- 1Department of Physics, Lawrence University, Appleton, WI, United States
- 2Department of Physics and Astronomy, Purdue University, West Lafayette, IN, United States
- 3Department of Curriculum and Instruction, Purdue University, West Lafayette, IN, United States
Cognitive load theory (CLT) posits the classic view that cognitive load (CL) has three-components: intrinsic, extraneous and germane. Prior research has shown that subjective ratings are valid measures of different CL subtypes. To a lesser degree, how the validity of these subjective ratings depends on learner characteristics has not been studied. In this research, we explored the extent to which the validity of a specific set of subjective measures depends upon learners’ prior knowledge. Specifically, we developed an eight-item survey to measure the three aforementioned subtypes of CL perceived by participants in a testing environment. In the first experiment (N = 45) participants categorized the eight items into different groups based on similarity of themes. Most of the participants sorted the items consistent with a threefold construct of the CLT. Interviews with a subgroup (N = 13) of participants provided verbal evidence corroborating their understanding of the items that was consistent with the classic view of the CLT. In the second experiment (N = 139) participants completed the survey twice after taking a conceptual test in a pre/post setting. A principal component analysis (PCA) revealed a two-component structure for the survey when the content knowledge level of the participants was initially lower, but a three-component structure when the content knowledge of the participants was improved to a higher level. The results seem to suggest that low prior knowledge participants failed to differentiate the items targeting the intrinsic load from those measuring the extraneous load. In the third experiment (N = 40) participants completed the CL survey after taking a test consisting of problems imposing different levels of intrinsic and extraneous load. The results reveals that how participants rated on the CL survey was consistent with how each CL subtype was manipulated. Thus, the CL survey developed is decently effective measuring different types of CL. We suggest instructors to use this instrument after participants have established certain level of relevant knowledge.
Introduction
Cognitive load theory (CLT) attends to the limited working memory capacity (Cowan, 2001) for instruction and learning. It posits that optimal design of instruction and learning should not overload learners’ working memory capacity (Sweller, 1988, 1994, 2010; Sweller et al., 1998). This is because novel information and previously learned information from long-term memory need to be consciously processed in working memory. However, working memory is limited in capacity and duration when processing novel information, especially without deliberate rehearsal (Baddeley, 1992; Cowan, 2001). This is in contrast to the long-term memory, which is an unlimited, permanent repository for organized knowledge that governs our cognitive processes (Sweller, 2010).
Cognitive load is defined as the working memory load experienced when performing a specific task (Kalyuga, 2011; Sweller et al., 2011; van Merriënboer and Sweller, 2005). This places a requirement on instruction to avoid overloading the working memory during learning. Cognitive load is a multifaceted construct. Historically, extraneous cognitive load (ECL) was the first CL subtype introduced by Sweller (1988). ECL refers to the working memory resources allocated to unproductive cognitive processes. The level of ECL is related to the presentation format (e.g., visual, audio, and text), spatial and temporal organization of various information, etc. For example, high ECL can be caused if the same information is presented simultaneously in both text and audio modalities, since the redundancy would cause cognitive resources to be wasted which could potentially hinder learning. The second CL subtype is the intrinsic cognitive load (ICL) which is related to the working memory resources allocated to dealing with the learning objectives (Sweller, 1994). Finally, a third kind of CL, germane cognitive load (GCL), refers to the working memory resources used for constructing, chunking and automating schemas (Sweller et al., 1998). From a common theoretical perspective, John Sweller suggested that all three subtypes of CL can be defined in terms of the core concept of element interactivity (Sweller, 2010). Under this theoretical formalism, the load is extraneous if the element interactivity can be reduced without altering the learning objective. The load is intrinsic if reducing the element interactivity alters the learning objective. Germane load simply refers to the working memory resources used for processing the intrinsic load, such as through chunking, schema generation and automation, and therefore is also tied to element interactivity. Kalyuga (2011) also argues germane load is not an independent load type, since there is no theoretical argument for any difference between GCL and ICL. Thus, it has been suggested that GCL can be readily incorporated into the definition of ICL by redefining the cognitive processes involving GCL as pertaining to the learning goals related to ICL. Thus, there are only two independent components in CLT: ECL and ICL, which are additive. Scientists would favor a two-component model if a two-component construct of CL has equal or more explanatory power than a three-component model. This comes from Occam’s razor argument which says the simpler model is usually the right one. Jiang and Kalyuga (2020) have provided evidence supporting a two-component model over a three-component model using subjectively rated CL surveys.
The aforementioned latest development in CLT suggests any measurement of CL should focus on differentiating ICL and ECL. Monitoring the levels of different types of CL perceived by students could help maximize the learning outcomes. However, Sweller (2010) has also argued that learners’ content knowledge level could affect their ability in discerning ICL from ECL. Learners with low content knowledge level may have difficulty differentiating irrelevant information from relevant information, or productive learning process from unproductive learning process. Therefore, the knowledge level moderates students’ capability to discern ICL from ECL. This certainly places even further challenges for educators since measuring different CL subtypes is already an ongoing challenge in the CLT research community (Kirschner et al., 2011). Thus, it begs researchers to design appropriate measurement tools and identify the condition for the appropriate use of the tools.
