- 1DIPF | Leibniz Institute for Research and Information in Education, Frankfurt am Main, Germany
- 2University of Wuppertal, Wuppertal, Germany
- 3Centre for International Student Assessment (ZIB), Frankfurt am Main, Germany
- 4Goethe University Frankfurt am Main, Frankfurt, Germany
- 5Centre for Educational Measurement (CEMO) at the University of Oslo, Oslo, Norway
This paper addresses the development of performance-based assessment items for ICT skills, skills in dealing with information and communication technologies, a construct which is rather broadly and only operationally defined. Item development followed a construct-driven approach to ensure that test scores could be interpreted as intended. Specifically, ICT-specific knowledge as well as problem-solving and the comprehension of text and graphics were defined as components of ICT skills and cognitive ICT tasks (i.e., accessing, managing, integrating, evaluating, creating). In order to capture the construct in a valid way, design principles for constructing the simulation environment and response format were formulated. To empirically evaluate the very heterogeneous items and detect malfunctioning items, item difficulties were analyzed and behavior-related indicators with item-specific thresholds were developed and applied. The 69 item’s difficulty scores from the Rasch model fell within a comparable range for each cognitive task. Process indicators addressing time use and test-taker interactions were used to analyze whether most test-takers executed the intended processes, exhibited disengagement, or got lost among the items. Most items were capable of eliciting the intended behavior; for the few exceptions, conclusions for item revisions were drawn. The results affirm the utility of the proposed framework for developing and implementing performance-based items to assess ICT skills.
Introduction
Information and communication technology (ICT) skills are considered a key competence for lifelong learning (European Communities, 2007), successful participation in the labor market (van Deursen and van Dijk, 2011), and participation in political and societal debates. ICT literacy has thus been termed a “survival skill” (Eshet-Alkalai, 2004) with significance across the lifespan (Poynton, 2005). Consequently, a lack of such skills leads to disadvantages in various life contexts. Knowledge tests or self-report questionnaires can be used to measure ICT skills (e.g., Richter et al., 2010; Goldhammer et al., 2016). However, emphasis should be placed on hands-on ICT skills–what individuals are actually capable of (International Computer and Information Literacy Study (ICILS); Jung & Carstens, 2015).
ICT skills refer to the capability to successfully solve tasks requiring the use of ICT. ICT skills therefore comprise skills and knowledge that refer to operating technology. ICT skills are frequently described as relying on capabilities not specific to the ICT domain, such as reading (e.g., International ICT Literacy Panel, 2002; Calvani et al., 2009; Fraillon and Ainley, 2010). In assessments such as ICILS, ICT tasks are presented as cognitive tasks that require “accessing” or “managing” information, for instance–but how exactly the ICT tasks relate to these conventional skills is not often considered. This of course makes it impossible for item development to be informed by theories addressing conventional skills, like reading, and to predict item difficulties by means of theoretically derived item properties (cf. Embretson, 1983). In light of these considerations, the first of this paper’s three goals is to identify what conventional skills are involved in ICT skills and to systematically apply established psychological theories regarding those skills to the ICT context. This makes it possible to identify task characteristics that can determine the item difficulties of information tasks.
Most frameworks describe how they organize the domain of interest–for instance, in terms of different cognitive tasks (International ICT Literacy Panel, 2002; Eshet-Alkalai, 2004; Calvani at al., 2009; Fraillon and Ainley, 2010)–but do not describe how the overarching framework itself is ultimately translated into items and a suitable assessment format. ICT skills are best assessed in a performance-based manner using computers, which can be expected to generate the best construct representation (Sireci and Zenisky, 2006). Highly authentic items involving software used in everyday life cannot be easily integrated into larger assessments (Parshall et al., 2002). Simulation environments, in contrast, can be resource-intensive to develop, and designers must make difficult decisions about which aspects to include in the simulation and which to omit (Mislevy, 2013, p.108). Such decisions are important in order to avoid construct underrepresentation on the one hand and adding irrelevant variance to the measured construct on the other (Messick, 1994). Balancing these aspects is crucial for the development of appropriate design principles for the simulated environment and procedures for scoring response behavior. Thus, the second goal of this study is to address various design questions in order to propose how a simulated environment suitable for measuring ICT skills in a performance-based way should look.
While the first two goals focus on conceptual issues, the third goal concerns validation. Whether these conceptual issues have been adequately addressed were evaluated by analyzing item difficulties and aspects of test-taker’s behavior. Behavioral indicators were identified and thresholds for malfunctioning defined that allow for drawing direct conclusions about the reason for malfunctioning and provide direction for item revision.
Intended Test Score Interpretation
According to Kane (2013), validation starts with an interpretation and use argument. Therefore, we seek to first describe the intended interpretation of test scores before developing the assessment framework. This assessment framework should focus not on specialized professional tasks, but on tasks that average users engaging with ICTs could potentially come across. However, even such everyday ICT tasks have a wide range of complexity. A simple task might be to open or send an email, while more complex tasks might also ask test-takers to decide what to do with an email (e.g., forward it or not). A simple task requires rather basic skills, such as knowledge about the technical environment. A more complex task would additionally require evaluating the content of the email, a higher-level skill, and might also require the application of ICT-specific knowledge such as knowledge about characteristics of spam. Thus, while a simple task encompasses one aspect of an everyday activity, a more complex task more comprehensively captures a typical everyday activity. This study focuses on such complex tasks, which require test-takers to make ICT-specific decisions in an ICT environment. Consequently, the mere command of skills for operating technology does not suffice to solve such ICT tasks successfully; higher-level skills are required. Consequently, in the present study, we intend for test scores to be interpreted as representing higher-order ICT skills.
In addition to “ICT skills”, other terms such as digital competence (e.g., Calvani et al., 2009) or ICT literacy (e.g., International ICT Literacy Panel, 2002) are also used. In a review, Siddiq et al. (2016) describe similarities between these concepts, such as a joint focus on retrieving and processing or producing information. Therefore, the various concepts refer to comparable situations and problems users face when operating ICTs. Ferrari et al. (2012) identify overlaps in conceptualizations of what they call “digital competence” based on policy documents and academic papers. For instance, many conceptualizations addressed information management and problem-solving. The term used often also defines the framework’s overarching goal: Thus, van Deursen and van Dijk (2009, 2014) use the term “Internet skills” and focus on skill-related problems in using the Internet. Eshet-Alkalai (2004) asks what so-called “survival skills” are necessary to accomplish Internet-based tasks, identifying highly specific skills such as “branching literacy”. In the present paper, we present a framework with the goal of developing a suitable assessment of skills necessary for accomplishing everyday ICT tasks, which we refer to as “ICT skills”. The specific skills and situations targeted are described in greater detail in First Goal: Developing an Assessment Framework.
First Goal: Developing an Assessment Framework
The construction of a performance-based test can follow either a task- or a construct-centered approach. While a task-centered approach focuses on the targeted actions to be performed, the construct-driven approach asks which skills and knowledge are required to perform these actions, with the nature of the construct guiding item development (Messick, 1994). The development of digital skills assessments can also follow tool-oriented approaches, which involve the application of specific software to initially structure the domain (cf. Ferrari et al., 2012). As this study focuses not on all tasks that may occur in ICT environments, but on tasks that require higher-order ICT skills, a construct-driven approach is needed to ensure that test scores can be interpreted as intended. Furthermore, the ICT skills we seek to measure are assumed to be independent of specific software. Relevant skills and their interplay with knowledge are defined in the following sections.
Components of ICT Skills
To solve tasks that require higher-order ICT skills, it is not enough to apply only ICT-specific knowledge. Additional skills are required to solve information problems in ICT environments. In the relevant literature, these skills are defined as “key competencies”, including reading, problem-solving, numeracy, logical, inferential, and metacognitive skills (Calvani et al., 2009, p.186); as “cognitive skills”, including reading, numeracy, critical thinking, and problem-solving (International ICT Literacy Panel, 2002, p.1); or as “conventional literacies” (Fraillon and Ainley, 2010, p.8). While the need for numerical skills, for instance, depends strongly on task content, we assume that problem-solving and comprehension skills are important for all kinds of tasks. This is because, first, information from the environment must be encoded, and second, the information problem must be solved by interacting with the environment.
Please note that this does not preclude the notion that other conventional skills might be important in specific ICT tasks. Instead, focusing on only a few skills that are assumed to play a role in almost all ICT tasks makes it possible to apply established psychological theories from these research areas to the context of ICT. This allows us to take a construct-driven approach and base item development on theoretically derived item properties. Such a procedure is important in order to ensure that test scores reflect the intended construct. Drawing on established theories from the domains of comprehension and problem-solving skills also emphasizes the fact that ICT skills should not be considered completely new skills. Rather, they involve skills that were important even before technological environments came into widespread use.
