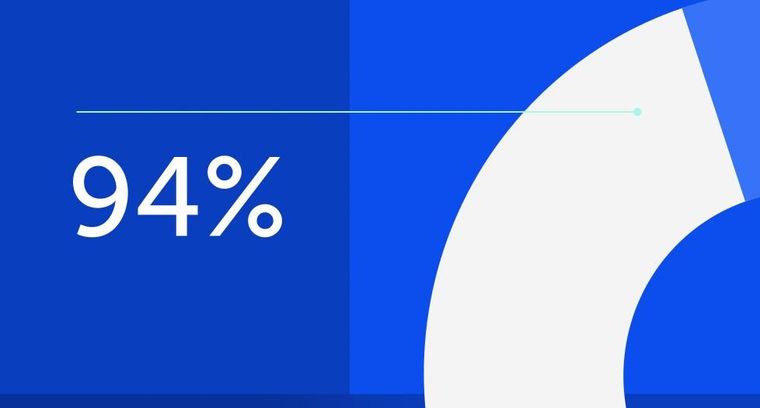
94% of researchers rate our articles as excellent or good
Learn more about the work of our research integrity team to safeguard the quality of each article we publish.
Find out more
HYPOTHESIS AND THEORY article
Front. Educ., 26 May 2021
Sec. Assessment, Testing and Applied Measurement
Volume 6 - 2021 | https://doi.org/10.3389/feduc.2021.653693
This article is part of the Research TopicThe Use of Organized Learning Models in AssessmentView all 6 articles
In education, taxonomies that define cognitive processes describe what a learner does with the content. Cognitive process dimensions (CPDs) are used for a number of purposes, such as in the development of standards, assessments, and subsequent alignment studies. Educators consider CPDs when developing instructional activities and materials. CPDs may provide one way to track students’ progress toward acquiring increasingly complex knowledge. There are a number of terms used to characterize CPDs, such as depth-of-knowledge, cognitive demand, cognitive complexity, complexity framework, and cognitive taxonomy or hierarchy. The Dynamic Learning Maps (DLM™) Alternate Assessment System is built on a map-based model, grounded in the literature, where academic domains are organized by cognitive complexity as appropriate for the diversity of students with significant cognitive disabilities (SCD). Of these students, approximately 9% either demonstrate no intentional communication system or have not yet attained symbolic communication abilities. This group of students without symbolic communication engages with and responds to stimuli in diverse ways based on context and familiarity. Most commonly used cognitive taxonomies begin with initial levels, such as recall, that assume students are using symbolic communication when they process academic content. Taxonomies that have tried to extend downward to address the abilities of students without symbolic communication often include only a single dimension (i.e., attend). The DLM alternate assessments are based on learning map models that depict cognitive processes exhibited at the foundational levels of pre-academic learning, non-symbolic communication, and growth toward higher levels of complexity. DLM examined existing cognitive taxonomies and expanded the range to include additional cognitive processes that demonstrate changes from the least complex cognitive processes through early symbolic processes. This paper describes the theoretical foundations and processes used to develop the DLM Cognitive Processing Dimension (CPD) Taxonomy to characterize cognitive processes appropriate for map-based alternate assessments. We further explain how the expanded DLM CPD Taxonomy is used in the development of the maps, extended standards (i.e., Essential Elements), alternate assessments, alignment studies, and professional development materials. Opportunities and challenges associated with the use of the DLM CPD Taxonomy in these applications are highlighted.
Jean Piaget proposed the first major theory of cognitive development in children beginning in the early 1920s and offered a complete description in a seminal 1983 publication. He suggested that children progress through qualitatively distinct stages in thinking and understanding from birth to maturity (Miller, 2011). He proposed four stages of mental growth: Sensorimotor stage—birth to 2 years, Preoperational stage—ages 2 to 7, Concrete operational stage—ages 7 to 11, and Formal operational stage—ages 12 and up. The theory was based on a constructivist approach, where early cognitive development involves processes based upon actions and later progresses to changes in mental operations and abstract thinking (Cherry, 2020). Piaget’s theory of cognitive development generated an enthusiasm that influenced educators to develop new teaching methods, particularly in science and mathematics (Demetriou et al., 2016). Teachers began to take this theory into account as they taught learners at different levels of intellectual development and developed curricula that supported cognitive development by learning concepts in logical steps (Bukatko and Daehler, 1995; Mwamwenda, 2009). Unfortunately, the distinct stages of thinking and understanding proposed by Piaget do not apply uniformly to students with disabilities, especially not to students with significant cognitive disabilities (Bernard et al., 2019). Furthermore, Piaget held that the development of thought required interactions with the physical environment, interactions that are not possible for many students with significant cognitive disabilities (Bruce and Borders, 2015).
Around the same time as Piaget, another school of thought developed. Drawing on the empiricist tradition, behaviorists conceptualized learning as a process of forming connections between stimuli and responses. Frequently using animals in laboratory studies, behaviorists theorized that motivation to learn was propelled primarily by drives, such as hunger, and the availability of external forces, such as rewards and punishments (e.g., Thorndike, 1913; Skinner, 1950). In the classroom setting, behaviorist theories of behavior modification predominately influenced teachers’ use of classroom management strategies. However, behaviorism also influenced instructional design. Observable behaviors were identified that connected to learning objectives determined through task analysis. Then specific measurable tasks were developed to measure learning success (Rostami and Khadjooi, 2010). This orientation made it difficult to study such phenomena as understanding, reasoning, and thinking (Pellegrino et al., 2016)—phenomena that are of paramount importance for education. Nonetheless, this orientation is the basis of evidence-based instructional practices ranging from approaches to prompting (Institute of Education Sciences 2018) to procedures like task analysis and constant time delay (e.g., Browder et al., 2014) to teach clearly articulated academic skills to students with significant cognitive disabilities.
Then in 1999, a National Research Council et al., 2001(NRC) Committee on Developments in the Science of Learning published a report, How People Learn, that summarized the implications of the changes in thinking about cognition for designing effective teaching and learning. This committee reviewed the extensive scientific literatures on cognition, learning, development, culture, and the brain, including: research on human learning and new developments from neuroscience; learning research that has implications for the design of formal instructional environments, primarily preschools, kindergarten through high schools (K–12), and colleges; and research that helps explore the possibility of helping all individuals achieve their fullest potential The continuing effects of this and other work including Knowing What Students Know (NRC 2001), along with next-generation rigorous college and career readiness standards (CCRS), have prompted a move away from requiring static knowledge proficiency. Instead of teaching memorization of facts, there is a new focus on higher-order thinking skills, problem-solving, and metacognitive skills, such as self-monitoring. This shift has resulted in efforts to generate models of cognition to classify these processes.
However, this evolving understanding of cognition and how people learn and its implications for how teachers teach has largely been confined to general education. Teachers of students with disabilities, especially those with the most significant cognitive disabilities, have generally been taught following behaviorists’ practices (Brown et al., 2020). These practices have their roots in an age of institutionalization and custodial care employing deficit-based views of what children can do and limiting learning to discrete, measurable, observable behaviors (Jackson et al., 2008; Kleinert et al., 2009). In this historical context, teaching and learning are framed as processes of training students to exhibit desired behaviors in order to mediate impairment and maximize life skills (Brown et al., 2020; Thomas and O’Hanlon 2007). As the demands to provide students with the most significant cognitive disabilities with access to the general education standards increased through the last two revisions of the Individuals with Disabilities Education Act (1997, 2004) and the Elementary and Secondary Education Act (2001, 2015), there have been few shifts away from these practices. In fact, they have gained status as evidence-based practices in textbooks (Brown et al., 2020; Mims 2020), practice guides (Browder et al., 2014), and sources like the What Works Clearinghouse (e.g., https://ies.ed.gov/ncee/wwc/Intervention/1374). Unfortunately, these approaches focus on teaching lower-order skills such as word identification (Browder et al., 2009) and mathematical computation (Browder et al., 2008) rather than the higher order skills that are the focus of current academic content standards and required by the (Every Student Succeeds Act, 2015).
This paper describes the theoretical foundations and processes used to develop an expanded cognitive processing dimension (CPD) taxonomy to characterize cognitive processes appropriate for map-based alternate assessments with particular attention to students with significant cognitive disabilities. We further explain how the expanded CPD taxonomy is used in the development of the maps, extended standards (i.e., Essential Elements), alternate assessments, and alignment studies for assessments of English language arts, mathematics, and science. Opportunities and challenges associated with the use of the expanded taxonomy in these contexts are highlighted. Applications in this paper are delimited to the case of the Dynamic Learning Maps (DLM™) Alternate Assessment System, the first map-based assessment system developed for large-scale use.
The CCRS for English language arts and mathematics represent the goals for what students should learn and identify what a student should know and be able to do at the end of each grade. Likewise, the three-dimensional next-generation science standards (NGSS) describe grade-level expectations but are arranged so that students have multiple opportunities—from elementary through high school—to build knowledge and skills. NGSS materials also include high level grade-span progressions for disciplinary core ideas, science and engineering practices, and crosscutting concepts (NGSS Appendices E, F, G 2013).
Assessment instruments based solely on these grade-level expectations provide information about the status of achievement at a point in time but lack information about what knowledge and skills students actually have or the reasons a student may be struggling with a skill or concept (Bechard et al., 2012). The discrepancy between what students know and what is slated to be taught causes many teachers to experience a dilemma that has serious implications for student learning: the dilemma of addressing students’ learning needs vs. maintaining the grade-level expectations (Confrey, 2019). Work ensued to develop adaptive systems that deliver curriculum and instruction to meet the needs of all the students, while at the same time ensuring progress at appropriate rates towards readiness for college and careers (Confrey, 2019). These systems included organized learning models, such as learning progressions, learning trajectories, and learning maps as models to describe ideas students are likely to hold at varying levels of sophistication, helping educators focus on students’ existing understanding, rather than on what students do not know.
Unlike grade-level standards, these organized learning models reflect the systematic consideration of interactions among the learner, the content, and the context for learning (e.g., situational, sociocultural, nature of support/scaffolding), as well as the dynamic, cumulative outcomes of these interactions (Bechard et al., 2012). Researchers and educators began to advance the position that learning ought to be coordinated and sequenced over longer periods of time; not over days of isolated lessons but rather over months and years of continuous development (Duschl, 2019). In addition, contemporary research now rejects age-based stages of development (e.g., Piaget’, 1983 concrete to formal) while maintaining the notion of developmental pathways. Specifically, developmental psychology research (NRC, 2007) indicates that learning progressions can start at early ages well before children enter formal schooling (Duschl, 2019). This research shows that young children ages three to four are, in select domains, capable of sophisticated reasoning (NRC, 2007). With such nuanced and structured models of student learning, assessments can not only check for whether students have the correct answer, but obtain a more accurate picture in the context of the domain of what students truly understand to inform instruction that is responsive to students’ learning needs (Alonzo, 2018).
With the emergence of the field of cognitive science, the processes of knowing became fertile grounds for research. This new science recognized that humans come to formal education with a range of prior knowledge, skills, beliefs, and concepts that significantly influence what they notice about the environment and how they organize and interpret it, which in turn, affects their abilities to remember, reason, solve problems, and acquire new knowledge. Beginning with the work of (Bloom et al., 1956) and others, taxonomies were created to codify the thought processes at work in most learning tasks by classifying learning objectives into levels of relative complexity. This concept of relative complexity has always been critical to understanding taxonomies, as complexity is not absolute. Instead, it must be interpreted relative to the content. These taxonomies were originally intended to help teachers develop educational objectives based on cognitive difficulty in order to provide a range of learning opportunities and observe student progress in the classroom. As the standards-based movement gained traction, these and other taxonomies were expanded or created to evaluate the coherence of the standards/instruction/assessment system. In this section we briefly describe the characteristics and uses of four commonly used taxonomies: Revised Bloom’s Taxonomy, Marzano’s New Taxonomy, Webb’s Depth of Knowledge, and Achieve’s Performance Centrality. The key components of the models are summarized in Figure 1.
FIGURE 1. Summary of common cognitive processing classifications from lowest to highest complexity levels.