There are two widely used approaches toward measuring CL: subjective (e.g., self-report) and objective (e.g., tests and physiological measures). Subjective measures of Sweller’s three subtypes of CL have been more extensively explored (Hart and Staveland, 1988; Paas, 1992; Kalyuga et al., 1998; Gerjets et al., 2004, 2006; Ayres, 2006; Leppink et al., 2013). Subjective measures typically require participants to evaluate their own cognitive processes during a learning task. Thus, they rely on the participant’s ability to introspect on their learning experience. A great deal of work has gone into developing such Likert scale style subjective measures of CL. In these studies, researchers generally manipulated ICL in terms of adjusting the amount of information presented to students, such as modular vs. molar solutions comparison (Gerjets et al., 2004, 2006), changing the number of arithmetic operations (Ayres, 2006), or adjusting the learning material complexity (Windell and Wieber, 2007). These studies have shown that subjective measures could discern different levels of ICL using a difficulty rating (Windell and Wieber, 2007; Cierniak et al., 2009), a mental effort rating (Ayres, 2006), sub-items of NASA-TLX, such as stress, devoted effort, task demands (Gerjets et al., 2004, 2006), and mental demands (Windell and Wieber, 2007). The authors manipulated ECL in terms of a split-attention effect (Kalyuga et al., 1998; Windell and Wieber, 2007), and a modality effect (Windell and Wieber, 2007). They showed that ECL can be measured using a weighted workload of NASA-TLX (Windell and Wieber, 2007), a difficulty rating (Kalyuga et al., 1998), and a rating of difficulty of interaction with the material (Cierniak et al., 2009). According to Sweller (2010), GCL is affected only by the learner’s motivation. Some researchers manipulated GCL/motivation through, instructional format (Gerjets et al., 2006). These studies showed GCL can be measured using, sub-items of NASA-TLX, such as task demands, effort, and navigational demands (Gerjets et al., 2006), or multiple survey items evaluating learning performance (Leppink et al., 2013).
In this work, we developed and validated a subjective survey for assessing the CL experienced by learners taking a conceptual physics test. The survey was adapted from the CL survey developed by Leppink et al. (2013). The motivation for developing this survey is that the survey developed by Leppink et al. (2013) and many previous subjective surveys were designed to measure the CL during instructional activities. In educational psychology, it has been suggested that quizzes and tests can be used as learning practice for learners (Roediger and Karpicke, 2006; Karpicke et al., 2014). This is contradictory to the traditional view that tests can only be used as summative evaluation of learner performance. Many different pedagogical methods require instructors to create problems of their own (Mazur, 2013). Instructors need to create different testing tasks if they want to use frequent testing as learning practice for students to construct knowledge. Thus, it is important to make sure the tasks on the test are optimally designed. For example, the task should not use confusing language in the statements. On the other hand, many different problem tasks purposefully provide more information than needed to solve the problem, such as context rich problem (Ogilvie, 2009). It is possible students will process the unnecessary information if they do not have the relevant knowledge, or they will report the statement of a problem task is confusing even if the statement is perfectly clear to an expert. A CL survey that can be used to measure the three types of CL could inform instructors if the tasks created used clear language and provide instructors feedback about how students process unnecessary information.
In this study, we adopt an argument-based approach to describe the validation of the CL survey we developed (Kane, 2013). During the development and validation of the CL survey, we payed attention to both reliability and validity. Reliability refers to the consistency of the items designed to measure the same theoretical attribute, and validity refers to the appropriateness of interpreting the subjective ratings on the survey.
We conducted three experiments to validate the CL survey. Together these three experiments contributed to establishing the validity of the CL survey and the condition under which it should be administered. The studies involving human participants were reviewed and approved by the IRB office at Purdue University. The participants provided their written informed consent to participate in this study.
Experiments
Experiment One
Materials and Procedure
We adapted the first six items on the survey used by Leppink et al. (2013), and adapted two other items targeting GCL based on previous literature (Paas, 1992; Salomon, 1984; see Table 1). Each item was rated on a Likert scale from 1 (not at all the case) to 9 (completely the case).
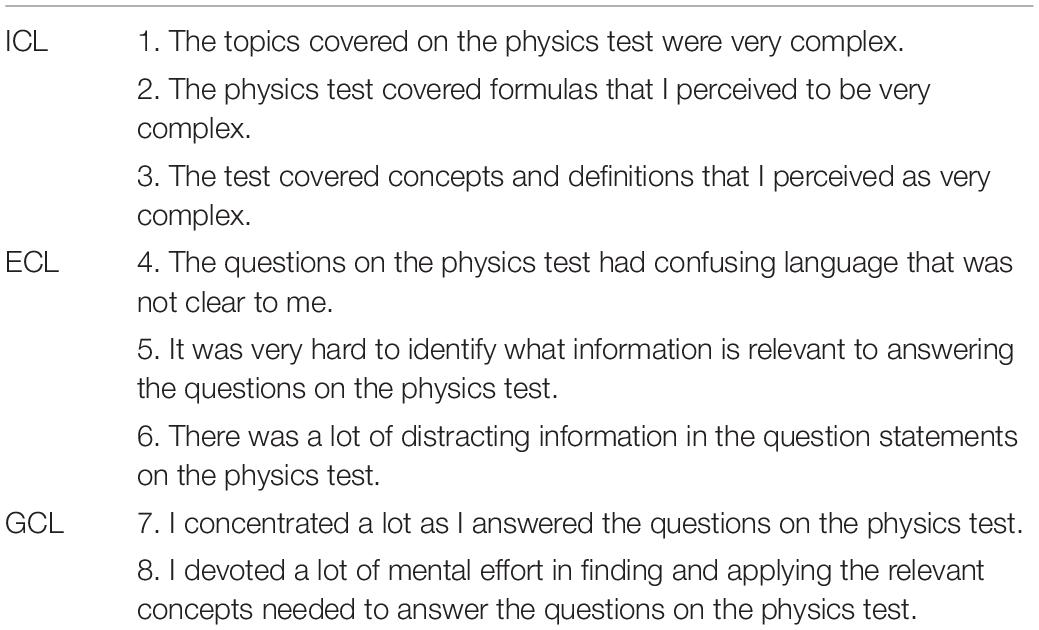
Table 1. A mapping between all items of the cognitive load survey and what they are constructed to measure.
