- 1Department of Psychological and Brain Sciences, DeLTA Center, Iowa Neuroscience Institute, University of Iowa, Iowa City, IA, United States
- 2Department of Psychology, Koç University, Istanbul, Turkey
- 3Department of Psychology, Sabanci University, Istanbul, Turkey
Social robots are receiving an ever-increasing interest in popular media and scientific literature. Yet, empirical evaluation of the educational use of social robots remains limited. In the current paper, we focus on how different scaffolds (co-speech hand gestures vs. visual cues presented on the screen) influence the effectiveness of a robot second language (L2) tutor. In two studies, Turkish-speaking 5-year-olds (n = 72) learned English measurement terms (e.g., big, wide) either from a robot or a human tutor. We asked whether (1) the robot tutor can be as effective as the human tutor when they follow the same protocol, (2) the scaffolds differ in how they support L2 vocabulary learning, and (3) the types of hand gestures affect the effectiveness of teaching. In all conditions, children learned new L2 words equally successfully from the robot tutor and the human tutor. However, the tutors were more effective when teaching was supported by the on-screen cues that directed children's attention to the referents of target words, compared to when the tutor performed co-speech hand gestures representing the target words (i.e., iconic gestures) or pointing at the referents (i.e., deictic gestures). The types of gestures did not significantly influence learning. These findings support the potential of social robots as a supplementary tool to help young children learn language but suggest that the specifics of implementation need to be carefully considered to maximize learning gains. Broader theoretical and practical issues regarding the use of educational robots are also discussed.
Introduction
Educational technologies are becoming commonplace in schools and homes across the world. While most attention has been given to screen technologies, such as tablets, and apps used with them (Herodotou, 2018; Papadakis et al., 2019), other digital devices such as robots are also becoming available for common use, to be used either independently or with screen technology. According to a 2020 report, the educational robot market is expected to grow 16% over the next 5 years across the world (Mordor Intelligence, 2020). The global trends are also reflected in academic research. By May 2017, 101 empirical papers (with 309 study results) concerning educational robots were published from different parts of the world such as North America, East Asia, Europe, and the Middle East, and 58% of these studies tested children (Belpaeme et al., 2018a). Importantly, however, most studies thus far not only focused on the affective components of the learning experience (e.g., whether the learner enjoyed the lesson or not) and did not evaluate learning gain but also have a small sample size and lack a control group. This study aims to address this limitation and to gain insights into specific ways to maximize educational robots' benefits for young learners.
Here, we exemplify second language (L2) teaching because fostering L2 skills is critical for the academic and social success of children in the increasingly globalized world, and because as described in the next section, it has been suggested that the unique characteristics of social robots may be particularly suited for language teaching. In addition, we aim to gain a better picture of how social robots should be used in language education, and examine the role of different scaffolds: hand gestures performed by the robot tutor and visual cues presented on the screen accompanying the robot.
Social Robot Tutors in Language Teaching
Social robots are autonomous or semi-autonomous robots that interact and communicate with humans while following the behavioral norms expected by the people with whom the robot is intended to interact (e.g., Bartneck and Forlizzi, 2004). A growing body of literature highlights the potential of social robots in education (e.g., Mubin et al., 2013; Belpaeme et al., 2018a), and more specifically, in teaching first (L1) and second language (L2) to typically- as well as atypically-developing children (e.g., Kanero et al., 2018; van den Berghe et al., 2019; Oranç et al., 2020). Kanero et al. (2018) list the adaptability and the ability to perform actions and gestures as the notable strengths of social robots to support teachers in educational settings. First, social robots can use their sensors to detect learners' motivational and educational needs and adapt their behavior accordingly. Therefore, robot tutors can provide individualized training on children's own time and offer opportunities for learning that might exceed what the teacher can offer in a given day. Second, with its physical body, a social robot can perform various gestures, which are known to facilitate language learning (e.g., Tellier, 2008; Macedonia et al., 2011; Wakefield et al., 2018). Some also suggest that not only the ability to perform gestures, but the physical presence per se might contribute to learning. For example, surveying 33 experimental works, Li (2015) identified the general pattern in which robots are more persuasive and perceived more positively when they are physically with the user than when the robot or another character was presented on the screen. A few studies found that children prefer physically-present robots over an on-screen avatar (Leite et al., 2008; Kose-Bagci et al., 2009; Jost et al., 2012; Looije et al., 2012), though whether the physical presence can affect language learning is unknown (but see Kennedy et al., 2015). Finally, teachers themselves also deem social robots as valid support in their classrooms (Fridin and Belokopytov, 2014; Serholt et al., 2014).
Despite social robots' unique characteristics and ever-increasing public interest in them, there have not been many carefully controlled experiments that examined the potential benefits of social robots in education (Belpaeme et al., 2018b; Kanero et al., 2018). More specifically for our purposes, the empirical findings on the effectiveness of robot tutors in language teaching to typically-developing children are mixed (e.g., Kanda et al., 2004; Moriguchi et al., 2011; Tanaka and Matsuzoe, 2012; Mazzoni and Benvenuti, 2015). Especially regarding vocabulary teaching, robots are found to be merely as effective or even less effective than human tutors and other digital devices (e.g., Hyun et al., 2008; Moriguchi et al., 2011; see Vogt et al., 2019 for a large-scale study). The effectiveness of a robot tutor might vary depending on the alignment between multiple factors in the learning environment, such as the type of learning task (Tazhigaliyeva et al., 2016), and the level of social support (Saerbeck et al., 2010). Based on the idea, we theorized that other scaffolds available in the teaching environment might interact with the effectiveness of robot-led language lessons.
Aligning Affordances of Scaffolds With the Learning Task: Role of Gestures and On-Screen Cues
According to recent instructional approaches, focusing on the effectiveness of technology for supporting learning on its own, i.e., simply testing whether technology is effective or not, provides a limited view; instead the facilitative role of any technology might vary depending on the specific topics or conditions (Lowe et al., 2011). A key question in educational designs is to what extent a particular scaffold aligns with the type of representation that enables a particular learner to successfully complete a specific task (Gibson, 1979). Thus, the focus is not just on the learner, the task, or the technology but the nexus of all three. While prior work on educational technology in general, and social robots in particular, focused on different features of the technology, here we evaluate the effectiveness of social robots in a wider context and examine how the role of social robots vary as a function of the scaffolds available in the learning environment.
Digital learning environments differ not only in the specific instructional technology they leverage but also on several other dimensions. In a typical L2 tutoring context, the teacher provides auditory information, i.e., L2 word, and the instruction is supported by a visual component, i.e., picture of the object to be labeled. To gather students' attention, teachers typically provide additional visual scaffolds, also referred to as focusing or signaling cues (Jones and Plass, 2002; Marulis and Neuman, 2010). Here we examine two types of scaffolds that are frequently used and have been effective in teaching vocabulary–static, attentional cues provided on a screen, also referred to as on-screen cues (Höffler and Leutner, 2007), and dynamic co-speech hand gestures (Wakefield et al., 2018).
The first set of scaffolds we consider are static visual attentional cues (i.e., focusing/signaling cues), which consist of cues such as arrows or highlighters (Lowe and Boucheix, 2011). Prior research showed that visual cues enable instruction to lead to more robust learning by focusing on the learner's attention to relevant information (e.g., De Koning et al., 2009). Robot tutors are typically used with other scaffolds such as touchscreen devices that can provide additional signaling cues to direct children's attentional focus. The literature on the effectiveness of on-screen cues has been mixed, and prior work primarily focused on adults (e.g., Tabbers et al., 2004; De Koning et al., 2009). Little is known about how on-screen cues influence children's learning, and how they compare to other cues. Almost nothing is known about the integration of on-screen cues in social robots' teaching although most studies implicitly combine robots with screens.
