- 1Friedl-Schöller Endowed Chair for Educational Psychology, TUM School of Education, Technical University of Munich (TUM), Munich, Germany
- 2Center for International Student Assessment Zentrum für Internationale Bildungsvergleichsstudien (ZIB), TUM School of Education, Technical University of Munich (TUM), Munich, Germany
The paper addresses cognitive processes during a teacher's professional task of assessing learning-relevant student characteristics. We explore how eye-movement patterns (scanpaths) differ across expert and novice teachers during an assessment situation. In an eye-tracking experiment, participants watched an authentic video of a classroom lesson and were subsequently asked to assess five different students. Instead of using typically reported averaged gaze data (e.g., number of fixations), we used gaze patterns as an indicator for visual behavior. We extracted scanpath patterns, compared them qualitatively (common sub-pattern) and quantitatively (scanpath entropy) between experts and novices, and related teachers' visual behavior to their assessment competence. Results show that teachers' scanpaths were idiosyncratic and more similar to teachers of the same expertise group. Moreover, experts monitored all target students more regularly and made recurring scans to re-adjust their assessment. Lastly, this behavior was quantified using Shannon's entropy score. Results indicate that experts' scanpaths were more complex, involved more frequent revisits of all students, and that experts transferred their attention between all students with equal probability. Experts' visual behavior was also statistically related to higher judgment accuracy.
Introduction
In day-to-day teaching, teachers have to continuously monitor a classroom full of students, respond to questions, observe students' learning progress, and assess how students react to their instructions—briefly, teaching is characterized by multi-dimensionality, immediacy, and simultaneity (Doyle, 2006). The success of a teacher to fulfill these various tasks depends heavily on their skill to visually perceive and process all of the information extracted from the classroom (Wolff et al., 2016). Eye-tracking provides an appropriate instrument for understanding the nature of teachers' visual perception processes and for uncovering differences therein that result from varying levels of expertise. Over the last decade, there has been a growing interest in teachers' eye-tracking data, particularly in terms of the number or duration of fixations (van den Bogert et al., 2014; Wolff et al., 2016; McIntyre et al., 2017; Stürmer et al., 2017; Seidel et al., 2020; Wyss et al., 2020). Such metrics were used in the above studies, for example, to demonstrate that expert teachers were able to process visual information more quickly than others, or that novice teachers focused more often on non-relevant classroom events. However, our knowledge of the underlying structure of this visual behavior, the so-called scanpath, is limited. Scanpaths represent the pattern of fixations and saccades constructed from the path of eye movements over a specific period (Holmqvist et al., 2015). Scanpath analyses have already been carried out in diverse domains (e.g., medicine; Kelly et al., 2016) to analyze and compare the visual behavior of experts and novices. In the teaching profession, such analyses may also be valuable to the identification of levels of expertise, since they would allow for the consideration of the sequential and dynamic nature of teaching (McIntyre and Foulsham, 2018). Analyzing teachers' scanpath can increase, for example, our knowledge about classroom management by uncovering how teachers deal with classroom distractions—do teachers succeed in refocusing their attention on what was relevant after an unimportant distraction from an object (e.g., cell phone) or another student, and are experts better able to refocus their attention? McIntyre and Foulsham (2018) found that experienced teachers prioritize and order the way they scan the classroom. The authors showed not only that experienced teachers were able to distribute their gaze more evenly among the students, but also that they followed a sequential pattern of observation. For example, their results suggest that experts initially fixate on a student and return to observe them more frequently and regularly than novices do after each diversion, which suggests that students are a consistent component in the experts' scanpath patterns. Novices, on the other hand, did not routinely return to the initially fixated student after a diversion and continue to observe other students. This first evidence of differences in teachers' scanpaths being related to expertise is essential to the development of knowledge about perceptional sequences in the teaching profession. While McIntyre and Foulsham (2018) analyzed teachers' scanpaths within the context of classroom management, no study to date has examined teachers' scanpaths in the context of an assessment situation, in which teachers must make inferences about students and their current states of underlying learning-relevant characteristics (e.g., self-concept). Accurate teacher assessments are crucial to adapting their pedagogical actions to students' individual needs and to support students' individual learning progress (Klieme et al., 2009).
In this study, teacher gaze data stems from one of our recent eye-tracking experiments (Seidel et al., 2020), in which teachers observed an authentic video clip of a lesson and were asked to assess marked students afterward. This assessment situation was appropriate to the detection of differences in visual gaze patterns, as teachers actively needed to search for and extract information from behavioral cues to form an accurate judgment. Previous research can provide very little insight into this complex process. It is not yet clear how teachers order and distribute their attention during an assessment situation like this one, nor whether there is a relationship between gaze sequences and teachers' assessment competence. For example, (1) do they monitor all students regularly in recurring sequences, or do they focus only on specific students? And (2) are teachers more successful in their subsequent assessment if they monitor all students equally because they avoid missing relevant behavioral cues? This paper aims to deepen our understanding of relationships between teachers' visual expertise and their ability to judge and assess students' underlying learning-relevant characteristics. Moreover, the present paper follows an expert–novice comparison paradigm, and aims to introduce new information about how exactly the visual behavior of expert and novice teachers differs during a diagnostic task, and whether a visual strategy can be extracted from experts' scanpaths, which differs from that of novices. In addition, we introduce a promising method to quantify the complexity of scanpath patterns (Shannon's entropy; Shannon, 1948) and explore how visual behavior is related to the ability to form accurate judgments about students' underlying learning-relevant characteristics.
Professional Vision: The Ability to Notice and Reason About Complex Events in the Classroom
Teachers cannot give equal attention to everything that happens in the classroom—instead, they must selectively focus their attention on specific events that seem significant at that particular moment, for example, a discussion between students. The concept of professional vision (Goodwin, 1994) deals with this crucial feature of teaching expertise. Professional vision is regarded as a situation-specific use of abilities and indicates how teachers' professional knowledge base is applied and linked to practical performance (Lachner et al., 2016). Professional vision implies two interconnected processes: (1) noticing, describing teachers' ability to direct their attention to relevant classroom events and cues; and (2) knowledge-based reasoning, referring to teachers' ability to interpret these events and anticipate consequences for further learning (Goodwin, 1994; Seidel and Stürmer, 2014).
The majority of prior research on professional vision is based on studies that used video examples of classroom teaching. These studies aimed (1) to measure professional vision (Seidel and Stürmer, 2014); (2) to identify differences between experts and novices (Wolff et al., 2016; Meschede et al., 2017; Wyss et al., 2020); or (3) to foster teachers' professional vision in teacher education programs (van Es and Sherin, 2010; Stürmer et al., 2013a,b; van Es et al., 2017). Studies such as the one conducted by Meschede et al. (2017) showed that novice teachers were distinctly less proficient at noticing relevant classroom features and events when compared to experts. Moreover, professional vision, like any other professional competence, is mainly acquired through deliberate and consistent practice over a long period (Berliner, 2001), indicating that professional vision is primarily a characteristic of experienced teachers (Berliner and Clarridge, 1991). To foster teachers' professional vision, van Es and Sherin (2010) used “video club” interventions in which teachers watched and discussed excerpts of authentic video lessons. They found substantial changes over time in what teachers noticed and how they interpreted these events.
Despite this increased focus on teachers' noticing process in recent years, little is known about their perceptual attention processes, which are strongly linked to the noticing component of professional vision (Lachner et al., 2016). Previous studies, outlined above, were mostly based on qualitative analyses of think-aloud protocols and transcripts (Berliner and Clarridge, 1991; van Es and Sherin, 2010) or of video observation with subsequent questionnaires (Meschede et al., 2017). However, eye-tracking offers a useful methodology for investigating teachers' professional vision and perceptual attention processes (Gegenfurtner et al., 2011). Eye-tracking metrics, such as fixations and fixation durations, can be used to identify where teachers direct their attention and process visual information. Previous studies have shown that expert teachers exhibit remarkable differences regarding eye-movement behavior when compared to novices (van den Bogert et al., 2014; Wolff et al., 2016; Seidel et al., 2020). For example, van den Bogert et al. (2014) have found that expert teachers are better able to distribute their attention (fixations) equally across all students while teaching, compared to novices. Wolff et al. (2016) reported that experts show (1) shorter fixation durations, (2) more task-relevant fixations, and (3) fewer fixations on task-redundant areas. These empirical results provide evidence that experts' visual perceptions approximate the principles of good classroom management (Levin and Nolan, 2014)—for example, equal distribution of attention to all students.
Human eye movements can be categorized into two main processes: bottom-up attention is driven by external features of a visual stimulus that are salient to the perceiver (bright colors, movements) while top-down attention is driven by task-related plans, intentions and current goals derived from professional knowledge (Gegenfurtner et al., 2011; Goldberg et al., 2020). After years of practical experience in teaching, teachers are likely to have restructured their professional knowledge base and formed practice-related cognitive schemata that guide their actions during classroom teaching (Boshuizen et al., 1995; Heitzmann et al., 2019). These cognitive schemata are, therefore, a central top-down driver for professional vision (Gegenfurtner et al., 2011; Seidel and Stürmer, 2014; Lachner et al., 2016).
Two further theoretical approaches can explain why noticing processes undergo changes with increased expertise (see a comprehensive meta-analysis across diverse professional domains; Gegenfurtner et al., 2011). First, based on a theory of long-term working memory, Ericsson and Kintsch (1995) found that experts were able to expand their working memory capacity; through hundreds of hours spent in the classroom, teachers had repeatedly experienced situations and stored this information in their long-term memory. Experienced teachers set up a retrieval structure in the long-term memory with which they activated interconnected knowledge such that it was readily available for use in working memory. This retrieval structure allowed experts to process more visual information and larger perceptual chunks in their domain of expertise. Second, the information-reduction hypothesis (Haider and Frensch, 1996) states that with increasing experience, teachers learn to separate task-relevant from task-redundant information. By reducing information that is not relevant to a particular task, experts can actively focus on the information relevant to the task and have a greater capacity to cognitively process relevant information.
