor (because two distractors of one item received the same number of responses) or no non-functioning distractors at all (because all distractors of one item received more than five percent of responses).
For the purposes of the first set of analyses, the best distractor for every single item was identified based on examinees’ responses. Once identified, its position within the options list (A, B, C, D, E) was registered. This allowed determining best distractor’s position at item level. On a second step, at a single-test level, each test taken by examinees was individually inspected to determine the frequency of best distractors at A, B, C, D, and E across all test items. Since not all items of a given test had indeed a best distractor, the absolute frequency of best distractors per position was converted, per test, to a percentage relative to the exact number of items containing an actual best distractor. Finally, a one-way repeated-measures ANOVA was conducted including all tests (regardless of domain and year), with Option Position as within factor (five levels: A, B, C, D, E) and percentage of best distractor’s presence (hereinafter called frequency) as dependent variable. The same procedure was implemented for non-functioning distractors.
In a second set of analyses, all distractors were ranked by attractiveness level for every single item, based on response frequency (best distractor > distractor 2 > distractor 3 > worst distractor), registering the position of each kind of distractor within the options list (A, B, C, D, E). At test level, distractors’ frequencies were compared at every position. Since totals varied per test and per positions, raw frequencies were again converted to percentages. For instance, if for a given test distractors were found 60 times (out of 80) for option A, raw frequencies of best distractor and remaining distractors were converted to percentages relative to a total of 60. Correct answers were excluded from all counts. Once this was completed, five one-way repeated-measures ANOVAs were conducted (one for each of the five option positions), with Distractor Type as within factor (four levels: best distractor, distractor 2, distractor 3, worst distractor) and percentage of occurrence (hereinafter called frequency) as dependent variable. ANOVAs assumptions were inspected and met by all conducted tests. Bonferroni post-hoc tests were conducted and were reported when relevant. Partial eta squared was reported as size effect measure.
Supplementary analyses were implemented to make sure that observed results were robust and generalizable. First, the distributions (percentages) of best and worst distractors were analyzed again, after defining distractors more conservatively, to make sure that observed results were not spurious. At this point, the most frequently selected erroneous response was labeled best distractor only when having received at least five percent more responses than the second-best distractor (distractor 2), and the least frequently selected erroneous response was labeled worst distractor only when having been selected five percent less than the second-worst distractor (distractor 3). This was done to confirm that findings were not attributable to the influence of option position on test-takers behavior (this influence being modest, with option position effects being generally smaller than five percent). Second, the distributions of best distractor and non-functioning distractors were analyzed for each tested domain (language, math, science, history) and year of test administration (2016, 2017, 2018, 2019, 2020) separately, to evaluate the generalizability and replicability of findings. Finally, the second data set was used to determine whether tests used in countries other than Chile presented similar distributions of distractors.
Results
A statistically significant difference was observed when comparing the frequency of best distractor between different option positions: F (4,436) = 50.267, p < 0.001, ƞ2p = 0.316. Best distractor was found the most frequently at option C and the least frequently at option E (all ps ≤ 0.004 in post-hoc tests, Figure 1A). When comparing the frequency of non-functioning distractors across option positions, a significant difference was also observed: F (4,420) = 41.598, p < 0.001, ƞ2p = 0.284. Non-functioning distractors were found the most frequently at option E and the least frequently at option C, an exact inversion of the pattern observed for best distractor (all ps < 0.001 in post-hoc tests, Figure 1B). In short, while frequencies for options A, B, and D did not hugely differ either when observing best distractor or non-functioning distractors, frequencies for options C and E did differ importantly and were completely reversed, with an eloquent bias towards option C for best distractors and an eloquent bias towards option E for non-functioning distractors. A visual inspection of these frequencies’ distribution showed that the strongest distractors were, in general, more likely to be found among middle options, whereas the weakest ones were mostly found at the last option.