The second set of scaffolds includes dynamic co-speech gestural cues. Speakers of all ages and backgrounds move their hands as they speak. These co-speech gestures come in different types including pointing, iconic, beat gestures, and emblems (McNeill, 1992), with pointing and iconic gestures being most frequently used in teaching contexts. Pointing gestures, also known as deictic gestures, are gestures that indicate an object, entity, or location through the extension of the index finger or whole hand. Iconic gestures are gestures that depict an action or shape of an object, e.g., drawing a circle in the air to describe round or opening hands wide to describe big. Teachers use gestures extensively in L2 teaching (Kusanagi, 2015), and gestures facilitate language learning in both first language (L1) and L2 (Goldin-Meadow and Wagner, 2005). Instruction that contains gestures typically promotes better learning compared to instruction that does not (e.g., Valenzeno et al., 2003, but see Singer and Goldin-Meadow, 2005; Hostetter, 2011; Congdon et al., 2018). When L2 instruction is accompanied by gestures, adults and children learn and retain novel nouns in L2 better compared to no gesture or meaningless gestures (Tellier, 2008; Macedonia et al., 2011). Further, the facilitating role of gesture is greater for children than adults (Hostetter, 2011).
Although a strength of social robots over other technological tools is their ability to gesture (Kanero et al., 2018), empirical evidence on the effectiveness of robot tutors' gesture remains scarce and mixed–some studies report that the use of gestures by robots might enhance learning (Conti et al., 2017), while others report that gesture might detrimentally influence performance (Yadollahi et al., 2018) or have no effect (Vogt et al., 2019). For example, one study found that 5- to 6-year-old children recalled stories more accurately when stories were narrated by an animated robot (that used gestures, eye gaze, and expressive intonation) than by an inexpressive human teacher (Conti et al., 2017). While this study was one of the first steps in understanding the role of social robots' gestures, the two conditions differed not only on gesture use but also on voice tone and eye gaze. In contrast, another study reported that a social robot's pointing gestures distract children from comprehending the text, especially for those with lower reading proficiency (Yadollahi et al., 2018). A recent study by Vogt and colleagues, which did not find an additional benefit of gestures over a touchscreen tablet, focused on iconic gestures only (Vogt et al., 2019). Overall, existing studies present mixed findings on the effects of gestures in children's learning with robots, and have focused on a specific type of gesture only. Thus, how different gesture types might influence a robot tutor's teaching effectiveness is another gap we aim to fill.
Overall, research on the effectiveness of robot tutors and how it could be influenced by different additional scaffolds remains scarce. To our knowledge, no prior study compared the role of different scaffolds in supporting robot tutors' teaching effectiveness.
Current Study
The current study asks whether (1) children can effectively learn new L2 words from a robot tutor, (2) scaffolds differ in how they support robot teaching (by comparing co-speech hand gestures and on-screen attentional cues), and (3) the type of gesture affects the effectiveness of L2 vocabulary teaching. To answer our main research questions, Study 1 tested a robot tutor. In Study 2, we tested the same questions with a human tutor to examine whether the pattern of results is unique to a robot tutor or would generalize across tutors. In both studies, 5-year-old Turkish-speaking children were taught English measurement adjectives such as big and high. We used measurement adjectives because these are typically covered in school curricula and are central for early STEM education (Bishop, 1988). Further, although the majority of the work focusing on the role of gestures in word learning focused on nouns and verbs (Wakefield et al., 2018; Aussems and Kita, 2019), prior work also established the benefit of using gestures for adjectives (O'Neill et al., 2002). We chose 5-years-old children as participants as they would be familiar with the measurement terms in their native language and because the previous studies suggest that younger children may struggle to be engaged in a lesson with a social robot (Moriguchi et al., 2011; Baxter et al., 2017). The gesture condition tested deictic gestures pointing the picture on a computer screen representing the word to be learned, and iconic gestures representing the meaning of the word. In the on-screen cue condition, a red rectangle was presented around the referent picture on the computer screen. Based on the prior literature, we hypothesized that children would effectively learn new words from a robot tutor. Given the small and mixed literature on scaffolds, however, we formed multiple predictions regarding the effect of scaffolds. On the one hand, given prior work on gestures in teaching, we may observe better learning in the gesture condition compared to the on-screen cue condition. Alternatively, if gestures simply serve as attentional cues, there would be no difference between gesture types, and between gesture vs. on-screen cue conditions.
Study 1: Robot Tutor
Our social robot tutor was NAO, a 54-cm-tall humanoid robot by Softbank Robotics (see Figure 1). NAO has a child-friendly appearance and abilities to perform hand gestures and has been used successfully in many human-robot interaction studies involving young children (e.g., Belpaeme et al., 2018b).
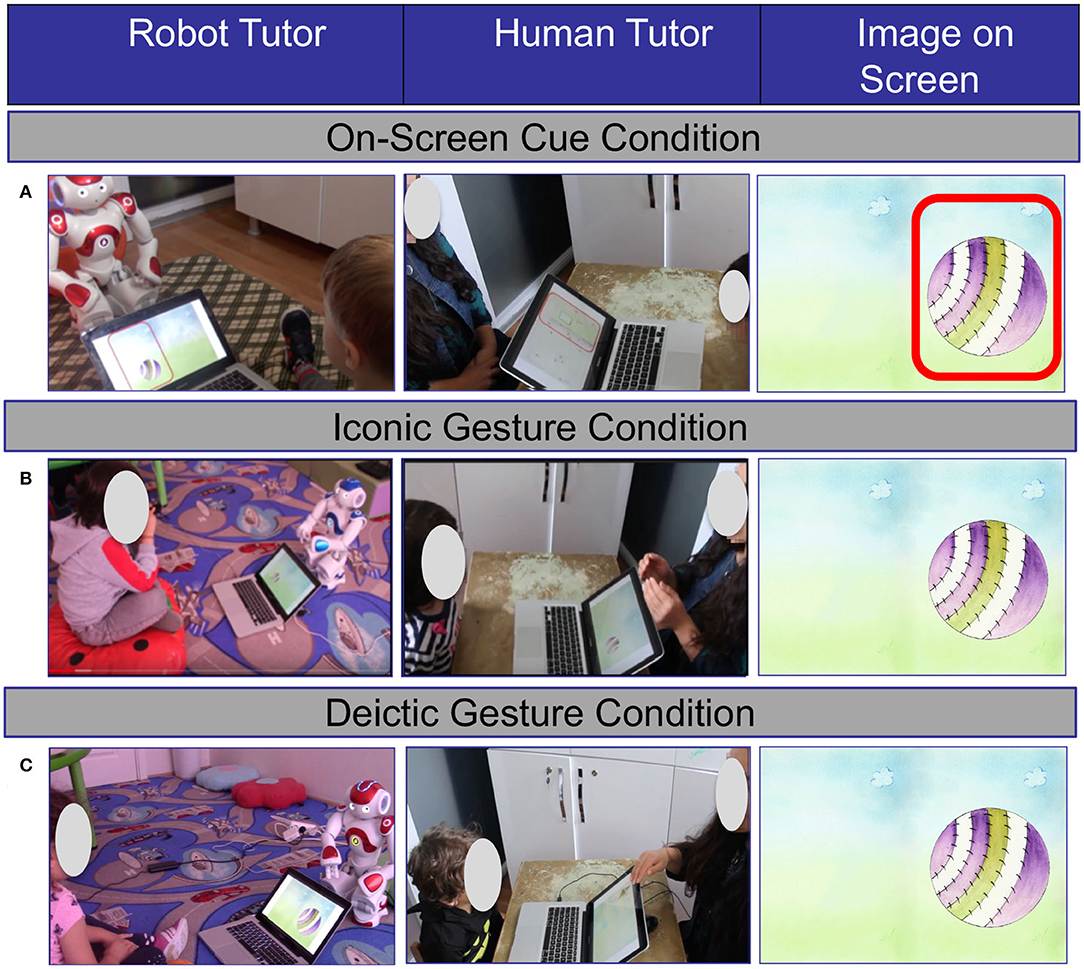
Figure 1. Study design when teaching the word small. (A) In the Screen Cue condition, no gesture is performed, and a red rectangle appears around the object to draw attention to the screen. (B) In the Iconic Gesture condition, an iconic gesture is performed. (C) In the Deictic Gesture condition, the experimenter points to the object on the screen.