In conclusion, the theories and empirical studies outlined so far point to the fact that teachers, through increasing experience, develop and restructure cognitive schemata (top-down processes) which in turn lead to different ways of visually perceiving and processing information when compared to novices. So far, most eye-tracking literature in teacher research has used raw gaze metrics such as the number of fixations (e.g., on single students or monitoring classroom events). These raw gaze metrics have been shown to be suitable for shedding light on important expert–novice differences, but such metrics cannot cover the processual nature of eye movement behavior. Looking more closely into gaze sequences, however, can yield rich information, especially for social interactions as they typically occur during teaching (McIntyre and Foulsham, 2018). Looking at the scanpath structure in this context might be a suitable approach, since the scanpath represents the exact spatial sequence of eye movements performed by an individual during a task. A scanpath also reflects the unfolding of visual attention over time, indicating exactly which contents of the visual information are attended to. In light of reported differences between the gazes of expert and novice teachers, it is conceivable that scanpath patterns are affected by teachers' experience. This paper aims to explore how scanpaths of teacher gaze change over the course of professional development. The so-called Scanpath Theory (Noton and Stark, 1971a,b) serves as a further theoretical framework and is reviewed in the next section.
Scanpath Theory
The Scanpath Theory was defined by Noton and Stark (1971a,b) and has become a highly relevant theory for understanding human eye movements and gaze patterns. Scanpath Theory argues that individuals looking at an image or a specific scene store both the scene features and the gaze sequence used to inspect that scene. Noton and Stark put forward the hypothesis that individuals who recognize a previously seen scene follow a scanpath similar to the one resulting from their initial viewing. In subsequent work, and based on their study of human facial recognition, Kanan et al. (2015) presented a less strict version of the Scanpath Theory, which they called “scanpath routines.” This version of the theory takes into account the fact that in real-world situations, humans rarely come across the same visual stimuli twice. Therefore, eye movements of individuals can rather be said to be similar between viewings of scenes or images from the same stimulus class—for example, classroom scenes from the teachers' perspective. These scanpath routines in a specific stimulus class evolve to enable improved visual processing (Kanan et al., 2015), for example, by filtering important and unimportant information (Haider and Frensch, 1996).
Experimental eye-tracking studies performed over the last decade have supported the Scanpath Theory. These studies found scanpaths to be repetitive and that an individual's scanpath pattern was idiosyncratic (Foulsham et al., 2012; Kanan et al., 2015; McIntyre and Foulsham, 2018; more similar within an individual than between individuals). This evidence supports the hypothesis that internal cognitive structures control not only eye-movements, but also the perception process itself. During the perceptual process, foveations enable the verification and adaptation of sub-features of cognitive structures. Based on these assumptions, human visual perception is seen mainly as a top-down process (Stark and Choi, 1996), which, however, still includes possibilities for adaptation of sub-features. If scanpaths are more guided by underlying cognitive schemata such as a professional knowledge base, it can be expected that scanpaths are, indeed, affected by experience. As a consequence, experts should produce significantly different scanpaths while viewing a professionally relevant scene than novices. To date, scanpath analysis has found application in the assessment of expertise level in domains such as medicine, for example detecting anomalies in radiographs (Kundel et al., 1978, 2007; Kelly et al., 2016); art (Antes and Kristjanson, 1991); and chess (Charness et al., 2001). These studies together showed significant differences in the scanpath routines of experts and novices, which in turn pointed to more efficient scanpaths and search patterns for experts. For instance, expert radiologists demonstrated that they were able to reduce an entire image more quickly to process a smaller section of the image, compared to novices. These findings indicate that through increasing expertise, radiologists change their visual behavior and implement a scanpath routine for this specific visual search task, for example a “global-to-foci” search strategy (Kundel et al., 1978).
The existing body of research about teachers' scanpath routines is limited and cannot yet give an accurate answer for the extent to which the underlying scanpaths of expert and novice teachers differ. However, the theoretical drivers behind the present study and results of previous research using eye-tracking metrics, such as fixation duration or the number of fixations (e.g., Wolff et al., 2016), emphasize the conclusion that expertise differences are also to be expected among teachers' gaze patterns. Experienced teachers gain knowledge-informed cognitive schemata (Stürmer et al., 2013a) and should therefore be more knowledge-driven compared to less experienced teachers (Seidel and Stürmer, 2014; McIntyre and Foulsham, 2018).
Recently, McIntyre and Foulsham (2018) made a major contribution to this line of study. In their real-world experiment using mobile eye-tracking data, they investigated the differences in scanpath patterns of teachers with varying levels of expertise. They found (a) that there were more similarities between a teacher's own scanpath patterns than when compared to those of other individuals (idiosyncratic), and (b) that scanpath patterns were more similar within expertise groupings. Furthermore, their qualitative scanpath analysis indicated that experts' scanpaths are more guided by strategy; for example, expert teachers restricted their gaze to the most task-relevant areas while novice teachers' scanpaths were more distracted by salient task-irrelevant events (McIntyre and Foulsham, 2018). In addition, the experts were able to refocus on the students after their gaze had been distracted. While these results provide important insight into how expertise affects teachers' scanpath routines, they are limited to the context of classroom management. This paper addresses the need for further investigations of teacher scanpaths in the context of observing and reasoning about individual students and their underlying learning-relevant characteristics. So far, we know little about the specific visual strategies that teachers use during a task in which they must monitor and assess several students.
Linking Teacher Gaze to Teachers' Assessment Competence
The aim of this section is to link teachers' visual behavior (noticing) to their ability to accurately judge underlying learning-relevant student characteristics. Teachers' ability to accurately judge student characteristics depends heavily on visual perception and attention allocation, because they gain information and collect behavioral cues. The primary source of information in this scenario is student observation during lessons.
Performance in Assessing Learning-Relevant Student Characteristics
Accurately assess the state of students' cognitive (e.g., pre-knowledge) and motivational-affective (e.g., self-concept) characteristics is an essential component of professional teacher competencies (Herppich et al., 2017) and a prerequisite for teachers to provide tailored instruction (Corno, 2008). Prior research tackled the question of how accurately teachers judge learning-relevant student characteristics and found that teachers assessed students' abilities (Machts et al., 2016) and achievement (Südkamp et al., 2012) relatively accurately, and tended to have more problems in assessing students' motivational-affective characteristics such as self-concept (Praetorius et al., 2013) or interest (Karing, 2009). Other research showed that teachers perceived students holistically, and intermingled distinct student characteristics when they were asked to judge cognitive abilities and motivational characteristics separately (Kaiser et al., 2013).
However, it remains relatively unclear which specific cognitive processes and behavioral activities underlie teachers' assessments (Leuders et al., 2018; Loibl et al., 2020; Schnitzler et al., 2020). Established models from social and general psychological (Brunswik, 1955; Fiske and Neuberg, 1990; Chaiken and Trope, 1999; Lens model; dual process theories) have helped to understand teachers' judgment processes. For example, the lens model of perception, introduced by Brunswik (1955), can be used to describe teachers' decision-making process: in trying to apprehend a latent distal trait (i.e., a student's characteristics), the teacher only has at their disposal imperfect indicators, or cues, of that distal trait (e.g., behaviors that are related to a specific student characteristic). Since there is typically more than one cue available, the teacher's task is to combine information gathered from these uncertain cues to reach the best judgment. Hence, teachers' decision-making process can be separated into a professional vision of student cues and the correct combination and subsequent interpretation of those cues with respect to the teacher's professional knowledge base. Teachers' judgment accuracy, therefore, depends first on whether critical student cues are noticed, then whether noticed cues are related to the actual distal trait, or—if cues are misleading (cue validity)—the degree to which teachers build their judgment on noticed cues (cue utilization) (Brunswik, 1955; Leuders et al., 2018). Moreover, other theories have their roots in social psychology and are generally described as dual-process theories of judgment under uncertainty (Fiske and Neuberg, 1990; Chaiken and Trope, 1999; Fiske et al., 1999; Ferreira et al., 2006), which can also be applied to better understand teachers' underlying cognitive processes during judgment processes. Dual-process theories have in common that mental processes are divided into two basic forms of reasoning, depending on whether they operate automatically or controlled. For example, Fiske and Neuberg (1990) continuum model of impression formation suggests people form impressions of others through various processes that operate along a continuum that reflects the degree to which people utilize category-related vs person-specific information. The category-related processing mode operates automatically and requires little cognitive effort because this mode involves the activation and processing of heuristic knowledge about the person to be judged. The person-specific processing mode is rather complex and associated with higher cognitive effort as more available information about the person to be judged is processed systematically. The model follows a sequence: First, the perceiver initially categorize others immediately upon easily noticeable cues (e.g., clothing, skin color) If the degree of personal relevance of the perceiver is high enough to warrant further processing (step 2), the perceiver will attend to other noticeable information to form an impression beyond the initial and rapid categorization (step 3). Fourth, the perceiver will try to assimilate the collected information about the target into the initially identified stereotype. If this is successful, the judgment about the target will be based on the initial categorization (step 1). However, if the target person's information is contradictory and cannot be categorized in the initial assessment, re-categorization will follow (step 5). During the re-categorization, the perceiver tries to find another adequate category by re-organizing the current amount of information or by including some extraordinary features of the target. If this is also not successful, the perceiver will process the model's most complex stage—the piecemeal integration (step 6). The perceiver will integrate all information available in an attribute-by-attribute assessment of the target's characteristics, if the perceiver has sufficient resources and motivation. It is assumed that more systematic information processing about a target leads to a more accurate judgment, whereby heuristic information processing results in less accurate judgments (Fiske et al., 1999). Fiske and Neuberg point out that piecemeal integration often occurs when perceivers assess many individuals based on a few specific characteristics. Dual-process theories have also found application in a recent model of teachers' assessment competence by Herppich et al. (2017). The authors point out that teachers form their judgments about students on the continuum of the two modes of processing described above and presented the following illustrative example (cf., Herppich et al., 2017): A new student will join the class, and the teacher's goal is to get a first impression of the students' achievement level in order to integrate the student the best way. The teacher heard from colleagues that the student achieved good grades in the last year. This information heuristically leads to inferences about the current achievement level, and the teacher decides to arrive at the judgment that the new student will be easily integrated into the class. The same teacher will execute a rather systematic and multi-cyclic assessment when the judgment has more consequences for the student (e.g., school tracking decisions). In this case, the teacher might integrate all available information to come to an accurate judgment.