To facilitate test development, Benjamin Bloom created one of the first systematic classifications of the processes of thinking and learning, by carefully defining a framework into which items measuring the same objective could be classified (Bloom et al., 1956). It was a one-dimensional cumulative hierarchical framework consisting of six categories of cognitive complexity, each requiring achievement of the prior skill or ability before moving to the next more complex category. In the late 1990s, a team of cognitive psychologists, curriculum theorists and instructional researchers, and testing and assessment specialists updated the taxonomy to make it more applicable to a wider audience and support its expanded use in classroom applications (Anderson and Krathwohl, 2001). The authors of the Revised Bloom’s Taxonomy (RBT) underscored a new dynamism, using verbs to label their categories and subcategories (rather than the nouns of the original taxonomy). These “action words” describe the cognitive processes by which thinkers encounter and work with knowledge (Armstrong, 2020). The revised taxonomy was subsequently enhanced by the addition of four knowledge dimensions (factual, conceptual, procedural, metacognitive) to intersect with the six cognitive process dimensions. With this addition, it became a two-dimensional taxonomy, acknowledging that thought processes occur within specific learning contexts.
Bloom recognized cognitive, affective, and psychomotor domains and measured the cognitive level students are expected to show in order to prove a learning experience occurred (Miller, 2011). When first published as a Taxonomy of Educational Objectives (1956), Bloom focused on the instructional uses of the taxonomy in order to help teachers create course objectives and to develop aligned classroom assessments. The RBT focused on the cognitive domain. It was based on the theory that the lower-order skills require less cognitive processing, but provide an important base for learning. Meanwhile, the higher levels require deeper learning and a greater degree of cognitive processing, which can presumably only be achieved once the lower-order skills have been mastered (Persaud, 2018). The RBT intended to provide a basis for moving curricula and tests toward objectives that would be classified in the more complex categories (Krathwohl, 2002).
Marzano and Kendall (2007) proposed a New Taxonomy in response to Bloom’s original and revised taxonomies. It was designed to be used: 1) for classifying educational objectives, 2) for enhancing state and district-level standards, 3) as a framework for designing educational objectives within a thinking skills curriculum, and 4) as a framework for educational assessments. The New Taxonomy shares many similarities with the RBT (Marzano and Kendall, 2007; Irvine, 2017). Both employ two dimensions; the RBT has a knowledge dimension (four dimensions) and a cognitive process dimension (six dimensions), and the New Taxonomy has a domain of knowledge dimension (three domains) and a levels-of-processing dimension (six levels). Both taxonomies classify educational tasks by considering the type of knowledge that is the focus of instruction and the type of mental processing the task imposes on that knowledge. The classification of the cognitive processing described in both systems is similar (e.g., problem solving requires greater cognitive demand than retrieval or comprehension). Both focus on educational objectives. One main difference involves the placement of metacognitive knowledge. While RBT includes it as one of the four types of knowledge in the same dimension as subject matter content, metacognition in the New Taxonomy represents a type of processing that is applied to subject matter content (Marzano and Kendall, 2007). Other significant differences are the inclusion of psychomotor procedures among the three domains of knowledge, and the addition of the self-system, which is listed as the highest level in the processing dimension, in the New Taxonomy. Marzano and Kendall state that the self-system is placed at the top of the levels of processing hierarchy “because it controls whether or not a learner engages in a new task and the level of energy or motivation allotted to the task if the learner chooses to engage” (Marzano and Kendall, 2007, 18). The psychomotor domain involves the physical procedures an individual uses to negotiate daily life and to engage in complex physical activities for work and for recreation. It is considered a type of knowledge because it is developmental and stored in memory, as are mental procedures. Marzano and Kendall posit that the theory underlying the New Taxonomy “improves on Bloom’s effort in that it allows for the design of a hierarchical system of human thought from the perspective of two criteria: 1) flow of information and 2) level of consciousness” (Marzano and Kendall 2007, 16).
(Webb’s, 1997) Depth of Knowledge (DOK) is a four-point scale (recall, skill/concept, strategic thinking, and extended thinking) that is widely used for alignment studies involving general education assessments, often with specificity per subject area in addition to the generic description (Herman, 2015). Webb asserted that standardized assessments measured how students think about a content and the procedures learned but did not measure how deeply students must understand and be aware of their learning so they can explain answers and provide solutions, as well as transfer what was learned to real world contexts. Webb defined alignment as “…the degree to which expectations and assessments are in agreement and serve in conjunction with one another to guide the system toward students learning what they are expected to know and do” (Webb, 1997, 4). In addition to DOK, Webb included criteria for categorical concurrence, range of knowledge correspondence, and balance of representation to evaluate the alignment of standards to assessments. DOK is probably the most widely used criterion, as it evaluates the match between the cognitive demand of the assessment tasks and the performance expectations of the standards. In practice, this is rated using the DOK rubric demonstrating that at least 50% of the items are at or above the DOK of the standard to which the items correspond. The main concern with this aspect of alignment is that assessment items should not be targeting skills that are below those required by the objectives to which the item is matched (Martone and Sireci, 2009). Essentially, the goal of DOK is to establish the context—the scenario, the setting, or the situation—in which students express the depth and extent of the learning (Francis, 2017).
In 2006, Achieve developed a model that addressed six criteria: accuracy of the test blueprint, content centrality, performance centrality, challenge, balance, and range. The performance centrality criterion is a rating of cognitive complexity similar to Webb’s DOK criterion (Forte, 2017) and describes cognitive complexity as the nature and level of higher order thinking required by a task (Achieve, 2018a). An item's cognitive complexity accounts for the content area and cognitive and linguistic demands required to understand, process, and respond successfully to it. In alignment studies, the focus of this criterion is to determine whether the assessment items, as well as the assessment program as a whole, reflect a range of cognitive demand that is sufficient to assess the depth and complexity of the state’s standards. Cognitive demand is best evaluated using classifications specific to the discipline. Reviewers consider to what extent the DOK of the items match the developer-claimed DOK and whether the documentation indicates processes to determine DOK and ensure that a range is represented on all forms across the assessment program (Achieve, 2018b). Two criteria are frequently employed by Achieve to evaluate cognitive complexity; the first is a variation of Webb’s DOK (Level 1—recall or basic comprehension, Level 2—application of skill/concept, Level 3—strategic thinking, Level 4—extended analysis, typically during an extended period of time), and the second is an evaluation of performance centrality (Achieve, 2002). Performance centrality is described as the match between the types of performance found in the components being evaluated. Content experts rate the match using a three-point scale (none, some, and all) primarily focusing on the verbs used in each component being examined.
Cognitive taxonomies have played a role in the design of educational assessments since Bloom developed the original taxonomy in the 1950s and have grown more prominent in response to legislative changes and advances in the field since 2000. The passage of the No Child Left Behind Act of 2001 (NCLB Act) incorporated major reforms, particularly in the areas of assessment, accountability, and school improvement. It required challenging academic content standards in academic subjects and a set of high-quality, annual academic assessments to monitor the achievement of all students, including, for the first time, students with disabilities in state accountability systems. The assessments had to include measures that assessed higher-order thinking skills and understanding, while providing reasonable adaptations and accommodations for students with disabilities and students with limited English proficiency. Peer review guidance (United States Department of Education, 2004) stipulated that assessments needed to reflect the full range of cognitive complexity and level of difficulty and depth of the concepts and processes described in the State’s academic content standards, meaning that the assessments were as demanding as the standards. Thus, an increased focus on alignment and complexity was initiated.
These mandates set off a flurry of activities to develop content standards and assessments that could provide valid measures of student achievement. National initiatives formed to develop high quality academic content standards [e.g., Common Core State Standards, 2021/CCRS (National Governors Association Center for Best Practices and Council of Chief State School Officers 2014) for English language arts and mathematics, and the NGSS (NGSS Lead States, 2013)]. Grade-specific learning goals were adopted by states. Federal funding was provided under the Race to the Top initiative to support consortia to develop valid and reliable academic assessments designed to measure the new standards [e.g., Partnership for Assessment of Readiness for College and Careers (PARCC, ETS, and Pearson, 2014) and the Smarter Balanced Assessment Consortium (Smarter Balanced); Department of Education 2010].
The most recent peer review guidance (USDE 2018) reflects the increased expectations that assessments measure more complex academic learning. For example, it includes the expectation that states follow processes to “ensure that each academic assessment is tailored to the knowledge and skills included in the State’s academic content standards, reflects appropriate inclusion of challenging content, and requires complex demonstrations or applications of knowledge and skills (i.e., higher-order thinking skills)” (USDE 2018; Critical Element 2.1, 36).
The Webb and Achieve taxonomies are frequently used to evaluate the alignment of assessments (as well as the assessment blueprint in Achieve) to the standards (e.g., Smarter Balanced Assessment Consortium, 2016), while other metrics are used to investigate the alignment of instruction to the standards (e.g., Surveys of Enacted Curriculum, Blank, 2005). In terms of alignment logic, a set of standards determines what measurement targets assessment scores are meant to reflect. Efforts to explicitly define exactly what assessments are measuring became an important component of test development and subsequent alignment evaluations. In the past couple of decades, many have turned to the principles of evidence-centered design (ECD; Mislevy and Haertel, 2006; Mislevy et al., 2002) to guide this process (Forte, 2017). However, there were challenges in applying these taxonomies and ECD principles to alternate assessments for students with the most significant cognitive disabilities (Bechard et al., 2019).
Federal regulations specified that alternate assessments are intended for students who cannot participate in general statewide assessments, even with accommodations [34 CFR 300.160(c)]. First introduced in 2000, early alternate assessments tended to focus on functional life skills aligned with dominant curricular models of the 1990s. In response to NCLB, states were required to shift their alternate assessments to measure student achievement in academic content. In 2005, guidance was published (USDE) to ensure that students with the most significant cognitive disabilities could be fully included in State accountability systems through alternate assessments (AAs) and have access to challenging instruction linked to State content standards through alternate achievement standards (AASs).
Alternate assessments are designed so the heterogeneous population of students with significant cognitive disabilities can demonstrate what they know and can do. Students with the most significant cognitive disabilities are a diverse group of learners with a broad range of cognitive, linguistic, physical, and sensory strengths and needs. By definition, all students with the most significant cognitive disabilities have cognitive disabilities that significantly impact their cognitive functioning and adaptive behavior, and who require extensive supports during instruction (Thurlow et al., 2019). They receive special education services under a variety of eligibility categories as defined by the Individuals with Disabilities Education Act (e.g., autism, multiple disabilities, intellectual disability), and almost all are educated in separate classrooms or special schools (Erickson and Geist 2016). Students with significant cognitive disabilities also tend to have additional disabilities that impact their instruction and assessment. Estimates are that 12% (Towles-Reeves et al., 2009) to 19% (Kearns et al., 2011) of students with the most significant cognitive disabilities have motor impairments significant enough that they use a wheelchair and/or require assistance for most or all activities. These motor impairments also impact their ability to independently access learning materials and technologies. An estimated 4% (Erickson and Geist 2016) to 8% (Cameto et al., 2010; Towles-Reeves et al., 2009) of students with the most significant cognitive disabilities have a known hearing loss, and 7–9% have uncorrected visual impairments (Erickson and Geist 2016; Kearns et al., 2011; Towles-Reeves et al., 2009). These sensory impairments impact a variety of processes from the earliest forms of joint attention (e.g., visual demonstrations of joint attention may not be possible) to the way that visual and auditory information is processed.
Students with the most significant cognitive disabilities also vary greatly with respect to the understanding and use of symbolic communication. A variety of surveys of teachers suggest that at least half of students with the most significant cognitive disabilities communicate at an abstract, symbolic level (Browder et al., 2008; Cameto et al., 2010; Erickson and Geist 2016). These students have full access to expressive language that allows them to communicate across contexts, in flexible ways. About 18% (Browder et al., 2008) to 30% (Erickson and Geist 2016) of students with significant cognitive disabilities demonstrate only concrete symbolic communication. This means their expressive communication is restricted to the use graphic symbols, objects, signs, and other symbols that look like, feel like, move like, or sound like the things they represent. Estimates of the percent of students with the most significant cognitive disabilities who have no symbolic communication range from 9% (Erickson and Geist 2016) to 30% (Cameto et al., 2010) to as high as 35% (Browder et al., 2008). Non-symbolic communication includes gestures, vocalizations, facial expressions and body movements that must be interpreted by communication partners (i.e., teachers, peers, family members). The size of this final group varies greatly because it is directly impacted by rates of access to augmentative and alternative communication (Kearns et al., 2011). Instruction for the full spectrum of students with significant cognitive disabilities is dominated by mastery-based approaches to teaching skills identified through traditions such as task analysis (Brown et al., 2020). For the subgroup of students who do not have symbolic communication, instruction is often focused on functional skills (Ayers et al., 2011), with participation in academic assessments limited by discontinuation rules (Georgia Department of Education, 2020) and development approaches that do not explicitly address the needs of this group (Kleinert et al., 2009).
