- 1Laboratoire ICAR, ENS de Lyon, CNRS, Université Lumière Lyon 2, Lyon, France
- 2Université de Lyon, Lyon, France
- 3Department of Psychology, Hallym University, Chuncheon-si, South Korea
- 4Laboratoire de Physique, Inst. Systèmes Complexes, ENS de Lyon, CNRS, Lyon, France
It is well-known that education related research is carried out within different disciplines and frameworks, but how is it specifically connected through citations to the larger social sciences and humanities? And how can this knowledge be mobilized to improve dialogue between researchers in different communities, given the benefits of integrating different frameworks and methods? We used different scientometric methods to show where exactly research in education connects to social sciences and humanities. This multidisciplinary context provokes a set of integration challenges for research in education. We propose how our work can supplement an existing model in order to give a framework for meeting these challenges with the goal of achieving broader education-related collective knowledge advancement.
Introduction
It is commonly argued whether diversity in a research field is a strength or a curse. Diversity of traditions is a strength when complex questions are answered with a broader perspective and when methodological complementarity can be discovered. It's a curse when participating researchers have difficulty communicating due to no shared vision or common understanding of the literature, thus leading to dispersion of discourse and an unstable epistemic community. The challenge is thus to build on the strengths while addressing the difficulties of such diversity. In this paper, given that educational research is carried out in close relationship to other research fields, we examine the nature of this diversity through bibliometric analyses, and ask four questions. The first two questions empirically examine the nature of educational research using a set of bibliometric methods whereas the second two questions ask what consequences such a nature implies, as well as what can be done, given these consequences.
1. What is the place of educational research in the larger context of social science research and how has it changed since the early 2000's?
2. How does educational research compare to the disciplinary composition of other fields?
3. What can such a disciplinary composition tell us about the challenges facing educational research?
4. And finally, what kind of model for broader education-related collective knowledge advancement can meet these challenges?
In what follows we motivate each of these research questions by giving preliminary definitions, explaining what we want to show, and describing why the second two questions are related to the first two. Finally, we illustrate why answering these questions are important for research in education.
The Connection of Research in Education to Research in Social Sciences and Humanities
Let's unpack the first research question. We are interested in understanding how research in education relates to social science research in terms of how closely connected the references of different publications are. When two publications cite another publication (i.e., a reference), we can surmise shared topics, but also shared theories and methods, depending on the nature of the shared reference. Publications that share references can be grouped into clusters that are either loosely or tightly connected around core references and relations between different clusters can also be evaluated in terms of which references connect clusters to each other. In this case, the notion of “place” refers first to sets of references in particular topic-based clusters that connect research in education to other research in education and second, to sets of references in particular topic-based clusters that connect research in education to research in the social sciences and humanities. This notion of “place” will have changed over some 20 years, between 2000–2004 (Education corpus 1) and 2015–2018 (Education corpus 2), the time periods studied for this article1.
Is Research in Education Undisciplined?
The second research question addresses the disciplinary composition of a field of inquiry. The report on Interdisciplinarity Problems of Teaching and Research in Universities Apostel and Centre for Educational Research Innovation (1972) defines discipline as referring to “the tools, methods, procedures, exempla, concepts, and theories that account coherently for a set of objects or subjects” (Klein, 1990, p. 104); Miller (1982) argues that “within each discipline there are rational, accidental and arbitrary factors responsible for the peculiar combination of subject matter, techniques of investigation, orienting thought models, principles of analysis, methods of explanation and aesthetic standards.” But a discipline is a fuzzy notion.
The level of integration of disciplinary approaches has survived as an indicator that distinguishes between the forms of so-called “non-disciplinary” research: multidisciplinarity, interdisciplinarity and transdisciplinarity. In (van den Besselaar and Heimeriks, 2001), neither theoretical perspectives nor actual results from different participating disciplines are integrated during multidisciplinarity. Rather, “the subject under study is approached from different angles, using different disciplinary perspectives (op. cit., p. 706).” Choi and Pak (2006) hold a similar view, arguing that multidisciplinarity draws on knowledge from different disciplines, but each researcher group stays within its own boundaries.
On the other hand, interdisciplinary research integrates contributing disciplines by creating its own theoretical, conceptual and methodological identity or in other words, “analyzes, synthesizes and harmonizes links between disciplines into a coordinated and coherent whole (Choi and Pak, 2006, p. 351).” The view emphasizing the integration of disciplinary perspectives as a marker of interdisciplinarity is a popular one [e.g., Birnbaum (1981), Cotterell (1979), Hanisch and Vollman (1983), Hausman (1979), Klein (1990, 1996), Kocklemans (1979), Epton et al. (1983), Hermeren (1986)]. But this notion of the necessity of some kind of integration for research to be labeled as interdisciplinarity is contested. Are participants in interdisciplinary projects purposefully taking an integrative stance? Lattuca (2003) argues that integrating presupposes a compatible framework in which such integration can take place — in other words, regardless of the disciplines concerned, interdisciplinary inquiry would naturally take the form of the scientific method found in the natural and physical sciences. This implies that each discipline's way of thinking about concepts, constructs, methods and theories are necessarily compatible if they can be integrated on an a priori basis into an agreed-upon general method of scientific inquiry. However, the measurement within a method is affected by the vantage point from which the phenomena in question are measured (Longino, 2013) and so if the general method of scientific inquiry or the levels of analysis are not compatible, then integration will be difficult. In addition, as Lattuca (op. cit.) also argues, perhaps some interdisciplinary projects attempt to redefine knowledge such as some scholarship in women's studies, ethnic studies, cultural studies and literary studies (Klein, 1996). So, while such redefinition might include integration of disciplinary perspectives, it may also include dismantling disciplinary perspectives rather than integrating them.
According to Gibbons et al. (1994), transdisciplinarity takes interdisciplinarity a step further. Whereas, interdisciplinary approaches explicitly formulate uniform discipline-transcending vocabulary or propose common methodologies, a transdisciplinary approach takes a common theoretical understanding and succeeds in integrating it into both participating disciplinary epistemologies. If enough researchers join in this effort, one could begin to refer to a new transdisciplinary field with a homogenized theory or set of models (e.g., social psychology or psycholinguistics). In other work, transdisciplinarity takes on a broader meaning. A transdisciplinary orientation works to overcome the disconnection between knowledge production on the one hand, and the demand for knowledge to contribute to the solution of persistent, complex, societal problems on the other hand (Jäger, 2007). For Hall et al. (2012), a hallmark of transdisciplinary research is its focus on advancing progress toward practical solutions to social problems - for example, translating research findings into practice and policy applications. This latter view of transdisciplinarity is at the heart of action-research in education in that researchers, teachers, and policy-makers need to work together to address how to organize education for the developing child, adolescent, and young adult, but also for life-long learners. Such organizational attempts are carried out in particular socio-cultural contexts and will vary across countries, and involve a variety of stakeholders, often illustrating differences in underlying value systems. Is learning a pleasure or is it equated with effort and pain? Why do teachers get high salaries in some countries and low salaries in others? Disentangling the forces that enter into tension in such complex problem solving is a major challenge for policy making around teaching and learning.
Our position is that research questions may be investigated through a set of theoretical and methodological lenses that stem from different disciplines but also from different frameworks within the same discipline, which is to say that even within a discipline, there can be epistemological diversity. These lenses are heavily influenced by the vantage point from which the phenomenon under study is measured (Longino, 2013). In discussing our results, we will assimilate the notion of “Scopus subject area” to the notion of discipline (see below).
Challenges for Research in Education, Given Its Broad Scope
The third research question asks what the challenges are that research in education faces, given its well-known diversity in assumptions, theories, objectives, and methods. No matter the research domain, there are general challenges to be met that occur in multi, inter, and transdisciplinary research. However, there are also specific challenges having to do with the nature of the precise frontiers between research in education and research in the other social sciences and humanities. Both are useful in defining the challenges that face researchers in education.
Meeting the Challenges of the Diversity of Research in Education
The fourth research question is designed to investigate a way for the academic communities involved to move forward with broader education-related collective knowledge advancement, given the challenges of diversity. Such advancement can be guided with a model that mobilizes multiple theories that come from different disciplines, according to analytic need and questions posed. Each theory allows for observations that are oriented in particular ways and methods of interpretation that are based on specific assumptions, but there needs to be a way for them to be considered in concert. In addition, there should be a way of exploring how different fields may be posing similar questions that could be combined for a broader view of the phenomenon under study.
In what follows, we present the method, including database selection and extraction, the notion of bibliographic coupling and how it constructs the network clusters in education and social sciences research. The extent to which two articles are related by virtue of them both referencing the same research is the basis for our analysis. The nature of the shared reference (e.g., theory, method, etc.) determines the nature of the relation. Next, we present the results that allow us to discuss the above research questions. We end with a conclusion, a presentation of the limitation of our work, and ideas about future perspectives.
Materials and Methods
Database Selection and Extraction
There are a number of electronic databases that index academic publications. They all provide wide coverage of academic publications and are practical to use. We chose to use Scopus. It has a similar overall coverage as the Web of Science, but a slightly wider coverage of non-English journals. That said, it remains unfortunate that so much research in other languages is not part of these large databases. As automatic translation progresses, hopefully the scope of the research world can be widened. Publication sources in Scopus are classified under four broad subject categories (Life Sciences, Physical Sciences, Health Sciences and Social Sciences & Humanities). These are further divided into 27 subject areas. The Scopus web interface does not provide a subject category corresponding to educational research. In order to select a corpus of publications in educational research, we relied on a list of educational research publication sources provided by AERES (AERES, 2014), the French national agency for the evaluation of research and higher education, which was in turn based on the European Educational Research Quality Indicator2.