Overall, the theories outlined above explain how teachers process information and translate collected information into judgments, but prior research that integrates attention allocation (e.g., gaze) to better understand the mechanism underlying judgment processes is still scarce. Karst and Bonefeld (2020) pointed out that (higher) attention allocation to the target person does not explicitly guarantee that the judgment accuracy is high or increases, but that attention allocation can be considered as a relevant prerequisite for judgments. Especially during person-specific, piecemeal integration of individual information, greater attention is required to process all additional information (Karst and Bonefeld, 2020).
Visual Expertise and Assessment Competence
Visual expertise and assessment competence so far have been studied separately from each other in a number of research strands (Gegenfurtner et al., 2011; Balslev et al., 2012; Praetorius et al., 2013). Therefore, limited knowledge has been provided with regard to the question of how visual expertise can affect professional outcomes, such as assessment competence. Research on visual expertise of physicians has provided nuanced understandings about visual behavior and diagnostic decision-making during the diagnosis of medical images (e.g., x-rays) (Tiersma et al., 2003; O'Neill et al., 2011) or patient video cases (Balslev et al., 2012). Balslev et al. (2012) demonstrated that experts were better diagnosticians compared to novices and that experts' visual behavior was more focused on diagnostically relevant features of the visual stimuli. O'Neill et al. (2011) found that experts demonstrated more systematic and circumferential gaze patterns, which were related to higher diagnostic accuracy. On the contrary, the gaze patterns of novices were more local, less systematic, and lacked diagnostically relevant features, which may have been the reason for their lower diagnostic accuracy. In addition, it was shown that regardless of expertise, a higher proportion of total time spent examining diagnostically relevant features went along with a more accurate diagnosis.
Taken together, eye-tracking literature from medical research indicates that visual behavior and scanning patterns are related to assessment competence. To the best of our knowledge, such studies are scarce in teacher education research. Thus, there is not yet a great deal of knowledge about how fixation pattern and gaze behavior are related to judgement accuracy in professional tasks—such as teachers' ability to assess learning-relevant student characteristics. In a recent study on this kind of professional task (Seidel et al., 2020), it was found that experts gave more visual attention to students who might require pedagogical action (e.g., students who were “struggling”). Another finding was that experts were more accurate in judging inconsistent combinations of learning-relevant student characteristics (e.g., underestimating students who demonstrated high expressions of characteristics in the cognitive domain, but low expressions with regard to self-concept of ability). Whether experts and novices might also differ in their visual strategy (scanpaths), and whether these differences are related to their assessment competence, have so far remained open questions.
The Present Study
The present study extends our previous work on the same dataset and experimental setup (Seidel et al., 2020), in which expert and novice teachers were asked to observe a video clip of an authentic teaching situation (see Methods–Procedure–Video Stimulus) and to assess five students based on their underlying learning-relevant student characteristics (see details in Seidel et al., 2020). The current research goals are to find first evidence of teachers' scanpath routines during the above-described assessment situation and to uncover whether scanpaths are affected by differences in level of expertise. Additionally, this study aims to shed light on the so far relatively unknown routines of experts' scanpaths, which only become visible when taking the spatial order of fixations into account. Finally, we want to bridge the gap between teachers' visual perception of student behavioral cues and their subsequent reasoning about underlying learning-relevant student characteristics. Therefore, we aim to explore whether different visual strategies are linked to differences in teachers' assessment competence. The following research questions and hypotheses guided this investigation:
RQ1a: Are teacher scanpaths (1) of an idiosyncratic nature and (2) more similar within expertise groups?
First, following the study by McIntyre and Foulsham (2018), we will examine whether scanpaths of teachers are also of an idiosyncratic nature in an assessment situation. The findings aim to support the idea that teachers' visual perception is mainly a top-down process. We therefore expect that teacher scanpaths are significantly more similar when compared within an individual than when compared between individuals (H1). Second, if scanpaths are, in fact, guided by cognitive schemata in a top-down process, then scanpaths of individual experts should be more similar to the scanpaths of other experts than those of a group of novices, and the scanpaths of individual novices should be more similar to those of other novices (H2).
RQ1b: Do scanpaths of experts include recurring sub-patterns—a consistent visual strategy—that differ from recurring sub-patterns in novice scanpaths?
If scanpaths of experts are more similar within the group of experts, then the question arises whether there are specific and regular patterns that indicate an expert visual strategy that differs from that of a novice. Based on previous findings showing that experts spread their eye movements more evenly across all students (van den Bogert et al., 2014), we expect that this might be the first indication that experts, in the process of assessing students, also demonstrate a visual strategy that is more consistent across all students (H3). We assume that experts are able to process all incoming information more effectively and that they are able to make more cross-comparisons between multiple students to form an accurate judgment. As Fiske et al. (1983) pointed out, experts' extra capacity potentially frees them to process additional relevant information, whereby novices do not yet can handle the amount of information, and they might become cognitive overwhelmed.
In addition, to confirm that top-down processes control teacher gaze, RQ1a and RQ1b were investigated in two different classroom context scenes, varying with regard to the assumed amounts of top-down vs. bottom-up drivers. The two different classroom scenes are described in detail further on (see Methods–Procedure–Video Stimulus).
RQ2: Is there (a) a relationship between teachers' visual strategy and their judgment accuracy, and (b) are there systematic differences between experts and novices?
This explorative research question aims to bridge the gap between teachers' visual behavior (the noticing component) with their ability to assess different student profiles accurately (reasoning about collected cues). Based on theoretical considerations of teacher professional competencies (Blömeke et al., 2015) while acknowledging limited prior research, we tentatively assume a positive relationship between teachers' visual behavior and their assessment competence. For example, experts may systematically and repeatedly spread their gaze to all targeted students and, therefore, manage to perceive more crucial behavioral cues. We assume that the greater the number of relevant behavioral cues that are perceived, the better teachers can assess individual students.
Methods
Participants
High-quality eye-tracking data (Mtrackingratio = 0.94 and average deviation x-axis = .58°, y-axis = 57°) were available for 44 participants. Among them were 35 novice teachers (female = 60.5%) enrolled at university level in a bachelor's teacher training program to become teachers in German high-track secondary schools for science or mathematics. Furthermore, data were available for nine in-service teachers (female = 70.5%) with an average teaching experience of 12.40 years (SD = 8.58, range = 1.5-25.0 years).
Procedure
Data collection took place in the university laboratory. First, participants were introduced to learning-relevant student characteristics (i.e., self-concept, pre-knowledge), as well as their complex combinations, so-called student profiles (Seidel, 2006; Seidel et al., 2016). Next, participants were instructed to carefully observe a video stimulus (11 min) showing a typical teaching-learning situation, and were then requested to assess the profiles of five target students. The five students to be assessed were continuously marked with five randomly selected letters (B, E, K, P, and T) throughout the video to ensure that participants were always aware of which students to observe and assess. After participants had watched the video and eye-tracking had been recorded, the assessment situation began, with participants assessing the profile of each marked student.
Video Stimulus
The video (11 min) originates from a previous video study on teacher-student interactions in classrooms (Seidel et al., 2016). The video clip showed an authentic eighth-grade mathematics lesson from a German high-track secondary school (22 students are constantly visible), and consisted of two segments. The first segment primarily comprised scenes of “whole-class instruction,” in which the teacher stands in front of the students and introduces a topic while some students occasionally raise their hands to give answers. The characteristics of this scene suggest that bottom-up drivers could be more involved because eye movements of observers may be controlled by salient cues from students (e.g., hand-raisings) — teacher gaze than follow mainly the course of the video. In contrast, the second segment consisted mostly of “individual work” in which the teacher speaks for a longer time, and students listen to the teacher and work alone on tasks. This scene is characterized by less motion from students (e.g., fewer hand-raisings) and should provide a context in which top-down drivers may be activated.
Student Profiles
Each of the five marked students in the video described above represented a so-called student profile (Seidel, 2006; Seidel et al., 2016). Student profiles were empirically identified in prior research studies (Seidel, 2006; Seidel et al., 2016) using latent profile analysis (LPA). This research tackles the question to identify homogenous subgroups of students that are statistically distinct from each other, meaning that each of the identified subgroups showed a unique pattern of cognitive (i.e., pre-knowledge) and motivational-affective characteristics (i.e., self-concept). For example, students with high self-concept and high pre-knowledge are grouped into a particular student profile (i.e., so-called strong students) and statistically separated from students who, for example, have a high self-concept but little pre-knowledge (i.e., so-called overestimating students). The student profiles we used in the present study stemmed from a larger video study (Seidel et al., 2016) and were created based on two learning-relevant student characteristics: students' self-concept and pre-knowledge in mathematics. Both student characteristics are important predictors of students' school achievement (e.g., Ausubel, 2000; Huang, 2011) and highly relevant for teachers because they often consider information about student characteristics when planning their pedagogical instruction and grading students (Landis, 1984).
The five marked students represented each of the following student profiles: strong (overall high values), struggling (overall low values), overestimating (high self-concept but low pre-knowledge), underestimating (low self-concept but high pre-knowledge), and intermediate (average self-concept and pre-knowledge). More detailed information about student profiles can be found in person-centered research (Seidel, 2006; Lau and Roeser, 2008; Linnenbrink-Garcia et al., 2012; Seidel et al., 2016).
