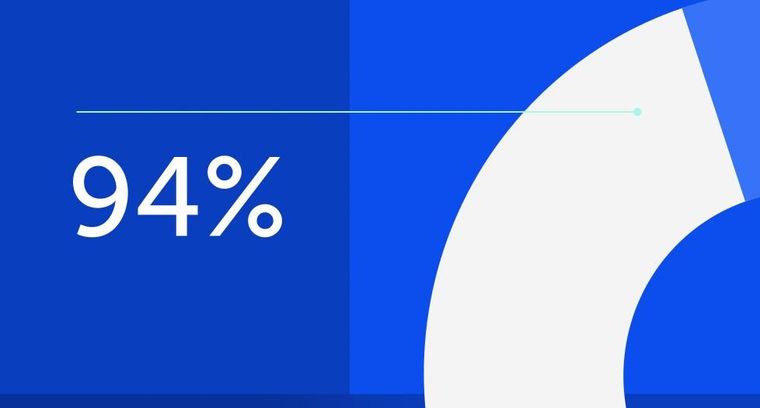
94% of researchers rate our articles as excellent or good
Learn more about the work of our research integrity team to safeguard the quality of each article we publish.
Find out more
ORIGINAL RESEARCH article
Front. Educ., 15 July 2021
Sec. STEM Education
Volume 6 - 2021 | https://doi.org/10.3389/feduc.2021.673850
This article is part of the Research TopicLearning Science in Out-of-School SettingsView all 20 articles
Several studies have investigated the way learners connect with science, re-emphasising persisting inequalities in science learning. This article combines the concept of intersectionality with the theoretical lens of science learning ecologies to focus on inequalities in connecting with science: Which factors influence the formation of a positive science attitude of young learners and how does the social background of young learners influence their opportunities of connecting with science, focusing on the intersections of class and gender? Based on a quantitative survey among 1,486 visitors of non-formal science education offers aged between 8 and 21, we analyze important factors for the development of a positive science attitude and investigate structural inequalities. The intersectional perspective was implemented in the sampling, survey design as well as its analysis. Using composite indicators of age and gender as well as gender and educational capital, we avoid a homogenisation of broadly defined groups. The results highlight that the development of a highly positive science attitude–as identified in a stepwise logistic regression model–is linked to supportive social environments, intrinsic motivation, science learning in school as well as regular engagement in arts-based learning, and self-directed science learning. The learning ecology perspective illustrates the influence of school on science attitudes in general. From an intersectional perspective, however, our findings demonstrate that the persistence of an androcentric and classist concept of science is not compatible with every learning ecology; male learners from educationally affluent backgrounds are most likely to enjoy science learning and see how science relates to their everyday realities. In turn, however, not only female learners with lower educational capital but also male learners with lower educational capital might find it more difficult to connect with science. The intersectional approach unveiled the multiple ways educational capital and gender shape individual learning ecologies. More equitable science learning spaces and offers have to adapt to a diversity of needs and preferences in order to make science activities enjoyable for all.
Popularly, science is connected to cleverness, intelligence, and academic success (Archer et al., 2013a; Archer et al., 2014). Access and inclusion in science-affine communities are based on the socialisation of given norms and practices as well as on the development of self-identities compatible with these communities (Carlone and Johnson 2007). In this sense, science is not for everyone, but for those complying with the “dominant cultural conventions of thought and action” (Grenfell 2004, 50), producing inequalities between young learners and their connection to science.
Several studies have investigated the way learners develop interests in science, learn about science, develop science attitudes or (aspire to) pursue science related careers putting an emphasis on single demographic features such as gender or social class (e.g., Bricheno 2001; Papanastasiou and Papanastasiou 2004; Barron 2006; Gorard and See 2009; Milgram 2011; Burns et al., 2016). In contrast to these studies, we focus on the intersection of class and gender and investigate 1) which factors influence the formation of positive science attitudes of young learners. 2) We explore how the social backgrounds of young learners potentially influences their opportunities of connecting with science (Archer et al., 2013a; Archer et al., 2014). Building on the results of a large-scale survey on science learning for youths aged between 8 and 21 in 17 countries across Europe and Israel/Palestine, we aim at identifying potential boundaries for young learners in connecting with science. This knowledge may support the development of more inclusive concepts of science learning and hence provide ways to tackle inequalities in science learning. The chosen theoretical lenses guiding this investigation are twofold:
Firstly, the perspective of learning ecologies is applied, highlighting the influence of young learners’ personal backgrounds on their opportunities to connect with science. The concept of learning ecologies explains a child’s development in relation to their environment. Based on Barron (2006), this article conceives learning ecologies as “the set of contexts found in physical or virtual spaces that provide opportunities for learning […]. Each context consists of a unique configuration of activities, material resources, relationships, and the interactions that emerge from them”(Barron 2006, 195). In the context of science learning, this perspective suggests that the specific relation between individuals and the environment shapes the way information is perceived and acquired. Whilst the conceptions of science learning ecologies have been criticised for failing to properly address “affective and extrarational influences” (Johnston et al., 2006, 909), our approach entails that (science) learning is understood as a cognitive, behavioural and affective process (Carlone and Johnson 2007; Falk et al., 2016) that is socio-culturally embedded. Prior knowledge of science topics that learners are interested in and their interactions with others (Anderson et al., 2015) shape youths’ educational experiences in and across formal and non-formal settings (Bevan 2016).
Secondly, an intersectional approach is applied, aiming at studying science attitudes and the engagement in various science activities at different intersections of identities, social positions or institutional practices in the educational context (Bowleg 2008; Bauer 2014). Originally developed for capturing the living realities of black women (Crenshaw 1989), the concept of intersectionality emphasises the interaction of gender and race as markers of structural inequalities on and across each other. Henceforth, the approach has been opened up to further categories of social differentiation and discrimination such as migration histories, class, dis_ability or sexual orientation. In our context, this research lens suggests that taking account of additional intersections can establish further layers of dis-/identifying with science (Brickhouse et al., 2000; Bell et al., 2009; Hazari et al., 2013). Thereby, intersectionality complements the concept of “science identities,” which is gaining attention in science education literature (Carlone and Johnson 2007). Despite the awareness that intersectionality itself offers a useful and valuable conceptual framework of studying science learning, until now, it has been hardly used in respective research (see e.g., Artiles 2013; Traxler et al., 2016; Avraamidou 2020; Cochran et al., 2020), and is difficult to operationalise from a quantitative perspective (see e.g., Bauer 2014; Rouhani 2014). Nevertheless, in this study intersectionality was considered at the level of survey design, sampling strategy as well as analysis.
The intersectional learning ecology approach pursuit in this paper demonstrates the relevance of intersectional analyses to obtain a fine-grained understanding of factors affecting (in)equity in science learning. Inspired by the work of Louise Archer and colleagues for the United Kingdom context (2012, 2013, and 2014) and studies following the concept of cultural reproduction (Bourdieu 2001), this paper specifically focuses on the intersections of gender and the socio-educational background that structure learners’ self-identities, their social environments and cultures, as well as their chances to connect with science.
As the approach of learning ecologies puts forward, concepts of science and those who partake in it do not exist in a vacuum. Families, attitudes of peers and the formal educational system are regarded as important contexts affecting the formation of science attitudes (Bricheno 2001).
The family is the first and most important place of primary socialisation; where knowledge, skills, norms, values, and traditions are learned (Anastasiu 2011). The family’s educational, financial, and occupational background and hence its social class and socio-economic status have been identified as stratifying factors of participation and accomplishment in the formal education system (Bell et al., 2009; Gorard and See 2009; Archer et al., 2012). Additionally, formal educational systems provide for the reproduction of the social status of those complying best with its norms and educational concepts (Goldthorpe 2007).
Consistent with this, researchers have found that the formal education system does not create spaces where multiple perspectives of knowing and showing science can emerge and hence, does not foster diversity (Barton and Osborne 2001). Narrowly defined and acknowledged science identities in turn do not appeal to a broad range of students coming from diverse living situations, entering the formal education system equipped with their own set of knowledge, cognitive skills and beliefs of how the world works (Bell et al., 2009; Jordan 2010). This is why the formal educational system acts as a gatekeeper potentially restricting the education pathways of learners not sharing the same habitus (Bourdieu 2001).
