- 1Aix-Marseille Université (AMU) and Centre National de la Recherche Scientifique (CNRS), Laboratoire de Psychologie Cognitive (LPC), UMR 7290, Marseille, France
- 2Aix-Marseille Université (AMU), Institut National Supérieur du Professorat Supérieur (INSPE), Marseille, France
Here we provide a proof-of-concept for the use of virtual reality (VR) goggles to assess reading behavior in beginning readers. Children performed a VR version of a lexical decision task that allowed us to record eye-movements. External validity was assessed by comparing the VR measures (lexical decision RT and accuracy, gaze durations and refixation probabilities) to a gold standard reading fluency test—the One-Minute Reading test. We found that the VR measures correlated strongly with the classic fluency measure. We argue that VR-based techniques provide a valid and child-friendly way to study reading behavior in a school environment. Importantly, they enable not only the collection of a richer dataset than standard behavioral assessments but also the possibility to tightly control the environment.
Introduction
Virtual reality (VR) techniques are a collection of software and hardware technologies that support the creation of synthetic, highly interactive three dimensional (3D) spatial environments, in which the user becomes a participant in a “virtually real” world (Psotka, 1995). An essential ingredient of VR technology is a tracked head-mounted display (HMD) that makes it possible for participants to see new views of the visual world as they move their head (Jensen and Konradsen, 2018). The key concept of VR is immersion (Jennett et al., 2008; Howard-Jones et al., 2014), a sense of “being” in the task environment, of being physically present in a non-physical world (Freina and Ott, 2015). The main motivation for using VR in education and training is that it provides the opportunity to experience situations that cannot be accessed physically (for review, see Freina and Ott, 2015; Stuart and Thomas, 1991) because of problems in time (e.g., visit different historical periods), distance (e.g., exploring the solar system or the functioning of a cell), dangerousness (e.g., training fire fighters to make decisions in life threatening situations) or ethics (e.g., performing surgery by non-experts). Here, we explore a very different advantage of using VR technology in an educational situation, namely the possibility to assess reading skills in a potentially noisy and distracting environment (i.e., classroom). Indeed, running experiments with children in a school environment is often a complex process that sometimes requires to control or measure eye movements and attention. We show that VR technology can provide such controls in a user-friendly way.
One of the keys to success in today’s world is becoming a skilled reader, and behavioral investigations of the mechanisms involved in achieving this skill are therefore of utmost importance. However, VR has rarely been used to study reading, which is hardly surprising, because reading provides a way to create a virtual reality without the need to use a computer-based system (Nell, 1988; Jacobs, 2015). Reading, quite naturally, allows one to shape events in a person’s brain. Much like a VR system, reading bridges gaps of time, space, and acquaintanceship (Pinker, 1994; Ziegler et al., 2020). The feeling of “getting lost in a book” (Nell, 1988) is probably very similar to the immersion in an artificially created virtual world. So why would one want to use VR to study reading? One possible reason has been put forward in the context of cognitive assessment and rehabilitation: “The potential power of VR to create human testing and training environments which allow for precise control of complex stimulus presentations as well as providing accurate records of targeted responses is a cognitive psychologist’s dream!” (Rizzo and Buckwalter, 1997). In recent work in our group, we have started to use VR to study reading behavior in adults (Mirault et al., 2020) and there has been a general rise in the use of VR techniques in cognitive psychology in general (for a review, see Mirault, 2020).
The goal of the present study was to test to what extent a VR system can provide a valid and reliable reading fluency assessment technique in primary school children. There are several reasons for why this is an interesting and potentially important issue. First, recent HMD systems (i.e., VR goggles) allow the recording of head (3D location and velocity) and eye movements (i.e., fixation locations, fixation durations). The eye movements recorded during silent reading provide a direct measure of reading fluency and the impact of linguistic complexity on reading behavior (Mirault et al., 2020; see Rayner, 1998, for a review of early research on eye movements and reading). Currently, the recording of eye movements requires a rather sophisticated laboratory setup and a rigorous calibration procedure, in which the head must be fixed using a chin rest and/or a bite bar. This complicates the use of eye movement measures in a classroom context. Second, most psycho-educational reading assessments take place in a school setting where children are potentially distracted by environmental factors. The immersive potential of VR technology makes it possible to blend out much of these distracting factors, thus facilitating testing in a classroom setting. Third, the assessment of the visual, orthographic and attentional factors involved in reading (Facoetti et al., 2010; Ziegler et al., 2010; Zorzi et al., 2012; Stein, 2014; Grainger et al., 2016) requires “the precise control of complex stimulus presentations” (Rizzo and Buckwalter, 1997), such as a fixed distance to the screen, which determines visual angle and stimulus size. Fourth, since VR goggles have become affordable in the past years (Ray and Deb, 2016) making their wide use in schools possible, it is crucial to investigate whether the reading and eye movement measures obtained with this technique are robust and externally valid. Finally, very little research on VR has been conducted with primary school children (Eleftheria et al., 2013) and it remains to be shown that VR systems can reproduce classic laboratory benchmarks of reading, such as effects of lexicality, frequency and length (Grainger and Jacobs, 1996; Coltheart et al., 2001; Perry et al., 2007, Perry et al., 2010). This is important if these systems were to be included in more sophisticated systems, in which participants can interact with letters, words and sentences in a virtual game environment (Pan et al., 2006).