Teacher Judgement Accuracy
The assessment situation required participants to assign each marked student to one of the five listed student profiles (as described above). In case they were uncertain, they were also able to assign an additional, alternative profile. If participants assigned the student to the correct profile, thereby judging the student correctly, they were awarded one point. If a teacher first assigned an incorrect profile but stated the correct profile in their alternative choice, they received half a point. If their first and second choices were both wrong, they received zero points. The total score could range between zero (no correct judgment) and five points (only correct judgments).
Apparatus
Eye movements were recorded using the static and lab-based SMI RED 500 binocular eye tracker using Experiment Center 3.7 software (SensoMotoric Instruments, 2017) on a 22-inch display monitor and at a sampling frequency of 500 Hz. Eye-tracking conditions were standardized for all participants (constant ceiling light, 65 cm distance to eye-tracker, use of a chinrest). Moreover, before beginning eye-tracking, a 9-point automatic calibration followed by a validation was implemented to ensure data quality. The calibration was performed again if the 9-point automatic failed.
Data Analysis
First, we wish to describe which preparatory work was conducted, and then illustrate which analytical steps were carried out. Preparatory work included the creation of five Areas of Interest (AOI). Each of the AOI represented a target student (Figure 1) and was drawn manually using SMI BeGaze 3.4 (SensoMotoric Instruments, 2017). Subsequently, we identified eight short teaching events in the scene: four events included primarily “individual work,” and four events included “whole-class instruction.” The teaching events were, on average, 43 seconds long. We then generated scanpaths for each of the eight teaching events, whereby the built-in saccade and fixation detector of SMI BeGaze 3.4 (see details: SensoMotoric Instruments, 2017) was used. The raw eye-tracking data were converted into strings using the conversion application smi2ogama (Dolezalova and Popelka, 2016), meaning that the fixation sequence was recoded into a sequence of strings representing the fixation locations. Finally, we obtained multiple scanpaths as strings (e.g., TEKBP) for every participant and each of the eight teaching events extracted from the two different classroom context scenes described above.
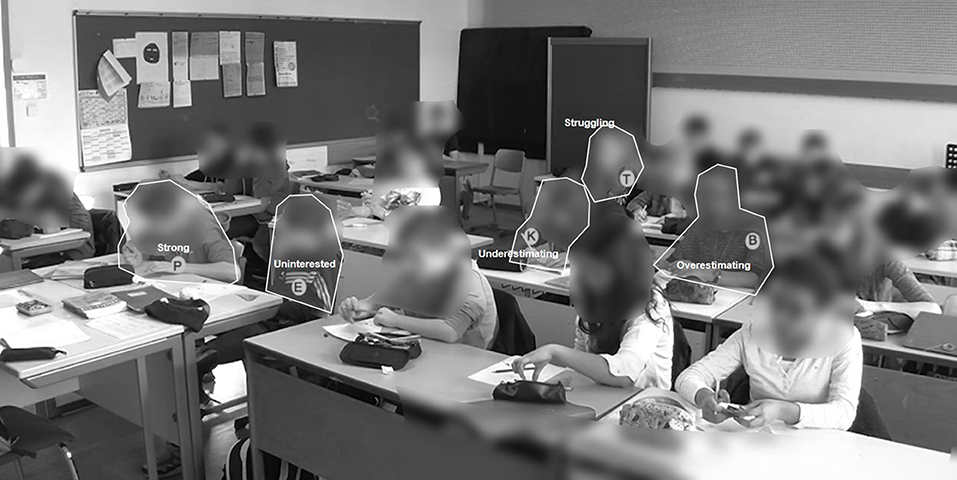
Figure 1. Video stimulus for eye movement analysis. This is an exemplary screenshot of the classroom and used AOIs. AOIs are only marked for illustration in this paper; they were not visible to the participants. The blurring of student faces is only added for the presentation in the publication to ensure the protection of data privacy; it was not visible when drawing the AOIs. Students were marked with letters not referring to any underlying profile: B, E, K, P, and T. This figure was previously published as Video stimulus for eye movement analysis by Seidel et al. (2020) and is licensed under CC BY 4.0.
One widely applied technique for comparing scanpaths is Levenshtein distance (LD) (Levenshtein, 1966), also known as the optimal matching analysis (Abbott and Tsay, 2016). This string-edit algorithm is used to measure the dissimilarity of character strings. In this method, a sequence of basic mathematical operations (deletion, insertion, or substitution) is used to transform one sequence of strings into another. The more similar two scanpaths are, the fewer mathematical operations need to be performed, and the lower the cost of converting one string to another. LD has been commonly used to analyze and compare scanpaths (Mathôt et al., 2012; Davies et al., 2016; McIntyre and Foulsham, 2018). After finishing the above-described preparatory work, we pursued the following data analysis strategy:
1. Use of LD to measure the similarity of scanpaths (a) within individuals compared across individuals (H1) and (b) across expertise groupings (H2). LD was calculated using the R package stringdist 0.9.5.5 (van der Loo, 2014). LDs were calculated for all pairwise scanpaths. To account for varying lengths of scanpaths, we normalized the LD by dividing the LD by the length of the longer scanpath and then subtracting from 1. As a result of this normalization, we obtained LD similarity scores (subsequently referred to as LDss) valued between [0, 1], whereby values near one indicated that both scanpaths were nearly identical.
2. For statistical analyses of the different sets of LDss, we ran repeated-measure ANOVA separately for each of the two different classroom context scenes.
3. To potentially detect and explore visual strategies and to uncover expert-novice differences (H3), we analyzed the data with the R package GrpString 0.3.2. (Tang and Pienta, 2017). GrpString enabled us to identify common sub-patterns (repetitive scanpath patterns), which are defined as sub-sequences within scanpaths that are found more than once with a minimum length of three characters (e.g., ABC). GrpString lists the sub-patterns with how many times they are seen in the scanpaths and how many scan paths are inclusive of the particular sub-pattern. Longer sub-patterns (e.g., ABCDCA) may contain multiple shorter sub-patterns (e.g., ABC, DCA, CDC etc.). Moreover, in order to explore gaze transitions from one AOI to another AOI (i.e., change of gaze from student A to student B), we computed transition matrices. A gaze transition is defined as a substring with two characters (e.g., AB).
4. We then introduced a rarely used but potentially valuable method of capturing and analyzing the complexity of scanpaths, Shannon's entropy of information, which is grounded in information theory (Shannon, 1948). Entropy measures the information in a variable in terms of ordering and complexity, and is defined as:
Where H(R) is the entropy in units (so-called bits) and p (ai) is the proportion of measurement ai (Shannon, 1948; see details: Hooge and Camps, 2013). Consider the example of a fair coin flip, in which the chance of each outcome is equal—it is a situation with maximum uncertainty because it is difficult to predict the outcome of the next coin flip. However, if we know that the coin is not fair and that the probability p(h) is higher compared to b(n), where p ≠ b, then we have less uncertainty, quantified in a lower entropy coefficient. Calculated for transition matrices, this means that the lowest possible entropy is zero. In this case, there is no uncertainty which transition between different AOI will occur (all cells in the transition matrix have the same value). The maximum entropy value occurs when all cells in the transition matrix have different values. Thus, from that analytical perspective, when Shannon's entropy coefficients are high, individuals look at every AOI with equal frequency and transition between all possible AOI combinations with approximately equal frequency (Hooge and Camps, 2013), indicating more complex scanpaths. In research about teacher's attentional processes, gaze entropy can be used to describe teachers' gaze distribution across multiple students or students and teaching-related objects (e.g., board) (McIntyre et al., 2017). A high entropy eye movement pattern occurs when the teacher distributes his/her attention equally among many students and when, after fixing one student, all other students have a similar probability of being looked at. McIntyre et al. (2017) refer to the term gaze flexibility, where greater gaze flexibility is related to a visual behavior where teachers can alternate their visual attention between different students or student-related material. Besides, Krejtz et al. (2014) pointed out that the calculation and use of Shannon's entropy coefficient has two advantages: first, it is possible to capture the complexity of visual behavior represented by a single value per individual, which can then be averaged across groups; and second, the Shannon's entropy coefficient allows for subsequent analysis using basic statistical methods (i.e., t-test, regression analysis) (Krejtz et al., 2014). Entropy analysis is an important step toward a better understanding of teacher attentional processes and is particularly useful for spotlighting the differences revealed in scanpath similarity studies.
5. In the last step, we performed a multiple regression analysis to investigate the relationship between entropy, teachers' assessment competence, and expertise level. Furthermore, two frequently used eye-tracking metrics (average fixation duration and number of fixations) were included as control variables in the regression analysis. We are not specifically interested in examining the relationship between averaged metrics and judgment accuracy in the present study, but we want to control the effect of both variables, as they may also be related to judgment accuracy.
Results
Nature of Teacher Scanpaths
Our first research question addresses the nature of teacher scanpaths and defines the extent to which teacher scanpaths are of an idiosyncratic nature and more similar within expertise groups.
Intra- vs. Inter-individual Scanpath Similarities
The first hypothesis states that scanpaths are more similar if they are from the same individual than if they are from different individuals. To evaluate this hypothesis, we calculated a set of intra-individual LDss (i.e., how similar multiple scanpaths are within an individual) as well as a set of inter-individual LDss (i.e., how similar scanpaths of an individual are when compared to all other individuals). The repeated-measure ANOVA revealed that for the “whole-class instruction” scene that a teacher's gaze behavior was more similar to itself (MLDss = 0.54) than to that of other teachers (MLDss = 0.49), F(1, 43) = 8.40, p = 0.006, η2 = 0.16. An analogous result was also found for the “individual work” scene [itself: MLDss = 0.52; other teachers: MLDss = 0.47; F(1, 43) = 11.09, p = 0.002, η2 = 0.21].