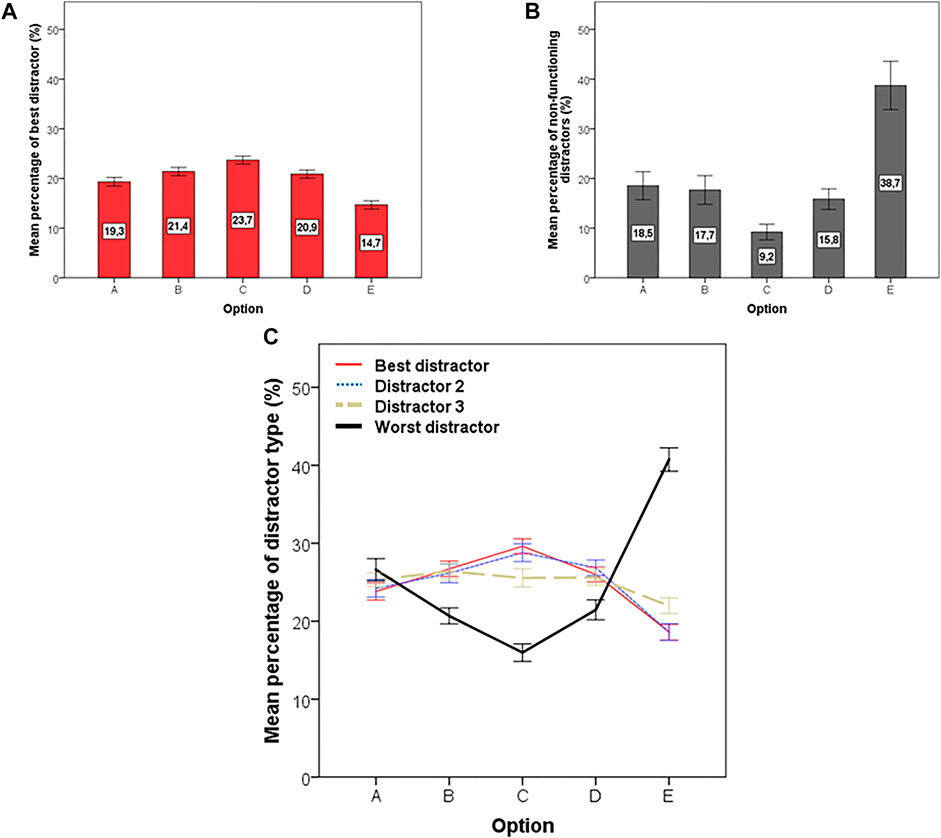
FIGURE 1. Distribution of different distractor types in multiple-choice tests. The distribution of best distractor, non-functioning distractors, and ranked distractors (best distractor, distractor 2, distractor 3, worst distractor) used in Chilean national examination to access higher education is presented in (A–C), respectively. All presented percentages are means across tests. In (A,B), percentages are calculated for every single analysed test by dividing the number of best distractors and non-functioning distractors found in each option position throughout the test by the total number of best and non-functioning distractors in test, respectively. In (C), percentages are computed differently: They are calculated for every single analysed test by counting the number of each distractor type found in each option position throughout the test and then dividing this number by the total number of distractors in that position in test. Error bars represent 95% confidence intervals.
When inspecting the frequency of distractor types (best distractor, distractor 2, distractor 3, worst distractor) at each option position (A, B, C, D, E), statistically significant differences were observed for all five positions: F (3,327) = 3.483, 21.177, 95.690, 14.726, and 245.512, respectively, all ps < 0.016, ƞ2p = 0.031, 0.163, 0.467, 0.119, and 0.693, respectively. Post-hoc analyses revealed that the worst distractor was found less frequently than the other distractors at options B, C, and D, but much more frequently at option E (all ps < 0.001). The best and second-best distractors were more frequently found at option C than the second-worst distractor was, and, conversely, they were both less frequently found at option E than the second-worst distractor (all ps < 0.012).