Comprehension of Text and Graphics
Nearly all ICT tasks require the processing of symbolically represented information to some degree. Even tasks that do not involve higher-order reading processes (such as installing a software program on a computer) require decoding, syntactic parsing, and the semantic integration of words (“Do you want to proceed?”). When using the Internet, text comprehension comes into play, as detailed in models such as the construction-integration (CI) model by Kintsch (1998). This model describes the text comprehension process as a cyclical interplay between bottom-up and top-down processes. Beginning with the physical representation of the text, processes (such as letter and word recognition, semantic parsing, and local coherence processes) are employed to build a propositional representation of the text content (textbase model). This model is integrated with prior knowledge in a top-down fashion, resulting in a situation model. As informational content can also be in the form of pictures or sounds, one can distinguish between the processing of visual-verbal (e.g., written language), visual-pictorial (e.g., iconic material), auditory-verbal (e.g., spoken language), and auditory-pictorial (e.g., sounds) information (cf. Integrated Model of Text and Picture Comprehension; Schnotz, 2005). The importance of processing pictorial information is further supported by Eshet-Alkalai (2004), who proposed photo-visual literacy as one aspect of digital literacy. In ICT environments, such comprehension processes are important for such tasks as identifying menu items or folders, discovering editing functions, or checking search result pages.
Problem-Solving
In ICT environments, problem-solving is required when performing tasks such as search queries or working with unfamiliar systems. According to Simon and Newell (1971), problem-solving takes place in a problem space, with nodes for different states of knowledge and the use of operators required to reach the next node. Problem-solving comprises the construction of the problem space as well as the solution. Within the problem-solving process, a problem-solver decides which node to choose as a point for further investigation and which operator might be best to achieve a desired goal. For example, such models can describe how users decide whether to go back and enter a new search term or to navigate to a web page listed in the search results. Brand-Gruwel et al. (2009) propose a model for solving such information problems using the internet (known as the IPS-I model). In this model, the solution process is divided into different steps, such as defining the information problem, organizing or ultimately presenting the relevant information.
Interplay with ICT-specific Knowledge
Kintsch (1998) proposed that different cognitive processes take place depending the amount of available knowledge. According to Funke and Frensch (2007), domain-specific knowledge is also highly important when solving problems. Hence, knowledge is assumed to guide both comprehension and problem-solving processes. It is further assumed that ICT skills are rooted in the three aforementioned skills: comprehension of text and graphics, problem-solving, and ICT-specific knowledge. Tasks that require conventional skills but no ICT-specific knowledge are not of interest for this study, as the resulting test scores would not capture ICT-specific skills. Tasks that require only ICT-specific knowledge and no conventional skills are also not the focus of this study. Such tasks are rather routine and do not require higher-order ICT skills. Therefore, in order for test scores to be interpreted as intended, task success must depend on ICT-specific knowledge (e.g., knowledge about characteristics of spam). Moreover, the item difficulty should be determined based on the difficulty level of the ICT-specific knowledge and the difficulty of integrating this knowledge into the task solution. This can be a starting point for developing items that can be interpreted as intended and have a certain level of difficulty.
Task Characteristics for the Development of ICT Skills Items
In addition to the skills and knowledge involved, the coverage of the content domain and generalizability also matter for assessment development (Messick, 1994). The broadness of the ICT skills construct necessitates an organization scheme to ensure that test scores include all important aspects of the construct. Various frameworks have been suggested to organize the broad domain of ICT skills, all of which have different aims and purposes (for an overview, see Ferrari et al., 2012; Siddiq et al., 2016). One very influential framework was proposed by the International ICT Literacy Panel (2002), which defines ICT literacy as being in command of a set of “critical components” (p. 3) for solving information tasks, which are identified as “access(ing)”, “manage(ing)”, “integrate(ing)”, “evaluate(ing)”, and “create(ing)” information. The ICT Literacy Panel’s framework has inspired other developments in recent years (cf. National Higher Education ICT Initiative, 2003; ICILS, Fraillon and Ainley, 2010) and overlaps with other conceptualizations of ICT skills (Eshet-Alkalai, 2004; Calvani et al., 2009). In light of the critical importance of this framework, we chose to take the information tasks defined in this model as the basis for our item development. As these are general information tasks unrelated to ICT-specific knowledge, current ICT topics (e.g., “fake news”) can be taken as item content and knowledge that must be integrated in order to solve the items. In addition, we seek to describe which ICT-specific demands the five cognitive ICT tasks of accessing, managing, integrating, evaluating, and creating impose on the user. Items representing these five tasks would be considered to adequately capture the target domain (cf. Kane, 2013, p.24).
In previous conceptualizations, skills and tasks were defined in a rather operational way. It was not defined what, for instance, constitutes an easy or hard task in terms of accessing and what makes it harder or easier in terms of ICT skills. However, addressing such questions is important to make sure that item difficulties vary sufficiently and solely due to construct-related task characteristics. Only then do the items capture individual differences in the target construct of ICT skills. In the following sections, task characteristics are derived based on the previously described skills that will be used to guide item development in order to systematically manipulate item difficulty. Table 1 summarizes the example items described below, the ICT-specific knowledge required, and the estimated difficulty.
Accessing
Accessing describes “knowing about and knowing how to collect and/or retrieve information” (International ICT Literacy Panel, 2002, p. 17). ICT-specific demands often involve navigating ICT environments in a variety of ways, which creates a risk that users may feel disorientated on the Internet (van Deursen, 2010; van Deursen and van Dijk, 2014). Unfamiliar navigational structures impede navigation (Chen et al., 2011). The breadth, depth and topology of the hypertext structure also matters (DeStefano and LeFevre, 2007). A prototypical item to measure individual skill in accessing information might be a task requiring the use of a search engine for a library database to find a reasonable selection of books on a certain topic (cf. Table 1). In such an example, task structures encouraging the use of more specific search queries, such as utilizing various filtering options or more than one search field might facilitate a more effective search process. If such structures are not available in the ICT environment at hand, the problem-solving process is less well-defined. As a result, less proficient users might perform an insufficiently structured search query. In such a case, prior knowledge about search engines and experience in specifying search queries have to be applied to solve the task. Consequently, accessing tasks should be harder if such prior knowledge is important for the task solution. Such a search engine task only requires ICT skills if conventional skills have to be used together with ICT-specific knowledge in order to solve the task. If a task requires only comprehension skills and/or problem-solving skills but not ICT-specific knowledge, it does not capture ICT skills. For instance, an information search task carried out at a library up until the mid-1990s would have meant accessing a card catalog, printed volumes of abstracts indexing journal articles, or a Microfiche catalog. Succeeding in such a task would have almost certainly depended on comprehension and problem solving skills, but not ICT-specific knowledge.
Managing
Managing refers to “applying an existing organizational or classification scheme” (International ICT Literacy Panel, 2002, p. 17). ICT-specific demands in managing information involve handling complex systems in order to accomplish an information management task. If the software is unfamiliar, users need to adapt their previous knowledge to the task (Calvani et al., 2009). The ease of transferring knowledge to an unfamiliar user interface depends on the similarity of structures, surfaces and contexts (Day and Goldstone, 2012), or whether general concepts exist (Singley and Anderson, 1985). A prototypical item that can be used to measure individual skill in managing information might require moving e-mails into a folder structure and/or renaming a folder (cf. Table 1). A basic understanding of folder structures in email inboxes and the possibility to move emails out of the inbox are required. In harder items, it could be necessary to create a new folder first before it can be named correctly, or the functions needed to complete these tasks might be not visible on the home screen. In such a case, knowledge and experience with such programs would support the solution process. The difficulty level of the knowledge necessary for task solution (e.g., about formats for saving documents or printing options) should drive item difficulty in managing tasks.
Integrating
Integrating requires “interpreting and representing information. It involves summarizing, comparing and contrasting” (International ICT Literacy Panel, 2002, p. 17). Metzger (2007) describes ICT-specific demands related to the enormous amount of accessible information, which requires users to integrate information obtained from different sources. The ease with which a user is able to create coherence (cf. Kintsch, 1998) with respect to information from different sources depends on the number of information units (like websites, documents, or e-mails), the degree to which information is comparable, and the degree of inconsistency between documents (Perfetti et al., 1999). The integration process is more complex if the sources differ in terms of breadth (Bhavnani et al., 2003) or contain conflicting information (Hämeen-Anttila et al., 2014). A prototypical item would be to select a specific language course by comparing the websites of different courses with respect to several criteria, such price, dates, and reviews by former participants (cf. Table 1). In easy integration tasks, the information units to be compared can easily be identified. In harder tasks, more knowledge must be applied to guide the integration process, such as knowledge about where information is located on websites.