Scientists are perceived as persons working with their minds. Accordingly, this popular image affects how children connect with science depending on their social backgrounds. Following the theory of cultural reproduction (Bourdieu 2001), Archer and colleagues introduce the concept of the “family habitus” (Archer et al., 2012, 886) to refer to the science capital of a family. This capital not only entails specific “resources, practices, values, cultural discourses” (Archer et al., 2012, 886), but also includes the family identity in which one is born into. As such, well-off middle-class families tend to condense science-specific cultural and social capital with a sense of a science-related image providing a supportive context for their children’s science interests (Archer et al., 2012). Working class families with a lower socio-economic status and less cultural capital in turn tend not to perceive science as part of their being. Instead, science is not part of their daily family practices and hence something rather “unthinkable” for their children (Archer et al., 2012). Carina Altreiter (2017) supports this argument by explaining that habitual rooting of career aspirations of the Austrian working-class is related to the idea of using one’s own hands and body instead of working predominantly with the mind.
Children from economically poorer families are not necessarily found to be less interested in science. However, they are found to be less likely to choose science as a subject, based on its perceived difficulty, its image within their social class and the influence of their families (Gorard and See 2009).
The popular image of natural scientists, as intelligent persons predominantly working in a lab, is also framed by the gendered division of labor. Working with one’s mind is not only predominantly attributed to higher educated people but has also historically been framed as masculine (Hausen 1976). In contrast to the feminine-constructed caring body and emotionality, the human brain and rationality are constructed as part of a male gender identity (Hausen 1976). Stemming from the 19th century, attributing science and brain work to men shapes the dominant image of science and scientists until today; an image re-creatable by children as young as the age of six (Carlone and Johnson 2007; Archer et al., 2013a). These relational gender stereotypes and conceptions of femininity and masculinity can make science seem “incompatible with girls’ performances of popular/desirable hetero-femininity” (Archer et al., 2013a, 181). On this basis, gender disparity in science was found to be reversed with students not identifying as heterosexual (Hughes 2018).
While at a young age, science interests do not statistically significantly vary with gender, binary (i.e., male and female only, as other groups have not been researched) gender differences manifest themselves as children grow older (Archer et al., 2012; DeWitt et al., 2013). Gender stereotypes have also been found to be reproduced by parents (Bell et al., 2009). In older studies, mothers overestimated the mathematical skills of their sons and underestimated those of their daughters. Mothers also tended to talk about science more with boys than girls (Frome and Eccles 1998). In more recent studies, fathers’ increasing gender stereotypes were observed to be negatively related to girls’ interests in mathematics, while positively related to boys’ enthusiasm in the subject (Jacobs et al., 2005). Further, fathers tended to employ more cognitively demanding speech with boys than girls (Tenenbaum and Leaper 2003). In short, parents and other adults support and encourage boys and girls differently (Falk et al., 2016). Given the influences of stereotyping and the social influences of peers, teachers and parents, some researchers still find female learners reporting less positive science attitudes than male learners (Bricheno 2001). Miller and Budd (1999) suggest that stereotyped views of science tend to decline for girls, while boys are more likely to hold stereotyped views of science (Bricheno 2001), stipulating more positive science attitudes in general.
In addition, (Archer et al., 2013a) underline that ideas of (hetero-) masculinity and (hetero-) femininity differ by social class. Boys from working-class contexts are less likely to see how science relates to their lives than boys from middle and upper classes (Archer et al., 2014). The class-gender intersection, however, exacerbates more strongly with regards to girls from working-class backgrounds, resulting in their exclusion from both corresponding to the androcentric ideal of science students and having science-related future aspirations (Archer et al., 2013a).
The paper at hand builds on these findings and is structured as follows: First, we start with the operationalisation of the main theoretical concepts used in this paper: positive science attitudes, non-identification with science and engagement in (science) learning. In this context, we also introduce the two datasets analyzed. Second, we present the empirical results, where we start with summarising the results of a regression analysis of the main parameters affecting the development of a positive science attitude. We then continue to explore group-based intersectional differences in the way young learners connect with science, focusing on gender and educational capital. In the conclusion, we discuss how our findings interrelate and potentially contribute to a more inclusive concept of science learning and highlight the benefits of our combined methodological approach.
To investigate differences in the way young learners connect with science on the basis of their learning ecologies and self-perception, we built on the work by John Falk et al., (2016) and developed a self-administered quantitative survey1. Dimensions addressed in the survey comprise the everyday engagement with science, the social environment, attitudes towards science in general and attitudes towards science lessons at school. In addition, to implement intersectional analyses later on, socio-demographic information about the learners’ age, gender identities (operationalised as an open question “What gender do you identify with” and coded afterwards), migration histories (operationalised as countries born in vs. country living in and languages spoken at home) and self-perceived dis_abilities was collected. While race/ethnicity exceeds the collected dimension of migration histories and was considered an important marker of inequality for intersectional research, its operationalisation in the European context turned out to be beyond the abilities of the survey. The multinational context of the project would have required a country-specific operationalisation of ethnic self-identification allowing for a context-specific interpretation of the collected results (Hoffmeyer-Zlotnik and Warner 2010). In addition, being a sensitive category, several EU Member States have legal frameworks strongly regulating the collection of data on ethnicity, with e.g., France prohibiting the collection of data on ethnic origin (Farkas 2017). As a result, race/ethnicity was not surveyed and is therefore excluded from this study. Questions about the highest level of education and current field of employment of the parent(s) were included in the consent sheet, completed by the parents (in case of minors) or young learners themselves (in case of older learners).
The research design was aligned with the project consortium of SySTEM 2020, an EU-funded research project focussing on non-formal science education, coordinated by Science Gallery Dublin and represented by research institutions and 19 museums, science centers, and maker spaces located in Austria, Belgium, Bulgaria, Czech Republic, France, Germany, Greece, Ireland, Israel/Palestine, Italy, Netherlands, Portugal, Serbia, Slovenia, Spain, Sweden, Switzerland, and the United Kingdom.
The targeted population was represented by learners aged between 8 and 21 who engaged in non-formal science educational events of the partner institutions. The specifically set-up convenience sampling strategy was embedded in an intersectional framework where the project partner institutions were asked to reach out to all their user groups putting an emphasis on engaging different age groups, gender identities and learners with migration histories. The project partners reached out to their visitor base as well as associations working with non-dominant youths and schools to engage survey respondents. To investigate possible changes of the learning ecologies over time (Barron 2006), the survey was set up in two waves, engaging the same participants twice within the timeframe of a year. The source questionnaire designed in English was piloted using cognitive probing interviews (see for example Prüfer and Rexroth 2005; Miller et al., 2014). Each of the 14 subsequently translated questionnaires was tested again using cognitive probing interviews with young learners to ensure the quality of the questionnaire and the resulting data.
In the first wave, learners were invited to participate in a workshop organised by each of the involved project partners where they completed the survey on paper. These workshops, which were all organised differently and focussed on different science-related topics, took place between February and April 2019. The same participants were reached out to in wave 2, between February and June 2020. In addition, several new respondents, who fit the sampling profile, were involved in the second wave, to reach a comparatively large sample size as in wave 1. During the second wave, the survey could also be completed online, an option that was particularly useful based on the specific COVID-19 induced measures of physical distancing during that time.
In total 1,468 individuals were engaged in the survey; 736 of them completed the survey twice and hence their responses could be investigated with regards to changes between measurement times. The data of wave 1 and wave 2 was matched by a pseudonymised ID, ensuring data protection rights. All data collected was analyzed descriptively. The sample surveyed twice (abbreviated “twice”) and a pooled sample of 732 learners, who answered the survey only once, either in wave 1 or wave 2, (abbreviated “once”), were also analyzed exploratorily, constructing a regression model with a focus on the impact of age, gender, the families’ educational capital, and their various intersections on the formation of science attitudes of young learners.
Most learners identified themselves within the gender binary as male or female or boys and girls respectively. Only 10 learners identifying beyond the gender binary participated in the survey, eight of them make part of the sample surveyed twice (1% of those answering twice). Based on this small sample size, their answers unfortunately needed to be excluded for gender-based analyses. From a binary perspective, both samples were about gender balanced.
In general, learners from low, medium, and highly educated households were part of the surveyed population. To explore the impact of different educational backgrounds of parents and their social and occupational status on their children’s possibilities to connect with science, an index measuring “educational capital” of the learners’ families was created. Following Bourdieu, educational capital can be defined as “incorporated cultural capital” (Bourdieu 2007, 95), an educational status that has been achieved by young learners’ parents and has become part of the self-identity of young learners, shaping their values and attitudes. In our study, educational capital was hence measured as index using the highest level of education, the current profession of the learners’ parents (collected according to ISCO-08 major groups) as well as the number of physical reading materials available in the household (DeWitt et al., 2013) (Cronbach’s α = 0.572). In case that both parents’ educational and professional status was collected, only the data of the higher-ranking parent was included in the index (International Labor Organisation 2008). The resulting index ranged from 2 (indicating the lowest score) to 10 (indicating the highest score). The scale was then summarised into three categories: low educational capital, ranging from 2 to 4.5; medium, ranging from 4.6 to 7.5, and high educational capital, ranging from 7.6 to 10.