Taken together, these results clearly revealed a bias in terms of how strong distractors and weak distractors spread between option positions. Strongest distractors were more likely to be found among middle options, preferentially at option C, whereas the weakest distractors were more likely to be found at last option, E. Supplementary analyses confirmed that these results were robust and generalizable. Frequencies for the best and worst distractors were biased even when distractors were defined more conservatively (see Data Analysis section and Supplementary Figure S1), revealing that these position biases cannot be explained (at least not wholly explained) by the fact that examinees tended to more frequently select any specific option position(s). Frequencies for the best distractor and for non-functioning distractors were found to be similarly biased in the four tested domains and in the 5 years exams were administered (Supplementary Figure S2), which confirmed generalizability and replicability of findings. Critically, a similarly biased pattern for distractors was observed again when inspecting multiple-choice tests used in countries other than Chile (Supplementary Figure S3), suggesting that the involved phenomenon is probably not cultural. Note that in this last analysis, bias was not only observed for five-option items, but also for four-option items.
Discussion
Previous studies about response options placement have shown that answer keys are not uniformly distributed in many multiple-choice tests, keys being more frequently positioned as a middle option than as an edge option (Attali and Bar-Hillel, 2003; Authors, 2021, under review; Metfessel and Sax, 1958). This keying bias reveals that test developers do not balance (or randomize) the position of answer keys in tests, ignoring guidelines provided by item-writing guides for decades now (Trump and Haggerty, 1952; Haladyna and Downing, 1989a; Haladyna et al., 2002; Haladyna and Rodriguez, 2013). Implications for the validity of test scores may be critical: if test takers become aware that answer keys are more frequent among middle options, they can develop position-based strategies to make more accurate guesses and provide correct responses by selecting more central positions (Bar-Hillel and Attali, 2002; Bar-Hillel et al., 2005).
Results from this study showed that neither strong nor weak distractors were uniformly distributed in tests: while the strongest distractors were most frequently found among middle options, the weakest distractor was most likely to be found at the end of the options list. These distribution biases are independent of the keying bias. Put differently, the best distractor of multiple-choice items tends to present itself before the worst one. This bias does not imply non-adherence to item-writing guidelines because no guide has provided any specific recommendations about distractors’ placement. However, it confirms that test developers do not usually randomize options order, contrary to recommendations from recent guides (Xu et al., 2016; Gierl et al., 2017).
Present findings have several implications. Most importantly, they have apparent implications for research exploring the effects of key position on item accuracy. Empirical literature about this topic reports conflicting results: While some studies have claimed that items are easier when key is placed in the middle (Attali and Bar-Hillel, 2003; DeVore et al., 2016) or among the first options (Hohensinn and Baghaei, 2017; Holzknecht et al., 2020), others have concluded that item performance is hardly affected by options position (Sonnleitner et al., 2016; Wang, 2019). Since position of distractors has been shown to impact item accuracy (Kiat et al., 2018; Shin et al., 2019) and since the present study shows that the distribution of distractors may be significantly biased, the above inconsistency in reported results may ultimately be driven by the fact that numerous studies about key position did not control for distractors’ position. In other words, the middle bias observed in the past among examinees’ responses might not always have been a correlate of keying bias but the result of placing the strongest distractors within the middle options. Future studies inspecting the effects of key position on item performance and test scores might need to consider distractors’ position as a potential confounding factor.
Implications for test takers are less clear. Indeed, it remains uncertain how examinees would adapt their item-solving strategies if they knew that strongest distractors are more likely to be found among earlier options than weaker ones. Examinees might assume that the last option(s) is (are) not worth being read with care and focus their cognitive efforts on the first options in the list, which would be consistent with the claim that test takers do not always read all the alternatives before responding (Clark, 1956; Fagley, 1987; Willing, 2013) and with the fact that they most frequently explore options in order (Holzknecht et al., 2020). Further research is needed to better understand the link between belief or awareness about options placement and how test takers read and solve multiple-choice items.
One possible explanation for presented results is that distractors are generated and listed in order of plausibility during item-design stage, this order remaining unaltered by test developers during the process of assembling a test once items have been designed. If this is the case, it is only natural that the weakest distractors are to be found at the last option, because a highly plausible, strong distractor is more likely to be retrieved from memory during the item-writing process than a less plausible, weak distractor (Attali and Bar-Hillel, 2003). Ultimately, then, distractors’ prominence/cognitive availability shapes the options order, consistently with our working hypothesis. Test developers might thus be highly interested in the results presented here because they provide, to the best of our knowledge, the first evidence supporting the claim that options are generated in order of plausibility. Future studies might analyze in much more depth the creation process of single items to explore this.
