- 1Umeå Science Education Research Group (UmSER), Department of Science and Mathematics Education, Umeå University, Umeå, Sweden
- 2Umeå Mathematics Education Research Centre (UMERC), Department of Science and Mathematics Education, Umeå University, Umeå, Sweden
Although motivation has been shown to have substantial influence on learning, the relative significance of Students’ motivational characteristics, compared to other school-related factors, for student learning and performance is still unclear. Furthermore, knowledge about the relative importance of different situational variables for predicting these motivational characteristics is crucial for educational decisions about how to enhance student motivation. This study examined (1) the relative importance of motivational characteristics derived from five different theories on motivation and epistemic beliefs, compared to almost 300 situational factors, for predicting student performance on the TIMSS 2011 achievement tests in science and mathematics, and (2) how student motivational characteristics can be predicted by the background variables in the TIMSS 2011 questionnaires and an additional questionnaire about motivation accompanying TIMSS in Sweden. Up to 52% of the variation in student performance could be predicted by models containing all background variables, and student motivational characteristics were among the most important variables in the model. Models that comprised only student motivational characteristics from several motivation theories predicted up to 27% of student performance on the achievement test, while models using only single motivational characteristics predicted, on average, 7%. Results emphasize teachers’ importance for student motivation. Five teacher features were consistently among the most important variables in predicting Students’ motivational characteristics. These five variables predicted as much of the variation in important student motivational characteristics as the remaining 300 situational variables together.
Introduction
Motivation is a prerequisite for learning and achievement as it instigates action and sustains and directs behavior. It is also associated with emotions which, in turn, affect memory functioning, and cognitive focus (attention) (Fredrickson and Branigan, 2005; Izard, 2007; Mikels et al., 2008). Thus, research on motivation is highly relevant to understand how Students’ adaptive behaviors, emotional wellbeing, and learning can be supported in school. However, motivation is a complex phenomenon, manifested in the abundance of theories that together comprise a wide range of variables proposed to affect the amount and character of motivation. Examples of such variables are: psychological needs of competence, autonomy and, relatedness (Deci and Ryan, 1985; Ryan and Deci, 2000, 2020), images of an “ideal self” (Carver and Scheier, 1990; Carver and Scheier, 2002), achievement goals (Elliot and Thrash, 2001), causal attributions (Weiner, 2000), value beliefs and expectations of success (Eccles et al., 1983; Wigfield and Eccles, 2000; Eccles and Wigfield, 2020). There is a wealth of studies examining the relationship between different motivational constructs and a wide range of “external” variables like, for example, emotions (Goetz et al., 2016), achievement (Chatzisarantis et al., 2016; Lüftenegger et al., 2016; Mouratidis et al., 2018), reading amount (Troyer et al., 2019), and epistemic beliefs (Winberg et al., 2018). Most of these studies take a single theoretical perspective on motivation, which may facilitate design of, for example, interventions, and theoretical clarity.
However, it has been shown that single theory approaches often predict only low or moderate amounts of Students’ achievement (e.g., Hulleman et al., 2010). Moreover, as motivation theories often are both overlapping and complementary, a single-theory approach may not only preclude us from drawing ecologically valid conclusions about the significance of different aspects of Students’ motivation for their engagement and learning, but also prevent us from distinguishing critical elements of the inherently multivariate educational practices to support Students’ motivation (Linnenbrink-Garcia et al., 2016). Different theories may provide complementary information about individuals’ motivation, hence increasing our ability to predict and understand their behavior and achievement. People simultaneously have certain amounts of, for example, performance goals, mastery goals, intrinsic motivation, expectancy of success, and different types of extrinsic motivation and value beliefs. It is reasonable to expect these aspects of Students’ motivational processes to act not as separate entities, but as a system of variables that exert a joint influence on Students’ behavior and cognition. Therefore, although previous single-theory approaches have generated invaluable information on the functioning of separate aspects of Students’ motivation, research on their joint influence is warranted. Due to the partially complementary nature of motivation theories, it is reasonable to expect such multi-theory models to be better at explaining different aspects of students learning than single-theory models. However, little is known about exactly how much better they are (i.e., to what degree theories are complementary) and the relative contributions of theories to the models’ ability to explain different outcomes. Moreover, in an authentic school situation, there are many other factors that may influence student motivation and learning in school, for example, the support and attitudes of parents, how teaching is organized (e.g., frequency of homework) and what goals are communicated through the teaching (e.g., school emphasis on academic success) (Martin et al., 2012). Hence, when making decisions about educational improvements, knowledge about the importance of different aspects of Students’ motivation for Students’ achievement in relation to other school-related variables is essential for efficient interventions.
Furthermore, if Students’ motivational characteristics are important, it is essential to find the situational variables in the learning environment that are most conducive to the prediction of motivational characteristics. Similar to the lack of multi-theory approaches to investigate the influence of motivation, there is little research on the joint influence of multiple supports for Students’ motivation. Linnenbrink-Garcia et al. (2016) argued that this limits researchers’ ability to provide efficient recommendations for educational practices and, consequently, called for more integrative approaches to this issue.
The present paper aims at investigating the importance of motivational variables from several contemporary theories of motivation, in relation to a wide range of other school related variables, for explaining student achievement in mathematics and science. The study includes comparisons between single-theory and multi-theory prediction models. In addition, the study examines the relative importance of a very large number of situational variables pertaining to home and school environment for the prediction of Students’ motivational characteristics. Hence, the present study adds to the literature on the importance of motivational characteristics for the prediction of academic performance and how Students’ motivation can be supported in authentic, multivariate, learning environments. The study is exploratory in its nature because (1) it is not feasible to formulate hypotheses about specific relationships between the large number of variables included in the study and (2) little is known about many of possible relationships between the included variables, although they all are theoretically relevant for explaining Students’ motivation and achievement.
Studying these relationships requires a very large number of survey items to provide valid and reliable measurements of the variables involved. In addition, large and carefully selected student samples are necessary to yield results that are of interest for decisions about school in a wider population. In this study, these conditions are met by using the Swedish data from a Trends in International Mathematics and Science Study (TIMSS). The TIMSS studies comprise nationally representative samples of students and aim at gaining “a deeper understanding of the effects of policies and practices across countries’ different systems of education” (Martin et al., 2012, p. 6). TIMSS include student achievement data and comprehensive student, parent, teacher, school, and curricular background data generated through questionnaires to students, teachers, and principals. This data is intended to provide “vital information on key curricular, instructional, and resource-related factors that can impact the teaching and learning process” (Martin et al., 2012, p. 2). In the present study, we have used the Swedish data from TIMSS 2011. The reason for this choice is that in this specific TIMSS study an extra questionnaire, focusing exclusively on the eighth-grade Students’ motivation and beliefs about learning, was included for the Swedish sample. This allows for a more fine-grained exploration of student motivation and its role for achievement than using data from other TIMSS studies.
Research Questions
The background leads us to the following research questions:
1. How important are motivational characteristics, in comparison to other variables in TIMSS 2011 questionnaires, for predicting Swedish student results on TIMSS achievement tests?
2. Which situational variables, measured in this study, are the best predictors of student motivational characteristics?
Situating Study Variables in Theories of Motivation
The items in the TIMSS questionnaires pertain to student motivational characteristics, Students’ perceptions of the teacher and the teaching, and many situational aspects of the school and teaching (e.g., teachers’ educational level, or extent of bullying at school). Most of these items are included in the present study as individual items. Some items have been considered by TIMSS to measure the same underlying construct and have therefore been grouped together accordingly. The extra questionnaire, accompanying the ordinary TIMSS package, includes items that form an additional number of variables describing Students’ motivational characteristics and situation variables from well-known motivation theories. In the following, the motivation constructs in the extra questionnaire and those formed from the items in the ordinary TIMSS questionnaires are described and situated in theories of motivation. The names of the variables included in the study are italicized. Examples of questionnaire items belonging to each of these variables are provided in the methods section. The purpose of situating the variables in the motivation theories to which they pertain is to describe their function in motivated behaviors and justify their inclusion in the study. The purpose of the study is to explore the relative importance of different variables for motivation and achievement, and not development of the individual theories.
Motivational Variables
The motivational variables pertain to five different theories of motivation and epistemology: Expectancy value theory, Self-determination theory, Attribution theory, Achievement goal theory, and theory on Epistemic beliefs. They represent some of the most prominent contemporary theories of motivation and individuals’ thinking about knowledge. More important, they constitute complementary parts of on the motivational process, as we will discuss below.
Expectancy-value theory
Expectancy value theory (EVT; Eccles et al., 1983; Wigfield and Eccles, 1992; Eccles and Wigfield, 2020) is one of the most comprehensive motivation theories, considering not only the individual’s perceptions of task value and probability of succeeding on the task, but also a wide range of variables influencing these perceptions and, ultimately, the resulting choices and behavior of the individual. As such, it offers an umbrella under which many theories on motivation can be incorporated (Eccles and Wigfield, 2020). Although the motivation theories used in this study are treated in their own right, Eccles and Wigfield (2020) argue that they all are important for a fuller understanding of individuals’ behavior as they explicitly or implicitly pertain to different parts of the network of interpretational, evaluative, and motivational factors and processes that constitute the EVT model.
According to EVT, expectancies of success and values are the two main determinants of motivated behavior. Expectancy of success can be defined as individuals’ “beliefs about how well they will do on upcoming tasks, either in the immediate or longer term future” (Wigfield and Eccles, 2000, p. 7), which is closely related to Bandura (2010) self-efficacy, defined as an individual’s belief that she can produce desired effects by her actions. Neither expectancy of success, nor self-efficacy are measured in TIMSS 2011. However, questions about Students’ ability beliefs are included. These ability beliefs are distinguished conceptually from expectancies of success and self-efficacy as they focus on present ability while expectancies and self-efficacy are future oriented. Moreover self-efficacy and expectancy for success are task specific, while ability beliefs pertain to a more general sense of competence in an area. Nevertheless, in terms of predictive patterns, ability beliefs, expectancies of success, and self-efficacy seem empirically closely related, especially within a domain such as mathematics (Wigfield and Eccles, 2000). Hence, for the sake of reducing the load on the participants in the study, we chose not to add the concepts of self-efficacy and expectancy for success, but to rely on the extant TIMSS measure of ability beliefs. These are measured by several items in TIMSS, brought together in this study to form the variable called Perceived Competence. Value is the other proximal determinant of motivation in EVT, comprising attainment value (importance), incentive (intrinsic value), and utility value (usefulness of the task) (Eccles et al., 1983; Wigfield and Eccles, 2000). While utility value and incentive value are measured through a number of items in the ordinary TIMSS questionnaire, there are no items pertaining to attainment value. Therefore, attainment value was included in the extra questionnaire.
In EVT, expectancies and values are assumed to be influenced by task-specific beliefs, such as ability beliefs, the perceived difficulty of the task, and the individual’s goals, self-schema, affective memories of previous tasks, and the perceived causality of the outcomes of these previous tasks. These social cognitive variables, in turn, are influenced by a variety of “socialization influences” (Wigfield and Eccles, 2000, p. 69). The importance of the influence of the social context, especially the role of parents and the school environment, for the appraisals and the options individuals consider in a specific situation was further emphasized in a recent update of the EVT model. The model was renamed SEVT by Eccles and Wigfield (2020) to illustrate this expansion. Many of these socialization influences are addressed in TIMSS (see examples under the heading Situational Variables) as they are assumed to contribute substantially to the explanation of Students’ achievement on the TIMSS achievement test. The SEVT provides theoretical support for this assumption.
Attribution theory
The causes students attribute to their past achievements is one example of an important social cognitive variable in SEVT that is measured in the complementary questionnaire. There are many possible causes for the outcome of an activity, but they can all be categorized by their underlying properties (Weiner, 1985, 2000). Three central property dimensions have been found–locus of causality, stability, and controllability–posited to affect expectancy of future success and value. The extra questionnaire includes items to which students are to indicate the extent they perceive the causes of successful and unsuccessful test results to be their efforts, aptitude, and test difficulty, respectively. Controllability was the only dimension that could be discerned in the statistical validation process (see the “Materials and Methods” section). Controllability beliefs has been shown to be related to emotions and the perceived value of achievement outcomes (Weiner, 2000), and such affects may influence future behavior (Scherer, 2005), for example, via the “value box” in expectancy value theory.