Apart from physical reading materials available at home, resources for learning were measured by counting the number of electronic devices available, as well as music instruments. Most of the surveyed learners (>90%, 1,398 < n < 1,410) have devices such as computers, smart phones and TVs in their homes that might enable science learning. 70% of all individuals, who answered this question (n = 1,418), indicated having musical instruments at home. Learners from higher educated households were more likely to have musical instruments at home (twice: χ2 (6) = 87, p < 0.001, once: χ2 (6) = 52, p < 0.001).
Since the sampling strategy targeted youth visiting and using non-formal science institutions, learners from households with higher educational capital are overrepresented in both samples. Comparing both samples, learners from households with low educational capital, learners with histories of migration and learners indicating facing serious difficulties with hearing, speaking or moving were more strongly represented in the sample surveyed once. More of these learners dropped out after wave 1 (n = 589), which included 1,322 respondents in total. In contrast, newly included members of wave 2 (n = 146), rolled out as an online survey among the institutions’ contacts fitting the description of the target group of the study, largely came from more privileged groups. Young learners who participated twice in the survey tended to live more often in cities, be slightly older and therefore tend to have a higher level of education themselves.
Learners who participated twice in the survey are also more likely to express a particularly positive science attitude (70% did so vs. 24% of one-time-respondents). The process of positive self-selection caused by the approximative longitudinal design impacted the representativeness of the groups, which is why the sample of wave 1 or wave 2 only respondents is more representative of the young learners reached by the institutions offering non-formal science learning programmes in general.
To measure science attitudes, engagement, and aspiration across European contexts we adapted survey questions suggested by the Synergies project in the US (Falk et al., 2016) and the ASPIRES project in the United Kingdom (Archer et al., 2013b) as both operationalised a learning ecologies perspective: To investigate the underlying, latent and multidimensional elements of science learning in various contexts and attitudes towards science-learning, an exploratory principal component analysis (PCA) was conducted with data collected from both samples at the time of wave 1 (n = 1,322). This method identifies the minimum numbers of factors consistently, summarising the interrelated items into a single, yet multidimensional variable (Field et al., 2012).
Exploring the underlying factors that explain young learners’ ways to connect with science, 18 items were included in the PCA.3 From this, five factors were identified: 1) a positive science attitude, 2) non-identification with science, 3) learners’ attitudes towards science lessons in school, 4) parental science relevance, and 5) friends’ science attitudes. Each factor was then modeled as a mean-based index summarising the related variables, values ranging from 1 to 5, with 1 implying the strongest possible opposition towards the measured concept and 5 the strongest agreement.
The factor-based index called “positive science attitude” (Cronbach’s α = 0.86, indicating high scale reliability) summarises the enjoyment and fascination with science, an interest in science and an idea of how science relates to one’s own life, including potential career paths. Science attitudes in general capture the emotional orientation of an individual to respond favourably to science (Papanastasiou and Papanastasiou 2004). The results of the factor analysis imply that a positive concept of science relates to the individual learners’ lives and hence touches on aspects which are often considered as part of the learner’s self-identity (e.g., Carlone and Johnson 2007).
The PCA also pinpoints the factor-based index “non-identification with science” (Cronbach’s α = 0.59), which is more explicitly related to the concept of science identity (Falk et al., 2016) summarising negative attitudes such as the feeling that science does not relate to oneself, to one’s way of learning and thinking and feeling that others relate more easily to science.
The two separate factors identified in our PCA explain 65% of overall variations and indicate the need to empirically differentiate between “positive science attitudes” and the “non-identification with science,” as both relate to different aspects of the multidimensional concept of science identities.
The other three factors (of five factors identified by the PCA) are considered as structuring young learners’ science ecologies, and hence as potentially explaining differences in the way young learners connect with science and build their own science identities.
The PCA identified index “attitude towards science lessons in school” (Cronbach’s α = 0.85) mirrors the learner’s excitement with science classes in the formal education system. The factor “parental science relevance” (Cronbach’s α = 0.79) captures parental influence on individual science attitudes, describes parental science interest and captures parent-child discussions about science. Lastly, the factor “friends’ science attitudes” (Cronbach’s α = 0.84) summarises the perceived positive science attitudes of the learners’ close friends.
A second obliquely rotated PCA was applied exploring the different ways young learners engage with science. As informal learning processes are ubiquitous, the activities probed here do not only include activities such as watching a video about science, but also learning to play a musical instrument, or gardening and were selected on the basis of the Synergies project (Falk et al., 2016). Among all activities three factors4 were identified, two of them are discussed hereafter: 1) The factor “self-directed science-learning” (Cronbach’s α = 0.63) summarises the regular engagement in building things, taking them apart or repairing them; doing science experiments at home; watching science-related videos; visiting a website about science, maths or technology outside of school. On this basis, a mean-based index (range 0–4) summarising the frequency of engagement in these four activities was constructed. 2) The factor “arts-based learning” (Cronbach’s α = 0.65) relates to regular engagement in arts activities, which were found positively relating to science achievement (e.g., Črnčec et al., 2006; Hille and Schupp 2015). This factor in our study includes the following variables: learning a musical instrument; pursuing dance; partaking in drama or acting classes; pursuing correlating after-school programmes. This factor was also remodeled as a mean-based index (range 0–4).
To explore the ways young learners connect with science, a stepwise logistic regression model was created and run with the two different samples (“twice” and “once”). The regression model provides insights on the main factors supporting the development of positive science attitudes (dependent variable) showing the relationship between each of the independent variables included in the model with this variable.
Answering the question how different groups of young learners connect with science the second part of the results section uses indicators to investigate significant (Bonferroni-corrected) group-based differences by age, gender and educational background of the family and the combined effect of age and gender (four groups, male and female below and above age 12), as well as gender and educational capital (six groups, low–medium–high per gender). This approach enabled to consider the intersections of gender and the learners’ social backgrounds and their manifestation in different age groups. Doing so, we followed the observations by Archer et al. (2012) as well as Miller and Budd (1999) that gender stereotypes exacerbate with age and differ according to the educational background and the socio-economic status of a family.5
Which dimensions of young learners’ learning ecologies influence the formation of positive science attitudes? In the following section the results of a stepwise logistic regression model are presented. To measure positive science attitudes the PCA-introduced mean-based index was used. In line with the approach of Hayes and Tariq (2000), we investigated the development of a positive science attitude, by recoding the science attitude mean-based index as a binary dependent variable with 0 indicating a negative or neutral science attitude (1–3 on the scale), and 1 indicating a positive science attitude (scoring 4 or 5 on the original index).
The model gives information on the probability of a positive science attitude developing, given the value of all included independent variables (Field et al., 2012). The regression model’s assumptions were tested investigating the linear relationship between predictors and the logit of the outcome variable, testing the independence of errors using the Durbin Watson Test and investigating levels of multicollinearity using variance inflation factors.
Parameters potentially influencing positive science attitudes included in the regression model were sociodemographic variables (such as age, gender, and educational capital), variables characterising the social context of learners (such as parental science relevance and friends’ science attitudes), learners’ engagement in (science) learning activities outside the classroom and their perception of science in school. A detailed list of all variables included in the final model can be found in Table 1.
The findings of earlier studies (e.g., Archer et al., 2013b; Falk et al., 2016) as well as our intersectional lens determined the order of variable inclusion with socio-demographic variables being included first (Bauer 2014). Model fits were judged using Cox and Snell’s R2 (R2CS) as approximated indicator for the share of explained variance as well as the Akaike Information Criterion (AIC), as goodness of fit measure. The model was tested with the two different samples, the sample surveyed twice, and the sample surveyed once. Only variables significantly improving the explanatory value were kept in the model, others were sequentially removed. A full list of all variables originally tested can be found in the Supplementary Material. For reasons of comparability, the final mode includes the same variables for both samples, except for the parameter of change in value of science attitudes between wave 1 and wave 2 (called “time-effect”), which could only be measured among the sample surveyed twice.
For the group surveyed twice, the logistic regression model comprised 14 independent variables that explained more than a third (R2CS = 0.39) of the variations of a positive science attitude (n = 614). Based on the overrepresentation of respondents from one participating organisation in the sample surveyed once and their significant influence on the model, the second model was weighted. The explanatory value of this model was R2CS = 0.29, with 13 dependent variables (n = 622). Although the same variables were tested with both samples, except for the “time-effect,” the applied independent variables tended to capture the variance of positive science attitudes of the sample surveyed twice better.
