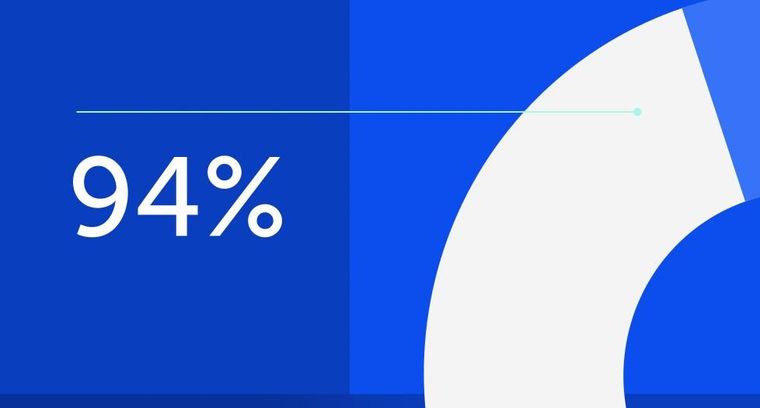
94% of researchers rate our articles as excellent or good
Learn more about the work of our research integrity team to safeguard the quality of each article we publish.
Find out more
ORIGINAL RESEARCH article
Front. Educ., 16 September 2021
Sec. Assessment, Testing and Applied Measurement
Volume 6 - 2021 | https://doi.org/10.3389/feduc.2021.727539
The Inference-Making and Reasoning in Biology (IMRB) measure is an assessment tool intended to 1) aid university personnel in advising students on course enrollment, 2) identify students in need of interventions to increase their reasoning skills and likelihood of completing STEM majors, 3) support instructors in determining growth in students’ reasoning skills, and 4) provide a measuring tool to gauge success of higher-education interventions intended to increase reasoning skills. Validity arguments for these four uses of the IMRB are provided by implementing a validity argument approach. This work exemplifies the advantages of framing validation studies within a validity argument framework.
Previous research has shown that making inferences during reading is beneficial, as it is associated with forming a better mental model of the depicted situation (Kintsch, 1998; McNamara, 2004; Butcher, 2006). In Kintsch (1998) Construction-Integration (CI) Model, readers form different representations of text (each of which can range from low to high quality) depending on the extent to which they incorporate their own prior knowledge: 1) a surface form or verbatim text model, similar to a “photographic memory” of the text; 2) a textbase or gist model of the text, which is a summary of what was read, but without adding any information from the reader’s prior knowledge; and 3) a situation model in which information from the text is incorporated with information from the reader’s prior knowledge. The situation model is posited to be a higher level of comprehension than the textbase (Royer et al., 1987; Graesser and Britton, 1996). From a practical standpoint, these comprehension quality differences arise because more inferences are generated when forming a situation model than a textbase representation of the text. Further, the during-reading processes (e.g., such as bridging inferences and elaborative inferences) and the reading strategies (e.g., summarizing, self-questioning, making a drawing) required to form a situation model are far more sophisticated than those required for forming a textbase representation of the text.
Many undergraduate students fail to perform well in freshman biology courses: one-half of students drop out of life sciences majors, and most after their first year (National Science Foundation, 2006). Poor performance in STEM is caused by a number of cognitive processes and motivation summarized by Pintrich (2000). In this manuscript we have focused on one of the higher order cognitive processes, deductive reasoning. Other cognitive skills interacting with deductive reasoning are activating prior knowledge, identifying key points, organizing material, and synthesizing materials (Van Meter et al., 1994; Gurlitt and Renkl, 2010). However, a student with complete mastery of these cognitive skills without self-motivation to put them into effect will perform poorly (Gutherie et al., 2004; Cleary et al., 2017; Cromley et al., 2020b). Measuring a student’s cognitive mastery and motivation for all of these variables would be overwhelming, which is why we focused on the higher order cognitive processes of deductive reasoning. A deficit in deductive reasoning may show up as students not being adept at drawing inferences from material learned in classes and from textbooks (Cromley et al., 2010)—akin to forming a situation model. Early intervention, additional supports, and course placement recommendations allow students to develop better reasoning abilities, leading to improved performance and less attrition. Over the last several years, researchers across universities developed a measure that assesses students’ inference-making and reasoning abilities in biology.
The construct of interest, inference-making and reasoning, is defined as “applied reasoning with recently presented information” (i.e., the ability to use evidence statements and artifacts to arrive at sensible and accurate conclusions). The context for this construct is undergraduate introductory biology coursework. The Inference-Making and Reasoning in Biology (IMRB) measure is intended to provide valid inferences for undergraduate students in introductory biology, mostly representing biochemistry and biology-related majors, including students at 4-year universities and colleges (but not 2-year schools) in the United States. Scores from the IMRB are intended to help academic advisors work with students to make appropriate course selections and seek additional supports when needed.
For any measure, it is imperative to evaluate whether it can be used in the ways it was intended. There are four intended uses for IMRB test scores and all four must be validated. The intended uses are to.
1. make student course placement recommendations (course placement);
2. identify students who could benefit from additional supports, such as workshops, tutoring, and mentoring (identification);
3. document biology reasoning growth over a semester (growth); and
4. support achievement and retention intervention research, such as comparing the effects of supports for at-risk students (intervention research).
Table 1 provides a comprehensive summary of appropriate and inappropriate uses for the IMRB.
This paper not only lays out the validity arguments that lend support for these uses, but it also provides an exemplar for constructing and presenting a validity argument for any kind of assessment. Acknowledging that validation efforts for any measure are ongoing (Kane, 2013), this paper also identifies gaps and possible counterarguments where more research could be conducted to strengthen this validity argument. The summary of the validity argument for the IMRB uses can be found in Figure 1 and Figure 2 and is a useful resource for the reader to refer to throughout.
The IMRB is designed to measure deductive reasoning from newly-presented biology information. The reasoning is deductive in that conclusions can be drawn from two pieces of presented information; this is distinguished from inductive reasoning or pattern detection, which is also important for biology learning. No information from prior knowledge is needed, beyond the most basic information such as “cells make up tissues.”
The assessment tasks for the IMRB are short paragraphs of content taught at the end of a semester of survey biology courses designed for science majors (e.g., biology, biochemistry, neuroscience). The stimuli, which focus on the immune system, are provided to examinees to read and then examinees are asked to respond to 15 multiple-choice questions. The multiple-choice questions are designed to elicit inference-making and reasoning skills as the distractors contain common misconceptions that students make based on the stimuli. Some items have associated graphics (e.g., diagrams, tables)—with an example in Figure 3.
FIGURE 3. Example IMRB test question1.
The first version of the IMRB was developed from student statements while reading from their biology textbook (Cromley et al., 2010). Students (n = 91) were asked to say everything they were thinking while learning from passages about the immune system, and—among other codes—students’ inferences were categorized as correct or incorrect. The think-aloud sessions (Cromley et al., 2021; Dai et al., 2018) were 40-min in length. The resulting passages and statements were then used to create brief deductive reasoning items, where the correct inferences from the think-aloud study were used as correct answers to 4-option multiple-choice reasoning items, and incorrect inferences (e.g., over-generalizations, under-generalizations, or restatements of a premise) were used as distractors.