Effect of Teaching Expertise on Scanpaths
In the second hypothesis, we expected for scanpaths of experts to be more similar to other experts than to novices and vice versa. To test this hypothesis, we calculated a second set of LDss. To assess expert-novice scanpath differences, we computed within-expertise mean LDss (i.e., teachers' scanpaths were compared within their level of expertise) and across-expertise mean LDss (i.e., experts' scanpaths were compared to novices' scanpaths). The repeated-measure ANOVA indicated for the “whole-class instruction” scene that teachers were more similar to other teachers with the same expertise (within expertise group LDss; MLDss = 0.61) than to teachers from different expertise groups (between expertise group LDss; MLDss = 0.50), F(1, 43) = 21,74, p = 0.003, η2 = 0.24. Next, within/between expertise LDss were analyzed for the “individual work” scene. Again, the repeated-measure ANOVA revealed that teachers' scanpaths were more similar within their expertise group (MLDss = 0.59) than when scanpaths were compared between experts and novices (MLDss = 0.48), F(1, 43) = 39,74, p < 0.001, η2 = 0.28.
Sub-patterns and Gaze Transitions of Experts and Novices
Our second research question aimed to uncover whether experts have a consistent visual strategy that is different from the visual strategy of novices. To get a better idea of the specific visual strategies shared by teachers from different expertise groups, we created transition matrices, including absolute and normalized numbers of transitions between two AOIs. We then extracted common sub-patterns from scanpaths. The results of these exploratory and qualitative analyses are presented in the following sections.
Gaze Transitions
The absolute and normalized frequency of transitions between two AOIs is shown in Table 1. This descriptive and qualitative analysis reveals both similarities and differences in gaze-switching behavior: On one hand, both experts and novices switched most frequently between student K and student T and vice versa. On the other hand, if the transitions are sorted by frequency, the gaze-switching behavior identified above (i.e., repetitive focus on two students) was more consistent among novices (see 10 most common transitions, sorted by frequency: KT, TK, PB, BP, KB, BK, EK, KE, TB, BT). Experts displayed these transitions considerably less frequently (see 10 most common transitions, sorted by frequency: KT, TK, PE, TB, BT, EK, ET, BK, KE, KB).
Sub-patterns
The overall frequencies and ratios of occurrence of common sub-patterns are presented in Table 2. We were able to identify common sub-patterns with a length of up to five strings. When comparing the most common sub-patterns, it became clear that the difference was more likely to be identified between expertise groups than between the two classroom scenes. Considering the sub-patterns of both expertise groups, it became visible that sub-patterns of experts covered a broader spectrum of different students compared to novices. For example, in the classroom talk scene, the most frequently identified sub-pattern of experts with a length of five strings (BTKET) included four different students who were inspected by experts one after another. On the contrary, the most frequently identified sub-pattern with a length of five strings (KTKTK) in the group of novices included only two different students. Moreover, the sub-pattern KTKTK was found multiple times within single scanpaths, as indicated by the high number of the overall frequency ratio (188%). These recurring sub-patterns were found in the group of experts only for sub-patterns with a length of four characters (the ratio of the sub-pattern EKTB was 144%). Besides, with regard to the sub-pattern consisting of three strings, it became clear that experts and novices prompted somewhat different visual behavior. Highly recurring sub-patterns were found for experts as well as for novices. The most recurring sub-pattern within the group of experts was KTB (422%) and included three different students, whereas in the group of novices, the most recurring sub-pattern was KTK (622%) and included only two different students.
The Relationship Between Visual Behavior and Teachers' Judgement Accuracy
In the final step of the study, we were interested in exploring the relationship between teachers' visual behavior and judgment accuracy. Before running multiple regression analysis, we computed a set of t-tests to identify expertise differences in entropy coefficients and judgment accuracy. We found a statistically significant difference between experts' (M = 4.56, SD = 0.77) and novices' (M = 3.79, SD = 0.86) mean entropy coefficients, t(42) = 2.95, p = 0.04. However, we found no significant difference between experts (M = 3.27, SD = 1.48) and novices (M = 2.57, SD = 1.23) in mean judgment accuracy scores, t(42) = 1.24, p = 0.22. We then conducted multiple regression to see if the entropy coefficient and expertise level explained variance in judgment accuracy. It was found that the entropy coefficient and expertise level explained a significant amount of the variance in teachers' judgment accuracy, F(4, 39) = 22.52, p < 0.001, = 0.48). The analysis confirmed that expertise level did not significantly predict judgment accuracy (βstd. = 0.12, p = 0.73), however entropy coefficient did significantly predict judgment accuracy (βstd. = 0.54, p < 0.001). We found no significant interaction term. The results suggest that the more frequently teachers varied their monitoring of students (higher entropy), the more they were able to judge students correctly. In addition, we found no significant effect of averaged eye-tracking metrics on judgment accuracy; average fixation duration (βstd. = 0.23, p = 0.65), and number of fixations (βstd. = 0.06, p = 0.51).
Discussion
The teaching profession heavily depends on visual information—teachers must visually perceive, collect, and process information and behavioral cues about their students in order to monitor their learning progress, adjust instruction, or draw conclusions about underlying student characteristics (Doyle, 2006; Wolff et al., 2016). Our eye-tracking experiment shed new light on teachers' visual behavior by taking into account the sequential nature of eye movements (i.e., scanpaths). Scanpath analyses in teacher research are very rare to date, and are more related to teachers' general classroom management skills (McIntyre and Foulsham, 2018). The present study aimed to bring together two lines of research: research about teachers' assessment competencies and research about visual expertise.
Teachers' Scanpaths Are Idiosyncratic and Driven by Expertise
The first research question was related to the assumed idiosyncratic nature of scanpaths in an assessment situation. We were able to provide support for the original descriptions by Noton and Stark (1971a,b) by showing that a teacher's own scanpaths resembled each other more closely than when compared to those of other teachers (H1). This result ties in well with previous studies across diverse psychological research areas—for example, face recognition (Kanan et al., 2015), memory and imagery research (Foulsham et al., 2012), and recently, teacher research (McIntyre and Foulsham, 2018). The results indicated that, in our experiment, teachers observed the authentic teaching video sequences in their own way; therefore, teachers seemed to be guided primarily by top-down (e.g., knowledge and schemata-driven gaze) rather than bottom-up (eye-catching visual features, e.g., light-colored clothing) visual processes. Furthermore, we extended the literature by showing that teachers' scanpaths were idiosyncratic even in a teaching video sequence in which much motion and salient cues were available (i.e., the whole-class instruction scene). Since we assumed that teacher gaze is mostly guided by cognitive schemata in a top-down process, we also expected cognitive structures to change throughout professional development (H2). Individual cognitive structures then converge to professionally shared cognitive schemata and, therefore, we expected experts to systematically differ from novices in their visual perception behavior (Gegenfurtner et al., 2011; McIntyre and Foulsham, 2018). Indeed, results from this study indicate that expert teachers share cognitive schemata that are more similar to other experts when compared to cognitive structures of novices.
Top-Down Driven Gaze: Experts' Scanpaths Included Complex Recurring Scans of All Students
We also found support for research question 1b by showing that the visual strategy of expert teachers differed from the visual strategy of novices on the micro-level, namely teachers' recurring sub-patterns of gaze. Qualitative sub-pattern analysis indicated systematic differences: Experts' most identified and recurring sub-patterns covered more individual students (i.e., four students) compared to novices (i.e., two students). Hence, experts' visual strategy maintained up-to-date information of target students by checking up on all of them more regularly. On the contrary, novices' sub-pattern analysis pointed out another visual strategy, as they made recurring transitions between just two students. Based on these findings, the second research question arose; which strategy was more successful in assessing students and their underlying characteristic profiles? We followed the idea that a quantification of the visual strategy was required to explore the relationship between visual perception and judgment accuracy. We quantified visual behavior using Shannon's Theory of entropy (Shannon, 1948), wherein higher entropy values display more complexity. The results supported the idea that expert and novice teachers followed rather distinct visual strategies. The visual behavior of experts was more complex compared to that of novices. Experts' significantly higher entropy values indicated that they monitored each student with more equal frequency and transferred their gaze between all possible combinations of students with approximately equal frequency. However, novices' significantly lower entropy values indicated that they focused more on specific students and also transferred their gaze between these specific students (which is in line with our qualitative analysis above). Moreover, we found that experts were more accurate in judging students and their underlying student characteristic profiles, but this difference did not reach statistical significance (perhaps due to the small sample size). Finally, we performed regression analysis to explore the relationship between entropy (as an indicator for the complexity of visual behavior) and judgment accuracy, and found that visual entropy explained a significant part of the variance in teachers' judgment accuracy. The more a teacher was able to follow an expert-like strategy (in the form of complex visual behavior), the better their judgment was of students' underlying learning-relevant characteristics.