Odds ratios (OR) reported hereafter, signify the change of odds for the outcome variable (positive science attitude) resulting from a unit change in the predicting variable, with ORs exceeding 1 implying a positive change, ORs below 1 a negative change (Field et al., 2012). Since ORs are difficult to compare both within and across models, average marginal effects (AME), interpreted as the average percentage change in likelihood, were used as additional measure of the effect of the independent variables on the variance of positive science attitudes (Wolf and Best 2010).
Interestingly, neither educational capital, nor gender significantly impacted the likelihood of developing a positive science attitude in both samples. Yet, for reference and for understanding their relation to other variables included, both variables were retained in the final model as discussed hereafter. Possibly, despite their hierarchical inclusion, their effects are mediated by other variables included in the model. Other tested socio-demographic variables such as migration histories, dis_abilities and fluency in multiple languages equally yielded no significant effects and were in turn excluded from the final model as listed in Table 1.
Only one variable turned out to influence positive science attitudes highly significantly among both samples; namely the enjoyment of science lessons in school (twice: b = 1.00 p < 0.001, once: b = 0.98, p < 0.001). Enjoying science classes more by one unit increases the likelihood of having a positive science attitude by 10% (AMEtwice) to 13% (AMEonce). The connection between the enjoyment of science lessons at school and the general science attitudes is reaffirmed by the impact of the learners’ own perception of their performance at school on the probability to develop a positive science attitude in the sample surveyed once. A better impression of a learner’s own performance at school supports the development of a positive science attitude in the sample surveyed once (b = 0.31, p < 0.05, AME = 0.04, OR = 1.36), whereas the impact of the same variable yields ambiguous results in the sample surveyed twice (b = 0.13, p < 0.05, AME = 0.03, OR = 0.86). Information from the involved project partners on the sampling strategy implemented further shows significant effects of school involvement when engaging survey/workshop participants. In case the non-formal institutions involved in the project cooperated with schools when recruiting survey participants (which was the case for 75% of engaged participants in total), the probability of the respondents enjoying science and ability to see how it relates to their world, rose by 3% (AME) in the group of respondents answering the survey twice and by 14% in the sample surveyed once. This might hint at a preselection of schools, who have built strong ties with non-formal science institutions and thereby, potentially, stipulated science interests of their students.
In addition, the model for both samples was improved once two specific age-groups, modeled according to age-based response tendencies of the dependent variable, were included. The youngest respondents (8–10 years) of the sample surveyed twice, were found less likely to exhibit a positive science attitude (b = -1.24, p < 0.01, AME = −0.13, OR = 0.29), possibly hinting at ongoing processes of attitude development. In turn, young adults (14–17 years) of both samples were more likely to have a positive science attitude (twice: b = 0.68, p < 0.05, AME = 0.06, OR = 1.97, once: b = 0.92, p < 0.01, AME = 0.12, OR = 2.51). This outcome potentially confirms results of former studies demonstrating that attitudes towards science become rather stable at age 14 and those learners might have positively self-selected (Archer et al., 2013b). The oldest age group (18–21 years), however, does not mirror this trend and –based on its insignificance (possibly group size related) –is not included in the final model (Table 1).
Involving the engagement in specific forms of (science) learning significantly improves the model for both samples. Concerning respondents only surveyed once, the regular engagement in arts-based learning, measured by the PCA-based index introduced earlier, influences the probability of also developing a positive science attitude (b = 0.30, p < 0.05, AME = 0.04, OR = 1.35). The sample surveyed twice does not indicate clearly positive effects of arts-based learning. Instead, this sample shows a significant impact of regular engagement in self-directed science learning (b = 0.43, p < 0.05, AME = 0.04, OR = 1.53), which yields comparable effects but lacks significance among the respondents surveyed once (b = 0.32, p < 0.1, AME = 0.04, OR = 1.37).
Having friends, with positive science attitudes makes it more likely to develop a positive science attitude (twice: b = 0.364, p < 0.01, AME = 0.04, OR = 1.40; once: b = 0.31, p < 0.05, AME = 0.04, OR = 1.38). Interestingly, the influence of parental science relevance on the science attitudes of learners is only identified as a parameter significantly impacting science attitudes of two-times respondents. While no significant impact of parental science relevance is evident in the sample surveyed once (b = 0.18, p > 0.1, AME = 0.02, OR = 1.20), parents who are interested in science and talk to their children about it significantly impact the positive science attitude in the sample surveyed twice (b = 0.56, p < 0.001, AME = 0.06, OR = 1.75). In turn, siblings supporting science learning have a negative influence on the learners’ probability to develop a positive science attitude. This effect is highly significant in the sample surveyed twice (b = −2.80, p < 0.01, AME = −0.29, OR = 0.06), but the same insignificant tendency, is visible in the sample surveyed once (b = −1.9555, p = 0.051, AME = −0.262, OR = 0.1421). 37% of the learners in the sample surveyed twice (n = 736) and 40% of the group of respondents surveyed once (n = 732) perceived their siblings as encouraging. Possibly, the siblings’ encouragement might be prompted by specific living conditions not included in the model, which also negatively correlate with the chance of developing a positive science attitude.
Among one-time respondents it is not so much the learners’ socio-cultural environments, but their own intrinsic motivation that highly significantly impacts their likelihood to develop positive science attitudes (b = 2.34, p < 0.001, AME = 0.32, OR = 10.43). Comparing the two waves of the sample surveyed twice, an effect of time on science attitudes is also evident. While only a small fraction (9%, ntwice = 728) of the learners experienced changes exceeding a one-point difference on the index between wave 1 and wave 2, a positive change between the two waves significantly improves the probability of a positive science attitude (b = 1.15, p < 0.001, AME = 0.12, OR = 3.15).
Summarising the results of the regression model, we see that the learners’ self-motivation to regularly engage in science related activities (including arts-based activities potentially fostering informal science learning), science interests at school and the self-perception of young learners’ performance at school explain the likelihood of having a positive science attitude best. Yet, who are the young learners with a positive science attitude and a low probability to non-identify with science? What differences do learners’ identities related to gender, age and educational capital make? To answer these questions the effects of age, gender and educational capital of young learners were explored.
Group differences were tested against the indices stemming from both earlier introduced PCAs identifying dimensions of young learners’ science attitudes and engagement with science. The effects of age, gender, and educational capital on the way young learners of different socio-economic status connect with science are reported according to the following dimensions: 1) science attitudes and non-identification with science 2) engagement in learning, and 3) importance of science for the social environment.6
In total, 70% of the sample surveyed twice (ntwice = 728), but merely a quarter (24% nonce = 730) of the sample surveyed once indicated a positive science attitude, hence enjoy science learning and see how science relates to their everyday lives. In contrast to the regression analysis, the comparison of means shows significant impacts of the educational capital of the learner’s probability to exhibit a positive science attitude. Learners from highly educated backgrounds are significantly more likely to exhibit a positive science attitude (twice: mhigh = 3.93, sd = 0.39; once: mhigh = 3.92, sd = 0.90) than learners with low (p < 0.01, 0.16 < r < 0.25) and medium educational capital (p < 0.01, 0.14 < r < 0.16).
These differences by educational capital intersect with gender across both samples, with the largest effects arising between male learners with high (mm-high = 4.08, sd = 0.37) and low educational capital (mm-low = 3.46, sd = 0.47 p < 0.01, r = 0.29) in the sample surveyed twice. In the sample surveyed once, about equally large effects can be found between male (mm-high = 3.95, sd = 0.89) and female learners (mf-high = 3.91, sd = 0.91) from highly educated families when compared with female learners with low educational capital (mf-low = 3.27, sd = 1.23, p < 0.001, r = 0.29).
In total, 15% of the learners in the sample surveyed twice (n = 724), and 19% of the respondents surveyed once (n = 724) do not consider science being a part of their identity, i.e., they do not identify with science. This non-identification with science does not significantly vary with time or age. However, also with this dimension of science identity it is the educational capital that significantly influences a learner’s probability to non-identify with science, with the largest effect being between learners with low educational capital backgrounds (twice: mlow = 3.13, sd = 0.56; once: mlow = 3.03, sd = 1.23) and respondents from highly educated families (twice: mlow = 3.93, sd = 0.38; once: mlow = 3.63, sd = 1.08), (with significance levels p < 0.01, and effect sizes between 0.25 < r < 0.26). While no significant gender-based differences were found at a general level, we see an impact of gender identity within groups of learners, with male learners from highly educated backgrounds being the least likely to non-identify with science (twice: mm-high = 3.9, sd = 0.37; once: mm-high = 3.75, sd = 1.00).
