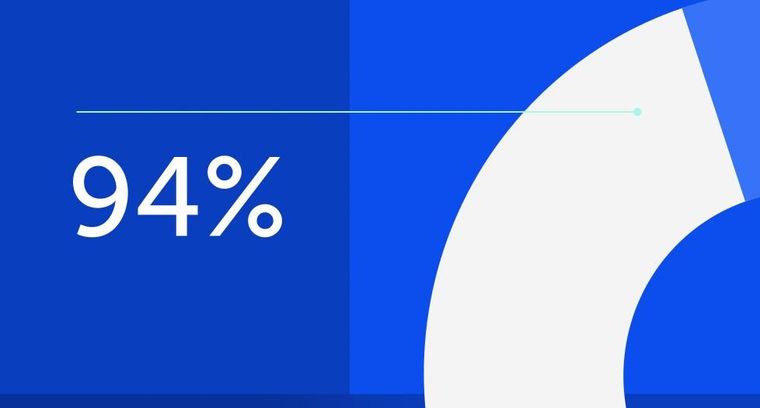
94% of researchers rate our articles as excellent or good
Learn more about the work of our research integrity team to safeguard the quality of each article we publish.
Find out more
ORIGINAL RESEARCH article
Front. Educ., 22 July 2021
Sec. Educational Psychology
Volume 6 - 2021 | https://doi.org/10.3389/feduc.2021.719539
This article is part of the Research TopicCOVID-19: Mid- and Long-Term Educational and Psychological Consequences for Students and EducatorsView all 18 articles
The American Psychological Association (APA) reports 81% of Gen Z teens (ages 13–17) have experienced more intense stress during the COVID-19 pandemic. This study uses a survey-based approach along with robust statistical analyses to identify key stressors from a set of students in a high school in Midwest United States. Our survey includes a broad range of stressors (15 explanatory variables) specific to high schoolers, controls (4 factors for pre-existing conditions), and mental health estimators (7 dependent variables) to identify changes in mental wellbeing during the pandemic. The results (n = 107) show good consistency in our estimators (Cronbach’s α = 0.78) and statistically significant (t = 0.636, p ≪ 0.001) degradation in the mental health. Correlation (r = 0.2, p = 0.034) and regression analysis showed that online learning (β1 = -0.96, p = 0.004) has the most influence on degradation in mental health, with some race-based differences. Exercise time helps reduce mental health degradation (β3 = -0.153, p = 0.037). Many other factors such as gender, homework time, school time, pre-existing mental health issues, and therapy did not have a significant influence on mental health degradation. Analysis of freeform feedback identified the following three recurring themes: increased stress due homework (13.2%), social isolation or lack of social interactions (8.5%), and lack of support for mental wellbeing (12.3%).
The COVID-19 pandemic has had a significant worldwide impact on the lives of more than 1.6 billion students at its peak (UNESCO 2021) and their schooling. Similar to many other countries, the United States also pursued a policy to lockdown schools, starting in mid-March 2020, to mitigate the pandemic. The lockdown caused the schools to rapidly adopt and pursue remote learning using video conferencing methods. The teachers, students, and their families have experienced several different challenges, including increased stress and anxiety that has led to degradation of mental health. The issues have been further compounded by stringent social distancing measures necessitated due to the lack of vaccines and high mortality rates (over two million deaths worldwide).
The issue of degrading mental health due to pandemics has been reported in the past (Hawryluck et al., 2004; McAlonan et al., 2007; Lau et al., 2010; Xiang et al., 2014). The circumstances surrounding the COVID-19 pandemic have increased the stress and anxiety in people (APA 2020; Tandon 2020; Wang et al., 2020), including high school students. Liang et al. (2020) report a much higher fraction of youth with psychological issues during the COVID-19 pandemic when compared to prior pandemics. The American Psychological Association (APA) reports [American Psychological Association (APA), 2020] that nearly 81% of Gen Z teens Gen Z teens (ages 13–17) experience more intense stress due to COVID-19, associated with schooling. However, APA’s report does not delve into the specific details of the stressors arising from the pandemic-related changes in schooling. The issues related to schooling and their impacts have been reported in many countries (Dolean and Lervag, 2021).