To start simple, in the present experiment, we had children in primary school (grade 2) make lexical decisions about words and pseudowords while wearing VR goggles. This allowed us to measure their reaction times, accuracy, initial fixation durations, total fixation durations, and number of fixations. Besides lexicality (words vs. pseudowords), we varied the length of words and pseudowords to test whether our measures are sensitive to word length, which is an excellent marker for the automatization of reading skills (Ziegler et al., 2003). To test the external validity of our VR test, we compared the VR measures with a One-Minute Reading (OMR) aloud test of words and pseudowords (similar to TOWRE, see Torgesen et al., 2012), which can be seen as the gold standard for measuring reading fluency (Bertrand et al., 2010). We expected to find faster and more accurate responses to words than to pseudowords. With respect to eye movements, we expected to see fewer and shorter fixations to words than to pseudowords. Finally, if our VR-based measures correlate strongly with the OMR gold standard, this could be taken as evidence that VR-based measures obtained during silent reading could potentially replace or complement more classic reading aloud assessments. This is important because the main goal of learning to read is fast, efficient, silent reading for meaning.
Method
Participants
A total of 102 children aged between 7 and 9 years were recruited from two schools in Marseille (France). Participants were either native speakers of French or grew up in a French-speaking environment since birth or early childhood. They reported having normal or corrected-to-normal vision and were naïve to the purpose of the experiment. Their parents signed an informed consent form in accordance with the provisions of the World Medical Association Declaration of Helsinki prior to the experiment. Ethics approval was obtained from the Comité de Protection des Personnes SUD-EST IV (No. 17/051).
Apparatus
The VR environment was created using the software Unity (Unity Technologies ApS) and displayed on a WQHD OLED screen (2,560 × 1,440 pixels) covering up to 100° of visual angle with a refresh rate of 70 Hz. Eye movements were recorded using the infra-red eye-tracker in the virtual reality headset Fove 0 HMD (FOVE, Inc.). The headset size was adapted to children with a strap at the back of the device in order to make this comfortable for the children and to achieve good immersion (i.e., no lights from the classroom). Children were free to move the head and the design of the experiment allow them to continually see the stimulus in front of their head location. Recording was binocular with a high spatial accuracy (<1°) and a sampling rate of 120 Hz (however, we recorded at 70 Hz in order to match the refresh rate of the screen). The position of the head was obtained by combining a USB Infra-Red position tracking camera with a refresh rate of 100 Hz and an Inertial Measurement Unit (IMU) placed in the headset. A recent graphic card (NVIDIA GeForce GTX 1650) was mounted on a laptop computer (ASUS ROG STRIX G) to display the VR environment in the Fove headset. The VR environment was also duplicated on the laptop LCD screen running with a high refresh rate (144 Hz) for the experimenter. The response was provided by pressing buttons on a gamepad (Trustmaster Dual Analog 4).
From the eye tracker, we recovered 6 measures: three for the origins (x, y, and z) and three for the Gaze Intersection Point (GIP). We defined the Origins as the viewer-local coordinates mapped from eye tracker screen coordinates to the near view plane coordinates. The GIP is given by the addition of a scaled offset to the view vector originally defined by the helmet position and central view line in virtual world coordinates (from Eye Tracking Methodology; Duchowski, 2007).1
Design and Stimuli
We created 100 items: 50 words and 50 pseudowords (see Stimuli on OSF link at the end of the article) that ranged in length from 4 to 8 characters (10 words and 10 pseudowords for each size). The words had an average frequency of 997.76 parts per million (ppm) (based on the Manulex frequency counts: Lété et al., 2004) which is equivalent to 5.99 Zipf (van Heuven et al., 2014). The pseudowords were constructed to look like real French words and were always pronounceable.