The requirement that alternate assessments must be based on grade-level academic content standards and alternate achievement standards introduced challenges for assessment designers and special education experts. The USDE released multiple guidance documents to clarify expectations. The 2004 Peer Review Guidance (USDE 2004) for alternate assessments stated that AA-AAS materials should show a clear link to the content standards for the grade in which the student is enrolled although the grade-level content may be reduced in complexity or modified to reflect pre-requisite skills. This guidance also introduced the concept of extended standards: “Extended” standards communicate the relationship between the State’s academic content standards and the content of the alternate assessment based on alternate achievement standards” (USDE 2004, Section 5.6). In 2005, the USDE provided guidance on Alternate Achievement Standards for Students with the Most Significant Cognitive Disabilities. It attempted to clarify how this group of students could be held to the same grade level academic content standard expectations as all students, but at the same time allow academic achievement standards at lower levels of depth and complexity. The guidance explained that “an alternate assessment based on alternate achievement standards may cover a narrower range of content (e.g., cover fewer objectives under each content standard) and reflect a different set of expectations in the areas of reading/language arts, mathematics, and science than do regular assessments…” (USDE 2005, 17). The guidance further explained that “an alternate achievement standard sets an expectation of performance that differs in complexity from a grade-level achievement standard” (USDE 2005, 21).
Despite federal guidance defining alternate assessment targets and alternate achievement standards, states struggled to define the academic content of their alternate assessments and align the assessments to grade-level content standards. This struggle was apparent in the results of external alignment studies conducted as a source of peer review evidence. Regardless of the alignment methodology applied, panelists considered whether states extended their content standards and developed assessment items without overstretching or losing the link to grade-level academic content standards. Alignment studies tended to confirm what was already expected to be true for alternate assessments: the assessments did not measure the full range of the state standards and primarily contained items measuring academic content at lower levels of cognitive complexity (as measured by Webb’s DOK) than the grade-level standards (Roach et al., 2005; Flowers et al., 2006). The DOK alignment findings suggested that alternate assessments generally did not leave much room to probe for more complex applications of extended academic content standards. In fact, some assessment items were rated as having DOK lower than the lowest point on Webb’s 4-point scale (Flowers et al., 2006), indicating a floor effect for Webb’s DOK taxonomy when applied to alternate assessment.
Beyond ratings on the cognitive dimension, alternate assessments of the mid-2000s did not meet other alignment criteria set for use with general assessments. For example, in a study on Webb’s alignment model applied to three states’ alternate assessments, the assessments generally did not meet Webb’s (1997) criteria for acceptable alignment based on categorical concurrence, range-of-knowledge, or balance of representation indices (Flowers et al., 2006). This pattern of findings prompted a re-evaluation of the criteria for acceptable alignment for alternate assessments as well as what needed to be evaluated in an alignment study—in other words, what parts of the educational system should be expected to be aligned.
Toward both of these goals, researchers developed the Links for Academic Learning (LAL) alignment model (Flowers et al., 2009). LAL included eight criteria to evaluate alignment within the student’s entire educational system, including the relationship of the enacted curriculum to the standards and assessments, and the degree to which instructional resources supported aligned instruction. Within the assessment system, LAL also extended beyond evaluation of the relationships between items and standards to include evaluation of administration and scoring procedures and the alternate achievement standards themselves.
LAL incorporated some metrics from Achieve and Webb alignment models but made several modifications to the metrics and criteria for acceptable alignment (Flowers et al., 2009). The cognitive taxonomy in the LAL alignment model (Flowers et al., 2009) used a modification of the original Bloom’s taxonomy, extended downward to capture minimal, intentional responses in the academic environment (“attend”), and it collapsed the upper end (analyze/synthesize/evaluate). This scale was also referred to as DOK.
Since the LAL was designed specifically for alignment studies on AA-AAS systems (including enacted curriculum), this taxonomy was more inclusive of the population of students with significant cognitive disabilities than Webb’s or Bloom’s DOK were. However, at the lowest end, LAL still assumed cognition began with joint attention, yet joint attention doesn’t begin to appear in typical development until 9 months of age and isn’t fully developed as a skill that promotes language learning until 18 months (Beuker et al., 2013). The floor of the taxonomy excluded the cognitive processes for the approximately 9–35% of students with significant cognitive disabilities who are still working toward intentional communication (Browder et al., 2008; Erickson and Geist 2016). Despite this limitation, LAL remained the recommended alignment model for alternate assessments for at least a decade (Forte, 2017).
Shifts toward more rigorous academic content standards and general statewide assessment prompted by the Race to the Top initiative also led to changes in academic expectations for students with significant cognitive disabilities. In response to states’ adoption of CCRS, states also revised their extended content standards or curriculum frameworks for students with significant cognitive disabilities. Table 1 illustrates the contrast between old and new expectations for what students with significant cognitive disabilities should know and be able to do by the end of fifth grade. Note that across all three subjects, students with significant cognitive disabilities are expected to engage in higher thinking and operations to accomplish more complex academic content.
TABLE 1. Shifts in academic expectations for students with the most significant cognitive disabilities under NCLB-era standards and current academic content standards, grade 5 examples.
Peer review guidance based on the 2015 reauthorization of Elementary and Secondary Education Act (USDE 2018) holds all academic assessments to the expectation that they be aligned to “the depth and breadth of the State’s academic content standards” (USDE 2018, 36). Recommended evidence for alternate assessments highlights the importance of cognitive complexity in new assessments based on college and career readiness content standards (USDE 2018, 37–38), including:
• Test blueprints that reflect content linked to the State’s grade-level academic content standards and the intended breadth and cognitive complexity of the AA-AAS;
• Description of the breadth of the grade-level academic content standards the assessments are designed to measure, such as an evidence-based rationale for the reduced breadth within each grade and/or comparison of intended content compared to grade-level academic content standards;
• Description of the strategies the State used to ensure that the cognitive complexity of the AA-AAS is appropriately challenging for students with the most significant cognitive disabilities; and
• Description of how linkage to different content across grades/grade spans and vertical articulation of academic expectations for students is maintained.
Given the new requirements for AA-AAS to be based on more rigorous expectations, the new generation of AA-AAS would require a cognitive taxonomy that could describe the full range of cognitive processes expected for the heterogeneous population of students with significant cognitive disabilities.
The new DLM Cognitive Processing Dimension Taxonomy (DLM CPD Taxonomy) was designed to overcome the limitations of the LAL DOK taxonomy, detect more incremental changes over time, and be based on contemporary research on cognitive processing for the full range of students with significant cognitive disabilities. The DLM CPD Taxonomy was created with specific attention to expanding other taxonomies to capture cognitive processes that are not intentional without losing the higher order processes. To accomplish this goal, several sources of information were integrated including the research literature tracking early symbolic language and conceptual development among students with the most complex multiple disabilities including combined vision and hearing loss (e.g., Stremel-Campbell and Matthews, 1988; Rowland and Schweigert, 2000).
Approximately 4% of students with the most significant cognitive disabilities are reported to interact with the environment and other people at a pre-intentional level (Erickson and Geist 2016). Cognitive processing for this group of students is often dependent on perception and experience (Chard and Bouvard, 2014; 2013). These pre-intentional, perceptually-focused cognitive processes are difficult for others to observe, understand, and acknowledge (Bruce and Borders, 2015), but perception is important as a specific, implicit type of processing and learning (Fahle and Poggio, 2002). Cognitive processes at the perceptual level can be pre-intentional or intentional. For example, perceptual processing helps students with the most complex, multiple disabilities including deafblindness begin to attend jointly and intentionally to other people and objects (Bruce 2005). After this intentional, joint attention is established, perceptual processing supports learners in differentiating objects, sustaining thought about objects, and associating representations with objects, which all leads to the development of language (Bloom, 1993). These multiple levels of perceptual processing include processes such as attention, visual discrimination, and categorization, which all lead to higher cognitive processes involving working memory, long-term memory, action planning, and decision making (Chard and Bouvard, 2014; 2013).
As students with significant cognitive disabilities begin to develop intentional and then symbolic communication skills, cognitive processing becomes easier to observe and understand (Bruce and Borders, 2015). Further, symbolic communication, or expressive language, accelerates the development of other cognitive processes (Scholnick 2002), but there are known differences or limitations in the ways students with significant disabilities engage in at least some cognitive processes. For example, many students with intellectual disabilities have limited short-term memory capacity and impairments in general knowledge (including long-term memory) and comprehension but relative strengths in visual-spatial thinking and auditory processing (Bergeron and Floyd, 2006). However, among individual students, there is not a single profile of strengths and weaknesses, rather their profiles are diverse (Bergeron and Floyd, 2006).
The DLM CPD Taxonomy was developed to capture the full range of cognitive processing and diversity found among the population of students with the most significant cognitive disabilities. Given that cognitive development begins at birth (e.g., Carey, 2009) and many students with the most significant cognitive disabilities exclusively exhibit pre-intentional cognitive processing and communication (Erickson and Geist 2016), the DLM Consortium believed it was theoretically possible to create a system that differentially captured the earliest levels of cognitive processing in a more fine-grained manner than had previously been attempted. Drawing heavily upon the research on the move from pre-intentional to intentional communication among children with and without disabilities (e.g., McLean, 1990; Yoder et al., 2001) the DLM CPD Taxonomy was created to include several levels of cognitive processing that have been noted before students acquire symbolic communication and can be inferred based on subtle changes in behavior states among students with extremely limited response modes (e.g., Cress et al., 2007).
DLM developed the DLM CPD Taxonomy for the cognitive domain with 10 ordinal levels of cognitive processing to characterize cognitive processes associated with content standards, assessments, and related instruction appropriate for the diversity of students with the most significant cognitive disabilities. The taxonomy describes the cognitive dimension, in the context of expressing academic knowledge, skills, and understandings (KSUs). The taxonomy is based on a view of CPD as one aspect of cognitive complexity, where complexity may vary by the number and range of cognitive processes needed to complete tasks (Burleson and Caplan, 1998) and, as seen in other cognitive taxonomies described above, complexity varies by context.
The DLM CPD Taxonomy expands revised Bloom’s taxonomy but incorporates and builds on LAL’s downward extension. It is defined as expanded because it integrates several research-based taxonomies and captures a much broader range of processes than any other taxonomy. It begins at the pre-intentional level to capture the early, perceptual processing that is characteristic of up to 4% of students with significant cognitive disabilities (Erickson and Geist 2016). The next level, attend, is consistent with the LAL model, but its definition was clarified to reflect the role of attention in cognitive processing for children with and without disabilities (Columbo 2013) and captures student-initiated individual and joint attention to objects and people. Joint attention that occurs in response to bids from others is captured in the DLM expanded taxonomy at the third level, which is labeled respond. The level labeled replicate was included to capture rote skills (e.g., rote counting) or demonstration of skills acquired through massed trials or repetition. As illustrated in Table 2, the upper end of the expanded DLM CPD Taxonomy was not collapsed as in LAL, but rather reflects the revised Bloom’s taxonomy in order to reflect the full range of processes included in the DLM learning map models. This adjustment makes it possible to use the expanded taxonomy in the development of appropriately challenging assessments for all students, including students with significant cognitive disabilities, as expectations for what students can learn change over time.