China was the first country to be impacted by the COVID-19 pandemic. Hence, several studies analyzing the impact of the pandemic on the mental health of students have been reported. Hou et al. (2020) discuss the issue of mental health problems and suicidality among senior high school students in China during the COVID-19 pandemic. Their study involved 859 high school students (61.4% male and 79.4% below 16 years of age). Their results showed that depression, anxiety, PTSD, and suicidal ideation, and suicidal attempts were 71, 54.5, 85.5, 31.3, and 7.5% respectively. However, their study did not specifically focus on specific stressors, though they surmise that educational pressure, pronged school closure, and social stigma of COVID infection might be stressors. Nevertheless, they report that higher exercise frequency, a control in our study, correlated with fewer symptoms of depression and anxiety. Another cross-sectional study of 532 Chinese high school students by Zhang et al. (2020) used three types of questionnaires from prior studies and showed that nearly 20% of the students’ mental health was impacted. Their analysis showed that resilience and positive coping were protective factors against depression, anxiety and stress symptoms. Another cross-sectional study (Liang et al., 2020) of 584 youth (age 14–35) in China showed that 40.4% were prone to psychological problems and 14.4% had PTSD symptoms. In the context of COVID-19, their study suggested that mental health was related to educational level, employment, and using negative coping styles.
Asanov et al. (2021) discuss results from a cross-sectional survey of over 1,500 high school students (age 14–18) in Ecuador. Their survey has been conducted via phone interviews with the participants, focusing on topics related to access to remote learning technologies and their impacts. They used the MHI-5 index proposed by Veit and Ware (1983) that uses a five-point Likert-like scale to assess their mental health along with a cutoff score of 17 (max score of 25), with higher scores indicating better mental health. Their survey found 16% of participants had scores that indicate major depression. Similarly, a longitudinal study involving 442 last year high school in Greece by Giannopoulou et al. (2021) showed an 15.3% increase in depression, 17% increase in severe depression, 25.7% increase in anxiety, and 16.7% increase in severe anxiety after just one month of lockdowns. They use Generalized Anxiety Disorder Scale (GAD-7), which is a 7-item questionnaire with each item using a 4-point Likert-type scale to assess anxiety. They used a modified version of the Patient Health Questionnaire-9, that also uses a 4-point Likert-like scale, to assess depression.
Similar to the works of Asanov et al. (2021) and Giannopoulou et al. (2021), our study also used a 5-point Likert-like scale to assess the mental health of high school students. Although our survey is not longitudinal, it includes self-reported mental health scores before and during the pandemic, giving it some longitudinal characteristics. Since the survey was conducted during the pandemic, the self-reported mental health scores prior to the pandemic are subject to recall bias, similar to the analysis reported by Giannopoulou et al. (2021). Moreover, similar to Giannopoulou et al. (2021) we have also used Cronbach’s alpha to establish good internal consistency of the survey results, which mitigates some of recall bias and increases confidence in our results and analysis.
The aforementioned investigations on COVID-19 pandemic and its impact on mental health have focused on more general aspects of mental health. They identify some of the socioeconomic stressors and their impact on anxiety and depression. They don’t delve into the specific stressors arising from the pandemic-related changes in schooling. In contrast, this study emphasizes several key stressors and controls along with their influence on mental health of high school students. Such a detailed analysis is exigent to identify and mitigate the impact of the stressors in order to improve the mental wellbeing of high school students. Our objective is to focus on the common stressors experienced by high school students. Hence, several stressors and controls were identified via a focus group of students and teachers. In addition, we include several controls to account for existing mental health issues. Our main research question is – has the mental health of high school students changed during the COVID-19 pandemic and what factors have contributed to the change in mental health? Moreover, we also aimed to elicit potential approaches to mitigate degradation in mental health from the participants.
This study uses a well-established scientific method for conducting a descriptive and exploratory research using a survey-based approach (Bhattacherjee 2019). An overview of the method is summarized in Figure 1. The first phase of the investigation focused on eliciting the three main categories of variables, namely ① stressors (independent variables) that influence mental health, ② controls to account for any pre-existing mental health conditions, and ③ mental health estimators. This phase of the research first involved literature survey of peer reviewed articles to identify key variables to be surveyed. The APA surveys (APA 2020) did not provide many parameters but suggested time invested on key activities such as school work, leisure, sports, and sleep. We also reviewed several instruments proposed in the literature for mental health assessments including MIH-5 (Viet and Ware, 1983), GAD-7 (Spitzer et al., 2006), PHQ-9 (Johnson et al., 2002).
At the time of this study, there was a paucity of technical articles pertaining to COVID-19 pandemic and its impact on mental health. Hence, we decided to pursue a focus group approach to obtain community input to elicit parameters and appropriately structure questions. The focus group consisted of a few high school students and two teachers. The focus group also motivated us to keep the questionnaire as short as possible and structure questions to be quickly understandable by students. Based on the focus group feedback, we have structured our mental health estimators along the lines of MIH-5, though our questions are different and included six questions, based on focus group feedback.