In experiment one our goal was to establish the construct validity of the survey to verify whether the survey items indeed measure the three different cognitive sub-loads that they were intended to measure. We asked a different group of participants (N = 45) to categorize the items on the CL survey into three groups based on the common theme. A subgroup of the participants (N = 13) were interviewed about how they perceived the items on the survey. Follow-up questions were asked during the interview process. Participants were asked to provide their reasoning for the way in which they grouped the items and were asked to express the similarities and differences among the items grouped together.
Results of Experiment One
In experiment one, we asked N = 45 participants to sort the eight items on the CL survey into three groups based on a common theme. Participants were offered extra credit equal to 1% of their total course grade for their participation. The items were presented to the participants in a randomized order. Twenty-nine of 45 participants (64%) sorted the items as expected (Group A: items 1, 2, 3; Group B: items 4, 5, 6; Group C: items 7, 8). Nine of 45 participants (20%) misplaced one or two items different from the expected grouping. Seven of 45 participants (16%) grouped the items following no apparent pattern.
Thirteen of the 29 participants were randomly selected for a follow-up interview after they completed the sorting task. Each participant was asked to provide the similarities and differences between the items of each group that they had created. Participants’ responses were audio recorded and coded. Ten (10) of the 13 participants grouped the survey statements in a way that was consistent with the CLT model. These interviews allowed us to detect participants’ perception of the meaning of these statements. The discussion with participants’ and their descriptions about each group are described below. The second author (JM) conducted the interview for experiment one since he was not associated with the course in any way at the time.
Discussion
Participants commonly responded that they grouped ICL items together because they were dealing with complexity. When confronted with questions about what makes a problem complex, participants often responded that a problem with several different elements was complex. One student remarked “circuit problems incorporate a lot of different elements. There is an element of knowing how bulbs in series function, but there also bulbs in parallel, and there is also a switch.” It was also commonly reported that not having a familiarity with the ideas contained in a question makes it complex. To that end, one student said, “For this question about the power delivered to these circuits you have to know the definition of power and resistors and understand how circuits work.” Here students’ argument clearly aligns well with how CLT defines ICL in terms of element interactivity which is reflected in the complexity (Sweller, 2010).
When participants were asked what differentiates these statements in this group from each other, they often remarked that the statements spoke to differing levels of organization. One student said “some of the items are about topics and the others are about sub-topics.” Statement one referred to topics, while statements two and three mentioned formulas and concepts, respectively. Participants generally agreed that topics are more general than concepts which is broader than formulas.
The common theme expressed by the participants about the ECL items was confusion. “These statements have to do with ambiguity, distraction, and confusion while taking a test and those things go together.” Some participants also related these items to the statement of the questions in the test, saying things like “These statements deal with the language of the question rather than the content,” while another said “These were within the question itself. Like language and extra information, the wording of the test.” Students’ understanding of the items seem to be consistent with what CLT describes ECL in terms of its detrimental impact for learning since they are mostly distracting to learning (Sweller, 1988, 2010).
When participants were asked what differentiates the statements in this group from each other, they replied that the statements were talking about different ways in which things can be confusing. For example, “These statements are different because (5) is about finding the relevant information (6) is about having too much information, and (4) is about the question being asked in a confusing way.” Participants were asked what makes confusing language different from distracting information. To which one replied “confusing information is related to things I don’t understand, where distracting information is related to having more information than I need to solve the problem,” while another observed “Language has a lot to do with the words you are using, distracting information deals with words and sentences that have no purpose.” Further probing, many respondents were asked what makes a test question confusing. Participants commented that questions they didn’t know how to answer were confusing. For example, “This question about electric field is confusing because I don’t know much about electric field.” This last response seems to indicate the possibility of conflating the reasons for grouping items as ICL or ECL.
Participants grouped the GCL items on the survey as thematically similar often reflected that they were related to one’s subjective experience of the test instead of difficult content or confusing language. One student stated, “These items covered what you felt toward the exam, not objective difficulty but more like your personal experience” while another indicated “These items dealt less with the test itself and more with the test taker, more about concentration and mental effort, having to put more thought into answering the questions instead of difficult concepts or the wording of questions.” Another participant said “Both items had to do with thinking. This one (7) was concentrating a lot, and this one (8) was exerting a lot of mental effort to figure out what was needed.” Here what the students reported were consistent with the definition of GCL as proposed by Sweller that GCL has to do with the personal experience of students, especially how much cognitive resources were devoted to learning as reflected by the words: “concentration” and “mental effort” (Salomon, 1984; Paas, 1992; Sweller, 2010).
When asked about what individuated these statements, participants often designated the difference in the concepts “mental effort” and “concentration.” In a series of subsequent questions most participants were unable to definitively make a distinction between the two constructs, with responses such as, “Concentration is more like focus. Mental effort is more like thinking about the information you already know to find the answer.” In order to further probe their interpretation of these statements the interviewer asked participants to reflect on “concentration” and “mental effort” in terms of the steps to for solving a physics problem. Participants generally stated that reading and taking in the information of the problem statement required concentration while building a model of the problem and formulating a solution required mental effort.