In contrast to general science attitudes, measured by the two indices of a positive science attitude and the non-identification with science, the attitudes towards science lessons in school neither vary significantly by educational capital nor gender. About two thirds (67%, ntwice = 656; 65%, nonce = 698) of all surveyed learners perceive their science lessons in school positively. Among the sample surveyed twice, younger learners are more likely to enjoy their science classes than older ones (p < 0.001, 0.22 < r < 0.33), with the largest effect between the youngest (m8–11 years = 4.23, sd = 0.47) and the oldest age groups (m18–21 years = 3.5, sd = 0.52, p < 0.001, r = 0.33). No such effects are visible among the group of one-time surveyed learners.
An investigation of activities, learners engage in out-of-the-classroom settings, which might foster informal (science) learning shows group-based differences based on age and gender. In general, 23% (ntwice = 735 and nonce = 727) of the learners indicated that they engage in self-directed science learning activities at least once a week (a score of 3 or 4 on the constructed index) and about one third (32%, ntwice = 735) of the two-times surveyed learners, and a quarter (25%, nonce = 731) of learners surveyed once engage at least weekly in arts-based activities which can potentially foster science learning.
Across both samples, male learners are more likely to engage in self-directed science learning (p < 0.001, 0.14 < r < 0.20). This gender-based difference is stronger at an early age as depicted in the data from the sample surveyed twice (p < 0.05, r = 0.31), but is also present among male and female teenagers in both samples (p < 0.01, 0.19 < r < 0.22). Gender-based differences in self-directed science engagement also intersect with educational capital; male learners from high education backgrounds are, on average, most likely to engage in self-directed science learning (twice: mm-high = 2.12, sd = 0.41; once: mm-high = 1.92, sd = 0.9). Their probability to do so significantly differs from female learners of all educational strata, with the strongest effects found in the sample surveyed twice, comparing them with female learners with high educational capital (mf-high = 1.53, p < 0.001, r = 0.34) or medium educational capital (mf-med = 1.52, p < 0.001, r = 0.34).
In contrast, female learners are more likely to engage in arts-based activities which can potentially foster science learning (p < 0.001, 0.17 < r < 0.18). Introducing information on the educational capital of the learners, we see learners from highly educated backgrounds significantly engage more often in arts-based science learning activities in both samples (p < 0.001, 0.22 < r < 0.33). The combined analysis of educational capital and gender demonstrates that gender differences do not manifest among learners from low educated households, but among learners with medium (twice and once, p < 0.01, 0.22 < r < 0.23) and high educational capital (once, p < 0.05, r = 0.19). Interestingly, the engagement in arts-based learning is the only form of engagement showing significant changes over time. Between wave 1 and wave 2, learners aged from 8 to 11 increased their engagement in arts-based activities (mtwicew1 = 1.74, sd = 0.45 to mtwicew2 = 1.97, sd = 0.45, p < 0.001, r = 0.25). These changes are particularly attributable to male learners (mtwicew1 = 1.39, sd = 0.40 to mtwicew2 = 1.57, sd = 0.40, p < 0.001, r = 0.20), thereby decreasing overall gender differences (rtwicew1 = 0.20 to rtwicew2 = 0.17, p < 0.001). Reasons for these changes can, however, only be hypothesized.
Parents play a major role in the learners’ engagement with science related activities in a broad sense (Falk et al., 2016): 90% of the learners in the sample surveyed twice and 88% of those respondents surveyed only once indicated that their parents encouraged them to engage in at least a quarter of all specified science-related activities. About one third of the surveyed learners also indicated that siblings (twice: 37%, once: 40%) and grandparents or other relatives (35%) encouraged them to engage in broadly science-related activities. Considering the general social environment of the learners, one can deduce that friends (twice: 63%, once: 51%) are perceived to be more supportive in science-learning than siblings and grandparents. In relation to the results of our regression model, support may have positive as well as negative effects on young learners’ science attitudes.
Given the important role of parents for individual learning ecologies a further investigation of parental science relevance was pursued. In contrast to questions of support structures elaborated above, these questions literally addressed the concept of science. Asked, whether science is present in their homes more than a third of the respondents answered that science does play an important role in their family (twice: 33%, once: 36%), while roughly an equal share of respondents (twice: 37%; once: 35%) indicated that their parents are not interested in science.
In line with earlier findings (e.g., Archer et al., 2012) our results confirm the influence of educational capital on science relevance in the learners’ homes; with learners with a high educational capital being more likely to perceive science as an important part of their home cultures than learners with medium and low educational capital (twice p < 0.001, 0.22 < r < 0.24; once: p < 0.01, 0.13 < r < 0.18). From an intersectional perspective, the strongest effect in the sample surveyed twice can be identified when comparing female learners with low educational capital (mf-low = 2.51, sd = 0.51) to male learners from highly educated backgrounds (mm-high = 3.35, sd = 0.46, p < 0.001, r = 0.30), where the latter regard science as being embedded in their homes. The intersectional gender-educational-capital-perspective does not yield significant results in the sample surveyed once. As gender distributions were comparable among the samples, this suggests that primarily educational capital and not so much the gender aspect influences whether science is perceived as an important part of a learner’s home-culture.
In addition, age was found to be influential when investigating science relevance at homes; with younger learners reporting a significantly higher presence of science in their homes than older ones (p < 0.05, 0.14 < r < 0.18). Differences by age might be related to changing parent-child interactions: once children grow older, they become more independent and more certain about their own interests. This correlates with findings from educational studies (Barron 2006; Stangl, 2021) suggesting that peers become more important for teenagers, while parental perspectives are likely to get scrutinised once children grow older.
In general, friends and peers represent an important element of a young person’s science learning ecology (Bevan 2016). More than a third of both samples (38%, ntwice = 716; 39%, nonce = 719) indicated that their close friends enjoyed science. With regard to their peers’ science attitudes, we see group-based differences by gender and age. Among the two-times respondents, the youngest age group of 8- to 11-year-olds (m8–11 = 3.40, sd = 0.62) is on average most likely to have a science-positive peer environment which significantly differs from the 12 to 14 age group (m12–14 = 2.86 sd = 0.62, p < 0.001, r = 0.23). Across both samples, young male learners below the age of 12 are significantly more likely to have friends interested in science, than female teenagers above the age of 12 (p < 0.05, 0.15 < r < 0.19). The sample surveyed twice additionally depicts significant, yet small differences by educational capital: Learners from highly educated backgrounds are slightly more likely to have friends who have a positive attitude towards science (mhigh = 3.21, sd = 0.60) than learners from medium (mmed = 2.94, sd = 0.59, p < 0.05, r = 0.12) and low educational households (mlow = 2.84, sd = 0.76, p < 0.05, r = 0.13).
In summary, to answer the questions leading to this chapter: Who are the young learners who positively connect with science? The results of the comparisons highlight the hierarchical relation between gender and the impact of educational capital, with low educational capital affecting young learners’ possibilities to connect with science more strongly than gender. However, the intersectional approach has made the variations between young learners with lower and higher educational capital visible, illustrating that even though gender and educational capital both impact the probability of developing a positive science attitude that favor male learners from highly educated backgrounds, it is not female, but male learners with low educational capital exhibiting the lowest probability of developing a positive science attitude. When it comes to engagement in specific forms of science learning, gender seems to be the main structuring influence with male learners being more likely to engage in self-directed science learning while female learners are slightly more likely to engage in arts-based learning.
In this paper, we have investigated how to explore the gradual spectrum of unequal opportunities of young learners in connecting with science. We analyzed science learning ecologies by applying an intersectional perspective that allows for a fine-grained understanding of factors impacting equity in science learning that does not blame single individuals for their “deficits,” but rather explores underlying structural inequalities shaping individual learning ecologies (Annamma and Booker 2020). Empirically, we first investigated the isolated effects of parameters influencing the development of a positive science attitude. Following the theory of learning ecologies, socio-demographic information, data on the learners’ social (support) structure, their engagement and their experience of school science lessons were integrated into the model. Secondly, we looked at intersecting group differences between young learners’ science attitudes and non-identification with science, the ways of engaging in activities potentially fostering science learning and their related social context, focusing not only on age, gender and educational capital, but also on their intersecting effects.