Procedure
In this study, children participated in two tasks: a VR lexical decision task (VR-LDT) and a One-Minute Reading (OMR) aloud test. In order to counterbalance task order, half of the children started with the VR-LDT while the other half started with the OMR. For the VR-LDT, they were seated in front of a school desk at 70 cm from the infra-red position detector and were free to move their head and torso. Testing did not occur in the classroom but in a small room right next to the classroom. Two children were tested at the same time. While one was doing the VR-LDT test, the other one did the OMR test. The instructions were explained to the children as a game, in which they had to detect “true” and “false” words. At the beginning of the experiment, the orientation and the position of the headset were tared, then, the participant’s eye position was calibrated using a 5-dot calibration phase. Dots appeared on the VR screen in green with a decreasing size. Children were instructed to focus on the center of the dots. They had the possibility to remove the headset at any time of the experiment. The instructions were repeated one more time and the experiment was initiated if the child was ready to start. Each trial started with a fixation dot during 1,000 ms located in the center of the screen (here, we use the term screen to refer to the calibrated visual field of the virtual environment). Then, the stimulus was displayed in black in the center of the virtual environment. We displayed the stimulus in monospaced vectorial police (no pixelization even if you zoom-in or zoom-out), with a font-size of 36 (it cannot be compared to normal font size to because of the depth of the Z axis). The background was a neutral virtual environment with a brown floor and a blue sky; the horizon line was light blue. Participants had to read the stimulus and press the right trigger on the gamepad if the word existed in French or the left trigger if not, as fast and as accurately as possible. We shuffled the list with all the items (N = 100) in order to create a random stimulus presentation and we used the same shuffled list for all children. Gamepad and desk were cleaned with a bactericidal wipe between each participation. There were no practice trials and feedback during the experiment, but the experimenter gave oral examples and invited the children to press the correct button. The experimenter then provided oral feedback and an explanation for any errors made.
Concerning the OMR test, we used the LUM test (“Lecture en Une Minute”) developed by Khomsi (1999). It consists of two reading aloud tests: one with a table of 35 existing words (in French), the other with a table of 30 pseudowords. The tables are presented in five rows. The test is explained to the child and then he or she starts by reading two test words (outside the table) that do not count in the number of words read. Then the timer is started when the child reads the first word in the table. Children were instructed to read the words from left to right and then to move to the next line. Scoring the test first involved counting the number of correctly read words and discarding incorrectly read words (mispronounced or not read after 5 s). After one minute the test was stopped. The number of correctly read words is the fluency value. If a child read all the words correctly in less than one minute, then the time to do so was recorded, and the number of words correctly read per minute calculated from that.
Pre-Processing the Eye Movements
We used the emov package (Schwab, 2016) in the R statistical computing environment (Pinheiro et al., 2014). This package implements a dispersion-based algorithm (I-DT) proposed by Salvucci and Goldberg (2000) which measures fixation durations and positions.
Analysis
We used Linear Mixed-Effects models (LMEs) to analyze our data, with items and participants as crossed random effects, including by-item and by-participant random intercepts (Baayen et al., 2008). Items in these analyses were the words/pseudowords. LMEs were used to analyze response time and fixation durations while Generalized (logistic) LMEs were used to analyze error and refixation rates. The models were fitted with the lmer (for LMEs) and glmer (for GLMEs) functions from the lme4 package (Bates et al., 2015) in R. We report regression coefficients (b), standard errors (SE) and |t-values| (for LMEs) or |z-values| (for GLMEs) for all factors. Fixed effects were deemed reliable if |t| or |z| > 1.96 (Baayen et al., 2008). All durations were inverse transformed (−1,000/duration) prior to analysis.
Following the main analyses, we will present post-hoc analyses concerning length and frequency effects and cross-task correlations.
Results
Prior to analysis we excluded participants who did not finish the experiment (N = 2) and those for whom there was a technical incident during the experiment (N = 10). The remaining group was composed of 90 participants.
Effects of Lexicality
Lexical Decision Error Rates
We observed a significant effect of lexicality in lexical decision error rates (b = 1.18; SE = 0.12; z = 9.81), with children making fewer errors to words (M = 5.30%; 95% CI = 4.68) compared to pseudowords (M = 13.38%; 95% CI = 7.11).
Lexical Decision Response Times
Prior to analysis, we excluded 3.32% of data points for being 2.5 SD below or above the participant’s mean such that extreme outlier values do not affect the inferential statistics. This is a standard procedure in experimental psychology (Ratcliff, 1993). We observed a significant effect of lexicality in lexical decision response times (b = 0.29; SE = 0.02; z = 13.87), with participants responding more rapidly to words (M = 1,627.87 ms; 95% CI = 197.70) than to pseudowords (M = 2,625.10 ms; 95% CI = 277.65). Figure 1 shows the condition means with response times transformed into reading speed.