Evaluating
Evaluating information involves “making judgments about the quality, relevance, usefulness, or efficiency of information” (International ICT Literacy Panel, 2002, p. 17). To deal with the growing amount of information on the Internet (Edmunds and Morris, 2000), users have to evaluate the value of incoming information (Whittaker and Sidner, 1996). ICT-specific demands in this area include evaluating the trustworthiness of information (Lorenzen, 2001), as material published on the Internet is not necessarily subject to peer-review processes or editorial control. A model of task-based relevance assessment and content extraction (TRACE model; Rouet, 2006) has been proposed to describe how the information provided is combined with prior knowledge and evaluated. The evaluation of information might depend on the ease of identifying relevant criteria, such as the truth, guidance, accessibility, scarcity, and weight of information (Simpson and Prusak, 1995), but also structural (e.g., domain names) and message-related (e.g., objectivity) features (Hahnel et al., 2016), or quality cues such as title and authority (Rieh, 2002). A prototypical item to measure individual skill in evaluating information would be to judge the relevance a set of e-mails in one’s personal inbox for a third party (cf. Table 1). In addition to the e-mails’ content, test-takers may also need to incorporate knowledge about characteristics of spam and available information about the senders of the e-mails. Task characteristics that support the identification of certain information as irrelevant, such as by helping to identify spam emails, make such items easier.
Creating
Creating describes “generating information by adapting, applying, designing, inventing, or authoring information” (International ICT Literacy Panel, 2002, p. 17). Choosing from among countless editing options to present information in a suitable way places unique demands on ICT users. Software for designing or painting has substantially expanded the possibilities to create and transform knowledge into graphical material compared to the pre-computer era. Nevertheless, using this kind of software might require particular cognitive skills (Horz et al., 2009; Cox et al., 2010). Bulletin boards, blogs and e-mails necessitate a different writing style (McVey, 2008) compared to traditional off-line writing, and users are no longer solely recipients but also producers of user-generated content (van Dijck, 2009). A prototypical item that can be used to measure individual skill in creating information would be to adapt the text of an e-mail to appropriately address a specific recipient (cf. Table 1). Creating tasks might be more difficult when the task instructions are ill-defined (see the cognitive process theory of writing; Flower and Hayes, 1981). When completing harder items, test-takers not only need to adapt to the setting based on their knowledge about norms, for example, but must also independently identify the need for adaptation in the first place.
Cognitive Tasks in Item Development
Tasks encountered in everyday life might not clearly fall within any one of these cognitive tasks. For example, when dealing with items in a real-life email inbox, it is likely that multiple emails will concern the same topic, meaning that integration processes are required. Moreover, one of those emails might also require evaluation skills. Consequently, the task of dealing with a real-life inbox would require both integration and evaluation processes. Similar examples can be found for different combinations of cognitive tasks. Even though such combined tasks are typical in everyday life, assessment items should focus on a single cognitive task, avoiding a mixture of cognitive tasks within any one item. Only then will an item’s difficulty stem from a single cognitive task, such as integrating or evaluating information. Such a procedure appears to have advantages for the specificity of items, which is necessary to ensure that test scores appropriately reflect all five cognitive tasks.
Second Goal: Item Implementation
Issues in Performance Assessment
Messick (1994) identified various issues in performance assessment. These issues focus on the central question of how to develop an assessment that maximizes construct representations and minimizes the irrelevant variance over and above the construct-relevant variance. These two problems are considered important because they can threaten construct validity. The crucial task of ensuring that test scores accurately measure the targeted skills guides not only item writing but also development. The following section begins by addressing emerging development issues and then makes decisions about them with an eye to ensuring that test scores can be interpreted as intended.
The first issue refers to the question of whether authentic or simulation-based environments should be used. ICT skills can be measured directly if the assessment uses the same technology that would be used in the situation making up the assessment target. In this case, the test situation would elicit the cognitive processes underlying the specific ICT skills intended to be assessed. However, according to Mislevy, a “higher fidelity of real-world situation does not necessary make for better assessment” (Mislevy, 2013 p.108). In the context of ICT skills assessment, reasons for favoring simulation environments rather than tasks in fully authentic settings can include the variety of existing operating systems, versions, and programs. Individual’s response behavior and associated test scores can only be compared if the test-takers are equally unfamiliar or familiar with an operating system or a certain software program. This requires either having each test-taker use the software he or she is most familiar with or utilizing simulation environments that abstract away from the systems test-takers use in everyday life. Simulation environments create a highly controlled testing situation that allows for measuring and comparing not only response outcomes but also interaction behavior. This is not possible in test formats in which different test-takers complete the tasks in different settings or test-takers have different levels of familiarity with the system. Furthermore, simulation environments can likely be used for a longer period of time, while real-life operating systems become outdated as soon as a newer version is released, or meaning that tasks involving these newer versions are no longer comparable to previous assessments. Moreover, we argue that adapting to new systems (such as the simulation environment) is an integral part of ICT skills. Hence, using a simulation environment should not add irrelevant variance to the construct and not conflict with the construct interpretation.
However, how to construct a simulation environment–which is always limited to certain extent–in order to evoke authentic processes that arise in ICT environments should be carefully thought out. Test developers need to decide which aspects of the target task should be modeled in the simulation environment and which can be omitted (Mislevy, 2013). First, the item’s level of task complexity should be defined. Messick (1994) describes task complexity along a continuum from structured to open-ended tasks. Structured tasks are efficient, while open-ended tasks might have higher domain coverage. However, open-ended and other highly complex tasks tend to be administered as standalone measures rather than as part of larger assessments (cf. Parshall et al., 2002, p.10). Note that this continuum from structured to open-ended assessment can refer both to the presented task and to the response mode (cf. Messick, 1994). We first consider the presented stimulus. A highly structured task guides cognitive processes in the intended direction, resulting in either a correct or an incorrect solution. In contrast, an open-ended task allows for a variety of reactions, including behavior that is largely unrelated to the targeted cognitive processes and therefore not of interest to the test developers. In problem-solving theory, the complexity of a task is described not in terms of structured vs. open-ended tasks but in terms of well-vs. ill-defined problems (Simon and Newell, 1971; OECD, 2012). When solving ill-defined problems, test-takers must first construct the problem space before they can engage in problem-solving activities, with both steps understood as part of problem-solving. Such open-ended tasks or ill-defined problems bear the risk that some test-takers might get lost, especially if they are not sure of how to solve the task. This could particularly affect test-takers who solve the item incorrectly. Highly structured tasks might be easier to solve than open-ended tasks because they provide guidance to test-takers in constructing a problem space.
Moreover, irrespective of the complexity of the stimulus, response modes can vary in the degree to which they are constrained (Messick, 1994), and can range from highly selective to constructive (Scalise and Gifford, 2006). Selective response formats within ICT environments can still be highly authentic–think of selecting the format in which a document should be saved from a drop-down menu. Constructive formats, on the other hand, might have test-takers enter text into an input field, edit a text, or produce a diagram. While it is quite easy to log test-taker’s behavior in simulated tasks, making sense of and interpreting such behavior can be quite challenging. Consequently, interpreting test-taker’s behavior in constructive tasks should be considered from an early stage (cf. Mislevy et al., 2002). Focusing on the intended interpretation during the item construction phase can provide clear directions on how to score test-taker’s behavior when completing the assessment (Mislevy et al., 2002).
The aforementioned points focus on how authentic cognitive processes can be evoked during test-taker’s interaction with a simulation environment. Simulation environments are always limited to certain extent, and test-takers becoming aware of these limitations might disrupt the emergence of authentic cognitive processes to a certain extent.
Design Principles
In order to evoke authentic processes, the implementation of ICT skills items should therefore seek to 1) give test-takers the impression that they are working within a complete environment for as long as possible, and 2) guide test-takers to the critical aspects of the task. Implementation issues can arise concerning the construction of the stimulus and the response format. While highly structured tasks provide more guidance to test-takers, less structured tasks may be necessary to represent certain construct-relevant aspects. Remaining oriented (cf. van Deursen, 2010; van Deursen and van Dijk, 2014) is an ICT-specific demand related to accessing information and conducting an unstructured search in a browser and might therefore represent an important construct-related aspect. In such a case, clear task instructions should ensure that the intended cognitive processes are indeed evoked despite the relative lack of guidance within the task.
A further problem with less structured tasks is that test-takers might spend far more time on the item than intended, especially if they are unable to find the correct solution. Time limits on the item level could help out here. However, this could have the disadvantage of disrupting test-takers from the simulation by providing feedback on their performance. Therefore, we instead seek to avoid breaking away from the simulation environment for as long as possible in order to avoid breaking the simulation effect for the test-taker. This includes having the test-taker proceed autonomously from one item to the next. Nevertheless, clear task instructions and as much guidance as possible should prevent test-takers from losing their way. In addition, as long as test-taker’s behavior remains closely linked to the task, they should not encounter restrictions in the task environment. On the other hand, aspects that are completely unrelated to the task solution can be omitted.