Figure 1A shows the evolution of the number of documents and corresponding number of journals in the AERES Education journal list being indexed by Scopus, since 1980. Scopus is known to focus mainly on recent articles (published after 1995), which explains the discontinuity observed in the figure. The increase in the number of indexed documents from 1996 to 2014, notably after 2005, is mainly due to the evolution of the number of journals coming into existence and being indexed. In this paper, we chose to compare two periods. The first is from 2000 to 2004 in order to get a sense of the research clusters before the steady increase of publications from 2005 (cf. Figure 1A). Choosing a time period before this initial increase also gave us a more manageable task. The second period is 2015–2018. Figure 1B shows the evolution of the number of documents within the Scopus “Social Sciences” broad category during the same period of 1995 to 2015. Similar to the “Education” list, we observe here a steady increase of the number of documents being indexed. This is also due to the evolution of journals coming into existence and being indexed by Scopus. In this paper, we chose to study the corpus of “Social Sciences” documents published in 2000. This snapshot allowed us to study the position of research in education within the whole field of social sciences while keeping the number of bibliographic records to extract from Scopus reasonable.
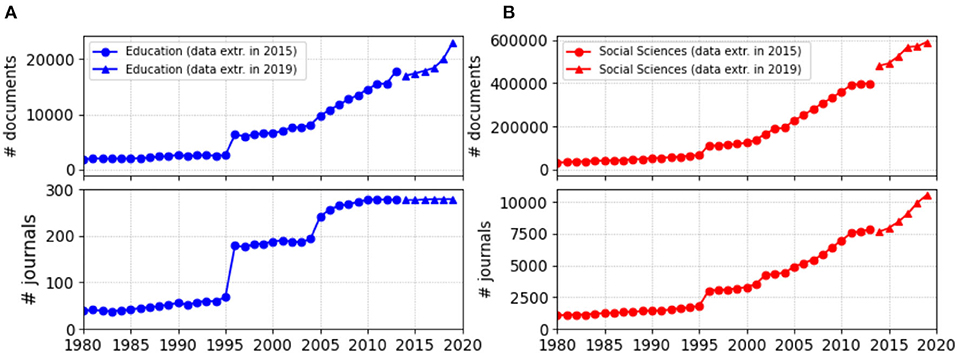
Figure 1. (A) Evolution of the number of documents and corresponding number of journals in the AERES Education journal list being indexed by Scopus, since 1980 and (B) evolution of the number of documents within the Scopus “Social Sciences and Humanities” broad category.
For the three corpora studied in this paper (on the one hand, for the two periods of the Education corpus and on the other hand, for the Social Sciences and Humanities corpus3, we saved the full records of the documents: authors, names of publication source, years of publication, publication titles, keywords (given by the authors and/or Scopus), and the lists of references included in the publications. From the references lists, we additionally extracted authors, year of publication, and title of the reference. References were not always formatted consistently throughout all the records in Scopus (e.g., use of different abbreviations and/or inclusion of subtitles) and/or included missing information (e.g., final publication year of pre-print articles). All in all, around 2% of the references were wrongly formatted in the Education corpora and it was 6% in the Social Sciences.
In total, the extracted Education corpus 1 contains 36,715 bibliographic records from 2000 to 2004, the extracted Education corpus 2 contains 75,037 bibliographic records from 2015 to 2018 and the Social Sciences and Humanities corpus 122,936 records from 2000 (5.1% of which also belong to Education corpus 1). Publications in the Education corpora (as follows % for Education corpus 1, % for Education corpus 2) are mainly written in English (94/92.5%) by authors from the United States (44/35%) followed by the United Kingdom (14/12%), and Australia (5/7%). Publications in the Social Sciences and Humanities corpus are also written mainly in English (92%) by authors from the United States (33%) followed by the United Kingdom (14%), and Germany (4%). Tables 1, 2 list the publication sources that contribute the most articles to Education corpus 1 & 2. It illustrates that Scopus indexes peer-reviewed journals as well as professional magazines (such as Phi Delta Kappan).
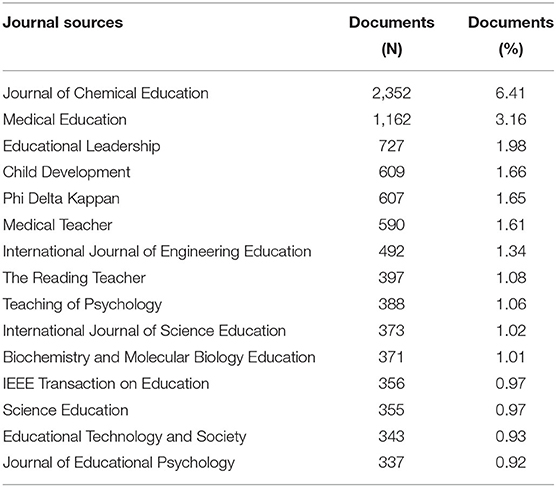
Table 1. Major contributing publication sources in Education corpus 1 and Social Sciences and Humanities corpus.
The reader should note that these are the major contributing publication sources so these are the journals in which appear the most publications in our corpus. The list of education journals was much broader in scope.
There are a number of observations to make regarding how these contributing publication sources have changed between the periods 2000–2004 and 2015–2018. The Journal of Chemical Education still leads and Medical Education and Medical Teacher (Teaching) remain strong. But most strikingly, research involving computers has gained much ground. In the first period, there were no journals that dealt with education and computers by name (but see Educational Technology and Society) whereas in the latter period the second most contributing journal is Computers in Human Behavior and Computers & Education is in the top five. Research in Developmental Disabilities has also gained in visibility; it was not previously present and it is now the third most contributing journal. Child Development has subsequently dropped and specific journals on psychology have also disappeared (Teaching of Psychology, Journal of Educational Psychology). The professional magazine Phi Delta Kappan, focused on discussions of research, policy, and practice in K-12 education remains a steady contributor. Finally, perhaps research on more foundational knowledge (The Reading Teacher, International Journal of Science Education, Biochemistry and Molecular Biology Education) have given way to transversal concerns (learning and individual differences), as well as methodological orientations (Quality & Quantity).
Bibliographic Coupling
Scientometrics is a well-established field that applies mathematical methods to academic publications in order to understand science organization and evolution (see Mingers and Leydesdorff, 2015 for a review). It can be used simply to describe a field and its boundaries or it can be used as a first step in understanding the nature of research in a particular domain. It capitalizes on the citations by scientists to detect linkages between different articles, leading to the bottom-up building of homogeneous scientific subfields by clustering methods to be detailed below. Two of us have been working on scientometrics for several years, building a variety of tools to map scientific institutions (Grauwin and Jensen, 2011), the field of complex systems (Grauwin et al., 2012), or assess the interdisciplinarity of several hundred French laboratories Jensen and Lutkouskaya (2014).
Here, we use the well-known bibliographic coupling approach (BC; Kessler, 1963) to create a network, using articles as nodes and their common references as links. As we have argued elsewhere (Grauwin et al., 2012), BC achieves a faithful representation of the fields, giving equal weight to all published articles, regardless of whether and how often they are cited. Moreover, the links are established on the basis of the author's own decisions (to include or not to include a given reference) rather than retrospectively from other scientists' citations, as in the popular co-citation approach (Small, 1973). Thus, bibliographic coupling can be used to analyze the research clusters as they are built by researchers themselves.
Bibliographic Coupling and Construction of Network Clusters
In order to determine how different articles are linked through common references, we systematically compared the reference lists of two publications and identified shared references. Articles are linked if they share at least two references, leading to a network of articles connected to each other. The resulting network is schematically represented in Figure 2A where nodes represent individual articles. The thicker the link, the more references are shared between the articles. The links are weighted by Kessler's (1963) cosine similarity
where Ri is the set of references of article i. By definition, the cosine similarity is equal to zero when two articles do not share any reference and is equal to 1 when their sets of references are identical.
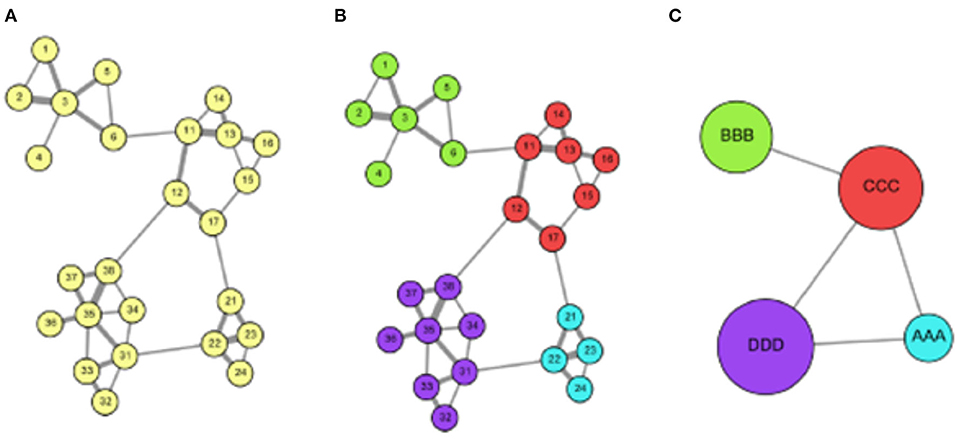
Figure 2. Cluster detection. (A) Nodes are individual papers. (B) Colored nodes represent emerging themes of clusters of papers. (C) Finalized clusters of papers with placeholder title names.
Clusters are then detected using modularity maximization (Newman and Girvan, 2004) and the fast Louvain Algorithm (Blondel et al., 2008). Modularity quantifies the possibility to split a network into clusters in such a way that the links between nodes (i.e., articles) are dense inside clusters but not between them. There are many techniques available for clustering the nodes of a graph into relevant “communities” (for a review, see Fortunato, 2010). Thanks to its conceptual simplicity and easiness of computation, modularity is by far the most popular, even if its results should be interpreted with care (Good et al., 2010). In previous work on similar bibliometrics networks (Grauwin and Jensen, 2011; Grauwin et al., 2012), we have shown that the clusters obtained by modularity maximization do represent the scientific structure of research in a meaningful way.