Method
Participants
Thirty-eight children participated in Study 1 with the Robot Tutor (Mage= 69.85 months, SD = 4.18, 21 females). All but two children were tested in a quiet room at their own preschool, located in a large city in Turkey (Istanbul or Bursa), the remaining two children were tested in the lab in Istanbul. All participants were free of vision or hearing impairments. The initial sample consisted of 42 children, but one child was excluded because they knew four of the eight English words to be taught and three children quit the study before it ended. A combination of convenience and snowball sampling techniques were used. Initially, multiple preschools that did not offer extensive English education were contacted. When a school expressed interest in the study, the consent forms were sent to families via the teacher or principal. All children included in the study had their parents provided consent and children themselves provided verbal assent at the beginning of the study. All procedures were approved by the Koç University Committee on Human Research.
Stimuli
Children learned four pairs of English measurement adjectives–small and big, wide and narrow, high and low, and tall and short. We first selected six pairs of words that were listed as measurement adjectives in the kindergarten math curricula in the Common Core in the US. The selected words were also balanced in terms of word frequency, familiarity, and imageability (see Coltheart, 1981; Zeno et al., 1995). In selecting the final set of words, we first identified gestures that would typically be used to describe the adjectives (see Table 1 for descriptions of iconic gestures produced for each adjective and its associated object). We then selected a subsample of these gestures that could be performed by NAO, and videotaped NAO performing the gestures. Twenty-seven adults (Mage = 33.19 years; SD = 6.50; 10 females) were asked to watch the videos of NAO gesturing, and rated how well the gesture represented the corresponding word (e.g., high) on a 5-point scale (1-not well at all to 5-very well). Word-gesture pairs that received an average rating of below two were excluded (thick and thin, near and far). The gestures for the remaining words were on average rated as 3.1 (SD = 0.05). We then created images to describe the target measurement adjectives using objects that should be familiar to children in our age range. These images included two balls (big and small), two doors (wide and narrow), two kites (high and low), and two flowers (tall and short) (see Figure 1 for an example and see Supplementary Material for all images as well as videos of NAO's gestures).
Design
In all conditions, the robot verbally taught the target adjectives and the images were presented using Microsoft Powerpoint on a 13-inch laptop screen, but the trials differed in terms of additional scaffolds provided. We used a mixed design where Gesture Type (Deictic, Iconic) was a between-subject factor and Scaffold Type (Gesture, Screen Cue) was a within-subject factor. All children went through two conditions: one of the two Gesture type conditions (Deictic or Iconic) and the On-Screen Cue condition. In the On-Screen Cue condition, the tutor did not perform any gestures. Instead, a red rectangle appeared around the corresponding object to draw attention to the image on the computer screen (Figure 1A). The presence of pictures for the words closely mimics typical learning contexts for L2 learning (e.g., Jones and Plass, 2002). In the Iconic Gesture condition, the tutor performed an iconic gesture while teaching the word (Figure 1B; see also Figure 2 for an example of phases for iconic gestures). In the Deictic Gesture condition, the tutor pointed to the object on the computer screen while teaching the word (Figure 1C). Because NAO has three fingers that cannot move independently of one another, both NAO and the human tutor used palm pointing, instead of index pointing.
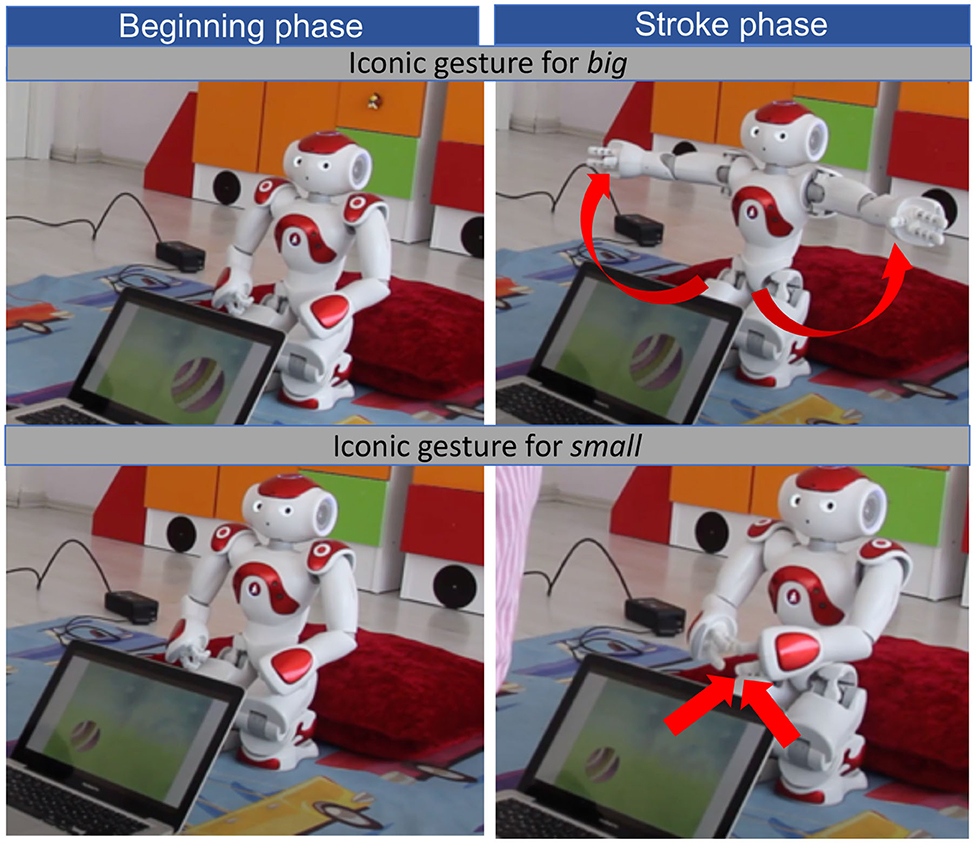
Figure 2. Example for phases (beginning, stroke) of iconic gestures when teaching the word big (top image) and small (bottom image).
In each Scaffold Type condition (Gesture vs. Screen Cue), children learned two pairs of words per condition. Each condition consisted of three blocks, where the word pairs were repeated. The word pairs were counterbalanced such that half of the children learned two pairs of words (e.g., big and small, high and low) in the Gesture condition, whereas half of them learned the same pairs in the On-Screen Cue condition. The order of conditions was also counterbalanced across children. Twenty-one of the 38 children participated in the Iconic Gesture + On-Screen Cue condition and 17 children participated in the Deictic Gesture + On-Screen Cue condition.