The Dynamic Learning Maps Alternate Assessment System made a shift away from thinking about academic content for AAs as a series of discrete, measurable, observable skills toward defining academic content for AAs in terms of conceptual understandings and cognitive dimensions. Using a map-based model of academic domains that is organized by cognitive complexity, DLM has successfully made this move away from viewing academic content for students with the most significant cognitive disabilities as a series of skills and behaviors to master sequentially and toward a nuanced view of academic content that focuses on the cognitive dimensions of thinking and learning across academic domains. This approach supported the consortium in providing the types of peer review evidence required to demonstrate coverage of the range of complexity of academic content standards. The DLM assessment system is based on a content structure (Figure 2) that extends the grade-level expectations from college and career ready standards to Essential Elements that describe the grade-level expectations for students with significant cognitive disabilities. The Essential Elements are located in large, fine-grained learning maps that span from preacademic to high school knowledge, skills, and understandings. Nodes in the map that align with each Essential Element make up the Target linkage level. Additional groups of nodes are identified before and after each Target level node, or group of nodes, to provide multiple access points at different levels of complexity for each Essential Element. Items are written to align to nodes at one linkage level. This structure is significantly different than that of general education assessments, which move directly from grade level standards to items. DLM allows for two opportunities to provide an increased range of content complexity for students with significant cognitive disabilities: 1) reducing the complexity of grade level standards by Essential Elements, and 2) creating a range of items at different levels of content complexity through identifying nodes in the map that are specified as Linkage Levels for each Essential Element. This content structure and applications of the DLM CPD taxonomy are described in more detail in the next sections.
FIGURE 2. Content relationships in the DLM assessment system.Source: DLM Consortium, 2016. Numbers indicate relationships evaluated in alignment studies. Linkage level abbreviations: initial precursor—IP, distal precursor—DP, proximal precursor—PP, target—T, successor—S.
The DLM Consortium took the position that standards, assessments, and instruction need to be rigorous and aligned. Since students with the most significant cognitive disabilities are working toward grade level content standards within a continuum of multiple measurable steps, the continuum needed to be defined. In addition, since these students often took different pathways toward the end-of-year learning targets, a single task-analyzed progression of skills would not be inclusive of all students’ learning and would most certainly introduce barriers for students with different learning profiles. DLM state partners made an important decision that the descriptions of expectations for student learning (i.e., grade level content standards or extended standards) were not sufficient to capture the within-year academic progress of these students, often accomplished slowly in small steps. Thus, learning map models were developed to show how students progressed toward grade level alternate standards, called the DLM Essential Elements (EEs).
Learning map models provide the basis for the DLM Alternate Assessment System (Kingston et al., 2016). Initially developed for English language arts and mathematics and later for science, the learning map models are derived from cognitive science and theories of learning and represent hypotheses about how learning progresses for most students (Romine et al., 2018). Cognitive learning maps, such as the DLM learning map models, are organized learning models that establish a foundation for the design of instructionally sensitive assessments for students in special populations that provide more useful and valid data about students’ progress in learning at the classroom, school, district, and state levels (Bechard et al., 2012). This approach has subsequently been expanded to include students instructed in grade-level general academic content standards (See https://enhancedlearningmaps.org/About).
Focusing on the grade level expectations in general education grade level content standards and the EEs linked to them in grades K-12, DLM constructed fine-grained organized learning models or maps representing multiple progressions between KSUs, resulting in a hypothesized framework of cognitive skills (nodes) and the relationships between them (connections) that is applicable to all students. To be included in the map, each node must be essential, unique, observable, and testable (Dynamic Learning Maps Consortium, 2016). The connections between nodes in the model are acyclic. Precursor skills precede mastery of a learning target, but throughout the map model, there are multiple pathways leading to learning targets. Together, the skills and their prerequisite connections map out the progression of learning within a given domain (Bechard et al., 2019, 188–205). For example, the DLM ELA map used for the assessment system that became operational in 2015 includes more than 1919 nodes representing cognitive KSUs ranging from foundational skills to high school-level expectations for students without disabilities. The ELA map has more than 5,045 connections between nodes.
The EEs in the DLM assessments are aligned to the grade-level CCRS but at reduced depth, breadth, and complexity. These grade-specific EEs are embedded within the maps. From the range of EEs developed to guide instruction, state partners prioritized certain EEs as foci for assessment. DLM alternate assessments are designed to measure academic KSUs aligned with these target EEs and at multiple levels of complexity associated with each EE. This allows the full range of students with the most significant cognitive disabilities to demonstrate their academic knowledge related to the EE at a level of complexity that balances rigor and access. The nodes selected as assessment targets sample the academic content at five levels of complexity in ELA and mathematics and three levels of complexity in science. Figure 3 shows a map section that articulates this progression for five levels of cognitive complexity for an ELA EE on vocabulary use in writing.
FIGURE 3. ELA map section showing five assessed levels of cognitive complexity for the grade 8 EE: Use domain specific vocabulary related to the topic (ELA.EE.W.8.2.d). Rectangles indicate tested nodes. Linkage level abbreviations:, initial precursor—IP, distal precursor—DP, proximal precursor—PP, target—T, successor—S, and untested—UN.
The DLM assessment system is organized into testlets (short assessments with three to eight items and an engagement activity). There are testlets at each linkage level for every EE on the blueprint. Within each testlet, items are written to align to one or more nodes in the linkage level. A student takes one testlet per EE, at the appropriate linkage level (i.e., the most challenging level where they are still likely to be able to demonstrate knowledge of academic content related to the EE). A student may test at different linkage levels for different EEs as they complete the assessment. Assessment results reported at the student level include student mastery of all linkage levels for each tested Essential Element, aggregated information about a student’s mastery of groups of Essential Elements called conceptual areas, and overall achievement in the subject.
Given the grain size and span of DLM’s learning map models and assessment targets, the DLM CPD Taxonomy with its expanded range of levels of complexity was applied to units within the map model and to the assessment, while also being used for a variety of purposes during the assessment design, development, and evaluation phases (including but not limited to alignment studies). The taxonomy would also inform the design of aligned professional development and instructional resources.
The DLM Consortium anchors the whole assessment system, including professional development, in the structure of the learning map models. Like other taxonomies, the DLM CPD Taxonomy provides one dimension for categorizing the content in the DLM assessment system. However, unlike other taxonomies, because DLM content and cognitive process are integrated through organized learning models, the cognitive process dimension is not intended to be interpreted in isolation of the content. This integration is evident at levels ranging from the nodes to score reports. Each node specifies what a student must do cognitively with given academic content. For example, “use domain-specific vocabulary in informative writing” requires the student to remember relevant vocabulary, understand its use in writing, and apply it in the context of a written message that informs the reader. A student score report describes the student’s skills in the conceptual areas of “using writing to communicate” and “integrating ideas and information in writing.”
The taxonomy plays an important but supporting role; it is not the primary lens for describing the content. For example, in the consortium’s theory of action, one of the claims is that “the combination of testlets administered throughout the year measure knowledge and skills at the appropriate breadth, depth and complexity” (Clark and Karvonen 2020, 51). As described in the next section, the CPD Taxonomy supports development and evaluation of assessments to ensure they support that claim.
Cognitive processes are dealt with differently across parts of the DLM assessment system, which encompasses the maps, assessments, and aligned professional development and instructional supports. Sometimes the DLM CPD Taxonomy is used explicitly, and at other times it is implicit within broader consideration of cognitive complexity (see Table 3). Implicit use means that developers rely on their general understanding of the taxonomy as one schema to guide their decisions. The DLM CPD Taxonomy is implicit when evaluating drafts of the maps, during EE development, and when selecting nodes for linkage levels. For explicit use, elements of the DLM assessment system are labeled with a DLM CPD level. The DLM CPD Taxonomy is explicitly used in map development, assessment design and development, and alignment evaluation. It is also used explicitly in the development of instructional supports that target various cognitive processes and promote progress toward higher levels and to create professional development. The taxonomy is implicit from the perspective of educators who complete the professional development.
As described previously, the learning map models are carefully constructed using a synthesis of research on student learning across academic domains. The DLM CPD Taxonomy is considered during map development as developers organize content for increasing cognitive complexity based on the literature. Map developers use verbs that explicate the DLM CPD Taxonomy levels (e.g., identify, describe, evaluate) for groups of nodes that represent the same component in a subject (e.g., key details or main idea in English language arts). Nodes in the group represents different levels of the DLM CPD Taxonomy. For example, in Figure 3, students recognize, then select, then use domain-specific vocabulary. The larger maps cycle through various combinations of the DLM CPD Taxonomy levels as they depict more complex content. In Figure 3, a student would use abstract words before recognizing domain-specific vocabulary. “Use” is equivalent to Apply in the CPD Taxonomy and “recognize” is associated with Remember. It is the difference in academic content in the two nodes that makes the directionality of the pathway make sense.
External review of sections of the maps relies on the broader notion of cognitive complexity. During this process, draft sections of maps are externally reviewed by educator panels (Romine et al., 2018). Panelists review the content of nodes and connections between the nodes using criteria related to content and accessibility. Content panelists use their judgment about cognitive complexity when they determine whether a node size is appropriate and whether the connections between criteria are accurate. Accessibility panelists judge whether each connection represents an appropriate learning sequence for all students.
EEs were first developed using educator panel judgments on how to reduce the complexity in the expectations in CCRS to an appropriate level of rigor for students with significant cognitive disabilities. Panelists were encouraged to ensure increasing complexity across grades in the drafted EEs to retain the highest possible expectations (DLM Consortium, 2016). During the process of aligning EEs to the ELA and mathematics maps, staff were able to confirm that EEs increased in complexity across grades by noting the location of the aligned nodes within the maps. For example, the nodes aligned to a fourth grade EE were precursors to the nodes for a related fifth grade EE.
When developing revised science EEs based on multidimensional science performance expectations in the NGSS, panels followed a process in which they considered each dimension separately and reduced complexity on each dimension before constructing the multidimensional EE. The external review process included a step in which the panel evaluates EEs across grades to ensure there is a vertical progression of the complexity of expectations (Koebley et al., 2020).
Nodes were identified as assessment targets in three to five linkage levels, depending on the subject (see example of five ELA linkage levels in Figure 3). Once the target level nodes were identified in the map, staff used a systematic process to identify assessment targets for the other linkage levels. Staff identified nodes that represented critical junctures on the pathway toward the target (DLM Consortium, 2016), or, for the successor level, provided an opportunity for the student to stretch toward the grade-level standard for students without significant cognitive disabilities. The map structure informed judgments about what constituted a critical juncture. For example, a node with many incoming or outgoing connections was often deemed an especially important precursor or critical juncture on the way to the target KSU. Figure 3 also illustrates that there are untested nodes (UN) between the linkage levels.
Student responses to items at different linkage levels provide empirical evidence of increasing complexity across linkage levels within an EE, as items are more difficult at higher linkage levels (Clark et al., 2014). This approach to empirical analyses is appropriate for localized evaluations but is not scalable to larger sections of the map. Other work is underway to develop methods for empirically evaluating map structure using diagnostic classification models (Thompson and Nash, 2019).
The DLM Consortium uses a unique approach to combining evidence-centered design and Universal Design for Learning (UDL) to create Essential Element Concept Maps (EECMs) to guide assessment development (Bechard et al., 2019). The EECM is a graphic organizer that incorporates some information commonly found in task templates and test specifications along with information based on UDL guidelines and content-specific information about accessibility for the full range of students with significant cognitive disabilities. The EECM supports item writers in developing testlets for each linkage level that are accessible to the population, free from sources of construct-irrelevant variance, and well-aligned to the intended nodes.
The original version of DLM EECMs dealt with cognitive complexity implicitly, by helping the item writer hone in on the expectations for a linkage level and distinguish between the expectations across linkage levels. The information provided for each node included a brief description of the node and the node observation, which is a longer description of how a student would demonstrate the KSU in the node during an assessment. The EECM also provided key vocabulary for each linkage level and node-specific misconceptions a student might have (DLM Consortium, 2016). The item writer would use the EECM to develop a testlet for a single linkage level but could also refer to the mini-map (Figure 3) and the EECM information at other linkage levels to clarify the assessment targets for the focal linkage level. The original EECMs specified a range of the number of items to be written for the linkage level.
The EECM for DLM science assessments is different from the EECMs for ELA and mathematics in a few ways. Science EECMs include information about each dimension in the multidimensional science EE and information for each of three linkage levels. Items within the testlet can be written to align to some part of the linkage level as long as the combination of items within the testlet cover the content in the linkage level statement. A planned improvement to the science EECM is to add a range of expected cognitive processes, using the DLM CPD Taxonomy, for each linkage level.