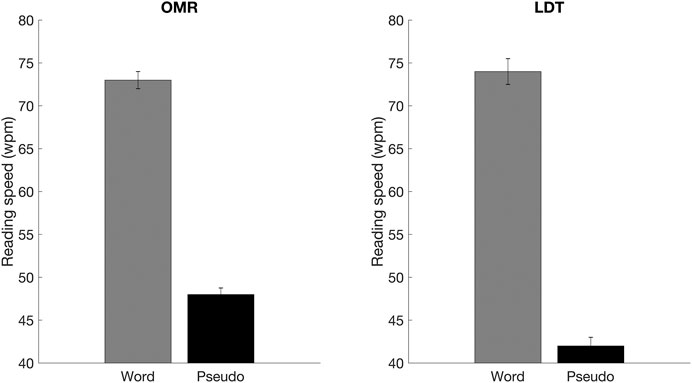
FIGURE 1. Mean correct word/pseudoword per minute for the One-Minute Reading test (OMR; left) and reading speed in the Lexical Decision Task (LDT; right) for words and pseudowords. Error bars represent 95% CIs.
One-Minute Reading Test
More words were read aloud correctly per minute than pseudowords (b = 24.84; SE = 1.49; z = 16.67). The condition means are shown in Figure 1.
Refixation Probability
We observed a significant effect of lexicality in refixation rates (b = 1.29; SE = 0.23; z = 5.48), meaning that participants made fewer refixations to words (M = 0.68; 95% CI = 0.09) than to pseudowords (M = 0.79; 95% CI = 0.08).
Fixation Durations
We recorded the first fixation duration (FFD), which is the duration of the first fixation on the word / pseudoword, and gaze duration (GD), which is the sum of all fixations on the word / pseudoword before the eyes left the stimulus. For each measure, we deleted durations beyond 2.5 standard deviations from the grand mean (FFD = 2.78%; GD = 3.18%) prior to statistical analysis. We observed a significant difference between words and pseudowords for FFD (b = 0.82; SE = 0.12; t = 6.55) and for GD (b = 1.19; SE = 0.19; t = 6.03), with longer durations for pseudowords compared to words. Condition means are reported in Figure 2.
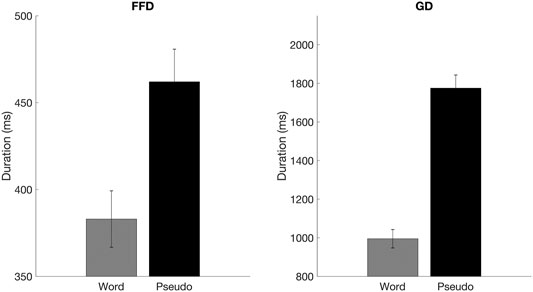
FIGURE 2. Mean first fixation duration (FFD; left) and gaze duration (GD; right) for words and pseudowords. Errorbars represent 95% CIs. The Y-axis scale is adapted to each measure.
Effects of Length
The average values for all dependent measures for the different lengths are shown in Tables 1 and 2.

TABLE 1. Average lexical decision response times and error rates, and averages of the three eye-tracking measures for each length (in number of letters) for words.

TABLE 2. Average lexical decision response times and error rates, and averages of the three eye-tracking measures for each length (in number of letters) for pseudowords.
Length Effects for Words
Concerning the length effect for words, we observed significant effects for all the dependent measures (Lexical Decision Error rate: b = 0.15; SE = 0.07; z = 2.04, Lexical Decision Response Time: b = 0.04; SE = 0.00; t = 4.82; Refixation rate: b = 0.67; SE = 0.06; z = 9.97, FFD: b = 0.31; SE = 0.05; t = 6.29, and GD: b = 0.61; SE = 0.06; t = 9.58).
Length Effects for Pseudowords
Concerning the length effect for pseudowords, we observed significant effects for Lexical Decision Response Time (b = 0.27; SE = 0.00; t = 4.49), Refixation rate (b = 0.54; SE = 0.06; z = 8.38), FFD (b = 0.02; SE = 0.00, t = 3.41), and GD (b = 0.36; SE = 0.05; t = 7.06). We found a marginally significant effect for Lexical Decision Error rates (b = 0.08; SE = 0.05; z = 1.63), with errors tending to decrease as pseudoword length increased.
Cross-Task Comparisons
Reading Speed
The comparison of reading speed for words and pseudowords in the lexical decision task and the One-Minute Reading test can be found in Figure 1. We calculated the correlations between average reading speed per child in these two tasks separately for words and pseudowords. The correlations were highly significant for both words (r = 0.63, p < 0.05) and pseudowords (r = 0.59, p < 0.05). Figure 3 shows the scatter plots of these correlations.
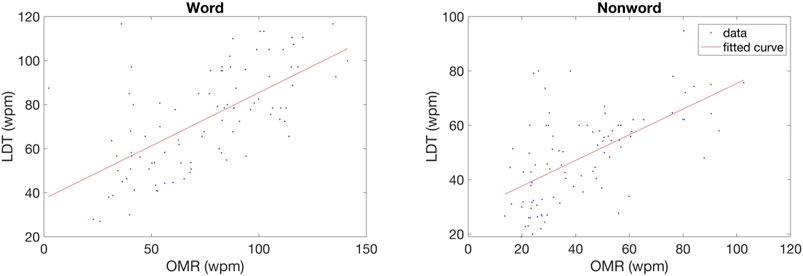
FIGURE 3. Scatter plots showing the relation between performance on the One-Minute Reading test (OMR) and the lexical decision task (LDT) for words (left panel, R2 = 0.40) and pseudowords (right panel, R2 = 0.35). Lines are best fitting linear regressions.