To fully represent the ICT skills construct, a variety of different cognitive tasks (e.g., access and evaluation tasks) involving different tools (e.g., spreadsheet and email software) are needed. However, covering such a broad spectrum of performance tasks can lead to a very heterogeneous item set. This can be very demanding for the test-taker, because the instructions have to be read carefully and the functioning of different tools in an unknown simulation environment have to be understood. If test-takers give up at early stages of item processing because they are disengaged or discouraged, this would conflict with the intended test score interpretation. Restricting items to only construct-related aspects should prevent items from becoming too complex and thus discouraging test-takers. In turn, this should prevent irrelevant variance from being added to the construct.
In addition, the response format should not disrupt the simulation effect, but should rather evoke the same cognitive processes as in realistic settings. For example, responses should be given within the simulated environment rather than on a separate answer sheet (e.g., in the form of multiple-choice questions on a separate platform). Test-taker’s behavior within an item can be captured with constructive (typing) but also selective (clicking a button or using a drop-down menu) response formats. Hence, forwarding an e-mail after evaluating its relevance for another person should be preferred to simply checking the e-mails that should be forwarded in a multiple-choice format. Such authentic response formats should not add irrelevant variance to the construct. In order to maintain the simulation effect, options for both correct and incorrect response behavior should be included in the simulated environment, while aspects irrelevant to the correct or incorrect item solutions can be omitted. Clearly distinguishing between different cognitive tasks and clearly focusing on ICT-specific knowledge and skills in the item development phase can help test developments clearly specific what the target behavior is and how it should be scored. Table 1 contains information on the response formats chosen for the prototypical items.
Third Goal: Application and Evaluation of the Framework
After specifying a framework for how items measuring ICT skills should be developed and implemented, we then applied the framework to develop and implement an ICT skills performance test for 15-year-old students.
Empirical Research Questions
The first and second goals of this paper concerned developing items based on a theory-based assessment framework and providing first evidence that the resulting test scores can be interpreted as intended (i.e., construct interpretation). The third goal is to also provide empirical evidence that the test scores validly measure the intended construct.
Item Difficulties
All five cognitive ICT tasks are considered important for fully capturing the broad construct of ICT skills. Consequently, each cognitive ICT task should be addressed with items representing the full difficulty range (i.e., easy, medium, and hard). For instance, if the items for a particular cognitive task all exhibited high difficulty, the assessment framework would not be helpful for manipulating item difficulty systematically. Thus, our first empirical research question examines whether the items for the five cognitive ICT tasks are comparable and equally distributed across the difficulty range. This research question tests validity evidence based on the internal structure (American Educational Research Association, American Psychological Association, and National Council on Measurement in Education [AERA, APA and NCME], 2014).
Research Question 1: Are the items for all five cognitive ICT tasks comparable and equally distributed across the item difficulty range?
Test-Taking Process
Messick (1994) differentiates between performance and product. As behavior constitutes the link between the cognitive processes executed during the item solution process and the item scores, our second research question asks: Do test-takers exhibit the expected response behavior? This research question examines validity evidence based on response processes (American Educational Research Association, American Psychological Association, and National Council on Measurement in Education [AERA, APA and NCME], 2014).
Response processes should be represented in the test scores (Embretson, 1983; Messick, 1994). If, for instance, some test-takers solve an item without taking into account all of the presented information, they did not execute the intended cognitive process, meaning that the derived scores would not be a valid measure of these cognitive processes. Cognitive processes become somewhat visible in test-taker’s interactions with items.
Research Question 2a: Does the number of test-takers’ interactions with items indicate that the intended cognitive processes were executed in all items?
Disengagement can also threaten the validity of test score interpretations. Disengagement is especially problematic in low-stakes assessments and can be identified by analysing response behavior (Goldhammer et al., 2017; Wise, 2017). If a considerable number of test-takers exhibit rapid guessing behavior, meaning that they gave up at an early stage of item processing, this could point to motivational issues among test-takers for certain items (Goldhammer et al., 2017; Wise, 2017). Scores from these items would then also represent motivational aspects, adding irrelevant variance to the construct (Messick, 1994).
Research Question 2b: Does time use within items indicate that many test-takers gave up too early on certain items?
Performance assessments with no time limits on the item level also bear the risk that some test-takers will spend too much time on certain items, especially if they cannot find the correct solution. If test-takers get lost within an item and simply run up the time clock while no longer interacting with it productively, test efficiency is threatened. In particular, test-takers might spend more time on less structured items–both those who solve the item correctly and those who solve it incorrectly. This does not threaten the validity of the test score interpretation, because exploring the environment can be seen as part of the ICT skills construct, but can be problematic in terms of test efficiency. Moreover, one goal of item construction was to ensure the test-takers do not get lost within items.
Research Question 2c: Does time use in items indicate that many test-takers spent too much time on certain items?
Method
Items
Seven item developers reciprocally reviewed item drafts to ensure that only items fitting within the framework and focusing on one of identified cognitive ICT tasks were included. Items were initially developed to capture one of the five cognitive tasks. They were then contextualized (cf. Messick, 1994) and embedded within either a personal, educational, or occupational situation. Moreover, some items reflected solely individual tasks, while others involved communicating with others, like sending an email. The item developers also specified the expected item difficulties and provided justifications for these (cf. Table 1). For item construction, the CBA ItemBuilder software was used (Rölke, 2012) to created simulated environments (e.g., a browser, e-mail inbox, word processing software, etc.) and implement the scoring rules. Cognitive interviews were conducted to ensure the user-friendliness of the testing environment and the clarity of all elements of the newly developed items (for a fully implemented item, see: Engelhardt et al., 2017; for further information about the study, see: Wenzel et al., 2016).
Sample and Data Collection
The sample consisted of N = 773 students from 34 schools in Germany. Volunteer schools equipped with suitable computers were selected from the two federal states of Baden-Württemberg and Rhineland-Palatinate. Students were 15.29°years old (SD = 0.66) on average and about half of them were male (male: 51%, female: 46%, not specified: 3%). Each test-taker received randomized subsets of items, as it would have taken too much time for test-takers to answer all 70 items. Eleven different item subsets were assembled based on a balanced incomplete booklet design (Frey et al., 2009). Test-takers worked on each item for an average of M = 108.24 s (SD = 42.00). The items were scored dichotomously (correct/incorrect) immediately after a response was given, and indicators of response behavior (i.e., number of interactions, time spent on task) were automatically extracted from the log data file. Omitted items (no test-taker interaction at all) were excluded from the analyses based on response behavior (test-taker interactions and time use).
Data Analyses
A one-dimensional Rasch model was fitted using the R package TAM (Kiefer et al., 2016; R Core Team, 2014), with the mean of the ability distribution set to 0 and the slope of the item characteristic curves set to 1 (in other words, the ability variance was freely estimated). One item was excluded due to an insufficient item fit (outfit: 2.18; cf. De Ayala, 2013); for all other items, the item fit was acceptable (range of infit: 0.87–1.11; range of outfit: 0.67–1.25). The expected a priori (EAP) reliability for the remaining 69 items was 0.70. To answer the first empirical research question, Levene’s test for homogeneity of variance was conducted for the items assessing the five cognitive tasks, with the item difficulties as the dependent variable and the cognitive tasks (access, manage, integrate, evaluate, create) as independent variables. Similarly, an ANOVA was calculated to examine whether the mean item difficulties differed between the five cognitive tasks.
To answer the second empirical research question, we examined two behavioral indicators to detect malfunctioning items, test-taker interactions and time use. To address Research Question 2a, the number of test-takers interactions among test-takers who solved the item correctly was examined, because these test-takers should have applied the intended cognitive processes. First, the smallest number of interactions that led to a correct solution was calculated for each item. This number was compared to an item-specific threshold, the theoretical minimum of interactions necessary to solve the item if executing the intended cognitive processes. If some test-takers required fewer interactions than the theoretical minimum, this indicates that some test-takers were able to solve the item correctly without performing the intended cognitive processes. Consequently, scores on this item could not be validly interpreted with respect to these intended cognitive processes.
Second, the amount of time test-takers who solved the item incorrectly engaged with each item was used as an indicator to detect malfunctioning items. The first quartile was used to identify item disengagement (Research Question 2b) in order to evaluate the behavior of a considerable number of test-takers. Their time use was compared to the fastest test-taker who solved the item correctly (cf. Goldhammer et al., 2017), which served as an item-specific threshold. If many test-takers spent far less time on this item and thus gave up at early stages, this could indicate disengagement, which threatens the validity of the test score interpretation.