The DLM assessment system is computer-based, but there are two types of testlets: computer-administered and teacher-administered. Some linkage levels are intended to be measured by testlets in which the student interacts directly with on-screen content. In these testlets, each item can be identified based on its cognitive process using the DLM CPD Taxonomy. The choice of testlet type is based on the linkage level assessed. For example, in Figure 4, the Proximal Precursor, Target, and Successor linkage levels related to sixth grade ELA Essential Element Reading Literature (Identify details in a text that are related to the theme or central idea) are answered by the student directly. In teacher-administered testlets, the teacher follows on-screen instructions that guide teacher-student interactions. These are the Initial Precursor and the Distal Precursor linkage levels shown in Figure 4. The teacher administers items and records the student’s response in the online system. In these testlets, the DLM CPD Taxonomy is used in two ways. First, each item can be identified for its cognitive process, the same way it is for computer-administered testlets. Second, when the teacher-administered testlet is used at the Initial Precursor linkage level to assess students operating at the lowest levels of the taxonomy, response options address different levels of the DLM CPD Taxonomy. An example of response options and their related relationships to the lowest levels of the DLM CPD Taxonomy are shown in an item for a teacher-administered testlet (Figure 5) for the same Essential Element shown in Figure 4.
FIGURE 5. Example Initial Precursor item for the node “Can match a real object with a picture or other symbolic representation of the object.” Response options represent levels in the CPD taxonomy.
Given this unique approach to incorporating the CPD levels in the student response rubric, test development staff take the extra step to develop testlet templates that serve as the starting point for item writers developing initial precursor testlets.
The DLM CPD Taxonomy is used explicitly through the item writing cycle, beginning with item writer training and continuing through internal and external review processes before items are field tested. For this purpose, the generic DLM CPD Taxonomy definitions have been interpreted for English Language Arts (ELA), Mathematics, and Science. Resources include the generic DLM CPD Taxonomy definition with content-specific examples (see Table 4). These examples extend beyond generic lists of verbs and illustrate the cognitive process in the context of the academic content. The purpose is to inform item writers with content-specific examples to help them assign a CPD level to each item after it is written. Unlike test development models in which item writers are assigned to create items at several cognitive levels for the same content (e.g., with the goal of covering Webb’s DOK levels 1–3), in the DLM model CPD levels are automatically constrained by the combination of content and cognitive process in the nodes to which items are aligned. The intent is not to write an item for each level of the taxonomy.
DLM item writers are educators who are trained on using the DLM CPD Taxonomy prior to beginning their work. They are asked to make a judgment on the complexity of the items they write. The DLM CPD Taxonomy levels they assign are included as metadata when items are entered in the online content management system. When identifying the item’s DLM CPD Taxonomy level, item writers are asked to use a holistic approach by considering the item, the response elicited by the item, and the skill described by the elicited response. If they cannot decide between two levels, they are advised to select the lower level. Item writers peer review each other’s draft testlets and are instructed to evaluate the accuracy of the assigned DLM CPD Taxonomy level for each item.
Once testlets are written, they are internally reviewed by staff or consultants with expertise in the academic content and in special education. These experts check the DLM CPD Taxonomy levels assigned to each item by the item writer, among other criteria. All guidance to internal reviewers in all three subjects contains the direction to refer to the DLM CPD Taxonomy and ensure that “the item has the appropriate CPD level listed” (DLM, 2018a; DLM, 2018b). DLM test development staff complete a final check for the accuracy of the DLM CPD Taxonomy level while processing feedback from the internal reviewers.
Once new testlets have passed all internal steps, they are ready for external review. The consortium facilitates external review events on an annual basis. Panelists may include local educators, state education agency staff, and other stakeholders. The panelists review for content, accessibility, and bias/sensitivity and use different criteria, for both individual items and testlets as a whole (Clark et al., 2016). The Content Review Panel evaluates the DLM CPD Taxonomy level assigned to each item for correspondence with the associated node. Test development staff use the combination of panel recommendations to accept, revise, or reject items and testlets to make decisions before advancing testlets for field testing.
The internal and external review steps described above are ways of monitoring and promoting alignment during the test development process. The DLM Consortium relies on external alignment studies to obtain unbiased evaluations of the degree to which the assessments are aligned as intended. Given the design of DLM assessments, alignment evaluation consists of the following relationships:
1) General education standards to EEs
2) EE to Target level node(s)
3) Vertical articulation of linkage levels associated with an EE
4) Items to nodes (or in science, items to linkage levels)
These relationships are illustrated by the numbered pathways in Figure 2, as portrayed for an alignment study conducted in 2016 for ELA and mathematics.
The DLM alignment studies were conducted using a subset of the criteria from LAL, with the methodology adapted for the DLM assessment design. For example, LAL’s modification of the Achieve performance centrality criterion was used to evaluate whether DLM accomplished its intended goals for the relationship between performance expectations in foci 1, 2, and 4. For study focus 3, panelists evaluated the series of linkage levels for each EE and determined whether there was an acceptable progression as defined by one of two conditions: a) there is an appropriate increase in the cognitive complexity of the skills described by the nodes assigned to the linkage levels; or b) a node or nodes at a lower linkage level represent clear prerequisite knowledge or skills for a node or nodes at a higher linkage level. In focus 4, the DLM CPD Taxonomy instead of the LAL DOK taxonomy was used to address the alignment evaluation question, Do the CPD levels in the assessment item reflect a full range of CPD levels and include challenging academic expectations for students with significant cognitive disabilities? Panelists determined whether they agreed with the DLM CPD Taxonomy level assigned by the item writer and confirmed by internal staff or experts. If they did not, they recommended a more appropriate level. When more than one cognitive process was required to answer an item, panelists rated based on the highest level evident in the item.
In ELA, most items were classified as having CPD levels ranging from respond to understand, although there were a few items at the attend level and some at the apply or analyze levels. In mathematics, most items were at the remember through analyze levels although some items in lower grades were rated attend or replicate and some items in upper grades extended to the evaluate or create levels (See Table 5).
The EECM graphic organizers used for item development are being revised to create Essential Element Instructional Concept Maps (EEICMs) for use in the development of instructional routines aligned with the DLM alternate assessments. This work will take a routines-based approach so that teachers have the flexibility to address content that matches the local curriculum while targeting collections of nodes and levels of CPD that align with the DLM blueprints. The EEICMs call out the range of levels from the DLM CPD Taxonomy to be addressed in instruction targeting each linkage level aligned with each EE in the DLM blueprints. The EEICMs also specifically call out the larger conceptual area that must be addressed to help students develop the full range of KSUs intended at each grade level, in each academic domain. Content development events will bring together teachers and content-area experts who will make explicit use of the levels of DLM CPD Taxonomy as part of the EEICMs to create instructional content; however, when teachers access and use the resulting content, there will not be explicit references to the taxonomy or the EEICMs.
Teachers also encounter implicit and explicit reference to CPD in the professional development that is part of the DLM Alternate Assessment System. Throughout a comprehensive set of 54 modules that focus on instruction aligned with the DLM alternate assessments, guidance is provided to help teachers deliver instruction that addresses the full range of cognitive processes reflected in the DLM CPD Taxonomy as well as the range of linkage levels aligned with the EEs. In some cases, there are more explicit references to CPD level. For example, in a module addressing beginning communicators, teachers are explicitly taught to look for and respond to pre-intentional forms of communication or perceptual processing in order to help students begin to attend and respond during academic instruction. Teachers are also explicitly supported in helping students develop more complex CPD levels through expanded descriptions of nodes aligned with the two lowest linkage levels (i.e., initial precursor and distal precursor) aligned with each EE. This implicit and explicit reference to the DLM CPD Taxonomy in the professional development system helps keep a focus toward more comprehensive views of KSUs, cognitive processing, and conceptual development and away from teaching mastery of isolated skills.
Cognitive classification systems were initially developed by Benjamin Bloom in the 1950s to facilitate test development by carefully defining a framework of educational objectives into which items measuring the same objective could be classified. In 2001, the No Child Left Behind Act supported standards-based educational reform, instituting the idea of a coherent system of standards, assessments, and instruction. Examining the coherence or alignment of the system’s components required metrics that could evaluate the relative difficulty of the expectations for students articulated by the three components. Cognitive processing taxonomies were created, including a revision of Bloom’s, for this purpose. These taxonomies generally described a hierarchical relationship of levels of cognitive processing, beginning with a level variously labeled as recall, retrieval, and remembering. At the same time, federal legislation also required that all students, including those with the most significant cognitive disabilities, be included in the standards-based assessments used for accountability purposes. The DLM Alternate Assessment System was created to serve this purpose. Given the characteristics of the target population and the heterogeneity of students identified in this group, it was apparent that a “one-size-fits-all” approach would not meet the needs of these students or the demands of the legislation. As a result, DLM learning map models were created to identify the foundational basis for all academic learning and the small, fine-grained steps necessary for developing conceptual understanding of academic content at increasing levels of sophistication and complexity via multiple pathways. This organized learning model required a new tool to describe the cognitive processes inherent in earliest stages of development. The DLM CPD Taxonomy was articulated based on research from early childhood and disability literature. The taxonomy is used to inform EEs and linkage level development in the DLM Alternate Assessment System. It is also employed explicitly in map, item and testlet development, and formal alignment studies, as well as implicitly and explicitly for professional development and designing instructional supports.
The DLM CPD Taxonomy provides the means to promote cohesion within the Alternate Assessment System and support a continuum of learning and assessment for the full range of learners with significant cognitive disabilities. Although the DLM CPD Taxonomy was more useful than past taxonomies would have been, there have been both opportunities and challenges in its use.
The DLM maps, EEs, and linkage levels were developed and evaluated using expert, staff, and educator judgments through many levels of review including evaluations of the relative cognitive complexity of each component. Empirical evaluation of the map structure is still in the very early stages (Thompson and Nash, 2019). The most sophisticated approaches to empirical evaluation are based on diagnostic classification models (DCM). In research, these approaches are traditionally applied to small groups of related skills and rely on large numbers of student responses to items measuring all of the skills. This approach could provide evidence that related content in the map, differing primarily in the cognitive process applied, is actually ordered appropriately. Unfortunately, the DLM assessment system design leads to sparsely populated data that limits the use of DCM for map validation. For example, students only test on one linkage level per EE, limiting the options for statistical evaluation of the vertical relationship between linkage levels. Until there are sufficient data for DCM approaches, the CPD taxonomy could be used in other studies to evaluate the ordering within localized clusters of nodes. For example, are items based on higher CPD levels more difficult than those at lower levels, within the same academic content? If future empirical evidence suggests different nodes or connections than the maps currently hypothesize, there could be implications for future revisions to EEs, linkage levels, or the maps themselves.
The DLM CPD Taxonomy also has potential for use in other assessment design research. For example, in cognitive labs evaluating students’ interactions with items (e.g., Karvonen et al., 2020), the items could be selected to include a range of CPD levels and student response data analyzed for evidence of use of the intended CPD. Furthermore, such cognitive labs would provide an opportunity for students to engage in think aloud processes that may reveal more about their response and approach to selecting a response than is possible through traditional item analysis.
The DLM CPD Taxonomy was essential for designing assessments that provide opportunities for the full continuum of students with significant cognitive disabilities to demonstrate what they know and can do on the assessment. From an assessment policy perspective, this inclusive approach eliminated the need to use discontinuation rules that assume a student without symbolic communication cannot demonstrate any knowledge on the assessment. Administering the full assessment using teacher-administered testlets provides more opportunities for students with inconsistent intentional responses or pre-symbolic means of communication to demonstrate a response and helps teachers learn to look for increasingly complex levels of processing. Offering teachers professional development that explicitly helps them look for pre-intentional cognitive processes and build on them through predictable instructional routines such as shared reading further supports the inclusive nature of the assessment.
Students’ correct responses to teacher-administered items requires students to demonstrate a CPD level of attend. However, the rubrics teachers use to record student interaction during the item administration reflect CPD levels extending down to the pre-intentional level. This design provides teachers with immediately actionable information they can take back into instruction, and it could support future score reports with more explicit diagnostic information about students’ incremental progress in their level of cognitive processing and expressive communication.