Based on the outlined findings, the question arises why complex gaze patterns were related to better judgment accuracy in the study's assessment task. In the following, we discuss and combine our findings from two research strands, teacher general judgment models and research with gaze metrics. With regard to the first strand, we point out that our data is limited with regard to being able to provide systematic theory testing. However, we tentatively want to link our findings with regard to possible explanations that can be supported by relevant teacher judgment models. Since these considerations are tentative, we secondly discuss our findings in the light of further psychological research from other application fields using similar metrics in order to underline the usefulness of this study's findings for teacher research. First, from the perspective of research about teachers' judgment processes and in the light of dual-process theories (Fiske and Neuberg, 1990; Chaiken and Trope, 1999; Fiske et al., 1999), both experts and novices may have formed their judgment in the more complex and systematic person-specific mode (Fiske and Neuberg, 1990), but experts may have managed to perform more effectively during the piecemeal integration of all available information: Based on their experience and knowledge, experts were better in identifying valid cues that are related to the target characteristic (Herbig and Glöckner, 2009), and experts were better at collecting many cues in a shorter period (Elstein et al., 1978). Our experiment may tentatively indicate that novices could not collect and combine all critical cues of many students and that they were required to reduce their attention to fewer students. Experts might know more about the validity of the collected cues (whether the identified cue is related to the target characteristic or multiple characteristics) and weighted their cues according to their knowledge about the validity (Chaiken and Trope, 1999; Herbig and Glöckner, 2009). All this suggests that experts, compared to novices, may have then been able to process more information effectively and had more time to monitor all students, make comparisons between them, and re-adjust their judgment until they terminated the search for cues and found a satisfying solution. Hence, experts' more effective systematic information processing resulted in a more complex gaze distribution. Previous research results by Dessus et al. (2016) underpin these assumptions. They found that novice teachers engaged with a larger amount of cognitive load and that the size of a gazed group of students was related to experience level. Expert teachers were able to scan a larger group of students and have a more comprehensive observation scheme, which allows them to collect more fine grated information about students. Furthermore, our results are, to some extent, in line with a recent study by Karst and Bonefeld (2020). They used a simulated classroom setting and novices' click frequency (more clicks indicating that the participant gathered more information) as an indicator of attention allocation and found that novices rated an individual within a group of students more highly when they gave that particular student more attention (more clicks). However, overall judgment accuracy was higher when teachers allocated their attention across all students more equally. Both investigations together indicate that teachers seem to be more able to assess or rank multiple students adequately when they follow a strategy in which they gather relevant information from all students approximately equally.
Second, cognitive psychology and visual perception research can help understand the relationship between gaze entropy and assessment accuracy. For example, Shic et al. (2008), as well as Krishna et al. (2018), suggest that a higher gaze entropy indicates a preference for detailed exploration of the visual stimuli, whereas a lower entropy corresponds to less exploratory behavior. Krejtz et al. (2014) conclude that a more evenly distributed gaze across different AOIs may be related to the viewer's increased interest in collecting information from all available AOIs. Furthermore, in their comprehensive review about gaze entropy, Shiferaw et al. (2019) pointed out that gaze entropy is related to the scene complexity, the task demand, and top-down processing level (see details in the integrated model of gaze orientation, Shiferaw et al., 2019). Gaze entropy increases relative to complexity, task demand, and with more top-down processing. Given the fact that the teachers were asked to diagnose students they did not know before and that the assessment task required that teachers assess two student characteristics at the same time (high complexity), experienced teachers' higher entropy, in this case, indicate increased compensatory top-down processing to meet task demand (Shiferaw et al., 2019). Hence, because top-down processes are mainly driven by task-related plans and current goals derived from professional knowledge (Gegenfurtner et al., 2011; Goldberg et al., 2020), the more complex and evenly distributed gaze on all students might be the product of the above-discussed strategy, namely that experts were able to collect more fine graded information from all students to reach a judgment.
While our study demonstrated that greater complexity (high entropy) of teachers' scanpath patterns contributed to a more accurate judgment of students and their underlying student profiles, previous literature, for example, about aircraft pilots (Kasarskis et al., 2001) reported rather contrary findings, indicating that experts employ lower entropy values compared to novices or that lower entropy values are in some cases beneficial (gaze guiding in advertisments; Hooge and Camps, 2013). To understand why it may be advantageous to show scanpaths with less complexity in some areas, it is essential to look at the type of visual stimuli being evaluated by the participants. For example, Kasarskis et al. (2001) were able to show that experienced pilots had lower entropy values and exhibit a clearly defined pattern of visual scanning during a landing task. The experts left out task-redundant instruments and focused only on the runway and the airspeed indicator. In this type of study, the experts were able to reduce complexity, mostly by hiding task-redundant instruments or regions, resulting in lower entropy values. While pilots can actively hide task-redundant instruments in trained routines (the exact method of scanning the instrument panel varies between pilots, but some basic features common to a “good” scan pattern are available), the reduction of complexity is much more difficult for teachers in the present study's assessment situation. Aircraft instruments are static, standardized, and a priority ranking in their importance can be made (Brams et al., 2018). On the other hand, teachers in our study had to observe several students simultaneously who acted dynamically and were asked to judge personal traits that are not readily identifiable —blinding out individual students might be much more complicated than instruments that are not required for the correct assessment (i.e., landing a plane).
As this was only a very first study, much more research is needed to understand the relation between gaze complexity and assessment competence in more detail.
These results are essential to a better understanding of teachers' judgment processes, and highlight the advantage of considering gaze sequences instead of only focusing on averaged eye-tracking metrics such as fixation duration as previously reported. In this context, it is crucial to note that we have statistically controlled for averaged eye-tracking metrics in our regression model and found no significant relationship between averaged metrics and judgment accuracy. Furthermore, earlier analysis with the same dataset (see details; Seidel et al., 2020) indicated that expert and novice teachers only showed relatively small differences in the number of fixations and average fixation durations regarding the five different students. The results of the present study underline that the way in which teachers ordered their gaze was essential to drawing accurate conclusions about underlying learning-relevant student characteristics.
Limitations and Further Research
The following limitations of the present study should be addressed. We had no variation in the authentic classroom video sequence used; thus, it remains unclear whether identical findings can be replicated when other video sequences (and other students) are presented. Furthermore, we used an event-based (dwell-based) scanpath comparison method to identify differences and similarities between experts and novices. Therefore, we have not taken into account the time a teacher spent at each AOI, which should be addressed in future research. The differences in scanpath similarity could be even greater when fixation time is taken into account, as previous research has repeatedly shown that experts process information faster than novices (Gegenfurtner et al., 2011). In addition, our analysis could show that expert and novice teachers differed in their visual behavior, but we know little about how they differ in their interpretation of what they saw. Future research should focus on a more comprehensive combination of eye-tracking and think-aloud protocols or subjective reports to better understand what cues teachers have noticed and, more importantly, how they reason these cues in relation to the target of the assessment (e.g., student characteristic). It should also be noted that in the present study, teachers were asked to diagnose students they did not know before and that the assessment task required that teachers assess two student characteristics at the same time. These research design factors may distort the eye-tracking metrics because, for example, we do not know which characteristics of the students caused more difficulty and may therefore have paid more attention to finding valid cues for those characteristics. Furthermore, the results of our statistical analysis need to be interpreted with caution, mainly due to our relatively small sample size, unequal number of experts and novices, and the absence of control variables in regression analysis. Hence, future studies might investigate whether our findings are replicable with a larger sample size. It should also be noted that although the average professional experience of the expert group is more than 12 years, the range was large and between 1.5 and 25 years. Even if we could not identify descriptive differences between experts with less work experience and experts with more work experience in the eye-tracking and assessment outcomes, the intermediates might distort the results in an unknowable direction.
Conclusion
The present study contributes to the understanding of teachers' process of assessing learning-relevant student characteristics. Results show that experts use qualitatively and quantitatively different strategies than novices in their visual behavior during an assessment situation, taking the sequential order of their gaze into account. We found that experts showed a more complex visual behavior in which more information about various students was used to form a judgment of students' underlying learning-relevant characteristics. Using Shannon's entropy value, we were able to quantify the complexity of visual behavior and found that the more a teacher included all students equally in the assessment process, the more students are judged accurately.
Data Availability Statement
The raw data supporting the conclusions of this article will be made available by the authors, without undue reservation.
Ethics Statement
Ethical review and approval was not required for the study on human participants in accordance with the local legislation and institutional requirements. The patients/participants provided their written informed consent to participate in this study.
Author Contributions
CK organized the data collection and database, performed statistical analysis, and wrote the first draft of the manuscript. All authors contributed to the conception and design of the study, manuscript revision, and read and approved the submitted version.
Funding
The present research project was funded by the Deutsche Forschungsgemeinschaft (German Research Foundation, grant no. SE1397/7-3). The funders had no role in the study's design, data collection and analysis, decision to publish, or preparation of the manuscript.
Conflict of Interest
The authors declare that the research was conducted in the absence of any commercial or financial relationships that could be construed as a potential conflict of interest.
References
Abbott, A., and Tsay, A. (2016). Sequence analysis and optimal matching methods in sociology. Sociol. Methods Res. 29, 3–33. doi: 10.1177/0049124100029001001
Antes, J. R., and Kristjanson, A. F. (1991). Discriminating artists from nonartists by their eye-fixation patterns. Percept. Mot. Skills 73, 893–894. doi: 10.2466/pms.1991.73.3.893
Ausubel, D. P. (2000). The Acquisition and Retention of Knowledge: A Cognitive View. Dordrecht: Kluwer Academic Publishers. doi: 10.1007/978-94-015-9454-7
Balslev, T., Jarodzka, H., Holmqvist, K., de Grave, W., Muijtjens, A. M. M., Eika, B., et al. (2012). Visual expertise in paediatric neurology. Eur. J. Paediatr. Neurol. 16, 161–166. doi: 10.1016/j.ejpn.2011.07.004
Berliner, D. C. (2001). Learning about and learning from expert teachers. Int. J. Educ. Res. 35, 463–482. doi: 10.1016/S0883-0355(02)00004-6
Berliner, D. C., and Clarridge, P. B. C. (1991). Perceptions of student behavior as a function of expertise. J. Classroom Interact. 26, 1–8.