Procedure
All children met individually with the human experimenter and the Robot tutor in a quiet room. Prior to the experiment, children were asked if they knew what each English target adjectives meant. One child who knew four of the eight target adjectives was excluded from the dataset. Four children who knew two adjectives (big and small) were included in the overall data analysis but their responses for the big and small questions were excluded.
The child was seated in front of a 13-inch laptop where all images were presented. The Robot tutor sat across from the child, behind the laptop (see Figure 1). A human experimenter first introduced the robot to the child, but had no further interaction with the child, and wirelessly controlled the robot using a Wizard of Oz technique while pretending to complete paperwork. The robot taught two pairs of measurement adjectives per block. Each pair of adjectives were taught with a specific object presented on the screen (e.g., a ball for the words small and big). At the end of each block, children were presented with the image of two objects (e.g., a small ball and a big ball) on the screen, and the robot asked the child to point to the object that corresponded to the target adjective (e.g., small). Children were asked 4 questions per block per condition. Thus, the maximum score on the test was 24 (4 questions x 3 blocks for the Gesture condition and 4 questions x 3 blocks for the Screen-Cue condition). Immediately after completing the three blocks, children also completed a generalization task designed to evaluate whether they could extend the newly learned adjectives to novel objects. Children were presented with a series of new images with new objects representing the same set of eight adjectives (e.g., cars for the adjectives big and small) on the computer screen, and asked to point to the object that corresponded to each of the eight adjectives (see Figure 3 for an example item). The maximum score on generalization questions was 8. Responses were coded online by another experimenter, but the sessions were also videotaped for possible offline coding. The entire session took 15–20 min (see the Appendix for a full description and verbatim transcription of the procedure).
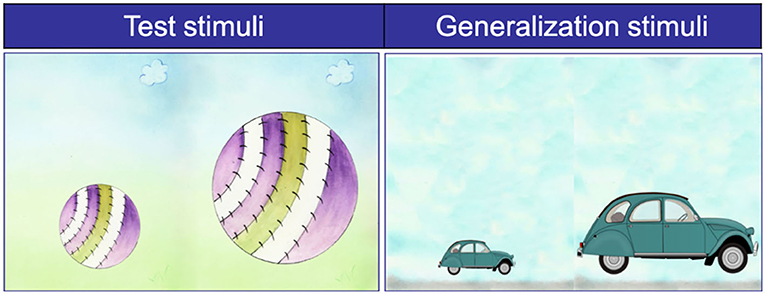
Figure 3. Example stimuli used in training (big and small ball) and test and stimuli used in generalization questions (big and small car).
Results
We first examined if children learned the L2 words. One sample t-tests showed that children performed significantly better than chance both on the test, t(36) = 10.536, p < 0.001, and generalization questions, t(36) = 4.672, p < 0.001. In both the Gesture condition and the Screen Cue condition, children performed significantly better than chance on the test (Gesture: t(37) = 8.047, p < 0.001; Screen Cue: t(36) = 9.481, p < 0.001), as well as the generalization questions (Gesture: t(37) = 4.762, p < 0.001; Screen Cue: t(36) = 4.905, p < 0.001). The percentage of accuracy did not vary as a function of Sex on the test, F(2,34) = 0.622, p = 0.543, partial eta-squared = 0.035, or on generalization questions, F(2,34) = 0.874, p = 0.427, partial eta-squared = 0.049.
Accuracy for the Test Questions as a Function of Gesture Type
Generalized Linear Mixed-Effects Models (GLMMs) were run using SPSS Statistics 23.0 (SPSS Inc., Chicago IL) with accuracy as the dependent variable. The logit was used as the link function to account for the dichotomous (correct vs. incorrect) dependent variable. This first model was to see if the specific Gesture Type (Iconic vs. Deictic) made a difference in children's learning. This first GLMM included Gesture type (Deictic, Iconic) with Block (1, 2, 3) as fixed factors and Subject and Word (referring to the specific adjective used) as random factors. No effects were significant, ps > 0.10. In other words, learning did not vary as a function of whether the tutor used a Deictic or Iconic gesture. Thus, in the subsequent analysis, we collapsed over Gesture type.
Accuracy for the Test Questions as a Function of Scaffold-Type
Another GLMM model was run to examine the role of Scaffold Type (Gesture vs. Screen Cue) on accuracy. This model included fixed effects for Scaffold Type (Gesture, Screen Cue), with Block (1, 2, 3) as fixed factors and Subject and Word as random factors. Figure 4 represents the average accuracy. Results revealed a main effect of Scaffold Type (F(1,906) = 3.931, p = 0.048). Bonferroni pairwise comparison post-hoc tests showed that Screen Cue condition was associated with higher accuracy than the Gesture condition (β = 0.455, SE =0.230, 95% CI [0.005, 0.906]). The odds of giving a correct response instead of the incorrect for the Screen Cue condition was estimated to be exp(0.455) = 1.58 times the corresponding odds for children in the Gesture condition, all other things being equal. Thus, children were more likely to give a correct response in the Screen Cue condition compared to the Gesture conditions. The fixed effect of Block was not significant (β = 0.375, SE = 0.224, 95% CI [−0.841, 0.0654], p = 0.094).
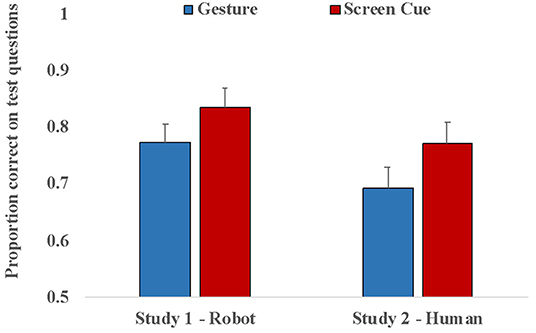
Figure 4. Accuracy on the test as a function of Scaffold Type (Gesture, Screen Cue) and Tutor (Robot, Human).
Accuracy for the Generalization Questions
Parallel models were run on generalization questions' accuracy. No effects reached statistical significance when examining the role of Scaffold Type or the role of specific Gesture Type, all ps > 0.10.
Interim Summary
Children were able to learn words from a Robot tutor and perform above chance on both test and generalization questions. Performance was also above chance both when teaching was accompanied by Gestures or On-Screen Cues. However, the accuracy was significantly higher when the robot tutor's teaching was supported by on-screen cues as compared to gestures. The main goal of the current study was to examine factors that examine the teaching effectiveness of robot tutors. However, these results raised a follow-up question of whether the role of scaffolds was unique to a robot tutor or would also generalize to a human tutor. Thus, we conducted a follow-up study using a human tutor to examine whether the pattern of results and the learning of the children would mimic the findings with the Robot tutor.
Study 2: Human Tutor
Method
Participants
A new group of 41 children participated in the follow-up study that used a human tutor (Mage = 67.6 months, SD = 4.98, 24 females). All but three children were tested in a quiet room at their own preschool, located in a large city in Turkey (Istanbul or Bursa), the remaining three children were tested in the lab in Istanbul. The initial sample consisted of 44 children, but one child was excluded because they knew four of the eight English words to be taught, one child was asked questions in the wrong order due to experimenter error, and one child quit the study before it ended. All other details (including recruitment methods) were the same as Study 1.
Stimuli
Same as Study 1.