To identify whether test-takers tended to get lost in certain items (Research Question 2c), the third quartile of time use was used to capture a considerable number of test-takers who spent a longer amount of time on the item. This indicator was compared to an item-specific threshold representing the slowest test-taker who solved the item correctly. In order to ensure that this measure was not affected by outliers who spent an inordinate amount of time on the item, outliers were excluded following the boxplot logic. Rather the examining the maximum time spent among test-takers who achieved a correct answer, the highest whisker was used as the threshold.
Results and Conclusions for Item Revision
Item Difficulties
The 69 items had an average difficulty of M = 0.38 (SD = 1.56) and were distributed across a wide difficulty range (Min = -2.84; Max = 4.27). Based on the results of the ANOVA (F (4, 64) = 1.12, p = 0.356) and the Levene test (F (4, 64) = 0.13, p = 0.971), the hypothesis of comparable item difficulties and homogeneous variances of the item parameter estimates across the five cognitive tasks could be confirmed (see also Figure 1).The results provide empirical support that the item development and construction process was successful with regard to the representativeness of all five cognitive tasks. Hence, test scores can be interpreted as representing ICT skills, defined as encompassing the five aforementioned cognitive ICT tasks. This represents support for the validity of the test score interpretation based on internal structure (American Educational Research Association, American Psychological Association, and National Council on Measurement in Education [AERA, APA and NCME], 2014).
Test-Taking Process
Figure 2 (cf. Research Question 2a) compares the theoretically assumed correct solution behavior (dashed line) and the empirically observed number of interactions (bar) for each of the 69 items. The dashed line describes the minimum number of test-takers’ interactions with items necessary for a correct item solution if all expected cognitive processes were executed. The interesting part of this figure concerns the cases in which the left ends of the bars (shortest correct item solution in the observed data) cross the dashed line.
In four items, test-takers achieved a correct solution with fewer interactions than expected. For two of these items (those with an expected minimum of 3 and 6, respectively), the log data file indicated that the test aborted just after the item was solved correctly. Thus, navigation to the next item was not included in the count of test-taker interactions for these test-takers, but was included in the item-specific threshold. This does not indicate a problem with item construction, and presumably occurred due to the global time limit, which stopped the test after 60 min. However, this was not the case for the other two items (those with an expected minimum of 8 and 11, respectively). These two items concerned the cognitive task of integrating information and were of medium difficulty (0.06 and 0.47). Both tasks asked test-takers to visit two different websites, compare the information available on each, and choose one. Thus, it was possible to solve this item with a lucky guess after having visited only one of the two websites. This happened in 9.45% and 25.56% of the correct solutions, respectively, indicating that these items should be revised for validity issues, because many test-takers did not perform the expected cognitive processes but were still able to the item correctly. Different options for revision are possible. 1) To encourage test-takers to apply the intended cognitive process (integration of information), test-takers could be more clearly instructed to first visit both websites. 2) The item scoring could be adjusted to include behavior as well: a correct response can only be coded if only if a test-taker selected the correct website after having visiting both. This second option should be implemented to ensure that scoring is valid. Please note that lucky guesses would still be possible in this case, but the inclusion of behavior in item scoring at least increases the chance of obtaining a valid test score. Whether the instructions should be adjusted (Option 1) depends on what the item score is intended to capture: only whether test-takers can compare websites or also whether test-takers recognize that they need to visit both websites in order to solve the task correctly. The latter could also be understood as part of the ICT skills construct, and its inclusion might increase the difficulty of an integrate item.
Figure 3A (Research Question 2b) compares the time spent on the item among test-takers who solved the item incorrectly (bars) compared to the fastest test-taker who solved the item correctly (bisecting, dashed line) for each of the 69 items. Items are sorted on the y-axis according to the fastest correct solution. The interesting part of this figure concerns cases in which the left ends of the bars (first quartile of time spent on item) intersect the dashed line. This indicates that a considerable number of test-takers (more than 25%) gave up on this item before the first person was able to achieve a correct solution.
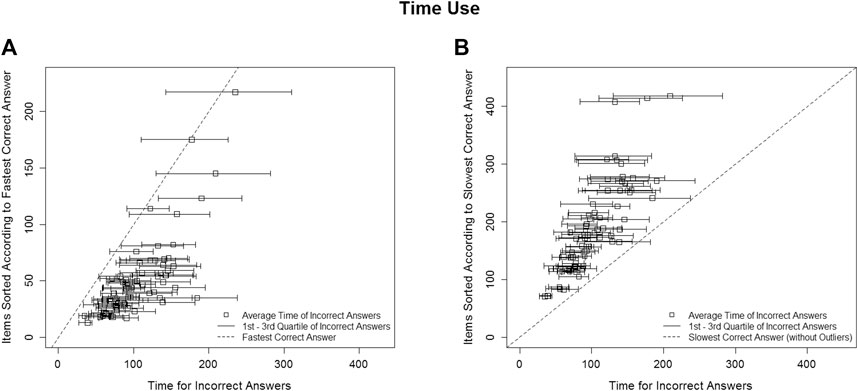
FIGURE 3. Empirical time use for incorrect item responses compared to fastest (A) and slowest (B) correct response.
According to Figure 3A, many test-takers gave up at an early stage for six items. These six items were all rather time-consuming (compare position on y-axis) in terms of the fastest correct item solution. Five of the six items were also rather hard (item difficulties greater than 2.37). Three of these six items concerned the cognitive task of integrating information, two items managing, and one item accessing. The test-taker’s reasons for giving up might have been 1) these item’s expected time intensity; 2) negative expectations of success due to the expected item difficulty, described by Goldhammer and colleagues (Goldhammer et al., 2017) as “informed disengagement” (p. 21); or 3) because these items might have seemed cognitively demanding for test-takers, which is likely at least for the integrate items, because dealing with information overload is an ICT-specific challenge in such items (Simpson and Prusak, 1995; Edmunds and Morris, 2000; Chen et al., 2011). Irrespective of the reason, such behavior calls into question the validity of the resulting test scores, because a higher test score would then also represent the decision to grapple with time-consuming, hard, or cognitively challenging items. Shortening or reducing the complexity of these six items could help to remove irrelevant variance (cf. Messick, 1994), which would also have the side effect of improving test efficiency due to the amount of time required to solve such items.
Figure 3B (Research Question 2c) compares the time spent on each item among those who solved it incorrectly (bar) compared to the slowest person who solved it correctly (bisecting, dashed line), not including outliers, for each of the 69 items. Items were sorted on the y-axis according to this slowest correct solution. The interesting part of this figure concerns cases in which the right ends of the bars intersect the dashed line, as this would indicate that many test-takers got lost when trying to solve the item. According to Figure 2, this was the case for one item, an access item. As ICT-specific challenges in accessing information include a feeling of disorientation on the Internet (van Deursen, 2010; van Deursen and van Dijk, 2014), this behavior does not threaten the validity of test score interpretation. Still, this item could be revised in order to increase efficiency.
General Discussion
Summary
The goal of this study was to propose a theoretical framework to inform theory-driven item development and implementation for the performance-based assessment of ICT skills. The described framework extends previous work by anchoring five cognitive ICT tasks, which had previously solely been defined operationally, into established theories. These five cognitive tasks were described in terms of what drives item difficulty and what important ICT-specific knowledge should be included in corresponding items. Proceeding from a definition of the construct intended to be measured, guiding principles for item implementation were derived and an empirical evaluation of the framework was conducted.
The proposed framework allowed us to evenly measure the targeted construct of ICT skills, as indicated by comparable ranges of item difficulties for all five cognitive ICT tasks (Research Question 1). Behavioral indicators were developed and extracted to evaluate the suitability of the framework and the guiding principles for item implementation. Based on the empirical analyses, most test-takers behaved as intended in most–but not all–items. These results allow conclusions to be drawn both in terms of concrete item revisions and with respect to assumptions for the item development process. Two items should be revised to ensure that the intended cognitive processes are executed (Research Question 2a). For these two items, scoring should consider not only the outcome of the task completion process (correct/incorrect response), but also behavior indicating whether important cognitive processes were actually performed. This conclusion can be applied more generally to performance assessment items to ensure the validity of the test score interpretation. Six items could be revised to ensure that motivational issues, like avoiding grappling with challenging or time-intensive items, are not represented in the test scores (Research Question 2b). This could be achieved by reducing needless item complexity. As a guiding principle, item revision should be rooted in a stronger focus on the ICT-specific difficulty. As a positive side effect, reducing complexity could also increase efficiency in terms of testing time. Getting lost (and thus wasting testing time on unproductive behavior) seemed to only be a problem for one item, even though no time limits were set on the item level (Research Question 2c). Based on the empirical results, giving up on items at early stages (Research Question 2b) seems to be a more serious problem than getting lost (Research Question 2c).
