- Chair of Psychology of Learning with Digital Media, Institute of Media Research, Faculty of Humanities, Chemnitz University of Technology, Chemnitz, Germany
The entity players compete with is an important element of competitive mechanisms. However, this crucial element is barely investigated within educational video games, as educational psychology research focuses mainly on supportive role models (e.g., pedagogical agents, intelligent tutorial systems). Nevertheless, the influence on learning must be explored, as interaction with an opponent might accompany the whole learning process. Thus, an experiment was conducted comparing three forms of social competition with an emphasis on external valid applications. More specifically, playing against a human competitive agent, playing against an artificial competitive agent, and playing against an artificial leaderboard were compared. Additionally, methods of adaptive difficulty adjustment were included within these groups to harness the potential of artificial systems. The results of the study (N = 102) revealed a beneficial effect of adaptive mechanisms on learning performance and efficiency. Furthermore, a difference in play behavior could be observed. The participants reported a lowered feeling of shame, increased empathy, and behavioral engagement when facing competitive agents. In contrast, calculations revealed no significant impact on mental strains by potentially demanding social competitors. These results highlight the potential for the future development of adaptive game systems and help choose the optimal implementation of social competition within different educational video games.
Introduction
Social interactions are a frequent element of video games, ranging from simple comparisons of achieved scores to complex collaborations within massively multiplayer online role-playing games. Furthermore, the use of computer technology enables the simulation of social entities, allowing game designers to create artificial social experiences. This approach has tremendously evolved throughout video game history, reaching a point where computer-controlled opponents might not only be worthy competitors for human players but even outperform them in board games (Mozur, 2018) and video games (Vincent, 2018). This highlights the potential of artificial elements when a human teammate or opponent is not available. More importantly for educational settings, computer-controlled entities offer features that might raise their value beyond that of placeholders for human players. For example, they enable deep control over the gameplay process. Instructors could adjust them with personalized or adaptive elements to suit their needs (Bakkes et al., 2012) and use them to guide learners’ progress. This might increase the efficiency of the underlying learning mechanism, as the system might be capable of optimizing each moment during gameplay. However, it is not yet clear how such adaptive and artificial components should be integrated within educational video games. Simply exchanging human opponents with artificial entities or abstract state representations might affect learning-related variables, such as emotions or cognitive load. Therefore, the influence of adaptive artificial competition within educational video games must be systematically analyzed, especially as investigations of individual game mechanics with systematic variations of their nuances are still scarce (Ke, 2016; Vandercruysse and Elen, 2017), and further experimental exploration of theory-driven design recommendations is requested (Clark et al., 2016). Therefore, the purpose of this study is to experimentally explore the impact of different social entities and the potential of adaptive elements within artificial entities on the learning process.
Literature Review
The following literature review will outline the competition game mechanic and elaborate the focus on social aspects. After artificial opponents are introduced, the question of how to configure the central aspect of difficulty will be discussed. For this, an adaptive approach is endorsed.
Artificial Social Competition
Current research stresses the importance of investigating distinct game mechanics to obtain generalizable insights into game-based learning (Nebel, 2017). In this context, game mechanics are described as the core components that define gameplay, which is responsible for creating patterns of experience (Salen and Zimmerman, 2004). Although not every game mechanic might be similarly beneficial for every player type, the competition mechanic seems to provide the broadest appeal (Orji et al., 2018), whereby competition can be defined as a process that unfolds over mutually exclusive desirable game states (Johnson et al., 1986) and is characterized by an inverse relationship of goal attainment between at least two entities (Griffiths et al., 2016). It is argued that competition might be one of the most important factors for enjoying video games (Vorderer et al., 2003), and that games are essentially competitive by nature (Salen and Zimmerman, 2004). Despite this importance, the empirical evidence regarding the educational context is not yet conclusive (Clark et al., 2016). Evidence in favor of competitive elements (e.g., Plass et al., 2013; Chen and Chen, 2014; Cagiltay et al., 2015), as well as evidence exploring boundary conditions (e.g., Vandercruysse et al., 2013; Chen, 2014; ter Vrugte et al., 2015) can be identified. Furthermore, research demonstrated that competition within educational video games involves several distinguishable processes that can be implemented with different game elements (media representations by which the user interacts with the game mechanic itself; Martens and Mueller, 2016). For example, the player could engage in a competitive mechanic through displays of ranks for comparisons (Seaborn and Fels, 2015), a game element often referred to as leaderboards (Nebel et al., 2016a, 2017) or visual representations of the competitor (Nebel et al., 2016b). To compare these different elements and mechanics, research must focus on specific aspects.
As argued in the introduction, changing the competitor type might influence the resulting social processes. Therefore, within this paper, social aspects are emphasized, and further clarification of such entities within the competitive process is needed. For this, social competition can be described as “a process which develops by competitive actions performed by individuals or social entities in order to maintain their own interests to the disadvantage of others” (Vorderer et al., 2003, p. 4). In addition to the previous definitions of competition, this approach underlines the importance of others, implying other players instead of abstract entities. Later, the authors amended that single-player games including (artificial) opponents should elicit similar social competitive processes as well. Hence, within this paper, competition with a human or artificial entity with humanlike features is referred to as social competition, whereas the degree of perceived social experiences may vary from comparably weak with only scarce perceivable social cues (e.g., online leaderboard) to strong with various social cues (e.g., arm wrestling). Different levels of such cues have been demonstrated as influential variables while investigating learning with multimedia (e.g., Mayer, 2014). If the opponent offers humanlike features without actually being human, this is referred to as artificial social competition. Naturally, this perspective excludes other aspects of competition, for example, competing with the game system itself (e.g., juggling) or artificial elements competing with one another (e.g., agent-based models).
Social Processes Within Artificial Social Competition
The underlying mechanics of competition have been most prominently described using Festinger’s Theory of Social Comparisons (1954). Regarding abilities, he postulated that humans have an inherent drive to evaluate themselves. Furthermore, if objective means are not available, they evaluate their abilities in comparison with others. Additionally, Festinger clarified that smaller differences in abilities will increase the tendency to compare oneself with others, and he postulated a unidirectional drive upward as long as no non-social restraints prevent the improvement regarding the underlying skills. This deeply rooted urge can explain why players engage in competitive activities, and why comparisons with others are appealing to many different player types (Orji et al., 2018). However, Festinger focused on real social interactions, whereas the previous introduction of artificial social competition clarified that it can include interactions with non-human entities as well. This might induce additional effects. For example, players report higher enjoyment, presence, and flow if they think they play against another human, even if this opponent is, in fact, a computer-controlled enemy (Weibel et al., 2008). In contrast, if players believe their opponent is computer-controlled, they report a different game experience, even if the opponent was, in reality, another human player (Gajadhar et al., 2008). Additionally, the mere presence of other players might moderate the negative effects of competitive gameplay, for example, by dampening aggressive behavior (Williams and Clippinger, 2002).
Highly abstracted systems lacking many humanlike features, such as leaderboards, offer different benefits. For instance, simple representations might help focus the player’s attention on the learning topic and reduce potentially disturbing strains induced by an opponent. Additionally, some artificial systems might provide better diagnostic features, as individual skill is not only communicated dichotomously (e.g., being better or worse than an opponent) but is characterized by the numerous leaderboard entries or the different rating scale nuances. However, the high degree of the abstraction of human features might dampen the social competition influences. Although Festinger (1954) did not address this issue explicitly, his discussion of relevance and attraction can be applied here. More specifically, he stated that the comparison group’s increased relevance and the attraction will increase the pressure toward uniformity of the compared ability. Transferred to the topic of social competition, it could be argued that not only the comparison partner’s identity but also his/her perception, representation, or interaction potential could influence the related mechanisms. For example, seeing and speaking with another player might increase the perceived relevance or attraction in contrast to reading his/her nickname on a leaderboard. The influence of human or even humanlike presences as supportive role models (e.g., teachers, pedagogical agents) is under investigation within the psychology field (e.g., social facilitation, Bond and Titus, 1983; parasocial interaction, Giles, 2002; anthropomorphism, Schneider et al., 2018; social agency, Schroeder et al., 2013). However, within the topic of artificial social competition, the interaction occurs with an opponent instead of a supporter. Therefore, further research is needed to explore whether these conclusions can be transferred. More specifically, this research will investigate the potential effects of the competitive agent on social presence, emotional variables, and mental strains.