An example of the resulting cluster membership of each node is represented in different colors in Figure 2B. Note that articles belonging to the same cluster (e.g., node 1 and 5 or node 12 and 15) are not always linked directly. Note also that articles belonging to different clusters may share links as well. The Louvain method detects clusters of nodes so that the number of “external” connections is as small as possible. In the networks studied in this paper, more than 70% of the links of an article are with articles belonging to the same cluster.
Clusters titles (Figure 2C shows placeholder names) were initially generated automatically as a function of frequent and significant title and keywords of their articles. This sometimes led to duplicate labels for the clusters, given shared overlap in research focus in educational research in general (e.g., two clusters with a “child” label). The final labels of the education network were determined after checking them against the references and other aspects of the clusters so that the labels would uniquely represent the clusters. Automatically generated labels were kept for social sciences and humanities clusters as they were distinct enough due to the diversity of research in social sciences and humanities research at large.
Cluster Cohesiveness as an Indicator of Cluster Focus
In Figure 2, the thickness of an edge between two clusters is proportional to the extent to which they share the same references, that is, the average weight between articles of both clusters. Note that this link arises mostly from shared references that are not within the clusters' core references. Summing up the weight of all edges of the cluster pairs, one obtains the weighted degree of a cluster, a “centrality” measure in the language of network theory, which indicates the overall connectivity of the cluster (see Tables 3, 4 in the results section). Clusters with a high W value share more references with other clusters than clusters with low W values, which use more specialized references. The layout algorithm tends to position highly connected clusters at the center of the map and less connected clusters on the periphery of the map4.
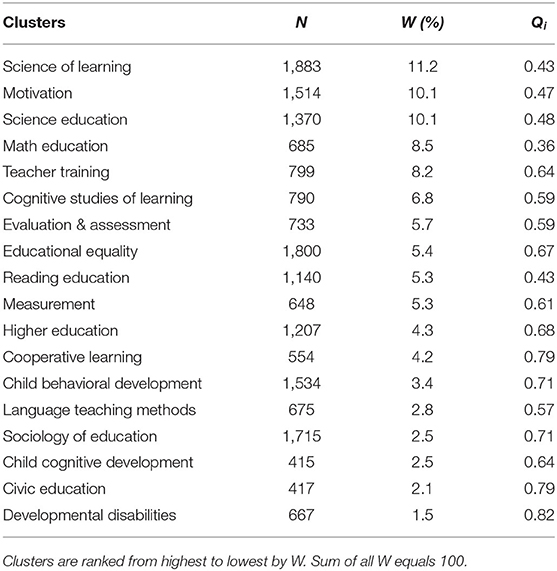
Table 3. Education corpus 1 cluster sizes (N), Normalized Weighted Degree (W) and Internal Modularity (Qi).
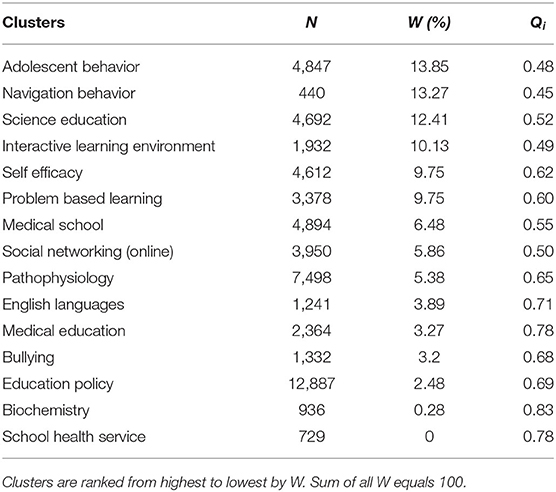
Table 4. Education corpus 1 cluster sizes (N), Normalized Weighted Degree (W) and Internal Modularity (Qi).
It follows then that clusters can vary in size and cohesiveness, that is, they can vary in the extent to which articles are connected to one another within the same cluster. In cohesive clusters, articles are highly connected to each other around a set of core references. In less cohesive clusters, the connections are not as strong and may not be homogeneously distributed so that further breakdown into sub-clusters can be done if needed. The relevance of such a sub-partition can be measured by an internal modularity measure Q varying between−1 and 1, where values above 0.4 are often considered as an indication of a relevant sub-partition.
Results
In reporting these results, we are working from the assumption that these clusters correspond to the different areas of educational research, given that articles in the same cluster share at least two references with another paper of that cluster. We first show through cluster cohesiveness how clusters in Education corpus 1 and Education corpus 2 focus either specifically on education or can be broken down into other clusters. In other words, one cluster will focus on education, but others will focus on other subjects that are aligned with the social sciences and/or humanities disciplinary focus of the cluster in question (cf. section Education clusters focus either clearly on education or in addition to other topics). We also point out how these clusters have evolved between the periods of 2000–2004 and 2015–2018, yet maintain their interdisciplinary footprint. Then, we show how a similar phenomenon plays out in the Social Sciences and Humanities corpus from 2000 where clusters that focus on education appear in different Scopus subject area regions of the map (section Overall Structure of Educational Research Within Social Sciences and Humanities). These two sections answer our first research question by illustrating the links that research in education has to research in social sciences and humanities, first from the bottom-up perspective of the cohesiveness and focus of clusters labeled as education with our bibliographic method, and then from the top-down perspective of in which Scopus subject area space such clusters are located within the larger social sciences and humanities.
In section Educational Research in Social Science Research at Large: A Deep Multidisciplinary Interface, we address our second research question and report on how education research compares to the disciplinary composition of other fields, illustrating its deep multidisciplinary interface. In section The Challenges that Face Researchers in Education we evoke the challenges of such multidisciplinarity and we propose a framework for meeting them.
Education Clusters Focus Either Clearly on Education or in Addition to Other Topics
Table 3 shows the cluster sizes (N), the normalized weighted degree (W) and the internal modularity (Qi) of each education cluster. The first clusters in Table 3 are highly cohesive and clustered around a set of core references. These core references reveal an education focus, once they are analyzed. The last clusters in Table 3 are less cohesive and clustered around multiple sets of core references. This means they have multiple foci having to do with multiple subject areas, one of which, at the very least, is education related. Clusters are designated here by their most significant keywords among the 20 most frequent ones (cf the online interactive map http://sebastian-grauwin.com/XYZ_EDUCMAP/BCclusters.html).
A closer look at the top five highly connected, cohesive and bottom five loosely connected clusters in Table 1 allow us to delve more deeply into the first research question: what is the place of educational research in the larger context of social science research? If we explore the top five highly connected, cohesive clusters from Table 3 (i.e., “Science of Learning,” “Motivation,” “Science Education,” “Math Education,” and “Teacher Training”), they appear to focus on teaching and learning directly. This can be in terms of theoretical approaches to education (e.g., socio-constructivist view), of teacher and student attitudes and identity, or practices in the classroom. The focus can also be more specific, on math and science teaching and learning practices. These clusters tend to be organized around references that connect the whole network (e.g., Lund et al., 2015, 2017). On the other hand, the bottom five loosely connected clusters (i.e., “Language Teaching Methods,” “Sociology of Education,” “Child Cognitive Development,” “Civic Education,” and “Developmental Disabilities”) deal only partially with teaching and learning practices. Their main focus is on different fields (i.e., linguistics, sociology, psychology) and education is only one object of inquiry, one example of application among others.
Some specific examples may help to understand why some clusters concentrate only peripherally on education. In the sub topic cluster “Developmental Disabilities,” most sub topic clusters are tied to the medical or psychology domain, as seen for example by their high use of the DSM manual (i.e., the Diagnostic and Statistical Manual of Mental Disorders). The sole strong connection to education flows through a psychology sub topic cluster, as a possible application of their more general work on disabilities. A similar phenomenon can be seen for the “Child Cognitive Development” cluster. Only a single sub-cluster is strongly connected to education, with articles focused specifically on the impact of child cognitive development on education. Similarly, education is but one example of a political phenomenon for the “Sociology of Education” sub topic cluster, which deals with general sociological matters as globalization, or colonialism. Take as an example the title of one of its most connected articles: “Re-thinking trust in a performative culture: the case of education” (Avis, 2003). Finally, despite what its name might imply, the “Language Teaching Methods” sub topic cluster does not solely focus on education either. Some research focuses on language policy and the politics of development or more specifically linguistic topics such as syntactic complexity in synchronous and asynchronous communication. Therefore, whereas the highly connected, cohesive sub topic clusters are more focused specifically on learning and teaching per se, at times in specific topic areas that make sense to the sub topic cluster (e.g., the science education sub topic cluster will focus on physics learning), the loosely connected, less cohesive sub topic clusters are recognized as focusing more on disciplines in their own right (e.g., the sociology of education sub topic cluster deals with general sociology in a variety of ways), in which there are links to many different foci of research, one or more of which happen to be education related.
This phenomenon of cohesiveness is repeated at the level of the social sciences and humanities corpus (see next section). In other words, the articles which deal with education in this larger corpus network are loosely connected together, yet span different sub topic clusters that each belong to a set of different Scopus subject areas. This characteristic will be further explored in section Educational Research in Social Science Research at Large: A Deep Multidisciplinary Interface.