The results from the literature survey and focus group analysis led to 29 questions pertaining to stressors, controls, and mental health estimators as summarized in Table 1. The complete survey administered to the participants is included in the supplements. Our survey started with a consent question (not shown in Table 1). The questions covered 15 stressors (i.e., independent variables) that influence mental health and were identified via literature survey (APA, 2020; Zhang et al., 2020; Asanov et al., 2021). In addition, the questions included stressors related to online vs. in-person learning that were identified via our focus group discussions. Our instrument included four controls to account for any pre-existing mental health issues that could be unrelated to COVID-19, but could be exacerbated due to the pandemic. The survey then included questions on mental health estimators to assess the mental wellbeing before and during the pandemic (Liang et al., 2020). The survey was administered during the pandemic and hence, the question on mental health prior to the pandemic is subject to recall bias, similar to other investigations (Giannopoulou et al., 2021). Lastly, the survey included freeform questions for participants to share their opinions on how their mental health could be improved.
TABLE 1. Category and summary of parameters included in our instrument. Full questionnaire is included in the supplementary materials.
The survey was designed to be administered to students in a high school in Mason, Ohio (Mid-West, United States). The survey questionnaire and protocol were approved by the school’s Institutional Review Board (IRB). After IRB approval was obtained, consent was also sought from the school’s administration. The school’s administration restricted us to administer the survey to a subset of high school students. The administration did not provide an explanation for their decision to restrict the survey to only a subset of students.
As part of the IRB approval, the school’s administration restricted us to administer the survey to a subset of students who were enrolled in the biomedical science program in the high school. As a result, the sample size (n = 107) of our data is somewhat limited. Nevertheless, the dataset has sufficient statistical power as discussed in the results section.
The survey was designed as a web-based (fully anonymized) survey using Google Forms (Google Inc, 2021) to ease distribution and completion. The survey link was shared with the students with the help of teachers in the biomedical science program in the high school. We also requested the teachers to periodically remind the students to complete the voluntary survey. The fully anonymized web-based survey was made available to participants for 30 days (Dec 1 to Dec 30, 2020). Participation was voluntary and no incentives (money, prize, extra credit, etc.) were provided to the participants. The fully anonymized data was obtained from Google Forms at the end of the survey time and stored safely in a password protected CSV file for further analysis.
The survey results were obtained from Google Forms as a Comma Separated Values (CSV) file. The data was analyzed using R statistical software (Core Team, 2020) and Microsoft Excel. As summarized in Figure 1, first we established internal consistency of the mental health estimators (dependent variables) using Cronbach’s alpha. Once we established good internal consistency, we tried to reduce the number of independent variables using Principal Component Analysis (PCA) (Jolliffe and Cadima, 2016).
To ease further analysis, we have also encoded the categorical questions to numeric values based on the Likert-like scales used for each question. In addition, to streamline analyses, we have added a dependent intermediate variable indicating change in mental health (Mt.Health.Change), which is computed as shown in the equation below.
In accordance with the high school administration’s conditions, the fully anonymous survey was made available to high school students (age 15–17) enrolled in the biomedical sciences program. The survey was made available for 30 days (from Dec 1–Dec 30, 2020). The voluntary survey was completed by 107 students whose demographics are summarized in Figure 2. The respondents were mostly Asian students (69 of 107 ∼ = 64%) and most of the students were female (75 of 107 ∼ = 70%). At the time of the survey, 80 of 107 participants (about 74.8%) were taking classes in-person while 27 participants (about 25.2%) were taking classes online. Out of the 107 participants, 102 participants were learning in their modality of choice (i.e., in-person or online) and had not recently switched learning modality. Four participants were considering switching learning modalities and only one participant had switched from online to in-person schooling.
Our survey questionnaire requested time (in hours) spent on several key activities related to schooling each day, including: time spent in school (online or in-person), average time spent on homework, approximate time spent in exercising or sports, and average sleeping time. We solicited this data because time pressure was reported as a key stressor during our focus group discussions. In our study we aimed to delve into specific categories of time use to identify the most influential time-related stressor, if any. The summary statistics for the time usage is shown in Figure 3. Only 14 out of the 107 students (i.e., ∼13%) were not involved in a student club or an honors society.
Prior to statistical analysis, the internal consistency, validity and reliability of mental health estimators (dependent variables) in the survey data was verified and using the well-established Cronbach’s alpha (Taber, 2018). Cronbach’s alpha requires that the data must all be on a consistent scale. Hence, for our alpha calculations, we have used the mental health change (Eq. 1) intermediate variable along with the estimators “Increased anxiety”, “Increased stress”, “Issues with daily tasks?”, and “Impact on relationships?” from Table 1. For the mental health estimators, the Cronbach’s alpha was α = 0.78, which in social sciences is considered a good value (Taber, 2018). Other investigations related to COVID-19 that used other instruments have reported comparable scores for Cronbach’s alpha: 0.79 for GAD-7 (Liang et al., 2020), 0.81 for GAD-7 (Giannopoulou et al., 2021), and 0.79 for GHQ-12 (Liang et al., 2020) to mention a few (Tavakol and Dennick, 2011). The results from our Cronbach’s alpha establish consistency, validity, and reliability of the responses. In other words, the α = 0.78, shows that the mental health assessment instrument was well designed, is unidimensional (i.e., focusing on mental health), and the responses are meaningful (i.e., students were not randomly or nonchalantly filling-in the survey, but were responding conscientiously).
