- Department of Psychology, UiT The Arctic University of Norway, Tromsø, Norway
Academic attrition is a worldwide problem representing a significant economic loss and a disadvantage for students in terms of health and career prospects. We focus on the roles of academic skills, academic self-efficacy, and students' integration in exploring their relation to attrition intentions. Based on existing research, we expected a negative relation between academic skills and attrition intentions, with academic self-efficacy and students' integration as possible mediators. Furthermore, it was expected that this relationship would be dependent on the outcome variable being measured (i.e., drop-out, transfer university, and transfer study field intentions). These hypotheses were investigated among Norwegian university students in a questionnaire study (total N = 756). Results supported, as predicted, the mediatory roles of academic self-efficacy and students' integration. Importantly, significant variability was indicated in comparison of the different outcome measures, with academic self-efficacy having a larger mediation effect in case of drop-out and transfer study field intentions. We conclude that academic self-efficacy is important in understanding the relationship between students' academic skills and attrition intentions. Our results provide an evidence that might facilitate development of assistance programs aiming to reduce academic attrition.
Introduction
Obtaining a higher education qualification has become more common in the transition from school to work leading to better economic success and well-being (Dalgard et al., 2007; OECD, 2019). However, according to the estimates by the EU research team on academic attrition rates across Europe, 19 to 40% of students withdraw from higher education (Vossensteyn et al., 2015). Although significant improvements have been achieved in the Norwegian higher education during the past years, the state of affairs on academic attrition is similar to other western societies (Ministry of Education and Research, 2018). According to recent estimates, 19% of bachelor students do not complete their academic degrees (Statistics Norway, 2019a). Students leaving before graduation represent an inefficient use of government funding (Statistics Norway, 2019b), and a considerable loss for students themselves in terms of health and career prospects (Muennig, 2007; Steingrímsdóttir et al., 2012).
Why do students leave at all? Departure before degree completion (i.e., attrition) has been extensively examined from different theoretical perspectives (for an overview, see Hossler and Bontrager, 2014; Burger, 2017). Common to these perspectives is that they focus on actual behavior. Despite extensive evidence on the role of intentions in predicting behavior, few studies have focused on attrition intentions as the primary outcome of interest (Sheeran, 2002). As behavioral intentions to leave is an excellent indicator of actual attrition (e.g., Bean, 1982; Mashburn, 2000), focus on attrition intentions may add valuable insights to the attrition problem, allowing for preventive measures before actual attrition. For example, the knowledge on antecedents for attrition intentions may aid in the development and utility assessment of prospective intervention programs such as academic skills training courses and more rigorous study plans.
Further, treating students who leave their university studies as a homogenous group is common among researchers. This tendency is problematic from both theoretical and practical perspectives (Grosset, 1993; Porter, 2000; Hoyt and Winn, 2004). First, from a theoretical perspective, one potential consequence is inaccuracy in prediction and explanation of students' attrition behavior. Second, from the practical perspective, treating these students as a uniform population might lead to the opposite outcomes than those being expected. For example, while a prospective assistance program might be effective in reducing system attrition, it might have no effect on students who change their academic institution. Although intentions are approximate indicators of actual attrition behavior (Bean, 1982), differences among students' intentions have not been previously addressed. As will be discussed, students leaving university permanently and students changing their place of education might have different reasons for leaving.
In the present study, we aim to investigate and facilitate the understanding of the factors that explain attrition intentions among students. These issues will be examined from the perspective of academic skills, academic self-efficacy, and students' integration. Although these factors are related to attrition behavior, they have not been examined in relation to the different categories of attrition intentions (e.g., intentions to change academic institution, intention to leave permanently).
Socio-Cognitive Factors
Academic and Social Integration
Research on academic attrition has addressed the issue from a variety of perspectives, e.g., psychological, sociological, organizational, cultural, and economic. In the current section, we provide a short summary of theories attempting to explain academic attrition (for an overview, see Hossler and Bontrager, 2014; Aljohani, 2016; Burger, 2017). Two theoretical models have been particularly important in guiding thinking and research on academic attrition for the last 40 years, the Institutional Departure Model by Tinto (1975) and the Students Attrition Model by Bean (1982).
The most prominent theory, the Institutional Departure Model, assumes a crucial role of students' integration for attrition-retention behavior. Tinto (1975) theoretical model expanded the debate on the causes of academic attrition by calling attention to institutional factors that affect attrition. According to the theory, the primary determinants of academic attrition can be broken down into student-related background factors (e.g., previous academic experiences, skills, and abilities) and factors related to university experience (e.g., academic and social integration). Academic integration refers to students' academic performance and intellectual development, whereas social integration can be defined as interaction with peers and faculty, sense of belonging to peers, and extracurricular activities (Tinto, 1975). The background factors influence students' initial goals and commitments that either facilitate or hinder their integration. In turn, academic and social integration transforms students' initial goals and commitments that leads to subsequent attrition or persistence. Tinto (1975, 1993) argues that both academic and social integration are important due to their reciprocal relationship (i.e., too much integration in the social domain may lead to lower integration in the academic domain). In other words, attrition is a function of interaction (or the fit) between students and their academic environments.
The crucial role of the interaction between student-related and institutional factors in explaining attrition behavior is also highlighted by Bean (1982, 1990) in the Students Attrition Model. This model addresses the issue of academic attrition from the perspective of organizational turnover. Further, the structure and content of the models are similar (e.g., the concepts of academic and social integration, commitment) to the previously described Tinto's theory (1975; 1993). However, the models differ in several aspects. First, it is assumed that factors external to academic environment (e.g., finances, employment, family responsibilities) should be considered in explaining students' attrition. Moreover, Bean and Metzner (1985) argue that although social integration is crucial for traditional students' persistence, it might be less important in case of non-traditional students. Second, the two models differ in factors that are assumed to determine students' attrition. Tinto (1975) attributes this role to students' commitment, while Bean (1982) argues that students' intentions is the most approximate determinant of actual attrition.
A third theoretical model proposed by Cabrera et al. (1993), the Student Retention Integrated Model, integrates the aspects of the two models discussed. Similar to Bean (1982, 1990) Students Attrition Model, environmental factors are argued to have a crucial role in explaining academic attrition. However, in comparison to Institutional Departure Model, the role of environmental factors is not constrained to shaping students' commitments, but it is also assumed to influence students' social and academic experiences (i.e., integration).
In sum, the models have many similarities. First, an interaction between student and institutional factors is common. The models also agree on the significance of the match between students and an institution (i.e., academic and social integration) in explaining academic attrition. Among the differences between the models are their views on the closest antecedent of academic attrition. According to Bean (1982, 1990) and Cabrera et al. (1993), the closest antecedent is the intention to leave. In contrast, Tinto (1975, 1993) attributes this role to students' goal and institutional commitment. Further, both Tinto (1975, 1993) and Bean (1990) admit the importance of students' skills and abilities in the process of academic attrition (i.e., background factors, academic variables), whereas Cabrera et al. (1993) do not include these factors into their integrated model.
Behavioral Intentions
Bean (1982) and Cabrera et al. (1993) argued that students' intentions are significant antecedents of actual behavior. Intentions are mental states of self-instruction to perform a behavior or to obtain a certain outcome (Webb and Sheeran, 2006). Intentions have been used to predict a wide range of behaviors, including consumer decisions, weight loss, smoking, gambling, and driver behavior. Based on a meta-analysis of meta-analyses by Sheeran (2002), intentions explain 28% of the variance (i.e., large effect size) in these behaviors. According to Bean (1982), intentions to leave university have the most substantial direct effect and explain the largest proportion of variation in actual attrition behavior. These findings are in line with different theoretical frameworks designed to explain and predict human behavior such as the Theory of Planned Behavior and the Mindset Theory of Action Phases (for an overview, see Fishbein and Ajzen, 1975; Webb and Sheeran, 2006; Gollwitzer, 2012).
Academic Skills
Even if intentions can predict students' attrition behavior, they do not contain information besides the fact that a person aims to perform a particular behavior. Identifying the factors that, in turn, determine behavioral intentions is of a great theoretical and practical value, e.g., understanding working mechanisms, assistance, and assessment. Here, academic skills provide a crucial stepping-stone to the solution. Academic skills have been consistently shown to promote students' performance, attrition intentions, and actual attrition behavior (Bean and Metzner, 1985; Rovai, 2003; Robbins et al., 2004; Credé and Kuncel, 2008; Cathey et al., 2016; Hattie and Donoghue, 2016; Bernardo et al., 2019).