The DLM CPD Taxonomy is a useful framework for item development and alignment evaluation. With the support of EECMs that reflect the full scope of concepts to be addressed in items, the taxonomy helps item writers construct items that accurately tap levels of cognitive processing that align with the intended EE and linkage level. Initial evidence of the success of this approach has led us to the upcoming modification of the EECMs to include a range of expected CPD levels for a node or linkage level. As we move forward, we will evaluate the impact of this upcoming EECM modification on the quality and alignment of newly developed items. The clearly articulated taxonomy supports multiple levels of review of the intended CPD level before the item is released for use.
Subsequently, the success of the item development approach can be checked empirically via alignment studies. Using the DLM CPD Taxonomy, rather than other taxonomies commonly used to evaluate alignment on the basis of cognitive process, had predictable trade-offs. A key benefit was that the results were more interpretable and supported evaluation of the item bank based on the same conceptualization of cognitive processing used during assessment development. The alternative (e.g., using Webb’s 4-point DOK or the original LAL DOK) would have restricted the range of possible ratings and resulted in lost information when trying to evaluate whether the assessments reflect a full range of CPD levels and include challenging academic expectations for all students. However, the wider range of levels on the DLM CPD Taxonomy provides more room for variability in ratings and requires more training on the distinctions between the levels. Interrater agreement statistics also need to be based on exact plus adjacent ratings to account for the range of possible values panelists could assign. Although it is common in alignment methodologies to evaluate content and cognitive process as separate dimensions, there is a risk of inaccurate ratings or incorrect interpretation of results based solely on the cognitive process dimension. An alignment methodology that evaluates relationships based on a broader view of cognitive complexity and where ratings are based on cognitive process in the context of the content, might be more appropriate. Redefining the role of the CPD in alignment evaluation could be part of a larger agenda in advancing alignment methodologies (Polikoff 2020).
Cognitive taxonomies were originally designed to support instruction and assessment. However, we intentionally have not brought the DLM CPD Taxonomy to the forefront in professional development for teachers. We were concerned about the potential pitfall of teachers learning to target instruction at a specific level based on nodes in the map models, linkage levels, or the taxonomy itself. We feared this would limit instruction based to a single level of processing, a single skill, or a narrowly defined learning target, which would all serve to lower expectations and reduce learning opportunities for the students. As such, the DLM CPD Taxonomy generally operates implicitly in the background, informing the design of instructional supports to prevent this outcome. The exception to this principle is the more explicit reference to the taxonomy in professional development and instructional supports that relates to students operating at the lowest levels of CPD. We made the choice to explicitly reference the lowest levels of the DLM CPD Taxonomy in professional development to help teachers move students operating at a pre-intentional level toward more intentional processes of attending and responding on their way to symbolic communication. Other assessment systems seek to move these students directly into symbolic communication (e.g., Kleinert et al., 2016) without intentionally addressing the levels of understanding and processing that can ultimately support such development.
Our work on EEICMs is the newest application of the DLM CPD Taxonomy in instruction, and the work is still underway. However, we believe that the inclusion of CPD in this work will help continue the movement toward instruction that is aligned with grade level standards, appropriate for each student, and designed and delivered in such a way that it results in the development of KSUs that meaningfully support progress from year to year. Taxonomies that start too high do not provide an opportunity for the full range of students with significant cognitive disabilities to demonstrate more complex levels of processing, and they fail to help teachers understand the role they play in helping students more through pre-intentional to intentional levels of cognitive processing.
The DLM CPD Taxonomy describes a wide range of cognitive processes but alone is insufficient for describing cognitive complexity. Cognitive processing in the context of academic content provides a better path toward characterizing cognitive complexity. While the field continues to develop new ways of operationally defining cognitive complexity differentiated for academic content (e.g., Achieve, 2019a; Achieve, 2019b; Achieve, 2019c), these definitions limit access for students with significant cognitive disabilities by setting a floor based on assumptions grounded in the literature on typical cognitive development. The DLM CPD Taxonomy based on the cognitive dimension provides one illustration of how to remove the floor.
The DLM CPD Taxonomy arguably incorporates a communication dimension at the lower end, which supports students reaching levels at which they can use symbolic communication to demonstrate higher-order thinking. Communication might be better positioned as a dimension of its own when considering the profiles of students with the most significant cognitive disabilities. If, as asserted by Webb, how deeply students understand and are aware of learning requires them to explain answers and communicate solutions, communication is a critical dimension of advanced levels of cognitive processing. Rather than considering symbolic communication as part of the lowest levels of the single dimension currently reflected in the DLM CPD Taxonomy, students with significant cognitive disabilities may be best served by a CPD that specifically addresses expressive communication development from pre-intentional levels to the advanced levels required to explain answers and communicate solutions.
Models of cognition for typically developing children are not inclusive of students with significant cognitive disabilities. For example, working memory is essential to complete tasks (Jensen 1998) but challenges with working memory are common for students with significant cognitive disabilities (Saeed and Tahir, 2016). Another potentially useful expansion of the DLM CPD Taxonomy would be a dimension reflecting working memory and other forms of metacognition. It would be important to include metacognition, currently treated as a separate system (along with the self-system and cognitive system) in Marzano’s taxonomy and as a type of knowledge used in cognition (along with factual, conceptual, and procedural) in revised Bloom’s taxonomy. Metacognition plays an important role in completing more complex mental tasks (Zelazo and Douglas, 1997). There are various theories about the best positioning of metacognition in models of cognition (Irvine, 2017), so further discussion about this dimension would be necessary for it to be meaningful and inclusive of all students.
Assuming prerequisite cognitive skills before considering instruction that targets metacognition might introduce new barriers for students with significant cognitive disabilities. The concepts of metacognition might have to be extended to include earlier forms of cognitive engagement that can lead to metacognition, which could also be put into an organized learning model. Perhaps this would constitute yet another dimension or a new map model that addresses metacognition as an element that intersects with all other cognitive processes. Strategies to develop metacognitive skills such as self-regulation are part of academic instructional models for students with high-incidence disabilities (e.g., Wehmeyer et al., 2012; What Works Clearinghouse 2017) but are only recently being extended to students with significant cognitive disabilities.
Thinking of metacognition along a continuum rather than as a higher level in a cognitive taxonomy also can be advanced through the lens of Universal Design for Learning (UDL). For example, the CAST UDL 2.0 guidelines include multiple guidelines and checkpoints for building student engagement, including options for recruiting interest, sustaining effort, and self-regulation (CAST, 2018). Designing instruction and assessment that integrates the affective domain can provide pathways for students with significant cognitive disabilities to develop self-regulation even while operating at lower levels on the DLM CPD Taxonomy. The pitfall would be viewing the CPD Taxonomy as rigidly hierarchical—for example, deciding that a student who is learning to “replicate” should only receive instruction that targets that cognitive process, may mean the student never has the opportunity to develop higher levels of engagement.
While there are two-dimensional learning models that include a well-defined psychomotor domain (e.g., Marzano and Kendall, 2007), we choose not to emphasize it because it risks limiting students with significant cognitive disabilities (so many of whom have challenges in this area). Students with significant cognitive disabilities can demonstrate their knowledge, skills and understandings without good eye-hand coordination or quick response times. There is value in supporting psychomotor development for all learners, but there is a risk of privileging this type of skill development over academic learning if we include psychomotor procedures as a domain that intersects with cognitive processing for the purpose of academic instruction and assessment.
A unidimensional cognitive process taxonomy will always have its limits. As the fields of cognitive and learning sciences evolve, we may be able to move toward more complex and integrated views of cognitive complexity and both cognitive and noncognitive factors in learning. Key to the design of useful educational assessments is that they are based on models of student cognition that “represent the most scientifically credible understanding of typical ways in which learners represent knowledge and develop expertise in the domain being assessed” (Pellegrino et al., 2016, 63). Lessons learned from creating and using the DLM CPD Taxonomy can support the goal of developing better models of cognition that are inclusive of students with significant cognitive disabilities. The DLM CPD Taxonomy could be useful for people designing curricula to be inclusive of all learners and support students learning at their own rate.
Publicly available datasets were analyzed in this study. This data can be found here: https://dynamiclearningmaps.org.
SB is a senior advisor to the Accessible Teaching, Learning and Assessment Systems and a contributor to the development of the Dynamic Learning Maps Alternate Assessment System. She provides the history and theoretical context for the DLM CPD Taxonomy. MK is the director of ATLAS and the Dynamic Learning Maps Alternate Assessment System. She contributes information on the uses of the DLM CPD Taxonomy within the DLM maps-based assessment system for test development and alignment studies. KE is the director of the Center for Literacy and Disability Studies at UNC Chapel Hill and an associate director of professional development at ATLAS. Her research on literacy assessment and instruction for students with significant disabilities contributed to the development of the DLM CPD Taxonomy and its use in test design and professional development.
This paper was developed under grant 84.373X100001 from the U.S. Department of Education, Office of Special Education Programs.
The views expressed herein are solely those of the authors and no official endorsement by the U.S. Department of Education should be inferred.
The authors declare that the research was conducted in the absence of any commercial or financial relationships that could be construed as a potential conflict of interest.
The authors wish to thank Jonathan Schuster for his feedback on the concept of cognitive complexity.
Achieve, Inc. (2019c). A Framework to Evaluate Cognitive Complexity in Science Assessments. Washington, DC: Achieve, IncAvailable at: https://www.nextgenscience.org/sites/default/files/Science%20Cognitive%20Complexity%20Framework_Final_093019.pdf.
Achieve, Inc. (2018b). Criteria for Procuring and Evaluating High Quality and Aligned Summative Science Assessments. Washington, DC: Achieve, IncAvailable at: https://www.achieve.org/files/Criteria03202018.pdf.
Achieve, Inc. (2018a). Independent Analysis of the Alignment of the ACT to the Common Core State Standards. Washington, DC: Achieve, IncAvailable at: https://www.achieve.org/files/ACTReport.pdf.
Achieve, Inc. (2002). Measuring up: A Standards and Assessments Benchmarking Report for Oklahoma. Washington, DC: Achieve, IncAvailable at: https://www.achieve.org/files/OK_Benchmark.pdf.
Achieve, Inc. (2019a). “A Framework to Evaluate Cognitive Complexity in Mathematics Assessments.” Achieve.Org. Available at: https://www.achieve.org/cognitive-complexity-mathematics (Accessed December 11, 2020).
Achieve, Inc. (2019b). “A Framework to Evaluate Cognitive Complexity in Reading Assessments.” Achieve.Org. Available at: https://www.achieve.org/cognitive-complexity-reading (Accessed December 11, 2020).
Alonzo, A. C. (2018). An Argument for Formative Assessment with Science Learning Progressions. Appl. Meas. Education 31 (2), 104–112. doi:10.1080/08957347.2017.1408630
Anderson, L., and Krathwohl, D. R. (2001). A Taxonomy for Learning, Teaching, and Assessing: A Revision of Bloom’s Taxonomy of Educational Objectives. New York: Longmans.
Armstrong, P. (2020). “Bloom’s Taxonomy. ” Center for Teaching: Vanderbilt University. doi:10.1287/d7ebc808-cf95-43cd-b8a9-f56c6cc1858f Available at: https://cft.vanderbilt.edu/guides-sub-pages/blooms-taxonomy/.
Assistance to States for the Education of Children with Disabilities (2006). Assistance to States for the Education of Children with Disabilities, U.S.C. 20.1400
Aungst, G. (2014). “Using Webb's Depth of Knowledge to Increase Rigor.” EdutopiaLast Modified September 4. Available at: https://www.edutopia.org/blog/webbs-depth-knowledge-increase-rigor-gerald-aungst.
Bechard, S., Hess, K., Camacho, C., Russell, M., and Thomas, K. (2012). Why Should Cognitive Learning Models Be Used as the Foundation for Designing Next Generation Assessment Systems? White Paper No. 1.1 presented at SRI International Symposium: Understanding Learning Progressions and Learning Maps to Inform the Development of Assessment for Students in Special Populations. Menlo Park, CA, 2011.