We also examined the relation between gaze durations, a gold standard for estimating word reading fluency in eye-movement research (e.g., Rayner, 1998), and Lexical Decision (r = −0.76, p < 0.05) and One-Minute Reading speed (r = −0.62, p < 0.05). The scatter plots of the correlations are shown in Figure 4.
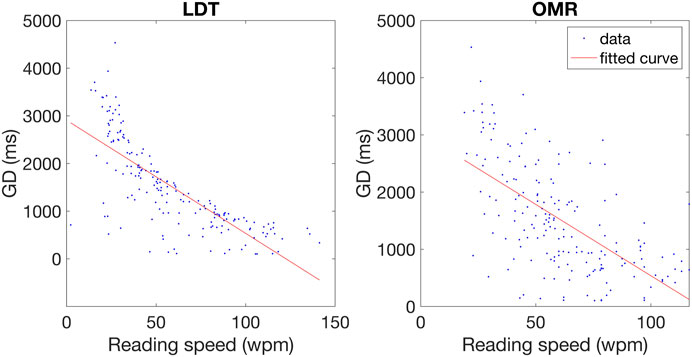
FIGURE 4. Scatter plots showing the relation between gaze duration (GD in milliseconds) and reading speed (wpm) in the Lexical Decision Task (LDT–left panel, R2 = 0.58) and the One-Minute Reading test (OMR–right panel, R2 = 0.38) averaged across words and pseudowords. Lines are best fitting linear regressions.
Finally, we examined the complete set of correlations across our different dependent measures. Table 3 provides the matrix of correlation coefficients between all dependent measures. We highlight values of |r| > 0.6. We observed 2 negative correlations with gaze durations (GD): with the OMR and LDT reading speeds, meaning that faster readers (higher reading speed) had shorter gaze durations. We also noted 3 positive correlations: one between the OMR and LDT reading speed scores, one between the refixation rate and first fixation durations, and another between refixation rate and gaze duration. The two latter correlations suggest that participants who made longer first fixations tended to refixate more often, hence the longer gaze durations.
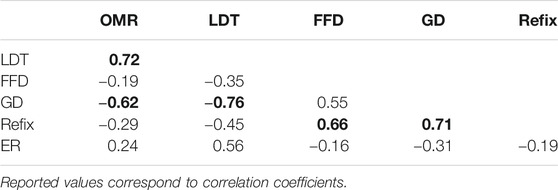
TABLE 3. Correlation matrix for the different dependent measures (OMR–One Minute Reading speed; LDT–Lexical Decision reading speed (wpm); FFD–first fixation duration; GD–gaze duration; Refix–refixation probability; ER–Lexical Decision error rates).
Discussion
The goal of the present study was to provide a proof-of concept that a virtual reality set-up can be used to measure reading fluency and eye movements during silent reading in primary school children. The internal validity was assessed using two classic benchmark measures of reading, effects of lexicality and word length. The external validity was assessed by comparing lexical decision performance and eye movement measures obtained in the virtual reality setting to a gold-standard reading fluency measure (OMR test). It is important to note that our research follows the AERA recommendations of “Standards for Educational and Psychological Testing” (American Educational Research Association (AERA), 2014).
Concerning internal validity, first of all, there were clear effects of lexicality in all our behavioral and eye movement measures obtained in the virtual reality setting. Children made more errors on pseudowords compared to words and took longer to respond to pseudowords compared to words. The two eye tracking measures (FFD and GD) also showed that children spent significantly more time inspecting pseudowords compared to words, and in line with this, children also re-fixated pseudowords more often than words. Secondly, there were clear effects of length on all our dependent measures except for lexical decision error rates to pseudowords. All other measures provided evidence that longer stimuli were harder to process, with longer response times and more errors in the lexical decision task, and longer fixation durations and more re-fixations in the eye movement measures. Given that the effects of word length are excellent measures for automatization of reading processes, they could be used to detect children who have not yet fully automatized word recognition procedures. That is, children who still exhibit some form of serial processing that is characteristic of dyslexia (Ziegler et al., 2003).