Design
Same as Study 1. Out of the 41 children, 23 participated in the Iconic Gesture + On-Screen Cue condition and 18 participated in the Deictic Gesture + On-Screen Cue condition.
Procedure
All children met individually with the human experimenter, who was an adult female, in a quiet room. The experimenter served as the tutor. The child was seated in front of a 13-inch computer screen where all images were presented. The Human tutor sat across from the child. All other details were the same as Study 1.
Results
One sample t-tests on children's percentage of accuracy showed that children performed significantly better than chance both on test questions, t(40) = 8.344, p < 0.001, and generalization questions, t(40) = 6.321, p < 0.001. In both the Gesture and Screen Cue conditions, children performed significantly better than chance on test (Gesture: t(40) = 6.245, p < 0.001; Screen Cue: t(40) = 7.326, p < 0.001), and generalization questions (Gesture: t(40) = 3.703, p = 0.001; Screen Cue: t(40) = 7.248, p < 0.001). The percentage of accuracy did not vary as a function of Sex on the test, F(1,39) = 0.460, p = 0.502, partial eta-squared = 0.012, or on generalization questions, F(1,39) = 1.632, p = 0.209, partial eta-squared =0.040.
Accuracy for the Test Questions as a Function of Gesture Type
GLMMs were run with accuracy as the dependent variable. This first model was to see if the specific Gesture Type (Iconic vs. Deictic) made a difference in children's learning. This first GLMM included Gesture type (Deictic, Iconic) with Block (1, 2, 3) as fixed factors and Subject and Word as random factors. No effects were significant, ps > 0.10. In other words, mimicking the results with the Robot tutor, there was no significant effect of Gesture type, meaning learning did not vary as a function of whether the Human tutor used a Deictic or Iconic gesture. Thus, in the subsequent analysis, we collapsed over Gesture type.
Accuracy of Test Questions as a Function of Scaffold-Type
Another GLMM was run with accuracy as the dependent variable to examine the role of Scaffold Type (Gesture vs. Screen Cue). This second model included fixed effects for Scaffold Type (Gesture, Screen Cue), with Block (1, 2, 3) as fixed factors and Subject and Word as random factors. Figure 4 represents the average accuracy. Results of the first GLMM analysis revealed a main effect of Scaffold Type (F(1,968) = 5.267, p = 0.022. Bonferroni pairwise comparison post-hoc tests showed that Screen Cue condition was associated with higher accuracy than the Gesture condition (β = 0.467, SE = 0.204, 95% CI [0.068, 0.867]). The odds of giving a correct response instead of the incorrect for the Screen Cue condition was estimated to be exp(0.467) = 1.59 times the corresponding odds for children in the Gesture condition, all other things being equal. Thus, children were more likely to give a correct response in the Screen Cue condition compared to the Gesture condition. Children performed better on the 3rd block compared to the 1st block representing learning over time (β = 0.530, SE = 0.199, 95% CI [−0.920, −0.0140], p = 0.008). Thus, the pattern of results mimicked the results with the Robot tutor.
Accuracy for the Generalization Questions
Parallel models were run on generalization questions' accuracy. No effects reached statistical significance, all ps > 0.10 when examining the role of Scaffold Type or the role of specific Gesture Type.
Interim Summary
Children were able to learn words from a Human tutor and perform above chance on both test and generalization questions. Performance was also above chance both when teaching was accompanied by Gestures or On-Screen Cues. Similar to the finding with the Robot tutor, accuracy was higher when teaching was accompanied by On-Screen Cues as compared to Gestures.
Exploratory Comparison of Robot and Human Tutor Across Study 1 and Study 2
Studies 1 and 2 were separately analyzed as one study was complete before the other and thus children were not randomly assigned to the two tutors. Nevertheless, we conducted an exploratory analysis to compare the robot and human tutors. We built an additional GLMM with accuracy as the dependent variable, Tutor (Human, Robot), Scaffold Type (Gesture vs. Screen Cue), and Block (1, 2, 3) as fixed factors, and Subject and Word as random factors.
There was a trend for the effect of Tutor, (F(1,1876) = 3.298, p = 0.070), with the Robot tutor condition being associated with higher accuracy than the Human tutor condition (β = 0.517, SE = 0.31, 95% CI [−1.129, 0.095]). The interaction between Tutor and Scaffold Type was not significant. Similar to results of the individual analysis–we again observed a main effect of Scaffold Type (F(1,1876) = 9.016, p = 0.003), where Screen Cue condition was associated with higher accuracy than the Gesture condition (β = 0.451, SE =0.230, 95% CI [0.001, 0.901]). There was also a main effect of Block, F(1,1876) = 5.285, p = 0.005): Block 1 accuracy was lower than Block 3 accuracy (β = −0.462, SE =0.149, 95% CI [−0.754, −0.171]), representing learning over time.
Discussion
Our goal was to examine the role of social robots in L2 vocabulary learning. We asked whether (1) children can effectively learn new words from a robot tutor, (2) scaffolds differ in how they support robot teaching, and (3) the type of gesture affects effectiveness in L2 vocabulary teaching. First, consistent with our hypothesis, we found that children were able to learn L2 words from a social robot. Second, we showed that children were able to learn when the tutor (robot or human) either gestured or used on-screen cues. Children were able to learn L2 words with both types of scaffolds, but learning outcomes were better when the teaching was supported by on-screen cues than when the tutor gestured. Finally, the type of gesture did not significantly influence L2 vocabulary learning. Below we further discuss our results.
Social Robot and Human Tutors in L2 Vocabulary Teaching
Our results showed that young children were able to effectively learn new L2 vocabulary from a social robot. Children performed above chance not only on the test but also generalization trials in which children were asked to associate the learned words with novel images. Our results are consistent with prior literature but provide novel insights by comparing both social robots and human robots in L2 vocabulary teaching. Although we refrain from emphasizing a trend indicating the robot promoted better learning outcomes than the human tutor, we can state that children successfully learned measurement adjectives in an L2 from a social robot tutor–as well as they learned from a human tutor. Possible explanations for the robot tutor's success include the robot's novelty. Anecdotally, children in the current study expressed great excitement about the robot tutor, and a recent review also emphasizes high enjoyment and anthropomorphic tendencies for robots in children in our age range (Ahmad et al., 2019; van Straten et al., 2020). A study similarly did not find an effect of tutor type, but showed that children gazed more at a robot tutor than a human tutor (Westlund et al., 2017). Another study with 10- to 13-year-olds also found more frequent gaze toward a robot compared to a human (Serholt and Barendregt, 2016). More frequent gaze might indicate a high interest in robots or simply novelty preference. One limitation of our study is that we did not have access to eye gaze data. Future studies should examine if eye gaze on the tutor or gestures mediates learning outcomes. Another limitation of our study is that the process of designing gestures could be improved. While we confirmed that robot gestures were interpretable by adults and while children's performance with the robot tutor did not differ from the human tutor condition, future research could leverage gestures produced by the children in this age range and also examine the information children gather from robot gestures in more detail.