Bechard, S., Clark, A., Swinburne Romine, R., Karvonen, M., Kingston, N., and Erickson, K. (2019). Use of Evidence-Centered Design to Develop Learning Maps-Based Assessments. Int. J. Test. 19 (2), 188–205. doi:10.1080/15305058.2018.1543310
Bergeron, R., and Floyd, R. G. (2006). Broad Cognitive Abilities of Children with Mental Retardation: An Analysis of Group and Individual Profiles. Am. J. Ment. Retard. 111, 417–432. doi:10.1352/0895-8017(2006)111[417:BCAOCW]2.0.CO;2
Bernard Paulais, M-A., Mazetto, C., Thiébaut, E., Nassif, M., Blanc, R., and Gattegno, M. P. (2019). Heterogeneities in Cognitive and Socio-Emotional Development in Children with Autism Spectrum Disorder and Severe Intellectual Disability as a Comorbidity. Front. Psychiatry 10, 508. doi:10.3389/fpsyt.2019.00508
Beuker, K. T., Rommelse, N. N. J., Donders, R., and Buitelaar, J. K. (2013). Development of Early Communication Skills in the First Two Years of Life. Infant Behav. Development 36 (1), 71–83. doi:10.1016/j.infbeh.2012.11.001
Blank, R. K. (2005). Surveys of Enacted Curriculum: Tools and Services to Assist Educators. Washington, D.C: Council of Chief State School OfficersAvailable at: https://eric.ed.gov/?id=ED484708.
Bloom, Benjamin. S., Engelhart, M. D., Furst, E. J., Hill, W. H., and Krathwohl, David. R. (1956). Taxonomy of Educational Objectives: The Classification of Educational Goals—Handbook I. New York, NY: Cognitive DomainDavid McKay.
Bloom, Lois. (1993). “The Transition to Language,”. In the Transition From Infancy To Language: Acquiring the Power Of Expression. Editor Lois. Bloom (Cambridge, MA: University of Cambridge), 79–109.
Bloom’s Taxonomy., () What Is Bloom’s Taxonomy?” BloomsTaxonomy.Net. Available at: https://www.bloomstaxonomy.net/.
Browder, D. M., Wood, L., Thompson, J., and Ribuffo, C. (2014). Evidence-based Practices For Students With Severe Disabilities (Document No. IC-3). CEEDAR CenterAvailable at: http://ceedar.education.ufl.edu/tools/innovation-configurations/.
Browder, D., Ahlgrim-Delzell, L., Spooner, F., Mims, P. J., and Baker, J. N. (2009). Using Time Delay to Teach Literacy to Students with Severe Developmental Disabilities. Exceptional Child. 75 (3), 343–364. doi:10.1177/001440290907500305
Browder, D. M., Spooner, F., Ahlgrim-Delzell, L., Harris, A. A., and Wakemanxya, S. (2008). A Meta-Analysis on Teaching Mathematics to Students with Significant Cognitive Disabilities. Exceptional Child. 74 (4), 407–432. doi:10.1177/001440290807400401
Brown, F., McDonnell, J. J., and Snell, M. E. (2020). Instruction of Students with Severe Disabilities. 9th edition. Pearson.
Bruce, S. M., and Borders., C. (2015). Communication and Language in Learners Who Are Deaf and Hard of Hearing with Disabilities: Theories, Research, and Practice. Am. Ann. Deaf 160 (4), 368–384. doi:10.1353/aad.2015.0035
Bukatko, D., and Daehler, M. W. (1995). Child Development: A Thematic Approach. 2nd edition. New Jersey: Houghton Mifflin Company.
Burleson, B. R., and Caplan, Scott. E. (1998). “Cognitive Complexity,” in Communication and Personality: Trait Perspectives. Editors J. C. McCroskey, J. A. Daly, M. M. Martin, and M. J. Beatty (Cresskill, NJ: Hampton Press), 233–286.
Burnes, J. J., and Clark., A. K. (2020). Characteristics Of Students Who Take Dynamic Learning Maps® Alternate Assessments (Technical Report No. 20-01). Lawrence, KS: University of KansasAvailable at: https://dynamiclearningmaps.org/sites/default/files/documents/publication/Characteristics_of_Students_Who_Take_DLM_AAs.pdf.
Carey, S. (2009). The Origin of Concepts. Oxford and New York: Oxford University Press. doi:10.1093/acprof:oso/9780195367638.001.0001
Cast, (2018). Universal Design for Learning Guidelines Version 2.2. ” Center for Applied Special TechnologyAvailable at: https://udlguidelines.cast.org/.
Center for Accessible Teaching, (2019). Learning, and Assessment Systems (ATLAS)DLM Item Writing Manual 2020. Lawrence, KS: University of Kansas.
Chard, M., Roulin, J.-L., and Bouvard, M. (2014). Visual Habituation Paradigm with Adults with Profound Intellectual and Multiple Disabilities: A New Way for Cognitive Assessment? J. Appl. Res. Intellect. Disabil. 27 (5), 481–488. doi:10.1111/jar.12079
Cherry, K. (2020). “What Is Cognition?” VeryWell Mind. Available at: https://www.verywellmind.com/what-is-cognition-2794982.
Clark, A. K., and Karvonen., M. (2020). Constructing and Evaluating a Validation Argument for a Next-Generation Alternate Assessment. Educ. Assess. 25 (1), 47–64. doi:10.1080/10627197.2019.1702463
Clark, A. K., Beitling, B., Bell, B., and Karvonen, M. (2016). Results from External Review during the 2015–2016 Academic Year. Lawrence, KS: University of Kansas, Center for Educational Testing and Evaluation.Technical Report No. 16-05
Clark, A. K., Kingston, N., Templin, J., and Pardos, Z. (2014). Summary of Results from the Fall 2013 Pilot Administration of the Dynamic Learning Maps™ Alternate Assessment System. Lawrence, KS: University of Kansas Center for Educational Testing and Evaluation.Technical Report No. 14-01
Common Core State Standards (2021). “About the Standards.” Common Core State Standards Initiative. Available at: http://www.corestandards.org/about-the-standards/ (Accessed January 2, 2021).
Confrey, Jere. (2019). Future of Education and Skills 2030: Curriculum Analysis – A Synthesis of Research on Learning Trajectories/Progressions in Mathematics. Paris: OECD Directorate for Education and Skills, Education Policy Committee.
Council of Chief State School Officers, (2014). Criteria for Procuring and Evaluating High-Quality and Aligned Summative Science Assessments. Available at: https://www.nextgenscience.org/sites/default/files/Criteria03202018.pdf (Accessed December 10, 2020).
Cress, C., Arens, K., and Zajicek, A. (2007). Comparison of Engagement Patterns of Young Children with Developmental Disabilities between Structured and Free Play. Education Train. Developmental Disabilities 42 (2), 152–164.
Demetriou, A., Shayer, M., and Efklides, A. (2016). Neo-Piagetian Theories of Cognitive Development: Implications and Applications for Education. London: Routledge.
Department of Education (2005). Alternate Achievement Standards for Students with the Most Significant Cognitive Disabilities, Non-regulatory Guidance. Washington, D.C: United States Department of EducationAvailable at: https://www2.ed.gov/policy/elsec/guid/altguidance.doc.
Department of Education (2010). April 9. Overview Information; Race to the Top Fund Assessment Program; Notice Inviting Applications for New Awards for Fiscal Year (FY) 2010. Fed. Regist. 75 (68), 18171–18185.
Department of Education (2015). Department of Education Peer Review of State Assessment Systems, Non-regulatory Guidance for States. Washington, D.C: U. S. Office of Elementary and Secondary Education.
Department of Education, n.d. “College- and Career-Ready Standards.” United States Department of EducationAvailable at: https://www.ed.gov/k-12reforms/standards.
Department of Education (2004). Standards and Assessments Peer Review Guidance: Information and Examples for Meeting Requirements of the No Child Left behind Act of 2001. Washington, D.C: United States Department of EducationAvailable at: https://files.eric.ed.gov/fulltext/ED483209.pdf.
Duschl, R. A. (2019). Learning Progressions: Framing and Designing Coherent Sequences for STEM Education. Discip Interdscip Sci. Educ. Res. 1 (November), 4. doi:10.1186/s43031-019-0005-x
Dynamic Learning Maps Consortium (2016). Dynamic Learning Maps 2015-2016 Technical Manual – Integrated Model. Lawrence, KS: University of Kansas Center for Educational Testing and Evaluation.
Dynamic Learning Maps Consortium (2018b). Dynamic Learning Maps 2016-2017 Technical Manual: Science. Lawrence, KS: University of KansasAvailable at: https://dynamiclearningmaps.org/sites/default/files/documents/publication/2016_2017_Science_Tech_Manual.pdf.
Dynamic Learning Maps Consortium (2020). Dynamic Learning Maps 2019-2020 Technical Manual Update: Science. Lawrence, KS: University of KansasAvailable at: https://2020-sci-techmanual.dynamiclearningmaps.org/.
Dynamic Learning Maps Consortium (2018a). Dynamic Learning Maps English Language Arts On-Site Item Writing Training. Lawrence, KS: University of Kansas.
Dynamic Learning Maps Consortium (2019). Dynamic Learning Maps Item Writing Manual 2020. Lawrence, KS: University of Kansas.
Dynamic Learning Maps Consortium (2013). Taxonomy for Type of Knowledge and Cognitive Process. Lawrence, KS: University of Kansas Center for Educational Testing and Evaluation.
Every Student Succeeds Act, (2015). Student Succeeds Act, U.S.C, 114-95. 20. Available at: https://www.congress.gov/bill/114th-congress/senate-bill/1177.
Flowers, C., Browder, D., and Ahlgrim-Delzell, L. (2006). An Analysis of Three States' Alignment between Language Arts and Mathematics Standards and Alternate Assessments. Exceptional Child. 72 (2), 201–215. doi:10.1177/001440290607200205
Flowers, C., Wakeman, S., Browder, D. M., and Karvonen, M. (2009). Links for Academic Learning (LAL): A Conceptual Model for Investigating Alignment of Alternate Assessments Based on Alternate Achievement Standards. Educ. Meas. Issues Pract. 28 (1), 25–37. doi:10.1111/j.1745-3992.2009.01134.x
Forehand, M. (2005). “Bloom's Taxonomy,” in In Emerging Perspectives On Learning, Teaching, and Technology. Michael Orey, 41–47. Available at: http://epltt.coe.uga.edu/index.php?title=Main_Page.
Forte, E. (2017). Evaluating Alignment in Large-Scale Standards-Based Assessment Systems. Washington, D.C.: Council of Chief State School Officers.
Francis, E. (2017). “What Is Depth of Knowledge?” ASCD InService. Available at: https://inservice.ascd.org/what-exactly-is-depth-of-knowledge-hint-its-not-a-wheel/. (Accessed May 9). doi:10.1093/wentk/9780190612269.001.0001
Georgia Department of Education, (2020). Test Administration Manual: Georgia Alternate Assessment 2.0 Spring 2020. Atlanta, Georgia: Georgia Department of EducationAvailable at: https://www.gadoe.org/Curriculum-Instruction-and-Assessment/Assessment/Documents/GAA_2/GAA-2.0-Spring-2020-TAM-Rev-1-29-20.pdf.
Herman, J. L. (2015). On the Road to Assessing Deeper Learning: What Direction Do Test Blueprints Provide? CRESST Report 849. Los Angeles, CAStandards, and Student Testing (CRESST): University of California, National Center for Research on Evaluation.
Institute of Education Sciences (1997). Individuals with Disabilities Education Act Amendments, U.S.C. 20, 1400.
Institute of Education Sciences (2004). Individuals with Disabilities Education Improvement Act, U.S.C. 20, 1400,
Institute of Education Sciences (2017). WWC Intervention Report: Self-Regulated Strategy Development. Washington, DC: IES What Works Clearinghouse.
Institute of Education Sciences (2018). “System of Least Prompts.” IES what Works Clearinghouse. Available at: https://ies.ed.gov/ncee/wwc/Intervention/1374.
Irvine, C. K. S., and Kevan, J. M. (2017). Competency-Based Education in Higher Education.” Res. Higher Education J. 33, no. 1, 1–27. Available at: https://eric.ed.gov/?id=EJ1161486 doi:10.4018/978-1-5225-0932-5.ch001
Jackson, L. B., Ryndak, D. L., and Wehmeyer, M. L. (2008). The Dynamic Relationship between Context, Curriculum, and Student Learning: A Case for Inclusive Education as a Research-Based Practice. Res. Pract. Persons Severe Disabilities 34 (1), 175–195. doi:10.2511/rpsd.33.4.175
National Research Council (1999). in How People Learn: Brain, Mind, Experience, and School. Division of Behavioral and Social Sciences. Editors J. Bransford, A. Brown, and R. Cocking. Center for Education (Washington, DC: The National Academies Press).