Self-determination theory
Motivation can vary both regarding the level (i.e., how much motivation), and the type of motivation (Ryan and Deci, 2020). The type of motivation refers to why the action is performed. Thus, different types of motivation can be distinguished, based on the reasons or goals behind an action. A basic distinction is between intrinsic motivation, which refers to doing an activity because it is experienced as interesting or enjoyable, and extrinsic motivation, which refers to doing something because it leads to a separable outcome. Thus, extrinsically motivated behaviors are undertaken and sustained because of expectancies of the outcome of the activities (e.g., a good grade). According to Self-determination theory (Deci and Ryan, 1985; Ryan and Deci, 2000, 2020) there are also different forms of extrinsic motivation. Different forms of extrinsic motivation differ in the extent to which a value or regulation, through a process of internalization, is taken in by an individual and transformed into their own so that it will emanate from their sense of self. In other words, extrinsic motivation differs in the degree to which it is autonomous. The least autonomous form of extrinsic motivation is entitled external regulation. Such motivated behaviors are performed to satisfy an external demand or obtain an externally imposed reward contingency (e.g., work on a task because a student has been told to do so by the teacher). A second form of extrinsic motivation is introjected regulation. Introjection refers to a partial internalization in which external regulations are taken in by the individual but are not accepted as his or her own. Introjected behaviors are experienced as being pressured by interpersonal or intrapsychic contingencies or demands, such as the feeling that the individual must achieve high grades to be a worthy person (Ryan, 1982). Although the regulation is internal to the person, introjected behaviors are not experienced as fully part of the self and thus still have an external perceived locus of causality. Identification refers to a fuller internalization in which the person identifies with the value of a behavior and accepts its regulation as his or her own. Through identification the person experiences a greater sense of choice, less internal conflict, and more responsibility for initiating and maintaining the behavior. Finally, integrated regulation occurs when identified regulations have been fully assimilated to the self. This occurs through self-examination and bringing new regulations into congruence with the individual’s other values and needs (Black and Deci, 2000; Ryan and Deci, 2000). A substantial amount of research has shown that these different forms of motivation (different forms of extrinsic motivation and intrinsic motivation) are associated with differences in both well-being and performance (Ryan and Deci, 2020). Hence, self-determination theory and expectancy-value theory overlap in the sense that both models include perceived value as driver of behavior. However, SDT expands this further by differentiating between intrinsic value and between different levels of internalization of extrinsic values (e.g., utility and attainment value). Moreover, SDT makes explicit the role of situational factors for the internalization of extrinsic norms and values (e.g., factors that are conducive to the satisfaction of the basic psychological needs of competence, relatedness, and autonomy). Intrinsic motivation and different forms of extrinsic motivation are measured in the complementary questionnaire by items adapted from the Academic Self-Regulation Questionnaire (SRQ-A), previously validated by Ryan and Connell (1989).
Achievement goal theory
Achievement goal theory is a strand within motivation research that focuses on how individuals’ goals influence performance and wellbeing. However, it seems that researchers have not been able to agree upon any clear definition of the achievement goal construct. Elliot and Thrash (2001) pointed out that there seems to be at least two approaches; one that focuses on the specific purposes of an individual’s engagement in an activity, and one that considers achievement goals as a system of several interrelated constructs that together form a general achievement orientation. In this study the “purpose approach” advocated by Elliot and Murayama (2008) is adopted which focuses on the explicit goals themselves. Within the “purpose approach,” goals are most often divided into mastery and performance goals. Performance goals are commonly conceptualized as driven by a desire to demonstrate competence, paired with a normative standard for evaluating competence, while mastery goals focus on the development of competence evaluated against either a task-based or intra-personal standard. While both mastery and performance goals are considered to have an approach and avoidance aspect (Elliot and Thrash, 2001), a trichotomous model have been argued to produce the most consistent results (Lau and Nie, 2008) and is frequently used in educational research (Murayama and Elliot, 2009; Vedder-Weiss and Fortus, 2012). In this model the mastery goal construct comprises only the approach dimension, which henceforth will be named mastery goal, while the performance goal construct includes both approach and avoidance goals. Recently, researchers have further distinguished between the standard and standpoint subcomponent of achievement goals. While the standpoint subcomponent focuses on whether the students pursue the goals to develop (mastery) or demonstrate (performance) competence, the standard subcomponent focuses on whether competence is evaluated against a task- or self-based (mastery) or an other-based (performance) point of reference (Elliot and Hulleman, 2017). The relative merits of the different achievement goals for Students’ achievement have for long been subject for discussion. In a review of over 90 studies, Linnenbrink-Garcia et al. (2008) showed that mastery goals and performance-approach goals in general are positively correlated with achievement, although mastery goals were argued to be more adaptive than performance approach goals when solving challenging tasks. In contrast, Senko (2019) found that mastery goals were associated with interest-based studying strategies and negatively related to achievement on closed- format tests, while performance approach goals (measured using the standard standpoint) were associated with vigilant study strategies and predicted high achievement when learning goals were clear. No positive effects were found for performance goals when learning goals were unclear, and for mastery goals on open-ended tests. However, researchers tend to agree that performance-avoidance goals are negative for achievement (Hulleman et al., 2010).
There are several points at which achievement goal theory align with the other theories in this paper. For example, researchers have found that considering to what extent Students’ reasons for pursuing different achievement goals are autonomous or controlled enhances the predictive ability of the achievement goals (Michou et al., 2014; Urdan and Kaplan, 2020). Moreover, attributions to controllable causes, for example effort, have been shown to influence mastery goal adoption (Song et al., 2020). In EVT, goals influence the individuals value appraisals of an activity (Eccles and Wigfield, 2020). For example, it is reasonable to expect individuals having performance-approach goals to ascribe high intrinsic and attainment value to learning activities on which they expect to perform well and the outcome has consequences for their appearance in the eyes of their peers, while tasks that do not have social consequences may not be perceived equally important or stimulating.
The complementary motivation questionnaire includes items representing the three dimensions of the trichotomous model (i.e., Mastery, Performance approach, and Performance avoidance goals). Research using the trichotomous model has used both the standard and standpoint subcomponents, either separately or together (Elliot and Hulleman, 2017). Although this has contributed to some of the ambiguity regarding the relative merits of the different goals, Senko and Tropiano (2016) argue that neither approach is clearly superior on theoretical grounds and that studying them together should be a more fruitful approach, capitalizing on their respective strengths. Elliot and Hulleman (2017) pointed out that combining the standard and standpoint components of performance goals could accentuate the negative implications of performance goals and therefore, through reduced satisficing (Podsakoff et al., 2003), lead to increased predictive power. For this paper, we therefore combined items from the Revised Achievement Goal Questionnaire (AGQ-R, Elliot and Murayama, 2008) and the Patterns of Adaptive Learning Scales (PALS; Midgley et al., 2000) to capture both the standard and standpoint subcomponents, similar to Korn et al. (2019). This combined measure has recently been validated in the Swedish context by Hofverberg and Winberg (2020b).
Epistemic beliefs
Epistemic beliefs are beliefs about knowledge and the process of knowing. Although epistemic beliefs are not always considered as a “motivational construct,” studies indicate that these beliefs are associated with Students’ achievement goals (Ricco et al., 2010; Winberg et al., 2018; Zhou et al., 2019) as well as their leaning behavior (Lindfors et al., 2017). There are also several models of motivation that implicitly incorporate Students’ thoughts about knowledge and knowing. For example, Muis (2007) argued that epistemic beliefs are intertwined with the motivational and cognitive conditions that influence Students’ task definition, affecting goal setting, planning, and eventually, enactment and achievement. In a similar vein, Wigfield and Cambria (2010) argued, in relation to the expectancy value model (Wigfield and Eccles, 2000), that individuals perceive tasks as important when they “… view them as central to their own sense of themselves, or allow them to express or confirm important aspects of self” (p. 4). Hence, a learning situation that aligns with the Student’s beliefs about the nature of knowledge would be perceived as more meaningful than one that does not, which would also result in a stronger sense of self-determination and more autonomous forms of motivation (e.g., identified, integrated or intrinsic regulation) (Ryan and Deci, 2020). Furthermore, in the expectancy-value model (Wigfield and Eccles, 2000; Eccles and Wigfield, 2020), the principal instigators of motivated behavior, task-value, and expectancies of success are determined by a wide range of cultural and social factors, including the individual’s own and socializers’ subject stereotypes. We argue that beliefs about knowledge and learning are part of these subject stereotypes, influencing the individuals view of themselves, and hence their appraisals of the value of a learning activity.
Hofer (2004) proposed two basic themes to constitute the core of epistemic beliefs: the nature of knowledge and the process of knowing, both comprising two sub dimensions, as shown below.
Nature of Knowledge
Certainty of knowledge, ranging from believing that absolute truth exists to a view that knowledge is preliminary and changing as we come to know more about something. Simplicity of knowledge, ranging from considering knowledge as discrete facts to a belief that knowledge is complex (facts are interrelated) and contingent to the specific circumstances of the situation.
Process of Knowing
Source of knowledge, perceptions that knowledge resides in authorities (and can be transferred to the learner), vs. actively constructed by the learner.
Justification of knowledge, pertains to the learner’s grounds for justifying or evaluating her or other’s knowledge, ranging from justification based on authority, or feelings, to critical evaluations of empirical evidence or statements by authorities.
To limit the load on the respondents, it was only possible to include one epistemic belief sub construct in the complementary TIMSS questionnaire. The simplicity construct was chosen because the item loading pattern showed better alignment with theory and higher stability between the validation rounds than the other epistemic beliefs constructs. Interviews with students in the validation studies also indicated fewer “alternative interpretations” of the items in this construct. It has also been shown to predict both motivation-related and cognitive aspects of learning, e.g., emotions during learning (Winberg et al., 2014) and information comprehension (Bråten and Strømsø, 2010).
Situational Variables
In this section some of the variables pertaining to the learning environment and Students’ perception of this environment are described. These variables may have explanatory power for student performance on the TIMSS achievement test (Research Question 1) but may also predict the student motivational characteristics (Research Question 2).
The type of motivation a student has for learning a subject partly depends on individual characteristics. However, it also depends on the learning situation, which can be influenced by the teacher. According to self-determination theory, individuals are motivated to act in ways they perceive may fulfill the three basic psychological needs for competence, autonomy, and relatedness (Ryan and Deci, 2000, 2020). Although all three are considered important in SDT, intrinsically motivated behaviors that satisfy the needs for competence and autonomy are considered “the prototype of self-determined behavior” (Ryan and Deci, 2000, p 65). Although the relative importance of the basic psychological needs still is under discussion, some evidence exists that fulfillment of the need for competence and autonomy is particularly important for academic achievement (e.g., Marshik et al., 2017).
hus, support for student autonomy is a central characteristic of the learning context to promote or undermine different forms of motivation. If a learning context is to support Students’ intrinsic motivation or productive forms of extrinsic motivation it must support Students’ opportunities to act in accordance with their own interest (intrinsic motivation) or identified or integrated values (extrinsic motivation) (Ryan and Deci, 2000). This means that students need to receive support for their decision-making and to feel that there is scope for making own choices in their learning. When their behavior is affected by extrinsic sources, such as the teacher telling them to work in groups or in their textbooks, they need to feel that their teacher considers and understands their perspective and needs, and they must have identified the value that these activities may be appropriate. However, complementing such teacher activities in the classroom with activities that ensure that there is a good working climate and that the students are learning (structure) may be important for some students. Both autonomy support and structure are variables included in the additional questionnaire. Autonomy support was measured by the short version of the Learning Climate Questionnaire (LCQ) (Black and Deci, 2000), while items for the structure construct were developed specifically for the complementary questionnaire. Support for Students’ sense of competence is measured in the ordinary TIMSS questionnaires. As there was a strong pressure to keep down the number of items in the extra questionnaire, and the possibly less central role of relatedness for academic achievement, the relatedness construct was not included in the extra questionnaire.