In summary, results of experiment one indicate that participants were remarkably astute not just in grouping the statements, but also in articulating their criteria for the groupings. Further they were able to describe with clarity how the various statements within each group were similar to and different from each other. These results support the face validity and construct validity of the items on the survey.
Experiment Two
Materials
In experiment two, our goal was to determine whether the validity of survey i.e., its alignment with the three-component model of CLT was conditional to the level of content knowledge of the participants. We know from literature that knowledge level can influence the perceived CL since low knowledge learners may not differentiate ICL from ECL, while high knowledge learners can distinguish between these two constructs (Sweller, 1994, 2010). Based on this, we hypothesize that the items used for assessing the ICL and ECL will load to the same component when they have low knowledge, and the items used for assessing ICL and ECL will load to two separate components when they have high knowledge level. We conducted a principal component analysis to confirm if different items on the survey aligned with the three different CL subtypes.
Procedure
N = 139 participants enrolled in a physics class for elementary education majors participated in the study. In a pre/post-test design, we asked participants to complete the DIRECT (Determining and Interpreting Resistive Electric Circuit Concepts Test) assessment at the beginning and at the end of an instructional unit on DC electric circuits. DIRECT was developed by Engelhardt and Beichner (2004) for assessing conceptual understanding of circuits. DIRECT has 29 multiple choice items on it. There is only one correct answer to each item. It usually took students ∼30 min to complete. After each test (pre- and post-test), we administered this CL survey individually. Presumably, participants had low knowledge level of the relevant concepts at the beginning of the instructional unit (as confirmed by their performance on the test); and they had higher knowledge level of the relevant material by the end of the instructional unit (again, confirmed by their performance on the test).
To validate the CL survey that has three underlying components, a principal component analysis (PCA) was conducted for the two sets (pre and post) of data using IBM SPSS version 24 software. PCA analysis was used since we are exploring how many components the 8 items of the CL survey would load on to. As such, PCA is a proper analysis. The results of the PCA are shown in Table 2 and Table 3.
Results of Experiment Two
For the CL survey results collected after participants had completed the DIRECT pre-test, there were no outliers or extreme skewness or kurtosis, as well as sufficient inter-item correlation; KMO (Kaiser-Meyer-Olkin test) = 0.839, Bartlett’s χ2(28) = 481.779, p < 0.001. A high value of KMO indicates the data is suitable for a factor analysis; Bartlett’s test of sphericity indicates the data is suitable for a factor analysis when the test achieves significance level (0.05). Both KMO and Bartlett tests indicated the data collected were fit for a PCA analysis. KMO is measure of sampling adequacy. Given the small sample size, a PCA was conducted. Varimax rotation was performed to investigate the correlational nature of the underlying components. When, eigenvalue one was used as criteria for determining the number of underlying components, a two-component model emerged with 68% of the variation explained by the two components. Items 1–6 are loaded to the first component and items 7–8 are loaded to the second component. This seems to support the idea that when participants do not have high knowledge level, they could not differentiate ICL from ECL as suggested by CLT (Sweller, 2010). Reliability analysis for the six components loaded to the same construct revealed Cronbach’s alpha values of 0.874 for Items 1, 2, 3, 4, 5, 6 (1, 2, 3 are expected to measure ICL; 4, 5, 6 are expected to measure ECL); and 0.782 for Items 7, 8 (expected to measure GCL).
For the CL survey results collected after participants had completed the DIRECT post-test, there were no outliers or extreme skewness or kurtosis, as well as sufficient inter-item correlation; KMO = 0.708, Bartlett’s χ2(28) = 496.201, p < 0.001. Both KMO and Bartlett tests indicated the data collected were fit for a PCA analysis. Given the small sample size, a PCA was conducted. Varimax rotation was performed to investigate the correlational nature of the underlying components. When, eigenvalue 1 was used as criteria for determining the number of underlying components, a three-component model emerged with 77% of the variation explained by the three-components. Items 1, 2, and 3 are loaded to the first component; items 4, 5, and 6 are loaded to the second component; items 7, and 8 are loaded to the third component. This provides evidence that when participants have high knowledge level (as on the post-test), they could differentiate ICL from ECL as suggested by CLT (Sweller, 2010). Reliability analysis for the three-components revealed Cronbach’s alpha values of 0.816 for Items 1, 2, 3 (1, 2, 3 are expected to measure ICL); and 0.763 for 4, 5, 6 (4, 5, 6 are expected to measure ECL); and 0.687 for Items 7, 8 (expected to measure GCL).
Discussion
In summary, results of experiment two indicate that, in a testing environment, the CL survey we developed could capture the three kinds of CL subtypes. Results confirmed that students’ capability of differentiating ICL from ECL depends on their content knowledge level. Thus, when students have relatively low content knowledge, they fail to differentiate ICL from ECL, which suggests even information related to learning can be confusing to students. This is consistent with what Sweller (2010) has suggested that content knowledge level could moderate how students self-perceive their CL. In addition, the items on our CL survey seem to be able to capture GCL regardless of the content knowledge level of students, which suggests students could introspect their level of effort for making sense and applying knowledge.
Experiment Three
Materials
In this experiment, we developed two pairs of tasks with clear manipulations using a 2 (High/Low ECL) × 2 (High/Low ICL) design (see Figure 1) based on Sweller (2010) and the redundancy effect of multimedia learning theory (Mayer, 2014).
The ICL level is the same (low) for both problems A and C because the underlying principle is series circuit law. Problem C has a higher ECL compared to A since it also presents redundant textual information. Problems B and D have higher ICL compared to problems A and C, since the underlying physics principle for both these problems (B and D) is a combination of series and parallel circuit laws. Compared to problem B, problem D has a higher ECL since it presents redundant textual information as well.