Twenty-five items were initially developed, and were piloted with 737 undergraduate introductory biology students. Items that did not perform well in reliability analysis were deleted, resulting in the first 15 items of the IMRB.
For the development of new IMRB items, the 15 old items were reviewed to uncover content-based perspectives for why those items performed well. Additionally, 86 think-alouds were conducted on the newly selected passages—also obtained from an introductory undergraduate biology textbook—using biology course alumni. Based on the information collected, item specifications were reverse engineered for the development of a new set of 21 items to be field tested and added to the IMRB pool. Of these, 15 new IMRB items were preserved—resulting in a total of 30 IMRB items. Thus, there are two 15-item parallel versions (i.e., Form A and Form B) of the IMRB.
While the Standards for educational and psychological testing (American Educational Research Association, American Psychological Association, and National Council on Measurement in Education, 2014) provide assessment practitioners with valuable guidance on the kinds of validity evidence available along with some methods for its assessment; they do not provide a framework within which to construct a validity argument. Kane (2006), Kane (2013) picks up where the Standards fall short by providing example lines of validation research through the use of chains of inference that lead to assumptions, evidence, and rebuttal. For educational assessments, the main goal of the validity argument is to provide support for the proposed interpretations and uses for the results of the assessment. The resulting conclusions and decisions are typically made based on a series of assumptions. Evidence must be collected to support these assumptions in order to place any faith in the decisions made based upon results. Further, what links evidence and assumptions are inferences which are generally backed by solid theory or experience. Oftentimes, there are alternative hypotheses that contradict the assumption. These alternative hypotheses can also be supported using evidence. In the absence of evidence to support the alternative hypotheses and in the presence of evidence that support the assumption, the assumption is considered plausible. A chain of these inferences can be built to further develop the validity argument where the assumption from a previous inference serves as the evidence for the following inference.
This process of chaining inferences was explained by Toulmin (1958) and has been found to be useful in measurement theory (Mislevy, 1996; Mislevy et al., 2002). Figure 4 shows an example adapted to a multiple-choice assessment that uses slightly different terminology than Toulmin (1958).
While this manuscript aims to exemplify the implementation of the validity argument approach to test score interpretation and use validation, it does not intend to compare various frameworks. For a thorough and critical comparison of various approaches to presenting validity evidence, within the context of language assessments, see Im et al. (2019) compelling review. These authors expand on the work of Chapelle and Voss (2014) who present an historical review of the evolution of test validity theories and practices (e.g., Messick, 1989; Bachman, 2005; Kane, 2006; Bachman, and Palmer, 2010).
Kane (2013) framework for validation presents a two-stage process for developing a validity argument that is based on Toulmin (1958) approach, but does streamline some aspects of the labor-intensive process. The first stage is to provide the interpretation/use argument—the internal logic for gathering information and making inferences that guides the user from test administration to reliable, accurate interpretation and appropriate use. Once the assertions are organized such that they hold together logically, a validity argument is built by gathering evidence to determine how well the assertions are factually supported by data.
The interpretation/use argument provides the necessary logic to support the interpretations and uses of test scores by making explicit the inferences, claims, and assumptions necessary to make links between the observed test score and intended interpretations and uses.
Chapelle and Enright (2010) compared the utility of the argument-based approach to more traditional presentations of validity evidence as applied to the Test of English as a Foreign Language™ (TOEFL®) and found the advantages to be four-fold in that it allows for a more natural unfolding of evidence to 1) frame the intended score interpretations and uses, 2) outline the essential validation research, 3) structure research results, and 4) posit challenges to the validity argument. Thus, the argument-based approach to validity is a principled method for the development of assumptions tied to inferences that ultimately support the intended score interpretations and uses of the measure under study. While the comparative efforts (e.g., Chapelle and Enright, 2010; Chapelle and Voss, 2014; Im et al., 2019) have been developed within the context of language assessments, they easily generalize to other assessment constructs, such as inference-making and reasoning—the focus of the IMRB.
For the IMRB uses, there are six levels of inference addressed: Description, Evaluation, Generalization, Explanation, Extrapolation, and Utilization. These are broad categories, each building on the previous. This “building” of inferences can be thought of as articulating each critical transition point where a type of modeling or sampling has taken place. For the IMRB, six such points have been identified. For example, within the Description inference, the construct is defined (or modeled through its verbal description); next, the construct is sampled through the development of items, representing the Evaluation inference. Likewise, scores on the IMRB are assumed to adequately reflect (or model) an examinee’s ability on the construct at the time they took the test leading to the interpretation that the test score serves as a good proxy for construct ability—making up part of the Generalization inference. We can make and support these progressively more abstracted assumptions within each inference level. Some assumptions are relevant for all the intended uses, while others may be more specific to just one. Assumptions specific to a particular use will be identified in the appropriate sections. At a high level, the six inferences can be defined in the following ways:
• Description—The construct of interest is defined, and the test design is developed.
• Evaluation—Examinees take the test and are evaluated, resulting in observed scores on the instrument; the test quality is also evaluated.
• Generalization—An argument is made that the observed score can be interpreted as an expected score on any test form or test occasion (i.e., scores are reliable).
• Explanation—The expected score is used to classify examinees into performance categories, thus allowing the expected score to explain performance on the criterion of interest.
• Extrapolation—Examinees’ skill levels on the construct are inferred based on the classification assigned or score obtained; that is, performance on the assessment is a proxy for performance on the criterion of interest.
• Utilization—Decisions are made based on the skill level inferred.
Table 2 provides the interpretation/use argument for the IMRB where the six inferences are further defined.
The validity argument provides the supporting evidence for the interpretation/use argument, ultimately resulting in the ability of IMRB assessment stakeholders to confidently use test scores in one of the four intended ways with the specified audience (students at 4-year colleges and universities). The validity argument also provides counterarguments for some assumptions that warrant them, identifies weaknesses in the argument, and highlights next steps for strengthening the argument—and ultimately, the IMRB assessment.
Throughout the validity argument that follows, various research studies (beginning in fall 2008 and concluding in spring 2018) based on data collected from consenting students from two universities is referred to. Table 3 provides a list of the various data collection time points by semester along with the sample size and phase of IMRB development. In total, 4,688 students participated in some aspect of the IMRB development. Of those, demographics are available for 1,784 students. Participants represented various races and ethnicities where groups historically underrepresented in STEM (e.g., Black, Hispanic) made up 23% of the samples. Fifty-nine percent were females. Forty percent were first-generation college students. For individual studies where demographic data of this type were collected, they are reported within each of the evidence summaries appearing within the next section.
The next sections are organized by each level of inference and contain both the evidence to support its associated assumptions along with a description and justification for the methods used to supply that evidence where warranted.
In the following sections, we define the construct and how it is measured. Observations of IMRB performance should reflect the identified attributes and appropriate assessment tasks that represent the full breadth and depth of the target domain. To support this inference, evidence has been collected to show that.
• the construct has been defined,
• observable attributes and relevant content areas are well-established and considered appropriate, and
• the assessment tasks provide evidence of observable attributes in relevant content areas.