In addition to the variables in the extra questionnaire, presented above, the TIMSS questionnaires include an extensive range of items and constructs, aiming at describing “…procedures and practices that have been shown to be effective in increasing achievement in mathematics and science” (Mullis et al., 2011, p. 93). The TIMSS questionnaires were distributed to students, teachers, and principals, respectively. The covered areas were:
For students
• Students’ home and school lives
• basic demographic information
• home environment
• school climate for learning
• self-perception and attitudes toward mathematics and science
For teachers
• teachers’ background
• views on opportunities for collaboration with other teachers
• job satisfaction
• education and training
• professional development
• instructional time for the classes tested in TIMSS
• materials and activities for teaching and promoting Students’ interest
• use of computers, assessment practices, and homework
For principals
• school characteristics
• instructional time, resources and technology, parental involvement
• school climate for learning, teaching staff, the role of the principal
• Students’ school readiness
It is not possible, in this paper, to give an account of all the situational variables in the TIMSS 2011 survey. Instead, for the justifications of the variables selected for the TIMSS questionnaires, we need to refer the reader to Mullis et al. (2011). A full display of the items can be found at the homepage of the International Association for the Evaluation of Educational Achievement (IEA, 2011b), where the questionnaires are available online. For detailed information on the constructs and scales created by TIMSS that were used in the present study we refer to Martin et al. (2011) and the IEA webpage (IEA, 2011a).
Materials and Methods
As mentioned earlier, due to the broad range of included variables, and the inherent complexity of their relationships, this study aims to explore these relationships rather than to test hypotheses pertaining to a smaller subset of variables.
Sample
In total, 5573 eighth grade students [48% female, age 14 (96%) or 13 (4%)] from 153 schools completed the TIMSS 2011 achievement test and questionnaires in Sweden. A nationally representative sample of schools was achieved by stratified sampling from all Swedish schools that had Grade 8 classes. The selection was made by the International Association for the Evaluation of Educational Achievement (IEA) in cooperation with the national research coordinators. Thereafter, one or two classes from each of the selected schools were chosen at random. All students in the chosen classes were asked to participate. However, students with cognitive or physical disabilities and students who were not proficient in the native language were excluded, according to TIMSS international standards. The resulting sample should provide for a standard error less than 0.035 standard deviation units for the country’s mean achievement. As it is beyond the scope of this paper to give an exhaustive account of the sampling procedure, we refer the interested reader to Joncas and Foy (2011) for details.
Within the frame of the TIMSS main study, the students completed a test of their knowledge and skills in mathematics and science (se next section) and a questionnaire concerning their motivation for learning and their perceptions of their learning environment (described in the background section). Questionnaire data regarding the school and the teaching was also collected from the headmasters and science and mathematics teachers at each school.
In addition to the TIMSS main study, students also completed a complementary questionnaire to expand the information about their motivation and perceived learning environment in either chemistry, physics, biology, or mathematics (hence, approximately 1390 students per subject).
Achievement Estimation by Plausible Values
In TIMSS 2011, a matrix sampling design was used for the collection of nationally representative achievement data (Joncas and Foy, 2011). A total of 217 test items were distributed on 14 different booklets, for mathematics and science, respectively. Each student completed one booklet in mathematics and one in science, comprising 12–18 items each. Since each student is only tested on a subset of all items the measurement of individual proficiency comes with a substantial amount of measurement error. To address this issue TIMSS uses a plausible value methodology by which five imputed scores are generated for each student. These plausible values are based on the Students’ responses to the items they receive, and other relevant background data from the questionnnaires. Plausible values are not intended to be the best estimates of the proficiency of specific individuals, “but rather are imputed scores for like students—students with similar response patterns and background characteristics in the sampled population” (IEA, 2011c, p. 6). A detailed account of the TIMSS scaling methodology is given in IEA (2011c). In this study, in accordance with TIMSS methodology, all analyses pertaining to the prediction of Students’ performance on the TIMSS achievement test were performed five times, once for each plausible value, and the reported results are averages from these five replications.
Data Analysis
In accordance with TIMSS methodology (Martin et al., 2011, p. 6) Principal Component Analysis (PCA) (Abdi and Williams, 2010) was used for the validation of constructs, and to summarize the participants’ responses to the items within each construct (i.e., a Student’s score is the Student’s coordinate value on the model component(s), that in turn is a linear weighted combination of the original variables). Participants’ scores on the principal components defining the constructs were subjected to Orthogonal Projection to Latent Structures analysis (OPLS) (Trygg and Wold, 2002) for analysis of the relative importance of motivational and situational variables for predicting student achievement (Research question 1) and the relative importance of situational variables for predicting Students’ motivational characteristics (Research question 2). The analyses were performed in the SIMCA P+ software (Umetrics, 2013), using the modified non-linear iterative partial least squares regression algorithm (NIPALS; Wold, 1975; Nengsih et al., 2019) for imputing missing data.
OPLS is a development of the well-known Projection to Latent Structure technique (PLS) (Abdi, 2010). PLS is a method for dimension reduction in multivariate regression, aiming at removing any multicollinearity from the predictor variables while finding the optimal set of latent variables (i.e., PLS components) to explain the covariance between original independent variables and the dependent variable. For the interested reader, Mateos-Aparicio (2011) offers an accessible depiction of the origin and applications of PLS. In PLS, the variation in the independent data (X) is divided into two parts, systematic variation and noise (residuals) and the systematic variation is modeled by a number of latent variables (components). At the same time, the relationship (i.e., covariance) between the systematic variation in X data and the dependent data (Y) is maximized. Since the systematic part of X data contains variation that is predictive of Y as well as variation that is not (i.e., orthogonal), interpretations of the relationships between X and Y may be difficult when orthogonal variation is substantial. In OPLS, otherwise similar to PLS, the systematic variation in X that is predictive of Y is separated from the orthogonal variation. This provides improved model interpretability and predictive ability, compared to PLS, since the predictive components are not contaminated by irrelevant variation. OPLS also improves detection of outliers due to eliminated influence of orthogonal variation on the calculations of, for example, Hotelling T2 or DmodX (described below) statistics (Trygg and Wold, 2002). PCA and OPLS are well suited for discerning trends and patterns in large datasets and cope well with “noise” and multicollinearity between variables—features that are common in questionnaire-based data and increasing with the number of variables. In this study PCA was chosen for the combined purpose of validating constructs and summarizing Students’, teachers’, and principals’ responses since it offers a convenient way to export student scores on these constructs for multivariate regression in OPLS. The OPLS approach is also consistent with a view that the predictors act as a system of interrelated variables that have a joint effect on outcomes, rather than a set of variables with additive individual effects. This also makes OPLS less sensitive to multicollinearity between variables, which tend to increase with the number of variables. In the present study, multicollinearity among predictor variables prevented the use of methods such as multilevel modeling and ordinary least squares regression, for example, multiple linear regression including dummy variables or with ad-hoc adjustments of standard errors (e.g., by using design effect measures).
In what follows, an overview is provided of the procedures used for analyzing data in relation to the different research questions, and details on the criteria used for validating variables and prediction models.
Schematic Description of Workflow
Steps common to all research questions
1. Distribution of the motivation questionnaire, the standard TIMSS background questionnaires, and achievement tests.
2. Merging data from the student, teacher, and school (principal) questionnaires and the student achievement datasheet.
3. Validation of constructs by PCA (Table 1). Students’ scores on the constructs were used in the subsequent analyses.
Additional steps pertaining to research question 1 (continuing from step 3)
For each subject, Students’, teachers’, and principals’ scores on the PCA constructs (step 3), and their answers on the separate items in the questionnaires that were not part of any construct, were subjected to orthogonal partial least squares analysis (OPLS) to assess their relative importance in the five consecutive predictions of Students’ Plausible Values. To obtain a clear picture of the relative importance of the different constructs and items for describing Students’ plausible values in the prediction model, the average “variable importance for projection value” (VIP) was calculated for each construct and item (Table 3).
For each subject, constructs describing student motivational characteristics were subjected to OPLS to investigate their ability to separately predict Students’ plausible values. The respective constructs were then entered into a OPLS top model to assess the ability of motivational characteristics to jointly predict Students’ five plausible values in the respective subjects. “Top model” refers to a hierarchical OPLS model where Students’ scores on the components of lower-level PCA models (e.g., describing their motivation type, epistemic beliefs, and so forth), instead of their responses to the original questionnaire items, are used in the regression. See Table 4 for statistics of these models.
Additional step pertinent to research question 2 (continuing from step 3)
Students’ motivation characteristics, described by their scores on the PCA models generated in step 3, were regressed on the situational variables from the student, teacher, and school questionnaires by OPLS. Models were calculated for each subject and the average importance of each situational variable for predicting the respective motivational characteristic was calculated (Table 6).
In all analyses, data were scaled to unit variance (UV) and mean-centered to reduce the impact of differences in variance between variables. This is regarded as the most objective approach when there are no a priori assumptions regarding the significance of the variables (Eriksson et al., 2006). In TIMSS, response scales in some cases have different range, as do Students’ score vectors in the different constructs calculated either by TIMSS or us. Furthermore, even if scales are of the same range, the variance of Students’ responses to two items/variables may differ. If left unscaled, variables with large variance would gain undue importance in the prediction models and there is a risk of neglecting potentially important variables with small, but systematic, variance.
Determining the Relative Importance of Variables in Prediction Models
Due to multicollinearity between predictor variables, variable importance for projection values (VIP) rather than regression coefficients of individual predictor variables have been used throughout the paper to indicate the relative significance of individual variables in the OPLS prediction models. Several methods for determining relative importance of collinear predictor variables exist; normally categorized as filter, wrapper, or embedded methods (Mehmood et al., 2012). Given the large number of variables involved in our study, we judged filter methods, to which VIP belongs, as the most appropriate due to their low computational load. Furthermore, both wrapper and embedded methods increase the risk of model overfitting, which would reduce the generalizability of the results. VIP values have been shown to perform very well compared to statistics provided by other regression methods, such as Lasso (embedded method) or Stepwise regression (wrapper method), for identifying important predictors. VIP is less sensitive to the proportion of relevant predictors, magnitude of correlation between predictors, structure of regression coefficients, and the amount of noise in the data (Chong and Jun, 2005). Also, while wrapper and embedded methods generally focus on identifying a “best subset” of predictor variables, VIP provides a ranking of individual variables, which is in line with the intention of this paper. VIP values are obtained by summarizing the squared normalized loadings of each variable on the predictive components in the OPLS model, weighted by the proportion of the variation in the outcomes (i.e., plausible values or motivation variables) that is predicted by the respective components (Galindo-Prieto et al., 2015). Hence the VIP values include variable inter-dependency in determining variable importance for projection. Variables with VIP > 1 are generally considered important (Eriksson et al., 2006; Gosselin et al., 2010), although cut-off values as high as 1.2 have been identified as “proper” when the proportion of important variables is low, or variables are highly correlated (Chong and Jun, 2005). In this paper, we use VIP > 1 as cut-off. Confidence intervals of the VIP values were calculated by Jack-knifing (Efron and Gong, 1983), using the multiple models on subsets of data generated in the cross-validation procedure (see below).
Validation and Reliability Testing of Models
Cross validation (Eastment and Krzanowski, 1982; Van der Voet, 1994) was used to decide on the number of components to retain in the PCA analyses of construct dimensionality and in the OPLS prediction models. In this procedure one seventh of the data is systematically left out and predicted by the remaining data until all data has been left out once, and the precision of each prediction has been calculated. The precision of this prediction is denoted Q2. Thus, Q2 can be considered as a measure of how well the model can predict new data that are not part of the model, while the R2 is a measure of the proportion of the variation that can be described by the model (i.e., how well the model fits existing data). In PCA models the default limits for Q2 corresponds to a significant reduction of prediction residuals, while in OPLS models they reflect the ability of the model to predict a significant amount of the variation in outcomes. In both cases significance is on the 95% level. For PCA models in Step 4 (validation of constructs) that did not have enough predictive ability to reach the default Q2 limits in SIMCA P+, decisions on whether the construct should be used in subsequent OPLS analyses were based on (1) whether the dimensionality and correlation structure between items was intelligible in relation to the theoretical underpinnings of the construct or not, (2) if the model could describe a substantial proportion of the variation in students responses to the items, (3) if eigenvalues of constructs > 1, and (4) if the correlation structure between items within a construct were similar between piloting rounds (data not shown). In total, four piloting rounds (not shown) had been performed before inclusion of items in the TIMSS complementary questionnaire. These piloting rounds included approximately 200 students in each round. Between each round, we performed qualitative analysis of PCA loading patterns, followed by interviews with students on their interpretations of items that did not show expected correlation patterns. After revision or deletion of items, items were tested again, until all items showed expected loading patterns.