An overall key question in this survey was to determine if students experienced any degradation in their mental health. In our survey, 24 out of 31 (77.4%) of White students and 37 out of 69 (53.6%) Asian students, two of 2 (100%) Hispanic/Latino, and one of 2 (50%) Black participants reported some degradation in mental health. Figure 4 shows a comparison of the distributions of mental health status reported by the participants before and during the COVID-19 pandemic. The boxplots in Figure 4B show a comparison of the mental health ratings. We conducted a paired two-sample t-test comparing the mental health of participants before and during the pandemic (see Table 1). We also verified that the change in mental health was normally distributed (a prerequisite for the t-test) using the Shapiro-Wilk normality test (W = 0.944, p-value = 0.00025). The t-test showed a statistically significant mental health degradation of 0.636 (p ≪ 0.001). Of the 64 participants who reported degradation in mental health during the pandemic, 45 (70%) of them were enrolled in online schooling. The proportions of males and females reporting mental health degradation was 18 (28%) and 45 (70%) respectively. Amongst the 64 participants, 24 were White and 37 were Asian. Therapy was followed by nine and six participants who reported degradation and no degradation respectively.
FIGURE 4. Mental health ratings reported on a 5-point Likert-scale by the survey respondents before and during the COVID-19 pandemic. Paired t-test showed a statistically significant degradation (the changes were normally distributed -- Shapiro-Wilk test W = 0.944, p-value = 0.00025) in mental health ratings with t = 0.636 (p ≪ 0.001). (A) Histogram of Mental Health ratings before and during the pandemic. (B) Box plots comparing the ratings before and during the pandemic.
Having established a good value of Cronbach’s alpha for mental health estimators, we focused on analysis of stressors (independent variables) and controls (pre-existing conditions). This part of the analysis aimed to identify influential parameters and reduce the number of parameters to be addressed in further analyses. Accordingly, we have used the widely used Principal Component Analysis (PCA) (Jolliffe and Cadima, 2016), to explore the feasibility of dimensionality reduction – i.e., check if some of the controls and stressors are redundant and can be discounted in further analyses. The key results from PCA are summarized in Figure 5. The Scree plot in Figure 5A shows that all of the parameters need to be included in order to account for 95% of the variance in the data. The monoplot also shows that all of the parameters have substantial contribution to the first two dimensions in PCA. In other words, the PCA results showed that our survey has very desirable features in that all of the stressors (independent variables) and controls are relatively uncorrelated and provide statistically significant information regarding each participant.
FIGURE 5. Results from Principal Component Analysis (PCA). (A) Scree Plot from PCA. (B) Monoplot from PCA.
Since PCA showed that all of the stressors (independent variables) and controls (pre-existing conditions) cannot be reduced, we pursued extensive correlation analysis to estimate their influence on mental health estimators (dependent variables). The analysis was conducted using R statistical software. Figure 6 shows a subset of results from correlation analysis with stressors (independent variables) and controls (pre-existing conditions) that showed statistically significant correlation with “Mt.Health.Change” intermediate variable (Eq. 1). The full correlogram with all stressors (independent variables), controls (pre-existing conditions), and mental health estimators (dependent variables) is included in the supplements. The upper right triangle of the correlogram shows the Spearman correlation values. Interestingly, for this data set, the Pearson correlation coefficients were exactly the same as Spearman coefficients, indicating that there is both a monotonic and linear relationships between the parameters.
FIGURE 6. Key subset of results from correlation analysis. The values in the upper-right triangle show the Spearman correlation between pairs of values. For example, 0.19 (p = 0.049) shows the correlation between Race (the row) and Mt.Health.Change (column). The red lines in the scatter plots show the Lowess curve to highlight correlation patterns within sub-ranges of data. The full correlogram with all stressors (independent variables), controls (pre-existing conditions), and mental health estimators (dependent variables) is included in the supplementary materials.
The key intermediate variable used for analysis was “MT.Health.Change”, which shows change in mental health. The correlation analysis showed that the controls (i.e., pre-existing mental health issues) had no correlation (p > 0.1) to change in mental health. Among the various stressors, only “In-person vs. Online” learning modality and race showed statistically significant correlation r = -0.20 (p = 0.034) and r = 0.19 (p = 0.049) to mental health degradation. That is, students learning online reported a statistically significant degradation in mental health. A large fraction (77.4%) of White participants reported some mental health degradation, and hence some correlation with race was observed. Sleeping time (Sleep.Time) and exercise Time (PT.Time) stressors showed no statistically significant correlation to change in mental health. However, these two stressors had a good negative correlation to increase in anxiety and stress -- that is, participants who spent more time sleeping or exercising reported lower stress and anxiety changes. These observations regarding the benefits of sleep and exercise are consistent with findings reported by other investigators (Liang et al., 2020; Giannopoulou et al., 2021).