Blömeke, S., Gustafsson, J.-E., and Shavelson, R. J. (2015). Beyond dichotomies. Zeitschrift für Psychologie 223, 3–13. doi: 10.1027/2151-2604/a000194
Boshuizen, H. P. A., Schmidt, H. G., Custers, E. J. F. M., and van de Wiel, M. W. (1995). Knowledge development and restructuring in the domain of medicine: the role of theory and practice. Learn. Instruct. 5, 269–289. doi: 10.1016/0959-4752(95)00019-4
Brams, S., Hooge, I., Ziv, G., Dauwe, S., Evens, K., De Wolf, T., et al. (2018). Does effective gaze behavior lead to enhanced performance in a complex error-detection cockpit task? PLoS ONE 13:e0207439. doi: 10.1371/journal.pone.0207439
Brunswik, E. (1955). Representative design and probabilistic theory in a functional psychology. Psychol. Rev. 62, 193–217. doi: 10.1037/h0047470
Chaiken, S., and Trope, Y. (1999). Dual-Process Theories in Social Psychology. Guilford Press. Available online at: http://www.loc.gov/catdir/bios/guilford051/98054246.html (accessed November 30, 2020).
Charness, N., Reingold, E. M., Pomplun, M., and Stampe, D. M. (2001). The perceptual aspect of skilled performance in chess: evidence from eye movements. Mem. Cogn. 29, 1146–1152. doi: 10.3758/BF03206384
Corno, L. (2008). On teaching adaptively. Educ. Psychol. 43, 161–173. doi: 10.1080/00461520802178466
Davies, A., Vigo, M., Harper, S., and Jay, C. (2016). “The visualisation of eye-tracking scanpaths: What can they tell us about how clinicians view electrocardiograms?,” in 2016 IEEE Second Workshop on Eye Tracking and Visualization (ETVIS) (Baltimore, MD: IEEE), 79–83. doi: 10.1109/ETVIS.2016.7851172
Dessus, P., Cosnefroy, O., and Luengo, V. (2016). “Keep your eyes on 'em all!”: a mobile eye-tracking analysis of teachers' sensitivity to students,” in European Conference on Technology Enhanced Learning, Lecture Notes in Computer Science, 72–84. doi: 10.1007/978-3-319-45153-4_6
Dolezalova, J., and Popelka, S. (2016). ScanGraph: a novel scanpath comparison method using visualisation of graph cliques. J. Eye Mov. Res. 9, 1–13. doi: 10.16910/jemr.9.4.5
Doyle, W. (2006). “Ecological approaches to classroom management,” in Handbook of Classroom Management: Research, Practice, and Contemporary Issues, eds C. M., and C. S. Weinstein (New Jersey: Lawrence Erlbaum Associates Publishers), 97–125.
Elstein, A. S., Shulman, L. S., and Sprafka, S. A. (1978). Medical problem solving: an analysis of clinical reasoning. Newsl. Sci. Technol. Hum. Values 3, 50–51. doi: 10.4159/harvard.9780674189089
Ericsson, K. A., and Kintsch, W. (1995). Long-term working memory. Psychol. Rev. 102, 211–245. doi: 10.1037/0033-295X.102.2.211
Ferreira, M. B., Garcia-Marques, L., Sherman, S. J., and Sherman, J. W. (2006). Automatic and controlled components of judgment and decision making. J. Personal. Soc. Psychol. 91, 797–813. doi: 10.1037/0022-3514.91.5.797
Fiske, S. T., Kinder, D. R., and Larter, W. M. (1983). The novice and the expert: Knowledge-based strategies in political cognition. J. Exp. Soc. Psychol. 19, 381–400. doi: 10.1016/0022-1031(83)90029-X
Fiske, S. T., Lin, M., and Neuberg, S. L. (1999). “The continuum model: ten years later,” in Dual-Process Theories in Social Psychology, eds S. Chaiken, and Y. Trope (The Guilford Press), 231–254.
Fiske, S. T., and Neuberg, S. L. (1990). A continuum of impression formation, from category-based to individuating processes: influences of information and motivation on attention and interpretation. Adv. Exp. Soc. Psychol. 23, 1–74. doi: 10.1016/S0065-2601(08)60317-2
Foulsham, T., Dewhurst, R., Nyström, M., Johansson, R., and Underwood, G. (2012). Comparing scanpaths during scene encoding and recognition: a multi-dimensional approach. J. Eye Mov. Res. 5, 1–14. doi: 10.16910/jemr.5.4.3
Gegenfurtner, A., Lehtinen, E., and Säljö, R. (2011). Expertise differences in the comprehension of visualizations: a meta-analysis of eye-tracking research in professional domains. Educ. Psychol. Rev. 23, 523–552. doi: 10.1007/s10648-011-9174-7
Goldberg, P., Schwerter, J., Seidel, T., Müller, K., and Stürmer, K. (2020). How does learners' behavior attract preservice teachers' attention during teaching? Teach. Teacher Educ. 97:103213. doi: 10.1016/j.tate.2020.103213
Goodwin, C. (1994). Professional vision. Am. Anthropol. 96, 606–633. doi: 10.1525/aa.1994.96.3.02a00100
Haider, H., and Frensch, P. A. (1996). The role of information reduction in skill acquisition. Cogn. Psychol. 30, 304–337. doi: 10.1006/cogp.1996.0009
Heitzmann, N., Seidel, T., Opitz, A., Hetmanek, A., Wecker, C., Fischer, M. R., et al. (2019). Facilitating diagnostic competences in simulations in higher education: a framework and a research agenda. Front. Learn. Res. 7, 1–24. doi: 10.14786/flr.v7i4.384
Herbig, B., and Glöckner, A. (2009). “Experts and decision making: first steps towards a unifying theory of decision making in novices, intermediates and experts,” in Preprints of the Max Planck Institute for Research on Collective Goods (Bonn). doi: 10.2139/ssrn.1337449
Herppich, S., Praetorius, A.-K., Förster, N., Glogger-Frey, I., Karst, K., Leutner, D., et al. (2017). Teachers' assessment competence: Integrating knowledge-, process-, and product-oriented approaches into a competence-oriented conceptual model. Teach. Teacher Educ. 76, 181–193. doi: 10.1016/j.tate.2017.12.001
Holmqvist, K., Nystrom, M., Andersson, R., Dewhurst, R., Jarodzka, H., and van de Weijer, J. (2015). Eye Tracking: A Comprehensive Guide to Methods and Measures. Oxford: Oxford University Press.
Hooge, I., and Camps, G. (2013). Scan path entropy and arrow plots: capturing scanning behavior of multiple observers. Front. Psychol. 4:996. doi: 10.3389/fpsyg.2013.00996
Huang, C. (2011). Self-concept and academic achievement: a meta-analysis of longitudinal relations. J. Sch. Psychol. 49, 505–528. doi: 10.1016/j.jsp.2011.07.001
Kaiser, J., Retelsdorf, J., Südkamp, A., and Möller, J. (2013). Achievement and engagement: how student characteristics influence teacher judgments. Learn. Instruct. 28, 73–84. doi: 10.1016/j.learninstruc.2013.06.001
Kanan, C., Bseiso, D. N. F., Ray, N. A., Hsiao, J. H., and Cottrell, G. W. (2015). Humans have idiosyncratic and task-specific scanpaths for judging faces. Vision Res. 108, 67–76. doi: 10.1016/j.visres.2015.01.013
Karing, C. (2009). Diagnostische Kompetenz von Grundschul- und Gymnasiallehr- kräften im Leistungsbereich und im Bereich Interessen. Zeitschrift für Pädagogische Psychologie 23, 197–209. doi: 10.1024/1010-0652.23.34.197
Karst, K., and Bonefeld, M. (2020). Judgment accuracy of preservice teachers regarding student performance: the influence of attention allocation. Teach. Teacher Educ. 94:103099. doi: 10.1016/j.tate.2020.103099
Kasarskis, P., Stehwien, J., Hickox, J., Aretz, A., and Wickens, C. D. (2001). “Comparison of expert and novice scan behaviors during VFR flight,” in Proceedings of the 11th International Symposium on Aviation Psychology, (Vol. 6) (Columbus, OH).
Kelly, B. S., Rainford, L. A., Darcy, S. P., Kavanagh, E. C., and Toomey, R. J. (2016). The development of expertise in radiology: in chest radiograph interpretation, “expert” search pattern may predate “expert” levels of diagnostic accuracy for pneumothorax identification. Radiology 280, 252–260. doi: 10.1148/radiol.2016150409
Klieme, E., Pauli, C., and Reusser, K. (2009). The pythagoras study. Investigating effects of teaching and learning in Swiss and German mathematics classrooms,” in The Power of Video Studies in Investigating Teaching and Learning in the Classroom (Waxmann).
Krejtz, K., Szmidt, T., Duchowski, A. T., and Krejtz, I. (2014). Entropy-based statistical analysis of eye movement transitions,” in Proceedings of the Symposium on Eye Tracking Research and Applications - ETRA '14 (Safety Harbor, FL). doi: 10.1145/2578153.2578176
Krishna, O., Helo, A., Rämä, P., and Aizawa, K. (2018). Gaze distribution analysis and saliency prediction across age groups. PLoS ONE 13:e0193149. doi: 10.1371/journal.pone.0193149
Kundel, H. L., Nodine, C. F., and Carmody, D. (1978). Visual scanning, pattern recognition and decision-making in pulmonary nodule detection. Invest. Radiol. 13, 175–181. doi: 10.1097/00004424-197805000-00001
Kundel, H. L., Nodine, C. F., Conant, E. F., and Weinstein, S. P. (2007). Holistic component of image perception in mammogram interpretation: gaze-tracking study. Radiology 242, 396–402. doi: 10.1148/radiol.2422051997
Lachner, A., Jarodzka, H., and Nückles, M. (2016). What makes an expert teacher? Investigating teachers' professional vision and discourse abilities. Instruct. Sci. 44, 197–203. doi: 10.1007/s11251-016-9376-y
Landis, C. (1984). Teachers' Decision Factors in Judging and Planning Discussion Activities for Elementary Art Programs. Marilyn Zurmuehlen Working Papers in Art, Iowa. doi: 10.17077/2326-7070.1091
Lau, S., and Roeser, R. W. (2008). Cognitive abilities and motivational processes in science achievement and engagement: a person-centered analysis. Learn. Ind. Differ. 18, 497–504. doi: 10.1016/j.lindif.2007.11.002
Leuders, T., Philipp, K., and Leuders, J. (2018). Diagnostic Competence of Mathematics Teachers: Unpacking a Complex Construct in Teacher Education and Teacher Practice. Mathematics Teacher Education: Vol. 11. Cham: Springer International Publishing. doi: 10.1007/978-3-319-66327-2
Levenshtein, V. (1966). Binary codes capable of correcting deletions, insertions and reversals. Soviet Phys. Doklady 10:707.