To assess the risk of the OPLS models being spurious, i.e., fitting the data well but predicting new observations well purely by chance, Cross validation ANOVA (CV-ANOVA; Ståhle and Wold, 1990) was performed on all OPLS models. CV-ANOVA uses the residuals from the cross-validation procedure described above to formally assess whether they are significantly smaller than just the variation around the mean of the dependent variable. The benefit of CV-ANOVA is that it provides a straightforward measure of the model’s reliability while also producing results consistent with other reliability/significance tests such as response permutation (Eriksson et al., 2008). Distance to Model (DModX) analysis and Observation Risk Analysis were performed to assess any undue leverage of single observations. While the first measure gives information on whether an observation should be regarded as an outlier or not, the latter is an estimate of the effect of a single observation on the models’ predictions (i.e., the residuals of the model when the observation is part of the model compared to when it is not). The value of DmodX for an observation is proportional to its residual standard deviation, normalized by the pooled residual standard deviation of the X space (predictor variables). The critical value of DmodX is calculated from the F-distribution. An observation was considered an outlier if the DmodX value was more than twice as large as the critical value. Observation risk is computed from the difference in residual standard deviation of the predicted variable when the observation is part of the model and when it is not. An observation risk of 1 means that there is no difference in residuals. Observations with an observation risk exceeding 1.5 were excluded and new models were computed and compared to the original model. In no case did the loading VIP values for the variables change after excluding these observations (normally less than 5 per model).
Validation of Constructs
Results show that all constructs but those pertaining to Students’ attributions were valid according to the cross-validation procedure (Table 1). The constructs were equally well modeled in all subjects. Correlation structures were consistent with theory in all constructs and showed similar patterns between subjects. The attribution constructs—stability, locus, and controllability—did not separate into distinct dimensions. Instead, two orthogonal dimensions were found, measuring controllability vs. non-controllability. Only the dimension measuring controllability was used in subsequent analyses. This variable (Controllability attributions) did not reach statistical validity according to cross validation. However, the correlation structure between items in this construct was intelligible and consistent with theory, and consistent between piloting rounds. Furthermore, a substantial proportion of the variation could be described by the components (71–74% in all subjects) and the eigenvalue for this construct was more than 1. Based on this information, the construct was considered as valid and included in the further analyses.
Results
To provide perspective on the importance of motivation for predicting student performance on the TIMSS achievement test (i.e., plausible values), OPLS models were computed for all subjects. The models utilized all available situational variables in the TIMSS School, Teacher and Student questionnaires, and the motivation constructs outlined in Table 1, to predict Students’ plausible values.
Results show that all variables included in the study jointly explained about 50% (and predict 40%) of the variation in Students’ plausible values (hereafter called PVs) in the different subjects (Table 2). The descriptive and predictive ability was similar for the science subjects while slightly higher for mathematics. Furthermore, when removing all variables with VIP < 1 in the prediction models, the predictive ability (Q2) was either almost fully retained or increased. For example, in biology, the 49 variables with VIP > 1 predicted as much as the model with all 308 variables. Performance measures for these reduced models are given within brackets in Table 2.
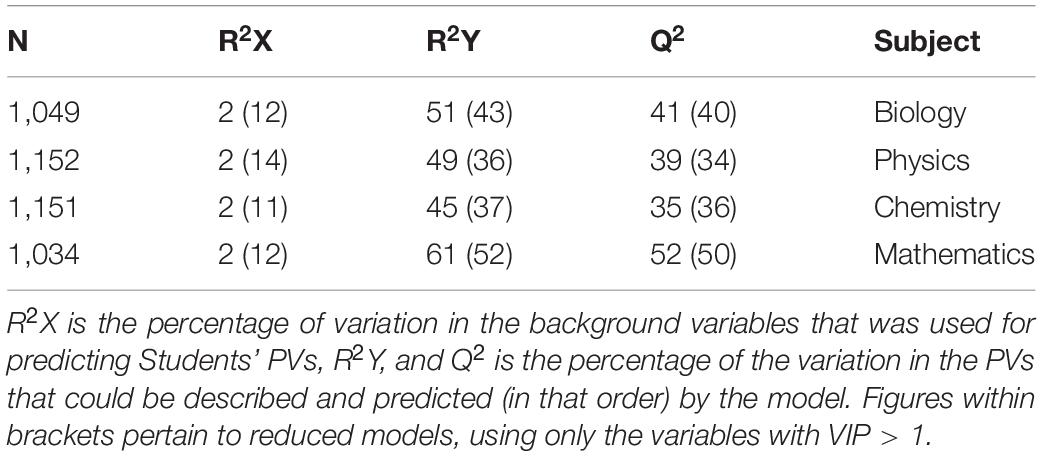
Table 2. Statistics of models for predicting PVs from background information, including student motivational characteristics, in the different subjects.
Cross-validation ANOVA strongly supported the validity of the models in Table 2 (p < 0.001 for all models). In most of the models, only 1–3 students showed a DModX exceeding critical levels. For chemistry, 10 students exceeded critical DModX levels in the model using only the predictive variables with VIP > 1. However, there were no effects on model performance or relationships between variables when deleting these students from the models. No students showed undue leverage in any of the models (Observation Risk values were under critical levels).
Research Question 1
How Important Are Motivational Characteristics, in Comparison to Other Variables in TIMSS 2011 Questionnaires, for Predicting Swedish Student Results on TIMSS Achievement Tests?
Table 3 shows that several motivational constructs are among the best predictors of student PVs, in relation to the other 290 variables included in the study (varying somewhat by subject). Perceived competence is one of the best predictors of student PVs, on level with or exceeding the importance of Students’ home educational resources for all subjects but biology. Furthermore, perceived competence is particularly important for predicting student PVs in mathematics, with the by far highest VIP of all variables. Other motivation-related variables that have considerable influence on the predictive ability of the models are: The subject makes the student confused and nervous, Teacher conveys trust in Student’s competence, and Student’s study expectations. The constructs of incentive value, epistemic beliefs, and mastery goals show high VIPs, at approximately the same level as parents’ educational level and the country of birth of the family members. Other motivation constructs that are more important than the average variables are: identified and intrinsic motivation, autonomy support and controllability attributions. The only motivation construct being less important than average is performance goals, which had an average VIP not significantly different from zero and therefore is not included in Table 3.
The motivation variables listed in Table 4 predict on average 27% of the variation in Students’ PVs when included in a common top model (Table 4). However, mathematics shows a substantial difference in comparison to the science subjects with a top model R2 of 41% and Q2 of 40%, which is almost twice as high as for any of the science subjects. The primary reason for this is the high predictive ability of perceived competence in mathematics, as also indicated in Table 3. When perceived competence is removed from the models, the differences in predictive ability between subjects are substantially reduced (last top model in Table 4).
The predictive ability is greater for models in which all the motivation constructs are used simultaneously to predict student PVs, compared to models based on individual constructs. As shown in Table 4, only a few motivation variables can individually predict a substantial proportion of the variation in student PVs. These are perceived competence (21%), epistemic beliefs (12%), controllability attributions (9%), and incentive value (9%). For three of these variables (perceived competence, controllability attributions, and incentive value) the predictive ability is more than twice as high in mathematics as in science. However, the motivation variables that are important for predicting student PVs in mathematics are also important for predicting their PVs in science.
For all models in Table 4, student DModX and Observation Risk values were below critical levels. Thus, no students exerted undue leverage on the models. Furthermore, Cross Validation ANOVA strongly supported the validity of the models (p < 0.001 for all models).
Research Question 2
Which of the Situational Variables Measured in This Study Are the Best Predictors of Student Motivational Characteristics?
For all the models in this section, Cross Validation ANOVA was < 0.005. No model had students with Observation Risk values exceeding limits. Most models had one, or no, students with DModX values exceeding critical levels. For these models no further measures were taken. One model had 6 students with observation risk exceeding limits. However, deletion of these students did not cause any changes in model performance or relationships between variables. Hence, all models were found valid and found to represent general patterns rather than a few extreme observations.
Table 5 shows that six student motivational characteristics can be predicted to 25–40% (on average across all subjects) from the situational variables, while performance goals, external/introjected motivation, controllability attributions and utility value are poorly predicted from the situational variables (Table 5).
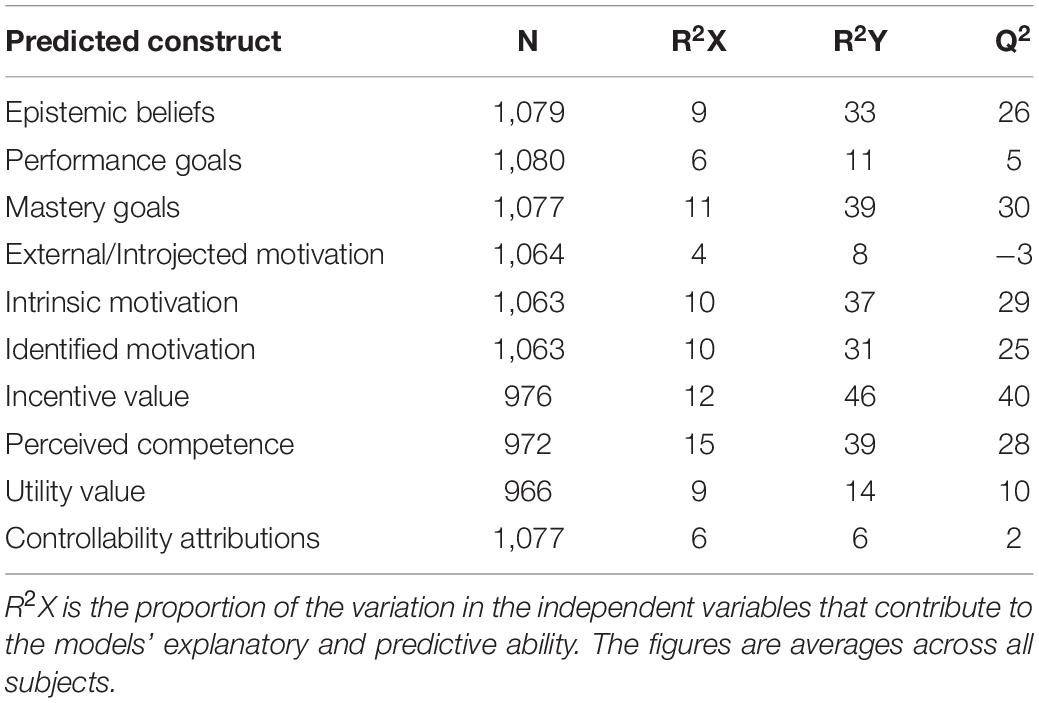
Table 5. Percentages of the variation in each motivation constructs that can be explained (R2Y) and predicted (Q2) by all situational variables in Table 6.
Most of the situational variables show very low importance for the prediction of student motivational characteristics. Less than 20 variables have substantially larger importance than the average variable for the predictive ability of the model. Furthermore, the confidence intervals for almost 75% of the situational variables’ VIPs were so large that their VIPs could be considered non-significant. Similar distributions were found for all constructs, and in all subjects. Table 6 shows the variables with higher-than-average ability (VIP > 1) to predict each motivational construct.