Interestingly and contrary to the findings of earlier studies, in our investigation neither educational capital, gender nor migration histories by themselves turned out to significantly improve the logistic regression model of positive science attitude development, outlining instead the importance of the learners’ own motivation and of their attitudes towards science learning in school. This is not a new finding, but e.g., supported by Barmby et al. (2008) who argue that science learning in school has a high influence on the formation of positive science attitudes and therefore represents a crucial point for strengthening young learners’ science attitudes. The results of our regression model hence demonstrate the value of applying the theoretical lens of learning ecologies conceptualising science learning as a culturally and socio-spatially embedded process across different learning contexts when investigating the way learners connect with science (Barron 2006). Even though schools are a place where the dominant concepts of science learning are reproduced, following a prescribed curriculum and (gendered) educational principles most congruent with the norms and conventions of highly educated middle-class families (Bourdieu 2001; Goldthorpe 2007), in our study no significant differences by gender and/or educational capital were identified using comparisons of means. The significant effect of school involvement for selecting survey participants, however, might add a possible explanation: Three quarters of our respondents were engaged using existing collaborations between schools and our partner organisations–museums, science centers or maker spaces offering non-formal science education. The significant effect might hence be not so much related to the equitability of formal education in general, but rather to these existing partnerships, which make formal as well as non-formal science education more accessible to all the students of a classroom. However, the strong impact of science lessons in school also suggests a high responsibility, as these school science experiences largely impact the science learning ecologies of students in a positive as well as a negative way which can cause lasting non-participation (Dawson 2012).
In contrast to the regression model, the intersectionally operationalised comparisons of means indicate the persisting effects of educational capital on the learners’ science attitudes, non-identification, parental science relevance and the learners’ regular engagement in activities, which potentially foster informal science learning. Despite our use of the order-sensitiveness of the stepwise regression model (Field et al., 2012), the (combined) effect of educational capital and gender might have equally influenced the other independent variables and therefore did not turn out statistically significant themselves. An example for this effect is the positive correlation of engaging in arts-based learning and positive science attitude development found for the sample surveyed once. While there are studies suggesting a direct relationship between cognitive processes of engaging with arts and music, such as enhancing creative thinking (Braund and Reiss 2019), spatiotemporal ability (Črnčec et al., 2006), and the learners’ motivation, self-confidence or perseverance (Winner and Cooper 2000), observed empirical correlations between engagement in arts and learning achievements can also be largely attributed to educational capital (Winner and Cooper 2000; Črnčec et al., 2006; Hille and Schupp 2015); learners from more educated families are more likely to get engaged in extracurricular activities entailing arts-based learning. Also, among our surveyed participants the availability of music instruments in the learners’ homes positively correlates with their educational capital across both samples. From the comparisons of means, we equally see intersecting effects of educational capital and gender. Since arts-based learning positively influences the likelihood to develop a positive science attitude among the one-time respondents–our more representative sample–we can assume that effects of educational capital, which obtained a p-value of 0.079, might have turned out significant in the regression analysis, if sample size was bigger. This is, however, not the case for the sample surveyed twice, where the effect of educational capital obtained a p-value of 0.778. One possible explanation for this stark difference between samples might be related to effects of self-selection among double-surveyed learners, who exhibit extremely positive science attitudes in general. For the sample of learners who participated twice (possibly smaller) effects of educational capital–potentially–condense in the independent variable of parental science relevance, suggesting that their home culture is among the most important factors explaining their probability to develop a positive science attitude, hinting at distinct support structures that shape their science learning ecologies. This is supported by former studies suggesting a positive impact of parental support on academic achievement of the learners of all classes (Gorard and See 2009). However, available resources for supporting the learners, such as time, again vary with socio-economic status of the parents (Jordan 2010). The comparatively lower support among the learners only surveyed once in our study, might have stipulated the higher importance of the learners’ own motivation and own interest to engage in science for the formation of a positive science attitude. The negative effect of supportive siblings found in both samples is interesting, yet not explainable from our results and needs further investigation, exceeding the scope of this paper.
While the results of the regression model put the focus on the individual learning ecologies (learning experiences in school, engagement in learning, development of science attitudes over time), the group comparisons provide more detailed analyses of the effects of unequal learning opportunities, introduced and (re-)produced by the prevailing normative concepts of science. The results of the group comparisons empirically support the findings of former studies carried out in other socio-cultural and geographically influenced regions (mainly the United Kingdom and US) and prove their validity also in the European context. Examining our findings more closely from an intersectional perspective enabled us to not only look into inter-categorical differences by educational capital or gender but visualised the many ways both intersect when shaping individual engagement and science learning (Harnois 2013). Social identities “interact to form qualitatively different meanings and experiences” (Warner 2008, 454) adding together to shape experiences over time (ibid). Importantly, these specific manifestations cannot be reduced to single elements analytically, as living realities are constantly shaped by the intersections of dimensions of social inequalities (Bowleg 2008).
Our findings on gender-based differences, differences by educational capital and on their intersections underline the importance of investigating the fine-grained influences of diverse group identities as aspects of individual science learning ecologies. Supported by the intersectional design, the results highlight the role of educational capital on the formation of positive science attitudes and a strong influence of parents on the engagement in science related activities can be observed. This influence becomes less important when young learners grow older, with peers potentially taking over their role (Stangl, 2021). The results of the comparisons also underline the potentially positive effect of the prevailing gendered understanding of science which supports male learners with high educational capital to positively connect with science.
In line with the findings of other researchers (e.g., Carlone and Johnson 2007; Archer et al., 2014; Annamma and Booker 2020) our findings suggest the need to diversify science learning to allow all learners to positively connect with science (Durall 2020). To overcome persisting inequalities, more equitable science learning spaces and offers have to adapt to a diversity of needs and preferences in order to make science activities enjoyable for all (Annamma and Booker 2020; Voigt et al., 2020).
The intersectional approach attempts to open the perspective to an integrated vision of the formation of social identities, including different aspects of social identities. These concepts influence how the formation of individual science ecologies can be investigated: They pinpoint the methodological limitations of an empirical research that has to decide which intersections of identities will be investigated in advance (Warner 2008). However, and importantly, this difficulty is not only related to the intersectional approach, but also to the concept of science identities (Carlone and Johnson 2007; Steinke 2017). Since unveiling, analysing and ultimately changing inequalities lie at the heart of the concept of intersectionality, the approach gives some guidelines about necessary structural -not individual–dimensions that need to be addressed (Annamma and Booker 2020). This is also why intersectionality might offer a useful conceptual frame for science identities to investigate their dimensions, relationality, and multiplicity (Avraamidou 2020). As much as the decision to focus on specific structural inequality leads to the necessary exclusion of other factors, the focus on gender-identities, educational capital, age, and their intersections selected on the basis of the collected data and the existing literature in this field, this paper provides evidence and exposes the negative effects of the narrow dominant concept of science learning.
For investigating science learning from an intersectional perspective, a diverse sample of respondents is needed that allows for large-enough group sizes, once subgroups are formed and need to be embedded in data collection, sampling procedures as well as methods for data analyses (Bauer 2014; Rouhani 2014; Seebacher 2016). While this approach had been taken into consideration from the very beginning of the research at hand, our operationalisation was limited by practical possibilities of the project setting: From an intersectional perspective it would have been fruitful to be able to analyze gender beyond a binary male-female self-identification (Traxler et al., 2016). Based on the low numbers of respondents identifying beyond the gender binary, only binary analysis of gender could be made. While operationalised, the strong context dependency of migration experiences potentially hindered the index from working equally well for all of the surveyed groups (Harnois 2013). Based on empirical evidence for different contexts, also the dimensions of race/ethnicity (Hazari et al., 2013) and dis_abilities (Bell et al., 2009) should have been included more strongly in the survey, the sampling strategy and the analysis, which however, exceeded the abilities of the study at hand. Future research investigating persisting inequities in science learning are, however, strongly recommended to do so.
Despite not succeeding in overcoming all challenges of embedding intersectionality in a quantitative framework, our two-step methodological (regression analysis followed by comparisons of means) and theoretical approach (integrating the concept of learning ecologies and an intersectional approach), has succeeded in showing the diverse and complex ways young learners’ science ecologies are shaped.
The datasets presented in this study can be found in online repositories. The names of the repository/repositories and accession number(s) can be found below: AUSSDA (https://aussda.at/en/) DOI https://doi.org/10.11587/MG9VQK
The studies involving human participants were reviewed and approved by the Ethics Committee of Center for Social Innovation, Linke Wienzeile 246, 1150 Vienna. Written informed consent to participate in this study was provided by the participants’ legal guardian/next of kin.
LS has collected the data and lead on the data analysis, IV has substantially contributed to the article, both of them share first authorship. CV and JT have provided feedback and helped to systematise the article.
The research was conducted in the realm of the SySTEM 2020 project, which has received funding 893 from the European Union’s Horizon 2020 Framework Programme for Research and Innovation under 894 Grant Agreement No. 788317.
The authors declare that the research was conducted in the absence of any commercial or financial relationships that could be construed as a potential conflict of interest.
The reviewer EU declared a shared affiliation, with the authors to the handling editor at the time of the review.