Concerning external validity, we found that the VR-LDT and OMR tasks produced almost identical effects of lexicality. Moreover, there was a very strong correlation between the VR-LDT and OMR reading speed measures. It is, of course, the case that silent reading and reading aloud measures naturally correlate and this alone should not be taken to suggest that VR methods produce more robust correlations with reading aloud than classic silent reading tasks. Yet, the high correlation is not a trivial result because the OMR task is a reading aloud (production) measure that requires the exact pronunciation of a letter string, while the LDT task is a silent reading/visual word recognition measure that does not require the computation of word’s pronunciation (Grainger and Jacobs, 1996; Dufau et al., 2012). In addition, OMR requires individual and supervised testing (i.e., an adult has to record the number of words read aloud), while the VR-LDT test can be done in an unsupervised, automatized fashion. The fact that these measures correlate so strongly points to a promising avenue for individualized high-quality assessment of reading fluency that does not require the intervention of an expert assessor.
Concerning the strong correlation between reading speed (wpm) in the lexical decision task and gaze durations (r = −0.76) found in the present study, this is in line with one prior study investigating such a relation with standard eye movement recording techniques during sentence reading (Schilling et al., 1998). However, given the results of more recent investigations that have revealed much lower correlations (Kuperman et al., 2013; Dirix et al., 2019), it seems likely that the high correlation found in our study is linked to the fact that the eye movement measures were obtained with isolated stimuli and not for words presented in a sentence context. In particular, the work of Dirix et al. (2019) demonstrates the limits of using lexical decision as a proxy to real-life reading, hence the importance of complementing this measure with eye movement measures as in the present work.
A major limitation of the present study is that we have not yet used any of the typical features of virtual reality environment related to the construction of a highly interactive 3D spatial environment. Also, as children moved their heads in our study, they did not get different views of the word they were looking at. While we fully acknowledge these limitations, it is important to note that these interesting aspects of VR were clearly beyond the scope of the present article. The primary goal of our study was to provide a proof-of-concept that VR technology can provide a reliable, valid, and child-friendly way to measure reading fluency in children, without the intervention of skilled assessors, and in a normal school environment. We successfully demonstrated that one can obtain reliable word recognition and eye movement measures with a procedure that can be applied in noisy school environments.
Data Availability Statement
The datasets presented in this study can be found in online repositories. The names of the repository/repositories and accession number(s) can be found below. https://osf.io/m8j2z.
Ethics Statement
The studies involving human participants were reviewed and approved by Ethics approval was obtained from the Comité de Protection des Personnes SUD-EST IV (No. 17/051). Written informed consent to participate in this study was provided by the participants’ legal guardian/next of kin.
Author Contributions
JM created the VR scripts, the analysis scripts and participated in the design of the experiments, in the creation of the stimuli, in the collection of the data and wrote the first draft of the manuscript. J-PA participated in the creation of the stimuli, in the analysis of the results, in the design of the experiments and in the data collection. JL helped in the creation of the stimuli and participated in the design of the experiments. JG participated in the design of the experiments and in the writing of the manuscript. JZ participated in the design of the experiments and in the writing of the manuscript.
Funding
This study was funded by grant ERC 742141 (JG) and the Ministry of Education eFran Grant scheme (JZ).
Conflict of Interest
The authors declare that the research was conducted in the absence of any commercial or financial relationships that could be construed as a potential conflict of interest.
Acknowledgments
We thank Julie Pynte for her help in running the participants in this study, Édouard Alavoine and Agnès Guerre-Genton for their help in the design of the experiment.
Footnotes
1All code used to program the experiment and to analyze the data can be found at: https://osf.io/m8j2z.
References
American Educational Research Association (AERA) (2014). Standards for Educational and Psychological Testing. New York, NY: American Educational Research Association American Psychological Association National Council on Measurement in Education.