Finally, there are some limitations to be mentioned. First, most of the results presented in this article were based on data gathered from five-option items. A large sample of four-option items and three-option items should be analyzed to determine whether (and how) the number of options modulates the distribution bias of distractors. More generally, items with a different set of traits (such as items with ordered numbers as options or with algebraic expressions as options) should be studied to confirm whether this position bias is present in all kinds of multiple-choice items or not. Second, the distribution of distractors was mainly analyzed in a set of real-life high-stakes tests. More in-house tests should be analyzed to confirm that the distribution bias of distractors exists at all educational levels and gauge the impact of test developers’ training/experience at item writing on this phenomenon. Finally, studies identifying different distractor types by means of a method not solely based on response frequency are needed to disentangle developers’ placement bias more clearly from examinees’ response bias. One interesting possibility is working on item sets having distractor types clearly identified by test developers’ boards before administration. Although predicting which distractors will be most or least selected by examinees is not an easy task that will probably be not 100% accurate, such an approach would possibly bring decisive evidence in favor or against our hypothesis.
In sum, this is the first study showing that a clear and widespread bias can be observed in the distribution of distractors in multiple-choice tests, suggesting that distractors were probably sequenced in a plausibility order when developers created items. Considering that distractors’ relative position and distance to correct response affect item performance and test scores (Kiat et al., 2018; Shin et al., 2019), test developers should be aware that the order of distractors could introduce noise on test results, especially when options order is scrambled to generate equivalent test forms. Researchers interested in conducting empirical studies focused on option position effects should consider controlling distractors position if they want to adequately capture the effects of key position on the item performance and/or test outcomes. In short, this study should draw educators and researchers’ attention to an item trait they have probably never or rarely considered.
Data Availability Statement
The raw data supporting the conclusion of this article will be made available by the authors, without undue reservation.
Author Contributions
SL, PD, and JL developed the study concept; MG, NC, and DJ handled the main data collection; SL and CM performed data analyses; MB and GO provided crucial information about item-writing guides and results’ presentation, respectively. SL drafted the manuscript, and all the other authors provided critical revisions. All authors have approved the final version of this manuscript.
Funding
This research was supported by the following grants from ANID: Fondecyt postdoctorado #3190273 and FONDEF ID16I10090. Support from ANID/PIA/Basal Funds for Centers of Excellence FB0003 (Center for Advanced Research in Education) and AFB170001 (Center for Mathematical Modeling) is also gratefully acknowledged.
Conflict of Interest
The authors declare that the research was conducted in the absence of any commercial or financial relationships that could be construed as a potential conflict of interest.
Publisher’s Note
All claims expressed in this article are solely those of the authors and do not necessarily represent those of their affiliated organizations, or those of the publisher, the editors and the reviewers. Any product that may be evaluated in this article, or claim that may be made by its manufacturer, is not guaranteed or endorsed by the publisher.
Acknowledgments
We thank María Leonor Varas, director of the Departamento de Evaluación, Medición y Registro Educacional (DEMRE), for her unconditional support and for making this collaborative research possible. We also thank Camilo Quezada Gaponov for editing the manuscript.
Supplementary Material
The Supplementary Material for this article can be found online at: https://www.frontiersin.org/articles/10.3389/feduc.2021.731763/full#supplementary-material
References
Ali, S. H., and Ruit, K. G. (2015). The Impact of Item Flaws, Testing at Low Cognitive Level, and Low Distractor Functioning on Multiple-Choice Question Quality. Perspect. Med. Educ. 4 (5), 244–251. doi:10.1007/s40037-015-0212-x
Ambu-Saidi, A., and Khamis, A. (2000). An Investigation into Fixed Response Questions in Science at Secondary and Tertiary Levels. Doctoral dissertation. Glasgow: University of Glasgow.