Impact of Difficulty
If artificial competitive elements are included, crucial parameters such as difficulty must be determined. However, perceived difficulty within social competition might differ relative to each learner’s performance (Abuhamdeh and Csikszentmihalyi, 2012). A player winning effortlessly might indicate a low individual difficulty, whereas losing by a wide margin indicates a relatively high difficulty even if the context was identical. This argumentation appears evident but gains importance, as individual difficulty might play a crucial part in influencing how the game is experienced and how the learning process evolves. For example, it was revealed that difficulty influences a game’s appeal and how often it is played (Xue et al., 2017). More specifically, an inverted U-shaped relationship can be described between the closeness of games (i.e., player performance is relatively close to the indicated performance goal) and subsequent gameplay frequency (Lomas et al., 2017). Overall, winning players are more likely to continue to play. However, even players who suffer a close defeat demonstrate comparably equal values in their subsequent engagement (Lomas et al., 2017). This is in line with the observation that reported enjoyment significantly correlated with perceived challenge even more strongly than with perceived skill. However, this relationship might be further moderated by other factors, such as intrinsic motivation or goal directions (Abuhamdeh and Csikszentmihalyi, 2012). Similar conclusions could be drawn from Festinger’s (1954) theory, as he hypothesized that people will be more drawn to situations in which others are comparably close in abilities than to scenarios where others are relatively divergent.
In contrast to this argumentation favoring closeness and balanced games, evidence in favor of difficult and easy constellations can be identified as well. For example, easy educational games might be played longer (Lomas et al., 2013, 2017), and higher achieved scores are related to perceived satisfaction (Cheon et al., 2015). Additionally, players who were informed that they played faster than others felt more competent and autonomous (Burgers et al., 2015). Overall, performing slightly better than opponents appeared to be the most enjoyable scenario, especially when compared to very imbalanced scenarios (Abuhamdeh and Csikszentmihalyi, 2012). However, especially in the educational psychology field, difficulty is less negatively connoted. For instance, the desirable difficulties approach (Bjork, 1994) emphasizes the use of specific additional difficulties for the learner. Moreover, an increased difficulty demonstrated a higher learning rate (Lomas et al., 2013), and feedback with negative valence increased the likelihood of immediately playing the game again (Burgers et al., 2015). Furthermore, such feedback mobilized a higher invested effort—a potential precondition of higher performance. In contrast, feedback with positive valence might lead to a reduced effort, and freed resources might be spent otherwise (Raaijmakers et al., 2017). A similar rationale can be identified within the Elaboration Retrieval Theory (Carpenter, 2009). Using this approach, it is argued that challenging tasks result in a higher invested effort. Consequentially, higher activation is induced, leading to increased knowledge recall. Subsequently, an adaption is needed to assign the optimal challenge to each individual learner (Endres and Renkl, 2015).
Adaptive Approach
As the previous chapter highlights, the selection of the appropriate difficulty might not be a trivial task, and a tradeoff between different considerations (e.g., motivation, learning) might be advised. Furthermore, as the most beneficial challenge might differ between individuals and competition could be perceived differently by each learner (Orji et al., 2017), adaptive approaches could be essential to optimize learning outcomes. In this context, adaptivity can be defined as “a player-centered approach by adjusting games’ mechanics and representational modes to suit games’ responsiveness to player characteristics with the purpose of improving in-game behavior, learning processes, and performance” (Schrader et al., 2017, p. 5). The relevance of such systems is highlighted by the amount of research addressing dynamic difficulty adjustments (DDAs, e.g., Spronck et al., 2004; Alexander et al., 2013; Altimira et al., 2017; Chen et al., 2017; Xue et al., 2017). Although adaptive mechanisms are not undisputed (e.g., Yong, 2018), as they influence crucial parts of gameplay without the players’ knowledge or involvement, they offer several benefits in educational settings. For example, depending on individual attributes, learners might not choose the optimal difficulty by themselves. Their individual gaming knowledge or ex-ante training self-efficiency could influence the chosen practice difficulty, and thus, the learning outcomes (Hughes et al., 2013). Additionally, learners might commit an inferential error when analyzing their perceived performance in combination with their learning progression (Bjork, 1994; Alexander et al., 2013).
Another benefit of adaptive systems is revealed during the gaming process. The learner’s subjective cognitive effort is reduced as their skill increases (Yeo and Neal, 2008). Thus, the optimal conception of a series of learning events could be a challenge. As a possible solution, adaptive systems could be used to maintain an adequate effort, with interventions adjusting the provided difficulty. Overall, adapted difficulty could lead to higher engagement (Altimira et al., 2017) and learning efficiency (Salden et al., 2004) and prevent frustration (van Lankveld et al., 2010). Furthermore, if the difficulty is correctly adjusted to the player’s skills, the uncertainty or suspense of not knowing the game’s outcome might promote deeper involvement in the activity (Abuhamdeh and Csikszentmihalyi, 2012). Altogether, adaptive approaches might significantly enhance learning outcomes and efficiency.
The Present Experiment
The literature review revealed significant challenges and the unexplored potential of adaptive approaches in combination with artificial social competition. To shed light on this topic, the present experiment (Figure 1) manipulates the game element competitive opponent within three typical scenarios [non-adaptive competition with strong social experiences (e.g., playing against another random player; HUMAN), adaptive artificial competition with strong social experiences (e.g., playing against an adaptive agent; AGENT), and adaptive artificial competition with weak social experiences (e.g., playing against an adaptive leaderboard; LEADRBD)].
Effects of Competitive Agents
The first segment will focus on the influence of human opponents or competitors potentially inducing strong social experiences, further referenced as competitive agents. Their humanlike features might trigger stronger awareness and involvedness with the competitive agent in comparison to artificial competitors with few humanlike features. This has been frequently addressed using the concept of social presence (de Kort et al., 2007). Therefore, it is postulated:
Hypothesis 1: Learners playing against competitive agents report higher social presence than players competing with a leaderboard.
Such an influence on presence could be relevant for learning, especially in combination with cognitive load (Schrader and Bastiaens, 2012). More specifically, cognitive learning theories, such as the Cognitive Load Theory (Sweller, 1994), suggest that learning irrelevant strains should be minimized to increase learning outcomes (Sweller et al., 2011). Presenting interesting information separated from the learning material, such as competitive elements or pedagogical agents (Dinçer and Doğanay, 2017; Schroeder and Traxler, 2017), could induce a potentially hindering load. In the context of virtual agents, facial expressions might particularly dampen comprehension (Frechette and Moreno, 2010). Therefore, it is hypothesized:
Hypothesis 2: Learners playing against competitive agents report higher cognitive strains than players competing with a leaderboard.
Theories overwhelmingly focusing on cognitive aspects have been expanded, providing a more exhaustive overview of the learning process (e.g., Integrated Cognitive Affective Model of Learning with Multimedia, Plass and Kaplan, 2016). Paradigmatically, Mayer (2018, p. 175) states: “The future (of educational psychology) involves overcoming some of the limitations of the cognitive revolution by incorporating motivation, metacognition, affect, and brain science into theories of academic learning.” Therefore, a more holistic perspective, including facets like emotions, should be pursued, and possible interactions should be revealed. For example, the emotional design field has demonstrated several connections between emotions and learning (e.g., Um et al., 2012; Schneider et al., 2016). Regarding competitive agents, it could be argued that social or parasocial interactions might amplify emotional experiences (Hartmann et al., 2004). Therefore, it is hypothesized:
Hypothesis 3: Learners playing against competitive agents report stronger emotional reactions than players competing with a leaderboard.
Adaptive Competition
The second segment will shift the focus toward the investigation of the adaptive elements. As the literature review highlighted, game mechanics with adaptive elements could substantially support the learning process in contrast to random competition against other learners. Therefore, it is postulated:
Hypothesis 4: Learners playing against adaptive competitive elements demonstrate higher retention scores than players competing against human opponents.
The insights into learning (H4) and mental strains (H2) might not be sufficient to interpret the impacts of adaptive social competition, as it is argued that an interpretation of cognitive strains could only be meaningful in combination with the associated performance (Paas and Van Merriënboer, 1994; Paas et al., 2003). For example, even if unbeneficial for learning outcomes (i.e., performance), adaptive adjustments or gameplay changes might nonetheless benefit process efficiency (Salden et al., 2004; Nebel et al., 2017) by influencing cognitive strains. More specifically, the lack of human competitors has been revealed as more efficient for learning purposes (Nebel et al., 2016b). Therefore, it is hypothesized:
Hypothesis 5: Learners playing against adaptive competitive elements demonstrate higher process efficiency than players competing against human opponents.