We would like to thank SySTEM 2020 project consortium coordinated by Science Gallery Dublin and especially the practice partners and third parties who tested the survey and collected the data this paper is based on. We would also like to thank the reviewers, who substantially enriched this paper with their comments.
The Supplementary Material for this article can be found online at: https://www.frontiersin.org/articles/10.3389/feduc.2021.673850/full#supplementary-material.
1The survey can be found in the Supplementary Material to this article.
2Cronbach-α measures the internal reliability among items (see e.g., Field 2012). Values above 0.7 are considered a good fit, values below 0.5 as inacceptable.
3The Kaiser–Meyer–Olkin measure of sampling adequacy was 0.89 and Bartlett’s test of sphericity was significant (p < 0.001). Indicating that correlations between items were sufficiently large for performing a PCA. Only factors with eigenvalues ≥1 were considered (Guttman 1954; Kaiser 1960). Examination of Kaiser’s criteria and the scree-plot yielded empirical justification for retaining four factors with eigenvalues exceeding 1 which accounted for 100% of the total variance.
4The Kaiser–Meyer–Olkin measure of sampling adequacy was 0.67 and Bartlett’s test of sphericity was significant (p < 0.001). Indicating that correlations between items were sufficiently large to perform a PCA. Only factors with eigenvalues ≥1 were considered (Guttman 1954; Kaiser 1960). Examination of Kaiser’s criteria and the scree-plot yielded empirical justification for retaining three factors with eigenvalues exceeding 1 which accounted for 100% of the total variance.
5The results of all group-based comparisons can be found in the Supplementary Material to this article.
6The results of all group-based comparisons can be found in the Supplementary Material to this article.
Altreiter, C. (2017). Subjekt und Klasse. Zur Dialektik von Position und Disposition junger IndustriearbeiterInnen. Vienna: University of Vienna. [dissertation].
Anastasiu, I. (2011). Family and School Understood as Agents of Socialization. Euromentor J. 2/2, 1.
Anderson, D., De Cosson, A., and McIntosh, L. (2015). Research Informing the Practice of Museum Educators: Diverse Audiences, Challenging Topics, and Reflective Praxis. Rotterdam: Sense Publishers – Springer.
Annamma, S. A., and Booker, A. (2020). “Integrating Intersectionality into the Study of Learning,” in In Handbook of the Cultural Foundations of Learning. Editors N. S. Nasir, C. D. Lee, R. Pea, and M. McKinney de Royston (New York: Routledge), 297–313. doi:10.4324/9780203774977-21
Archer, L., DeWitt, J., Osborne, J., Dillon, J., Willis, B., and Wong, B. (2013a). 'Not Girly, Not Sexy, Not Glamorous': Primary School Girls' and Parents' Constructions of Science Aspirations1. Pedagogy, Cult. Soc. 21, 171–194. doi:10.1080/14681366.2012.748676
Archer, L., DeWitt, J., Osborne, J., Dillon, J., Willis, B., and Wong, B. (2012). Science Aspirations, Capital, and Family Habitus. Am. Educ. Res. J. 49, 881–908. doi:10.3102/0002831211433290
Archer, L., DeWitt, J., and Willis, B. (2014). Adolescent Boys' Science Aspirations: Masculinity, Capital, and Power. J. Res. Sci. Teach. 51, 1–30. doi:10.1002/tea.21122
Archer, L., DeWitt, L., Osborne, J., Dillon, J., Wong, B., and Willis, B. (2013b). ASPIRES Report: Young People’s Science and Career Aspirations, Age 10 –14. London: King's College London.
Artiles, A. J. (2013). Untangling the Racialization of Disabilities. Du Bois Rev. 10, 329–347. doi:10.1017/S1742058X13000271
Avraamidou, L. (2020). Science Identity as a Landscape of Becoming: Rethinking Recognition and Emotions through an Intersectionality Lens. Cult. Stud. Sci. Educ. 15, 323–345. doi:10.1007/s11422-019-09954-7
Barmby, P., Kind, P. M., and Jones, K. (2008). Examining Changing Attitudes in Secondary School Science. Int. J. Sci. Educ. 30, 1075–1093. doi:10.1080/09500690701344966
Barron, B. (2006). Interest and Self-Sustained Learning as Catalysts of Development: A Learning Ecology Perspective. Hum. Develop. 49, 193–224. doi:10.1159/000094368
Barton, A. C., and Osborne, M. D. (2001). in “Marginalized Discourses and Pedagogies: Constructively Confronting Science for All in Classroom Practice,” in Teaching Science in Diverse Settings: Marginalized Discourses and Classroom Practice. Editors A. C. Barton, and M. D. Osborne (New York: P. Lang), 7–32.
Bauer, G. R. (2014). Incorporating Intersectionality Theory into Population Health Research Methodology: Challenges and the Potential to advance Health Equity. Soc. Sci. Med. 110, 10–17. doi:10.1016/j.socscimed.2014.03.022
Bell, P., Shouse, A., and Feder, M. (2009). Learning Science in Informal Environments: People, Places, and Pursuits, Vol. 4. Washington D.C.: The National Academies Press. doi:10.1179/msi.2009.4.1.113
Bourdieu, P. (2007). “Die drei Formen des kulturellen Kapitals,,” in Absolute Pierre Bourdieu. Editor J. Jurt (Freiburg, Germany Freiburg: orange press), 95–102.
Bourdieu, P. (2001), Wie die Kultur zum Bauern kommt. Über Bildung, Schule & Politik. Schriften zu Politik & Kultur, 4. Hamburg: VSA-Verlag.
Bowleg, L. (2008). When Black + Lesbian + Woman ≠ Black Lesbian Woman: The Methodological Challenges of Qualitative and Quantitative Intersectionality Research. Sex Roles 59, 312–325. doi:10.1007/s11199-008-9400-z
Braund, M., and Reiss, M. J. (2019). The 'Great Divide': How the Arts Contribute to Science and Science Education. Can. J. Sci. Math. Techn. Educ. 19 (3), 219–236. doi:10.1007/s42330-019-00057-7
Bricheno, P. (2001). Pupil Attitudes: A Longitudinal Study of Children's Attitudes to Science at Transfer from Primary to Secondary School. Greenwich: University of Greenwich. dissertation.
Brickhouse, N. W., Lowery, P., and Schultz, K. (2000). What Kind of a Girl Does Science? the Construction of School Science Identities. J. Res. Sci. Teach. 37, 441–458. doi:10.1002/(sici)1098-2736(200005)37:5<441::aid-tea4>3.0.co;2-3
Burns, H. D., Lesseig, K., and Staus, N. (2016). Girls' Interest in STEM, Girls' interest in STEM IEEE Frontiers in Education Conference; Oct. 2016; Erie, PA, USA. IEE), 1–5. doi:10.1109/FIE.2016.7757645
Carlone, H. B., and Johnson, A. (2007). Understanding the Science Experiences of Successful Women of Color: Science Identity as an Analytic Lens. J. Res. Sci. Teach. 44, 1187–1218. doi:10.1002/tea.20237
Cochran, G. L., Boveda, M., and Prescod-Weinstein, C. (2020). “Intersectionality in STEM Education Research,” in “Intersectionality in STEM Education and Research,” in Handbook of Research on STEM Education. Editors C. C. Johnson, M. J. Mohr-Schroeder, T.J. Moore, and L. D. English (New York, London: Routledge), 257–266. doi:10.4324/9780429021381-24
Crenshaw, K. (1989). “Demarginalizing the Intersection of Race and Sex: A Black Feminist Critique of Antidiscrimination Doctrine,” in Feminist Theory and Antiracist Politics (University of Chicago Legal Forum), 1, 31.
Črnčec, R., Wilson, S. J., and Prior, M. (2006). The Cognitive and Academic Benefits of Music to Children: Facts and Fiction. Educ. Psychol. 26/4, 579–594. doi:10.1080/01443410500342542
Dawson, E. (2012). “I Could’n Think of Anything Worse Than Going There to Be Honest”; Science Museums, Science Centers, and Non-participation. Informal Learn. Rev. 115, 1–6.
DeWitt, J., Osborne, J., Archer, L., Dillon, J., Willis, B., and Wong, B. (2013). Young Children's Aspirations in Science: The Unequivocal, the Uncertain and the Unthinkable. Int. J. Sci. Educ. 35, 1037–1063. doi:10.1080/09500693.2011.608197
Durall, E. (2020). Design-Principle Toolkit. Brussels: Ecsite. Available at: https://system2020.education/resources/design-principles-and-methods-toolkit-for-supporting-science-learning-outside-the-classroom/(Last accessed 02 28, 2021).doi:10.1190/segam2020-3415521.1
Falk, J. H., Staus, N., Dierking, L. D., Penuel, W., Wyld, J., and Bailey, D. (2016). Understanding Youth STEM Interest Pathways within a Single Community: The Synergies Project. Int. J. Sci. Educ. B 6, 369–384. doi:10.1080/21548455.2015.1093670
Farkas, L. (2017). European Commission. DG Justice and Consumers. Luxembourg: EU Publications OfficeAvailable at: https://data.europa.eu/doi/10.2838/447194.Analysis and Comparative Review of Equality Data Collection Practices in the European Union: Data Collection in the Field of Ethnicity
Field, A., Miles, J., and Field, Z. (2012). Discovering Statistics Using R. LondonThousand Oaks, Calif: Sage.