Academic skills can be defined as a student's ability to manage time, use different study strategies, and manage their resources to reach their goals and complete academic tasks (Tressel et al., 2019, p.122). However, students receive little instruction on how they should acquire and properly use these skills, and such instruction is usually not included in study curricula (Dunlosky et al., 2013). In the present paper, we focus on a specific and important category of academic skills, time-management skills which many students struggle to acquire. For example, in a study by Sauvé et al. (2018), half of the participants reported problems with time management. Time-management skills can be generally defined as students' knowledge and ability to effectively manage study time to achieve an academic outcome. Also, these skills are generally assumed to predict students' learning, academic performance, and attrition (Credé and Kuncel, 2008; George et al., 2008, Goldfinch and Hughes, 2007; Kitsantas et al., 2008; Dunlosky et al., 2013; Xuereb, 2014).
Time management is a part of the broader concept of self-regulated learning (SRL), seen as an integral and inseparable part of higher education (Zimmerman, 1998, 2002; Foerst et al., 2017). SRL is defined as students' active engagement in self-generated thoughts, feelings, and actions that are oriented toward the attainment of academic goals. At the university level, external support is typically limited to only specific deadlines (e.g., dates for assignments and exams). Thus, independent regulation of one's own education is important. Self-regulated learners are usually academically successful, achieve higher grades, and procrastinate less (Zimmerman, 2002; Steel, 2007). In turn, SRL process can be divided into four interdependent phases: planning, monitoring, control, reflection (Zimmerman, 1998; Pintrich, 2000). Students' ability to manage their time is a crucial component of this process.
In sum, students' time-management skills are important for academic success and retention. Since planning academic activity is an initial step of a study process, good time-management skills are crucial for the overall study process. Even if students possess good academic skills and apply them correctly (e.g., relating ideas in preparing for essay form of an exam), they might ineffectively devote their time to different competing goals. This might lead to poor performance and negative experience reducing the sense of student's self-efficacy which is crucial for subsequent effort, persistence, and self-regulation of behavior (Bandura, 1997; Heikkilä and Lonka, 2006). Further, the relationship of time management with other important aspects of academic-related skills and competences (e.g., rehearsal, elaboration, metacognitive skills) is medium-to-large (Pintrich et al., 1993; Weinstein et al., 2016). Therefore, time-management skills were chosen as the main independent variable in the current study.
Academic Self-Efficacy
Even if students possess knowledge about “healthy” time-management skills, they may not practice them and approach academic tasks in unproductive ways. Some indirect evidence (Schunk, 1985; Pintrich and De Groot, 1990; Foerst et al., 2017) and research findings1 show that academic skills are related to self-efficacy being a potential determinant of their implementation and practice. For example, Foerst et al. (2017) indicated that doubt about the ability to implement SRL strategies and lack of time were among the most popular self-reported reasons for not using them.
Like time-management skills, academic self-efficacy is an important part of academic attrition puzzle. The concept of self-efficacy refers to the conviction or belief that one can successfully perform a behavior required to achieve the desired outcome (Bandura, 1977). Extensive research evidence indicates a crucial role of self-efficacy in human agency including choice of behavior, effort, persistence, engagement, and emotional reactions (Bandura, 1997). Also, empirical evidence supports the importance of the construct in the domain of education and students' learning (Robbins et al., 2004).
According to the Social Cognitive Theory, self-efficacy is constructed from mastery experience (previous experience success), vicarious experience (observation), social persuasion, and physiological/affective states. According to Bandura (1997), mastery experience is the most influential source of self-efficacy. Changes in self-efficacy are more likely to arise following self-observations of improved performance and experienced development of skills or abilities to meet future challenges (Bandura, 1977). The notion is supported by the research findings indicating changes in self-efficacy as the result of skill-based interventions (e.g., Smith, 1989; Wernersbach et al., 2014).
How are self-efficacy beliefs related to students' attrition intentions? Here, the Theory of Planned Behavior (TPB) can provide a theoretical explanatory framework (Ajzen, 1991). Based on this theory, self-efficacy is a crucial dimension of behavioral control which is a central aspect in the formation of behavioral intentions and actual behavior (Ajzen, 1991, 2002). Further, according to Bean and Eaton (2001) psychological model of academic attrition, self-efficacy is an important precondition of students' intentions to persist and actual persistence. The assumption is in line with several findings indicating a negative relationship between academic self-efficacy and attrition intentions (Willcoxson, 2010; Willcoxson et al., 2011).
As discussed, academic skills and self-efficacy are important determinants of academic success and attrition intentions. However, even if students possess the skills required in post-secondary education (e.g., time management, critical thinking, selecting main ideas), they might not use them due to low self-efficacy beliefs (Schunk, 1985; Bandura, 1986, 1997; Pintrich and De Groot, 1990; Foerst et al., 2017). This relationship between academic skills and attrition intentions mediated by academic self-efficacy will be investigated in the current paper.
Variability of Attrition
According to Hoyt and Winn (2004), students not following a prescribed educational path can be differentiated into drop-outs, stop-outs, opt-outs, and transfer-outs. In the present study, we focus on the drop-out and transfer-out student categories. Dropping out can be defined as leaving an academic institution before degree completion, having no concrete intentions of returning to higher education. Transferring out is commonly referenced when an act of moving from a university (where students commenced their studies) to another higher education institution has taken place (Hovdhaugen, 2009). Students changing their initial study field could be also included in the category of transfer-outs (i.e., transfer study field).
In support, multiple researchers agree that treating non-returning students as a single cohort is inappropriate (Grosset, 1993; Porter, 2000; Hoyt and Winn, 2004; Hovdhaugen, 2011). Indeed, based on the dichotomy of system and institutional attrition, different sets of factors are found significant in explaining drop-out and transfer-out behaviors (Hovdhaugen, 2009). For example, Hovdhaugen (2009) indicated that background characteristics such as gender, age, and school grades were particularly more important in explaining students' drop-out than transfer-out behaviors. In contrast, students' motivation, educational goals, and field of the study were stronger related to a subsequent transfer to another university.
However, relatively few studies have compared the relationships between investigated variables and types of attrition intentions. Research has concentrated primarily on either intention to withdraw entirely or intention to change university (e.g., Raciti, 2012; Farr-Wharton et al., 2018). Consequently, previous findings may not be equally applicable to different categories of attrition intentions (e.g., dropping out, transferring out). For example, Willcoxson (2010) investigated factors that are related to students' intentions to persist vs. drop-out. The author excluded those students who reported an intention to change their academic institution. Hence, the results indicating the relationship between academic self-efficacy beliefs might be not applicable to those students who indicated their interion to transfer-out. Thus, we aim to address this issue through an assessment of students drop-out, transfer university, and transfer study field intentions.
The Current Study
In the present paper, we assess the relationship of academic skills (i.e., time-management skills) with attrition intentions, given self-efficacy, academic and social integration as possible mediating factors (see Figures 1 and 2). There are two possible mechanisms through which time-management skills could be related to drop-out and transfer-out intentions. First, based on Tinto's (1975, 1993) and Bean's (1990) models, the interaction between students-related factors (e.g., academic skills) and university's environment is crucial for their subsequent attrition or retention. Second, academic self-efficacy beliefs are closely related to students' academic skills and attrition (Bandura, 1997; Robbins et al., 2004; Foerst et al., 2017). Thus, we expect that academic integration, social integration, and academic self-efficacy would mediate the relationship of time-management skills with drop-out and transfer-out intentions.
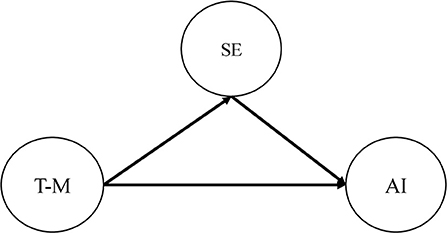
Figure 1. Conceptual model. T-M, Time-management skills; SE, Academic self-efficacy; AI, Attrition Intentions (Drop-out, Transfer University, Transfer Study Field).
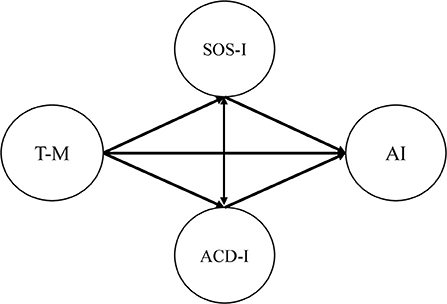
Figure 2. Conceptual model. T-M, Time-management skills; SOS-I, Social integration; ACD-I, Academic integration; AI, Attrition Intentions (Drop-out, Transfer University, Transfer Study Field).
As discussed, intentions are closely related to actual behavior and evidence shows variability of factors related to drop-out and transfer-out behaviors (e.g., Hovdhaugen, 2009). Also, according to Tinto (1993) and Quinn-Nilas et al. (2019) transfer-out students might perform as good as those students who persist at university, whereas this is not the case for dropouts. Since academic skills facilitate students' performance and are related to attrition (e.g., Robbins et al., 2004; Credé and Kuncel, 2008), differences in the relationship of time-management skills with attrition intentions might be expected.