Additional Measures and Calculations
The majority of game-based learning research focuses on pre-and posttest measures (Smith et al., 2015). Therefore, additional data collection during gameplay is required. For example, as the analysis of individual player experience appears to be a challenge in the field (Young et al., 2012), explorative calculations addressing this topic were added. For example, adaptive components might ensure learners play faster but also make more errors (Jagušt et al., 2018). Therefore, we explore a potential difference regarding playstyle and game experience between the groups. Finally, if the analysis of the hypothesis reveals significant results, we will conduct comparisons of each individual group to gather deeper insights into the underlying processes.
Materials and Methods
Participants
Overall, 102 students (Mage = 22.38, SD = 3.41; 84.3% female) participated in the study. This sample size was aimed for, as a priori sample planning using the intended design, a small to medium effect (Cohen, 1988) similar to other experiments (Nebel, 2017), and sufficient sensibility (f2 = 0.06, α = 0.05, 1-β = 0.80) revealed a necessary sample of 102 participants for three groups with two dependent variables [e.g., mental load (ML) and mental effort (ME)]. Most were enrolled in Bachelor Media Communications (49.0%), Master Media-and Instructional Psychology (12.7%), and Elementary School Pedagogy (12.7%). The remaining students (25.6%) were distributed over 14 other fields of study. They were either rewarded with a 1.5 course credit or 9€. After the data collection, one participant had to be excluded, as she reported a mild form of dyslexia. Additionally, as the participants could play rather unobserved and might only pretend to learn to earn the course credit, the dataset was checked for unusual patterns. Several Grubbs tests (Grubbs, 1969) using the GraphPad outlier calculator (GraphPad Software, 2018) were conducted. More specifically, knowledge measures (as an indicator of an unwillingness to learn) and the frequency in which the participants did not answer the questions within the game (as an indicator of an unwillingness to play) were analyzed. This led to the exclusion of four more participants, resulting in a final sample of n = 97. There was no significant difference between the experimental groups regarding gender (p = 0.56) or age (p = 0.11). Additionally, prior knowledge was measured during the pretest questionnaire with self-created scales addressing general domain knowledge, the familiarity with the learning material (animals), and prior game experience. Domain knowledge was assessed with three questions asking “How would you describe your prior knowledge in the field of…geography (climate zones, ecological systems); biology (animals, plants); exotic animals (reptiles, amphibians).” Participants had to rate their answers on a five-point Likert scale ranging from “very good” to “very poor.” Game experience was measured with the same scale and two questions asking “How would you describe your prior knowledge in the field of…video games (e.g., consoles, PC, mobile, handhelds); quiz games (e.g., QuizDuel, Who Wants to Be a Millionaire).” Familiarity with the learning material was measured four questions addressing each of the included animals. More specifically, the players where asked “Do you know this animal?.” They could rate their answer on a four-point Likert scale including “Not at all,” “I’ve heard it before,” “Yes, a little bit,” and “Yes, very well.” A multivariate analysis of variance (MANOVA) addressing these measures revealed significant differences [Wilk’s Λ = 0.853, F(6,184) = 2.54, p = 0.022] between the experimental groups. Follow-up analyses of variance (ANOVA) demonstrated a significant difference regarding prior game experience F(2,94) = 3.52, p = 0.034 and prior experience regarding the animals F(2,94) = 3.43, p = 0.036 but not regarding general prior knowledge F(2,94) = 0.62, p = 0.54. Therefore, game and animal knowledge will be included as covariates.
Design
To address the postulated hypotheses and research questions, a design with three groups was chosen (Table 1).
Thus, three different versions of the same game were necessary (Figure 1). However, to foster comparability, the visual game elements should be kept as constant as possible and the interface should not change substantially throughout the game. This process started with the HUMAN (non-adaptive competition with strong social experiences) version. To gather valid data within complex social interactions, a controllable situation without many confounding factors was used. Thus, instead of letting the players play side by side, an interaction through the game interface appeared more appropriate. More specifically, a webcam (Logitech C920 HD PRO) was used to stream a video of the player’s face to the competitor’s monitor (26″) and vice versa. This particular image detail was selected, as the whole body might only be crucial in extreme situations to correctly attribute the emotional valence (Aviezer et al., 2012). Following, the AGENT (adaptive artificial competition with strong social experiences) condition was created. First, the webcam image was replaced with that of an artificial agent. Based on the assumption that humans are capable of identifying emotions even within rudimentary humanlike avatars (Korn et al., 2017), the representation could be kept simple, especially as fine details are non-essential for face recognition (Sinha et al., 2006). More specifically, focus was placed on the most important facial features for communicating affective states (Frechette and Moreno, 2010), such as the eyebrows, eyes, and mouth. To foster comparability with the webcam image, it was ensured that the agent was capable of demonstrating simple emotions. Therefore, different facial expressions were designed and triggered if the game status changed. More specifically, an emotion was selected: for the learning phase; during the presentation of a question; while the players waited for the correct answer; while the answers of the player, the agent, and the correct answer were presented; after the points were rewarded. For example, if the player gave the wrong answer, the agent showed malicious joy (Figure 2).
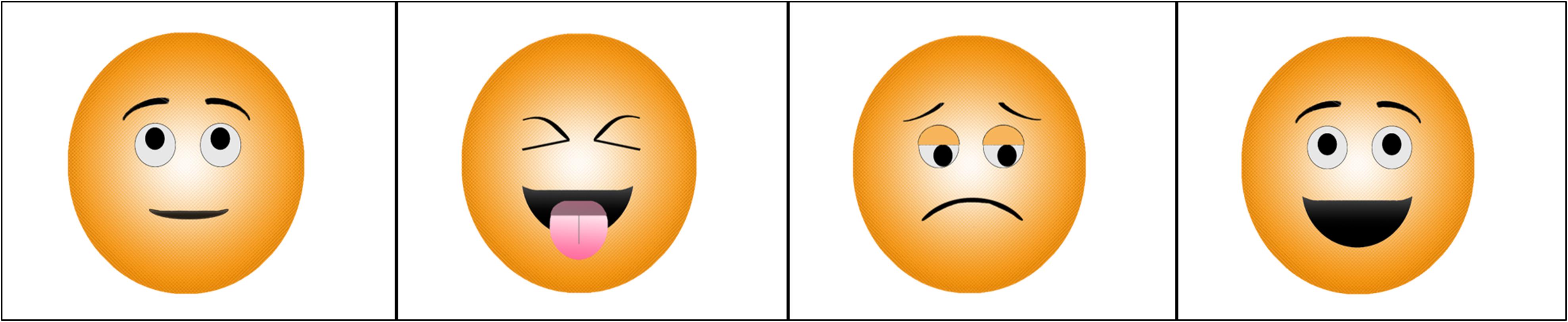
Figure 2. Examples of AGENT emotions (from left to right: neutral, malicious joy, sad, happy; translated from German).
Finally, the LEADBRD (adaptive artificial competition with weak social experiences) version had to be created. To foster constant visual strains in comparison with the two previous experimental groups (AGENT and HUMAN), appropriate counterparts for the necessary representations were developed. The point’s overview was replaced with an extract from a leaderboard. Furthermore, the competitive agent’s image was replaced by a number indicating the player’s position within a leaderboard. As the AGENT and HUMAN conditions reacted to the player’s actions, rudimentary visual stimuli within the LEADBRD group were included as well. More specifically, after the players received a point for their performance, arrows appeared, indicating the progression within the leaderboard.
Tasks
The game was intended to teach simple factual knowledge. Hence, the game task should be aligned with this learning goal. In this context, testing might be an appropriate learning mechanism for simple recognition tasks, as the testing effect appears to be more beneficial if the learning material can be characterized by low element interactivity (Hanham et al., 2017). Additionally, testing has become more relevant, as response tools such as Kahoot! (2018) or Quizlet Inc (2018) have gained popularity in the classroom. More specifically, the learners received 10 short texts, each with an average of M = 94.2, SD = 16.83 words, regarding different comparably obscure animals (Thorny Devil, Pacman Frog, Shoebill, Lungfish). The average learning time held constant at 200 words/min. During this phase, the interface was mostly identical to the quiz segment of the game (Figure 1). As they were not necessary during this phase, no questions or answers were presented. Instead, the learning text was shown on the left side of the screen. Thus, players could still see their opponents or leaderboard rank depending on their experimental group. After each text, the players had to answer quiz questions regarding the learning material. Within this game phase, the players could choose one of the four possible answers. Once the players made their choices, the correct answer was presented. If the players responded correctly, they received points. Otherwise, the opponent received them. Additionally, the point value increased during the course of the game, creating the chance for players to catch up. However, this simple point-based reward system demanded a modification within the LEADBRD condition. Within the competitive agent groups, a certain time pressure exists, as the opponent might answer faster and steal the potential reward. To ensure this confounding influence was dampened, the mechanic was changed to a points-per-time-based system. The players in the LEADBRD group were informed that they would receive the maximum points per question only if they answered quickly. Hence, this condition included the time pressure factor as well.