For any measure, there is a target for what is intended to be assessed. Clear definition of the construct helps ensure better test design and test score use. The construct for the IMRB is inference-making and reasoning, described as “applied reasoning with recently presented information.” Applied is defined as “the application of a principle to a specific situation,” reasoning is defined as “a deductive inference,” and recently presented is defined as “new facts and relations gleaned from stimuli” (e.g., a passage or diagram) for the associated item or from stimuli previously presented within the same assessment.
Instructors and textbooks do not make every relation in the domain explicit; students must draw their own conclusions (i.e., engage in inference-making) to fully understand the course material. Inferences are critical for deep understanding and play a vital role in the transfer of learning to new contexts (Cromley et al., 2010; see also; Cromley et al., 2013). Poor reasoning skills can have detrimental direct and indirect effects on persistence in STEM majors (Lawson et al., 2007). Thus, the IMRB is intended to identify students who may struggle with reasoning. The context within which inferences are elicited is new information that has not been previously taught. The content is not necessarily important beyond it being biological in nature and not previously learned. For the IMRB, immune system content is suitable for reasoning with new information as students typically do not learn the material in high school except at the most superficial level. Therefore, all learners have equal opportunity to acquire and reason with the new information. The endocrine system is another example of an often-untaught area of high school biology. Future IMRB development could consider including stimuli and test items that address the endocrine system.
Further, immunology is like other often-taught areas of biology, e.g., evolution, ecology, and neurology—there is basic terminology, and the main parts of the systems are defined. However, one must also understand the positive and negative interactions of the basic parts to have in-depth understanding.
For the IMRB to remain viable, it is important for regular evaluations to continue for those students who have been observed to lack an in-depth understanding of the immune system. Should teaching practices change (e.g., the immune system is given more emphasis in secondary education), the interpretation of IMRB test scores would also change.
Observable attributes (i.e., drawing inferences and reasoning) can be categorized into various types of inferences:
• Hypothesis Generation (HYP)—posing a hypothesis about how something might work when the hypothesis is not already stated in a stimulus
• Local Inference (INFLOC)—making a conclusion across two adjacent sentences in a stimulus
• Global Inference (INFGLOB)—drawing a conclusion across nonadjacent segments of a stimulus
• Knowledge Elaboration Before Test (KEBT)—combining information from a stimulus with information not found in the stimulus (i.e., prior knowledge) to draw a conclusion
• Knowledge Elaboration Earlier in Test (KEET)—combining information found in a stimulus with information found in a previous item’s stimulus (i.e., information learned while taking the test) to draw a conclusion
Some inferences are intended to be elicited by test items (HYP, INFLOC, INFGLOB), and others are not (KEBT, KEET). The intended inferences are those made based only on the information presented in the stimulus for the associated items. The unintended inferences are those made due to prior knowledge the examinee may have, which is a threat to the premise that the information presented is new and not previously taught. However, this only applies to the KEBT inference. The KEET inference reflects learning that may occur while taking the test, but it does threaten the assumption that the test items are locally independent; responses to items are supported only by the stimulus specific to the item and not by stimuli or material presented for other items. A violation of local independence does not necessarily threaten the use of test scores.
The assessment tasks for the IMRB are short paragraphs containing content typically not taught in most gateway biology courses, though they are later covered in courses designed for science majors (e.g., biology, neuroscience). The stimuli are read by examinees, who are then asked to respond to multiple-choice questions that are designed to elicit inference-making and reasoning skills with distractors (answer choices) containing common misconceptions students might make based on the content of the stimulus. These misconceptions were discovered in two think-aloud studies, the first with 91 students (68% female, 31% male, 1% unidentified; 40% White, 23% Black, 31% Asian, 6% mixed race or other race; 40% first-generation college students) in introductory biology courses (2008 fall semester; Cromley et al., 2010) and the second with an additional 37 biology students (2017 fall semester; 51% White, 37% Asian, 7% Latino/Latina, 6% of other races; 21% first-generation college students). In both studies, students read the stimuli and researchers noted any inferences—accurate or inaccurate—that the students made. The inaccurate inferences (or reasoning errors) were then used as distractors for items developed to accompany the stimuli on the IMRB.
While the description inference requires individual assessment tasks to adequately reflect the target domain, the evaluation inference requires the test—an organized sampling of the assessment tasks—to produce an observed score reflective of inference-making and reasoning in the target domain. Thus, the focus of the evaluation inference is to provide evidence that methods for test assembly, administration, and scoring are appropriate. To support this inference, evidence has been collected to show that.
• the assessment is constructed to draw from the pool of available items such that the underlying domain is adequately sampled,
• the assessment is administered under appropriate conditions,
• scoring procedures produce accurate scores that are reflective of inference-making and reasoning ability, and
• items on the assessment demonstrate appropriate statistical quality.
The stimuli in the IMRB are taken from one of the later chapters of a widely used biology textbook (Campbell and Reece, 2001) not often covered in high school. This is a sufficient sampling technique for immune system content, but it may be worth broadening the scope of sampled content if it is desirable to generalize to all biology content not taught in high school. This would allow for building the argument that inference-making in the immune system content area generalizes to other new content areas as well. While this assumption is not fully supported, it does not detract from the utility of the IMRB.
It is desirable to allow for the IMRB to be administered under a range of conditions, including in a proctored computer laboratory or with a self-guided Blackboard module (a virtual learning environment). More research is needed to evaluate whether IMRB test scores are comparable under the variety of conditions anticipated. Institutions are encouraged to make use of necessary policies that confirm the identity of test-takers. In all cases (except for students with prearranged accommodations), IMRB test sessions are timed (30 min).
A formal user manual (Cromley et al., 2020a) has been developed with entry-level biology course instructors as the intended audience and includes instructions for administering the IMRB. Adherence to the test administration guidelines has not yet been evaluated. Once the manual becomes operational, observational studies could be performed to ensure standardized administration of the IMRB, which would strengthen the ability to compare IMRB scores across individuals and timepoints.
A think-aloud study consisting of 86 participants (fall 2016 semester) who had completed an introductory environmental and organismal biology course required for life sciences majors within two prior years was designed and implemented to determine whether the IMRB tests the intended construct—inference-making and reasoning ability. They were 51% White, 37% Asian, 7% Latino/Latina, and 6% of other races. Twenty-one percent of examinees were first-generation (neither parent with a Bachelor’s degree) college students. In an individual 1-h session, participants were asked to “think aloud” as they answered 15 multiple-choice questions. Responses were audio-recorded, transcribed, and coded for item-response-strategy-use based on a modified and previously published coding scheme (Cromley et al., 2010), resulting in a total of 9,705 coded utterances. For each code, a within-subjects analysis was used to compare the proportion of utterances verbalized when questions were answered incorrectly to when questions were answered correctly in order to determine which codes were associated with correct answers. Eight predictions based on construct validity arguments, prior test-taking research, and problem-solving research were made and evaluated.