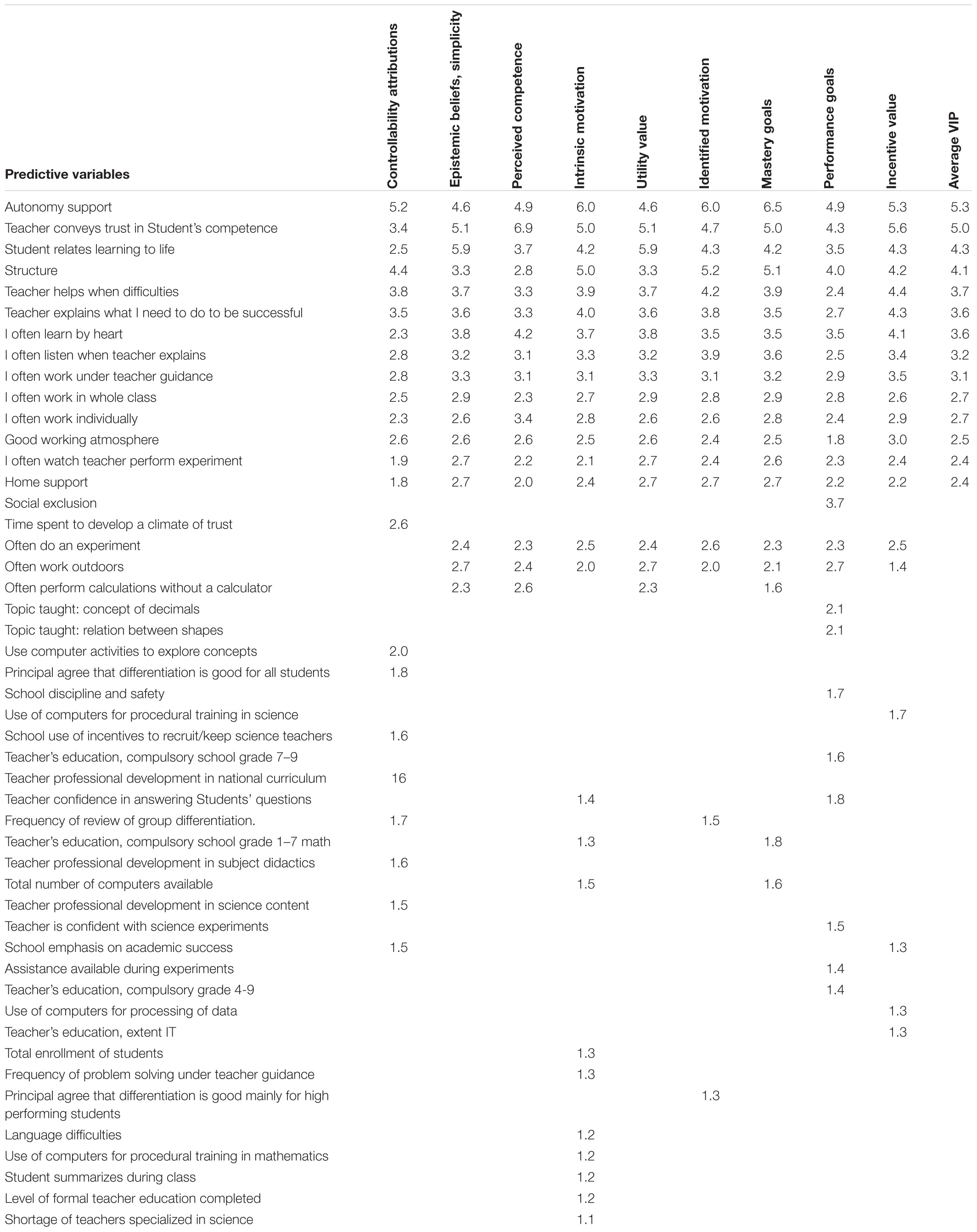
Table 6. Average VIPs (across all subjects) of situational variables in models predicting motivation constructs.
Five of the most important variables for predicting student motivational characteristics are teacher variables. These are: Autonomy support, Teacher conveys trust in Student’s competence, Structure, Teacher helps when difficulties and Teacher explains what I need to do to be successful. All these variables come from the student questionnaires and describe the Students’ views of their teachers. These situation variables describe teachers that convey their belief in Students’ capabilities to learn and provide help and explanations when needed. They provide support for Students’ autonomy and help them take responsibility for their learning, while at the same time providing supporting boundaries for classroom behavior. They take an interest in Students’ thoughts and make them feel understood. Autonomy support is, on average, the best predictor of motivational characteristics, followed by Teacher conveys trust in Students’ competence. Among the individual motivational characteristics, Autonomy support is the best predictor of Intrinsic and Identified motivation and for Mastery goals, while Teacher conveys trust in Students’ competence is the best predictor for Epistemic beliefs, Perceived competence, and Incentive value.
Interestingly, these five teacher variables together can predict even more of the student motivational characteristics (i.e., the variation in the Students’ responses related to the different motivation constructs) than all situational variables together. Six of the student motivational characteristics can be predicted to 30–42% (Table 7) using only the top five teacher variables, which can be compared to 25–40% for the models including all situational variables (Table 5). Furthermore, as shown in Table 6, these five teacher variables are consistently strong predictors for all motivational constructs, with only minute rank-order differences between subjects (not shown).
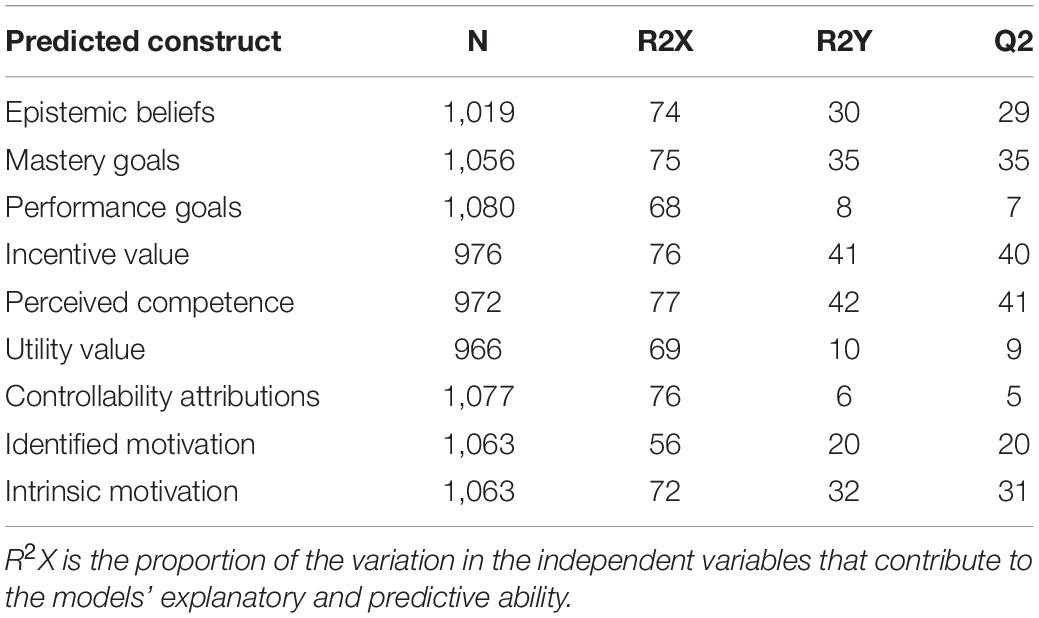
Table 7. Average proportions (across all subjects) of variation in each motivation construct that can be explained (R2Y) and predicted (Q2) by the top five VIP situational (teacher) variables in Table 6.
Discussion
Importance of Motivational Characteristics for Student Achievement
This study shows that several student motivational characteristics are important for student achievement. However, the main contribution of the study is the relative importance of motivational aspects, compared to other school and teaching related variables. The results show that student motivational characteristics play a more important role than most of the nearly 300 situational variables measured in TIMSS, and this holds for all of the four subjects.
Similar to other studies (e.g., Areepattamannil and Kaur, 2013), Students’ personal factors were abundant among the most important variables for predicting Students’ achievement. Many of the important variables (i.e., VIP > 1) for predicting student performance in the full models (Table 3) are related to motivation. Furthermore, even in relation to the full model including all variables, motivation top models using only motivational characteristics in a conjoint manner predicted a large proportion of the variation in student performance. For example, the model including all measured variables predicted 53% of the variation in student results in mathematics (Table 2), while the model including only motivational variables predicted 40% (Table 4). These top models, including several motivational characteristics, were also significantly better at predicting performance than models based on one motivational characteristic only. This is not surprising but emphasizes that Students’ behavior and performance in school is best understood and described by complex relationships between “clusters of related factors” (Covington, 2000, p. 187), and points to the potential value of studies that bring together several perspectives on motivation. For example, Hulleman et al. (2010) showed in a meta-analysis of 243 studies that correlations between four different types of achievement goals and performance ranged between −0.20 and 0.13, corresponding to R2s around 2–4%. This is on the same level as the R2s found for the models in our study, predicting performance from Students’ mastery and performance goals, respectively, and considerably lower than R2s for the two motivation top models using several motivation constructs simultaneously to predict performance (Table 4).
This study also describes the motivational profile of students that are successful on the TIMSS achievement test. They have confidence in their ability to perform and learn the subject, enjoy learning the subject, perceive the subject knowledge taught as coherent rather than consisting of unrelated pieces of information or areas and believe that understanding how things are related is a prerequisite for successful learning, and have a strong focus on knowledge development. Furthermore, the learning behavior of these successful students seems to be driven by the full integration of the instrumental value of learning the subject, as well as by the enjoyment of learning and the belief that the quality of the outcome of learning is under their control (e.g., by investing more effort). This pattern provides partial support for the SEVT (Eccles and Wigfield, 2020) notion that expectancy of success and value appraisals are important instigators of Students’ motivation to learn and, eventually, their performance on the TIMSS test. Expectancy of success (competence beliefs and study expectations), named perceived competence in our study, was the strongest motivation-related predictor of achievement, followed by incentive value of learning the subject (i.e., it is fun) and, somewhat less important, intrinsic motivation to learn (similar to incentive value) and identified motivation (cf. attainment value). However, the perceived utility of the knowledge does not seem to be as important for achievement as a strong focus on knowledge development (mastery goals) and a perception that knowledge should be viewed as a whole rather than a collection of isolated fragments (reverse of simplicity of knowledge). The simplicity of knowledge could be regarded not only as an epistemic belief (i.e., about what knowledge is, in terms of coherence of information), but also as an implicit goal regarding how knowledge should be constructed in the learning process. Although Students’ epistemic beliefs and achievement goals are not direct indicators of perceived value in themselves, the students can use them to evaluate to what extent the learning activities are conducive to goal fulfillment, and thus provide information of their value. It is clear from our data that (1) we need to include several independent value subcomponents to adequately portray the relation between Students’ motivation and their learning, as suggested by Trautwein et al. (2013) and, (2) as proposed by Eccles and Wigfield (2020), the meanings of those subconstructs need to be interpreted in the light of, for example, students beliefs and goals to understand how their relative weights differ between individuals and situations. In our study, the utility value items were predefined by the TIMSS organization and focused on the usefulness of the subject to learn other subjects or as a key to a desired study or work career (e.g., I need to learn XX to get into university; …to get the job I want). Hence, the utility value component mainly assessed an instrumental value. In contrast, important values and goals for Students’ mathematics and science achievement were related to Students’ enjoyment of learning and their own beliefs about knowledge and knowledge development. Moreover, the values and goals important for science and mathematics achievement in the present study are to a high extent concerned with the immediate learning, rather than future achievements or careers. Hence, although expectancies of success, a perceived importance of doing well in the subject (identified motivation, cf. attainment value), and enjoyment of learning (incentive value) were among the most important factors for predicting achievement, our results corroborate Eccles and Wigfield (2020) proposition that Students’ expectancies of success and value appraisals of the SEVT model need to be both complemented and differentiated to adequately predict Students’ achievement in mathematics and science in school. In this paper, we have shown that Students’ achievement goals, attributions, motivation type, and epistemic beliefs, in addition to contributing to a joint model in their own right, can be used to discern possible directions for the expansion of the SEVT.