To implement adaptivity within the tasks, two different approaches for each artificial system had to be implemented. For the LEADBRD condition, the approach of Nebel et al. (2016a, 2017) was used. In these experiments, a static leaderboard for one specific task was artificially created, and the player could progress through its ranks after a repetition of the same task. In contrast to these static approaches, however, the leaderboard within this experiment was not only presented after the completion of the task (i.e., the game). Instead, it offered dynamic content throughout the complete game and had to be continuously updated after each quiz question. Thus, a progress that ensured the player could perceive a sense of self-efficacy (Bandura, 1977) hat to be induced differently. For this, a mechanism was included that artificially calculated a new player rank after each individual question. If a player performed well, the rank was increased, and if the player performed poorly, the rank was lowered. Afterward, the remaining entries of the leaderboard were calculated identically to the previously used approach of Nebel et al. Thus, the progression within the leaderboard was kept similar for all participants. This was especially important, as research has demonstrated that different positions and progressions within a leaderboard could influence learning-related variables (Nebel et al., 2016a, 2017). The adaptive mechanism within the AGENT group consisted of two separate elements that were continuously used throughout the game. First, it had to be determined when the artificial opponent would answer. Based on a predetermined probability curve, the answer time was adapted to each individual player. Second, the issue of what the opponent would answer had to be solved. For this, an adaptive mechanism ensured that the agent answered comparably equal to the player. This process was repeated for each individual quiz question throughout the game. In contrast to a specific set of pre-defined opponents, this approach ensured that each participant faced a competition matching their individual playstyle and compare their performance in real-time with an opponent (similar to the HUMAN or LEADBRD condition). Overall, this design led to a small delay in the experimental manipulations. More specifically, the social comparison will be visible from the beginning, whereas the adaptivity can only begin during the first interaction (the first quiz question).
Measures
Because of the ongoing criticism of Cronbach’s α (McNeish, 2018), the coefficient Revelle’s ω (McDonald, 1999; Revelle and Zinbarg, 2008) was chosen to calculate the reliability estimates for all measures.
Questions identical to the 39 quiz items within the game were used to assess retention knowledge (ω = 0.81). Thus, retention was not assessed with the quiz itself, but with an additional questionnaire after the gameplay phase. ML and ME were measured with Krell’s (2015). Six items assessed the ML, and six items assessed the ME. Statements similar to “the tasks were challenging” were rated on a seven-point Likert scale ranging from “not at all” to “totally.” The questionnaire was implemented twice to measure ML and ME during gameplay and during the retention test (0.89 ≤ ω ≤ 0.95). In addition, the time the participants needed to respond to the questions as well as their performance (i.e., the number of correct answers) were used for the efficiency calculations. More specifically, the efficiency during the learning process was calculated using the following formula (Salden et al., 2004) with standardized (z) values: E = [(zPerformance – zMentalStrainGame – zTime)/31/2]. As two types of mental strains were measured (ML and ME), two types of process efficiency could be investigated depending on whether ML or ME is included as mental strain component within the formula. Game experience was assessed with the core module of the Game Experience Questionnaire (IJsselsteijn et al., 2013). The relevant subscales for the current investigation were challenge, competence, and flow (five items each; 0.81 ≤ ω ≤ 0.89). Statements like “I felt competent” were rated on a five-point Likert scale ranging from “completely true” to “not true at all.” The Achievement Emotions Questionnaire (Pekrun et al., 2011) was used to measure emotions during gameplay. In line with the prior research (e.g., Nebel et al., 2017), only 15 items that measured class-related emotions could be implemented after the test phase (0.70 ≤ ω ≤ 0.75). Statements like “I am happy that I understood the material” were rated on a five-point Likert scale ranging from “strongly disagree” to “strongly agree.” Social presence was measured with the Social Presence in Gaming Questionnaire (SPGQ; de Kort et al., 2007). This includes the subfacets psychological involvement–empathy with seven items, psychological involvement–negative feelings with six items, and behavioral engagement with eight items (0.86 ≤ ω ≤ 0.93). Statements like “I sympathized with the other(s)” were rated on a five-point Likert scale ranging from “completely true” to “not true at all.” Finally, different playstyles were investigated. More specifically, fast response times along with high false answer rates could indicate a potentially risky playstyle, and slow response times with comparably high correct answer rates could indicate a thoughtful play strategy. For this, an indicator was calculated: INDIC = zCorrectAnswerRate + zResponseTime. Because of the addition, high INDIC values indicated a thoughtful strategy, whereas low values indicated a riskier playstyle.
Procedure
The experiment took place in the laboratories of the Technical University of Chemnitz. Two players participated within each session and were assigned to the conditions by drawing lots. Because of the constant group size, the participants could not guess their experimental condition beforehand. and the matchups within the HUMAN condition were randomized and naturally non-adaptive. Additionally, as the participants were unaware of the other conditions, biases could be avoided. After eliminating the outliers, the AGENT group contained 29, the LEADBRD group 29, and the HUMAN group 39 learners. First, the participants were greeted and informed about their upcoming task, in line with the APA ethical guidelines (American Psychological Association, 2010). If no questions remained, they signed a consent form and completed the pretest questionnaire. Following, they played the game, including a small tutorial section explaining the overall gameplay. The experimental phase of the game itself took, on average, 20.87 (SD = 1.57) min. Afterward, the players answered the posttest questionnaire, received 9€ or a 1.5 course credit, and were dismissed.
Results
As described within the participants section, game and animal knowledge were included as covariates. Furthermore, the AGENT, HUMAN, and LEADBRD groups were used as factor levels, and Sidak corrections will be applied to the pairwise comparisons between these groups. To foster readability, calculations regarding the test requirements were only reported if significantly violated.
Effects of Competitive Agents
Hypothesis 1
To check whether competitive agents lead to an increase in social presence compared to competition within a leaderboard, a multivariate analysis of covariance (MANCOVA) was conducted with SPGQ scores as a dependent measure. Significant main effects were found (Wilk’s Λ = 0.430), F(6,180) = 15.74, p < 0.001, = 0.34. Follow-up analyses of covariance (ANCOVAS) (Table 2) for the three subscales revealed effects within empathy and behavioral engagement, though not within negative feelings.
Further Sidak-corrected pairwise comparisons for empathy show that competition against a human opponent leads to a higher perception of empathy (p < 0.001) than competition against an artificial agent, and a higher perception of empathy (p < 0.001) compared to a leaderboard. In addition, there is no significant difference between the AGENT and LEADBRD groups. Sidak-corrected pairwise comparisons for behavioral engagement revealed that competition against a human opponent leads to a higher perception of behavioral engagement (p = 0.007) than competition against an artificial agent, and a higher perception of engagement (p < 0.001) compared to a leaderboard. In this case, the AGENT group also reported a significantly higher engagement than the LEADBRD group (p = 0.003). Therefore, H1 can be supported for behavioral engagement but not for negative feelings. Furthermore, empathy analysis revealed mixed results.
Hypothesis 2
To check whether competitive agents lead to an increase in mental strains compared to competition within a leaderboard, a MANCOVA was conducted with the ML and ME scores as dependent measures. No significant main effects were found, (Wilk’s Λ = 0.954), F(4,182) = 1.09, p = 0.36, = 0.02. The results indicate that H2 must be rejected. Additionally, descriptive data are shown in Table 3.
Hypothesis 3
To check whether competitive agents lead to differences in emotional states compared to competition within a leaderboard, enjoyment, pride, anger, shame, and hopelessness scores were used as dependent measures. As Box’s M (30, 24,443.22) = 3.203, p < 0.001, was found to be significant, Kruskal–Wallis tests were calculated for each dependent variable (Table 4). To prevent Type-I error inflation (as no overall MANCOVA could be conducted), a Sidak-corrected p threshold of 0.0102 instead of 0.05 should be applied. Therefore, only shame reached significance. A follow-up Mann–Whitney test revealed a significant difference between the HUMAN and LEADBRD conditions (U = 362.0, z = −2.74, p = 0.006) and the AGENT and LEADBRD conditions (U = 263.5, z = −2.62, p = 0.009. Overall, H3 cannot be supported.