This study revealed partial-to-full support for seven of the eight predictions. Cromley et al. (2021) provide a full discussion of the methods and results. Briefly, the findings supported three patterns from IMRB study participants. First, they more often made accurate inferences when responding correctly to a test question than when responding incorrectly to test questions. Second, those responding correctly to test questions did not have greater prior knowledge than study participants responding incorrectly to test questions. Third, those who responded correctly to test questions did not show any greater understanding of the vocabulary in the stimuli and test questions than study participants responding incorrectly to test questions.
While recent research (Sato et al., 2019) shows that many students take nonbiological approaches to answering questions and arrive at the correct response despite wrong thinking, our findings support that the IMRB questions do elicit inference-making behavior (not just test-taking strategies or prior knowledge) in participating students, and this is associated with a higher probability of responding correctly to items (Cromley et al., 2021). Thus, the IMRB test score is a good proxy for deductive reasoning.
The IMRB user manual (Cromley et al., 2020b) contains technical details on the statistical quality of IMRB test questions and test forms. Analyses conducted include the calculation and review of item difficulty, point biserial correlations, IRT parameter estimates, differential item functioning (DIF), and IRT-model fit. These analyses were conducted on the 15 original items and 21 field test items used to create two parallel test forms. Field test items that exhibited serious violations of statistical quality criteria were eliminated from potential use on future test forms.
Additionally, an exploratory factor analysis (EFA) and confirmatory factor analysis (CFA) using different datasets were conducted. The EFA employed principal axis factoring using responses to the 15 IMRB items from students in a fall 2016 organismal biology course. Data were collected in the semester’s first 2 weeks. Students (n = 267) were instructed to take the IMRB on their own time using personal computers through a Blackboard module as a pretest for an intervention study. The EFA revealed one significant factor, suggesting that the IMRB is a unidimensional assessment, as intended.
The 1-factor CFA was conducted using fall 2017 item response data (n = 1,511) and was determined to fit the data well (RMSEA = 0.018, CFI = 0.987, TLI = 0.985). All 15 items loaded on the latent factor with a standardized loading of 0.400 or higher.
The generalization inference requires the observed score on a single test form be reflective of the expected score on any test form. This can be achieved through task and test specifications that ensure form parallelism, form equating, and scaling procedures that ensure score equivalency. To support this inference, evidence was collected to show that.
• stimuli are selected and items are developed using a similar process to elicit desired inference-making skills,
• the test form construction process results in forms of similar distribution of task types and psychometric properties,
• statistical analyses of observed scores on specific forms show them to be good predictors of expected score on any form, and
• equating and scaling methods accurately place scores from different forms onto a common scale.
IMRB test development follows a principled approach: The stimuli for IMRB test items are taken or modified from a widely used biology textbook (Campbell and Reece, 2001). The stimuli come from a chapter on the immune system, which ensures that the majority of IMRB test-takers are seeing new material.
Participants (n = 91) from an introductory biology course (2008 fall semester) were presented with an illustrated passage from their own biology textbook and were asked to think aloud while learning from the passage during a 40-min session (Cromley et al., 2010). The material was later covered in their course. The sessions were audio-recorded and coded for several strategies (e.g., paraphrasing), verbalizations about vocabulary, prior knowledge activation, word reading, and inferences. Coded inferences were separated into within-text inferences and prior-knowledge-to-text inferences. Both correct and incorrect deductions made by students were included. In writing items, the relevant content was located within the long text that was used to draw correct within-text inferences, and then question stems were written that would require the correct inference. Distractors were developed based on incorrect statements and inferences provided by participants in the think-alouds. Twenty-five items were initially developed and piloted with 737 undergraduate introductory biology students across three semesters (i.e., 2008 fall, 2009 spring, and 2009 fall semesters; see Table 3). Items that did not perform well in reliability analyses were deleted, resulting in the 15 items of the IMRB.
For the development of new IMRB items, these original 15 items were reviewed to uncover content-based perspectives on why these items performed well. Additionally, 86 think-alouds with biology course alumni were conducted on the newly selected passages in the 2017 fall semester. Based on the information collected, item specifications for the development of a new set of 21 items were drafted. A larger item pool allowed for the development of alternate (i.e., parallel) test forms.
The 21 new IMRB items were field tested (2017 fall semester, n = 1,511), and 15 of the best performing items were retained, resulting in a pool of 30 IMRB test items (i.e., 15 original items and 15 new items). From these 30 items, two 15-item test forms were developed. Items were allocated to forms based on IRT item parameter estimates: the difficulty parameter and discrimination parameter. Test characteristic and information curves were simultaneously optimized to reflect the smallest difference between forms. Content experts then reviewed the selected forms and made adjustments to account for the imbalance of items with diagrams and to avoid multiple items coming from a single passage. Once items were selected, they were ordered within the forms to avoid instances of clueing.
The IMRB consists of two test forms, A and B, built to be parallel to one another (scores on Form A are interchangeable/comparable with scores on Form B). Evidence to support comparability comes from the secondary analysis of examinee performance on test forms (2018 spring semester), including a comparison of descriptive statistics and test characteristic curves.
Among essentially equivalent samples, Form A and Form B of the IMRB are comparable with mean test scores of 8.75 (SD = 3.44) for Form A (n = 122) and 8.20 (SD = 3.12) for Form B (n = 118). Cronbach alpha reliability estimates are 0.75 for Form A and 0.71 for Form B. These reliability estimates are modest; typically, reliability estimates of 0.85 or greater are expected, but considering the IMRB is used for making low-stakes decisions and consists of only fifteen items, a reliability of 0.70 or greater is considered adequate (Nunnally, 1978).
Most convincingly, based on the test characteristic curves (see the left panel of Figure 5), interchangeable scores between the two forms are supported—note that the curves plotted are nearly overlapping.
Test forms were developed using preequated IRT parameter estimates. In spring 2018, both test forms were delivered to students in introductory biology courses at two universities. Participants (n = 192) took only one test form, and forms were randomly assigned to participants. Postequating of the forms was conducted (a commonly accepted practice for accurately placing scores for two or more forms onto the same scale). The resulting number correct equivalents between test forms can be provided upon request.
The explanation inference requires that expected scores be attributed to proficiency in the target domain through implementation of appropriate standard setting procedures, test assembly protocols that ensure score precision near cut scores, and item classifications that agree with item performance. Thus, scores are used to classify examinees into categories of performance or ability. To support this inference, evidence needs to be collected to show that.
• the cut score is established through appropriate standard setting or statistical methodologies,
• tests are assembled to provide adequate precision along the score scale near the cut score,
• use of test-based strategies alone do not result in increased likelihood of correct responses, and
• correct responses result from the use of accurate inference-based strategies.
The original goal for the IMRB was to establish a cut score that could serve to classify examinees into one of two categories: likely or unlikely to successfully complete a postsecondary introductory biology course with a grade of C or better. However, after analyzing the semester-end course grades, SAT/ACT scores, and fall 2017 IMRB scores, data revealed that the predictive ability of the IMRB is strongest in combination with SAT/ACT scores. That is, SAT/ACT and IMRB scores have a compensatory relationship with one another, where a low score on one and a high score on the other may still result in successful course completion. Therefore, a single IMRB cut score is not needed to best utilize the IMRB test results. Based on these findings, ordinary least squares (OLS) regression-based prediction models were used to develop a course grade prediction calculator, contained in a spreadsheet, into which an advisor or professor can input various test scores (e.g., IMRB score, SAT/ACT quantitative score, and/or SAT/ACT verbal score). Once scores are input, the calculator applies the most appropriate linear regression weights to predict the final introductory biology course grade for a student.