Most results were similar across subjects. One exception was the relative importance of Students’ perceived competence. The strong position of perceived competence in relation to the other variables in this study is noteworthy and corroborates its central role in many motivation theories. However, although perceived competence was the best predictor of performance in both science and mathematics subjects, similar to Shen and Pedulla (2000) and Lee et al. (2014), its predictive ability was substantially higher in mathematics than in science subjects (Tables 3, 4). One possible explanation could be differences in the types of classroom activities and assessment that dominate in mathematics and the science subjects, respectively. In Sweden, working with tasks in textbooks is the dominant activity in mathematics classrooms and written tests, like the TIMSS achievement test, is the dominant method of testing Students’ competence. In the science subjects, on the other hand, several different types of activities are common both for learning and testing. These include not only written tests, but also (inter alia) laboratory exercises, lab reports and excursions/field work. Thus, while the activities available for students to judge their competence in mathematics are similar to the TIMSS achievement test, the Students’ perceived competence in science is partly based on appraisals of their performance on activities other than what is tested on the TIMSS achievement test. Since such activities may require other subject matter competencies than those required in written tests, evaluations of competence including performance on these activities may correlate less with TIMSS written test scores. Several studies have found correlations of Students’ test achievement with perceived competence (e.g., Shen and Pedulla, 2000) and with self-efficacy (Areepattamannil and Kaur, 2013; Lee et al., 2014; Burns et al., 2021). Common explanations for this are that perceived competence and self-efficacy leads to high engagement (Ryan and Deci, 2020) or self-regulation (Schunk and Pajares, 2005; Lee et al., 2014) which in turn lead to high achievement. However, by using a random intercept cross lagged panel analysis, Burns et al. (2020) recently found that students perceived competence was strongly influenced by prior achievement, while no evidence were found for the reverse relationship. According to self-determination theory, fulfillment of the need to feel competent promotes autonomous forms of motivation, which in turn leads to improved achievement and wellbeing. Indeed, several studies have corroborated this proposition by showing effects of fulfillment of the need for competence on autonomous motivation (Gnambs and Hanfstingl, 2016; Van den Broeck et al., 2016; Litalien et al., 2017) and engagement (Jang et al., 2016) and effects of autonomous motivation on achievement, mediated by engagement (Froiland and Worrell, 2016). Although the chicken-and-egg question of causality raised by, among others, Schunk and Pajares (2005) is still not solved, we argue that it is reasonable to expect a high perceived competence to lead to at least a temporary increase in autonomous forms of motivation and engagement.
Performance goals were unimportant in comparison to other variables for predicting Students’ achievement, with very low VIPs in all subjects and an average VIP not significantly above zero. This lack of association between performance goals and achievement is in line with the results of a recent study by Hofverberg and Winberg (2020a), based on another large sample and using a different measure of achievement. In the present study, the performance goal construct included items pertaining to both performance approach and avoidance goals. Hypothetically, this could have confused the results since performance approach and avoidance goals have been argued to have different implications for performance (Covington, 2000; Elliot and Murayama, 2008). However, this was not the case. The validation studies showed that performance approach goals could not be empirically separated from performance avoidance goals in this study, in terms of their predictive patterns. In addition, treating performance approach and performance avoidance goals as separate constructs did not improve their importance for prediction of student performance in any of the models (data not shown). The integrated nature of performance approach and avoidance goals in science and mathematics have been observed in several studies on different Swedish samples, using different scales and statistical methods for the analysis of the goal structures (Blomgren, 2016; Hofverberg and Winberg, 2020b). Furthermore, in an international cross-cultural comparative study, Hofverberg and Winberg (2020b) argued that this pattern may depend on to what extent social comparison occur and is accepted in the society. Swedish culture is considered as one of the least competitive in the world (Hofstede et al., 2010) and social comparisons in the classroom are often actively avoided by teachers. It might be tempting to speculate that the Swedish classroom goal structures (Ames, 1992) in general may have emphasized mastery goals before performance goals and, according to the interaction effect model by Murayama and Elliot (2009), thereby rendered performance goals less adaptive for learning. However, the study by Hofverberg and Winberg (2020a) found no support for such an interaction effect in a Swedish sample. Thus, although our study shows very weak influence of performance goals on Students’ achievement when other features of the school environment are also considered, the reasons for this require further attention.
To conclude, besides providing evidence that students motivation is essential for achievement, our results clearly point out the importance of an intrinsic or identified value of learning (i.e., autonomous motivation), mastery focus, perceptions of being in control of the learning, and a view that knowledge is contextual and coherent. In contrast, external motivation (i.e., being driven by rewards and threats), and introjected motivation (i.e., feeling forced to perform to preserve self-worth) were clearly negative for achievement. Performance goals, that is, focusing on own performance compared to peers, are at best non-significant for achievement. Hence, for teaching to be efficient, it is important to attend also to the “soft” aspects of learning, besides the subject content knowledge. That is, we need instructional practices that foster identified and intrinsic motivation, “sophisticated” epistemological beliefs, mastery goals and controllability attributions. Next section discusses what features of the instructional practices that may be most efficient for accomplishing this.
Importance of Situational Variables for Students’ Motivational Characteristics
Another main contribution of the study is the relative importance of situational variables for predicting student motivational characteristics. The study shows that teacher variables are among the most important situational variables and identifies five such variables that can predict as much of the student motivational characteristics as all situational variables together (Table 7, compared to Table 5). These five situational variables (autonomy support, teacher conveys trust in Student’s competence, structure, teacher helps when difficulties, and teacher explains what I need to do to be successful) can predict 20–41% of the variation in six motivational characteristics that are strongly associated with achievement (Table 7). This pattern was found in mathematics and in all four science subjects (Table 6). The significance of this result lies in the identification of the five teacher variables as important in relation to other variables, and the strength of their combined predictive power in relation to other variables. For example, earlier research has shown that high levels of autonomy support are associated with mastery goals (Shih, 2008; Ciani et al., 2010) and this study shows that the association is similar for all four subjects and that autonomy support not only is a good predictor of mastery goals, compared to all situational variables available in the TIMSS 2011 questionnaires and the supplementary questionnaire, it is on average the best variable to predict all nine student motivational characteristics. This is important since teachers can learn how to engage in more autonomy-supportive behavior (Reeve et al., 2004). In addition, the identification of the predictive power of these five teacher variables emphasizes teachers’ importance for student motivation, compared to other school and home variables. Moreover, the results suggest that these few variables should be considered in both mathematics and science classrooms when designing instructional practices to promote student motivation.
While autonomy support seems universally important, there are some differences in the prediction patterns for the other “top five” predictors. For example, to promote a view that the subject content is coherent and that successful learning requires the learner to see how the different parts relate to each other (epistemic beliefs, simplicity), it is important to provide choice in learning and show interest in Students’ way of thinking (autonomy), but even more important to give them opportunities to reflect on how the subject content pertains to life outside school and to show confidence in their ability to do so (e.g., by communicating high expectations). Hence, it seems that to be understandable, the real-life context requires different aspects of Students’ school subject knowledge be brought together. The beneficial effects of handling complex, and sometimes ambiguous, scientific information in real-life context have been highlighted in research on, for example, situated learning (Anderson et al., 1996), socio-scientific issues (Sadler, 2009), and context-based learning (Gilbert et al., 2011). However, for integration of concepts to happen, our data suggest that it is not enough to provide a complex context for the treatment of the information, but the teacher must also express in what way they expect students to treat the information, and support Students’ confidence in doing so. In contrast, to predict identified and intrinsic motivation among students, autonomy is the most important variable (among those measured). Within self-determination theory, the fulfillment of the basic psychological need of autonomy is considered essential for the promotion of autonomous forms of regulation, such as identified, integrated, and intrinsic motivation (Ryan and Connell, 1989; Ryan and Deci, 2020), a proposition that has been corroborated in many studies (Van den Broeck et al., 2016). Hence, the association between autonomy support and identified motivation found in our study was expected. Moreover, the fact that teacher’s confidence in Students’ competence is important for students identified motivation is not surprising since it is reasonable to assume that such explicitly shown confidence can influence students own sense of competence, which has been shown to be associated with autonomous motivation (Van den Broeck et al., 2016). The second strongest predictor of identified motivation in our study was structure, that is; the teacher keeps track of students learning, make sure that they know what they are supposed to do, and helps them stay focused during the lesson. Although it might seem counterintuitive that a high degree of structure promotes identified and intrinsic motivation, the level of self-determination is not primarily linked to the freedom to choose what to do, but to the degree to which the choice to act (e.g., to comply with the teacher’s instructions) emanates from within, rather from external pressure. In fact, autonomy support in combination with clarity of goals, high expectations, and rich efficacy feedback conveyed in a positive manner is viewed as “the most positive teaching and parenting style” (Ryan and Deci, 2020, p. 4). The combined positive effect of autonomy support, teachers trust in Students’ competence and structure on identified and intrinsic motivation found in our study corroborates this.
Limitations
The results in this study are correlational. The term “prediction” used in the paper refers to the cross-validation procedure, where parts of the data set were deleted and predicted by the remaining data. This should not be confused with predictions in a temporal sense. Hence, conclusions about causality based on these results should be made with caution. In addition, the results do not specify, for example, teachers’ actions that will always facilitate the development of Students’ motivational characteristics beneficial for their achievement. One reason for this is that the situation variables refer to how situations are perceived by the students, and different students may perceive the same situation differently. But Students’ perceptions constitute their reality, and this information is what they act upon and need to be considered when orchestrating learning situations. Nevertheless, the set of situational variables identified as particularly important in this study may still be useful in development of principles for motivation-promoting instructional designs in authentic teaching as well as in research studies on the effectiveness of motivation-promoting teaching. It would be valuable to extend such studies to include measures of Students’ motivation manifested as observable actions in the classroom learning situations.
For the sake of ecological validity, the study included a large number of variables to describe Students’ motivation as completely as possible, and the context in which it operates. Hence, high levels of multicollinearity were expected, which precluded the use of ordinary least squares based multiple regression methods and multilevel modeling, which may produce inflated standard errors for coefficient estimates (Shieh and Fouladi, 2003). Huang (2014, 2016) showed that ordinary least squares regression (OLS), fixed effects models (FE), Taylor series linearization (TSL), and multilevel modeling (MLM) produce similar coefficient estimates for all levels of nested data. However, while providing unbiased estimates of variables on all levels, OLS generally underestimate the standard error of higher level variables. As the nested nature of the data may have resulted in underestimation of standard errors for the class- and school-level variables, their significance for the prediction of Students’ achievement on the TIMSS test may have been overestimated. If this is the case, the relative importance of Students’ motivation, compared to class- and school-related variables, may in reality be even more pronounced than shown in our study.
Data Availability Statement
The datasets presented in this article are not readily available because, data were collected by the Swedish National Agency of Education, who claim ownership of the data. Requests to access the datasets should be directed to cmVnaXN0cmF0b3JAc2tvbHZlcmtldC5zZQ==.
Ethics Statement
Ethical review and approval was not required for the study on human participants in accordance with the local legislation and institutional requirements. Written informed consent from the participants’ legal guardian/next of kin was not required to participate in this study in accordance with the national legislation and the institutional requirements.
Author Contributions
Both authors listed have made a substantial, direct and intellectual contribution to the work, and approved it for publication.
Funding
This study was funded by the Swedish Research Council (Grant No. 2007-3216).
Conflict of Interest
The authors declare that the research was conducted in the absence of any commercial or financial relationships that could be construed as a potential conflict of interest.