Baayen, R. H., Davidson, D. J., and Bates, D. M. (2008). Mixed-effects Modeling with Crossed Random Effects for Subjects and Items. J. Mem. Lang. 59, 390–412. doi:10.1016/j.jml.2007.12.005
Bates, D., Maechler, M., Bolker, B., Walker, S., Christensen, R. H. B., Singmann, H., et al. (2015). Package ‘lme4’. Convergence 12, 2. doi:10.1007/s00265-020-02842-z
Bertrand, D., Fluss, J., Billard, C., and Ziegler, J. C. (2010). Efficacité, sensibilité, spécificité : comparaison de différents tests de lecture. Année psy 110, 299–320. doi:10.4074/s000350331000206x
Coltheart, M., Rastle, K., Perry, C., Langdon, R., and Ziegler, J. (2001). DRC: A Dual Route Cascaded Model of Visual Word Recognition and reading Aloud. Psychol. Rev. 108, 204–256. doi:10.1037/0033-295x.108.1.204
Dirix, N., Brysbaert, M., and Duyck, W. (2019). How Well Do Word Recognition Measures Correlate? Effects of Language Context and Repeated Presentations. Behav. Res. 51, 2800–2816. doi:10.3758/s13428-018-1158-9
Duchowski, A. T. (2007). Eye Tracking Methodology. Theor. Pract. 328, 2–3. doi:10.1007/978-1-84628-609-4
Dufau, S., Grainger, J., and Ziegler, J. C. (2012). How to Say “no” to a Nonword: A Leaky Competing Accumulator Model of Lexical Decision. J. Exp. Psychol. Learn. Mem. Cogn. 38, 1117–1128. doi:10.1037/a0026948
Eleftheria, C. A., Charikleia, P., Iason, C. G., Athanasios, T., and Dimitrios, T. (2013). An Innovative Augmented Reality Educational Platform Using Gamification to Enhance Lifelong Learning and Cultural Education. IISA 2013, 1–5. doi:10.1109/IISA.2013.6623724
Facoetti, A., Corradi, N., Ruffino, M., Gori, S., and Zorzi, M. (2010). Visual Spatial Attention and Speech Segmentation Are Both Impaired in Preschoolers at Familial Risk for Developmental Dyslexia. Dyslexia 16, 226–239. doi:10.1002/dys.413
Freina, L., and Ott, M. (2015). A Literature Review on Immersive Virtual Reality in Education: State of the Art and Perspectives. Int. Scientific Conf. eLearning Softw. Edu. 1, 10–1007. doi:10.1016/j.compedu.2019.103778
Grainger, J., Dufau, S., and Ziegler, J. C. (2016). A Vision of Reading. Trends Cogn. Sci. 20, 171–179. doi:10.1016/j.tics.2015.12.008
Grainger, J., and Jacobs, A. M. (1996). Orthographic Processing in Visual Word Recognition: A Multiple Read-Out Model. Psychol. Rev. 103, 518–565. doi:10.1037/0033-295x.103.3.518
Howard-Jones, P., Ott, M., van Leeuwen, T., and De Smedt, B. (2014). The Potential Relevance of Cognitive Neuroscience for the Development and Use of Technology-Enhanced Learning. London: Learning, Media and Technology, 1–21.
Jacobs, A. M. (2015). Towards a Neurocognitive Poetics Model of Literary reading. London: Cognitive Neuroscience of Natural Language Use, 135–159.
Jennett, C., Cox, A. L., Cairns, P., Dhoparee, S., Epps, A., Tijs, T., et al. (2008). Measuring and Defining the Experience of Immersion in Games. Int. J. Human-Computer Stud. 66, 641–661. doi:10.1016/j.ijhcs.2008.04.004
Jensen, L., and Konradsen, F. (2018). A Review of the Use of Virtual Reality Head-Mounted Displays in Education and Training. Educ. Inf. Technol. 23, 1515–1529. doi:10.1007/s10639-017-9676-0
Khomsi, A. (1999). Epreuve d'évaluation de la compétence en lecture: lecture de mots et compréhension-révisée. Paris: Les Editions du Centre de Psychologie Appliquée.
Kuperman, V., Drieghe, D., Keuleers, E., and Brysbaert, M. (2013). How Strongly Do Word reading Times and Lexical Decision Times Correlate? Combining Data from Eye Movement Corpora and Megastudies. Q. J. Exp. Psychol. 66, 563–580. doi:10.1080/17470218.2012.658820
Lété, B., Sprenger-Charolles, L., and Colé, P. (2004). MANULEX: A Grade-Level Lexical Database from French Elementary School Readers. Behav. Res. Methods Instr. Comput. 36, 156–166. doi:10.3758/bf03195560
Mirault, J., Guerre-Genton, A., Dufau, S., and Grainger, J. (2020). Using Virtual Reality to Study reading: An Eye-Tracking Investigation of Transposed-word Effects. Methods Psychol. 3, 100029–100034. doi:10.1016/j.metip.2020.100029
Nell, V. (1988). Lost in a Book: The Psychology of Reading for Pleasure. New Haven, London: Yale University Press. doi:10.2307/j.ctt1ww3vk3
Pan, Z., Cheok, A. D., Yang, H., Zhu, J., and Shi, J. (2006). Virtual Reality and Mixed Reality for Virtual Learning Environments. Comput. Graphics 30, 20–28. doi:10.1016/j.cag.2005.10.004
Perry, C., Ziegler, J. C., and Zorzi, M. (2010). Beyond Single Syllables: Large-Scale Modeling of reading Aloud with the Connectionist Dual Process (CDP++) Model. Cogn. Psychol. 61, 106–151. doi:10.1016/j.cogpsych.2010.04.001
Perry, C., Ziegler, J. C., and Zorzi, M. (2007). Nested Incremental Modeling in the Development of Computational Theories: the CDP+ Model of reading Aloud. Psychol. Rev. 114, 273–315. doi:10.1037/0033-295x.114.2.273
Pinheiro, J., Bates, D., DebRoy, S., and Sarkar, D. (2014). R Core Team (2014) Nlme: Linear and Nonlinear Mixed Effects Models. R. Package Version 3.1-117 11, 23. doi:10.1002/9781118445112.stat05514Available at: http://CRAN.R-project.org/package=nlme
Psotka, J. (1995). Immersive Training Systems: Virtual Reality and Education and Training. Instr. Sci. 23, 405–431. doi:10.1007/bf00896880
Ratcliff, R. (1993). Methods for Dealing with Reaction Time Outliers. Psychol. Bull. 114 (3), 510–532. doi:10.1037/0033-2909.114.3.510
Ray, A. B., and Deb, S. (2016). Smartphone Based Virtual Reality Systems in Classroom Teaching―a Study on the Effects of Learning Outcome. In: 2016 IEEE Eighth International Conference on Technology for Education (T4E)IEEE, 68–71.