Procedure
A group (different from Experiments 1 and 2) of N = 40 elementary education majors participated in this study. We first asked participants to solve the four problem tasks on a short physics quiz. After participants completed the quiz, they were shown three pairs of problems, that they had just solved juxtaposed with each other: Pair A–B, where both problems were manipulated to impose low ECL but different ICL (A < B); Pair A–C, where both problems were manipulated to impose low ICL, but different ECL (A < C); and Pair A–D, where the problems were manipulated to impose different ICL (A < D) and ECL (A < D).
Although there were six potential problem pairs, we chose these three pairs because they would allow us to probe the extent to which participants were able to discern differences in both ICL and ECL when only one of those two had been manipulated to be different (as in pairs A–B and A–C), and one in which both had been manipulated to be different (pair A–D).
Each of the eight items on our CL survey were presented to the participants, and then they were asked to answer a question like: “If you are asked to rate: “The topics covered on the physics question were very complex” on a scale from 1 (Not at all the case) to 9 (Completely the case)”, select from one of three options: (i) I would rate A is higher than C on the scale; (ii) I would rate A is the same as C on the scale; (iii) I would rate A is lower than C on the scale”. An example is shown in Figure 2.
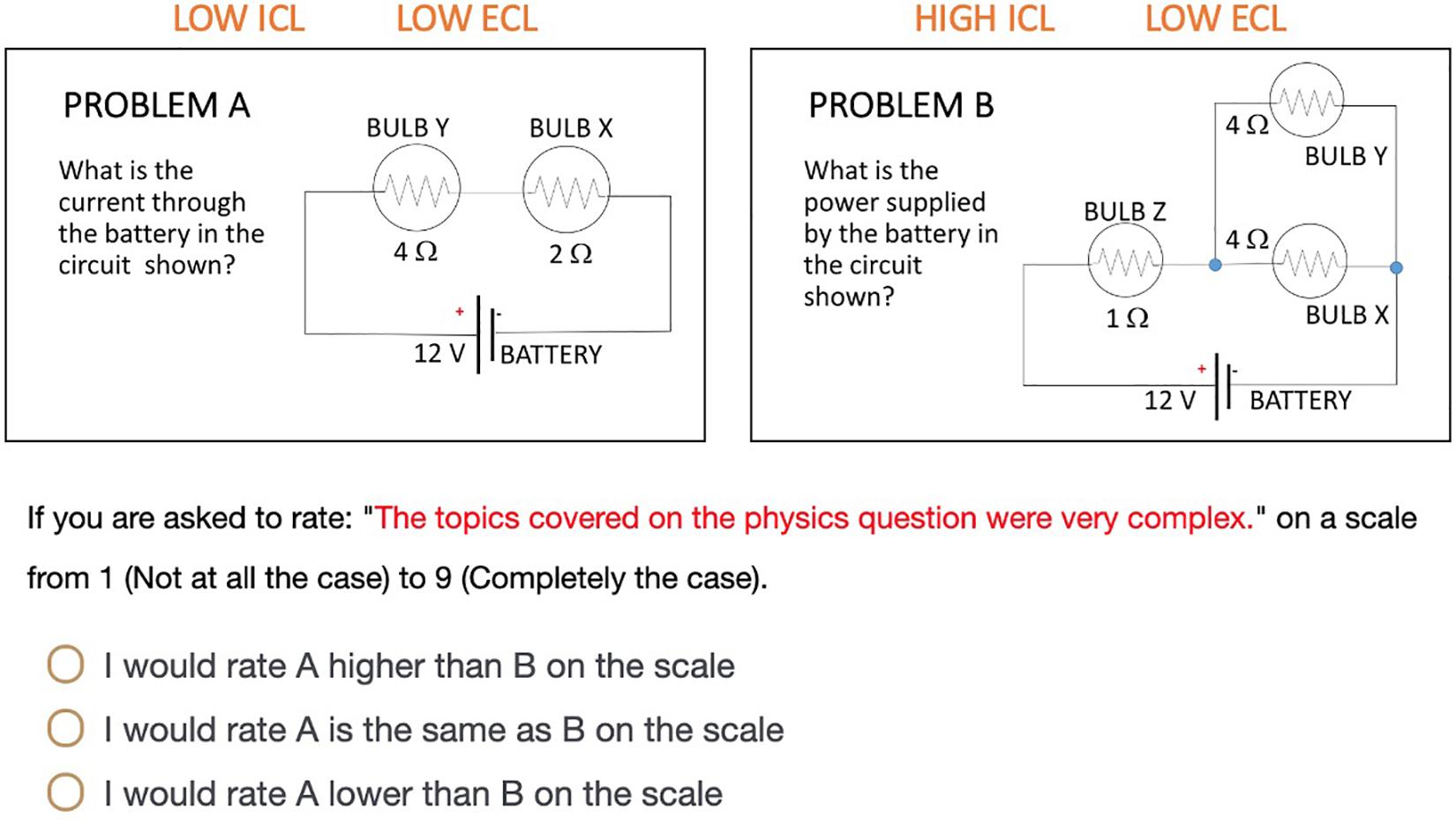
Figure 2. Example of questions participants had to respond to during the cognitive load survey rating session.
Results
We collapsed the answers to the items on the survey targeting the same underlying construct and calculated the percentage of participants selecting each of the three options. The results can be found in Tables 4–6.