Role of Gestures and On-Screen Cues in L2 Vocabulary Teaching
We demonstrated that the effectiveness of the tutor (social robot and human) varies as a function of the other scaffolds in the environment. In doing so, we extended the prior literature by explicitly focusing on the role of different scaffolds for robot tutors–thus we explored not only whether or not social robots aid learning but also how they might aid learning. More specifically, our results showed that children's learning outcomes were better when the tutor's (both social robot and human) teaching was supported by on-screen cues compared to co-speech hand gestures. Although the results are inconsistent with our hypothesis and were initially surprising, our results dovetail with a recent large-scale study showing no beneficial effect of robot's gestures for learning in teaching English vocabulary to young children (Vogt et al., 2019). Not all studies observe the facilitating effects of gesture on learning (Congdon et al., 2018). Individuals greatly vary in the amount of information they glean from gestures (Demir-Lira et al., 2018; Kartalkanat and Göksun, 2020). Some of this variability is due to age and prior knowledge level (Puccini and Liszkowski, 2012; Post et al., 2013; Novack et al., 2016), and gestures seem to help learners who are ready to learn, but not learners with low background knowledge (Post et al., 2013; Congdon et al., 2018). For example, when learning grammar rules from animations, children with low language skills performed worse when the rules were taught with gestures than when there were no gestures (Post et al., 2013). In a recent study, when social robots used pointing gestures during a reading comprehension task, children with higher proficiency benefited, whereas children with lower proficiency did not (Yadollahi et al., 2018). The children in our study did not know much English, and thus our results are consistent with previous research suggesting that gestures might only help learners who have some prior knowledge. We also did not find differences between the iconic and deictic gesture conditions–again, gesture type might make a difference for learners with a certain level of background knowledge.
In many earlier studies, gesture condition has been compared to control conditions where children were presented with speech only–in other words, conditions with no other scaffold in the environment (e.g., Demir-Lira et al., 2018). Some studies compared gestures to conditions with other educational supports such as with real objects (e.g., Novack et al., 2016). These studies present mixed results–gestures are sometimes more but sometimes less effective than interacting with real objects (Congdon et al., 2018). The mixed findings highlight the importance of comparing and contrasting the educational effectiveness of gestures to other educational scaffolds available in the learning environment such as other scaffolds provided by a screen device as was the case in our study. Taken together, our findings add to the literature by being the first study that compared gestures to an on-screen cue condition where attentional support was provided on a screen.
In terms of task characteristics, for beginner learners, focusing on a single visual scene can aid processing (Atkinson, 2005; Kalyuga, 2005). A previous study on discovery learning using NAO and the touchscreen device also suggests that it might be natural for children to look mostly at the screen instead of the robot (Kennedy et al., 2015). In the current study, children heard the measurement word to be learned (e.g., big) and were also presented with an image associated with the word on the computer screen (e.g., big ball). This context closely mimics typical L2 teaching contexts where children need to coordinate information presented by the tutor and supplementary visuals (such as pictures on a book or screen) to gather the meaning of a word (e.g., Jones and Plass, 2002). The gesture condition required children to shift their attention back and forth between the screen and the tutor. On the contrary, in the on-screen cue condition, the visual image and on-screen cue were both on the computer screen. Overall, gestures might have placed higher attentional demands than on-screen cues. A rich body of literature does support the use of non-verbal aids in children's learning and emerging work compares different types of non-verbal scaffolds in children's learning. For example, a recent study on word learning in preschoolers reported that pictures, compared to gestures, might reduce demands on working memory (Rowe et al., 2013). For beginner learners in our study, providing the visual information and cues on the same screen might have made the matching of images to auditory labels easier. More research should be conducted to understand the role of educational technologies and should further evaluate how different features interact with each other to help or to hinder learning. The ways in which the role of gestures might evolve as children become more proficient in L2 can also be explored.
In terms of the educational implications of our findings, our results are consistent with an emerging broader literature suggesting that the role of scaffolds might vary depending on multiple factors in the learning environment, more specifically the design/technology, the learner as well as task characteristics. Instead of a one-size-fits-all approach, our study also emphasizes the importance of considering the alignment of any educational technology with the particular task at hand as well as the particular characteristics of the learner (Lowe and Boucheix, 2011). Going forward, gestural vs. screen cues can be leveraged to different extents, depending on the background knowledge of the learner. For example, beginner learners could benefit more from static, concrete cues, and over time cues can become more representational and abstract as the learner gathers further background information–a possibility that should be tested in future studies. If robots will be introduced to educational contexts, their role should be evaluated in relation to other supports available in the teaching environment. Moreover, although our design did not provide this feature, social robots could be programmed to respond contingently and vary instruction depending on child needs which is an important future direction for educational research.
In summary, the findings suggest that children can learn new words equally well from a robot tutor or a human tutor when a screen is used as an intermediary medium to present the learning material. Given their potential in the classroom, identifying factors that facilitate the use of social robots in teaching will benefit the development of more supportive environments for L2 teaching. Our results also emphasize the importance of tailored educational environments as opposed to a one-size-fits-all approach. Future education designs could reach maximal effectiveness by leveraging tools that best match the constraints of the task, learning goals, and the learner's needs.
Data Availability Statement
The raw data supporting the conclusions of this article will be made available by the authors, without undue reservation.
Ethics Statement
The studies involving human participants were reviewed and approved by Koç University Committee on Human Research. Written informed consent to participate in this study was provided by the participants' legal guardian/next of kin.
Author Contributions
ÖD-L, JK, CO, TG, and AK conceived the study with feedback from SK and IF. ÖD-L, JK, CO, SK, and IF were in charge of collecting the data. ÖD-L analyzed the data in consultation with JK, CO, TG, and AK. ÖD-L drafted the manuscript, and all authors critically edited it. All authors extensively contributed to the project and approved the final submitted version of the manuscript.
Funding
This study was conducted as part of L2TOR, the European Union's Horizon 2020 research and innovation programme under the Grant Agreement No. 688014 awarded to AK as the PI and TG as the co-PI for the Koç University site.
Conflict of Interest
The authors declare that the research was conducted in the absence of any commercial or financial relationships that could be construed as a potential conflict of interest.
Acknowledgments
We thanked all the schools who participated in the study and families and children who generously gave their time. We also thanked Merve Aslan and Orhun Uluşahin for help with data collection and Sevval Konyali for illustrations.
Supplementary Material
The Supplementary Material for this article can be found online at: https://www.frontiersin.org/articles/10.3389/feduc.2020.599636/full#supplementary-material
References
Ahmad, M. I., Mubin, O., Shahid, S., and Orlando, J. (2019). Robot's adaptive emotional feedback sustains children's social engagement and promotes their vocabulary learning: a long-term child–robot interaction study. Adapt. Behav. 27, 243–266. doi: 10.1177/1059712319844182
Atkinson, R. K. (2005). “Multimedia learning of mathematics,” in The Cambridge Handbook of Multimedia Learning, ed R. E. Mayer (Cambridge: Cambridge University Press), 393–408. doi: 10.1017/CBO9780511816819.026
Aussems, S., and Kita, S. (2019). Seeing iconic gestures while encoding events facilitates children's memory of these events. Child Dev. 90, 1123–1137. doi: 10.1111/cdev.12988
Bartneck, C., and Forlizzi, J. (2004). “A design-centered framework for social human–robot interaction,” in Proceedings of the 2004 IEEE International Workshop on Robot and Human Interactive Communication (Kurashiki), 591–594.