References
Abdi, H. (2010). Partial least squares regression and projection on latent structure regression (pls regression). Wiley Interdisci. Rev. Comput. Stat. 2, 97–106. doi: 10.1002/wics.51
Abdi, H., and Williams, L. J. (2010). Principal component analysis. Wiley Interdisci. Rev. Comput. Stat. 2, 433–459. doi: 10.1002/wics.101
Anderson, J. R., Reder, L. M., and Simon, H. A. (1996). Situated learning and education. Educ. Res. 25, 5–11. doi: 10.3102/0013189X025004005
Ames, C. (1992). Classrooms: Goals, structures, and student motivation. J. Educ. Psychol. y 84, 261–271. doi: 10.1037/0022-0663.84.3.261
Areepattamannil, S., and Kaur, B. (2013). Factors predicting science achievement of immigrant and non-immigrant students: a multilevel analysis. Int. J. Sci. Math. Educ. 11, 1183–1207. doi: 10.1007/s10763-012-9369-5
Bandura, A. (2010). “Self-Efficacy,” in The Corsini Encyclopedia of Psychology, Vol. 4, eds C. B. Nemeroff and W. E. Craighead (Hoboken, NJ: John Wiley and Sons Inc), 1–3. doi: 10.1002/9780470479216.corpsy0836
Black, A. E., and Deci, E. L. (2000). The effects of instructor’ autonomy support and students’ autonomous motivation on learning organic chemistry: a self-determination theory perspective. Sci. Educ. 84, 740–756. doi: 10.1002/1098-237X(200011)84:6<740::AID-SCE4<3.0.CO;2-3
Blomgren, J. (2016). Den Svårfångade Motivationen: Elever i en Digitaliserad Lärmiljö [The elusive motivation: Students in a Digitalized Learning Environment]. (Doctoral thesis), University of Gothenburg, Gothenburg.
Bråten, I., and Strømsø, H. I. (2010). When law students read multiple documents about global warming: Examining the role of topic-specific beliefs about the nature of knowledge and knowing. Instr. Sci. 38, 635–657. doi: 10.1007/s11251-008-9091-4
Burns, E. C., Martin, A. J., Kennett, R. K., Pearson, J., and Munro-Smith, V. (2021). Optimizing science self-efficacy: a multilevel examination of the moderating effects of anxiety on the relationship between self-efficacy and achievement in science. Contemp. Educ. Psychol. 64, 101937. doi: 10.1016/j.cedpsych.2020.101937
Burns, R. A., Crisp, D. A., and Burns, R. B. (2020). Re-examining the reciprocal effects model of self-concept, self-efficacy, and academic achievement in a comparison of the cross-lagged panel and random-intercept cross-lagged Panel frameworks. Br. J. Educ. Psychol. 90, 77–91. doi: 10.1111/bjep.12265
Carver, C. S., and Scheier, M. F. (1990). Origins and functions of positive and negative affect: A control-process view. Psychol. Rev. 97, 19–35. doi: 10.1037/0033-295X.97.1.19
Carver, C. S., and Scheier, M. F. (2002). Control processes and self-organization as complementary principles underlying behavior. Personal. Soc. Psychol. Rev. 6, 304–315. doi: 10.1207/S15327957PSPR0604_05
Chatzisarantis, N. L. D., Bing, Q., Xin, C., Kawabata, M., Koch, S., Rooney, R., et al. (2016). Comparing effectiveness of additive, interactive and quadratic models in detecting combined effects of achievement goals on academic attainment. Learn. Individ. Differ. 50, 203–209. doi: 10.1016/j.lindif.2016.08.015
Chong, I.-G., and Jun, C.-H. (2005). Performance of some variable selection methods when multicollinearity is present. Chemom. Intell. Lab. Syst. 78, 103–112. doi: 10.1016/j.chemolab.2004.12.011
Ciani, K. D., Middleton, M. J., Summers, J. J., and Sheldon, K. M. (2010). Buffering against performance classroom goal structures: the importance of autonomy support and classroom community. Contemp. Educ. Psychol. 35, 88–99. doi: 10.1016/j.cedpsych.2009.11.001
Covington, M. V. (2000). Goal theory, motivation, and school achievement: an integrative review. Annu. Rev. Psychol. 51, 171–200.
Deci, E. L., and Ryan, R. M. (1985). Intrinsic Motivation and Self-Determination in Human Behavior. New York, NY: Plenum Press.
Eastment, H., and Krzanowski, W. (1982). Crossvalidatory choice of the number of components from a principal component analysis. Technometrics 24, 73–77. doi: 10.1080/00401706.1982.10487712
Eccles, J. S., Adler, T. F., Futterman, R., Goff, S. B., Kaczala, C. M., Meece, J. L., et al. (1983). “Expectancies, values, and academic behaviors,” in Achievement and Achievement Motivation, ed. J. T. Spence (San Francisco, CA: W.H. Freeman).
Eccles, J. S., and Wigfield, A. (2020). From expectancy-value theory to situated expectancy-value theory: a developmental, social cognitive, and sociocultural perspective on motivation. Contemp. Educ. Psychol. 61:101859. doi: 10.1016/j.cedpsych.2020.101859
Efron, B., and Gong, G. (1983). A leisurely look at the bootstrap, the jack-knife, and cross-validation. Am. Stat. 37, 36–48. doi: 10.1080/00031305.1983.10483087
Elliot, A. J., and Hulleman, C. S. (2017). “Achievement goals,” in Handbook of Competence and Motivation: Theory and Application, 2 Edn, eds A. J. Elliot, C. S. Dweck, and D. S. Yeager (New York, NY: Guilford Publications), 43–60.
Elliot, A. J., and Murayama, K. (2008). On the measurement of achievement goals: Critique, illustration, and application. J. Educ. Psychol. 100, 613–628. doi: 10.1037/0022-0663.100.3.613
Elliot, A. J., and Thrash, T. M. (2001). Achievement goals and the hierarchical model of achievement motivation. Educ. Psychol. Rev. 13, 139–156. doi: 10.1023/A:1009057102306
Eriksson, L., Johansson, E., Kettaneh-Wold, N., Trygg, J., Wikström, C., and Wold, S. (2006). Multi and Megavariate Data Analysis. Part i: Basic Principles and Applications, Second Edn. Umeå: Umetrics Inc.
Eriksson, L., Trygg, J., and Wold, S. (2008). CV-ANOVA for significance testing of PLS and OPLS§models. J. Chemom. 22, 594–600. doi: 10.1002/cem.1187
Fredrickson, B. L., and Branigan, C. (2005). Positive emotions broaden the scope of attention and thought - action repertoires. Cogn. Emot. 19, 313–332. doi: 10.1080/02699930441000238
Froiland, J. M., and Worrell, F. C. (2016). Intrinsic motivation, learning goals, engagement, and achievement in a diverse high school. Psychol. Sch. 53, 321–336. doi: 10.1002/pits.21901
Galindo-Prieto, B., Eriksson, L., and Trygg, J. (2015). Variable influence on projection (VIP) for OPLS models and its applicability in multivariate time series analysis. Chemom. Intell. Lab. Syst. 146, 297–304. doi: 10.1016/j.chemolab.2015.05.001
Gilbert, J. K., Bulte, A. M. W., and Pilot, A. (2011). Concept development and transfer in context-based science education. Int. J. Sci. Educ. 33, 817–837. doi: 10.1080/09500693.2010.493185
Gnambs, T., and Hanfstingl, B. (2016). The decline of academic motivation during adolescence: an accelerated longitudinal cohort analysis on the effect of psychological need satisfaction. Educ. Psychol. 36, 1691–1705. doi: 10.1080/01443410.2015.1113236
Goetz, T., Sticca, F., Pekrun, R., Murayama, K., and Elliot, A. J. (2016). Intraindividual relations between achievement goals and discrete achievement emotions: an experience sampling approach. Learn. Instr. 41, 115–125. doi: 10.1016/j.learninstruc.2015.10.007
Gosselin, R., Rodrigue, D., and Duchesne, C. (2010). A bootstrap-vip approach for selecting wavelength intervals in spectral imaging applications. Chemom. Intell. Lab. Syst. 100, 12–21. doi: 10.1016/j.chemolab.2009.09.005
Hofer, B. K. (2004). Exploring the dimensions of personal epistemology in differing classroom contexts: Student interpretations during the first year of college. Contemp. Educ. Psychol. 29, 129–163. doi: 10.1016/j.cedpsych.2004.01.002
Hofstede, G., Hofstede, G. J., and Minkov, M. (2010). Cultures and Organizations: Software of the Mind, 3 Edn. New York, NY: McGraw-Hill.
Hofverberg, A., and Winberg, M. (2020a). Achievement goals and classroom goal structures: do they need to match? J. Educ. Res. 113, 145–162. doi: 10.1080/00220671.2020.1759495
Hofverberg, A., and Winberg, M. (2020b). Challenging the universality of achievement goal models: a comparison of two culturally distinct countries. Scand. J. Educ. Res. 64, 333–354. doi: 10.1080/00313831.2018.1544170
Huang, F. L. (2014). Analyzing group level effects with clustered data using taylor series linearization. Pract. Assess. Res. Eval. 19:13. doi: 10.7275/ntnk-d929
Huang, F. L. (2016). Alternatives to multilevel modeling for the analysis of clustered data. J. Exp. Educ. 84, 175–196. doi: 10.1080/00220973.2014.952397
Hulleman, C. S., Schrager, S. M., Bodmann, S. M., and Harackiewicz, J. M. (2010). A meta-analytic review of achievement goal measures: different labels for the same constructs or different constructs with similar labels? Psychol. Bull. 136, 422–449. doi: 10.1037/a0018947
IEA (2011a). Creating and Interpreting the Timss and Pirls Context Questionnaire Scales: Context Questionnaire Scales Details. Available online at: http://timss.bc.edu/methods/t-context-q-scales.html (accessed March 17, 2021).
IEA (2011b). Timss 2011 Contextual Questionnaires. Available online at: http://timss.bc.edu/timss2011/international-contextual-q.html (accessed March 17, 2021).
IEA (2011c). Timss and Pirls Achievement Scaling Methodology. Available online at: http://timss.bc.edu/methods/pdf/TP11_Scaling_Methodology.pdf (accessed March 17, 2021).
Izard, C. E. (2007). Basic emotions, natural kinds, emotion schemas, and a new paradigm. Perspect. Psychol. Sci. 2, 260–280. doi: 10.1111/j.1745-6916.2007.00044.x
Jang, H., Kim, E. J., and Reeve, J. (2016). Why students become more engaged or more disengaged during the semester: a self-determination theory dual-process model. Learn. Instr. 43, 27–38. doi: 10.1016/j.learninstruc.2016.01.002
Joncas, M., and Foy, P. (2011). Sample Design in Timss and Pirls. Available online at: http://timss.bc.edu/methods/pdf/TP_Sampling_Design.pdf (accessed March 17, 2021).
Korn, R. M., Elliot, A. J., and Daumiller, M. (2019). Back to the roots: The 2× 2 standpoints and standards achievement goal model. Learn. Individ. Differ. 72, 92–102. doi: 10.1016/j.lindif.2019.04.009
Lau, S., and Nie, Y. (2008). Interplay between personal goals and classroom goal structures in predicting student outcomes: a multilevel analysis of person-context interactions. J. Educ. Psychol. 100, 15–29. doi: 10.1037/0022-0663.100.1.15
Lee, W., Lee, M.-J., and Bong, M. (2014). Testing interest and self-efficacy as predictors of academic self-regulation and achievement. Contemp. Educ. Psychol. 39, 86–99. doi: 10.1016/j.cedpsych.2014.02.002
Lindfors, M., Winberg, T. M., and Bodin, M. (2017). The role of students’ scientific epistemic beliefs in computer-simulated problem solving. Scand. J. Educ. Res. 63, 124–144. doi: 10.1080/00313831.2017.1324907
Linnenbrink-Garcia, L., Patall, E. A., and Pekrun, R. (2016). Adaptive motivation and emotion in education:research and principles for instructional design. Policy Insights Behav. Brain Sci. 3, 228–236. doi: 10.1177/2372732216644450
Linnenbrink-Garcia, L., Tyson, D. F., and Patall, E. A. (2008). When are achievement goal orientations beneficial for academic achievement? A closer look at main effects and moderating factors. Rev. Int. De Psychol. Soc. 21, 19–70.