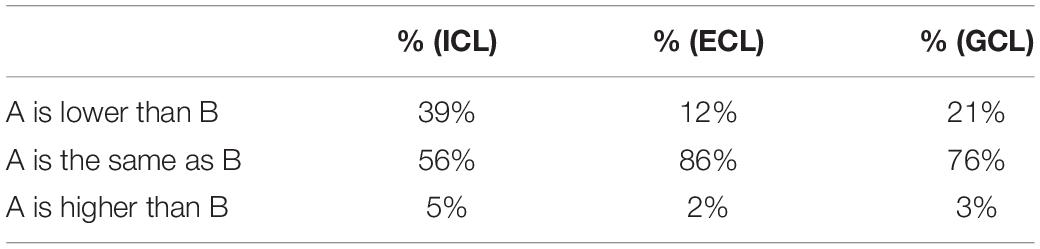
Table 4. Percentage of each option selected by participants for each aspect of cognitive load for (A,B) comparison.
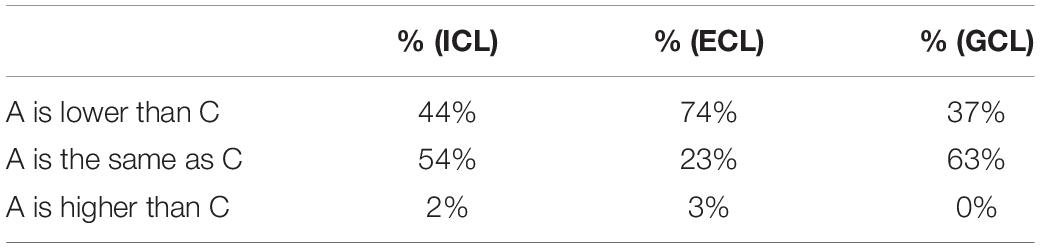
Table 5. Percentage of each option selected by participants for each aspect of cognitive load for (A,C) comparison.
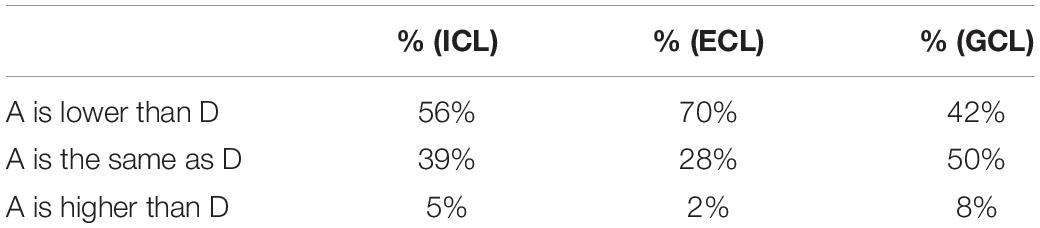
Table 6. Percentage of each option selected by participants for each aspect of cognitive load for (A,D) comparison.
For problem pair (A, B) comparison, 56% of participants rated A and B as having the same ICL. Eighty-six (86%) of participants rate A and B have the same ECL, and 76% of participants rated that they devoted the same total energy when solving A and B. This means that participants did not perceive the combination of series and parallel circuits (Problem B) as more complicated than the simple series circuit (Problem A). They perceived no distracting information for both A and B. They perceived the same level of germane load for solving these two problems. Notice the percentage for GCL is not close to the percentage of ICL suggesting that GCL and ICL can be differentiated by the measurement.
For problem pair (A, C) comparison, 54% of participants rated A and C have the same ICL. 74% of participants rated A had less ECL than B. Sixty-three (63%) of participants rated the same level of germane load when solving A and C. This means that participants did realize that the A and C are investigating the same underlying principles. They also perceived more distracting information for A than C. Again, the percentage for GCL is not the close to the percentage of ICL suggesting that GCL and ICL can be differentiated by measurement.
For problem pair (A,D) comparison, most (56%) of participants rated A had lower ICL than D. Most (70%) of participants rated A had lower ECL than D, and most (50%) of participants rated the same germane load when solving A and D. This means that participants did realize that combination of series circuit and parallel law is more complicated than the simple series circuit law. They also perceived more distracting information for A than D. Again, the ratings for “A is the same as D” is different for items corresponding to ICL than items corresponding to GCL suggesting that ICL and GCL might be differentiated on a subjective survey.
Discussion
In general, experiment three results are consistent the three-component model of CLT (Sweller, 2010). Specifically, we found that for all problem pair comparisons, participants rated the GCL differently than the ICL, which is consistent with the notion that GCL and ICL should be independent constructs, even though GCL may not provide an independent source of CL as suggested by Sweller (2010) and Kalyuga (2011). In problem pairs with the same ECL level (e.g., A–B), indeed most participants (86%), as expected rated the ECL of the two problems to be the same. In problem pairs with different ECL levels (e.g., A–C and A–D), most participants rated C (74%) and D (70%) as imposing a higher ECL than A as expected. In problem pair (e.g., A–B), where both problems were manipulated to impose low ECL but different ICL levels (e.g., A < B), most participants (56%) rated the ICL level of A and B to be the same. However, in a problem pair (e.g., A–D) where problems were manipulated to impose different ECL (A < D) as well as different ICL (A < D), participants indeed rated A as imposing lower ICL than D. This result demonstrates participants’ perceived differences in ICL might depend on the levels of ECL. When ECL is high, they might have confused ECL with ICL as in the A–D comparison case. Given that these participants were students in an introductory physics class for elementary education majors and were unfamiliar with material before being exposed to it in the class, we can assume that they were low prior knowledge students. Therefore, this result seems to be consistent with the notion that participants may not be able to differentiate ICL from ECL when they have low prior knowledge. This result is consistent with the results for experiment two in this study as well as the theoretical formalism from Sweller (2010), and certainly calls for more research to further understand low prior knowledge learners’ ability to distinguish between changes in ICL and ECL.