Before we turn to examining the overall structure of educational research within social sciences and humanities, let's review the top five highly connected, cohesive and bottom five loosely connected clusters in Table 4. This will allow us to compare the Education corpus clusters from 2000 to 2004 to the clusters from 2015 to 2018 and give evidence for how the place of educational research in the larger context of social science research may have evolved. First, it's striking how much the landscape has changed. Science education is the only cluster remaining from the period 2000–2004. It is still a highly connected and cohesive cluster, and research here has continued to have this characteristic for almost 20 years. This aside, Table 4 is not as clear cut as Table 3 where the highly connected, and cohesive clusters were focused on learning and teaching. Here, apart from the third cluster (“Science Education,”) “Adolescent Behavior,” “Navigation Behavior,” “Interactive Learning Environment,” and “Self Efficacy” focus rather on behavioral and contextual aspects of learning. In addition, the sources referenced are quite multidisciplinary, connecting also to psychology for Adolescent Behavior, and Navigation Behavior. This latter also connects to the field of education sciences through sources that are highly influenced by psychology (Educational Psychologist, Learning and Instruction, Cognition and Instruction, and Instructional Science). Unsurprisingly, the “Interactive Learning Environment” cluster's most frequent references' sources include journals from computer science, psychology, and education. And looking to the loosely connected clusters (“Bullying,” “Education Policy,” “Biochemistry,” and “School Health Service”), only “Biochemistry” stands out as focused on teaching and learning of that subject, whereas the others connect to other disciplines that treat the topic in question from different perspectives (e.g., “School Health Service” is examined by pediatricians, but also through public health and behavioral psychology; “Bullying” is the focus of child development, but also studied through the lens of child psychology and psychiatry, through disability research, and also by taking into account aggression and adolescent health).
In sum, the latter period of research in education (2015–2018) exhibits a more multidisciplinarity approach for a given topic, whether or not the clusters are highly connected and cohesive or loosely connected. We could dare to hypothesize that research in this area is slowly accomplishing more and more integration across frameworks and approaches.
Overall Structure of Educational Research Within Social Sciences and Humanities
We can further deconstruct the notion of “place” of educational research in the larger context of social sciences and humanities research if we begin by illustrating the top-down characterization that Scopus proposes through its categorization of articles by Scopus subject area. The cluster map in Figure 3 was formed by gathering all “social science and humanities” articles published in year 2000 from the Scopus database. This yielded 122,936 articles, and the colors signify the Scopus subject areas in which the journals publishing articles are categorized. We performed our cluster detection analysis on these articles, which allowed us to combine our bottom-up approach of bibliometric coupling to the top-down approach subject area categorization of Scopus. But we need to figure out where research in education is situated, within the network. There are five major regions which we can characterize according to how Scopus puts publication sources into subject areas: neuroscience (top right, orange), psychology (also orange, but in addition green, pink, middle red and brown), general social science/sociology (light blue, in the middle/right), business and management (yellow) and economics and finance (bottom, pink/purple)5.
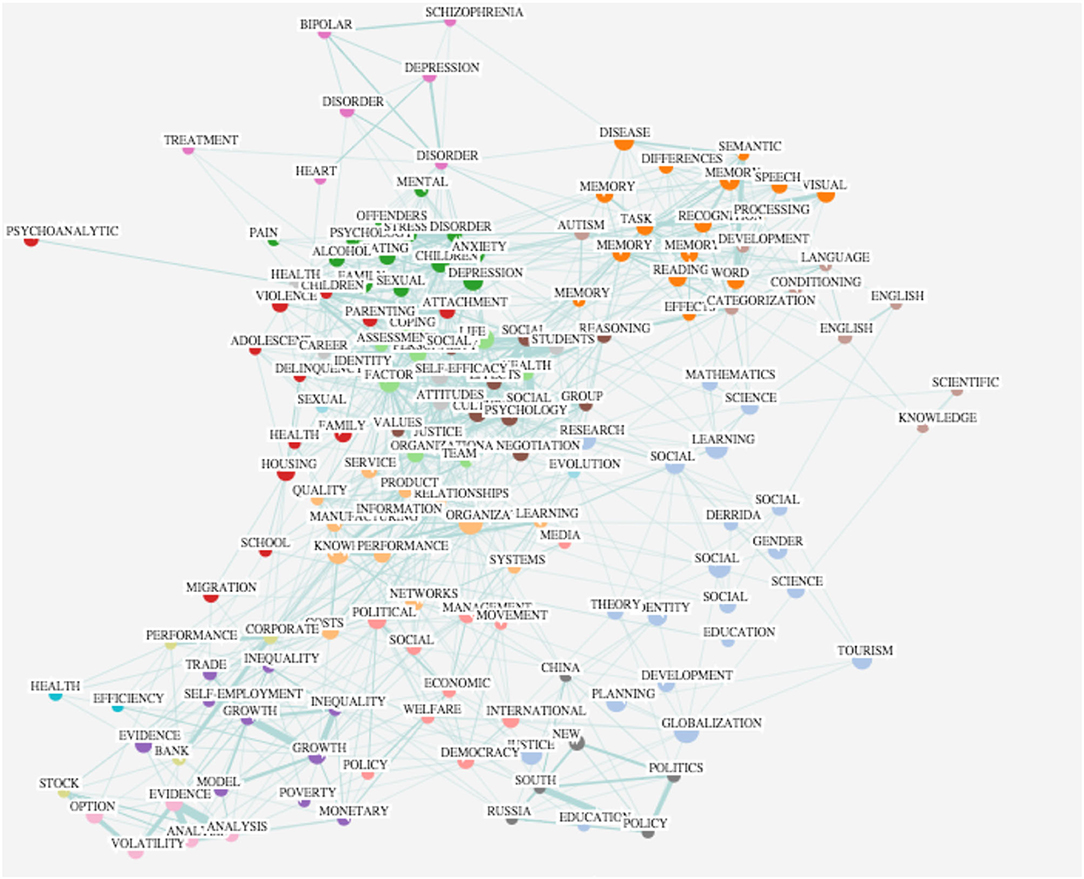
Figure 3. Social Sciences and Humanities network sub topic clusters (in colors) obtained by the partitioning algorithm on the bibliographic network built on around 123,000 articles published in year 2000 in the Scopus “Social sciences and humanities” broad category. The area of the nodes corresponds to the number of articles of that cluster and the colors to the corresponding topic clusters, each of which would subsume all of colored sub topic clusters into one colored cluster. The labels correspond to the most common title keyword of the source publication. A few sub topic clusters, disconnected from the main network, containing mostly biology articles from Nature, Science and Proceedings of the National Academy of Sciences (PNAS), are not shown.
Education does not automatically appear as a single region with its own specific color, because Scopus does not have a subject area for education (see the materials and methods section where we explain how AERES and EERQI help us create this subject area). This paper can be viewed as an attempt to answer why Scopus might not have such a subject area called education. In order understand the extent to which research in education was present in the Social Sciences and Humanities corpus, we examined the relative proportion of the articles from the “education journals” database in each of the clusters of Figure 3; this is plotted in Figure 4 on a red scale. To simplify the analysis, we restricted it to the social science and humanities clusters that contain more than 100 articles.
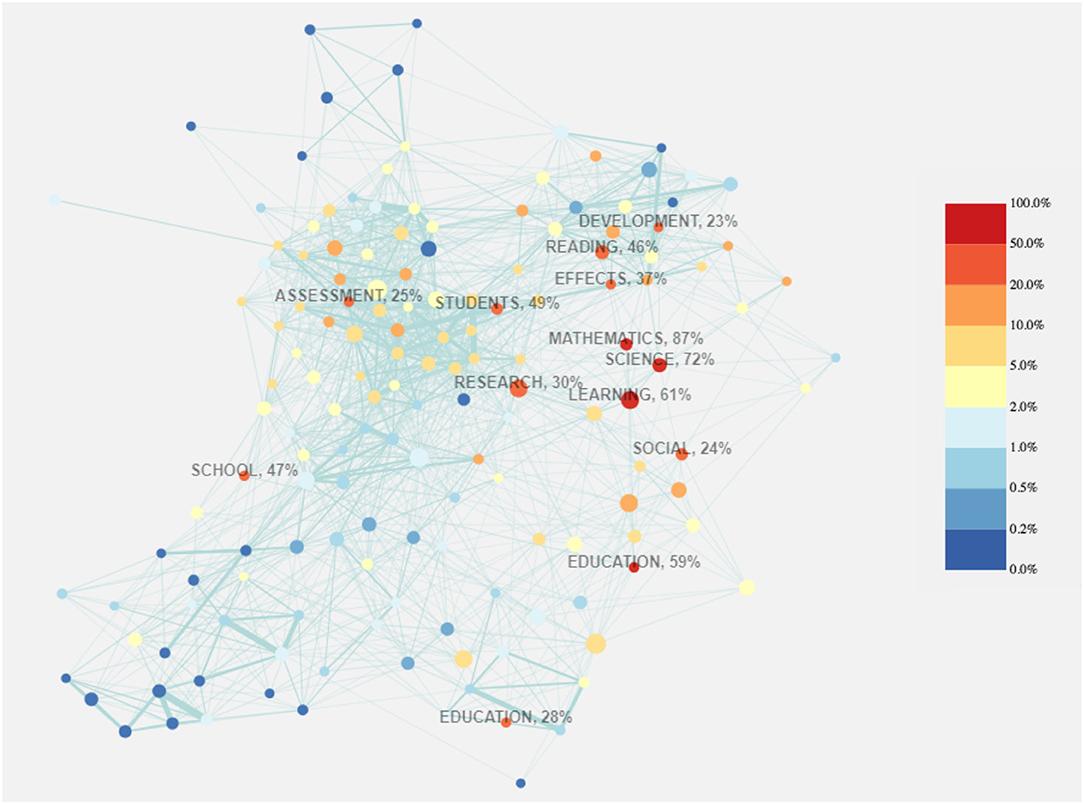
Figure 4. Social Sciences and Humanities clusters (Figure 3) according to the proportion of articles of each sub top cluster that are also found in the Education corpus. The closer to red, the higher the proportion, the closer to blue, the lower. Four sub topic clusters contain more than half of the articles from education: “mathematics” (87%), “science” (72%), “learning” (61%), and “education” (59%).
Results revealed four major and nine minor education sub topic clusters within the social sciences and humanities map (labeled and shown in red in Figure 4). If education had been a subject area that was well-defined in relation to the boundaries it shared with other Scopus subject areas, we could have expected there to be a clearly defined region. Instead, education is distributed over many different regions, which points to a loosely connected field with multiple areas of study connecting to different subfields of social sciences and humanities, corresponding in nature to the bottom clusters of Table 2, within Education corpus 1 and Education corpus 2.