Indicators Derived from Test-Taking Behavior
Computer-based performance assessments are nowadays the rule rather than the exception, and allow test developers to focus not only on the outcome (e.g., scored response) but also solution behavior during the task solution process (Messick, 1994). When focusing on task performance, log data files or even already extracted indicators may be available. In this study, test-taker interactions and processing times were used. These indicators are often available in computer-based studies and can be calculated for any item independent of its content. For more homogeneous items like questionnaire data, the complete log data file might provide a more detailed picture of response processes. In such a case, for instance, the log data could be filtered based on theoretical process models (Kroehne and Goldhammer, 2018). However, the ICT skills items used in this study are very heterogeneous. ICT skills items encompass different cognitive tasks, such as accessing or evaluating information; are based on different applications, such as email software or websites; require different numbers and kinds of interactions (clicking or typing); and are differently time-consuming. This means that different log data files are produced for each ICT skills item. Hence, analyses can be only conducted for each item separately or by using general indicators, as was the case in this study.
In order to draw conclusions about malfunctioning items and necessary item revisions, item-specific indicators as well as item-specific thresholds for detecting malfunctioning items were calculated. These indicators and thresholds are generic and can be applied to any performance item for which test-taker interactions and processing times are available. Three approaches were used: (a) The empirical minimum number of test-taker interactions among test-takers obtaining a correct answer (indicator) was compared to an item-specific theoretical minimum of test-taker interactions necessary for a test-taker executing all intended cognitive processes (threshold). This approach makes it possible to identify items in which the intended cognitive processes were not executed. (b) The first quartile of time spent on a given item was calculated for persons who solved the item incorrectly (indicator) and compared to the fastest person who solved the item correctly (threshold). This approach (cf. Goldhammer et al., 2017) makes it possible to identify items in which a considerable number of test-takers gave up at a very early stage–potentially due to disengagement. (c) The third quartile of time spent on a given item was calculated for persons who solved the item incorrectly (indicator) and compared to the slowest person who solved the item correctly (threshold), not taking into account outliers. This approach makes it possible to identify items in which a considerable number of test-takers got lost.
In the present study, all three indicators pointed to items that should be revised. Interestingly, infit and outfit statistics did not identify these items, supporting the notion that heterogeneous, performance-based items might require more detailed, item-specific analyses. As an advantage compared to item fit statistics, the indicators used in this study also lead to conclusions on how to revise these problematic items in order to improve the validity of the test score interpretation. More effortful and item-specific in-depth analyses can now be conducted and could focus on whether sub-goals were reached or which kinds of mistakes test-takers made.
In this study, the two indicators and three approaches applied led to the identification of different items. In items in which cognitive processes are reflected by test-taker interactions, such as navigating through a simulated environment, interactions might be a good indicator of malfunctioning. Note, however, that interactions do not necessary indicate that test-takers reasoned about the material because interactions can be also performed rather quickly (cf. rapid guessing behavior) or can occur unintended, without representing a unique cognitive step, such as when a user inadvertently clicks next to a button rather than on it. In items in which cognitive processes are not at all linked to navigational steps, but rather involve the mental processing of information, test-taker interactions might be less meaningful. In such cases, processing times might be more informative, although they do not necessarily mean that the test-taker reasoned about the item for this full amount of time. These indicators are of course non-exhaustive and do not guarantee that items function well. They also do not replace classical analyses like item fits or differential item functioning, but rather complement them. We assume that such analyses are very useful to get a first impression of whether performance items have been successfully constructed or whether and how to revise those items.
Further Evidence for the Validity of Test Score Interpretations
This study provided evidence for the validity of the targeted test score interpretation based on the internal structure by analyzing and comparing the distributions of item difficulties for all five cognitive tasks, and based on response processes by analyzing response behavior in terms of interactions and testing time (American Educational Research Association, American Psychological Association, and National Council on Measurement in Education [AERA, APA and NCME], 2014). However, these empirical analyses cannot be regarded as sufficient evidence for the validity of the test score interpretation.
The assessment framework suggests further test of the validity of the test score interpretations that should be conducted. First, the fact that the definition of ICT skills refers to other constructs suggests analyzing convergent sources of validity evidence, namely the relations with ICT-specific variables as well as problem-solving and reading comprehension (validity evidence based on relation to other variables; American Educational Research Association, American Psychological Association, and National Council on Measurement in Education [AERA, APA and NCME], 2014). In an empirical study (Engelhardt et al., 2020), positive relations between ICT skills items and reading and problem-solving skills as well as technical knowledge were reported. As expected, the effect of technical knowledge tended to be stronger for harder items, but not the effect of the conventional skills of reading and problem-solving skills, supporting the assumption that ICT-specific knowledge rather than reading or problem-solving skills requirements should drive item difficulty. Furthermore, the assumed role of problem-solving skills with respect to interacting with the environment was supported, because item-specific effects of problem-solving were moderated by the number of unique test-taker interactions.
The developed ICT skills test is intended to measure higher-order ICT skills. Higher-order ICT skills include not only skills in operating technology (e.g., sending an email) but also making ICT-specific decisions (e.g., how to treat an email based on knowledge about characteristics of spam). Whether these decisions do indeed contribute to item difficulty was investigated in an experimental validation study (Engelhardt et al., 2017). Manipulating the difficulty of these decisions did indeed change the item difficulties–in both directions–without changing the construct interpretation. Items in which such decisions were eliminated were not only easier but partially measured a different construct. This supports the notion that ICT-specific decisions drive item difficulty and are also important for test score interpretation. This study provided validity evidence based on response processes (American Educational Research Association, American Psychological Association, and National Council on Measurement in Education [AERA, APA and NCME], 2014). Consequently, these two empirical studies provide support that ICT skills items contain difficulties resulting from the need to apply ICT-specific knowledge. They thus empirically support the interplay between conventional skills and ICT-specific knowledge described above (see Interplay with ICT-specific Knowledge).
Target Group of the Assessment
In the present research, the ICT skills items were developed to measure ICT skills in a defined population, 15-year-old students. This target group naturally influenced item development, for example, regarding the linguistic presentation of the items, their contextualization, and the targeted difficulty level. According to prior empirical research, younger individuals have higher photo-visual skills and fewer operational or formal skill-related problems compared to older individuals, but perform worse when it comes to evaluating information (Lorenzen, 2001; Eshet-Alkalai and Amichai-Hamburger, 2004; van Deursen and van Dijk, 2009; Eshet-Alkalai and Chajut, 2010). Therefore, it is likely that the item difficulties would change if the items developed here were presented to individuals from different age groups or with different levels of education. In addition, a different sample might take more or less time to solve the items due to the differences mentioned above–for instance, more or fewer problems with operational or formal skills. Accordingly, if these items were to be used in a different age group, further evidence on the validity of the intended test score interpretations would need to be collected. If the item difficulty of a few items had to be adjusted, one approach might be to change the amount of ICT-specific knowledge required (cf. Engelhardt et al., 2017). Further adaptations for different target groups could concern the linguistic presentation of the items and their contextualization. Consequently, item development and outcomes may also depend on sample characteristics such as age or education level. However, we consider the assessment framework, design principles, and even the presented strategies for considering test-taker behavior to be independent of the sample characteristics.
Conclusion
In the present study, we demonstrated that allegedly new domains such as ICT skills can be related back to theories concerning well-established constructs. Design principles completed the foundation of the item development process. We assume that the presented approach to item development and implementation is useful not only for assessing ICT skills, but also other contemporary constructs, such as 21st century skills, assessed in computer-based simulations. Moreover, we assume that the presented strategies for analyzing test-taking behavior, namely comparing empirical test-taker interactions and processing times to a theoretically defined minimum, could be useful for screening item functioning and that the presented approaches can be applied to performance items from other domains.
Data Availability Statement
The raw data supporting the conclusions of this article will be made available by the authors, without undue reservation.
Ethics Statement
Ethical review and approval was not required for the study on human participants in accordance with the local legislation and institutional requirements. Written informed consent to participate in this study was provided by the participants' legal guardian/next of kin.
Author Contributions
LE, JN, FG, AF, HH, KH, and FW developed the Assessment Framework which served as basis for the development and implementation of items. LE wrote the first draft of the manuscript and discussed it with JN, FG, AF, HH, KH, and FW. LE developed and applied the approaches for the empirical analyses and discussed them with JN, FG, and AF, who also contributed to the revision in terms of language and content. LE, JN, FG, AF, HH, KH, and FW approved the final version of the manuscript for submission.
Funding
This work was supported by the German Federal Ministry of Education and Research (grant numbers: 01LSA010, 01LSA010A, 01LSA010B). The publication of this article was funded by the Open Access Fund of the Leibniz Association.
Conflict of Interest
The authors declare that the research was conducted in the absence of any commercial or financial relationships that could be construed as a potential conflict of interest.
Acknowledgments
Some of this manuscript’s content was previously published as part of LE’s dissertation (Engelhardt, 2018).