The correlation analysis showed that pandemic-time degradation in mental health (i.e., the Mt.Health.Change intermediate variable) is correlated only to learning modality (In-person vs. online) and the race of students. Other stressors did not show any influence on changes in mental health during the pandemic. We have cross validated this inference by identifying the best-fitting generalized regression model that explains the observations in mental health changes during the COVID-19 pandemic. For this analysis we have removed one outlier participant who was taking most of classes via the College Credit Plus (CCP) program. The best fitting regression model (Johnson and Wichern, 2015) was determined using R statistical software which identifies parameters that minimize the Akaike Information Criterion (AIC) (Rabbi et al., 2020). The model automatically identified included a School.Time parameter that was not significant (p >> 0.05). When we removed it from the regression model, the Switched parameter was no longer significant (p >> 0.05). Hence, these two parameters have been removed from our final regression model as they were not statistically significant. The results from multivariate regression analysis using the best fitting model are shown in Figure 7.
FIGURE 7. Regression results from best fitting generalized regression model. The best fitting regression model was determined using R statistical software which identifies parameters that minimize AIC.
Consistent with the correlation analysis, changes in mental health is best explained by online learning and race. Online learner’s mental health degraded by about −0.9 (on a 5-point Likert scale) (p = 0.004) while Asian participants reported lower degradation of 0.89 (p = 0.003) when compared to other races. Exercise time (PT.Time) had only mild protective influence of 0.15 (p = 0.037) on the mental health of participants. This is corroborated by the correlation analysis (Figure 6) where PT.Time and Therapy was only correlated to stress and anxiety levels, but not on changes in mental health. Controlling for gender did not have any impact (p-value >> 0.05) showing that mental health issues were equally experienced by all of the genders in our survey.
Our regression analysis identified three explanatory parameters, namely learning modality (in-person vs. online), race, and PT.Time. The first two parameters were also highlighted by correlation analysis. In order to assess the influence of PT.Time, we compared the explanatory power with and without PT.Time. We also chose this minimal subset to avoid overfitting our regression model to the survey data. Figure 8 shows a comparison of the predictions of the full regression model vs. the simpler model. Note that, in both the models, we are attempting only to predict degradation (i.e., Mt.Health.Change <0), if any, and not the magnitude of degradation.
FIGURE 8. Confusion matrix to compare the effectiveness of a regression model using just two explanatory variables, namely: learning-modality (In-person vs. online) and Race. (A) Confusion matrix with 4 factors: Learning modality, Race, Therapy, and PT. Time. (B) Confusion matrix with just 2 factors: Learning modality and Race.
The confusion matrix shows that overall, this simple model with the two explanatory variables (see Table 1 for full list) can predict potential mental health issues with an accuracy 62.6% (p = 0.003). On the other hand, inclusion of PT.Time in the regression model improves the accuracy to 72.0% (p << 0.001) by primarily decreasing the number of false positives (FP) from 40 to 25.
The biomedical science program is an elective program in the high school. Hence, the proportion of students in this program is different and hence the demographics of the survey was different than that of the high school. The percentage of male vs. female respondents was ∼27 and ∼70% respectively, while the overall high school’s proportions are 51 and 49% (US News, 2021). Similarly, the proportion of Asian (including S. E. Asian) students who responded to our survey are much higher at ∼64% whereas the overall high school’s proportions is 20% Asians. This skew introduces a bias in our study. The survey did not track students who had access to the survey but chose not to participate -- prior investigations report a “pre-existing interest” bias, where participants with interest in mental health issues are more inclined to respond, which can introduce bias in the data. However, Giannopoulou et al. (2020) report that such “pre-existing interest” bias applies to most mental health surveys of COVID-19, which have mostly used web-based convenience samples (Pierce et al., 2020). The survey then included questions on mental health estimators to assess the mental wellbeing before and during the pandemic. The survey was administered during the pandemic and hence, the question on mental health prior to the pandemic is subject to recall bias, similar to other investigations (Giannopoulou et al., 2021). Although our study included freeform comments, we did not specifically ask participants to list aspects that cause mental health issues. Hence, our study does not draw inferences on additional factors such as family situation, social isolation, etc. (APA 2020; Tandon 2020; Wang et al., 2020) that may degrade mental health. Lastly, the mental health estimators used in this study, despite its good internal consistency, is not a well-established instrument and hence may have an impact on the results from this study.