However, we did not have any specific expectations about the direction and significance of this relationship due to the limited research evidence on the issue. Based on the findings by Hovdhaugen (2009) on the role of students engagement (i.e., effort) and the role of time management in self-regulation (i.e., engagement into learning), it might be expected that students time-management skills would be negatively related to both drop-out and transfer-out behaviors (Zimmerman, 1998, 2002; Pintrich, 2004). Similar conclusion can be made about the relationship of self-efficacy beliefs which are positively related to students' effort (Bandura, 1986, 1997). Nevertheless, to the best of our knowledge, the direct relationship between academic skills and self-efficacy with different categories of attrition intention has not been investigated.
Also, the relationship of integration factors with different categories of attrition intentions is less clear. Research shows that social and academic integration can be positively related to transfer-out behaviors (Nora and Rendon, 1990; Tinto, 1993). However, according to Wood et al. (2012) the evidence is inconclusive, with several studies indicating weak or no relationship of students' integration with transfer-out behaviors. Further, these and other studies have been concerned with students transferring from 2- to 4-year institutions (i.e., vertical transfer). Nevertheless, the evidence on students' integration and transferring from 4- to 4-year institutions (i.e., horizontal transfer), which is more relevant for Norwegian education system, is lacking.
In sum, the current study will test the following assumptions. First (Hypothesis 1), the relationship between time-management skills and attrition intentions is mediated by their self-efficacy beliefs. Second (Hypothesis 2), the relation of time-management skills with attrition intentions is mediated by the level of students' integration. However, as discussed, the relationship between the variables of interest could be dependent on the measured outcome (e.g., drop-out, transfer-out intention). Hence (Hypothesis 3), we aim to conduct an exploratory analysis if the mediated effects of time-management skills would differ depending on the outcome measure–drop-out, transfer university, or transfer study field intentions (Tinto, 1993; Hovdhaugen, 2009).
Methods
Sample and Setting
Participants were 756 students (72% females) in different stages of their studies at the university: first year (25%), second-year (24%), third-year (17%), fourth-year (13%), fifth-year (11%), and sixth-year or more (10%). Age ranged from 18 to 54 with a mean of 24.3 years (SD = 4.83). The relatively large proportion of females reflects the fact that the student population is female-dominated (i.e., 61%) in Norway (Statistics Norway, 2020). Also, some study programs (i.e., psychology) have even larger proportion of females (i.e., 70% and higher).
Assessment and Measures
Students participating in this study were recruited through Facebook and via e-mail sent to the active students registered at Norwegian universities. Participants from UiT The Arctic University of Norway and UiO University of Oslo were recruited via e-mail sent to active students. Data collection was done with the online survey tool Qualtrics2, which participants could access using either a mobile device or a computer.
Ethics
Participants were presented with a consent form, informed that they were anonymous and could refrain from answering or withdraw from the study at any time. The study was approved by the Norwegian Center for Research Data (NSD) in accordance with the requirements of data protection legislation (reference code 651244). Further, the current study was preregistered on Open Science Framework (OSF)3 where the supplementary materials and preregistration protocol could be retrieved. It is worth mentioning that Hypothesis 3 was not explicitly specified in our initial preregistration. However, based on the previous discussion, differences might be expected. Since we did not have any specific expectations about the direction and significance of direct and indirect effects, Hypothesis 3 is defined as exploratory (see The Current Study section).
Background Factors
Students were asked to report their age, gender, high-school GPA, university GPA, study field, university affiliation, parents' education, and if they have previously changed a study field or university. Age was an open-ended question and recorded as a continuous variable. High-school and university's GPA were categorical variables (1 = Lowest grade; 6 = Highest grade). Study field was an open-ended question, but subsequently re-coded into five different categories: psychology, STEM field, medicine and health science, biology and fishery field, humanitarian and social field. Parents education was categorical and included four categories: lower-secondary education, upper-secondary education, higher education, and other. Responses of students who chose “other,” were recorded as missing resulting into three main categories of parents' education. Parents education was not distinguished into mother's and father's level of education based on data-privacy considerations. University affiliation initially consisted of seven categories which were reduced to three because of small number of students from other Norwegian universities (recorded as “other”): University of Tromsø (UiT), University of Oslo (UiO), and other. Number of years studied at university was a categorical variable (1 = 1 year; 6 = 6 years or more). We also included single question about students' initial intention to receive an academic degree (0 = No; 1 = Yes), question about previous history of changing study field (0 = No; 1 = Yes), and question about previous history of changing academic institution (0 = No; 1 = Yes). Parents' education (with university's education as the reference group), university affiliation (with students from UiT as the reference group), and study field (with psychology as the reference group) were dummy coded for subsequent mediation analyses. The descriptive statistics can be found in the OSF depository.
Time-Management Skills
The time-management skills subscale (four items) from Approaches and Study Skills Inventory for Students (ASSIST) inventory was chosen based on its internationally validated stable factor structure and being tested with Norwegian samples (e.g., Entwistle et al., 2000; Diseth, 2001; Bonsaksen, 2018). An example item is: “I organize my study time carefully to make best use of it.” Response options ranged from 1 = Totally agree to 5 = Totally disagree with lower scores interpreted as showing worse time-management skills. In the study by Diseth (2001), internal reliability of the subscale was 0.72. In the current sample Cronbach's alpha was 0.78.
Academic Self-Efficacy
The measurement index was borrowed from a Danish study by Herrmann et al. (2017). The scale is based on MSLQ (Motivated Strategies for Learning Questionnaire) by Pintrich (1991). Self-efficacy, as it is used here, refers to the students' self-appraisal of their ability to master a task and includes judgment about their ability to accomplish a task as well as their confidence in their ability to perform that task (Pintrich, 1991). Three items (1 = Totally agree; 5 = Totally disagree) were chosen based on the reported highest loadings (i.e., Herrmann et al., 2017) with lower scores interpreted as showing lower academic self-efficacy beliefs. An example item is: “I am confident that I can acquire the skills necessary to excel within my field of study.” Original Cronbach's alpha (five items) was 0.83. Internal reliability for the current sample was 0.78.
Academic and Social Integration
The academic and intellectual development subscale from the Institutional Integration Scale was chosen as a measure of academic integration (Pascarella and Terenzini, 1980). Response alternatives were given on five-point Likert scale ranging from 1 = Not true of me to 5 = Totally true of me. An example item is: “I am satisfied with the extent of my intellectual development since enrolling in this university.” Original Cronbach's alpha (seven items) was 0.74. Internal reliability of three items for the current sample was 0.84. Three items from the Peer-group interaction subscale were borrowed from the same measurement index (Pascarella and Terenzini, 1980). An example item is: “Since coming to this university I have developed close personal relationships with other students.” Original Cronbach's alpha (seven items) was 0.84. Internal reliability of the three items was 0.84. These two subscales have been chosen based on personal communication with V. Tinto (August 16, 2019). He pointed out the significance of making distinction between academic and social integration clear for students. Hence, other dimensions of Pascarella and Terenzini (1980) academic and social integration (e.g., interaction with faculty members) were not included.
Drop-Out Intentions
Two items were taken from the study by Hardre and Reeve (2003): “I sometimes consider dropping out of university before graduation,” “I intend to drop out of school before graduation.” Original Cronbach's alpha (Three Items) was 0.79. Based on the Mindset Theory of Action Phases (Gollwitzer, 2012), two additional items were designed for these study, intending to measure the degree of intention's formation (“I sometimes think that other job opportunities suit me better than those I can get with my current education”; “I know what I am going to do if I withdraw from my studies”). The second item was subsequently excluded based on the low factor loading of 0.40. Cronbach's alpha was 0.67, which is lower than advised 0.70. However, internal consistency is considered sufficient given the number of items (Cortina, 1993; Streiner et al., 2015).
Transfer University Intentions
Two items were taken from the same study by Hardre and Reeve (2003) but rephrased with a focus on transfer university intentions: “I sometimes consider changing university before graduation,” “I intend to change university before graduation.” Similar to the drop-out intentions' measure, two items were devised based on the Mindset Theory of Action Phases (see Supplementary Material). Internal reliability of four items for intentions to change university (Cronbach's alpha) was 0.82.
Transfer Study Field Intentions
Two items were taken from the study by Hardre and Reeve (2003) but rephrased with a focus on transfer study field intentions: “I sometimes consider changing study field before graduation,” “I intend to change study field before graduation.” Similar to the previous measures of attrition intentions, two items were devised based on the Mindset Theory of Action Phases (see Supplementary material). Internal reliability of four items for intentions to change study field (Cronbach's alpha) of the scale was 0.82.