References
American Educational Research Association, American Psychological Association and National Council on Measurement in Education [AERA, APA and NCME] (2014). Standards for Educational and Psychological Testing. Washington, DC: American Psychological Association.
Bhavnani, S. K., Jacob, R. T., Nardine, J., and Peck, F. A. (2003). “Exploring the Distribution of Online Healthcare Information,” in CHI'03 Extended Abstracts on Human Factors in Computing Systems, Ft. Lauderdale, FL, April 5–10, 2003 (New York, NY: Association for Computing Machinery), 816–817.
Brand-Gruwel, S., Wopereis, I., and Walraven, A. (2009). A Descriptive Model of Information Problem Solving while Using Internet. Comput. Educ. 53, 1207–1217. doi:10.1016/j.compedu.2009.06.004
Calvani, A., Cartelli, A., Fini, A., and Ranieri, M. (2009). Models and Instruments for Assessing Digital Competence at School. J. e-Learning Knowledge Society-English Version 4 (3), 183–193. doi:10.20368/1971-8829/288
Chen, C.-Y., Pedersen, S., and Murphy, K. L. (2011). Learners' Perceived Information Overload in Online Learning via Computer-Mediated Communication. Res. Learn. Technol. 19, 101–116. doi:10.1080/21567069.2011.586678
Cox, A. M., Vasconcelos, A. C., and Holdridge, P. (2010). Diversifying Assessment through Multimedia Creation in a Non‐technical Module: Reflections on the MAIK Project. Assess. Eval. Higher Educ. 35, 831–846. doi:10.1080/02602930903125249
Day, S. B., and Goldstone, R. L. (2012). The Import of Knowledge Export: Connecting Findings and Theories of Transfer of Learning. Educ. Psychol. 47, 153–176. doi:10.1080/00461520.2012.696438
De Ayala, R. J. (2013). The Theory and Practice of Item Response Theory. New York, NY. Guilford Publications.
DeStefano, D., and LeFevre, J.-A. (2007). Cognitive Load in Hypertext Reading: A Review. Comput. Hum. Behav. 23, 1616–1641. doi:10.1016/j.chb.2005.08.012
Edmunds, A., and Morris, A. (2000). The Problem of Information Overload in Business Organisations: a Review of the Literature. Int. J. Inf. Manage. 20, 17–28. doi:10.1016/S0268-4012(99)00051-1
Embretson, S. E. (1983). Construct Validity: Construct Representation versus Nomothetic Span. Psychol. Bull. 93, 179–197. doi:10.1037/0033-2909.93.1.179
Engelhardt, L. (2018). Fertigkeiten für die Lösung von kognitiven ICT-Aufgaben - Entwicklung und empirische Erprobung eines Erhebungs- und Validierungskonzepts. [dissertation]. Frankfurt am Main, Germany: Universitätsbibliothek Johann Christian SenckenbergAvailable at: http://publikationen.ub.uni-frankfurt.de/frontdoor/index/index/docId/46804 (Accessed May 4, 2021).
Engelhardt, L., Goldhammer, F., Naumann, J., and Frey, A. (2017). Experimental Validation Strategies for Heterogeneous Computer-Based Assessment Items. Comput. Hum. Behav. 76, 683–692. doi:10.1016/j.chb.2017.02.020
Engelhardt, L., Naumann, J., Goldhammer, F., Frey, A., Wenzel, S. F. C., Hartig, K., et al. (2020). Convergent Evidence for the Validity of a Performance-Based ICT Skills Test. Eur. J. Psychol. Assess. 36, 269–279. doi:10.1027/1015-5759/a000507
Eshet-Alkalai, Y., and Chajut, E. (2010). You Can Teach Old Dogs New Tricks: The Factors that Affect Changes over Time in Digital Literacy. JITE:Res. 9 (1), 173–181. doi:10.28945/1186 Available at: http://www.jite.org/documents/Vol9/JITEv9p173-181Eshet802.pdf
Eshet-Alkalai, Y. (2004). Digital Literacy: A Conceptual Framework for Survival Skills in the Digital Era. J. Educ. Multimedia Hypermedia, Norfolk, VA: Association for the Advancement of Computing in Education (AACE) 13, 93–107. Available at: http://www.openu.ac.il/Personal_sites/download/Digital-literacy2004-JEMH.pdf (Accessed May 4, 2021).
Eshet-Alkalai, Y. E., and Amichai-Hamburger, Y. (2004). Experiments in Digital Literacy. CyberPsychology Behav. 7, 421–429. doi:10.1089/cpb.2004.7.421
European Communities (2007). Key Competences for Lifelong Learning: European Reference Framework. Luxembourg: Publications Office of the European UnionAvailable at: https://www.erasmusplus.org.uk/file/272/download (Accessed 04 12, 2021).
Ferrari, A., Punie, Y., and Redecker, C. (2012). “Understanding Digital Competence in the 21st Century: an Analysis of Current Frameworks,” in 21st Century Learning for 21st Century Skills. EC‐TEL 2012. Lecture Notes in Computer Science (Berlin, Heidelberg: Saarbrücken, Germany, September 18‐21, 2012. Editors A. Ravenscroft, S. Lindstaedt, C. D. Kloos, and D. Hernández‐Leo Springer), 79–92.
Flower, L., and Hayes, J. R. (1981). A Cognitive Process Theory of Writing. Coll. Compost. Commun. 32, 365–387. doi:10.2307/356600
Fraillon, J., and Ainley, J. (2010). The IEA International Study of Computer and Information Literacy (ICILS). Available at: http://www.researchgate.net/profile/John_Ainley/publication/268297993_The_IEA_International_Study_of_Computer_and_Information_Literacy_%28ICILS%29/links/54eba4330cf2082851be49a9.pdf (Accessed May 4, 2021).
Frey, A., Hartig, J., and Rupp, A. A. (2009). An NCME Instructional Module on Booklet Designs in Large-Scale Assessments of Student Achievement: Theory and Practice. Educ. Meas. Issues Pract. 28, 39–53. doi:10.1111/j.1745-3992.2009.00154.x
Funke, J., and Frensch, P. A. (2007). “Complex Problem Solving: The European Perspective - 10 Years after,” in Learning to Solve Complex Scientific Problems. Editor D. H. Jonassen (New York, NY, US: Lawrence Erlbaum Associates), 25–47.
Goldhammer, F., Gniewosz, G., and Zylka, J. (2016). “ICT Engagement in Learning Environments,” in Assessing Contexts of Learning: An International Perspective. Editors S. Kuger, E. Klieme, N. Jude, and D. Kaplan (Dordrecht: Springer International Publishing), 331–351. doi:10.1007/978-3-319-45357-6_13
Goldhammer, F., Martens, T., and Lüdtke, O. (2017). Conditioning Factors of Test-Taking Engagement in PIAAC: an Exploratory IRT Modelling Approach Considering Person and Item Characteristics. Large-scale Assess. Educ. 5, 1–25. doi:10.1186/s40536-017-0051-9
Hahnel, C., Goldhammer, F., Naumann, J., and Kröhne, U. (2016). Effects of Linear Reading, Basic Computer Skills, Evaluating Online Information, and Navigation on Reading Digital Text. Comput. Hum. Behav. 55, 486–500. doi:10.1016/j.chb.2015.09.042
Hämeen-Anttila, K., Nordeng, H., Kokki, E., Jyrkkä, J., Lupattelli, A., Vainio, K., et al. (2014). Multiple Information Sources and Consequences of Conflicting Information about Medicine Use during Pregnancy: a Multinational Internet-Based Survey. J. Med. Internet Res. 16, e60. doi:10.2196/jmir.2939
Horz, H., Winter, C., and Fries, S. (2009). Differential Benefits of Situated Instructional Prompts. Comput. Hum. Behav. 25, 818–828. doi:10.1016/j.chb.2008.07.001
International ICT Literacy Panel (2002). Digital Transformation: A Framework for ICT Literacy: ETS. Available at: http://www.ets.org/Media/Research/pdf/ICTREPORT.pdf (Accessed 10 14, 2019).
Kane, M. T. (2013). Validating the Interpretations and Uses of Test Scores. J. Educ. Meas. 50, 1–73. doi:10.1111/jedm.12000
Kiefer, T., Robitzsch, A., and Wu, M. (2016). TAM: Test Analysis Modules. R package version 1.99-6. Available at: http://CRAN.R-project.org/package=TAM (Accessed April 16, 2016).
Kintsch, W. (1998). Comprehension: A Paradigm for Cognition. Cambridge, UK: Cambridge University Press.