The ongoing COVID-19 pandemic has not only caused considerable socioeconomic impacts but has also caused mental health degradation worldwide. The American Psychological Association (APA) reports that nearly 81% of Gen Z teens Gen Z teens (ages 13–17) experience more intense stress due to COVID-19, associated with schooling. Several recent investigations have confirmed the technological challenges and psychological impacts due to the COVID-19 pandemic on school students in different countries. However, similar to the APA’s report, the recent studies have not focused on eliciting key stressors that cause mental health degradation in school students.
This study pursued a data-driven, scientific, and ethical approach to assess the impact of COVID-19 pandemic on the mental health of high schoolers. There are a few distinguishing aspects of this study. First, it used a focus-group based approach to elicit key stressors that impact the population under study. We found this approach to be very useful particularly due to the paucity of articles that focus on the impact of the pandemic on the high school population. Similar to recent publications to assess stress and anxiety changes, our study also used 5-questions on a 5-point Likert-like scale to elicit the mental health of the participants. The results from Cronbach’s alpha (α = 0.78) analysis showed good internal consistency of our instrument. Our alpha value (α = 0.78) is considered a good value (Taber, 2018) and is consistent with the values reported by recent investigations conducted using different assessment instruments. PCA was used to establish that the controls and stressors (explanatory/independent variables) contain important information about this survey population, adding further credence to the survey used in this study.
Our fully anonymous survey was made available via a Google Form to students enrolled in the Biomedical science program in William Mason Highschool (Ohio, United States). The school administration restricted the survey to this subset of students. No incentives of any kind were provided to the participants. Yet, a majority of the students in the program completed the survey over a period of 30 days. This outcome suggests that students are open to sharing information about their wellbeing.
A comparison of mental health ratings showed a statistically significant mental health degradation t = 0.636 (p ≪ 0.001) before and during the pandemic as summarized in Figure 5. Correlation analysis (Figure 6) and regression analysis (Figure 7) showed that the most influential factor was Online schooling (estimate = −0.90), followed by race (estimate = 0.89), and exercise time (0.15). In our study, Asian students reported a much lower rate of mental health degradation. This could possibly be due to bias in our survey population because 64% of the respondents were of Asian descent. However, despite 70% of the respondents being female, no differences were found when controlling for gender, suggesting that both males and females experienced comparable mental health issues. Other factors such as pre-existing mental health issues and therapy did not influence mental health degradation.
The survey gathered time spent by the participants on several daily activities, namely: school time, homework time, exercise time, and sleeping time. We used an unpaired t-test to compare these four times reported by participants who did and did not experience mental health degradation. None of the times in the four categories showed any statistical difference. Prior to the unpaired t-test, we verified that all of the times in the four categories were normally distributed, verified using the Shapiro-Wilk normality test. In addition, except for homework time, they all had similar variances, verified using F-test.
Nevertheless, exercise time (PT.Time) was found to have explanatory power, in addition to learning-modality and race, in multivariate regression analyses. Consequently, we analyzed its contribution by comparing it with a regression model without it just two explanatory variables, namely learning-modality (In-person vs. online) and race. The simpler model was able to predict potential degradation in mental health with an accuracy of 62.3% (p = 0.014) vs. 73.6% with PT.Time included (see Figure 8). When compared to the full regression model, the false positive rate for the simpler model is higher. The key difference between the two models is attributed to the known benefits of exercise time (PT.Time). This finding is consistent with results reported by recent investigations (Liang et al., 2020). Adding PT.Time to the model essentially reduced the number of false positives from 40 to 25. That is, the simpler model tends to be more cautious and predict potential mental health degradation, which in this context, may be an acceptable risk. On the other hand, the number of false negatives from the simpler model is zero, showing that the chances we would miss a potential mental health problem is rather low, which is a positive aspect of the simpler model. The overall good prediction rates suggests that the two explanatory variables, namely learning-modality, and race are strong indicators of mental health changes in our survey data, with PT.Time helping to mitigate impact of stressors on mental health.
Multiple statistical analyses clearly showed degradation in mental health of students pursuing online schooling. Several recent articles suggest that this degradation can be attributed to a feeling of social isolation, loss of social structure in the form of friends or clubs (85% of students reported being in a club or student organizations), and family issues (Zhang et al., 2020). Although, our questionnaire did not specifically solicit factors impacting mental health, 45.8% of the participants provided freeform comments. Reviewing the freeform feedback identified three recurring themes in the feedback. First, 14 participants (13.2%) indicated that stress caused by homework as being an influential factor and suggested coordination between instructors to spread the workload. Second, nine participants (8.5%) reported social isolation or lack of social interactions as being a factor. Third, 13 participants (12.3%) suggest increasing community support and social interactions through flexible times or during short breaks during school time could improve mental wellbeing.