Adaptive Competition
Hypothesis 4
To check whether an adaptive competition leads to an increase in retention compared to a real opponent, an ANCOVA was conducted with the retention scores as dependent measures. The analysis revealed a significant difference, F(2,92) = 7.92, p = 0.001, = 0.15. A further Sidak-corrected pairwise comparison of all the groups demonstrated that the group with a real opponent, MHUMAN = 30.40 (SD = 2.68), had lower (p = 0.03) retention scores than the group with the agent, MAGENT = 32.17 (SD = 2.76), and lower (p = 0.001) retention scores than the group with a leaderboard, MLEADBRD = 32.92 (SD = 2.78). The LEADBRD and AGENT groups did not differ significantly (p = 0.68). In conclusion, H4 was confirmed.
Hypothesis 5
To check whether adaptive competition leads to an increase in process efficiency compared to the HUMAN condition, a MANCOVA was conducted with the process efficiency for both ML and ME. The analysis showed a significant difference (Wilk’s Λ = 0.88), F(4,182) = 3.37, p = 0.01, = 0.07. Follow-up ANCOVAS revealed significant differences for the ML and ME process efficiency (Table 5). Sidak-corrected pairwise comparisons regarding the ML process efficiency demonstrated that the group with the agent was more efficient (p = 0.011) than the group with a real opponent, and more efficient (p = 0.014) than the group with a leaderboard. Sidak-corrected pairwise comparisons regarding the ME process efficiency also revealed that the AGENT group was more efficient (p = 0.006) than the group with a real opponent, and more efficient (p = 0.024) than the group with a leaderboard. In both analyses, the LEADBRD and HUMAN groups did not significantly differ (p = 0.99). As a consequence, H5 cannot be supported.
Additional Measures
To investigate how fast participants responded during gameplay, an ANCOVA with time as a dependent variable and the groups as independent variables was conducted. A significant effect with a high effect size was found (Table 6). Sidak-corrected pairwise comparisons revealed that participants in the LEADBRD condition responded slower than participants in the AGENT condition (p < 0.001) and HUMAN condition (p < 0.001). The AGENT and HUMAN conditions did not differ from each other (p = 0.89). The same results pattern was found, even when only the response times to the correct answers were included within the calculations, F(2,92) = 21.14, p < 0.001, = 0.32.
To investigate different playstyles, a Kruskall–Wallis H test with INDIC as a dependent measure and experimental groups as factor levels was conducted, since the Levene test was infringed, F(2,94) = 8.25, p = 0.001. A significant influence of the experimental groups on the INDIC score was found (Table 6). Follow-up Mann–Whitney tests revealed that higher INDIC values could be observed in the LEADBRD condition in comparison with the AGENT (p < 0.001) and HUMAN conditions (p < 0.001). The AGENT and HUMAN conditions did not differ from each other (p = 0.72).
To address the perceived game experience, a MANCOVA with the Game Experience Questionnaire subscales (challenge, competence, and flow) as dependent variables and the experimental groups as factor levels was performed. The results revealed a significant effect with a medium to high effect size, Wilk’s Λ = 0.75, F(6,180) = 4.56, p < 0.001, = 0.13. However, follow-up ANCOVAs demonstrated only one significant effect for competence (Table 6). Sidak-corrected pairwise comparisons revealed that participants in the LEADBRD condition reported a lower perceived competence than participants in the AGENT (p = 0.005) and HUMAN conditions (p < 0.001). The AGENT and HUMAN conditions did not differ from each other (p = 0.91).
Discussion
Overall (Table 7), the experiment demonstrated that competitive agents influenced social presence, as empathy and behavioral engagement were significantly increased when a competitive agent was present. As expected, the HUMAN condition achieved the highest scores. Regarding behavioral engagement, the AGENT group was rated higher than the LEADBRD group. In contrast, competitive agents induced no significant difference regarding ML and ME. Similarly, no stable effect on emotions could be observed. The following analysis revealed a significant influence on efficiency. However, the results did not follow the hypothesized pattern. Further exploratory investigations revealed that the participants within the competitive agent groups answered faster than those within the LEADBRD condition. Furthermore, the LEADBRD group revealed lowered perceived competence and more cautious play behavior.
Implications and Conclusion
The results regarding social presence partially supported H1, demonstrating that a higher level of social competition increases the connection between competitive entities. Additionally, it could be demonstrated that a simple depicted artificial opponent might not be enough to trigger empathy but triggers behavioral engagement nonetheless. This large effect might be especially remarkable. Players rated items measuring others’ influence on their actions higher, indicating that an opponent could be utilized to guide the learner within educational scenarios. Besides, the lack of a significant effect on negative feelings might support the assumption that opponents are useful within educational scenarios, as jealousness or malicious delight are potentially undesirable states within a classroom. In contrast to our expectations, no influence on ML or ME could be observed. This could indicate that the social cues (mostly facial) used within the experiment might not have a strong influence on the measured mental strains. This is supported by other research that states that reading non-verbal information could be categorized as biological communication (Kirschner et al., 2018), which does not induce a heavy cognitive load. Although not in line with the postulated hypothesis, the lack of significant differences might still be important for the design of educational material, especially as previous experiments indicated the potentially harmful effects of social competition on mental strains (Nebel et al., 2016b). In contrast, the presented experiment indicates that the benefits (e.g., increased behavioral engagement) of carefully implemented social cues might outweigh the potential harm (e.g., the chance of cognitive overload).
However, the sparse presentation of social cues might have additional drawbacks, as no gain in emotional reactions could be observed. Again, some effect might have been overlooked, as the direction of the descriptive differences follows a clear pattern, but the non-parametric tests reduced the test strength. The pattern of lower negative emotions within the AGENT+HUMAN conditions deserves special attention, as shame reached significance. This effect is noteworthy, as one might assume that social cues might trigger such negative emotions (e.g., feeling ashamed for performing insufficiently). Within the experiment, however, performing insufficiently on a leaderboard induced a stronger feeling of shame than an interaction with a competitively linked agent.
The implementation of adaptive elements successfully increased retention knowledge, providing a valuable method for increasing learning outcomes without needing to change the learning material itself. Thus, the experiment provides crucial evidence and further motivation to investigate and optimize adaptive elements within educational video games. However, the results revealed no difference between the adaptive and HUMAN groups on how efficiently the knowledge structures were developed. In contrast to the hypothesis, only the AGENT condition was more efficient, whereas the HUMAN and LEADBRD conditions did not differ significantly. The reason could be found within the time element. The explorative calculations of gameplay behavior revealed that participants within the LEADBRD condition took significantly more time to give their answers. Therefore, only the AGENT condition combined the advantage of being adaptive and sufficiently triggering fast learning.
This first glimpse into play behavior was accompanied by an INDIC investigation. The results further strengthen the rationale that the high score list triggered fewer social processes than the experimental counterparts, resulting in a lowered influence of the competitive elements on the learner. As a consequence, they played and learned more thoughtful but less purposefully on efficient learning. The subsequent game experience analysis demonstrated no effect regarding flow and challenge, indicating a rather similar experience. This could indicate that the effort to increase comparability and ensure internal validity was successful. However, the difference regarding competence should be addressed, especially as ensuring that the player feels competent about the topic might be important in educational settings. The results indicate that demonstrating personal skills toward a rather social competitor seems to foster a feeling of competence.
Altogether, the presented experiment provides a unique comparison of three different approaches to adaptive and social competitive gameplay, revealing individual strengths and weaknesses. The results imply that competitive agents with sufficient social cues could be used to guide learner behavior, influence play behavior, alter perceived competence, and induce acceptable demands on mental resources, if not presented too intrusively. In addition, the experiment not only highlighted the potential of adaptive mechanisms but provided insights into the differences between the implementations. Overall, the AGENT group might combine the strengths of both approaches, social competition, and adaptivity.
Limitations and Future Research
In addition to the general challenges within the measurements of cognitive strains (Korbach et al., 2017; Orru and Longo, 2019), the measures within this experiment are subject to several limitations. For example, some researchers suggest that the implications of the Cognitive Load Theory should not be applied to complex, multigoal-learning environments but rather to specific phases or activities (Kalyuga and Singh, 2016). This research tried to follow this recommendation with a narrow focus on a specific game mechanic. However, only one instructional goal was investigated (a simple retention task). If another goal is of interest (e.g., generating procedural knowledge, a creativity task), the results might differ. The study’s timespan raises further limitations, as prior studies indicated that delayed tests revealed more consistently positive effects within the context of the testing effect (Halamish and Bjork, 2011). Consequently, immediate measures might not have revealed the full impact of the learning environment. Adaption needs time to adapt to the individual player as well. Within the cold-start stage (Xue et al., 2017), the player parameters are unknown, and an optimal play experience cannot be realized. Additionally, identification with avatars emerges over time (Turkay and Kinzer, 2014). Similarly, emotional attachment and a deeper connection with artificial and social competitors and the connected effects might only develop after longer periods of gameplay. The sample inherits some limitations as well as that the appeal and importance of competitive games may vary within different cultural backgrounds. For instance, there appears to be a difference in competitive game use within moderately and highly stratified societies (Peoples et al., 2017). The economic validity is enhanced through the close orientation to common quiz games and social cues. However, some limitations must be discussed as well. For example, DDA is discussed controversially because players might feel patronized if the game adjusts the difficulty for them. As an additional consequence, this might impede competitive behavior and effects. Furthermore, if the game involves non-transparent mechanisms, self-efficiency might be negatively influenced.