Baxter, P., de Jong, C., Aarts, A., de Haas, M., and Vogt, P. (2017). “The effect of age on engagement in preschoolers child–robot interactions,” in Companion Proceedings of the 2017 ACM/IEEE International Conference on HRI (New York, NY). doi: 10.1145/3029798.3038391
Belpaeme, T., Kennedy, J., Ramachandran, A., Scassellati, B., and Tanaka, F. (2018a). Social robots for education: a review. Sci. Robot. 3:eaat5954. doi: 10.1126/scirobotics.aat5954
Belpaeme, T., Vogt, P., van den Berghe, R., Bergmann, K., Göksun, T., de Haas, M., et al. (2018b). Guidelines for designing social robots as second language tutors. Int. J. Soc. Robot. 10, 325–341. doi: 10.1007/s12369-018-0467-6
Bishop, A. J. (1988). “Mathematical enculturation: a cultural perspective on mathematics education,” in Mathematics Education Library, Vol. 6, eds O. McNamara and R. Barwell (Dordrecht: Kluwer Academic Publishers). doi: 10.1007/978-94-009-2657-8
Coltheart, M. (1981). The MRC psycholinguistic database. Q. J. Exp. Psychol. 33, 497–505. doi: 10.1080/14640748108400805
Congdon, E. L., Kwon, M. K., and Levine, S. C. (2018). Learning to measure through action and gesture: children's prior knowledge matters. Cognition 180, 182–190. doi: 10.1016/j.cognition.2018.07.002
Conti, D., Di Nuovo, A., Cirasa, C., and Di Nuovo, S. (2017). “A comparison of kindergarten storytelling by human and humanoid robot with different social behavior,” in Proceedings of the Companion of the 2017 ACM/IEEE International Conference on Human-Robot Interaction (New York, NY), 97–98. doi: 10.1145/3029798.3038359
De Koning, B. B., Tabbers, H. K., Rikers, R. M., and Paas, F. (2009). Towards a framework for attention cueing in instructional animations: guidelines for research and design. Educ. Psychol. Rev. 21, 113–140. doi: 10.1007/s10648-009-9098-7
Demir-Lira, Ö. E., Asaridou, S. S., Raja Beharelle, A., Holt, A. E., Goldin-Meadow, S., and Small, S. L. (2018). Functional neuroanatomy of gesture–speech integration in children varies with individual differences in gesture processing. Dev. Sci. 21:e12648. doi: 10.1111/desc.12648
Fridin, M., and Belokopytov, M. (2014). Acceptance of socially assistive humanoid robot by preschool and elementary school teachers. Comput. Human Behav. 33, 23–31. doi: 10.1016/j.chb.2013.12.016
Gibson, J. J. (1979). The Theory of Affordances. The Ecological Approach to Visual Perception. Boston, MA: Houghton Mifflin.
Goldin-Meadow, S., and Wagner, S. M. (2005). How our hands help us learn. Trends Cogn. Sci. 9, 234–241. doi: 10.1016/j.tics.2005.03.006
Herodotou, C. (2018). Young children and tablets: a systematic review of effects on learning and development. J. Comput. Assist. Learn. 34, 1–9. doi: 10.1111/jcal.12220
Höffler, T. N., and Leutner, D. (2007). Instructional animation versus static pictures: a meta-analysis. Learn. Instruct. 17, 722–738. doi: 10.1016/j.learninstruc.2007.09.013
Hostetter, A. B. (2011). When do gestures communicate? A meta-analysis. Psychol. Bull. 137:297. doi: 10.1037/a0022128
Hyun, E. J., Kim, S. Y., Jang, S., and Park, S. (2008). “Comparative study of effects of language instruction program using intelligence robot and multimedia on linguistic ability of young children,” in Proceedings of the 17th IEEE International Symposium on Robot and Human Interactive Communication (Munich), 187–192.
Jones, L. C., and Plass, J. L. (2002). Supporting listening comprehension and vocabulary acquisition in French with multimedia annotations. Modern Lang. J. 86, 546–561. doi: 10.1111/1540-4781.00160
Jost, C., Le Pevedic, B., and Duhaut, D. (2012). “Robot is best to play with human!,” in 2012 IEEE RO-MAN: The 21st IEEE International Symposium on Robot and Human Interactive Communication (Paris), 634–639. doi: 10.1109/ROMAN.2012.6343822
Kalyuga, S. (2005). “Prior knowledge principle in multimedia learning,” in The Cambridge Handbook of Multimedia Learning, ed R. E. Mayer (Cambridge: Cambridge University Press), 325–337. doi: 10.1017/CBO9780511816819.022
Kanda, T., Hirano, T., Eaton, D., and Ishiguro, H. (2004). Interactive robots as social partners and peer tutors for children: a field trial. J. Human Comput. Interact. 19, 61–84. doi: 10.1207/s15327051hci1901andamp;2_4
Kanero, J., Geçkin, V., Oranç, C., Mamus, E., Küntay, A. C., and Göksun, T. (2018). Social robots for early language learning: current evidence and future directions. Child Dev. Perspect. 12, 146–151. doi: 10.1111/cdep.12277
Kartalkanat, H., and Göksun, T. (2020). The effects of observing different gestures during storytelling on the recall of path and event information in 5-year-olds and adults. J. Exp. Child Psychol. 189:104725. doi: 10.1016/j.jecp.2019.104725
Kennedy, J., Baxter, P., and Belpaeme, T. (2015). Comparing robot embodiments in a guided discovery learning interaction with children. Int. J. Soc. Robot. 7, 293–308. doi: 10.1007/s12369-014-0277-4
Kose-Bagci, H., Ferrari, E., Dautenhahn, K., Syrdal, D. S., and Nehaniv, C. L. (2009). Effects of embodiment and gestures on social interaction in drumming games with a humanoid robot. Adv. Robot. 23, 1951–1996. doi: 10.1163/016918609X12518783330360
Kusanagi, Y. (2015). The Roles and Functions of Teacher Gesture in Foreign Language Teaching. Ann Arbor, MI: ProQuest Dissertations Publishing, 3745822.
Leite, I., Pereira, A., Martinho, C., and Paiva, A. (2008). “Are emotional robots more fun to play with?,” in RO-MAN 2008 - The 17th IEEE International Symposium on Robot and Human Interactive Communication (Munich), 77–82. doi: 10.1109/ROMAN.2008.4600646
Li, J. (2015). The benefit of being physically present: a survey of experimental works comparing copresent robots, telepresent robots and virtual agents. Int. J. Hum. Comput. Stud. 77, 23–37. doi: 10.1016/j.ijhcs.2015.01.001
Looije, R., van der Zalm, A., Neerincx, M. A., and Beun, R.-J. (2012). “Help, I need some body the effect of embodiment on playful learning,” in 2012 IEEE RO-MAN: The 21st IEEE International Symposium on Robot and Human Interactive Communication (Paris), 718–724. doi: 10.1109/ROMAN.2012.6343836
Lowe, R., and Boucheix, J. M. (2011). Cueing complex animations: does direction of attention foster learning processes? Learn. Instruct. 21, 650–663. doi: 10.1016/j.learninstruc.2011.02.002
Lowe, R., Schnotz, W., and Rasch, T. (2011). Aligning affordances of graphics with learning task requirements. Appl. Cogn. Psychol. 25, 452–459. doi: 10.1002/acp.1712
Macedonia, M., Müller, K., and Friederici, A. D. (2011). The impact of iconic gestures on foreign language word learning and its neural substrate. Hum. Brain Mapp. 32, 982–998. doi: 10.1002/hbm.21084
Marulis, L. M., and Neuman, S. B. (2010). The effects of vocabulary intervention on young children's word learning: a meta-analysis. Rev. Educ. Res. 80, 300–335. doi: 10.3102/0034654310377087
Mazzoni, E., and Benvenuti, M. (2015). A robot-partner for preschool children learning English using socio-cognitive conflict. J. Educ. Techno. Soc. 18, 474–485.
Mordor Intelligence (2020). Educational Robot Market - Growth, Trends, and Forecast (2020 - 2025). Available online at: https://www.prnewswire.com/news-releases/educational-robots-2020-2025-market-insights-trends-and-lucrative-segments-301038232.html (accessed October 25, 2020).