Frome, P. M., and Eccles, J. S. (1998). Parents' Influence on Children's Achievement-Related Perceptions. J. Personal. Soc. Psychol. 74, 435–452. doi:10.1037/0022-3514.74.2.435
Goldthorpe, J. (2007). “Cultural Capital”: Some Critical Observations. Sociologica 1/2, 1–23. doi:10.2383/24755
Gorard, S., and See, B. H. (2009). The Impact of Socio‐economic Status on Participation and Attainment in Science. Stud. Sci. Educ. 45, 93–129. doi:10.1080/03057260802681821
Grenfell, M. (2004). ““Bourdieu in the Classroom,” in,” in Culture and Learning: Access and Opportunity in the Classroom. International Perspectives on Curriculum. Editor M. Olssen (Charlotte, NC: Information Age Publishing)), 49–72.
Guttman, L. (1954). Some necessary conditions for common-factor analysis. Psychometrika 19, 149–161. doi:10.1007/BF02289162
Harnois, C. E. (2013). Feminist Measures in Survey Research. Los Angeles: SAGE Publications. doi:10.4135/9781452269955
Hausen, K. (1976). “„Die Polarisierung der ‚Geschlechtscharaktere‘. Eine Spiegelung der Dissoziation von Erwerbs- und Familienleben,” in Sozialgeschichte der Familie in der Neuzeit Europas. Editor W. Conze (Stuttgart: Klett-Cotta), 363–393.
Hayes, B. C., and Tariq, V. N. (2000). Gender Differences in Scientific Knowledge and Attitudes toward Science: A Comparative Study of Four Anglo-American Nations. Public Underst Sci. 9, 433–447. doi:10.1088/0963-6625/9/4/306
Hazari, Z., Sadler, P., and Sonnert, G. (2013). The Science Identity of College Students: Exploring the Intersection of Gender, Race, and Ethnicity. J. Coll. Sci. Teach. 42 (5), 82–91.
Hille, A., and Schupp, J. (2015). How Learning a Musical Instrument Affects the Development of Skills. Econ. Educ. Rev. 44, 56–82. doi:10.1016/j.econedurev.2014.10.007
Hoffmeyer-Zlotnik, J., and Warner, U. (2010). The Concept of Ethnicity and its Operationalisation in Cross-National Social Surveys. Metodoloski Zvezki 7/2, 107.
Hughes, B. E. (2018). Coming Out in STEM: Factors Affecting Retention of Sexual Minority STEM Students. Sci. Adv. 4, eaao6373. doi:10.1126/sciadv.aao6373
International Labour Organisation (2008). ISCO-08 Structure, index Correspondence with ISCO-88 ‘Part I - Introductory and Methodological Notes’. ILOAvailable at: https://www.ilo.org/public/english/bureau/stat/isco/isco08/index.htm.
Jacobs, J., Davis-Kean, P., Bleeker, M., Eccles, J., and Malanchuk, O. (2005). ““’I Can but I Don’t Want to’: The Impact of Parents, Interests and Activities on Gender Differences in Math,”,” in Gender Differences in Mathematics. An Integrative Psychological Approach. Editors A. Gallagher, and J. Kaufman (New York: Cambridge University Press), 246–263.
Johnston, A., Southerland, S. A., and Sowell, S. (2006). Dissatisfied with the Fruitfulness of “Learning Ecologies”. Sci. Ed. 90, 907–911. doi:10.1002/sce.20161
Kaiser, H. F. (1960). The Application of Electronic Computers to Factor Analysis. Educ. Psychol. Meas. 20, 141–151.
Jordan, W. J. (2010). Defining Equity: Multiple Perspectives to Analyzing the Performance of Diverse Learners. Rev. Res. Educ. 34, 142–178. doi:10.3102/0091732X09352898
Milgram, D. (2011). How to Recruit Women and Girls to the Science, Technology, Engineering, and Math (STEM) Classroom. Technol. Eng. Teach. 71/3, 4–11.
Miller, K., Willson, S., Chepp, V., and Padilla, J. L. (2014). Cognitive Interviewing Methodology. Hoboken, NJ: Wiley.
Miller, L., and Budd, J. (1999). The Development of Occupational Sex‐role Stereotypes, Occupational Preferences and Academic Subject Preferences in Children at Ages 8, 12 and 16. Educ. Psychol. 19, 17–35. doi:10.1080/0144341990190102
Papanastasiou, C., and Papanastasiou, E. C. (2010). Major Influences on Attitudes toward Science. Educ. Res. Eval. 10, 239–257. doi:10.1076/edre.10.3.239.30267
Rouhani, S. (2014). Intersectionality-Informed Quantitative Research: A Primer. Am. J. Public Health 103/6, 1082–1089.
Seebacher, L. M. (2016). A Feminist Standpoint Theoretical Perspective on Quantitative Methods & Questionnaire Construction. Vienna: Vienna University of Economics and Business ([master thesis]. Counting People, Not Objects
Stangl, W. (2021). Jugendliche im Spannungsfeld zwischen Eltern und Peers. werner stangl‘s arbeitsblätter.Available at: https://arbeitsblaetter.stangl-taller.at/JUGENDALTER/Spannungsfeld-Eltern-Peers.shtml (Last accessed 02 28, 2021).
Steinke, J. (2017). Adolescent Girls' STEM Identity Formation and Media Images of STEM Professionals: Considering the Influence of Contextual Cues. Front. Psychol. 8, 716. doi:10.3389/fpsyg.2017.00716
Tenenbaum, H. R., and Leaper, C. (2003). Parent-child Conversations about Science: The Socialization of Gender Inequities? Develop. Psychol. 39, 34–47. doi:10.1037/0012-1649.39.1.34
Traxler, A. L., Ximena, C., Cid, J. B., and Barthelem, R. (2016). Enriching Gender in Physics Education Research: A Binary Past and a Complex Future. Phys. Rev. Phys. Educ. Res. 12/2, 020114.
Voigt, C., Seebacher, L. M., and Vana, I. (2020). Overcoming Inequalities in Informal Science Learning; ICLS 2020: The 14th International Conference of the Learning Sciences; June 19-23, 2020; Nashville. Tennessee.
Warner, L. R. (2008). A Best Practices Guide to Intersectional Approaches in Psychological Research. Sex Roles 59 (5-6), 454–463. doi:10.1007/s11199-008-9504-5
Winner, E., and Cooper, M. (2000). Mute Those Claims: No Evidence (Yet) for a Causal Link between Arts Study and Academic Achievement. J. Aesthet. Educ. 34 (3/4), 11. doi:10.2307/3333637
Keywords: science learning, intersectionality, learning ecology, structural inequalities, gender, educational capital, science attitudes
Citation: Seebacher LM, Vana I, Voigt C and Tschank J (2021) Is Science for Everyone? Exploring Intersectional Inequalities in Connecting With Science. Front. Educ. 6:673850. doi: 10.3389/feduc.2021.673850
Received: 28 February 2021; Accepted: 30 June 2021;
Published: 15 July 2021.
Edited by:
Chantal Lise Barriault, Laurentian University, CanadaReviewed by:
Spela Godec, University College London, United KingdomCopyright © 2021 Seebacher, Vana, Voigt and Tschank. This is an open-access article distributed under the terms of the Creative Commons Attribution License (CC BY). The use, distribution or reproduction in other forums is permitted, provided the original author(s) and the copyright owner(s) are credited and that the original publication in this journal is cited, in accordance with accepted academic practice. No use, distribution or reproduction is permitted which does not comply with these terms.
*Correspondence: Lisa M. Seebacher, c2VlYmFjaGVyQHpzaS5hdA==
†These authors have contributed equally to this work and share first authorship
Disclaimer: All claims expressed in this article are solely those of the authors and do not necessarily represent those of their affiliated organizations, or those of the publisher, the editors and the reviewers. Any product that may be evaluated in this article or claim that may be made by its manufacturer is not guaranteed or endorsed by the publisher.
Research integrity at Frontiers
Learn more about the work of our research integrity team to safeguard the quality of each article we publish.