Items for the test forms were selected to provide approximately similar information curves based on the IRT item parameter estimates used for preequating test forms (see right panel of Figure 5). There is no single cut score that has been identified to determine whether students should be offered remediation or advised to take remedial courses before their introductory biology course. Instead, in most cases, the IMRB can be used in conjunction with SAT/ACT scores to determine the best guidance for individual students. That is, low scores on the IMRB can be compensated with high scores on the SAT/ACT and vice versa. Therefore, either a matrix of cut scores or a course calculator can be used to incorporate available test scores to make student advising decisions. Thus, it is anticipated that precision should be adequate across a range of scores and not particularly peaked at a single cut score. The evaluation of IRT-based information graphics is helpful for determining where along the score continuum the IMRB is most useful.
As observed in the right panel of Figure 5, test forms are most informative between -1.0 and +1.2 theta, which translates to a range of 5–12 number correct score points (see left panel). Thus, scores between 5 and 12 will be the most precise and will be most useful for making decisions about students.
Cognitive think-aloud results from the 2016 fall semester (n = 86) show that the use of test-based strategies alone (e.g., re-reading questions) do not increase the likelihood of correct responses (Cromley et al., 2021). Findings show that using test-based strategies hindered performance and prolonged the time spent choosing a correct response. Test-taking strategies did not result in a greater likelihood of responding correctly to test questions during the think-alouds. In some cases, such strategies resulted in a greater likelihood of responding incorrectly. Thus, test-wiseness does not help a student perform well on the IMRB; rather, as intended, engaging in accurate inference-making and reasoning does help a student perform well. A more detailed discussion of these findings is available (Cromley et al., 2021).
Cognitive think-aloud results from the 2016 fall semester (n = 86) provide support that inference-based strategies increase the likelihood of correct responses (Cromley et al., 2021). Inference-based strategies resulting in accurate inferences did result in a greater likelihood of responding correctly to test questions during the think-aloud sessions.
The extrapolation inference requires that the classification decision be reflected in contexts outside the exam environment through correlations to valid external criteria; that is, assigned classifications should accurately represent the examinee’s skill level on the construct. To support this inference, evidence has been collected to show that.
• assessment tasks adequately reflect performance outside of the testing environment;
• an IMRB score is a predictor of gateway biology achievement;
• an IMRB score is a predictor of retention in STEM;
• other measures of inference-making and reasoning correlate, as theoretically expected, with the IMRB scores; and
• the IMRB is capable of showing growth over a semester.
Outside the IMRB testing environment (i.e., in biology courses), students are asked to read and listen to instructional material. Much of the material must be used for making inferences because explicit connections between concepts are not always made in course readings and lectures. It is this skill that the IMRB is intended to identify and access. In addition to course expectations, results of the student cognitive interviews from the 2016 fall semester (n = 86) show that students are making inferences (Cromley et al., 2021) as intended and expected in their courses. Therefore, the IMRB test questions are designed such that the inference-making skill must be employed to arrive at a correct response.
Course grade in gateway biology courses is the outcome measure used to determine course achievement. Therefore, IMRB student scores should correlate with course grade to be considered a good predictor of achievement. Previous research shows that when the IMRB is given at the beginning of the semester, the score has a significant correlation with the end-of-semester grade (Dai and Cromley, 2014). For instance, scores were significantly correlated with course grade at week 2 (r = 0.27) and week 12 (r = 0.55), with a greater correlation observed toward the end of the semester. Using the 2018 spring semester administrations (n = 196), the correlation between course grade and IMRB scores is 0.20. More factors than the student’s ability to reason with new information inform a final course grade in any course; therefore, this correlation is quite good and expected.
One could argue that the intent of college entrance exam scores (e.g., SAT/ACT) is to predict performance in first-year college courses, making additional assessment unnecessary. However, a series of secondary data analyses collected from research studies using the IMRB shows that use of the IMRB score in regression analyses with other outcome measures results in increased ability to predict course grade. For example, including the SAT Critical Reading score, previous chemistry grade, and prior GPA as predictors of course performance, the IMRB score was still a significant independent predictor, contributing an additional 13.5% of the variance that explained course grade. Additionally, several regression analyses show that the IMRB score explains additional variance (from 0.6 to 14.4%, depending on sample) associated with undergraduate introductory biology course grades above that explained by SAT/ACT verbal and math scores. Across nine samples, the average added value of the IMRB score is the ability to explain 6.5% additional variation in course grade.
One use of the IMRB is identifying and supporting students who may be at risk for dropping out of STEM-related majors. It should follow that if the IMRB score has predictive validity for retention in STEM majors, then the IMRB is useful for identifying students who might be at risk for attrition. Unpublished findings show that students with higher IMRB scores, regardless of whether they took the IMRB at the beginning or end of the semester, tended to self-report that they would likely remain in STEM, while those with lower IMRB scores at either the beginning or end of the semester tended to end the semester by self-reporting that they were unlikely to remain in STEM. Additionally, another study showed that students who began biology with higher IMRB scores (based on the original set of 15 items) were less likely to drop out of STEM majors 2 years later (Dai and Cromley, 2014).
Correlational studies with other tests that measure inference-making and reasoning would help to further support that the IMRB does measure what it intends. Plans for introducing other measures into similar research studies are currently underway. Presently, the lack of this evidence is noted as a limitation.
It is necessary to show that the IMRB provides enough range in item difficulty to measure growth from the beginning to the end of the semester. The 30 IMRB test items range in difficulty along the IRT-based ability metric of −1.18 to 2.66 with a mean of 0.25 (SD = 1.03). This metric (i.e., theta) can be interpreted like a z-score along a normal curve. Results show item difficulty is well dispersed and tends to be slightly more difficult than the ability of average examinees (mean = 0.0, SD = 1.0) to which items were scaled.
The test design needs to allow for precise estimation of performance at both the beginning and end of the semester and should, therefore, include item-selection methods that avoid floor and ceiling effects for test scores. Mean number correct scores during the first 2 weeks of the semester, calculated for spring 2018 examinees (n = 192), are 8.72 (SD = 3.65) for Form A and 8.74 (SD = 3.11) for Form B. This distribution shows that the IMRB is suitable for assessing reasoning skills at the start of a semester. Previous secondary analyses of collected data show that changes in scores from week 2–12 have a large effect on final course grade (b = 2.37). That is, a 1-point score increase was associated with 2.37 percentage points above the course mean of 60.5, suggesting that the IMRB is sensitive to detecting an increase in reasoning skill.
In addition to maintaining parallel test forms, it may also be wise to develop alternate test forms where one is meant to assess incoming students (as a pretest) and the other to assess reasoning skills of students completing their introductory biology course (as a posttest). These pretest and posttest forms would be evaluated to ensure the same construct is measured and calibrated to the same scale of measurement. The forms would be developed so that they would be most informative at different ranges along the scale, better allowing for the precise measurement of growth on the reasoning construct.