The regression analysis showed that exercise has a positive impact on mental wellbeing. Hence, online schooling can explore opportunities to engage students by providing breaks for physical activity. Electronic approaches for gaming such as Wii exercise could be used for exercising and their results can be used to provide bonus points for students to encourage regular exercising. Exercises can be combined with the aforementioned actionable approaches proposed by participants to further alleviate some of the stressors experienced by high schoolers.
Combining with the data published by the APA (2020), this study further helps our community to move from correlation to causality, which is a critical step in addressing the rising amount of mental health issues in younger generations in the United States. Although our results are subject to some bias in race, there is no gender bias. Hence, our results are also applicable in other communities and possibly in other regions as well. This study provides survey instruments and valuable inferences to many communities to help improve mental health of their children. By conducting further research, the scientific community would be able to learn more about what impacts mental health and how to help those in need.
The datasets presented in this study can be found in online repositories. The names of the repository/repositories and accession number(s) can be found below: http://pc2lab.cec.miamioh.edu/documents/RawMentalHealthSurvey.xlsx.
The studies involving human participants were reviewed and approved by Institutional Review Board, William Mason High School, Mason, OHIO, United States. Written informed consent from the participants legal guardian/next of kin was not required to participate in this study in accordance with the national legislation and the institutional requirements.
MR conceived the research, designed the study, developed survey, collected data, helped with data analysis, helped with creating charts and figures, and writing the article. DR helped with data analysis, drawing inferences, assisted with creating charts and figures, and writing the article.
The authors declare that the research was conducted in the absence of any commercial or financial relationships that could be construed as a potential conflict of interest.
The authors thank Susana Maldonado and Victoria Rao for their continuous encouragement and support.
The Supplementary Material for this article can be found online at: https://www.frontiersin.org/articles/10.3389/feduc.2021.719539/full#supplementary-material
American Psychological Association (APA) (2020). Stress in America, 2020: A National Mental Health Crisis. Available at: https://www.apa.org/news/press/releases/stress/2020/report-october (Accessed January 20, 2021).
Asanov, I., Flores, F., McKenzie, D., Mensmann, M., and Schulte, M. (2021). Remote-learning, Time-Use, and Mental Health of Ecuadorian High-School Students during the COVID-19 Quarantine. World Dev. 138, 105225. doi:10.1016/j.worlddev.2020.105225
Bhattacherjee, A. (2019). Social Science Research: Principles, Methods and Practices (Revised Edition). Tampa, FL: University of South FloridaAvailable at: https://scholarcommons.usf.edu/cgi/viewcontent.cgi?article=1002&context=oa_textbooks.
Core Team (2020). R: A Language and Environment for Statistical Computing. Vienna, Austria: R Foundation for Statistical Computing. Available at:.
Dolean, D. D., and Lervag, A. (2021). Variations of Homework Amount Assigned in Elementary School Can Impact Academic Achievement. J. Exp. Edu., 1–18. doi:10.1080/00220973.2020.1861422
Giannopoulou, I., Efstathiou, V., Triantafyllou, G., Korkoliakou, P., and Douzenis, A. (2021). Adding Stress to the Stressed: Senior High School Students' Mental Health amidst the COVID-19 Nationwide Lockdown in Greece. Psychiatry Res. 295, 113560. doi:10.1016/j.psychres.2020.113560
Google Inc (2021). Google Forms. Retrieved from: https://www.google.com/forms/about/( Accessed March 20, 2021).
Hawryluck, L., Gold, W. L., Robinson, S., Pogorski, S., Galea, S., and Styra, R. (2004). SARS Control and Psychological Effects of Quarantine, Toronto, Canada. Emerg. Infect. Dis. 10 (7), 1206–1212. doi:10.3201/eid1007.030703
Hou, T.-y., Mao, X.-f., Dong, W., Cai, W.-p., and Deng, G.-h. (2020). Prevalence of and Factors Associated with Mental Health Problems and Suicidality Among Senior High School Students in Rural China During the COVID-19 Outbreak. Asian J. Psychiatry 54, 102305. doi:10.1016/j.ajp.2020.102305
Johnson, R. A., and Wichern, D. (2015). “Multivariate Analysis,” in Wiley StatsRef: Statistics Reference Online (John Wiley & Sons, Inc.), 1–20. doi:10.1002/9781118445112.stat02623.pub2