The four major sub topic clusters we see in Figure 4 refer to social sciences and humanities clusters in which more than 50% of the articles are published in education journals. These are the sub topic clusters labeled “Mathematics” (87% of articles published in education journals), “Science” (72%), “Learning” (61%) and the larger “Education” sub topic cluster at 59%. Given their high rate of publication in journals that are labeled as education journals, these four sub topic clusters have a strong education coloring.
It is noteworthy that overall, the highlighted sub topic clusters that tend toward red in the social sciences and humanities map can be matched to a single Education corpus 1 sub topic cluster in Table 3, the “Science Education” sub topic cluster. Seventy-two percentage of the articles within the social sciences and humanities sub topic cluster “Science” are published in a journal in our list of education source publications. And among these, 80% can be found in the “Science Education” sub topic cluster of Table 3 while the rest are distributed among other sub topic clusters. This observation shows that Scopus' top-down method of categorizing articles according to the Scopus subject area of the journal in which they are published and our bottom-up method of clustering articles together if they share references can triangulate. They both show a focus on Science in the year 2000 that come together in the respective analyses6.
The nine minor education sub topic clusters in Figure 4 refer to clusters containing between 20 and 50% of education articles and reach toward other Scopus subject areas in terms of the nature of references of articles that are shared. They therefore have a weaker education coloring. There are three sub topic clusters within the orange neuroscience nodes of Figure 3 (“Reading” 46%, “Effects” 37%, and “Development” 23%), four within the green, pink, brown, and red nodes of psychology (“Students” 49% “Research” 30%, “School” 47%, and “Assessment” 25%) and two within the light blue nodes of general social science and sociology (“Social” 24% and the smaller “Education” cluster at 28%).
Educational Research in Social Science Research at Large: A Deep Multidisciplinary Interface
In this section, we set out to answer our second research question:
How does educational research compare to the disciplinary composition of other subject areas?
As can be seen in Figure 4, education clusters are scattered over a wide region of the map, suggesting that educational research is carried out in close connection with a number of other research areas in social sciences and humanities research, rather than forming an isolated region. This scattering of education clusters over multiple disciplinary areas of the Social Sciences and Humanities corpus suggests that research in education is quite multi-disciplinary and wide-reaching, in that different intellectual traditions and areas of study coexist in a loosely connected fashion. In what follows, we quantify this interpretation in three ways. First, we compute the extent to which articles are connected via references to articles of the same Scopus subject area. We use the Scopus subject areas of the journals where the articles are published and remind the reader that journals may belong to several Scopus subject areas. The main Scopus subject areas used here are: “Business, Management, and Accounting,” “Decision Sciences,” “Economics, Econometrics and Finance,” “Medicine,” “Psychology.” We add “Education” for those articles published in the list of education journals we built, for as we have mentioned, the Education subject area does not exist in Scopus. The results confirm that articles having to do with education are more likely to connect to articles in other Scopus subject areas than articles of other Scopus subject areas. For example, “Education” articles are equally likely to be linked to articles belonging to Education and to articles belonging to Psychology (39% of the links for both Scopus subject areas). And on the contrary, “Economics” articles are mostly linked to other Economics articles (60%).
A second way is to look at the sub topic cluster level. We qualify whether these sub topic clusters gather articles from different Scopus subject areas or not. The multidisciplinary indicator M (d1, d2) quantifies the co-presence of two Scopus subject areas d1 and d2 within sub topic clusters. By extension, one can define a single Scopus subject area indicator M (d, d) to quantify the proportion of articles of a single Scopus subject area d within the clusters. Formally:
where fi (d1) represents the percentage of articles of Scopus subject area d1 within sub topic cluster i and sub topic size i its size. This indicator computes the proportion of articles of Scopus subject area 2 for the clusters where there are articles of Scopus subject area 1 [i.e., fi (d1) is not 0], weighting the contributions by the number of articles of d1 [the factor fi (d1) * size i]. In other words, our indicator quantifies whether articles from different Scopus subject areas share many references with articles from other Scopus subject areas, which would indicate commonalities. Note that since many articles are attributed by Scopus to several Scopus subject areas, the sum of the frequencies of the different Scopus subject areas is not exactly equal to 1. Note that this indicator is independent of the number of articles of the Scopus subject area.
One obtains the following single-subject area relations: M (Education, Education) = 0.34; M (Economics, Economics) = 0.52; M (Psychology, Psychology) = 0.65; M (Business, Business) = 0.44; M (Decision, Decision) = 0.16 (see Figure 5). The value is the highest in psychology followed by economics, indicating that the psychology and economics sub topic clusters tend to be mono-disciplinary, i.e., articles from these Scopus subject areas share references mostly with articles from the same Scopus subject areas. On the other hand, education articles belong to social science and humanities sub topic clusters that contain only a third of articles from education. Similarly, “Decision sciences” articles belong to sub topic clusters that contain only a quarter of articles from Decision Sciences, showing that “Decision sciences” articles are more connected to articles belonging to Business and Economics Scopus subject areas.
From which Scopus subject areas do the rest of the articles concerning education come? As can be seen in Figure 5, the two most important multi-disciplinary indicators for Education are: M (Education, Psychology) = 0.35 and M (Education, Medicine) = 0.13. This means that, on average, education articles belong to clusters that contain, beyond the third of education articles, a third of Psychology articles and 13% of articles belonging to Medicine. In other words, Education articles share many references with articles of these Scopus subject areas, which indicates commonalities. These results suggest that Education as well as Decision sciences, instead of forming a single, well-delineated closed discipline, tend to form a loosely-connected and distributed discipline that actively incorporates diverse knowledge bases in social sciences and humanities fields.
The third way of confirming the multidisciplinary character of Education uses the clustering coefficient (cc) to quantify the disciplinary breadth to which articles are linked. This standard characterization of the nodes of a graph is whether the neighborhood of a node is tightly connected, that is, whether an article connects articles that are already connected or whether it connects otherwise unconnected articles. Formally, it is defined as the ratio of the number of links among the neighbors of a node to the number of possible links, that is, d*(d-1)/2 where d is the degree of the node. For a given article, cc = 0 means that there is no connection (no common references) among its neighbors, while cc = 1 means that all the articles connected to it are also connected among themselves. In most social networks (Newman, 2010), average cc values are around 0.2–0.5, meaning that there is a high probability that two of my “acquaintances” also know each other. In our network, cc corresponds to the proportion of articles in the neighborhood of an article that share more than two references. One can expect that cohesive subject areas show high values of cc, since many articles share similar references, while subject areas that connect distinct thematic areas are likely to have low cc values, as articles in those distant subject areas are not often connected.
We have computed the cc for all the articles in the social sciences and humanities network. The average cc is 0.29, while that of articles published in Economics journals is significantly higher (0.32, p < 0.001), and that of articles published in Education journals is significantly lower (0.28, p < 0.02). This means that the neighbors of articles in Economics also share references between each other whereas the neighbors of articles in Education do not necessarily share references between each other, and may share references with distant articles, in terms of Scopus subject area. We note that the Scopus subject area with the highest cc is Psychology (0.34, p < 0.001) which means that an article in Psychology has the most neighbors who share references. The same result is found when looking at the cc at the level of the journals, where nodes are entire journals that are linked whenever they contain articles sharing at least 2 references. In this aggregated network, the average cc is 0.38, Economics journals have an average cc of 0.41 (p < 0.001), Psychology journals have an average cc of 0.42 (p < 0.001), while Education journals are below average (0.36, p < 0.01). The same result is obtained when averaging cc for the articles in the different clusters of Figure 4: clusters that contain mostly Psychology articles show a larger average cc than those in Economics and Education.
In summary, education related research is widely scattered across research in social sciences and humanities, sharing references with different subject areas. First, articles having to do with education are more likely to connect to articles in other Scopus subject areas (in particular, psychology) than articles of other Scopus subject areas. Secondly, in qualifying whether sub topic clusters gather articles from different Scopus subject areas or not, we see that Education first gathers articles essentially equally from both Education and Psychology and then next from Medicine. Thirdly, in quantifying the disciplinary breadth to which articles are linked, we see that Psychology has the least breadth, but the most cohesiveness whereas Education has the most breadth and the least cohesiveness. In conclusion, Education is the most multidisciplinary.
The Challenges That Face Researchers in Education
Given that we have empirically shown the cross-disciplinary connections between research and education and social sciences and humanities, in this section we discuss our third research question, which arises naturally from this state of affairs:
3. What can the disciplinary composition of the subject area of Education tell us about the challenges facing educational research?
Earlier in the paper, we presented the different types of cross-disciplinary relations, including multi, inter, and transdisciplinarity. In what follows, we discuss both the drawbacks of working across disciplines, and the reasons for venturing outside of disciplinary boundaries (Lund, 2016). Each of these are first presented from a general point of view and then specifically from the point of view of education related research. In this paper, we will argue in favor of working across disciplines in ways that are adapted to the objectives for and the context of education research. We will propose a model in response to our fourth research question that deals with meeting the challenges that we detail below.
Drawbacks of Working Across Disciplines
Klein (1990) notes three major difficulties facing interdisciplinary scholarship: general uncertainly over definition, lack of professional identity and dispersion of discourse. First, is interdisciplinarity just nostalgia for a lost wholeness or is it a new stage in the evolution of science? Is it a historical quest for unified knowledge or is its goal to develop the frontiers of knowledge? Second, some proponents of such scholarship are wary of organizing professional interdisciplinary movements because institutionalization may bring about insularity, and avoiding insularity was one of main reasons they were attracted to interdisciplinarity in the first place. Third, the discourse on interdisciplinarity is widely dispersed and so commonalities that could be shared are simply not available for those who could benefit. That said, the explorable on-line maps that we provide are meant to remediate this.