The utilization inference requires that estimates of ability (i.e., classification on the IMRB) are useful to the stakeholders for the decisions they make. This requires sufficient understanding by stakeholders of what constitutes valid, as well as limits on, score interpretations and uses such that decisions are beneficial to stakeholders and fair to test-takers. To support this inference, evidence should be collected to show that.
• stakeholders understand the meaning of IMRB test scores, appropriate use and interpretation of those scores, and any limitations on their interpretation and use;
• decisions based on IMRB scores are useful to those making the decisions; and
• decisions based on IMRB scores are fair and just.
The IMRB user manual (Cromley et al., 2020a), with stakeholders as its audience, describes how to appropriately use and interpret IMRB scores. To date, IMRB test data have been used for research purposes only. Once test scores have been used for their primary intended purposes (i.e., course enrollment recommendations, identifying students for intervention/support, and evaluating growth over a semester), significant effort will be placed on ensuring stakeholders understand the utility of the IMRB. For example, IMRB test scores alone should not be used for making high-stakes decisions. Rather, preliminary findings show that SAT/ACT scores used in combination with IMRB scores is a better predictor of final biology course grades. In fact, IMRB and SAT/ACT scores are compensatory to one another (i.e., if a student has a low SAT/ACT score and high IMRB score, the student is likely to still perform well, receiving a C or better in the course). The reverse is also true: Poor performance on one does not dictate poor performance on the other, and strong performance on one can compensate for poor performance on the other. These interactions must be shared and understood by test score stakeholders (e.g., academic advisors). An addendum to the user manual is likely to include a tutorial video to make test score interpretation more transparent. Other supports, like an interactive Excel spreadsheet macro, are being developed for instructors and advisors to use. Such tools would incorporate multiple sources of data for guiding advisement decisions for students.
The IMRB could be instituted at the department or classroom level. At the department level, it is recommended the IMRB be administered (with supervision) before the start of the semester. If the department is unable to accommodate such an administration, the IMRB could be administered the first day or week of class. In either case, a student’s IMRB score should be examined in conjunction with the SAT/ACT verbal score. Advisors and professors should discuss with students who have a low IMRB score or composite (SAT/ACT/IMRB) score that they may encounter coursework challenges, which could result in a nonpassing course grade. It is strongly recommended that advisors and professors, together with these students, construct a proactive mitigation plan to increase the chance for success in the course. Advisors and professors should have a separate discussion with those students whose composite score suggests they could earn a course grade within the B–C range. While a C would allow a student to proceed in their major, it could also indicate the need for a proactive mitigation plan or a shift in the student’s major concentration/emphasis.
Tools are under development to assist advisors and professors with interpreting SAT/ACT verbal scores in combination with IMRB scores. Relevant university personnel will be trained on the use of these tools once they are piloted and approved for use.
The IMRB is intended to help support advising recommendations for course enrollment. However, it is not meant to exclude or prevent students from pursuing their major or field of interest. Ultimately, it is a tool to be used to help students make informed academic decisions.
To prevent adverse impact through advising decisions made based on IMRB scores, it is important to evaluate whether the test items on the IMRB function differentially among subgroups historically impacted. Differential item functioning (DIF) analyses were conducted for samples where important demographic information was available including the 2016 fall semester for the original 15 IMRB items and the 2018 spring semester for the new items added to the IMRB pool. For the original 15 items, no significant DIF between sex, ethnic, or family education level groups was observed. In the analysis of the new items, the original 15 items served as the purified anchor item set (essentially free from bias), where the item parameters remain fixed for all subgroups to stabilize the calibration within smaller subgroup samples for items being tested for DIF. This anchor set was used for testing DIF among the new items using an IRT-based approach, where item parameters are separately calibrated for the respective subgroups and parameter estimates are tested for statistical differences (p < 0.05). This analysis also resulted in an absence of DIF detection, except one item was impossible to calibrate within the Female group due to inconsistent performance and was not used on the final IMRB forms. Thus, the IMRB items chosen for operational administration are free from significant DIF for the identified subgroups of interest.
Cronbach alpha reliability estimates were calculated for each subgroup of interest and test form, Figure 1 and Figure 2 provide a summary. Subgroups of interest include sex, race, and first-generation college student status. Due to the low sample sizes within the subgroups, race can only be disaggregated such that one group represents the reference group (White and/or Asian), and the underrepresented minority group consists of non-White and non-Asian students. A first-generation college student is one who has neither parent that earned a bachelor’s degree or higher level of education. A college student who is not considered first generation has at least one parent that earned a bachelor’s degree or higher level of education. Table 4 provides the disaggregated test form descriptive statistics for each subgroup. While there is variability between subgroups for each statistic (including reliability), the number of examinees available within each subgroup is small, which capitalizes on the sample-dependent nature of these descriptive statistics. Note that when reliability is low (below 0.70) for one subgroup on Form A (0.62 for underrepresented minority students), it tends to be higher (above 0.70) on Form B (0.76 for underrepresented minority students)—again, displaying sample-dependency. When more subgroup data are available, these analyses should be revisited.
The IMRB has undergone several refinements to better support its use for aiding student course recommendations, identifying students in need of academic interventions and support, documenting student growth in reasoning skills, and measuring the success of intervention research. These uses of the IMRB are supported to varying degrees. Figure 1 and Figure 2 provides a summary of the validation research findings, including the five strongest supporting arguments:
1. The construct of reasoning is clearly defined.
2. The use of cognitive think-alouds aided in the development of strong multiple-choice items that draw upon the misconceptions and inaccurate inferences that undergraduate introductory biology students make.
3. The use of cognitive think-alouds revealed that accurate inference use increased a student’s chance of responding correctly to items.
4. There is a positive correlation between IMRB score and retention in STEM after 2 years.
5. The IMRB score has good strength as a predictor of biology course grade.
These arguments provide strong support for the use of the IMRB as an aid (in conjunction with other measures) for advising students on course placement for biology courses and for identifying students in need of intervention. While the IMRB might be used to assess the success of interventions and growth on reasoning skills, more analysis and test refinement is needed to provide confidence in these uses; and thus could be considered a use case that is still under investigation. To address some of the limitations of the IMRB validity argument discussed throughout this manuscript further analyses and supplemental test development processes could include the following activities:
1. Investigate how different test administration conditions may affect student motivation and have an impact on the utility of the IMRB.
2. Consider constructing alternative test forms that sample other domains.
3. Develop test forms of varying difficulty to aid in growth assessment.
4. Conduct correlational studies of the IMRB with other measures of reasoning skills.
5. Train advisors and other stakeholders on appropriate uses of IMRB test scores.
Validation efforts for any measure are ongoing, and these additional studies and processes are recommended to provide further support for IMRB development, improvement, and proper use.
In conclusion, students who are more adept at drawing inferences from material learned in classes and from textbooks are likely forming situation models to represent text and are more equipped to successfully complete their STEM-related majors. Thus, we have created two test forms of the Inference-Making and Reasoning in Biology (IMRB) measure to assess students’ inference-making skills with the goal of identifying students who may need more support or interventions to enhance their inference-making skills and increase the likelihood of completing their STEM-related major.