Levin, J., and Nolan, J. F. (2014). Principles of Classroom Management: A Professional Decision-Making Model. London: Pearson.
Linnenbrink-Garcia, L., Pugh, K. J., Koskey, K. L. K., and Stewart, V. C. (2012). Developing conceptual understanding of natural selection: the role of interest, efficacy, and basic prior knowledge. J. Exp. Educ. 80, 45–68. doi: 10.1080/00220973.2011.559491
Loibl, K., Leuders, T., and Dörfler, T. (2020). A framework for explaining teachers' diagnostic judgements by cognitive modeling (DiaCoM). Teach. Teacher Educ. 91:103059. doi: 10.1016/j.tate.2020.103059
Machts, N., Kaiser, J., Schmidt, F. T. C., and Möller, J. (2016). Accuracy of teachers' judgments of students' cognitive abilities: a meta-analysis. Educ. Res. Rev. 19, 85–103. doi: 10.1016/j.edurev.2016.06.003
Mathôt, S., Cristino, F., and Gilchrist Theeuwes, J. (2012). A simple way to estimate similarity between pairs of eye movement sequences. Eye 5, 1–15. doi: 10.16910/jemr.5.1.4
McIntyre, N. A., and Foulsham, T. (2018). Scanpath analysis of expertise and culture in teacher gaze in real-world classrooms. Instruct. Sci. 46, 435–455. doi: 10.1007/s11251-017-9445-x
McIntyre, N. A., Mainhard, M. T., and Klassen, R. M. (2017). Are you looking to teach? Cultural, temporal and dynamic insights into expert teacher gaze. Learn. Instruct. 49, 41–53. doi: 10.1016/j.learninstruc.2016.12.005
Meschede, N., Fiebranz, A., Möller, K., and Steffensky, M. (2017). Teachers' professional vision, pedagogical content knowledge and beliefs: on its relation and differences between pre-service and in-service teachers. Teach. Teacher Educ. 66, 158–170. doi: 10.1016/j.tate.2017.04.010
Noton, D., and Stark, L. (1971b). Scanpaths in saccadic eye movements while viewing and recognizing patterns. Vision Res. 11, 929-IN8. doi: 10.1016/0042-6989(71)90213-6
O'Neill, E. C., Kong, Y. X. G., Connell, P. P., Ong, D. N., Haymes, S. A., Coote, M. A., et al. (2011). Gaze behavior among experts and trainees during optic disc examination: does how we look affect what we see? Invest. Ophthalmol. Vis. Sci. 52, 3976–3983. doi: 10.1167/iovs.10-6912
Praetorius, A.-K., Berner, V.-D., Zeinz, H., Scheunpflug, A., and Dresel, M. (2013). Judgment confidence and judgment accuracy of teachers in judging self-concepts of students. J. Educ. Res. 106, 64–76. doi: 10.1080/00220671.2012.667010
Schnitzler, K., Holzberger, D., and Seidel, T. (2020). connecting judgment process and accuracy of student teachers: differences in observation and student engagement cues to assess student characteristics. Front. Educ. 5, 1–28. doi: 10.3389/feduc.2020.602470
Seidel, T. (2006). The role of student characteristics in studying micro teaching–learning environments. Learn. Environ. Res. 9, 253–271. doi: 10.1007/s10984-006-9012-x
Seidel, T., Jurik, V., Häusler, J., and Stubben, S. (2016). “Micro-environments within classrooms: how cognitive and motivational-affective student characteristics affect students' classroom perception and behavior,” in Conditions and Effects of Good Teaching (Bedingungen und Effekte guten Unterrichts), eds N. McElvany, W. Bos, H. G. Holtapples, M. M. Gebauer, and F. Schwabe (Münster: Waxmann), 65–88.
Seidel, T., Schnitzler, K., Kosel, C., Stürmer, K., and Holzberger, D. (2020). Student characteristics in the eyes of teachers: differences between novice and expert teachers in judgment accuracy, observed behavioral cues, and gaze. Educ. Psychol. Rev. 15:98. doi: 10.1007/s10648-020-09532-2
Seidel, T., and Stürmer, K. (2014). Modeling and measuring the structure of professional vision in preservice teachers. Am. Educ. Res. J. 51, 739–771. doi: 10.3102/0002831214531321
SensoMotoric Instruments. (2017). Experiment Center (Version 3.7) [Computer software]. Available online at: http://www.humre.vu.lt/files/doc/Instrukcijos/SMI/ExperimentCenter.pdf (accessed November 30, 2020).
Shannon, C. E. (1948). A mathematical theory of communication. Bell Syst. Tech. J. 27, 379–423. doi: 10.1002/j.1538-7305.1948.tb01338.x
Shic, F., Chawarska, K., Bradshaw, J., and Scassellati, B. (2008). “Autism, eye-tracking, entropy,” in Development and learning, 2008. ICDL 2008,” in 7th IEEE International Conference on IEEE (New Haven, CT), 73–78 doi: 10.1109/DEVLRN.2008.4640808
Shiferaw, B., Downey, L., and Crewther, D. (2019). A review of gaze entropy as a measure of visual scanning efficiency. Neurosci. Biobehav. Rev. 96, 353–366. doi: 10.1016/j.neubiorev.2018.12.007
Stark, L. W., and Choi, Y. S. (1996). Experimental metaphysics: the scanpath as an epistemological mechanism. Adv. Psychol. 116, 33–69. doi: 10.1016/S0166-4115(96)80069-0
Stürmer, K., Könings, K. D., and Seidel, T. (2013b). Declarative knowledge and professional vision in teacher education: effect of courses in teaching and learning. Br. J. Educ. Psychol. 83, 467–483. doi: 10.1111/j.2044-8279.2012.02075.x
Stürmer, K., Seidel, T., Müller, K., Häusler, J., and Cortina, K. S. (2017). What is in the eye of preservice teachers while instructing? An eye-tracking study about attention processes in different teaching situations. Zeitschrift für Erziehungswissenschaft 20, 75–92. doi: 10.1007/s11618-017-0731-9
Stürmer, K., Seidel, T., and Schäfer, S. (2013a). Changes in professional vision in the context of practice. Gruppendynamik und Organisationsberatung 44, 339–355. doi: 10.1007/s11612-013-0216-0
Südkamp, A., Kaiser, J., and Möller, J. (2012). Accuracy of teachers' judgments of students' academic achievement: a meta-analysis. J. Educ. Psychol. 104, 743–762. doi: 10.1037/a0027627
Tang, H., and Pienta, N. J. (2017). GrpString: Patterns and Statistical Differences Between Two Groups of Strings. [Computer software]. Available online at: https://CRAN.R-project.org/package=GrpString (accessed November 30, 2020).
Tiersma, E. S. M., Peters, A. A. W., Mooij, H. A., and Fleuren, G. J. (2003). Visualising scanning patterns of pathologists in the grading of cervical intraepithelial neoplasia. J. Clin. Pathol. 56, 677–680. doi: 10.1136/jcp.56.9.677
van den Bogert, N., van Bruggen, J., Kostons, D., and Jochems, W. (2014). First steps into understanding teachers' visual perception of classroom events. Teach. Teacher Educ. 37, 208–216. doi: 10.1016/j.tate.2013.09.001
van der Loo, M. P. J. (2014). The stringdist package for approximate string matching. R J. 6, 111–122. doi: 10.32614/RJ-2014-011
van Es, E. A., Cashen, M., Barnhart, T., and Auger, A. (2017). Learning to notice mathematics instruction: using video to develop preservice teachers' vision of ambitious pedagogy. Cogn. Instruct. 35, 165–187. doi: 10.1080/07370008.2017.1317125
van Es, E. A., and Sherin, M. G. (2010). The influence of video clubs on teachers' thinking and practice. J. Math. Teacher Educ. 13, 155–176. doi: 10.1007/s10857-009-9130-3
Wolff, C. E., Jarodzka, H., van den Bogert, N., and Boshuizen, H. P. A. (2016). Teacher vision: expert and novice teachers' perception of problematic classroom management scenes. Instruct. Sci. 44, 243–265. doi: 10.1007/s11251-016-9367-z
Keywords: eye tracking, scanpath analysis, student profiles, professional vision, teacher gaze, assessment competence
Citation: Kosel C, Holzberger D and Seidel T (2021) Identifying Expert and Novice Visual Scanpath Patterns and Their Relationship to Assessing Learning-Relevant Student Characteristics. Front. Educ. 5:612175. doi: 10.3389/feduc.2020.612175
Received: 02 October 2020; Accepted: 09 December 2020;
Published: 26 January 2021.
Edited by:
Robbert Smit, University of Teacher Education St. Gallen, SwitzerlandReviewed by:
Karina Karst, Universität Mannheim, GermanyNora McIntyre, University of Cambridge, United Kingdom
Els Boshuizen, Open University of the Netherlands, Netherlands
Copyright © 2021 Kosel, Holzberger and Seidel. This is an open-access article distributed under the terms of the Creative Commons Attribution License (CC BY). The use, distribution or reproduction in other forums is permitted, provided the original author(s) and the copyright owner(s) are credited and that the original publication in this journal is cited, in accordance with accepted academic practice. No use, distribution or reproduction is permitted which does not comply with these terms.
*Correspondence: Christian Kosel, christiankosel@tum.de