Kroehne, U., and Goldhammer, F. (2018). How to Conceptualize, Represent, and Analyze Log Data from Technology-Based Assessments? A Generic Framework and an Application to Questionnaire Items. Behaviormetrika 45, 527–563. doi:10.1007/s41237-018-0063-y
Lorenzen, M. (2001). The Land of Confusion? Res. Strateg. 18, 151–163. doi:10.1016/S0734-3310(02)00074-5
McVey, D. (2008). Why All Writing Is Creative Writing. Innov. Educ. Teach. Int. 45, 289–294. doi:10.1080/14703290802176204
Messick, S. (1994). The Interplay of Evidence and Consequences in the Validation of Performance Assessments. Educ. Res. 23, 13–23. doi:10.3102/0013189x023002013
Metzger, M. J. (2007). Making Sense of Credibility on the Web: Models for Evaluating Online Information and Recommendations for Future Research. J. Am. Soc. Inf. Sci. 58, 2078–2091. doi:10.1002/asi.20672
Mislevy, R. J. (2013). Evidence-centered Design for Simulation-Based Assessment. Mil. Med. 178, 107–114. doi:10.7205/milmed-d-13-00213
Mislevy, R. J., Steinberg, L. S., Breyer, F. J., Almond, R. G., and Johnson, L. (2002). Making Sense of Data from Complex Assessments. Appl. Meas. Educ. 15, 363–389. doi:10.1207/S15324818AME1504_03
M. Jung, and R. Carstens (2015). ICILS 2013 User Guide for the International Database (Amsterdam: IEA). Available at: http://pub.iea.nl/fileadmin/user_upload/Publications/Electronic_versions/ICILS_2013_IDB_user_guide.pdf (Accessed 10 14, 2019).
National Higher Education ICT Initiative (2003). Succeeding in the 21st Century: What Higher Education Must Do to Address the Gap in Information and Communication Technology Proficiencies. Available at: http://www.ets.org/Media/Tests/Information_and_Communication_Technology_Literacy/ICTwhitepaperfinal.pdf.
OECD (2012). Literacy, Numeracy and Problem Solving in Technology-Rich Environments: Framework for the OECD Survey of Adult Skills. Paris, France: OECD Publishing. doi:10.1787/9789264128859-en
Parshall, C. G., Spray, J. A., Kalohn, J. C., and Davey, T. (2002). “Considerations in Computer-Based Testing,” in Practical Considerations in Computer-Based Testing. Editors C. G. Parshall, J. A. Spray, L. Kalohn, and T. Davey (New York: Springer), 1–12. doi:10.1007/978-1-4613-0083-0_1
Perfetti, C. A., Rouet, J.-F., and Britt, M. A. (1999). “Toward a Theory of Documents Representation,” in The Construction of Mental Representations during Reading. Editors H. van Oostendorp, and S. R. Goldman (Mahwah, NJ, US: Lawrence Erlbaum Associates Publishers), 99–122.
Poynton, T. A. (2005). Computer Literacy across the Lifespan: A Review with Implications for Educators. Comput. Hum. Behav. 21, 861–872. doi:10.1016/j.chb.2004.03.004
R Core Team (2014). R: A Language and Environment for Statistical Computing. Vienna, Austria: R Foundation for Statistical ComputingAvailable at: http://www.R-project.org/.
Richter, T., Naumann, J., and Horz, H. (2010). Eine revidierte Fassung des Inventars zur Computerbildung (INCOBI-R). Z. für Pädagogische Psychol. 24, 23–37. doi:10.1024/1010-0652/a000002
Rieh, S. Y. (2002). Judgment of Information Quality and Cognitive Authority in the Web. J. Am. Soc. Inf. Sci. 53, 145–161. doi:10.1002/asi.10017
Rölke, H. (2012). “The Item Builder: A Graphical Authoring System for Complex Item Development,” in Proceedings of World Conference on E-Learning in Corporate, Government, Healthcare, and Higher Education. Editors T. Bastiaens, and G. Marks (Chesapeake, VA: AACE), 344–353.
Rouet, J.-F. (2006). The Skills of Document Use: From Text Comprehension to Web-Based Learning. Mahwah, NJ, US: Lawrence Erlbaum Associates Publishers. doi:10.4324/9780203820094
Scalise, K., and Gifford, B. R. (2006). Computer-Based Assessment in E-Learning: A Framework for Constructing "Intermediate Constraint" Questions and Tasks for Technology Platforms. J. Teach. Learn. Assess. 4 (6). Available at: https://ejournals.bc.edu/ojs/index.php/jtla/article/view/1653/0(Accessed May 4, 2021).
Schnotz, W. (2005). “An Integrated Model of Text and Picture Comprehension,” in The Cambridge Handbook of Multimedia Learning. Editor R. E. Mayer (New York, NY, US: Cambridge University Press), 49–70. doi:10.1017/cbo9780511816819.005
Siddiq, F., Hatlevik, O. E., Olsen, R. V., Throndsen, I., and Scherer, R. (2016). Taking a Future Perspective by Learning from the Past - A Systematic Review of Assessment Instruments that Aim to Measure Primary and Secondary School Students' ICT Literacy. Educ. Res. Rev. 19, 58–84. doi:10.1016/j.edurev.2016.05.002
Simon, H. A., and Newell, A. (1971). Human Problem Solving: The State of the Theory in 1970. Am. Psychol. 26, 145–159. doi:10.1037/h0030806
Simpson, C. W., and Prusak, L. (1995). Troubles with Information Overload-Moving from Quantity to Quality in Information Provision. Int. J. Inf. Manage. 15, 413–425. doi:10.1016/0268-4012(95)00045-9
Singley, M. K., and Anderson, J. R. (1985). The Transfer of Text-Editing Skill. Int. J. Man-Machine Stud. 22, 403–423. doi:10.1016/S0020-7373(85)80047-X
Sireci, S. G., and Zenisky, A. L. (2006). “Innovative Item Formats in Computer-Based Testing: In Pursuit of Improved Construct Representation,” in Handbook of Test Development. Editors S. M. Downing, and T. M. Haladyna (Mahwah, New Jersey: Lawrence Erlbaum Associates, Inc), 329–347.
van Deursen, A. J. A. M., and van Dijk, J. A. G. M. (2009). Using the Internet: Skill Related Problems in Users' Online Behavior. Interacting Comput. 21 (5), 393–402. doi:10.1016/j.intcom.2009.06.005
van Deursen, A. J. (2010). Internet Skills: Vital Assets in an Information Society. Netherlands: University of Twente. doi:10.3990/1.9789036530866
Van Deursen, A. J., and Van Dijk, J. A. (2014). Digital Skills: Unlocking the Information Society. Springer.
van Deursen, A., and van Dijk, J. (2011). Internet Skills and the Digital Divide. New Media Soc. 13, 893–911. doi:10.1177/1461444810386774
van Dijck, J. (2009). Users like You? Theorizing Agency in User-Generated Content. Media, Cult. Soc. 31, 41–58. doi:10.1177/0163443708098245
Wenzel, S. F. C., Engelhardt, L., Hartig, K., Kuchta, K., Frey, A., Goldhammer, F., et al. (2016). “Computergestützte, adaptive und verhaltensnahe Erfassung Informations- und Kommunikationstechnologie-bezogener Fertigkeiten (ICT-Skills) (CavE-ICT),” in Forschung in Ankopplung an Large-Scale Assessments. Editor BMBF (Hrsg.) (Bonn, Berlin: BMBF), 161–180.
Whittaker, S., and Sidner, C. (1996). “Email Overload,” in, CHI96: CHI '96 ACM Conference on Human Factors, Vancouver, BC, April 13‐18, 1996. Editor J. T. Michael (New York, NY: Association for Computing Machinery). doi:10.1145/238386.238530
Keywords: information and communication technology skills, assessment framework, performance items, validation, behavioral indicators
Citation: Engelhardt L, Naumann J, Goldhammer F, Frey A, Horz H, Hartig K and Wenzel SFC (2021) Development and Evaluation of a Framework for the Performance-Based Testing of ICT Skills. Front. Educ. 6:668860. doi: 10.3389/feduc.2021.668860
Received: 17 February 2021; Accepted: 27 April 2021;
Published: 13 May 2021.
Edited by:
Bernhard Ertl, Munich University of the Federal Armed Forces, GermanyReviewed by:
Małgorzata Kisilowska, University of Warsaw, PolandSirje Virkus, Tallinn University, Estonia
Copyright © 2021 Engelhardt, Naumann, Goldhammer, Frey, Horz, Hartig and Wenzel. This is an open-access article distributed under the terms of the Creative Commons Attribution License (CC BY). The use, distribution or reproduction in other forums is permitted, provided the original author(s) and the copyright owner(s) are credited and that the original publication in this journal is cited, in accordance with accepted academic practice. No use, distribution or reproduction is permitted which does not comply with these terms.
*Correspondence: Lena Engelhardt, ZW5nZWxoYXJkdGxAZGlwZi5kZQ==