Finally, the three conditions were intended to be as comparable as possible. However, some of the necessary adjustments might unintendedly influence the resulting game experience and impede the correct entanglement of cause-and-effect patterns. For instance, a leaderboard condition enabled a comparison with a larger group of individuals, whereas the other conditions did only provide one opponent, potentially decreasing the focus on performance. Additionally, negative feedback was not part of the manipulation. However, within the AGENT condition, about one-third of the participants won the game. Naturally, within the HUMAN group, 50% won the match. However, within the LEADBRD condition, the participants started, on average, on the mid-range position 25 (SD = 2.8) and finished lower on position 41 (SD = 6.4). Compared to the other groups, this could indicate that the participants felt they lost the game, leading to a reduced sense of competence. Also, it is a constant challenge for educational videogame research to create adequate comparison groups, as not all scientifically advised manipulations would result in viable games. For example, a non-adaptive LEADBRD or AGENT condition would feel arbitrary. However, clear rules and perceived impact of player effort are essential to games (Juul, 2003). Other scenarios lack external validity. For example, a fully adaptive HUMAN group would require a Wizard of Oz approach that is not feasible in a school setting. Nonetheless, future research should intensify their efforts separating the effects of difficulty adjustments and competitive agents as the mixture of the aspects of social agents and difficulty adjustments limit the interpretability of this study. Furthermore, a complex interaction like the investigated gameplay behavior might be moderated by additional variables not assessed within this experiment. For example, the effect on play behavior could be influenced by individual risk aversion.
Despite these limitations, this experiment manages to provide valid insights, especially as the learning content itself is often varied within difficulty research (e.g., Sampayo-Vargas et al., 2013), creating challenges for inferring generalizable design recommendations. However, much more research needs to be done. To gain further insights into the aspect of group size and social cues, additional variations of the AGENT group should be considered. For instance, the opponent could be replaced by a textual representation or the specific emotional responses could be varied. Additionally, it might be beneficial to vary the difficulty throughout the different gameplay phases. Reducing the difficulty during the early game phases and increasing the difficulty during later gameplay might prevent an educational game from becoming boring (Lomas et al., 2017). Furthermore, adaptive systems can be partially applied to HUMAN scenarios as well (e.g., actively assigning who plays against whom), and future research is needed if such methods could compensate for the negative effects. Beyond this, the adaptive mechanics could be used to learn something about the learning material itself. For example, if the adaptive mechanism lowers the difficulty during a specific segment of the learning material, instructors can adjust the content’s relative difficulty. This further amplifies the potential of adaptive systems within educational video games. Finally, future research might utilize additional methods of data collection (e.g., physiological measures; Nebel and Ninaus, 2019) to gather even deeper insights into the underlying processes.
Data Availability Statement
The datasets generated for this study are available on request to the corresponding author.
Ethics Statement
Ethical review and approval was not required for the study on human participants in accordance with the local legislation and institutional requirements. The patients/participants provided their written informed consent to participate in this study.
Author Contributions
SN created the material, conducted the experiments and statistical analyses, and wrote major parts of the manuscript. MB and SS wrote minor parts and contributed to proofreading. GR contributed to proofreading. All authors contributed to the article and approved the submitted version.
Funding
The publication of this article was funded by Chemnitz University of Technology. The funder was involved in paying the publication costs and was not involved in the conduction of the experiments.
Conflict of Interest
The authors declare that the research was conducted in the absence of any commercial or financial relationships that could be construed as a potential conflict of interest.
Acknowledgments
We want to thank every participant for their valuable time and effort.
References
Abuhamdeh, S., and Csikszentmihalyi, M. (2012). the importance of challenge for the enjoyment of intrinsically motivated, goal-directed activities. Personal. Soc. Psychol. Bull. 38, 317–330. doi: 10.1177/0146167211427147
Alexander, J. T., Sear, J., and Oikonomou, A. (2013). An investigation of the effects of game difficulty on player enjoyment. Entertain. Comput. 4, 53–62. doi: 10.1016/j.entcom.2012.09.001
Altimira, D., Mueller, F., Clarke, J., Lee, G., Billinghurst, M., and Bartneck, C. (2017). Enhancing player engagement through game balancing in digitally augmented physical games. Int. J. Hum. Comput. Stud. 103, 35–47. doi: 10.1016/j.ijhcs.2017.02.004
American Psychological Association (2010). Publication Manual of the American Psychological Association, 6th Edn. Washington, DC: American Psychological Association.
Aviezer, H., Trope, Y., and Todorov, A. (2012). Body cues, not facial expressions, discriminate between intense positive and negative emotions. Science 338, 1225–1229. doi: 10.1126/science.1224313
Bakkes, S., Tan, C. T., and Pisan, Y. (2012). “Personalised gaming: a motivation and overview of literature,” in Proceedings of the 8th Australasian Conference on Interactive Entertainment: Playing the System, Vol. 4, New York, NY: ACM, 10.
Bandura, A. (1977). Self-efficacy: toward a unifying theory of behavioral change. Psychol. Rev. 84, 191–215. doi: 10.1037/0033-295X.84.2.191
Bjork, R. A. (1994). “Memory and metamemory considerations in the training of human beings,” in Metacognition: Knowing About Knowing, eds A. P. Shimamura and J. Metcalfe (Cambridge, MA: MIT Press), 185–205.
Bond, C. F., and Titus, L. J. (1983). Social facilitation: a meta-analysis of 241 studies. Psychol. Bull. 94, 265–292. doi: 10.1037/0033-2909.94.2.265
Burgers, C., Eden, A., van Engelenburg, M. D., and Buningh, S. (2015). How feedback boosts motivation and play in a brain-training game. Comput. Hum. Behav. 48, 94–103. doi: 10.1016/j.chb.2015.01.038
Cagiltay, N. E., Ozcelik, E., and Ozcelik, N. S. (2015). The effect of competition on learning in games. Comput. Educ. 87, 35–41. doi: 10.1016/j.compedu.2015.04.001
Carpenter, S. K. (2009). Cue strength as a moderator of the testing effect: the benefits of elaborative retrieval. J. Exp. Psychol. Learn. Mem. Cogn. 35, 1563–1569. doi: 10.1037/a0017021
Chen, Z., Xue, S., Kolen, J., Aghdaie, N., Zaman, K. A., Sun, Y., et al. (2017). “EOMM: an engagement optimized matchmaking framework,” in Proceedings of the 26th International Conference on World Wide Web, New York, NY: ACM, 1143–1150.
Chen, Z.-H. (2014). Exploring students’ behaviors in a competition-driven educational game. Comput. Hum. Behav. 35, 68–74. doi: 10.1016/j.chb.2014.02.021
Chen, Z.-H., and Chen, S. Y. (2014). When educational agents meet surrogate competition: impacts of competitive educational agents on students’ motivation and performance. Comput. Educ. 75, 274–281. doi: 10.1016/j.compedu.2014.02.014
Cheon, J., Chung, S., and Lee, S. (2015). The roles of attitudinal perceptions and cognitive achievements in a serious game. J. Educ. Comput. Res. 52, 3–25. doi: 10.1177/0735633114568851
Clark, D. B., Tanner-Smith, E. E., and Killingsworth, S. S. (2016). Digital games, design, and learning: a systematic review and meta-analysis. Rev. Educ. Res. 86, 79–122. doi: 10.3102/0034654315582065
Cohen, J. (1988). Statistical Power Analysis for the Behavioral Sciences, 2nd Edn. Hillsdale: Lawrence Erlbaum Associates, Inc.