Moriguchi, Y., Kanda, T., Ishiguro, H., Shimada, Y., and Itakura, S. (2011). Can young children learn words from a robot? Interact. Stud. 12, 107–118. doi: 10.1075/is.12.1.04mor
Mubin, O., Stevens, C. J., Shahid, S., Al Mahmud, M., and Dong, J. (2013). A review of the applicability of robots in education. J. Technol. Educ. Learn. 1:209-0015. doi: 10.2316/Journal.209.2013.1.209-0015
Novack, M. A., Wakefield, E. M., and Goldin-Meadow, S. (2016). What makes a movement a gesture? Cognition 146, 339–348. doi: 10.1016/j.cognition.2015.10.014
O'Neill, D. K., Topolovec, J., and Stern-Cavalcante, W. (2002). Feeling sponginess: the importance of descriptive gestures in 2-and 3-year-old children's acquisition of adjectives. J. Cogn. Develop. 3, 243–277. doi: 10.1207/S15327647JCD0303_1
Oranç, C., Baykal, G. E., Kanero, J., Küntay, A., and Göksun, T. (2020). “A look into the future: how digital tools advance language development,” in International Perspectives on Digital Media and Early Literacy: The Impact of Digital Devices on Learning, Language Acquisition and Social Interaction, eds K. Rohlfing and C. Müller-Brauers (London: Routledge), 122–140. doi: 10.4324/9780429321399-10
Papadakis, S., Zaranis, N., and Kalogiannakis, M. (2019). Parental involvement and attitudes towards young Greek children's mobile usage. Int. J. Child Comput. Interact. 22:100144. doi: 10.1016/j.ijcci.2019.100144
Post, L. S., Van Gog, T., Paas, F., and Zwaan, R. A. (2013). Effects of simultaneously observing and making gestures while studying grammar animations on cognitive load and learning. Comput. Human Behav. 29, 1450–1455. doi: 10.1016/j.chb.2013.01.005
Puccini, D., and Liszkowski, U. (2012). 15-month-old infants fast map words but not representational gestures of multimodal labels. Front. Psychol. 3:101. doi: 10.3389/fpsyg.2012.00101
Rowe, M. L., Silverman, R. D., and Mullan, B. E. (2013). The role of pictures and gestures as nonverbal aids in preschoolers' word learning in a novel language. Contemp. Educ. Psychol. 38, 109–117. doi: 10.1016/j.cedpsych.2012.12.001
Saerbeck, M., Schut, T., Bartneck, C., and Janse, M. D. (2010). “Expressive robots in education: varying the degree of social supportive behavior of a robotic tutor,” in Proceedings of the SIGCHI Conference on Human Factors in Computing Systems (New York, NY), 1613–1622. doi: 10.1145/1753326.1753567
Serholt, S., and Barendregt, W. (2016). “Robots tutoring children: longitudinal evaluation of social engagement in child-robot interaction,” in Proceedings of the 9th Nordic Conference on Human-Computer Interaction (New York, NY), 1–10. doi: 10.1145/2971485.2971536
Serholt, S., Barendregt, W., Leite, I., Hastie, H., Jones, A., Paiva, A., et al. (2014). “Teachers' views on the use of empathic robotic tutors in the classroom,” in The 23rd IEEE International Symposium on Robot and Human Interactive Communication (Edinburgh: IEEE), 955–960. doi: 10.1109/ROMAN.2014.6926376
Singer, M. A., and Goldin-Meadow, S. (2005). Children learn when their teacher's gestures and speech differ. Psychol. Sci. 16, 85–89. doi: 10.1111/j.0956-7976.2005.00786.x
Tabbers, H. K., Martens, R. L., and Van Merriënboer, J. J. (2004). Multimedia instructions and cognitive load theory: effects of modality and cueing. Br. J. Educ. Psychol. 74, 71–81. doi: 10.1348/000709904322848824
Tanaka, F., and Matsuzoe, S. (2012). Children teach a care-receiving robot to promote their learning: field experiments in a classroom for vocabulary learning. J. Human Robot Interact. 1, 78–95. doi: 10.5898/JHRI.1.1.Tanaka
Tazhigaliyeva, N., Diyas, Y., Brakk, D., Aimambetov, Y., and Sandygulova, A. (2016). “Learning with or from the robot: exploring robot roles in educational context with children,” in International Conference on Social Robotics (Kansas City, MO), 650–659. doi: 10.1007/978-3-319-47437-3_64
Tellier, M. (2008). The effect of gestures on second language memorisation by young children. Gesture 8, 219–235. doi: 10.1075/gest.8.2.06tel
Valenzeno, L., Alibali, M. W., and Klatzky, R. (2003). Teachers' gestures facilitate students' learning: a lesson in symmetry. Contemp. Educ. Psychol. 28, 187–204. doi: 10.1016/S0361-476X(02)00007-3
van den Berghe, R., Verhagen, J., Oudgenoeg-Paz, O., van der Ven, S., and Leseman, P. (2019). Social robots for language learning: a review. Rev. Educ. Res. 89, 259–295. doi: 10.3102/0034654318821286
van Straten, C. L., Peter, J., and Kühne, R. (2020). Child–robot relationship formation: a narrative review of empirical research. Int. J. Soc. Robot. 12, 325–344. doi: 10.1007/s12369-019-00569-0
Vogt, P., van den Berghe, R., de Haas, M., Hoffmann, L., Kanero, J., Mamus, E., et al. (2019). “Second language tutoring using social robots. A large-scale study,” in IEEE/ACM Int. Conf. on Human-Robot Interaction (Daegu). doi: 10.1109/HRI.2019.8673077
Wakefield, E. M., Hall, C., James, K. H., and Goldin-Meadow, S. (2018). Gesture for generalization: gesture facilitates flexible learning of words for actions on objects. Develop. Sci. e12656. doi: 10.1111/desc.12656
Westlund, J. M. K., Dickens, L., Jeong, S., Harris, P. L., DeSteno, D., and Breazeal, C. L. (2017). Children use non-verbal cues to learn new words from robots as well as people. Int. J. Child Comput. Interact. 13, 1–9. doi: 10.1016/j.ijcci.2017.04.001
Keywords: second language learning, gesture, language learning, social robot, children
Citation: Demir-Lira ÖE, Kanero J, Oranç C, Koşkulu S, Franko I, Göksun T and Küntay AC (2020) L2 Vocabulary Teaching by Social Robots: The Role of Gestures and On-Screen Cues as Scaffolds. Front. Educ. 5:599636. doi: 10.3389/feduc.2020.599636
Received: 27 August 2020; Accepted: 17 November 2020;
Published: 18 December 2020.
Edited by:
Stamatis Papadakis, University of Crete, GreeceReviewed by:
Hatice Kose, Istanbul Technical University, TurkeyWing Chee So, The Chinese University of Hong Kong, China
Copyright © 2020 Demir-Lira, Kanero, Oranç, Koşkulu, Franko, Göksun and Küntay. This is an open-access article distributed under the terms of the Creative Commons Attribution License (CC BY). The use, distribution or reproduction in other forums is permitted, provided the original author(s) and the copyright owner(s) are credited and that the original publication in this journal is cited, in accordance with accepted academic practice. No use, distribution or reproduction is permitted which does not comply with these terms.
*Correspondence: Ö. Ece Demir-Lira, ZWNlLWRlbWlybGlyYUB1aW93YS5lZHU=