Analysis
Model Specification and Estimation
A structural equation model (SEM) analysis was employed since it allows estimation of cross-equation error correlation (see Bollen, 1989). Allowing such correlations is important, because academic and social integration are generally assumed to be related constructs (Tinto, 1993). The models specified are similar in terms of independent (i.e., time-management skills) and dependent (i.e., drop-out, transfer-out intentions) variables. The models differ in mediators being specified. The first set of models have academic self-efficacy as the mediator, while for the second set academic and social integration variables are specified as the mediators (see Figures 1 and 2). It is worth mentioning that readers should not interpret our data analysis approach as an indicator of causality. As discussed, the causality in the present study is theory-driven but cannot be directly supported by the study design.
Further, the weighted least squares parameter (WLSMV) estimation was implemented, which is appropriate when manifest variables are categorical or ordinal, and the sample size is relatively large (Muthén and Muthén, 1998-2017). Bootstrapping (based on 10,000 draws), which is a preferable method for testing significance of indirect effects (MacKinnon et al., 2004), was also implemented. Model fit data were examined using the chi-square test (χ2), Comparative Fit Index (CFI), Tucker-Lewis Fit Index (TLI), Root Mean Square Error of Approximation (RMSEA), and Standardized Root Mean Square Residual (SRMR). These model fit indices have been selected based on their satisfactory performance in Hu and Bentler (1999) simulation studies. For a more detailed description and discussion of the fit indices the reader is referred to Hu and Bentler (1999); Brown (2015).
Standard fit cut-off values were applied: CFI, TLI values >0.95, SRMR <0.08, and RMSEA <0.06 (Hu and Bentler, 1999). Values equal to or lesser/higher than cut-off values indicate good and close fit. Confirmatory factor analysis (CFA) was performed to assess the validity of the measurement model (i.e., time-management skills, academic self-efficacy, academic and social integration). The results of CFA indicated a good fit for time-management skills scale while academic self-efficacy, academic and social integration were just-identified (see OSF) 3 Hence, model-fit indices are not applicable in case of academic self-efficacy and integration constructs. Nevertheless, factor loadings were high (all above 0.60) and in expected direction. The aim of the current study was to test the described mediatory models and not to confirm factorial structure of the constructs. Thus, the results were deemed acceptable and we proceeded with the test of hypothesized mediatory models (see The Current Study section). Furthermore, the results of observed indirect effects were interpreted in concordance with Zhao et al. (2010), stating that a significant total effect is not a requirement for an indirect effect to be established. Items (i.e., questions) were used as indicators of the factors described in the method section. Analyses were performed with Mplus version 8.
Control Variables
The analyses were performed accounting for the effects of other variables that were previously found to influence students' attrition behaviors (e.g., gender, age, parents' education). Participants' age, time spent at university in years, initial goal of obtaining an academic degree, previous history of changing university or study field, parent's education, grade-average from upper-secondary school, and university affiliation were significant and were included in the final model. Only results for main effects are reported (i.e., control variables are included in the models but not presented). For detailed information on the effects of control variables see OSF4.
Results
Time-Management Skills and Attrition Intentions Via Academic Self-Efficacy
Drop-Out Intentions
The overall model fit for drop-out intentions was very good. The chi-square test was significant (χ2 = 126.032, df = 50, p < 0.001), CFI = 0.987; TLI=0.983; RMSEA = 0.045 (90% CI 0.035–0.055); SRMR = 0.038. As seen in Table 1, time-management skills is positively related to academic self-efficacy (β = 0.458, boot SE = 0.041, p < 0.001), which in turn was negatively related to drop-out intentions (β = −0.434, boot SE = 0.053, p < 0.001). The direct effect from time-management skills to drop-out intentions was insignificant (β = −0.074, boot SE = 0.055, p = 0.177). In other words, the better time-management skills that was reported, the higher was their academic self-efficacy, which was related to lower drop-out intentions. The indirect effect of time-management skills on drop-out intentions through self-efficacy was significant (β = −0.199, boot SE = 0.032, p < 0.001), indicating that academic self-efficacy indirect-only mediated the relationship of time-management skills with drop-out intentions. This implies that academic self-efficacy “fully” mediated the relationship between time-management skills and drop-out intentions.
Transfer University Intentions
The overall model fit for transfer university intentions was good. The chi-square test was significant (χ2 = 228.576, df = 121, p < 0.001), CFI = 0.983; TLI=0.980; RMSEA = 0.035 (90% CI 0.028–0.042); SRMR = 0.080. As seen in Table 1, the direct effects reveals that academic self-efficacy is positively related to time-management skills (β = 0.451, boot SE = 0.044, p < 0.001), which in turn was negatively related to transfer university intensions (β = −0.216, boot SE = 0.059, p < 0.001). The direct effect from time-management skills to transfer university intentions was insignificant (β = 0.083, boot SE = 0.056, p = 0.135). That is, the better time-management skills that were reported, the higher was their academic self-efficacy, which was related to lower transfer university intentions. The indirect effect of time-management skills on transfer university intentions through self-efficacy was significant (β = −0.098, boot SE = 0.030, p = 0.001). These results indicate the indirect-only mediation of academic self-efficacy when transfer university intentions is an outcome variable. This implies that academic self-efficacy “fully” mediated the relationship between time-management skills and transfer university intentions.
Transfer Study Field Intentions
The overall model fit for transfer study field intentions was very good. The chi-square test was significant (χ2 = 192.841, df = 91, p < 0.001), CFI = 0.987; TLI=0.985; RMSEA = 0.039 (90% CI 0.031–0.046); SRMR = 0.051. As in the abovementioned result, the direct effect from time-management skills are positively related to academic self-efficacy (β = 0.459, boot SE = 0.042, p < 0.001), which in turn was negatively related to transfer study field intensions (β = −0.265, boot SE = 0.054, p < 0.001). The direct effect from time-management skills to transfer study field intentions was insignificant (β = −0.022, boot SE = 0.052, p = 0.674). That is, the better time-management skills, the higher was the academic self-efficacy, which was related to lower transfer study field intentions. The indirect effect of time-management skills on transfer study field intentions through self-efficacy was significant (β = −0.122, boot SE = 0.028, p < 0.001), which indicated that academic self-efficacy also indirect-only mediated the relationship between time-management skills and students' transfer study field intentions. In other words, academic self-efficacy “fully” mediated the relationship between time-management skills and transfer study field intentions.
Summary
Overall, these results indicate “indirect-only” mediation of time-management skills on drop-out and transfer-out intentions by academic self-efficacy. The indirect only mediation overlaps with Baron and Kenny (1986) conceptualization of full mediation effect excluding precondition of significant total and direct effects (Zhao et al., 2010; Rucker et al., 2011). We hypothesized (Hypothesis 1) that relation of time-management with attrition intentions would be mediated by academic self-efficacy. The hypothesis was supported despite generally small effect size of time-management skills. The indirect-only mediation was found in all three cases (see Table 1). Further, comparison of mediation effect sizes (completely standardized mediation effects) showed that the effect of academic self-efficacy was larger in case of drop-out (β = −0.199, p < 0.001) and transfer study field intentions (β = −0.122, p < 0.001) than transfer university intentions (β = −0.098, p < 0.001). Thus, Hypothesis 3 which assumed that the mediated effects might differ depending on the category of intention (i.e., drop-out, transfer-out) was supported.
Time-Management Skills and Attrition Intentions Via Academic and Social Integration
Drop-Out Intentions
The overall model fit for drop-out intentions was very good. The chi-square test was significant (χ2 = 301.647, df = 83, p < 0.001), CFI = 0.985; TLI=0.981; RMSEA = 0.059 (90% CI 0.052–0.066); SRMR = 0.052. The direct effects reveals that academic integration and social integration are positively related to time-management skills (β = 0.321, boot SE = 0.044, p < 0.001 and β = 0.218, boot SE = 0.045, p < 0.001, respectively), which in turn was negatively related to drop-out intensions (β = −0.287, boot SE = 0.057, p < 0.001 and β = −0.244, boot SE = 0.050, p < 0.001, respectively). The direct effect from time-management skills to drop-out intentions was significant (β = −0.126, boot SE = 0.049, p = 0.01). In other words, the better time-management skills, the higher was the academic and social integration, which was related to lower drop-out intentions. The indirect effect of time-management skills on drop-out intentions through academic integration was significant (β = −0.092, boot SE = 0.022, p < 0.001). Similarly, social integration was a significant mediator (β = −0.053, boot SE = 0.016, p = 0.001). Thus, the results indicate complimentary mediation of time-management skills on drop-out intentions by academic and social integration. This implies that academic and social integration “partially” mediated the relationship between time-management skills and drop-out intentions (see Table 2).