One of the more practical difficulties of working across disciplines is the time it takes to perform the intellectual work necessary to consider their compatibility or their incommensurability (Latour, 2005). It may be more efficient to stay within the boundaries of a discipline where the frameworks are well-defined and where the type of maneuvering is well-understood. Kuhn (1962/1970) called it doing “normal science” where details are slowly accumulated in accordance with an established broad theory and where there is no need to question or challenge the underlying assumptions of that theory. In this way, a researcher's energy can be put toward reaching specific disciplinary scientific objectives. It's difficult to use a framework in an effective way while you are questioning it.
Another drawback of working across disciplines is difficulty in framing research to be published so that it both fits the aims and scope of existing journals and so that it does not fall victim to be found lacking in one way from one disciplinary perspective and in another way from another disciplinary perspective.
Reasons for Venturing Outside of Disciplinary Boundaries
Klein (1990) notes a wide range of objectives that educators, researchers and practitioners have pursued through interdisciplinary work: answer complex questions, address broad issues, explore disciplinary and professional relations, solve problems that are beyond the scope of any one discipline, and achieve unity of knowledge, whether on a limited or grand scale.
In addition, communities of researchers may work separately on seemingly similar objects of study while ignoring each other's efforts. Asking how the efforts of others can relate to one's own efforts is another reason for venturing into another discipline's territory. In fact, the key word here is “seemingly.” Once researchers gather data and make explicit their purpose of analysis and methods, it becomes clear that what is considered the same phenomenon is in fact very different. Although the phenomenon in question may seem to be the same at a general level (e.g., group interactions), it often is not the same phenomenon once the data has been gathered and the purpose of analysis is made explicit. For example, experimental psychologists will typically gather data on group interactions in controlled laboratory situations that are specifically designed to test a hypothesis concerning how a variable affects either group process or outcome whereas interactional linguists will more likely gather data on group interactions in naturally occurring situations with the goal of describing the ways that participants co-organize their actions. At first glance, it may not seem obvious what these psychologists and linguists would say to each other.
But are they missing opportunities for the advancement of scientific knowledge on group interactions by staying anchored in their respective communities? How is it different to do research within one discipline vs. in a way that reaches across disciplines? Could the latter be more productive or at least provide new opportunities for innovative research questions? If the group interactions that are in question have learning as a process as their goal, there are indeed ways that psychologists and linguists can bring their unique focus to bear on this question. The former can pinpoint which variables have effects on process in particular situations and the latter can describe in detail how these effects are manifested and co-constructed in the process. Such an approach gives a broader and richer view of the data.
Meeting the Challenges That Arise From the Diversity of Research in Education
Given the nature of research in education and how it is strongly connected to research in social sciences and humanities, and also given the various challenges that this situation provokes for the researcher, in this section, we discuss our fourth research question:
4. What kind of model for broader education-related collective knowledge advancement can meet these challenges?
So far, we've seen that research in education is carried out from within different institutional vantage points that may or may not have particular advantages. Different disciplinary viewpoints are taken on the same object, different theoretical frameworks are mobilized, data of different natures are focused on, and a variety of methodological approaches are used. Even if such diversity makes for fascinating research, it also brings about missed opportunities.
We begin by giving five examples of missed opportunities involving communication between researchers in fields relating to research in education and then we present a model that is supplemented with the exploration of our Education and Social Sciences and Humanities corpora. The combination of this model and the exploration of our corpora is designed to build broader collective knowledge by bringing together existing expertise to address missed research opportunities, given the diversity of research in education related topics.
Absence of Communication
A first type of missed opportunity is the absence of communication between disciplines or between sub-domains in a given field of inquiry. In what follows, we note five examples that all have to do with different deficiencies in communication between researchers. First, delving into our scientometric maps can illustrate the different ways in which theoretical constructions are used. Scientific analyses are fragile when they are carried out from one point of view. This approach radically limits conclusions. Operationalizing theoretical constructions under different foci makes them more robust (Rosé and Lund, 2013). Our approach allows us to identify zones where theoretical constructions are operationalized differently. For example, the research that treats personal epistemology, epistemic cognition and development, beliefs, theories and epistemological resources are dispersed in different clusters and do not share the same references, although these constructions could benefit from being compared.
A Divided Field That Leads to Pursuing Different Objectives
A second example of missed opportunity around communication occurs when a field divides in order to pursue specific objectives, but does not maintain contact with the evolution of the other part of the field. The two domains Computer Supported Collaborative Work (CSCW) and Computer Supported Collaborative Learning (CSCL) are an example. This division allowed them to concentrate, respectively, on work and learning. But now there are attempts to re-integrate findings through the study of CSCL @ work (Goggins et al., 2013).
Foundational Differences That Hinder Collaboration
A third example of missed opportunity around communication occurs when two fields share a goal, but due to differences in foundational theories and models, do not build on each other's research (Kirby et al., 2005). For example, Learning Sciences and Instructional Systems Design share an interest in the application of technology for advancing human learning. But Learning Sciences is founded on theories and methods from Cognitive Science and Psychology (e.g., information processing models of learning; constructionist models of learning) whereas Instructional Systems Design is founded on theories and methods from design (instructionist models of learning). Kirby et al. (2005) showed that although there was a trend for increased cross-field citation, this trend was led by a small number of prominent researchers in both communities. The authors argued that combining the strengths of both communities—cognition in context on the one hand (Learning Sciences) and design (Instructional Systems Design) on the other—would give researchers a better chance of “effecting meaningful change in education through the creation and effective application of technology-enhanced learning environments” (op. cit., p. 46).
Specific Foci Lead to Researcher Isolation
A fourth example of missed opportunity around communication is when researchers who focus on particular aspects of a topic (e.g., human behavior) remain isolated from researchers who focus on others aspects. For example, in behavioral research in psychology, factors influencing dispositions of individuals are seen as more important than questions about the variation across differently situated populations (Longino, 2013). It follows that the set of references cited by a paper is very specific to the different disciplines and communities of readership for which the research is meant (op. cit.), despite the interest of combining approaches to gain broader understanding of the topic.
Same Broad Focus but Different Choices of Explanada
A fifth example of missed opportunity around communication is when researchers focus on the same phenomenon, but give attention to different explanatory aspects of it; an explanandum/explananda is the object(s) to be explained (Hempel and Oppenheim, 1948). Chevrot and Foulkes (2013) have noted the approaches of two fields in the study of language variation: Cognitive Sociolinguistics and Sociolinguistic Cognition. The first field explores cross-linguistic variation linked to social dimensions (Kristiansen and Dirven, 2008) and views linguistic knowledge and patterns of thought as properties shared by communities. These communities are seen as heterogeneous and are described at sociological, cultural, ideological or political levels thus sharing assumptions with sociolinguistics and employing a social approach to cognition (Kaufmann and Clément, 2011). The second field focuses on individual cognitive and cerebral mechanisms that underpin a person's ability to produce sociolinguistic variation and to process it during perception (Chevrot and Foulkes, 2013). For example, efforts include understanding the cognitive encoding of variants, describing the influence of social knowledge and its retrieval on the processing of variation, and studying the cerebral mechanisms that process indexical information. These authors argue that Sociolinguistic Cognition is close to psycholinguistics and represents the approach that Kaufmann and Clément (2011) call a cognitive approach to social facts. Indeed, it would seem that both Cognitive Sociolinguistics and Sociolinguistic Cognition should be considered in a relationship of reciprocal causality, in that knowledge about social life in relation to language and how we produce and understand language are mutually influencing each other.
Mobilizing Expertise at the Boundaries of Disciplines or Frameworks
The five examples of missed opportunities having to do with communication deficiencies discussed above also have another characteristic in common; they illustrate a deep expertise at boundaries between disciplines or frameworks. Such expertise includes building theoretical constructs, choosing which application domain to apply research, deciding on what part of analytic objects to focus, deciding which aspect of a topic to pay attention to, and choosing to focus on different explanatory aspects of the same phenomenon. Gaining this expertise takes time and effort.
In this final results section, inspired by Klein (1990) and deWachter (1982), we propose a slightly modified model (see Figure 6) that is set up to meet the challenges detailed in the previous section regarding broader education-related collective knowledge advancement. It is therefore a prospective model.
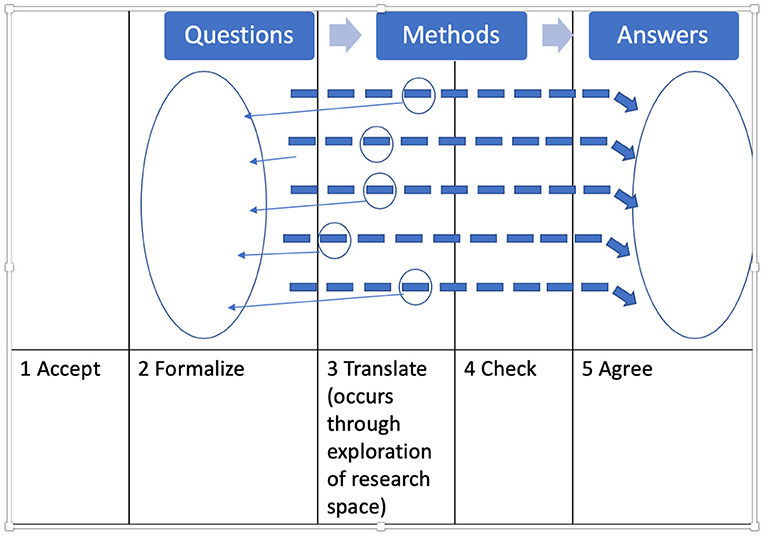
Figure 6. Inspired by deWachter (1982) and Klein (1990), an abstract model of the interdisciplinary process redrawn to integrate exploration of the maps of the research space of education within social sciences and humanities.