Johnson, J. G., Harris, E. S., Spitzer, R. L., and Williams, J. B. W. (2002). The Patient Health Questionnaire for Adolescents. J. Adolesc. Health 30 (3), 196–204. doi:10.1016/S1054-139X(01)00333-0
Jolliffe, I. T., and Cadima, J. (2016). Principal Component Analysis: A Review and Recent Developments. Phil. Trans. R. Soc. A. 374 (2065), 20150202. doi:10.1098/rsta.2015.0202
Lau, J. T., Griffiths, S., Choi, K. C., and Tsui, H. Y. (2010). Avoidance Behaviors and Negative Psychological Responses in the General Population in the Initial Stage of the H1N1 Pandemic in Hong Kong. BMC Infect. Dis. 10, 139. doi:10.1186/1471-2334-10-139
Liang, L., Ren, H., Cao, R., Hu, Y., Qin, Z., Li, C., et al. (2020). The Effect of COVID-19 on Youth Mental Health. Psychiatr. Q. 91, 841–852. doi:10.1007/s11126-020-09744-3
McAlonan, G. M., Lee, A. M., Cheung, V., Cheung, C., Tsang, K. W., Sham, P. C., et al. (2007). Immediate and Sustained Psychological Impact of an Emerging Infectious Disease Outbreak on Health Care Workers. Can. J. Psychiatry 52 (4), 241–247. doi:10.1177/070674370705200406
Pierce, M., McManus, S., Jessop, C., John, A., Hotopf, M., Ford, T., et al. (2020). Says Who? the Significance of Sampling in Mental Health Surveys During COVID-19. Lancet Psychiatry 7 (7), 567–568. doi:10.1016/S2215-0366(20)30308-410.1016/s2215-0366(20)30237-6
Rabbi, F., Khan, S., Khalil, A., Mashwani, W. K., Shafiq, M., Göktaş, P., et al. (2020). Model Selection in Linear Regression Using Paired Bootstrap. Commun. Stat. - Theor. Methods 50, 1629–1639. doi:10.1080/03610926.2020.1725829
Spitzer, R. L., Kroenke, K., Williams, J. B. W., and Löwe, B. (2006). A Brief Measure for Assessing Generalized Anxiety Disorder. Arch. Intern. Med. 166 (10), 1092–1097. doi:10.1001/archinte.166.10.1092
Taber, K. S. (2018). The Use of Cronbach's Alpha when Developing and Reporting Research Instruments in Science Education. Res. Sci. Educ. 48, 1273–1296. doi:10.1007/s11165-016-9602-2
Tandon, R. (2020). COVID-19 and Mental Health: Preserving Humanity, Maintaining Sanity, and Promoting Health. Asian J. Psychiatry 51, 102256. doi:10.1016/j.ajp.2020.102256
Tavakol, M., and Dennick, R. (2011). Making Sense of Cronbach's Alpha. Int. J. Med. Edu. 2, 53–55. doi:10.5116/ijme.4dfb.8dfd
UNESCO (2021). COVID-19 Educational Disruption and Response. Retrieved from: https://en.unesco.org/covid19/educationresponse. [Data from April 4, 2021] (Accessed March 20, 2020).
Veit, C. T., and Ware, J. E. (1983). The Structure of Psychological Distress and Well-Being in General Populations. J. Consult. Clin. Psychol. 51 (5), 730–742. doi:10.1037/0022-006X.51.5.730
Wang, G., Zhang, Y., Zhao, J., Zhang, J., and Jiang, F. (2020). Mitigate the Effects of home Confinement on Children during the COVID-19 Outbreak. The Lancet 395 (10228), 945–947. doi:10.1016/S0140-6736(20)30547-X
Xiang, Y. T., Yu, X., Ungvari, G. S., Correll, C. U., and Chiu, H. F. (2014). Outcomes of SARS Survivors in China: Not Only Physical and Psychiatric Co-morbidities. East. Asian Arch. Psychiatry 24 (1), 37–38.
Keywords: mental health, online education, COVID-19, high school students, survey-based
Citation: Rao ME and Rao DM (2021) The Mental Health of High School Students During the COVID-19 Pandemic. Front. Educ. 6:719539. doi: 10.3389/feduc.2021.719539
Received: 02 June 2021; Accepted: 07 July 2021;
Published: 22 July 2021.
Edited by:
Maria Jose Alvarez-Alonso, Nebrija University, SpainReviewed by:
Cristina Ma García-Fernández, University of Cordoba, SpainCopyright © 2021 Rao and Rao. This is an open-access article distributed under the terms of the Creative Commons Attribution License (CC BY). The use, distribution or reproduction in other forums is permitted, provided the original author(s) and the copyright owner(s) are credited and that the original publication in this journal is cited, in accordance with accepted academic practice. No use, distribution or reproduction is permitted which does not comply with these terms.
*Correspondence: Dhananjai M. Rao, cmFvZG1AbWlhbWlvaC5lZHU=
Disclaimer: All claims expressed in this article are solely those of the authors and do not necessarily represent those of their affiliated organizations, or those of the publisher, the editors and the reviewers. Any product that may be evaluated in this article or claim that may be made by its manufacturer is not guaranteed or endorsed by the publisher.
Research integrity at Frontiers
Learn more about the work of our research integrity team to safeguard the quality of each article we publish.