General Discussion
In this study, we developed an eight-item cognitive load (CL) survey measuring intrinsic load, extraneous load, and germane load of participants while taking a multiple-choice conceptual physics test. We conducted three experiments to validate the survey.
In the first experiment participants were asked to sort the items into groups according to the common theme. A vast majority of the participants sorted the items consistent with the CLT formalism. Namely, participants grouped items relevant to ICL together, items relevant to ECL together, and items relevant to GCL together. In follow-up interviews we found evidence that participants understand the items on the survey consistent with how ICL, ECL, and GCL were theorized in CLT (Sweller, 2010).
In the second experiment, we administered the CL survey both at the beginning and at the end of an instructional unit on electric circuits in a conceptual physics class for elementary education majors. A PCA revealed a two-component model when knowledge level was low (beginning of the unit) confirming what Sweller (2010) has proposed that low knowledge level participants might not differentiate relevant information from irrelevant information. All the items relevant to ICL and ECL loaded onto one component and all the items relevant to GCL loaded onto another component. A PCA revealed a three-component model when knowledge level was high (end of the teaching unit) confirming what Sweller (2010) has proposed that participants can differentiate relevant and irrelevant information at a high knowledge level. All the items relevant to ICL loaded onto one component; all the items relevant to ECL were loaded onto a second component; and all items relevant to GCL were loaded onto yet another component. This seems to indicate that this survey is better able to distinguish between ICL and ECL on a post-test rather than on a pretest.
In the third experiment, we asked participants to solve four physics problems of varying levels of ICL and ECL. Two of the four problems had the same ICL (low level), the other pair had the same ICL (high level). For each pair of problems with same level of ICL, one had low ECL, and the other had high ECL. After having solved the four problems, we asked participants to compare how they would rate the eight items on the CL survey differently when comparing selected pairs of problems. The results showed that most participants selected the option that they devoted the same amount of GCL to both problems in each of the compared pairs of problems. However, their ratings were not the same as on the items corresponding to ICL suggesting GCL and ICL can be measured separately, contrary to what the theoretical construct suggests (Kalyuga, 2011; Jiang and Kalyuga, 2020). When they were asked to compare a problem of high ECL with a problem of low ECL, they rated ECL as expected. When they were asked to compare a pair of problems of the same ICL, they rated the ICL as expected. When they were asked to compare two problems—one of high ICL with one of low ICL—when both problems had a low ECL, they rated the problems having the same ICL. However, when asked to compare two problems—one of high ICL and ECL with another of low ICL and ECL, they rated the problems as having different levels of ICL (A < D) which might indicate how participants perceive ICL depends on the existence of ECL. When ECL is high, they might have confused ECL with ICL. This seems to be consistent with the idea that participants may not be able to differentiate ICL from ECL when they have low prior knowledge as shown by experiment one in this study as well as the theoretical formalism from Sweller (2010). Overall, the results of the three experiments taken together provide clear evidence supporting the classic theoretical construct of CLT, i.e., a three-component construct (Sweller, 2010). These results also provide clear validation of the CL survey items.
Cognitive load theory proposes a multi-faceted construct of CL. Given the significance of CL in learning and instruction, the measurement of sub aspects of the load is important. This work will be beneficial to assessment designers who are interested in attending to the issues of CL in the design of assessment instruments. Our work adds to the existing literature by developing and adapting a subjective survey for measuring three aspects of CL.
Prior studies have not looked at if their CL survey/items were stable over the progression of students’ learning. This is an overlooked area in the CLT community. This work offers evidence supporting what Sweller (2010) has argued for a long time that students’ capability of differentiating ICL from ECL depends on their knowledge level. This is a challenge for CLT community if we want to measure the three types of CL reliably, we have to take the knowledge level of students into consideration. As for the proper use of the CL survey developed in this work, we suggest using it when students have developed certain level of knowledge. In terms of instruction, when instructors design questions, it usually happens post-instruction when students have already constructed a certain level of knowledge which is a good time for using the survey.
Data Availability Statement
The raw data supporting the conclusions of this article will be made available by the authors, without undue reservation.
Ethics Statement
The studies involving human participants were reviewed and approved by IRB office at Purdue University. The patients/participants provided their written informed consent to participate in this study.
Author Contributions
TZ conceptualized and drafted the initial article and incorporated edits from coauthors. JM conducted interviews for experiment two and drafted the section in the initial article. JM and NR offered suggestions for terms and assisted with editing. NR reviewed and edited the manuscript. All authors read and approved the final manuscript for submission.
Funding
This work was supported in part by the U.S. National Science Foundation Grant No. 1348857.
Conflict of Interest
The authors declare that the research was conducted in the absence of any commercial or financial relationships that could be construed as a potential conflict of interest.