Transfer University Intentions
The overall model fit for transfer university intentions was very good. The chi-square test was significant (χ2 = 378.714, df = 175, p < 0.001), CFI = 0.986; TLI=0.984; RMSEA = 0.040 (90% CI 0.034–0.045); SRMR = 0.078. The direct effects reveals that academic integration and social integration are positively related to time-management skills (β = 0.332, boot SE = 0.045, p < 0.001 and β = 0.222, boot SE = 0.046, p < 0.001, respectively), which in turn was negatively related to transfer university intensions (β = −0.126, boot SE = 0.054, p = 0.02 and β = −0.306, boot SE = 0.054, p < 0.001, respectively). The direct effect from time-management skills to transfer university intentions was insignificant (β = 0.094, boot SE = 0.050, p = 0.06). In other words, the better time-management skills, the higher was the academic and social integration, which was related to lower transfer university intentions. The indirect effect of time-management skills on transfer university intentions through academic integration was significant (β = −0.042, boot SE = 0.019, p = 0.03). Similarly, social integration was a significant mediator (β = −0.068, boot SE = 0.019, p < 0.001). Thus, the results indicate indirect-only mediation of time-management skills on transfer university intentions by academic and social integration. This implies that academic and social integration “fully” mediated the relationship between time-management skills and transfer university intentions (see Table 3). In comparison to the two other models in Tables 2, 4, the direct effect from time-management skills to transfer university intentions had a positive sign. In addition, only this model produced a non-significant total effect (β = −0.015, boot SE = 0.049, p = 0.768). This finding is in line with the result found when academic self-efficacy was specified as the mediator (see Table 1).
Transfer Study Field Intentions
The overall model fit for transfer study field intentions was very good. The chi-square test was significant (χ2 = 332.436, df = 136, p < 0.001), CFI = 0.988; TLI=0.986; RMSEA = 0.044 (90% CI 0.038–0.050); SRMR = 0.063. The direct effects reveals that academic integration and social integration are positively related to time-management skills (β = 0.321, boot SE = 0.046, p < 0.001 and β = 0.224, boot SE = 0.046, p < 0.001, respectively), which in turn was negatively related to transfer study field intensions (β = −0.171, boot SE = 0.058, p = 0.003 and β = −0.262, boot SE = 0.054, p < 0.001, respectively). The direct effect from time-management skills to transfer study field intentions was insignificant (β = −0.030, boot SE = 0.048, p = 0.53). In other words, the better time-management skills, the higher was the academic and social integration, which was related to lower transfer study field intentions. The indirect effect of time-management skills on transfer study field intentions through academic integration was significant (β = −0.055, boot SE = 0.021, p = 0.01). Similarly, social integration was a significant mediator (β = −0.059, boot SE = 0.017, p = 0.001). Thus, the results indicate indirect-only mediation of time-management skills on transfer study field intentions by academic and social integration. This implies that academic and social integration “fully” mediated the relationship between time-management skills and transfer study field intentions (see Table 4).
Summary
In sum, it was hypothesized (Hypothesis 2) that effect of time management on attrition intentions would be mediated by academic and social integration. The hypothesis was supported despite generally small effect sizes of time-management skills. The indirect-only mediation was found in case of transfer intentions (see Tables 3, 4). The indirect only mediation overlaps with Baron and Kenny (1986) conceptualization of full mediation effect excluding precondition of significant total and direct effects (Zhao et al., 2010; Rucker et al., 2011). Further, the complementary mediation was found in case of drop-out intentions (see Table 2). Thus, academic and social integration only “partially” explained the proposed pattern of relationship. Thus, Hypothesis 3 which assumed that the mediated effects might differ depending on the category of intention (i.e., drop-out, transfer-out) was supported. Of note, academic integration is relatively more important for drop-out intentions than social integration (as indicated by the beta coefficients), while the opposite is true for transfer intentions.
Results Summary
The results of the present study lend support to our initial hypotheses and can be summarized as follows. The relationship between time-management skills and attrition intentions was mediated by students' academic self-efficacy beliefs. Similar results were obtained when analyzing mediatory effects of academic and social integration. However, taking differences in the outcome variables (i.e., attrition intentions) into account, the effects of time-management skills mediated by academic self-efficacy were on average larger than those mediated by academic and social integration constructs. Furthermore, the total effect was insignificant in both models specifying transfer university intentions as the outcome variable. Clearly, one reason for this is the generally weaker effect between the mediators and transfer-out intentions. Also, in both the transfer university models the sign of the direct and indirect effect are of opposite directions, which leads to a reduction of the total effect. However, the primary aim of the study was to establish mediation, which is possible without a significant total effect (Zhao et al., 2010; Rucker et al., 2011; Agler and De Boeck, 2017).
Discussion
Behavioral intentions are mental states that are generally assumed to capture commitment or motivation to act and readiness of a person to perform a specific behavior (Fishbein and Ajzen, 1975; Ajzen, 1991; Webb and Sheeran, 2006; Gollwitzer, 2012). However, despite the theoretical and practical utility of behavioral intentions, few studies have focused on intentions in the context of academic attrition. The objective of this paper was to investigate mechanisms that could explain different types of attrition intentions, i.e., drop-out, transfer university, and transfer study field intentions. Thus, we investigated if three potential factors would facilitate attrition intentions among Norwegian university students by mediating the effect of time-management skills. In particular, we focused on the mediatory effects of academic self-efficacy, academic integration, and social integration (Tinto, 1975, 1993; Robbins et al., 2004; Willcoxson et al., 2011).
The findings of the present study lend support to previous research (e.g., Tinto, 1975, 1993; Robbins et al., 2004; Willcoxson et al., 2011), but also contribute to research on academic attrition in the following ways. First, the findings that time-management skills and academic self-efficacy are important in explaining students' academic performance and attrition intentions are in line with previous research (Robbins et al., 2004; Willcoxson et al., 2011; Dunlosky et al., 2013). However, addressing either of them separately with an aim to reduce attrition might be tricky. For example, findings by Jairam (2019) indicate that despite being explicitly taught effective study strategies, students continued to use the ones that are commonly found to be less productive. The author concluded that traditional approaches used to reduce attrition and improve retention such as teaching students academic skills might be ill-suited practice. One of the potential reasons proposed by Wernersbach et al. (2014) might be the neglect of students' academic self-efficacy beliefs. This assumption is in line with our results showing that although time-management skills were not directly related to attrition intentions, while the indirect effect through academic self-efficacy was.
Second, we tested the mediatory role of academic and social integration in the relationship of students' time-management skills with attrition intentions. Both factors were significant in mediating the effects of time-management skills. The findings indirectly support assumptions of Tinto (1975, 1993), Bean (1982, 1990), and Cabrera et al. (1993) on the importance of academic environment in the process of academic attrition. Nevertheless, a comparison of the proposed mediatory models showed that cognitive factors (i.e., academic self-efficacy) had generally larger effects in explaining students' attrition intentions than traditionally considered social factors of the academic environment (i.e., academic and social integration). These results support Tinto (2017) recent assumptions that students' perspectives and perceptions should be also addressed when devising interventions and assistance programs.
Also, the results of the current study showed that both academic and social integration were significantly related to students' intentions to drop-out, transfer to another university, and transfer to another study field. The findings contradict Bean and Metzner's (1985) idea that social factors are less important for non-traditional students. These researchers defined non-traditional students as individuals who are either older than 24 years, do not live on campus, are part-time students, or who have all these characteristics. According to the definition, the students from the current sample can be defined as non-traditional (e.g., 35% were older than 24 years and none of the students live on campus). Hence, further investigation of social and academic integration factors in the Norwegian context adjusting the definition of non-traditional students might provide valuable insights into academic attrition. For example, culture-specific validity and reliability of the theories on academic attrition. Similar conclusions can be made based on the negative relationship between academic and social integration with transfer university intentions. These findings stand in contrast to what has been found on the issue of vertical transfer (i.e., positive relationship) among American students (Nora and Rendon, 1990; Tinto, 1993).
Finally, the results of the present study show that the pattern and magnitude of the effects were dependent on the outcome variable being measured. Specifically, academic and social integration complementary or “partially” mediated the relationship between time-management skills and drop-out intentions. In contrast, both factors indirect-only or fully mediated the same relationship in the case of transfer intentions. Also, the effects mediated by academic self-efficacy were two times larger for drop-out intentions than transfer university intentions. These results are in line with previous findings that students transferring to other universities might be equally able as students who persist and more able than those who drop-out entirely (Tinto, 1993; Quinn-Nilas et al., 2019). Moreover, our results showed that time-management skills were positively related to students' intentions to change university. Although the relationship was insignificant, it provides an indirect support to the same notion. Further, the findings indicate the significance of distinction among students' attrition intentions which is in line with previous research on attrition behavior (Hovdhaugen, 2009). To the best of our knowledge, this is the first study addressing the same issue in terms of behavioral intentions.
These findings are important from several perspectives. First, from the theoretical perspective, students should be distinguished into more than two groups when behavioral intentions are used as a proxy of students' future behavior. The problem with dichotomization of students (i.e., returning and non-returning students) is inaccuracy in prediction and explanation of students' behavior. The same consequences could be assumed when students who leave are treated as a single population. Second, from the perspective of the government and universities, decisions based on the findings treating attrition students as a single population might potentially lead to the opposite outcomes than those being expected.