Rayner, K. (1998). Eye Movements in reading and Information Processing: 20 Years of Research. Psychol. Bull. 124, 372–422. doi:10.1037/0033-2909.124.3.372
Rizzo, A. A., and Buckwalter, J. G. (1997). Virtual Reality and Cognitive Assessment. Virtual Reality In Neuro-Psycho-Physiology: Cognitive. Clin. Methodological Issues Assess. Rehabil. 44, 123.
Salvucci, D. D., and Goldberg, J. H. (2000). Identifying Fixations and Saccades in Eye-Tracking Protocols. Proc. 2000 Symp. Eye tracking Res. Appl. 1, 71–78. doi:10.1145/355017.355028
Schilling, H. E. H., Rayner, K., and Chumbley, J. I. (1998). Comparing Naming, Lexical Decision, and Eye Fixation Times: Word Frequency Effects and Individual Differences. Mem. Cogn. 26, 1270–1281. doi:10.3758/bf03201199
Schwab (2016). Software R. Available at: https://cran.r-project.org/web/packages/emov/index.html.
Stein, J. (2014). Dyslexia: the Role of Vision and Visual Attention. Curr. Dev. Disord. Rep. 1, 267–280. doi:10.1007/s40474-014-0030-6
Stuart, R., and Thomas, J. C. (1991). The Implications of Education in Cyberspace. Multimedia Rev. 2 (2), 2–17.
Torgesen, J. K., Wagner, R., and Rashotte, C. (2012). Test of Word Reading Efficiency: (TOWRE-2). London: Pearson Clinical Assessment
van Heuven, W. J. B., Mandera, P., Keuleers, E., and Brysbaert, M. (2014). SUBTLEX-UK: A New and Improved Word Frequency Database for British English. Q. J. Exp. Psychol. 67, 1176–1190. doi:10.1080/17470218.2013.850521
Ziegler, J. C., Pech-Georgel, C., Dufau, S., and Grainger, J. (2010). Rapid Processing of Letters, Digits and Symbols: what Purely Visual-Attentional Deficit in Developmental Dyslexia? Dev. Sci. 13, F8–F14. doi:10.1111/j.1467-7687.2010.00983.x
Ziegler, J. C., Perry, C., Ma-Wyatt, A., Ladner, D., and Schulte-Körne, G. (2003). Developmental Dyslexia in Different Languages: Language-specific or Universal? J. Exp. Child Psychol. 86, 169–193. doi:10.1016/s0022-0965(03)00139-5
Ziegler, J. C., Perry, C., and Zorzi, M. (2020). Learning to Read and Dyslexia: From Theory to Intervention through Personalized Computational Models. Curr. Dir. Psychol. Sci. 29, 096372142091587. doi:10.1177/0963721420915873
Keywords: reading fluency, virtual reality, lexical decision task, eye-tracking, beginning readers
Citation: Mirault J, Albrand J-P, Lassault J, Grainger J and Ziegler JC (2021) Using Virtual Reality to Assess Reading Fluency in Children. Front. Educ. 6:693355. doi: 10.3389/feduc.2021.693355
Received: 10 April 2021; Accepted: 31 May 2021;
Published: 10 June 2021.
Edited by:
Yong Luo, Educational Testing Service, United StatesReviewed by:
Jennifer Randall, University of Massachusetts Amherst, United StatesMatthew Ryan Lavery, South Carolina Education Oversight Committee, United States
Copyright © 2021 Mirault, Albrand, Lassault, Grainger and Ziegler. This is an open-access article distributed under the terms of the Creative Commons Attribution License (CC BY). The use, distribution or reproduction in other forums is permitted, provided the original author(s) and the copyright owner(s) are credited and that the original publication in this journal is cited, in accordance with accepted academic practice. No use, distribution or reproduction is permitted which does not comply with these terms.
*Correspondence: Jonathan Mirault, jonathan.mirault@univ-amu.fr