National Research Council (2001). in Knowing what Students Know: The Science and Design of Educational Assessment. Editors J. W. Pellegrino, N. Chudowsky, and R. Glaser (Washington, DC: The National Academies Press).
Karvonen, M., Russell, S., and Clark, A. K. (2020). Response Process Evidence for Academic Assessments of Students with Significant Cognitive Disabilities.Romine
Kearns, J. F., Towles-Reeves, E., Towles-Reeves, H. L., Kleinert, J. O. R., and Thomas, M. K.-K. (2011). Characteristics of and Implications for Students Participating in Alternate Assessments Based on Alternate Academic Achievement Standards. J. Spec. Educ. 45 (1), 3–14. doi:10.1177/0022466909344223
Kingston, N. M., Karvonen, M., Bechard, S., and Erickson, K. (2016). The Philosophical Underpinnings and Key Features of the Dynamic Learning Maps Alternate Assessment. Teach. Coll. Rec. (Yearbook) 118, 1–30. Available at: https://eric.ed.gov/?id=EJ1110770.
Kleinert, H., Kleinert, J., and Kearns, J. (2016). NCSC GSEG Policy Paper: Communicative Competence for Students with the Most Significant Disabilities: A Three-Tiered Model of Intervention. Minneapolis, MN: University of Minnesota, National Center and State Collaborative. doi:10.1142/9915
Kleinert, H. L., Browder, D. M., and Towles-Reeves, E. A. (2009). Models of Cognition for Students with Significant Cognitive Disabilities: Implications for Assessment. Rev. Educ. Res. 79 (1), 301–326. doi:10.3102/0034654308326160
Koebley, S., Russell, S., McCann, M., and Glore, C. (2020). Interim Report on Development and Revision of Essential Elements in Science for the Dynamic Learning Maps Alternate Assessment System. Lawrence, KS: University of Kansas, Accessible Teaching, Learning, and Assessment Systems.
Krathwohl, D. R. (2002). A Revision of Bloom's Taxonomy: An Overview. Theor. into Pract. 41 (4), 212–218. doi:10.1207/s15430421tip4104_2
Martone, A., and Sireci, S. G. (2009). Evaluating Alignment between Curriculum, Assessment, and Instruction. Rev. Educ. Res. 79 (4), 1332–1361. doi:10.3102/0034654309341375
Marzano, R. J., and Kendall, J. S. (2007). The New Taxonomy of Educational Objectives. Thousand Oaks, CA: Corwin Press.
McLean, L. (1990). Communication Development in the First Two Years of Life: A Transactional Process. Zero Three Bull. Natl. Cent. Clin. Infant Programs 11, 13–19.
Miller, P. H. (2011). “Piaget's Theory: Past, Present, and Future,” in In the Wiley-Blackwell Handbook Of Childhood Cognitive Development. Editor U. Goswami (Hoboken, NJ: Wiley-Blackwell).649–672.
Mislevy, R. J., and Haertel, G. D. (2006). Implications of Evidence-Centered Design for Educational Testing. Educ. Meas. Issues Pract. 25 (4), 6–20. doi:10.1111/j.1745-3992.2006.00075.x
Mislevy, R. J., Steinberg, L. S., and Almond, R. G. (2003). Focus Article: On the Structure of Educational Assessments. Meas. Interdiscip. Res. Perspective 1 (1), 3–62. doi:10.1207/S15366359MEA0101_02
Mwamwenda, T. S. (2009). Educational Psychology: An African Perspective. 3rd edition. Durban: Heinemann.
National Governors Association (2010). Common Core State Standards. Washington, DC: National Governors Association Center for Best Practices, Council of Chief State School Officers.
Nemeth, Y. M., and Justin, P. (2017). Dynamic Learning Maps Science Alternate Assessment: Alignment Study. Alexandria, VA: Human Resources Research Organization.
NextGenScience (2013). Appendix E – Progressions within the Next Generation Science Standards. San Francisco, CA: NextGenScience at WestEdAvailable at: https://www.nextgenscience.org/sites/default/files/resource/files/AppendixE-ProgressionswithinNGSS-061617.pdf.
NextGenScience (2013). Appendix F – Science and Engineering Practices in the NGSS. San Francisco, CA: NextGenScience at WestEdAvailable at: https://www.nextgenscience.org/sites/default/files/resource/files/Appendix%20F%20%20Science%20and%20Engineering%20Practices%20in%20the%20NGSS%20-%20FINAL%20060513.pdf.
NextGenScience, (2013). Appendix G – Crosscutting Concepts. San Francisco, CA: NextGenScience at WestEdAvailable at: https://www.nextgenscience.org/sites/default/files/resource/files/Appendix%20G%20-%20Crosscutting%20Concepts%20FINAL%20edited%204.10.13.pdf.
NextGenScience (2021). “Today’s Science Standards.” NextGenScience at WestEd. Available at: https://ngs.wested.org/todays-science-standards/ (Accessed January 2, 2021).
NGSS Lead States (2013). Next Generation Science Standards: For States, by States. Washington, DC: The National Academies Press.
Orey, M. (2001). in Emerging Perspectives on Learning, Teaching, and Technology (Athens, GA: University of Georgia). Available at: http://epltt.coe.uga.edu/index.php?title=Main_Page.
PARCC, ETS, and Pearson (2014). Development of Cognitive Complexity Measures for PARCC. Philadelphia, PA.” Paper presented at the Annual Meeting of the American Educational Research AssociationAvailable at: https://www.aera.net/LinkClick.aspx?fileticket=v1rGlSYcCqk%3D&portalid=38.
Pellegrino, J. W., DiBello, L. V., and Goldman, S. R. (2016). A Framework for Conceptualizing and Evaluating the Validity of Instructionally Relevant Assessments. Educ. Psychol. 51 (1), 59–81. doi:10.1080/00461520.2016.1145550
Persaud, C. (2018). “Bloom’s Taxonomy: The Ultimate Guide.” Top Hat. Available at: https://tophat.com/blog/blooms-taxonomy/.(Accessed August 13, 2018
Piaget, J. (1983). ““Piaget’s Theory,”. In Handbook Of Child Psychology. Editors P. H. Mussen, and W. Kessen (New York: Wiley), Vol. 1.
National Research Council (2007). in Taking Science to School: Learning and Teaching Science in Grades K–8. Editors R. A. Duschl, H. A. Schweingruber, and A. W. Shouse (Washington, DC: The National Academies Press).
Roach, A. T., Elliott, S. N., and Webb, N. L. (2005). Alignment of an Alternate Assessment with State Academic Standards. J. Spec. Educ. 38 (4), 218–231. doi:10.1177/00224669050380040301
Romine, S., Russell, L. A., Schuster, J., and Karvonen, M. (2018). Developing and Evaluating Learning Map Models in Science: Evidence from the I-SMART Project. Lawrence, KSand Assessment Systems (ATLAS): University of Kansas, Accessible Teaching, Learning.
Rostami, K., and Khadjooi, K. (2010). The Implications of Behaviorism and Humanism Theories in Medical Education. Gastroenterol. Hepatol. 3 (2), 65–70.
Rowland, C. (2012). Communication Matrix: Description, Research Basis, and Data. Portland: OR: Oregon Health and Science UniversityAvailable at: https://www.communicationmatrix.org/Uploads/Pdfs/CommunicationMatrixDataandResearchBasis.pdf.
Rowland, C., and Schweigert, P. (2000). Tangible Symbols, Tangible Outcomes. Augmentative Altern. Commun. 16 (2), 61–78. doi:10.1080/07434610012331278914
Saeed, T., and Tahir, S. (2016). Working Memory in Children with Intellectuality Disability (ID). Jpcpy 6 (4), 00366. doi:10.15406/jpcpy.2016.06.00366
Schultz, S. R., Wiley, C. R. H., Dvorak, R. L. N., and Michaels, H. R. (2014). Smarter Balanced Assessment Consortium: Alignment Study Report. Alexandria, VA: Human Resources Research Organization.
Skinner, B. F. (1950). Are Theories of Learning Necessary? Psychol. Rev. 57, 193–216. doi:10.1037/h0054367
Smarter Balanced Assessment Consortium (2016). Smarter Balanced Assessment Consortium: Alignment Study Report (Revised). Alexandria, VA: Human Resources Research Organization.
State University, (2013). “Taxonomies of Educational Objectives.” StateUniversity.Com Education Encyclopedia. Available at: https://education.stateuniversity.com/pages/2475/Taxonomies-Educational-Objectives.html.
Stremel-Campbell, K., and Matthews, J. (1988). “Development of Emergent Language,” in Monmouth, OR: Communication Skills Center for Young Children with Deaf-Blindness Teaching Research. Editors M. Bullis, and G. Fielding (Division, Oregon State System of Higher Education), 165–201.
Thompson, W. J., and Nash, B. (2019). Empirical Methods for Evaluating Maps: Illustrations and Results, Paper presented at Symposium on Beyond Learning Progressions: Maps as Assessment Architecture, Toronto, Ontario, Canada, April 4–8, 2019. National Council of Measurement in Education Annual Meeting.
Thorndike, E. L. (1913). Educational Psychology, Vols. 1 and 2. New York: Columbia University Press.
Thurlow, M. L., Sheryl, S., Larson, E. D., and Liu, K. K. (2019). 2018-19 Participation Guidelines and Definitions for Alternate Assessments Based on Alternate Academic Achievement Standards. NCEO Report 415. Minneapolis, MN: University of Minnesota, National Center on Educational OutcomesAvailable at: https://nceo.info/Resources/publications/OnlinePubs/report415/default.html.
Towles-Reeves, E., Kleinert, H., and Muhomba, M. (2009). Alternate Assessment: Have We Learned Anything New? Exceptional Child. 75 (2), 233–252. doi:10.1177/001440290907500206
Webb, N. L. (1997). Criteria for Alignment of Expectation and Assessments in Mathematics and Science EducationResearch Monograph No. 6. Madison, WIWashington, DC: National Institute for Science EducationUniversity of Wisconsin-MadisonCouncil of Chief State School Officers.
Wehmeyer, M. L., Shogren, K. A., Palmer, S. B., Williams-Diehm, K. L., Little, T. D., and Boulton, A. (2012). The Impact of the Self-Determined Learning Model of Instruction on Student Self-Determination. Exceptional Child. 78 (2January), 135–153. doi:10.1177/001440291207800201
Yoder, P. J., McCathren, R. B., McCathren, S. F., and Warren, A. L. (2001). Important Distinctions in Measuring Maternal Responses to Communication in Prelinguistic Children with Disabilities. Commun. Disord. Q. 22 (3), 135–147. doi:10.1177/152574010102200303
Keywords: cognitive process dimensions, learning map model, taxonomy, alternate assessment, alignment, depth of knowledge
Citation: Bechard S, Karvonen M and Erickson K (2021) Opportunities and Challenges of Applying Cognitive Process Dimensions to Map-Based Learning and Alternate Assessment. Front. Educ. 6:653693. doi: 10.3389/feduc.2021.653693
Received: 15 January 2021; Accepted: 10 May 2021;
Published: 26 May 2021.
Edited by:
Alicia Alonzo, Michigan State University, United StatesReviewed by:
Emily Toutkoushian, The American Board of Anesthesiology, United StatesCopyright © 2021 Bechard, Karvonen and Erickson. This is an open-access article distributed under the terms of the Creative Commons Attribution License (CC BY). The use, distribution or reproduction in other forums is permitted, provided the original author(s) and the copyright owner(s) are credited and that the original publication in this journal is cited, in accordance with accepted academic practice. No use, distribution or reproduction is permitted which does not comply with these terms.
*Correspondence: Sue Bechard, c3VlLmJlY2hhcmRAa3UuZWR1
Disclaimer: All claims expressed in this article are solely those of the authors and do not necessarily represent those of their affiliated organizations, or those of the publisher, the editors and the reviewers. Any product that may be evaluated in this article or claim that may be made by its manufacturer is not guaranteed or endorsed by the publisher.
Research integrity at Frontiers
Learn more about the work of our research integrity team to safeguard the quality of each article we publish.