Attali, Y., and Bar-Hillel, M. (2003). Guess where: The Position of Correct Answers in Multiple-Choice Test Items as a Psychometric Variable. J. Educ. Meas. 40 (2), 109–128. doi:10.1111/j.1745-3984.2003.tb01099.x
Bar-Hillel, M. (2015). Position Effects in Choice from Simultaneous Displays: A Conundrum Solved. Perspect. Psychol. Sci. 10 (4), 419–433. doi:10.1177/1745691615588092
Bar-Hillel, M., and Attali, Y. (2002). Seek Whence. The Am. Statistician 56 (4), 299–303. doi:10.1198/000313002623
Bar-Hillel, M., Budescu, D., and Attali, Y. (2005). Scoring and Keying Multiple Choice Tests: A Case Study in Irrationality. Mind Soc. 4 (1), 3–12. doi:10.1007/s11299-005-0001-z
Carnegie, J. A. (2017). Does Correct Answer Distribution Influence Student Choices when Writing Multiple Choice Examinations. cjsotl-rcacea 8 (1), 11. doi:10.5206/cjsotl-rcacea.2017.1.11
Clark, E. L. (1956). General Response Patterns to Five-Choice Items. J. Educ. Psychol. 47 (2), 110–117. doi:10.1037/h0043113
DeVore, S., Stewart, J., and Stewart, G. (2016). Examining the Effects of Testwiseness in Conceptual Physics Evaluations. Phys. Rev. Phys. Educ. Res. 12 (2), 020138. doi:10.1103/PhysRevPhysEducRes.12.020138
Downing, S. M. (2005). The Effects of Violating Standard Item Writing Principles on Tests and Students: the Consequences of Using Flawed Test Items on Achievement Examinations in Medical Education. Adv. Health Sci. Educ. Theor. Pract. 10 (2), 133–143. doi:10.1007/s10459-004-4019-5
Fagley, N. S. (1987). Positional Response Bias in Multiple-Choice Tests of Learning: Its Relation to Testwiseness and Guessing Strategy. J. Educ. Psychol. 79 (1), 95–97. doi:10.1037/0022-0663.79.1.95
Friel, S., and Johnstone, A. H. (1979). Does the Position of the Answer in a Multiple-Choice Test Matter. Educ. Chem. 16, 175. Available at: https://eric.ed.gov/?id=EJ213396.
Gierl, M. J., Bulut, O., Guo, Q., and Zhang, X. (2017). Developing, Analyzing, and Using Distractors for Multiple-Choice Tests in Education: a Comprehensive Review. Rev. Educ. Res. 87 (6), 1082–1116. doi:10.3102/0034654317726529
Gustav, A. (1963). Response Set in Objective Achievement Tests. J. Psychol. 56 (2), 421–427. doi:10.1080/00223980.1963.9916657
Haladyna, T. M., and Downing, S. M. (1989a). A Taxonomy of Multiple-Choice Item-Writing Rules. Appl. Meas. Educ. 2 (1), 37–50. doi:10.1207/s15324818ame0201_3
Haladyna, T. M., and Downing, S. M. (2004). Construct-Irrelevant Variance in High-Stakes Testing. Educ. Meas. Issues Pract. 23 (1), 17–27. doi:10.1111/j.1745-3992.2004.tb00149.x
Haladyna, T. M., Downing, S. M., and Rodriguez, M. C. (2002). A Review of Multiple-Choice Item-Writing Guidelines for Classroom Assessment. Appl. Meas. Educ. 15 (3), 309–333. doi:10.1207/s15324818ame1503_5
Haladyna, T. M., and Downing, S. M. (1989b). Validity of a Taxonomy of Multiple-Choice Item-Writing Rules. Appl. Meas. Educ. 2 (1), 51–78. doi:10.1207/s15324818ame0201_4
Haladyna, T. M., and Rodriguez, M. C. (2013). “Developing and Validating Test Items,” in Developing and Validating Test Items. Editors T. M. Haladyna, and M. C. Rodriguez (New York: Routledge), 89–110. doi:10.4324/9780203850381
Hohensinn, C., and Baghaei, P. (2017). Does the Position of Response Options in Multiple-Choice Tests Matter. Psicológica 38 (1), 93–109. Available at: https://files.eric.ed.gov/fulltext/EJ1125979.pdf.