The interdisciplinary researcher mobilizes multiple theories that come from different disciplines and from different frameworks within the same discipline, according to analytic need. Each theory allows for observations that are oriented in particular ways and methods of interpretation that are based on specific assumptions. It is important to note that research questions should drive the choice of theories and methods, but that if researchers put the cart before the proverbial horse by choosing a particular theory and methods before thinking about research questions, this will seriously limit possibilities. In the same way, if a researcher only focuses on the specific theories and original methods with which they were trained, then answers will be limited in scope (Lund et al., 2013). Working from within bioethics, deWachter (op. cit.) proposes five stages to the interdisciplinary process, the foundation of which is a temporary suspension of all known methods, which he calls methodical epoché:
1. Accept that all involved disciplines abstain from approaching the topic using their own monodisciplinary methods;
2. Formalize the global question in an interdisciplinary way, acknowledging all of its aspects, as well as the total network;
3. Translate the global question into the specific language of each participating discipline;
4. Check the answer to this translated question to verify its relevance to the global question;
5. Agree upon a global answer which is not produced by any one disciplines, but integrates all particular available answers.
In Figure 6, we suggest that in each of the cases of missed opportunities around communication, researchers can follow this process model of interdisciplinarity in order to reach broader education-related collective knowledge advancement. Our proposed contribution to the model appears in phase 3 where researchers translate the global question into the specific language of each participating discipline. Finding out how to do this for different questions can be achieved by exploring the bibliometric coupling maps and the analyses thereof that we provide. One can explore the maps we used to generate the clusters in many other ways, by searching for most frequent keywords, most frequent authors' keywords, most frequent title words, most frequent subject categories, most frequent journal sources, most frequent countries, most frequent institution, most frequent references, most frequent references' sources, most representative papers, most cited papers, or most cited authors. For a given sub topic cluster, one can see the collective answers to all of these searches and be pointed to both papers and authors on google scholar. Such a target search tool specifically scaffolds the reformulation of questions in terms of different frameworks and disciplines.
In summary, we propose that this model be used, coupled with exploration of our maps, to meet the challenges that we previously defined and that in general require mobilizing expertise at the boundaries of disciplines or frameworks, a phenomenon that our analyses show, both at the Education corpus level and at the Social Sciences and Humanities corpus level. We argue that — given the will — operationalizing this proposal will help researchers to connect who have the same broad focus but different choices of explanada. It may also alleviate researcher isolation due to specific foci, if bridges can be built to other foci in order to gain a broader understanding. Given that a divided field leads to pursuing different objectives, touching base with other community branches can help a larger field to integrate results and perspectives. Finally, foundational differences that hinder collaboration can be specifically addressed, but again, given the will of the involved researchers.
Conclusion
In this paper, we set out to understand the nature of education research and how it is connected through citations to the larger social sciences and humanities. We answered four research questions regarding (1) the place of research in education in this larger context, (2) how research in education compares to the disciplinary composition of other fields, (3) what such a composition can tell us about the challenges that research in education faces, and (4) what kind of model for broader education-related collective knowledge advancement can meet these challenges.
We argued that education cannot be considered to be a single discipline and is instead very multidisciplinary. We illustrated this in multiple ways. First, bibliographic coupling shows that research in education exists on a continuum, from highly connected, cohesive clusters of articles on a particular topic to loosely connected, less cohesive clusters. The former focus more directly on learning and teaching and specifically address science whereas the latter reach out to different subject areas such as linguistics, sociology, and psychology, with education being only one object of inquiry, amongst others. Secondly, this result is mirrored at the social sciences and humanities level in that research in education is distributed over many subject area regions, corresponding to the loosely connected, less cohesive clusters of the Education corpus. That said, we also see a focus on Science at this level because a large percentage of the education related articles within the social sciences and humanities sub topic cluster “Science” are published in Education corpus journals and a large percentage of these are in the “Science Education” sub topic cluster in the Education corpus. Thirdly, articles having to do with education are more likely to connect to articles in other Scopus subject areas (in particular, Psychology) than articles of other Scopus subject areas. Fourthly, in qualifying Education related research first gathers articles essentially equally from both Education and Psychology and then next from Medicine subject areas. Fifthly, the Psychology subject area has the least breadth in terms of linked articles, but the most cohesiveness whereas the Education subject area has the most breadth and the least cohesiveness.
All of these measures point to the Education subject area as the most multidisciplinary both in terms of being less cohesive (lack of closely linked neighboring articles) and in terms of linking widely to different subject areas. A lack of cohesiveness may seem negative, but it is not necessarily a value judgement. And linking widely to different subject areas may seem positive in the sense of illustrating interest in theories and methods from other subject areas, and thereby exhibiting openness, but it may also be the curse of diversity we referred to in the introduction. If participating researchers have difficulty communicating due to no shared vision or common understanding of the literature, there will be dispersion of discourse and an unstable epistemic community. This seems to be the case today in research that is related to education, in light of its wide spread multidisciplinarity. We gave examples of the challenges that needed to be met and examples of the dispersion of discourse in education research. We then proposed a method based on the exploration of the Education and Social Sciences and Humanities corpora that can reduce dispersion, while building on expertise, and contribute to achieving broader education-related collective knowledge advancements, despite an unstable epistemic community.
Limitations and Perspectives
The main limitation of our work is that although we were able to compare publications in education from two periods (2000–2004 and 2015–2018), we were only able to compare the first period to publications in social sciences and humanities to a year within that period (i.e., 2000). We were not able to compare the second period to publications in social sciences and humanities for the same period, due to the explosion in publication numbers rendering our method unworkable. Our results from 2000 to 2004 illustrate a snapshot in time before an initial major increase in new journals being indexed, so it is both a time before such a comparison may drastically change, and it's a way to keep the number of bibliographic records manageable. However, given the changes in the two Education corpora (2000–2004 and 2015–2018), coupled with more current literature that discusses the broader state of research in the social sciences and humanities, we hypothesized that the pressing need for methods to scaffold integration between disciplines and frameworks has not let up.
The second limitation is that articles from Scopus only give a partial view of the research in education, Scopus essentially only gives an anglophone view. Similar work using google scholar would be more comprehensive, but meta data is lacking. In addition to continuing to use our maps for the study of fine-tuning research questions from an interdisciplinary standpoint, perspectives include using them for other activities, such as training early career researchers, finding partners for collaboration, co-developing primers with practitioners that target conceptual constructs that are useful for teaching, and pinpointing gaps in the literature and thus opportunities for new research.
Data Availability Statement
The datasets presented in this study can be found in online repositories. The names of the repository/repositories and accession number(s) can be found at: http://sebastian-grauwin.com/XYZ_EDUCMAP/.
Author Contributions
PJ and SG provided the knowledge and methods regarding bibliometric coupling. SG built the algorithms, produced Figures 1–4, Tables 1–4, and also constructed the website where the underlying data can be visualized. PJ built the algorithms, calculated the data, and produced Figure 5. HJ and KL provided the expertise needed to interpret the data in terms of research on education. KL contributed the challenges and benefits of multidisciplinarity, and the connection with deWachter's model, (Figure 6) the bibliometric maps and their interpretation to aid researchers to use interdisciplinarity in order to alleviate missed opportunities. All authors worked together weekly for a number of months, discussing how to frame the paper and re-read, and made extensive comments on the manuscript. All authors approved the submitted version.
Funding
The authors would like to acknowledge support in 2014 and 2015 from the Programme Exploratoire Premier Soutien (PEPS) COMUE à Projets Interdisciplinaires Université de Lyon – CNRS. In addition, the authors are grateful to the ASLAN project (ANR-10-LABX-0081) of Université de Lyon, for its financial support within the program Investissements d'Avenir (ANR-11-IDEX-0007) of the French government operated by the National Research Agency (ANR). This work was also supported by the National Research Foundation of Korea (Grant No. NRF-2016R1D1A1B03935697, 2016).
Conflict of Interest
The authors declare that the research was conducted in the absence of any commercial or financial relationships that could be construed as a potential conflict of interest.
Acknowledgments
The authors would like to acknowledge colleagues and students who have participated in various workshops with us on the EducMap project, among them Sanne F. Akkerman, Christine Detrez, Stephen M. Fiore, Judith Harackiewicz, Barbara K. Hofer, Emmanuelle Picard, and Romane Rozencwajg.
Footnotes
1. ^We examine in detail the relation of research in education in the period 2000-2004 to research in the social sciences and humanities in the year 2000, but we cannot do a similar comparison for 2015-2018 and a more recent year in the social sciences and humanities corpus. For example, the 2000 corpus has around 120,000 publications and in 2018, it would have 600,000. The extraction alone for 2018 would be a challenge and the analysis would unfortunately require more computer power than we have available. In addition, given the large difference in the corpora, we could expect some surprises regarding the Louvain algorithm (cf. section Bibliographic Coupling and Construction of Network Clusters), thus requiring additional analyses and interpretations. We plan to address the challenge of examining the relation of research in education to research in human and social sciences with more recent data in a future publication. But we will describe the Education corpus for both 2000-2004 (Education corpus 1) and 2015-2018 (Education corpus 2) and this allows us to extrapolate on the recent relation between education aon the one hand nd social sciences and humanities on the other.
2. ^EERQI project: http://www.eerqi.eu.
3. ^Education corpus 1 is referred to as Educmap 2000-2004, Education corpus 2 is referred to as Educmap 2015-2018 and the Social Sciences and Humanities corpus is referred to as Sociomap 2000 in the on-line visualizations at http://sebastian-grauwin.com/XYZ_EDUCMAP/).
4. ^The overall spatial structure of such clusters presented in Figure 4 is obtained thanks to a force-directed algorithm, used to draw the network in an aesthetically pleasing way. This algorithm simulates a physical system by assigning 3 types of forces among the nodes and links of the network: (1) a gravity force, that attracts every node to the center of the graph, (2) a spring-like attractive force, that attracts linked nodes toward each other, (3) a repulsive force, that tends to separate all pairs of nodes. The final layout results from an equilibrium between all these forces.
5. ^See http://sebastian-grauwin.com/XYZ_EDUCMAP/BCclusters.html for more details.
6. ^Compare the clusters in Sociomap 2000 at the topic level to the clusters in Educmap 2000-2004 at the topic or sub topic level http://sebastian-grauwin.com/XYZ_EDUCMAP/BCclusters.html.