de Kort, Y. A. W., IJsselsteijn, W. A., and Poels, K. (2007). Digital Games as Social Presence Technology: Development of the Social Presence in Gaming Questionnaire (SPGQ). 195–203. Avaliable online at: https://astro.temple.edu/~lombard/ISPR/Proceedings/2007/de%20Kort,%20IJsselsteijn,%20and%20Poels.pdf
Dinçer, S., and Doğanay, A. (2017). The effects of multiple-pedagogical agents on learners’ academic success, motivation, and cognitive load. Comput. Educ. 111, 74–100. doi: 10.1016/j.compedu.2017.04.005
Endres, T., and Renkl, A. (2015). Mechanisms behind the testing effect: an empirical investigation of retrieval practice in meaningful learning. Front. Psychol. 6:1054. doi: 10.3389/fpsyg.2015.01054
Festinger, L. (1954). A theory of social comparison processes. Hum. Relat. 7, 117–140. doi: 10.1177/001872675400700202
Frechette, C., and Moreno, R. (2010). The roles of animated pedagogical agents’ presence and nonverbal communication in multimedia learning environments. J. Med. Psychol. 22, 61–72. doi: 10.1027/1864-1105/a000009
Gajadhar, B. J., de Kort, Y. A. W., and IJsselsteijn, W. A. (2008). “Shared fun is doubled fun: player enjoyment as a function of social setting,” in Fun and Games, eds P. Markopoulos, B. de Ruyter, W. IJsselsteijn, and D. Rowland (Berlin: Springer), 106–117. doi: 10.1007/978-3-540-88322-7_11
Giles, D. C. (2002). Parasocial interaction: a review of the literature and a model for future research. Media Psychol. 4, 279–305. doi: 10.1207/S1532785XMEP0403_04
GraphPad Software (2018). GraphPad QuickCalcs: Outlier calculator. Avaliable at: https://www.graphpad.com/quickcalcs/Grubbs1.cfm (accessed August 22, 2018).
Griffiths, R. P., Eastin, M. S., and Cicchirillo, V. (2016). Competitive video game play: an investigation of identification and competition. Commun. Res. 43, 468–486. doi: 10.1177/0093650214565895
Grubbs, F. E. (1969). Procedures for detecting outlying observations in samples. Technometrics 11, 1–21. doi: 10.2307/1266761
Halamish, V., and Bjork, R. A. (2011). When does testing enhance retention? A distribution-based interpretation of retrieval as a memory modifier. J. Exp. Psychol. Learn. Mem. Cogn. 37, 801–812. doi: 10.1037/a0023219
Hanham, J., Leahy, W., and Sweller, J. (2017). Cognitive load theory, element interactivity, and the testing and reverse testing effects. Appl. Cogn. Psychol. 31, 265–280. doi: 10.1002/acp.3324
Hartmann, T., Schramm, H., and Klimmt, C. (2004). Personenorientierte medienrezeption: ein zwei-ebenen-modell parasozialer interaktionen. Publizistik 49, 25–47. doi: 10.1007/s11616-004-0003-6
Hughes, M. G., Day, E. A., Wang, X., Schuelke, M. J., Arsenault, M. L., Harkrider, L. N., et al. (2013). Learner-controlled practice difficulty in the training of a complex task: cognitive and motivational mechanisms. J. Appl. Psychol. 98, 80–98. doi: 10.1037/a0029821
IJsselsteijn, W. A., Kort, Y. A. W., and Poels, K. (2013). The Game Experience Questionnaire. Avaliable at: https://research.tue.nl/en/publications/the-game-experience-questionnaire (accessed December 28, 2019).
Jagušt, T., Botički, I., and So, H.-J. (2018). Examining competitive, collaborative and adaptive gamification in young learners’ math learning. Comput. Educ. 125, 444–457. doi: 10.1016/j.compedu.2018.06.022
Johnson, R. T., Johnson, D. W., and Stanne, M. B. (1986). Comparison Of computer-assisted cooperative, competitive, and individualistic learning. Am. Educ. Res. J. 23, 382–392. doi: 10.3102/00028312023003382
Juul, J. (2003). “The game, the player, the world: looking for a heart of Gameness,” in Level Up: Digital Games Research Conference Proceedings, eds M. Copier and J. Raessens (Utrecht: Utrecht University), 30–45.
Kahoot!. (2018). Kahoot! | Learning Games | Make Learning Awesome!. Avaliable at: https://kahoot.com/ (accessed August 22, 2018).
Kalyuga, S., and Singh, A.-M. (2016). Rethinking the boundaries of cognitive load theory in complex learning. Educ. Psychol. Rev. 28, 831–852. doi: 10.1007/s10648-015-9352-0
Ke, F. (2016). Designing and integrating purposeful learning in game play: a systematic review. Educ. Technol. Res. Dev. 64, 219–244. doi: 10.1007/s11423-015-9418-1
Kirschner, P. A., Sweller, J., Kirschner, F., and Zambrano, R. (2018). From cognitive load theory to collaborative cognitive load theory. Int. J. Comput. Supp. Coll. Learn. 13, 213–233. doi: 10.1007/s11412-018-9277-y
Korbach, A., Brünken, R., and Park, B. (2017). Measurement of cognitive load in multimedia learning: a comparison of different objective measures. Instr. Sci. 45, 515–536. doi: 10.1007/s11251-017-9413-5
Korn, O., Stamm, L., and Moeckl, G. (2017). “designing authentic emotions for non-human characters: a study evaluating virtual affective behavior,” in Proceedings of the 2017 Conference on Designing Interactive Systems, Edinburgh, 477–487.
Krell, M. (2015). Evaluating an instrument to measure mental load and mental effort using item response theory. Sci. Educ Rev. Lett. 6:1280256.
Lomas, D., Patel, K., Forlizzi, J. L., and Koedinger, K. R. (2013). “Optimizing challenge in an educational game using large-scale design experiments,” in Proceedings of the SIGCHI Conference on Human Factors in Computing Systems, New York, NY: ACM, 89–98.
Lomas, J. D., Koedinger, K., Patel, N., Shodhan, S., Poonwala, N., and Forlizzi, J. L. (2017). “Is Difficulty overrated?: the effects of choice, novelty and suspense on intrinsic motivation in educational games,” in Proceedings of the 2017 CHI Conference on Human Factors in Computing Systems, (New York, NY: ACM), 1028–1039.
Martens, A., and Mueller, W. (2016). “Gamification—A structured analysis,” in Proceedings of the 2016 IEEE 16th International Conference on Advanced Learning Technologies (ICALT), Piscataway, NJ: IEEE, 138–142.
Mayer, R. E. (2014). “Principles based on social cues in multimedia learning: personalization, voice, image, and embodiment principles,” in The Cambridge Handbook of Multimedia Learning (2. Aufl., S, ed. Mayer, and R. (Cambridge, MA: Cambridge University Press), 345–368.
Mayer, R. E. (2018). Educational psychology’s past and future contributions to the science of learning, science of instruction, and science of assessment. J. Educ. Psychol. 110, 174–179. doi: 10.1037/edu0000195
McDonald, R. P. (1999). Test Theory: A Unified Treatment. Mahwah, NJ: Lawrence Erlbaum Associates Publishers.
McNeish, D. (2018). Thanks coefficient alpha, we’ll take it from here. Psychol. Methods 23, 412–433. doi: 10.1037/met0000144
Mozur, P. (2018). Google’s AlphaGo Defeats Chinese Go Master in Win for A.I. New York, NY: The New York Times.
Nebel, S. (2017). Investigating the Mechanisms of Competition within Educational Video Games—Die Mechanismen des Wettbewerbs in Digitalen Lernspielen. Doctoral dissertation, Technische Universität Chemnitz, Chemnitz.
Nebel, S., Beege, M., Schneider, S., and Rey, G. D. (2016a). The higher the score, the higher the learning outcome? Heterogeneous impacts of leaderboards and choice within educational videogames. Comput. Hum. Behav. 65, 391–401. doi: 10.1016/j.chb.2016.08.042
Nebel, S., Schneider, S., and Rey, G. D. (2016b). From duels to classroom competition: social competition and learning in educational videogames within different group sizes. Comput. Hum. Behav. 55, 384–398. doi: 10.1016/j.chb.2015.09.035
Nebel, S., and Ninaus, M. (2019). “New perspectives on game-based assessment with process data and physiological signals,” in Game-Based Assessment Revisited, eds D. Ifenthaler and Y. Jeon (Berlin: Springer Nature), 141–161. doi: 10.1007/978-3-030-15569-8_8
Nebel, S., Schneider, S., Beege, M., and Rey, G. D. (2017). Leaderboards within educational videogames: the impact of difficulty, effort and gameplay. Comput. Educ. 113, 28–41. doi: 10.1016/j.compedu.2017.05.011
Orji, R., Nacke, L. E., and Di Marco, C. (2017). “towards personality-driven persuasive health games and gamified systems,” in Proceedings of the 2017 CHI Conference on Human Factors in Computing Systems, Denver, CO, 1015– 1027.