Limitation and Future Studies
One of the main limitations of the current study regards the psychometric properties of the attrition intentions scale. Four items used to measure the degree of intention's formation did not show an expected factor structure. A more precise formulation of the response items should be evaluated. Similarly, increasing the number of items measuring attrition intentions is a possible solution and should be addressed in future studies. This would require development of a specific measurement scale due to researchers' preference to use single-item measures in the field of academic attrition.
Second, the measure of academic self-efficacy that has been used in the current study measures a more general perception of students' academic-related beliefs. Thus, the observed effect sizes might be underestimated (Bandura, 1997). Future research studies might consider devising and validation of a time management specific scale to validate this assumption.
Third, academic skills is a multifaceted construct (Tressel et al., 2019). Even if time-management skills is a key process of students' self-regulation and academic success, it does not cover all aspects of academic skills and competences (Zimmerman, 2002; Credé and Kuncel, 2008). Thus, future studies should address other important aspects of the phenomenon, such as critical thinking, metacognition, depth of information processing.
Fourth, the design of the current study does not allow to make firm conclusions about causality of the observed patterns of relationships (i.e., time-management skills, self-efficacy). Based on the available evidence, the relationship may be accounted for by alternative models (MacCallum et al., 1993). For example, the relationship between self-efficacy and skills (study strategies) may be bi-directional (Phan, 2011). Thus, validation of the results by experimental or/and longitudinal studies is required. The research findings by van Dinther et al. (2011); Bartimote-Aufflick et al. (2016), and Weinstein et al. (2000) might provide some valuable insights on potential study designs.
Fifth, it is worth mentioning an exploratory aspect of the present study. Non-probability based sampling method (i.e., convenience sampling) has been used for data collection purposes. Thus, generalization of the results of the present study to the whole population of Norwegian students should be done with caution. Future studies should preferably acquire the probability based sampling methods to make more valid statistical inferences.
Finally, actual attrition behaviors (e.g., registry data, university records on students' academic status) should also be considered in future studies. As discussed, attrition intentions are closely related to students' actual behavior (Bean, 1982; Mashburn, 2000). Although behavioral intentions can be assumed to be a close approximation of future behaviors, they might not necessarily lead to the actual implementation of those intentions (Wu and Du, 2012). Further clarification of the relationship of the proposed mechanisms with actual behaviors is important and will be addressed in future studies by the current research group.
Conclusion
The findings of the current study indicate the significance of distinction among students' attrition intentions which is in line with previous research on attrition behavior (Hovdhaugen, 2009). Thus, future studies should be explicit on what is the primary outcome of their study. Further, consistent with previous research findings our results provide preliminary evidence on the mechanism being involved in the process of academic attrition. Providing students the tools (i.e., skills) required for academic success might not be enough, they should also believe that that they can succeed if implementing these tools. Although future experimental studies are required to support the indicated pattern of relationship between time-management skills and attrition intentions. These studies would provide a more solid scientific evidence for development of effective assistance programs for students (Jairam, 2019). What is clear is that “students' perceptions of their experiences add another dimension to our understanding of the complex process of persistence and completion” (Tinto, 2017, p.264).
Data Availability Statement
The data will be made available at Open Science Framework (https://osf.io/gszjq/?view_only=64754bda6648487ba4e821e4b9272a16) at a latter point.
Ethics Statement
Ethical review and approval was not required for the study on human participants in accordance with the local legislation and institutional requirements. The patients/participants provided their written informed consent to participate in this study.
Author Contributions
EN wrote the draft and did the statistical analyses under the supervision of FS. TG-K assisted with data analyses. RS, TG-K, and FS edited the manuscript. All authors contributed to the article and approved the submitted version.
Funding
This research was facilitated by funds from the UiT The Arctic University of Norway and publication charges were funded by a grant from the publication fund of UiT The Arctic University of Norway.
Conflict of Interest
The authors declare that the research was conducted in the absence of any commercial or financial relationships that could be construed as a potential conflict of interest.
Acknowledgments
We would like to thank the administrative staff at the University of Oslo who have distributed our questionnaire. Also, we wish to thank Hege Elise Tønsberg for assistance in distribution of gift cards. Special thanks to the committee of the Research Program at UiT (Sarah Martiny, Kamilla Rognmo, Gabor Csifcsak, and Heidi Solberg) and my peer-students for trust, support, and making this research project possible.
Supplementary Material
The Supplementary Material for this article can be found online at: https://www.frontiersin.org/articles/10.3389/feduc.2020.606291/full#supplementary-material
Footnotes
1. ^The findings of the correlational study by Svartdal et al. (2020) provide indirect support on the mediatory role of academic self-efficacy in the relation of academic skills with procrastination.
3. ^https://osf.io/gszjq/?view_only=64754bda6648487ba4e821e4b9272a16 where readers can find preregistration protocol and additional materials: https://osf.io/gszjq/?view_only=8bf1d551536441f1a8e8478143b8932c.
4. ^https://osf.io/gszjq/?view_only=64754bda6648487ba4e821e4b9272a16 where readers can find preregistration protocol and additional materials. https://osf.io/gszjq/?view_only=8bf1d551536441f1a8e8478143b8932c (Table 1).
References
Agler, R., and De Boeck, P. (2017). On the interpretation and use of mediation: multiple perspectives on mediation analysis. Front. Psychol. 8:1984. doi: 10.3389/fpsyg.2017.01984
Ajzen, I. (1991). The theory of planned behavior. Organ. Behav. Hum. Decis. Process 50, 179–211. doi: 10.1016/0749-5978(91)90020-T
Ajzen, I. (2002). Perceived behavioral control, self-efficacy, locus of control, and the theory of planned behavior1. J. Appl. Soc. Psychol. 32, 665–683. doi: 10.1111/j.1559-1816.2002.tb00236.x
Aljohani, O. (2016). A comprehensive review of the major studies and theoretical models of student retention in higher education. High. Educ. Stud. 6, 1–18. doi: 10.5539/hes.v6n2p1
Bandura, A. (1977). Self-efficacy: toward a unifying theory of behavioral change. Psychol. Rev. 84, 191–215. doi: 10.1037/0033-295X.84.2.191
Bandura, A. (1986). Social Foundations of Thought and Action: A Social Cognitive Theory. Englewood Cliffs, NJ: Prentice-Hall.
Baron, R. M., and Kenny, D. A. (1986). The moderator-mediator variable distinction in social psychological research: conceptual, strategic, and statistical considerations. J. Pers. Soc. Psychol. 51, 1173–1182. doi: 10.1037/0022-3514.51.6.1173
Bartimote-Aufflick, K., Bridgeman, A., Walker, R., Sharma, M., and Smith, L. (2016). The study, evaluation, and improvement of university student self-efficacy. Stud. High. Educ. 41, 1918–1942. doi: 10.1080/03075079.2014.999319
Bean, J. (1982). Student attrition, intentions, and confidence: interaction effects in a path model. J. Assoc. Inst. Res. 17, 291–320. doi: 10.1007/BF00977899
Bean, J., and Eaton, S. B. (2001). The psychology underlying successful retention practices. J. Coll. Student Reten. 3, 73–89. doi: 10.2190/6R55-4B30-28XG-L8U0
Bean, J. P. (1990). “Why students leave: insights from research,” in The Strategic Management of College Enrollments, eds D. Hossler and Associates (San Francisco: Jossey-Bass) 147–169
Bean, J. P., and Metzner, B. S. (1985). a conceptual model of nontraditional undergraduate student attrition. Rev. Educ. Res. 55, 485–540. doi: 10.3102/00346543055004485
Bernardo, A., Esteban, M.-A., Cervero, A., Cerezo, R., and Herrero, F. J. (2019). the influence of self-regulation behaviors on university students' intentions of persistance. Front. Psychol. 10:2284. doi: 10.3389/fpsyg.2019.02284
Bonsaksen, T. (2018). Psychometric properties of the short assist scales. Uniped 41, 164–181. doi: 10.18261/issn.1893-8981-2018-02-07
Brown, T. (2015). Confirmatory Factor Analysis for Applied Research, 2nd Edn. New York, NY: Guilford Press.