For the IMRB measure, we have gathered a multitude of compelling evidence to support its reliability, fairness, and validity for four purposes. Cognitive interviews and predictive regression data support the use of the IMRB for academic advising and course placement. The measure does not require knowledge beyond basic biology facts, students answer with moderate internal consistency, and the measure is fair across race, sex, and socioeconomic groups. We believe the IMRB will be useful to biology instructors and advisors at 4-year university and college settings, as well as to science education researchers.
The IMRB could be useful at either the department or classroom level. The IMRB is best administered via a proctored setting before the start of the semester (or within the first week of class). A student’s IMRB score could be reviewed alongside verbal SAT/ACT scores, if available. For students with low IMRB scores or a composite (SAT/ACT and IMRB combination) indicating a possible nonpassing course grade, advisors and professors should discuss with students the possibility that they may encounter coursework challenges that could result in a nonpassing course grade. It is strongly recommended that advisors and professors, together with these students, construct a proactive mitigation plan to increase the chance for success in the course. As evidence of feasibility, one university is actively trialing this level of academic advisement with over 1,000 entering biology majors each year. There are some interventions within the literature that may be considered and include elaborative interrogation (Seifert, 1993), worked examples (Dyer et al., 2015), prescribed active learning (Freeman et al., 2007), and direct training on how to make inferences (Elleman, 2017). More research in this area is encouraged.
For researchers and test developers, we show, by example, how an argument-based approach to validity can be structured to support intended score interpretations and uses. We encourage other test developers to adopt similar approaches: The supporting evidence for the use of a measure can be read in a logical, transparent, and narrative form that leads the reader to a comprehensive understanding of proper assessment use with healthy skepticism.
Data is available upon request by contacting JC at amNyb21sZXlAaWxsaW5vaXMuZWR1.
The use of human subjects in this study complied with all relevant federal guidelines and institutional policies regarding informed consent and confidentiality. All procedures in studies involving human participants were performed in accordance with the ethical standards of the institutional and/or national research committee and with the 1964 Helsinki Declaration and its later amendments or comparable ethical standards. The studies were reviewed and approved by each institution’s institutional review board (IRB): IRB number 16896 and IRB number 23848. Informed consent was obtained from all individual participants included in the studies.
TF designed the validity argument framework. JC led the conception and design of the IMRB measurement instrument. FN supplied content expertise during the IMRB development. All authors contributed to the design and implementation of measure evaluation and validity studies. YD organized the database. TF wrote the first draft of the manuscript. JG, FN, TD, and TF wrote sections of the manuscript. All authors contributed to manuscript revision, read, and approved the submitted version.
This research was funded by the Institute of Education Sciences, U.S. Department of Education, Grant R305A160335 to University of Illinois, Urbana-Champaign. The opinions expressed are those of the authors and do not represent views of the Institute or the U.S. Department of Education.
The authors declare that the research was conducted in the absence of any commercial or financial relationships that could be construed as a potential conflict of interest.
All claims expressed in this article are solely those of the authors and do not necessarily represent those of their affiliated organizations, or those of the publisher, the editors and the reviewers. Any product that may be evaluated in this article, or claim that may be made by its manufacturer, is not guaranteed or endorsed by the publisher.
We thank the professors who allowed us to administer the IMRB to their students, and those who provided feedback to inform the IMRB refinement and development of score interpretation tools. We also thank the students who voluntarily consented to participate in the research to develop and refine the IMRB.
1Illustration from Anatomy and Physiology by J. G. Betts, K. A. Young, J. A. Wise, E. Johnson, B. Poe, D. H. Kruse, O. Korol, J. E. Johnson, M. Womble, and P. DeSaix, 2013. Houston, TX: OpenStax (https://openstax.org/books/anatomy-and-physiology/pages/18-4-leukocytes-and-platelets) Betts et al., 2013. Illustration used and modified under CC BY 4.0 license. The authors of this manuscript added text below the illustration to provide an example of a test question that might accompany a graphic-based stimulus
American Educational Research Association, American Psychological Association, and National Council on Measurement in Education (2014). Standards for Educational and Psychological Testing. Washington, DC: American Psychological Association.
Bachman, L. F. (2005). Building and Supporting a Case for Test Use. Lang. Assess. Q. 2, 1–34. doi:10.1207/s15434311laq0201_1
Bachman, L. F., and Palmer, A. S. (2010). Language Assessment in Practice: Developing Language Assessments and Justifying Their Use in the Real World. Oxford: Oxford University Press.
Betts, J. G., Young, K. A., Wise, J. A., Johnson, E., Poe, B., Kruse, D. H., et al. (2013). Anatomy and Physiology. Houston, TX: OpenStax. Available at: https://openstax.org/books/anatomy-and-physiology/pages/18-4-leukocytes-and-platelets.
Butcher, K. R. (2006). Learning from Text with Diagrams: Promoting Mental Model Development and Inference Generation. J. Educ. Psychol. 98 (1), 182–197. doi:10.1037/0022-0663.98.1.182
Chapelle, C. A., Enright, M. K., and Jamieson, J. (2010). Does an Argument-Based Approach to Validity Make a Difference? Educ. Meas.-Issues. Pra. 29 (1), 3–13. doi:10.1111/j.1745-3992.2009.00165.x
Chapelle, C. A., and Voss, E. (2014). “Evaluation of Language Tests Through Validation Research,” in The Companion to Language Assessment. Editor A. J. Kunnan (Chichester: Wiley), 1079–1097. doi:10.1002/9781118411360.wbcla110
Cleary, T. J., Velardi, B., and Schnaidman, B. (2017). Effects of the Self-Regulation Empowerment Program (SREP) on Middle School Students' Strategic Skills, Self-Efficacy, and Mathematics Achievement. J. Sch. Psychol. 64, 28–42. doi:10.1016/j.jsp.2017.04.004
Cromley, J. G., Bergey, B. W., Fitzhugh, S., Newcombe, N., Wills, T. W., Shipley, T. F., et al. (2013). Effects of Three Diagram Instruction Methods on Transfer of Diagram Comprehension Skills: The Critical Role of Inference while Learning. Learn. Instruction. 26, 45–58. doi:10.1016/j.learninstruc.2013.01.003
Cromley, J. G., Dai, T., Fechter, T., Van Boekel, M., Nelson, F. E., and Dane, A. (2021). What Cognitive Interviewing Reveals About a New Measure of Undergraduate Biology Reasoning. J. Exp. Education. 89 (1), 145–168. doi:10.1080/00220973.2019.1613338
Cromley, J. G., Fechter, T. S., Dai, T., and Nelson, F. (2020a). Inference-Making and Reasoning in Biology (IMRB) User Manual & Technical Report, Urbana: US Department of Education, Institute for Education Sciences.