References
Ayres, P. (2006). Using subjective measures to detect variations of intrinsic cognitive load within problems. Learn. Instr. 16, 389–400. doi: 10.1016/j.learninstruc.2006.09.001
Cierniak, G., Scheiter, K., and Gerjets, P. (2009). Explaining the split-attention effect: is the reduction of extraneous cognitive load accompanied by an increase in germane cognitive load? Comput. Hum. Behav. 25, 315–324. doi: 10.1016/j.chb.2008.12.020
Engelhardt, P. V., and Beichner, R. J. (2004). Participants’ understanding of direct current resistive electrical circuits. Am. J. Phys. 72, 98–115. doi: 10.1119/1.1614813
Gerjets, P., Scheiter, K., and Catrambone, R. (2004). Designing instructional examples to reduce intrinsic cognitive load: molar versus modular presentation of solution procedures. Instr. Sci. 32, 33–58. doi: 10.1023/B:TRUC.0000021809.10236.71
Gerjets, P., Scheiter, K., and Catrambone, R. (2006). Can learning from molar and modular worked examples be enhanced by providing instructional explanations and prompting self-explanations? Learn. Instr. 16, 104–121. doi: 10.1016/j.learninstruc.2006.02.007
Hart, S. G., and Staveland, L. E. (1988). Development of NASA-TLX (Task Load Index): results of empirical and theoretical research. Adv. Psychol. 52, 139–183. doi: 10.1016/s0166-4115(08)62386-9
Jiang, D., and Kalyuga, S. (2020). Confirmatory factor analysis of cognitive load ratings supports a two-factor model. Tutor. Quant. Methods Psychol. 16, 216–225. doi: 10.20982/tqmp.16.3.p216
Kalyuga, S. (2011). Cognitive load theory: how many types of load does it really need? Educ. Psychol. Rev. 23, 1–19. doi: 10.1007/s10648-010-9150-7
Kalyuga, S., Chandler, P., and Sweller, J. (1998). Levels of expertise and instructional design. Hum. Factors 40, 1–17. doi: 10.1518/001872098779480587
Kane, M. T. (2013). Validating the interpretations and uses of test scores. J. Educ. Meas. 50, 1–73. doi: 10.1111/jedm.12000
Karpicke, J. D., Lehman, M., and Aue, W. R. (2014). “Retrieval-based learning: an episodic context account”, in Psychology of Learning and Motivation Vol. 61, ed. B. H. Ross (San Diego, CA: Academic Press), 237–284.
Kirschner, P. A., Ayres, P., and Chandler, P. (2011). Contemporary cogni- tive load theory research: the good, the bad and the ugly. Comput. Hum. Behav. 27, 99–105. doi: 10.1016/j.chb.2010.06.025
Leppink, J., Paas, F., Van der Vleuten, C. P., Van Gog, T., and Van Merriënboer, J. J. (2013). Development of an instrument for measuring different types of cognitive load. Behav. Res. Methods 45, 1058–1072. doi: 10.3758/s13428-013-0334-1
Mayer, R. E. (2014). Incorporating motivation into multimedia learning. Learn. Instr. 29, 171–173. doi: 10.1016/j.learninstruc.2013.04.003
Paas, F. G. (1992). Training strategies for attaining transfer of problem-solving skill in statistics: a cognitive-load approach. J. Educ. Psychol. 84:429. doi: 10.1037/0022-0663.84.4.429
Ogilvie, C. A. (2009). Changes in students’ problem-solving strategies in a course that includes context-rich, multifaceted problems. Phys. Rev. Phys. Educ. Res. 5:020102. doi: 10.1103/PhysRevSTPER.5.020102
Roediger III, H. L., and Karpicke, J. D. (2006). Test-enhanced learning: taking memory tests improves long-term retention. Psychol. Sci. 17, 249–255. doi: 10.1111/j.1467-9280.2006.01693.x
Salomon, G. (1984). Television is “easy” and print is “tough”: the differential investment of mental effort in learning as a function of perceptions and attributions. J. Educ. Psychol. 76, 647–658. doi: 10.1037/0022-0663.76.4.647
Sweller, J. (1988). Cognitive load during problem solving: effects on learning. Cogn. Sci. 12, 257–285. doi: 10.1207/s15516709cog1202_4
Sweller, J. (1994). Cognitive load theory, learning difficulty, and instructional design. Learn. Instr. 4, 295–312. doi: 10.1016/0959-4752(94)90003-5
Sweller, J. (2010). Element interactivity and intrinsic, extraneous, and germane cognitive load. Educ. Psychol. Rev. 22, 123–138. doi: 10.1007/s10648-010-9128-5
Sweller, J., Van Merrienboer, J. J., and Paas, F. G. (1998). Cognitive architecture and instructional design. Educ. Psychol. Rev. 10, 251–296.
van Merriënboer, J., and Sweller, J. (2005). Cognitive load theory and complex learning: recent development and future directions. Educ. Psychol. Rev. 17, 147–177. doi: 10.2307/23363899
Keywords: cognitive load, subjective measure, validity, test, content knowledge
Citation: Zu T, Munsell J and Rebello NS (2021) Subjective Measure of Cognitive Load Depends on Participants’ Content Knowledge Level. Front. Educ. 6:647097. doi: 10.3389/feduc.2021.647097
Received: 29 December 2020; Accepted: 12 April 2021;
Published: 10 May 2021.
Edited by:
Fred Paas, Erasmus University Rotterdam, NetherlandsReviewed by:
John Sweller, University of New South Wales, AustraliaPatricia O’Sullivan, University of California, San Francisco, United States
Copyright © 2021 Zu, Munsell and Rebello. This is an open-access article distributed under the terms of the Creative Commons Attribution License (CC BY). The use, distribution or reproduction in other forums is permitted, provided the original author(s) and the copyright owner(s) are credited and that the original publication in this journal is cited, in accordance with accepted academic practice. No use, distribution or reproduction is permitted which does not comply with these terms.
*Correspondence: Tianlong Zu, dGlhbmxvbmcuenVAbGF3cmVuY2UuZWR1