Burger, A. (2017). Factors and Experiences Related to the Academic Success of Students in the Faculty of the Humanities. (Doctoral dissertation, University of the Free State). Available online at: http://hdl.handle.net/11660/6515
Cabrera, A. F., Nora, A., and Castaneda, M. B. (1993). College persistence: structural equations modeling test of an integrated model of student retention. J. High. Educ. 64, 123–139. doi: 10.2307/2960026
Cathey, C. L., Visio, M. E., Whisenhunt, B. L., Hudson, D. L., and Shoptaugh, C. F. (2016). Helping when they are listening: a midterm study skills intervention for introductory psychology. Psychol. Learn. Teach. 15, 250–267. doi: 10.1177/1475725716646319
Cortina, J. M. (1993). What is coefficient alpha? An examination of theory and applications. J. Appl. Psychol. 78, 98–104. doi: 10.1037/0021-9010.78.1.98
Credé, M., and Kuncel, N. R. (2008). Study habits, skills, and attitudes: the third pillar supporting collegiate academic performance. Perspec. Psychol. Sci. 3, 425–453. doi: 10.1111/j.1745-6924.2008.00089.x
Dalgard, O. S., Mykletun, A., Rognerud, M., Johansen, R., and Zahl, P. H. (2007). Education, sense of mastery and mental health: results from a nation wide health monitoring study in Norway. BMC Psychiatry 7:20. doi: 10.1186/1471-244X-7-20
Diseth, Å. (2001). Validation of a Norwegian Version of the Approaches and Study Skills Inventory for Students (ASSIST): application of structural equation modelling. Scand. J. Educ. Res. 45, 381–394. doi: 10.1080/00313830120096789
Dunlosky, J., Rawson, K. A., Marsh, E. J., Nathan, M. J., and Willingham, D. T. (2013). Improving students' learning with effective learning techniques. Psychol. Sci. Public Interest 14, 4–58. doi: 10.1177/1529100612453266
Entwistle, N., Tait, H., and McCune, V. (2000). Patterns of response to an approaches to studying inventory across contrasting groups and contexts. Eur. J. Psychol. Educ. 15, 33–48. doi: 10.1007/BF03173165
Farr-Wharton, B., Charles, M. B., Keast, R., Woolcott, G., and Chamberlain, D. (2018). Why lecturers still matter: the impact of lecturer-student exchange on student engagement and intention to leave university prematurely. High. Educ. 75, 167–185. doi: 10.1007/s10734-017-0190-5
Fishbein, M., and Ajzen, I. (1975). Belief, Attitude, Intention and Behavior: An Introduction to Theory and Research. Reading, MA: Addison-Wesley.
Foerst, N. M., Klug, J., Jöstl, G., Spiel, C., and Schober, B. (2017). Knowledge vs. action: discrepancies in university students' knowledge about and self-reported use of self-regulated learning strategies. Front. Psychol. 8:1288. doi: 10.3389/fpsyg.2017.01288
George, D., Dixon, S., Stansal, E., Gelb, S. L., and Pheri, T. (2008). Time diary and questionnaire assessment of factors associated with academic and personal success among university undergraduates. J. Am. Coll. Health 56, 706–715. doi: 10.3200/JACH.56.6.706-715
Goldfinch, J., and Hughes, M. (2007). Skills, learning styles and success of first-year undergraduates. Active Learn. High. Educ. 8, 259–273. doi: 10.1177/1469787407081881
Gollwitzer, P. (2012). “Mindset theory of action phases,” in Handbook of Theories of Social Psychology: Vol. 1 (Vol. 1, pp. 526–546), eds P. A. Van Lange, A. W. Kruglanski and E. T. Higgins (London: SAGE Publications Ltd). doi: 10.4135/9781446249215.n26
Grosset, J. M. (1993). A profile of community college stop-outs. Commun. Coll. Rev. 20, 51–58. doi: 10.1177/009155219302000406
Hardre, P. L., and Reeve, J. (2003). A motivational model of rural students' intentions to persist in, versus drop out of, high school. J. Educ. Psychol. 95, 347–356. doi: 10.1037/0022-0663.95.2.347
Hattie, A. C. J., and Donoghue, M. G. (2016). Learning strategies: a synthesis and conceptual model. NPJ Sci. Learn. 1:16013. doi: 10.1038/npjscilearn.2016.13
Heikkilä, A., and Lonka, K. (2006). Studying in higher education: students' approaches to learning, self-regulation, and cognitive strategies. Stud. High. Educ. 31, 99–117. doi: 10.1080/03075070500392433
Herrmann, K. J., Bager-Elsborg, A., and McCune, V. (2017). Investigating the relationships between approaches to learning, learner identities and academic achievement in higher education. High. Educ. 74, 385–400. doi: 10.1007/s10734-016-9999-6
Hossler, D., and Bontrager, B. (2014). Handbook of strategic enrollment management, 1st Edn. San Francisco, CA: Wiley.
Hovdhaugen, E. (2009). Transfer and dropout: different forms of student departure in Norway. Stud. High. Educ. 34, 1–17. doi: 10.1080/03075070802457009
Hovdhaugen, E. (2011). Do structured study programmes lead to lower rates of dropout and student transfer from university? Irish Educ. Stud. 30, 237–251. doi: 10.1080/03323315.2011.569143
Hoyt, J. E., and Winn, B. A. (2004). Understanding retention and college student bodies: differences between drop-outs, stop-outs, opt-outs, and transfer-outs. NASPA J. 41, 395–417. doi: 10.2202/1949-6605.1351
Hu, L.-T., and Bentler, P. M. (1999). Cutoff criteria for fit indexes in covariance structure analysis: conventional criteria versus new alternatives. Struc. Equat. Model. Multidiscip. J. 6, 1–55. doi: 10.1080/10705519909540118
Jairam, D. (2019). First-year seminar focused on study skills: an ill-suited attempt to improve student retention. J. Further High. Educ. 44, 513–517. doi: 10.1080/0309877X.2019.1582757
Kitsantas, A., Winsler, A., and Huie, F. (2008). Self-regulation and ability predictors of academic success during college: a predictive validity study. J. Adv. Acad. 20, 42–68. doi: 10.4219/jaa-2008-867
MacCallum, R. C., Wegener, D. T., Uchino, B. N., and Fabrigar, L. R. (1993). the problem of equivalent models in applications of covariance structure analysis. Psychol. Bull. 114, 185–199. doi: 10.1037/0033-2909.114.1.185
MacKinnon, D. P., Lockwood, C. M., and Williams, J. (2004). Confidence limits for the indirect effect: distribution of the product and resampling methods. Multivariate Behav. Res. 39, 99–128. doi: 10.1207/s15327906mbr3901_4
Mashburn, A. J. (2000). A psychological process of college student dropout. J Coll. Stud. Retent. 2:173190. doi: 10.2190/U2QB-52J9-GHGP-6LEE
Ministry of Education Research (2018). Report to Storting (white paper). Long-term plan for research and higher education 20192028. (Meld. St. 4). Oslo: Norwegian Ministry of Education and Research. Available online at: https://www.regjeringen.no/en/dokumenter/meld.-st.-4-20182019/id2614131/ (Retrieved December 8, 2020).
Muennig, P. A. (2007). “Consequences in health status and costs,” in The Price We Pay: Economic and Social Consequences of Inadequate Education, eds C. Belfield and H. M. Levin (Washington, DC: Brookings Institution Press).
Muthén, L. K., and Muthén, B. O. (1998-2017). Mplus User's Guide. 8th Edn. Los Angeles, CA: Muthén & Muthén.
Nora, A., and Rendon, L. I. (1990). Determinants of predisposition to transfer among community college students: a structural model. Res. High. Educ. 31, 235–255. doi: 10.1007/BF00992310
OECD (2019). Education at a Glance 2019. Available oline at: https://www.oecd-ilibrary.org/content/publication/f8d7880d-en
Pascarella, E. T., and Terenzini, P. T. (1980). Predicting freshman persistence and voluntary dropout decisions from a theoretical model. J. Higher Educ. 51, 60–75. doi: 10.1080/00221546.1980.11780030
Phan, H. P. (2011). Interrelations between self-efficacy and learning approaches: a developmental approach. Educ. Psychol. 31, 225–246. doi: 10.1080/01443410.2010.545050
Pintrich, P. (2004). A conceptual framework for assessing motivation and self-regulated learning in college students. Educ. Psychol. Rev. 16, 385–407. doi: 10.1007/s10648-004-0006-x
Pintrich, P. R. (2000). “Chapter 14—The role of goal orientation in self-regulated learning,” in Handbook of Self-Regulation, eds M. Boekaerts, P. R. Pintrich, and M. Zeidner. (Academic Press) 451–502. doi: 10.1016/B978-012109890-2/50043-3
Pintrich, P. R., and De Groot, E. V. (1990). Motivational and self-regulated learning components of classroom academic performance. J. Educ. Psychol. 82, 33–40. doi: 10.1037/0022-0663.82.1.33
Pintrich, P. R., Smith, D. A. F., Garcia, T., and Mckeachie, W. J. (1993). Reliability and predictive validity of the motivated strategies for learning questionnaire (Mslq). Educ. Psychol. Meas. 53, 801–813. doi: 10.1177/0013164493053003024
Pintrich, P. R. (1991). A Manual for the Use of the Motivated Strategies for Learning Questionnaire (MSLQ). Ann Arbor, MI: National Center for Research to Improve Post-Secondary Teaching.