Litalien, D., Morin, A. J., Gagné, M., Vallerand, R. J., Losier, G. F., and Ryan, R. M. (2017). Evidence of a continuum structure of academic self-determination: a two-study test using a bifactor-ESEM representation of academic motivation. Contemp. Educ. Psychol. 51, 67–82. doi: 10.1016/j.cedpsych.2017.06.010
Lüftenegger, M., Klug, J., Harrer, K., Langer, M., Spiel, C., and Schober, B. (2016). Students’ achievement goals, learning-related emotions and academic achievement. Front. Psychol. 7:603. doi: 10.3389/fpsyg.2016.00603
Marshik, T., Ashton, P. T., and Algina, J. (2017). Teachers’ and students’ needs for autonomy, competence, and relatedness as predictors of students’ achievement. Soc. Psychol. Educ. 20, 39–67. doi: 10.1007/s11218-016-9360-z
Martin, M. O., Mullis, I. V. S., Foy, P., and Arora, A. (2011). Creating and Interpreting the Timss and Pirls 2011 Context Questionnaire Scales. Available online at: http://timss.bc.edu/methods/pdf/TP11_Context_Q_Scales.pdf (accessed March 17, 2021).
Martin, M. O., Mullis, I. V. S., Foy, P., and Stanco, G. M. (2012). Timss 2011 International Results in science (ED544560). Available online at: https://eric.ed.gov/?id=ED544560 (accessed March 17, 2021).
Mateos-Aparicio, G. (2011). Partial least squares (PLS) methods: origins, evolution, and application to social sciences. Commun. Stat. Theory Methods 40, 2305–2317. doi: 10.1080/03610921003778225
Mehmood, T., Liland, K. H., Snipen, L., and Sæbø, S. (2012). A review of variable selection methods in partial least squares regression. Chemom. Intell. Lab. Syst. 118, 62–69. doi: 10.1016/j.chemolab.2012.07.010
Michou, A., Vansteenkiste, M., Mouratidis, A., and Lens, W. (2014). Enriching the hierarchical model of achievement motivation: autonomous and controlling reasons underlying achievement goals. Br. J. Educ. Psychol. 84, 650–666. doi: 10.1111/bjep.12055
Midgley, C., Maehr, M. L., Hruda, L. Z., Anderman, E., Anderman, L., Freeman, K. E., et al. (2000). Manual for the Patterns of Adaptive Learning Scales. Ann Arbor, MI: University of Michigan.
Mikels, J. A., Reuter-Lorenz, P. A., Beyer, J. A., and Fredrickson, B. L. (2008). Emotion and working memory: evidence for domain-specific processes for affective maintenance. Emotion 8, 256–266. doi: 10.1037/1528-3542.8.2.256
Mouratidis, A., Michou, A., Demircioðlu, A. N., and Sayil, M. (2018). Different goals, different pathways to success: performance-approach goals as direct and mastery-approach goals as indirect predictors of grades in mathematics. Learn. Individ. Differ. 61, 127–135.
Muis, K. R. (2007). The role of epistemic beliefs in self-regulated learning. Educ. Psychol. 42, 173–190. doi: 10.1080/00461520701416306
Mullis, I. V. S., Martin, M. O., Ruddock, G. J., O’Sullivan, C. Y., and Preuschoff, C. (2011). Timss 2011 Contextual Frameworks TIMSS 2011 Assessment Frameworks. Chestnut Hill, MA: TIMSS and PIRLS International Study Center, 91–116.
Murayama, K., and Elliot, A. J. (2009). The joint influence of personal achievement goals and classroom goal structures on achievement-relevant outcomes. J. Educ. Psychol. 101, 432–447. doi: 10.1037/a0014221
Nengsih, T. A., Bertrand, F., Maumy-Bertrand, M., and Meyer, N. (2019). Determining the number of components in PLS regression on incomplete data set. Stat. Appl. Genet. Mol. Biol. 18, 1–16. doi: 10.1515/sagmb-2018-0059
Podsakoff, P. M., MacKenzie, S. B., Lee, J.-Y., and Podsakoff, N. P. (2003). Common method biases in behavioral research: a critical review of the literature and recommended remedies. J. Appl. Psychol. 88, 879–903. doi: 10.1037/0021-9010.88.5.879
Reeve, J., Jang, H., Carrell, D., Barch, J., and Jeon, S. (2004). Enhancing students’ engagement by increasing teachers’ autonomy support. Motiv. Emot. 28, 147–169. doi: 10.1023/B:MOEM.0000032312.95499.6f
Ricco, R., Schuyten Pierce, S., and Medinilla, C. (2010). Epistemic beliefs and achievement motivation in early adolescence. J. Early Adolesc. 30, 305–340. doi: 10.1177/0272431609333299
Ryan, R. M. (1982). Control and information in the intrapersonal sphere: an extension of cognitive evaluation theory. J. Pers. Soc. Psychol. 43, 450–461. doi: 10.1037/0022-3514.43.3.450
Ryan, R. M., and Connell, J. P. (1989). Perceived locus of causality and internalization: examining reasons for acting in two domains. J. Pers. Soc. Psychol. 57, 749–761. doi: 10.1037/0022-3514.57.5.749
Ryan, R. M., and Deci, E. L. (2000). Intrinsic and extrinsic motivations: classic definitions and new directions. Contemp. Educ. Psychol. 25, 54–67. doi: 10.1006/ceps.1999.1020
Ryan, R. M., and Deci, E. L. (2020). Intrinsic and extrinsic motivation from a self-determination theory perspective: definitions, theory, practices, and future directions. Contemp. Educ. Psychol. 61:101860. doi: 10.1016/j.cedpsych.2020.101860
Sadler, T. D. (2009). Situated learning in science education: socio-scientific issues as contexts for practice. Stud. Sci. Educ. 45, 1–42. doi: 10.1080/03057260802681839
Scherer, K. R. (2005). What are emotions? And how can they be measured? Soc. Sci. Inform. 44, 695–729. doi: 10.1177/0539018405058216
Schunk, D. M., and Pajares, F. (2005). “Self-efficacy and self-concept beliefs: jointly contributing to the quality of human life,” in New Frontiers for Self Research, eds H. W. Marsh, R. G. Craven, and D. M. McInerney (Charlotte, NC: Information Age Publishing, Inc).
Senko, C. (2019). When do mastery and performance goals facilitate academic achievement? Contemp. Educ. Psychol. 59:101795. doi: 10.1016/j.cedpsych.2019.101795
Senko, C., and Tropiano, K. L. (2016). Comparing three models of achievement goals: goal orientations, goal standards, and goal complexes. J. Educ. Psychol. 108, 1178–1192. doi: 10.1037/edu0000114
Shen, C., and Pedulla, J. J. (2000). The relationship between students’ achievement and their self-perception of competence and rigour of mathematics and science: a cross-national analysis. Assess. Educ. Princ. Policy Pract. 7, 237–253. doi: 10.1080/713613335
Shih, S.-S. (2008). The relation of self-determination and achievement goals to taiwanese eighth graders’ behavioral and emotional engagement in schoolwork. Elem. Sch. J. 108, 313–334. doi: 10.1086/528974
Shieh, Y.-Y., and Fouladi, R. T. (2003). The effect of multicollinearity on multilevel modeling parameter estimates and standard errors. Educ. Psychol. Meas. 63, 951–985. doi: 10.1177/0013164403258402
Song, J., Kim, S. I., and Bong, M. (2020). Controllability attribution as a mediator in the effect of mindset on achievement goal adoption following failure [Article]. Front. Psychol. 10:2943. doi: 10.3389/fpsyg.2019.02943
Ståhle, L., and Wold, S. (1990). Multivariate analysis of variance (manova). Chemometr. Intell. Lab. Syst. 6, 259–272. doi: 10.1016/0169-7439(90)80094-M
Trautwein, U., Nagengast, B., Marsh, H. W., Gaspard, H., Dicke, A.-L., Lüdtke, O., et al. (2013). “Expectancy-value theory revisited: from expectancy-value theory to expectancy-ValueS theory?,” in Theory Driving Research: New Wave Perspectives on Self-Processes and Human Development, eds D. M. McInerney, H. W. Marsh, and R. G. Craven (Charlotte, NC: IAP Information Age Publishing), 233–249.
Troyer, M., Kim, J. S., Hale, E., Wantchekon, K. A., and Armstrong, C. (2019). Relations among intrinsic and extrinsic reading motivation, reading amount, and comprehension: a conceptual replication. Read. Writ. 32, 1197–1218. doi: 10.1007/s11145-018-9907-9
Trygg, J., and Wold, S. (2002). Orthogonal projections to latent structures (o-pls). J. Chemom. 16, 119–128. doi: 10.1002/cem.695
Urdan, T., and Kaplan, A. (2020). The origins, evolution, and future directions of achievement goal theory. Contemp. Educ. Psychol. 61:101862. doi: 10.1016/j.cedpsych.2020.101862
Van den Broeck, A., Ferris, D. L., Chang, C.-H., and Rosen, C. C. (2016). A review of self-determination theory’s basic psychological needs at work. J. Manag. 42, 1195–1229. doi: 10.1177/0149206316632058
Van der Voet, H. (1994). Comparing the predictive accuracy of models using a simple randomization test. Chemometr. Intell. Lab. Syst. 25, 313–323. doi: 10.1016/0169-7439(94)85050-X
Vedder-Weiss, D., and Fortus, D. (2012). Adolescents’ declining motivation to learn science: a follow-up study. J. Res. Sci. Teach. 49, 1057–1095. doi: 10.1002/tea.21049
Weiner, B. (1985). An attributional theory of achievement motivation and emotion. Psychol. Rev. 92, 548–573. doi: 10.1037/0033-295X.92.4.548
Weiner, B. (2000). Intrapersonal and interpersonal theories of motivation from an attributional perspective. Educ. Psychol. Rev. 92, 548–573. doi: 10.1023/A:1009017532121
Wigfield, A., and Cambria, J. (2010). Students’ achievement values, goal orientations, and interest: definitions, development, and relations to achievement outcomes. Dev. Rev. 30, 1–35. doi: 10.1016/j.dr.2009.12.001
Wigfield, A., and Eccles, J. S. (1992). The development of achievement task values: a theoretical analysis. Dev. Rev. 12, 265–310. doi: 10.1016/0273-2297(92)90011-P
Wigfield, A., and Eccles, J. S. (2000). Expectancy-value theory of achievement motivation. Contemp. Educ. Psychol. 25, 68–81. doi: 10.1006/ceps.1999.1015
Winberg, M., Hofverberg, A., and Lindfors, M. (2018). Relationships between epistemic beliefs and achievement goals: developmental trends over grades 5-11. Eur. J. Psychol. Educ. 34, 295–315. doi: 10.1007/s10212-018-0391-z
Winberg, T. M., Hellgren, J. M., and Palm, T. (2014). Stimulating positive emotional experiences in mathematics learning: influence of situational and personal factors. Eur. J. Psychol. Educ. 29, 673–691. doi: 10.1007/s10212-014-0220-y
Wold, H. (1975). “11 – Path models with latent variables: the NIPALS approach,” in Quantitative Sociology eds H. M. Blalock, A. Aganbegian, F. M. Borodkin, R. Boudon, and V. Capecchi (Cambridge, MA: Academic Press), 307–357. doi: 10.1016/B978-0-12-103950-9.50017-4
Keywords: achievement, mathematics, science, achievement goals, epistemic beliefs, self-determination theory, TIMSS, orthogonal projection to least squares analysis (OPLS)
Citation: Winberg M and Palm T (2021) Antecedents and Relative Importance of Student Motivation for Science and Mathematics Achievement in TIMSS. Front. Educ. 6:575926. doi: 10.3389/feduc.2021.575926
Received: 24 June 2020; Accepted: 10 March 2021;
Published: 29 March 2021.
Edited by:
Emilie Prast, Leiden University, NetherlandsReviewed by:
Marian Hickendorff, Leiden University, NetherlandsMeryem Yilmaz Soylu, University of Nebraska-Lincoln, United States
Copyright © 2021 Winberg and Palm. This is an open-access article distributed under the terms of the Creative Commons Attribution License (CC BY). The use, distribution or reproduction in other forums is permitted, provided the original author(s) and the copyright owner(s) are credited and that the original publication in this journal is cited, in accordance with accepted academic practice. No use, distribution or reproduction is permitted which does not comply with these terms.
*Correspondence: Mikael Winberg, bWlrYWVsLndpbmJlcmdAdW11LnNl