Cromley, J. G., Perez, T., Kaplan, A., Dai, T., Mara, K., and Balsai, M. J. (2020b). Combined Cognitive-Motivational Modules Delivered via an LMS Increase Undergraduate Biology Grades. Technol. Mind, Behav. 1 (2). doi:10.1037/tmb0000020
Cromley, J. G., Snyder-Hogan, L. E., and Luciw-Dubas, U. A. (2010). Cognitive Activities in Complex Science Text and Diagrams. Contemp. Educ. Psychol. 35, 59–74. doi:10.1016/j.cedpsych.2009.10.002
Dai, T., and Cromley, J. G. (2014). Changes in Implicit Theories of Ability in Biology and Dropout from STEM Majors: A Latent Growth Curve Approach. Contemp. Educ. Psychol. 39 (3), 233–247. doi:10.1016/j.cedpsych.2014.06.003
Dai, T., Van Boekel, M., Cromley, J., Nelson, F., and Fechter, T. (2018). Using Think-Alouds to Create a Better Measure of Biology Reasoning. SAGE Res. Meth. Cases. doi:10.4135/9781526437167
Dyer, J. O., Hudon, A., Montpetit-Tourangeau, K., Charlin, B., Mamede, S., and van Gog, T. (2015). Example-Based Learning: Comparing the Effects of Additionally Providing Three Different Integrative Learning Activities on Physiotherapy Intervention Knowledge. BMC Med. Educ. 15, 37. doi:10.1186/s12909-015-0308-3
Elleman, A. M. (2017). Examining the Impact of Inference Instruction on the Literal and Inferential Comprehension of Skilled and Less Skilled Readers: A Meta-Analytic Review. J. Educ. Psychol. 109, 761–781. doi:10.1037/edu0000180
Freeman, S., O'Connor, E., Parks, J. W., Cunningham, M., Hurley, D., Haak, D., et al. (2007). Prescribed Active Learning Increases Performance in Introductory Biology. CBE Life Sci. Educ. 6, 132–139. doi:10.1187/cbe.06-09-0194
Graesser, A., and Britton, B. K. (1996). “Five Metaphors for Text Understanding,” in Models of Understanding Text. Editors B. K. Britton, and A. Graesser (Mahwah, NJ: Erlbaum), 341–351.
Gurlitt, J., and Renkl, A. (2010). Prior Knowledge Activation: How Different Concept Mapping Tasks lead to Substantial Differences in Cognitive Processes, Learning Outcomes, and Perceived Self-Efficacy. Instr. Sci. 38 (4), 417–433. doi:10.1007/s11251-008-9090-5
Guthrie, J. T., Wigfield, A., Barbosa, P., Perencevich, K. C., Taboada, A., Davis, M. H., et al. (2004). Increasing Reading Comprehension and Engagement Through Concept-Oriented reading Instruction. J. Educ. Psychol. 96 (3), 403–423. doi:10.1037/0022-0663.96.3.403
Im, G.-H., Shin, D., and Cheng, L. (2019). Critical Review of Validation Models and Practices in Language Testing: Their Limitations and Future Directions for Validation Research. Lang. Test. Asia 9 (14). doi:10.1186/s40468-019-0089-4
Kane, M. T. (2013). Validating the Interpretations and Uses of Test Scores. J. Educ. Meas. 50, 1–73. doi:10.1111/jedm.12000
Kane, M. (2006). “Validation,” in Educational Measurement. Editor R. L. Brennan. 4th ed. (Washington, DC: American Council on Education/Praeger), 17–64.
Kintsch, W. (1998). Comprehension: A Paradigm for Cognition. New York, NY: Cambridge University Press. Available at: https://psycnet.apa.org/record/1998-07128-000
Lawson, A. E., Banks, D. L., and Logvin, M. (2007). Self-efficacy, Reasoning Ability, and Achievement in College Biology. J. Res. Sci. Teach. 44 (5), 706–724. doi:10.1002/tea.20172
McNamara, D. S. (2004). SERT: Self-Explanation Reading Training. Discourse Process. 38 (1), 1–30. doi:10.1207/s15326950dp3801_1
Messick, S. (1989). “Validity,” in Educational Measurement. Editor R. L. Linn. 3rd ed. (New York: American Council on Education & Macmillan), 13–103.
Mislevy, R. J. (1996). Test Theory Reconceived. J. Educ. Meas. 33, 379–416. doi:10.1111/j.1745-3984.1996.tb00498.x
Mislevy, R. J., Wilson, M. R., Ercikan, K., and Chudowsky, N. (2002). Psychometric Principles in Student Assessment (CSE Technical Report 583). Los Angeles, CAStandards, and Student Testing: University of California Center for the Study of Evaluation, National Center for Research on Evaluation Graduate School of Education & Information Studies.
National Science Foundation (2006). Women, Minorities, and Persons with Disabilities in Science and Engineering. Available at: https://www.nsf.gov/statistics/2017/nsf17310/.
Pintrich, P. R. (2000). “The Role of Goal Orientation in Self-Regulated Learning,” in Handbook of Selfregulation. Editors M. Boekaerts, P. Pintrich, and M. Zeidner (Academic Press), 451–502. doi:10.1016/b978-012109890-2/50043-3
Royer, J. M., Greene, B. A., and Sinatra, G. M. (1987). The Sentence Verification Technique: A Practical Procedure for Testing Comprehension. J. Reading 30 (5), 414–422. Available at: https://www.jstor.org/stable/40029713.
Sato, B. K., Hill, C. F. C., and Lo, S. M. (2019). Testing the Test: Are Exams Measuring Understanding? Biochem. Mol. Biol. Educ. 47 (3), 296–302. doi:10.1002/bmb.21231
Seifert, T. L. (1993). Effects of Elaborative Interrogation With Prose Passages. J. Educ. Psychol. 85, 642–651. doi:10.1037/0022-0663.85.4.642
Keywords: reasoning, biology, validation, retention, stem, assessment, academic guidance, inference-making
Citation: Fechter T, Dai T, Cromley JG, Nelson FE, Van Boekel M and Du Y (2021) Developing a Validity Argument for an Inference-Making and Reasoning Measure for Use in Higher Education. Front. Educ. 6:727539. doi: 10.3389/feduc.2021.727539
Received: 18 June 2021; Accepted: 23 August 2021;
Published: 16 September 2021.
Edited by:
Elizabeth Archer, University of the Western Cape, South AfricaReviewed by:
Prashant Mahajan, R. C. Patel Institute of Technology, IndiaCopyright © 2021 Fechter, Dai, Cromley, Nelson, Van Boekel and Du. This is an open-access article distributed under the terms of the Creative Commons Attribution License (CC BY). The use, distribution or reproduction in other forums is permitted, provided the original author(s) and the copyright owner(s) are credited and that the original publication in this journal is cited, in accordance with accepted academic practice. No use, distribution or reproduction is permitted which does not comply with these terms.
*Correspondence: Tia Fechter, dGlhY29ybGlzc0Bob3RtYWlsLmNvbQ==
Disclaimer: All claims expressed in this article are solely those of the authors and do not necessarily represent those of their affiliated organizations, or those of the publisher, the editors and the reviewers. Any product that may be evaluated in this article or claim that may be made by its manufacturer is not guaranteed or endorsed by the publisher.
Research integrity at Frontiers
Learn more about the work of our research integrity team to safeguard the quality of each article we publish.