Porter, S. R. (2000). Including Transfer-Out Behavior in Retention Models: Using the NSLC Enrollment Search Data. AIR Professional File, 82(Winter), 116.
Quinn-Nilas, C., Kennett, D. J., and Maki, K. (2019). Examining explanatory style for failure of direct entry and transfer students using structural equation modelling. Educ. Psychol. 39, 749–767. doi: 10.1080/01443410.2019.1574340
Raciti, M. M. (2012). Predicting first year student transfer intentions: do relationships matter? Aust. Market. J. 20, 65–72. doi: 10.1016/j.ausmj.2011.10.016
Robbins, S. B., Lauver, K., Le, H., Davis, D., Langley, R., and Carlstrom, A. (2004). Do psychosocial and study skill factors predict college outcomes? A meta-analysis. Psychol. Bull. 130, 261–288. doi: 10.1037/0033-2909.130.2.261
Rovai, A. P. (2003). In search of higher persistence rates in distance education online programs. Internet High. Educ. 6, 1–16. doi: 10.1016/S1096-7516(02)00158-6
Rucker, D. D., Preacher, K. J., Tormala, Z. L., and Petty, R. E. (2011). Mediation analysis in social psychology: current practices and new recommendations. Soc. Personal. Psychol. Compass 5, 359–371. doi: 10.1111/j.1751-9004.2011.00355.x
Sauvé, L., Fortin, A., Viger, C., and Landry, F. (2018). Ineffective learning strategies: a significant barrier to post-secondary perseverance. J. Further High. Educ. 42, 205–222. doi: 10.1080/0309877X.2016.1224329
Schunk, D. H. (1985). Self-efficacy and classroom learning. Psychol. Sch. 22, 208–223. doi: 10.1002/1520-6807(198504)22:2<208::AID-PITS2310220215>3.0.CO
Sheeran, P. (2002). Intention—behavior relations: a conceptual and empirical review. Eur. Rev. Soc. Psychol. 12, 1–36. doi: 10.1080/14792772143000003
Smith, R. E. (1989). Effects of coping skills training on generalized self-efficacy and locus of control. J. Pers. Soc. Psychol. 56, 228–233. doi: 10.1037/0022-3514.56.2.228
Statistics Norway (2019a). Completion Rates of Students in Higher Education. Retrieved from: https://www.ssb.no/en/utdanning/statistikker/hugjen (accessed August 7, 2019).
Statistics Norway (2019b). Facts About Education in Norway 2019. Retrieved from https://www.ssb.no/en/utdanning/artikler-og-publikasjoner/facts-about-education-in-norway-2019 (accessed August 7, 2019).
Statistics Norway (2020). Students in Higher Education. Retrieved from https://www.ssb.no/en/utuvh (accessed November 16, 2020)
Steel, P. (2007). The nature of procrastination: a meta-analytic and theoretical review of quintessential self-regulatory failure. Psychol. Bull. 133, 65–94. doi: 10.1037/0033-2909.133.1.65
Steingrímsdóttir, Ó., Næss, Ø., Moe, J., Grøholt, E.-K., Thelle, D., Strand, B., et al. (2012). Trends in life expectancy by education in Norway 1961–2009. Affiliat. Eur. Epidemiol. Federation 27, 163–171. doi: 10.1007/s10654-012-9663-0
Streiner, D. L., Norman, G. R., and Cairney, J. (2015). Health Measurement Scales 5th Edn. Oxford: Oxford University Press.
Svartdal, F., Sale, R. G., Dahl, T. I., Nemtcan, E., and Gamst-Klaussen, T. (2020). Academic Skills and Procrastination: The Role of Academic Self-Efficacy. Manuscript in preparation.
Tinto, V. (1975). Dropout from higher education: a theoretical synthesis of recent research. Rev. Educ. Res, 45, 89–125. doi: 10.3102/00346543045001089
Tinto, V. (2017). Through the eyes of students. J. Coll. Stud. Reten. Res. Theory Prac. 19, 254–269. doi: 10.1177/1521025115621917
Tinto, V. (1993). Leaving College: Rethinking the Causes and Cures of Student Attrition 2nd Edn. Chicago, IL: The University of Chicago Press.
Tressel, T., Lajoie, S. P., and Duffy, M. C. (2019). A guide for study terminology: reviewing a fragmented domain. Can. Psychol. Psychol. Can. 60, 115–127. doi: 10.1037/cap0000138
van Dinther, M., Dochy, F., and Segers, M. (2011). Factors affecting students' self-efficacy in higher education. Educ. Res. Rev. 6, 95–108. doi: 10.1016/j.edurev.2010.10.003
Vossensteyn, J. J., Kottmann, A., Jongbloed, B. W. A., Kaiser, F., Cremonini, L., Stensaker, B., et al. (2015). Dropout and Completion in Higher Education in Europe: Main Report. Luxembourg: Publication Office of the European Union. doi: 10.2766/826962
Webb, T. L., and Sheeran, P. (2006). Does changing behavioral intentions engender behavior change? A meta-analysis of the experimental evidence. Psychol. Bull. 132, 249–268. doi: 10.1037/0033-2909.132.2.249
Weinstein, C. E., Palmer, D. R., and Acee, T. W. (2016). LASSI User's Manual: For Those Administering the Learning and Study Strategies Inventory 3th Edn. H & H Pub.
Weinstein, C. E., Husman, J., and Dierking, D. R. (2000). “Chapter 22—Self-Regulation Interventions with a Focus on Learning Strategies,” in Handbook of Self-Regulation, eds M. Boekaerts, P. R. Pintrich, and M. Zeidner (San Diego: Elsevier Science and Technology), 727–747. doi: 10.1016/B978-012109890-2/50051-2
Weinstein, C. E., Palmer, D. R., and Acee, T. W. (2020). LASSI User's Manual: For Those Administering the Learning and Study Strategies Inventory 3th Edn. Clearwater: H & H Publishing Company.
Wernersbach, B., Crowley, S., Bates, S., and Rosenthal, C. (2014). Study skills course impact on academic self-efficacy. J. Dev. Educ. 37, 14–33.
Willcoxson, L. (2010). Factors affecting intention to leave in the first, second and third year of university studies: a semester-by-semester investigation. Higher Educ. Res. Dev. 29, 623–639. doi: 10.1080/07294360.2010.501071
Willcoxson, L., Cotter, J., and Joy, S. (2011). Beyond the first-year experience: the impact on attrition of student experiences throughout undergraduate degree studies in six diverse universities. Stud. Higher Educ. 36, 331–352. doi: 10.1080/03075070903581533
Wood, J. L., Nevarez, C., and Hilton, A. A. (2012). Determinants of transfer among community college students. J. Appl. Res. Commun. Coll. 19, 64–69.
Wu, J., and Du, H. (2012). Toward a better understanding of behavioral intention and system usage constructs. Eur. J. Inform. Syst. 21, 680–698. doi: 10.1057/ejis.2012.15
Xuereb, S. (2014). Why students consider terminating their studies and what convinces them to stay. Active Learn. Higher Educ. 15, 145–156. doi: 10.1177/1469787414527395
Zhao, X., Lynch, J. G., and Chen, Q. (2010). Reconsidering baron and kenny: myths and truths about mediation analysis. J. Consumer Res. 37, 197–206. doi: 10.1086/651257
Zimmerman, B. J. (1998). Academic studing and the development of personal skill: a self-regulatory perspective. Educ. Psychol. 33, 73–86. doi: 10.1080/00461520.1998.9653292
Keywords: academic attrition, attrition intentions, drop-out intentions, transfer university intentions, transfer study field intentions, integration, academic skills, academic self-efficacy
Citation: Nemtcan E, Sæle RG, Gamst-Klaussen T and Svartdal F (2020) Drop-Out and Transfer-Out Intentions: The Role of Socio-Cognitive Factors. Front. Educ. 5:606291. doi: 10.3389/feduc.2020.606291
Received: 14 September 2020; Accepted: 27 November 2020;
Published: 23 December 2020.
Edited by:
Evely Boruchovitch, Campinas State University, BrazilReviewed by:
Lilly Augustine, Jönköping University, SwedenKathryn Holmes, Western Sydney University, Australia
Copyright © 2020 Nemtcan, Sæle, Gamst-Klaussen and Svartdal. This is an open-access article distributed under the terms of the Creative Commons Attribution License (CC BY). The use, distribution or reproduction in other forums is permitted, provided the original author(s) and the copyright owner(s) are credited and that the original publication in this journal is cited, in accordance with accepted academic practice. No use, distribution or reproduction is permitted which does not comply with these terms.
*Correspondence: Efim Nemtcan, ZWZpbS5uZW10Y2FuJiN4MDAwNDA7dWl0Lm5v