References
AERES website: http://www.aeres-evaluation.fr/ AERES list of publication sources in educational research (2014). Available online at: http://www.aeres-evaluation.fr/Publications/Methodologie-de-l-evaluation/Listes-de-revues-SHS-de-l-AERES
Apostel, L., and Centre for Educational Research and Innovation Organisation for Economic Co-operation and Development (1972). Interdisciplinarity Problems of Teaching and Research in Universities. (Paris: Organization for Economic Cooperation and Development), 75–139.
Avis, J. (2003). Re-thinking trust in a performative culture: the case of education. J. Educ. Polut. 18, 315–332.
Birnbaum, P. H. (1981). Academic interdisciplinary research: characteristics of successful projects. J. Soc. Res. Adminis. 13, 5–16.
Blondel, V. D., Guillaume, J. L., Lambiotte, R., and Lefebvre, E. (2008). Fast unfolding of communities in large networks. J. Stat. Mech. Theory Exp. 2008:P10008. doi: 10.1088/1742-5468/2008/10/P10008
Chevrot, J.-P., and Foulkes, P. (2013). Introduction: language acquisition and sociolinguistic variation. Linguistics 51, 251–254. doi: 10.1515/ling-2013-0010
Choi, B. C. K., and Pak, A. W. P. (2006). Multidisciplinarity, interdisciplinarity and transdisciplinarity in health research, services, education and policy: 1. Definitions, objectives, and evidence of effectiveness. Clin. Invest. Med. 29, 351–364. doi: 10.25011/cim.v30i6.2950
deWachter, M. (1982). Interdisciplinary bioethics: but where do we start? A reflection on Epoché as Method. J. Med. Philos. 7, 275–287.
Epton, S. R., Payne, R. L., and Pearson, A. W., (eds.). (1983). Managing Interdisciplinary Research. (New York, NY: Wiley).
Fortunato, S. (2010). Community detection in graph. Phys. Rep. 486, 75–174. doi: 10.1016/j.physrep.2009.11.002
Gibbons, M., Limoges, C., Nowotny, H., Schwartzman, S., Scott, P., and Trow, M. (1994). The New Production of Knowledge. (London: Sage).
Goggins, S., Jahnke, I., and Wulf, V., (eds.). (2013). “CSCL@Work: case studies of collaborative learning at work,” in Computer Supported Collaborative Learning Series, Vol. 14, eds C. Hoadley, and N. Miyake (New York, NY: Springer), 243–268. doi: 10.1007/978-1-4614-1740-8
Good, B. H., Montjoye, Y.-A., and Clauset, A. (2010). Performance of modularity maximization in practical contexts. Phys. Rev. E 81:046106204010. doi: 10.1103/PhysRevE.81.046106
Grauwin, S., Beslon, G., Fleury, E., Franceschelli, S., Robardet, C., Rouquier, J. B., et al. (2012). Complex systems: dreams of universality, reality of interdisciplinarity. J. Am. Soc. Inform. Sci. Tech. 63, 1327–1338. doi: 10.1002/asi.22644
Grauwin, S., and Jensen, P. (2011). Mapping scientific institutions. Scientometrics 89, 943–954. doi: 10.1007/s11192-011-0482-y
Hall, K. L., Vogel, A. L., Stipelman, B. A., Stokols, D., Morgan, G., and Gehlert, S. (2012). A four-phase model of transdisciplinary team-based research: goals, team processes, and strategies. Transl. Behav. Med. 2, 415–430. doi: 10.1007/s13142-012-0167-y
Hanisch, T. E., and Vollman, W., (eds.). (1983). Interdisciplinarity in Higher Education, United Nations Educational, Scientific, and Cultural Organization. Bucharest. European Centre for Higher Education.
Hausman, C. R. (1979). “Introduction: disciplinarity or interdisciplinarity?,” in Interdisciplinarity and Higher Education, ed J. Kockelmans (University Park, PA: The Pennsylvania State UP), 1–10.
Hempel, C. G., and Oppenheim, P. (1948). Studies in the logic of explanation. Philos. Sci. 15, 567–579. doi: 10.1086/286983
Hermeren, G. (1986). “Interdisciplinarity revisited promises and problems,” in Interdisciplinarity Revisited: Re-assessing the Concept in Light of Institutional Experience, eds L. Levin, and I. Lind (Stockholm: Organisation for Economic Cooperation and Development, Swedish Board of Universities and Colleges), 15–25.
Jäger, J. (2007). “Foreword,” in Handbook of Transdiscplinary Research, eds G. Hirsch Hadorn, H. Hoggmann-Riem, S. Biber-Klemm, W. Grossenbacher-Mansuy, D. Joye, C. Pohl, et al. (Bern: Springer), vii–viii.
Jensen, P., and Lutkouskaya, K. (2014). The many dimensions of laboratories' interdisciplinarity. Scientometrics. 98, 619–631. doi: 10.1007/s11192-013-1129-y
Kaufmann, L., and Clément, F. (2011). “L'esprit des sociétés bilan et perspectives en sociologie cognitive,” in La Sociologie Cognitive, eds F. Clément, and L. Kaufmann (Paris: Orphys et Maison des Sciences de l'Homme), 7–40.
Kessler, M. M. (1963). Bibliographic coupling between scientific articles. Am. Document. 24, 123–131.
Kirby, J. A., Hoadley, C. M., and Carr-Chellman, A. A. (2005). Instructional systems design and the learning sciences: a citation analysis. Educ. Technol. Res. Dev. 53, 37–48. doi: 10.1007/BF02504856
Klein, J. T. (1990). Interdisciplinarity: History, Theory, and Practice. (Detroit, MI: Wayne State University).
Klein, J. T. (1996). Crossing Boundaries: Knowledge, Disciplinarities, and Interdisciplinarities. (Charlottesville: University of Virginia Press).
Kocklemans, J. (1979). “Why interdisciplinarity?” in Interdisciplinarity and Higher Education, ed J. Kocklemans, (University Park, PA: Pennsylvania State UP), 123–160.
Kristiansen, G., and Dirven, R., (eds.). (2008). Cognitive Sociolinguistics: Language Variation, Cultural Models, Social Systems. (Berlin: Mouton de Gruyter).
Kuhn, T. S. (1962/1970). The Structure of Scientific Revolutions (Edition 1970). (Chicago, IL: The University of Chicago Press).
Latour, B. (2005). Reassembling the Social: An Introduction to Actor-Network Theory. (Oxford: Oxford University Press).
Lattuca, L. R. (2003). Creating interdisciplinarity: grounded definitions from College and University faculty. Hist. Intell. Cult. 3, 1–20.
Longino, H. E. (2013). Studying Human Behavior: How Scientists Investigate Aggression and Sexuality. (Chicago, IL: University of Chicago Press).
Lund, K. (2016). Modeling the Individual Within the Group: an Interdisciplinary Approach to Collaborative Knowledge Construction. Education: Université Grenoble – Alpes.
Lund, K., Jeong, H., Grauwin, S., and Jensen, P. (2015). A Scientometric Map of Global Educational Research. Working paper.
Lund, K., Jeong, H., Grauwin, S., and Jensen, P. (2017). Une carte scientométrique de la recherche en éducation vue par la base de données internationales Scopus. Les Sci. Éduc. 50, 67–84. doi: 10.3917/lsdle.501.0067
Lund, K., Rosé, C. P., Suthers, D. D., and Baker, M. (2013). “Epistemological encounters in multivocal settings,” in Productive Multivocality in the Analysis of Group Interactions, eds D. Suthers, K. Lund, C. P. Rosé, C. Teplovs, N. Law, C. Hoadley, et al. (New York, NY: Springer), 659–682.
Miller, R. C. (1982). Varieties of interdisciplinary approaches in the social sciences: a 1981 overview. Issues Integrat. Stud. 1, 1–37.
Mingers, J., and Leydesdorff, L. (2015). A review of theory and practice in scientometrics. Eur. J. Operation. Res. 246, 1–19. doi: 10.1016/j.ejor.2015.04.002
Newman, M. E. J., and Girvan, M. (2004). Finding and evaluating community structure in networks. Phys. Rev. E Stat. Nonlin. Soft. Matter Phys. 69:026113. doi: 10.1103/PhysRevE.69.026113
Rosé, C. P., and Lund, K. (2013). “Methodological pathways for avoiding pitfalls in multivocality”, in Productive Multivocality in the Analysis of Group Interactions, eds D. D. Suthers, K. Lund, C. P. Rosé, C. Teplovs, and N. Law (New York, NY: Springer), 613–637.
Small, H. (1973). Co-citation in the scientific literature: a new measure of the relationship between two documents. J. Am. Soc. Inform. Sci. 24, 265–269. doi: 10.1002/asi.4630240406
Keywords: education research, social sciences and humanities (SSH), bibliometics, discipline (s) (of knowledge), multidisciplinarity, interdisciplinarity, transdisciplinarity, integration challenges
Citation: Lund K, Jeong H, Grauwin S and Jensen P (2020) Research in Education Draws Widely From the Social Sciences and Humanities. Front. Educ. 5:544194. doi: 10.3389/feduc.2020.544194
Received: 19 March 2020; Accepted: 28 October 2020;
Published: 08 December 2020.
Edited by:
Arthur C. Graesser, University of Memphis, United StatesReviewed by:
Chih Ming Chu, National Ilan University, TaiwanSusan R. Goldman, University of Illinois at Chicago, United States
Copyright © 2020 Lund, Jeong, Grauwin and Jensen. This is an open-access article distributed under the terms of the Creative Commons Attribution License (CC BY). The use, distribution or reproduction in other forums is permitted, provided the original author(s) and the copyright owner(s) are credited and that the original publication in this journal is cited, in accordance with accepted academic practice. No use, distribution or reproduction is permitted which does not comply with these terms.
*Correspondence: Kristine Lund, a3Jpc3RpbmUubHVuZCYjeDAwMDQwO2Vucy1seW9uLmZy