Holzknecht, F., McCray, G., Eberharter, K., Kremmel, B., Zehentner, M., Spiby, R., et al. (2020). The Effect of Response Order on Candidate Viewing Behaviour and Item Difficulty in a Multiple-Choice Listening Test. Lang. Test. 38, 41–61. doi:10.1177/0265532220917316
Kiat, J. E., Ong, A. R., and Ganesan, A. (2018). The Influence of Distractor Strength and Response Order on MCQ Responding. Educ. Psychol. 38 (3), 368–380. doi:10.1080/01443410.2017.1349877
Metfessel, N. S., and Sax, G. (1958). Systematic Biases in the Keying of Correct Responses on Certain Standardized Tests. Educ. Psychol. Meas. 18 (4), 787–790. doi:10.1177/001316445801800411
Shin, J., Bulut, O., and Gierl, M. J. (2019). The Effect of the Most-Attractive-Distractor Location on Multiple-Choice Item Difficulty. J. Exp. Educ. 88, 643–659. doi:10.1080/00220973.2019.1629577
Siddiqui, Z. S. (2018). Errors in the Construction of Multi-Choice Questions: An Analysis. The Pakistan J. Med. Dentistry 7 (4), 4. Available at: https://research-repository.uwa.edu.au/files/39825784/ERRORS_IN_THE_CONSTRUCTION_OF_MULTI_CHOI.pdf.
Sonnleitner, P., Guill, K., and Hohensinn, C. (2016). “Effects of Correct Answer Position on Multiplechoice Item Difficulty in Educational Settings: Where Would You Go,” in International Test Commission Conference, Vancouver, Canada, August 2, 2016. Available at: http://hdl.handle.net/10993/29469.
Tarrant, M., and Ware, J. (2008). Impact of Item-Writing Flaws in Multiple-Choice Questions on Student Achievement in High-Stakes Nursing Assessments. Med. Educ. 42 (2), 198–206. doi:10.1111/j.1365-2923.2007.02957.x
Tarrant, M., Ware, J., and Mohammed, A. M. (2009). An Assessment of Functioning and Non-functioning Distractors in Multiple-Choice Questions: a Descriptive Analysis. BMC Med. Educ. 9 (1), 40–48. doi:10.1186/1472-6920-9-40
Trump, J. B., and Haggerty, H. R. (1952). Basic Principles in Achievement Test Item Construction. Washington: The Adjutant General’s Office. Personnel Research Section Report 979. doi:10.1037/e523682009-001
Wang, L. (2019). Does Rearranging Multiple‐Choice Item Response Options Affect Item and Test Performance. ETS Res. Rep. Ser. 2019 (1), 1–14. doi:10.1002/ets2.12238
Willing, S. (2013). Discrete-option Multiple-Choice: Evaluating The Psychometric Properties of a New Method of Knowledge Assessment. Doctoral dissertation. Duesseldorf: University of Düsseldorf. Available at: https://docserv.uni-duesseldorf.de/servlets/DerivateServlet/Derivate-29719/Dissertation%20Sonja%20Willing.pdf.
Keywords: assessment, educational tests, multiple-choice, response placement, distractors
Citation: Lions S, Monsalve C, Dartnell P, Godoy MI, Córdova N, Jiménez D, Blanco MP, Ortega G and Lemarié J (2021) The Position of Distractors in Multiple-Choice Test Items: The Strongest Precede the Weakest. Front. Educ. 6:731763. doi: 10.3389/feduc.2021.731763
Received: 28 June 2021; Accepted: 16 September 2021;
Published: 28 October 2021.
Edited by:
Yong Luo, Educational Testing Service, United StatesReviewed by:
Georgios Sideridis, Harvard Medical School, United StatesDuy Pham, Educational Testing Service, United States
Copyright © 2021 Lions, Monsalve, Dartnell, Godoy, Córdova, Jiménez, Blanco, Ortega and Lemarié. This is an open-access article distributed under the terms of the Creative Commons Attribution License (CC BY). The use, distribution or reproduction in other forums is permitted, provided the original author(s) and the copyright owner(s) are credited and that the original publication in this journal is cited, in accordance with accepted academic practice. No use, distribution or reproduction is permitted which does not comply with these terms.
*Correspondence: Séverin Lions, severin.lions@ciae.uchile.cl