Orji, R., Tondello, G. F., and Nacke, L. E. (2018). “Personalizing persuasive strategies in gameful systems to gamification user types,” in Proceedings of the SIGCHI Conference on Human Factors in Computing Systems - CHI ′18, New York, NY: ACM, 15.
Orru, G., and Longo, L. (2019). “The evolution of cognitive load theory and the measurement of its intrinsic, extraneous and germane loads: a review,” in Human Mental Workload: Models and Applications, Vol. 1012, eds L. Longo and M. C. Leva (Berlin: Springer), 23–48. doi: 10.1007/978-3-030-14273-5_3
Paas, F., Tuovinen, J. E., Tabbers, H., and Gerven, P. W. M. V. (2003). Cognitive load measurement as a means to advance cognitive load theory. Educ. Psychol. 38, 63–71. doi: 10.1207/S15326985EP3801_8
Paas, F. G. W. C., and Van Merriënboer, J. J. G. (1994). Instructional control of cognitive load in the training of complex cognitive tasks. Educ. Psychol. Rev. 6, 351–371. doi: 10.1007/BF02213420
Pekrun, R., Goetz, T., Frenzel, A. C., Barchfeld, P., and Perry, R. P. (2011). Measuring emotions in students’ learning and performance: the achievement emotions questionnaire (AEQ). Contemp. Educ. Psychol. 36, 36–48. doi: 10.1016/j.cedpsych.2010.10.002
Peoples, S. M., Hardecker, S., Watts, J., Greenhill, S., Colleran, H., and Haun, D. (2017). “The Transmission of Cultural Values via Games,” in Gemeinsame Tagung der Fachgruppen Entwicklungspsychologie und Pädagogische Psychologie (PAEPSY) 2017 presented at the Münster, Münster.
Plass, J. L., and Kaplan, U. (2016). “Chapter 7—emotional design in digital media for learning,” in Emotions, Technology, Design, and Learning, eds S. Y. Tettegah and M. Gartmeier (Cambridge, MA: Academic Press), 131–161. doi: 10.1016/b978-0-12-801856-9.00007-4
Plass, J. L., O’Keefe, P. A., Homer, B. D., Case, J., Hayward, E. O., Stein, M., et al. (2013). The impact of individual, competitive, and collaborative mathematics game play on learning, performance, and motivation. J. Educ. Psychol. 105, 1050–1066. doi: 10.1037/a0032688
Quizlet Inc (2018). Quizlet—Simple Tools for Learning Anything. Avaliable at: https://quizlet.com/. (accessed August 22, 2018)Google Scholar
Raaijmakers, S. F., Baars, M., Schaap, L., Paas, F., and van Gog, T. (2017). Effects of performance feedback valence on perceptions of invested mental effort. Learn. Instr. 51, 36–46. doi: 10.1016/j.learninstruc.2016.12.002
Revelle, W., and Zinbarg, R. E. (2008). Coefficients Alpha, Beta, Omega, and the glb: comments on sijtsma. Psychometrika 74:145. doi: 10.1007/s11336-008-9102-z
Salden, R. J. C. M., Paas, F., Broers, N. J., and van Merriënboer, J. J. G. (2004). Mental effort and performance as determinants for the dynamic selection of learning tasks in air traffic control training. Instr. Sci. 32, 153–172. doi: 10.1023/B:TRUC.0000021814.03996.ff
Salen, K., and Zimmerman, E. (2004). Rules of Play: Game Design Fundamentals. Cambridge, MA: MIT Press.
Sampayo-Vargas, S., Cope, C. J., He, Z., and Byrne, G. J. (2013). The effectiveness of adaptive difficulty adjustments on students’ motivation and learning in an educational computer game. Comput. Educ. 69, 452–462. doi: 10.1016/j.compedu.2013.07.004
Schneider, S., Nebel, S., Beege, M., and Rey, G. D. (2018). Anthropomorphism in decorative pictures: benefit or harm for learning? J. Educ. Psychol. 110, 218–232. doi: 10.1037/edu0000207
Schneider, S., Nebel, S., and Rey, G. D. (2016). Decorative pictures and emotional design in multimedia learning. Learn. Instr. 44, 65–73. doi: 10.1016/j.learninstruc.2016.03.002
Schrader, C., and Bastiaens, T. J. (2012). The influence of virtual presence: effects on experienced cognitive load and learning outcomes in educational computer games. Comput. Hum. Behav. 28, 648–658. doi: 10.1016/j.chb.2011.11.011
Schrader, C., Brich, J., Frommel, J., Riemer, V., and Rogers, K. (2017). “Rising to the challenge: an emotion-driven approach toward adaptive serious games,” in Serious Games and Edutainment Applications, eds M. Ma and A. Oikonomou (Berlin: Springer), 3–28. doi: 10.1007/978-3-319-51645-5_1
Schroeder, N. L., Adesope, O. O., and Gilbert, R. B. (2013). How effective are pedagogical agents for learning? A meta-analytic review. J. Educ. Comput. Res. 49, 1–39. doi: 10.2190/EC.49.1.a
Schroeder, N. L., and Traxler, A. L. (2017). Humanizing instructional videos in physics: when less is more. J. Sci. Educ. Technol. 26, 269–278. doi: 10.1007/s10956-016-9677-6
Seaborn, K., and Fels, D. I. (2015). Gamification in theory and action: a survey. Int. J. Hum. Comput. Stud. 74, 14–31. doi: 10.1016/j.ijhcs.2014.09.006
Sinha, P., Balas, B., Ostrovsky, Y., and Russell, R. (2006). Face recognition by humans: nineteen results all computer vision researchers should know about. Proc. IEEE 94, 1948–1962. doi: 10.1109/JPROC.2006.884093
Smith, S. P., Blackmore, K., and Nesbitt, K. (2015). “a meta-analysis of data collection in serious games research,” in Serious Games Analytics, eds C. S. Loh, Y. Sheng, and D. Ifenthaler (Berlin: Springer), 31–55. doi: 10.1007/978-3-319-05834-4_2
Spronck, P., Sprinkhuizen-kuyper, I., and Postma, E. (2004). “Difficulty scaling of game AI,” in Proceedings of the 5th International Conference on Intelligent Games and Simulation (GAMEON’2004, Belgium, 33–37.
Sweller, J. (1994). Cognitive load theory, learning difficulty, and instructional design. Learn. Instr. 4, 295–312. doi: 10.1016/0959-4752(94)90003-5
ter Vrugte, J., de Jong, T., Vandercruysse, S., Wouters, P., van Oostendorp, H., and Elen, J. (2015). How competition and heterogeneous collaboration interact in prevocational game-based mathematics education. Comput. Educ. 89, 42–52. doi: 10.1016/j.compedu.2015.08.010
Turkay, S., and Kinzer, C. K. (2014). The effects of avatar-based customization on player identification. Int. J. Gam. Comput. Med. Simul. 6, 1–25. doi: 10.4018/ijgcms.2014010101
Um, E. “R. ”, Plass, J. L., Hayward, E. O., and Homer, B. D. (2012). Emotional design in multimedia learning. J. Educ. Psychol. 104, 485–498. doi: 10.1037/a0026609
van Lankveld, G., Spronck, P., van den Herik, H. J., and Rauterberg, M. (2010). “Incongruity-based adaptive game balancing,” in Advances in Computer Games, eds H. J. van den Herik and P. Spronck (Berlin: Springer), 208–220. doi: 10.1007/978-3-642-12993-3_19
Vandercruysse, S., and Elen, J. (2017). “Towards a game-based learning instructional design model focusing on integration,” in Instructional Techniques to Facilitate Learning and Motivation of Serious Games, eds P. Wouters and H. van Oostendorp (Berlin: Springer), 17–35. doi: 10.1007/978-3-319-39298-1_2
Vandercruysse, S., Vandewaetere, M., Cornillie, F., and Clarebout, G. (2013). Competition and students’ perceptions in a game-based language learning environment. Educ. Technol. Res. Dev. 61, 927–950. doi: 10.1007/s11423-013-9314-5
Vincent, J. (2018). AI Bots Trained for 180 Years a Day to Beat Humans at Dota 2. Avaliable at: https://www.theverge.com/2018/6/25/17492918/openai-dota-2-bot-ai-five-5v5-matches (accessed December 28, 2019).
Vorderer, P., Hartmann, T., and Klimmt, C. (2003). “Explaining the enjoyment of playing video games: the role of competition,” in Proceedings of the Second International Conference on Entertainment Computing, Pittsburgh, 1–9.
Weibel, D., Wissmath, B., Habegger, S., Steiner, Y., and Groner, R. (2008). Playing online games against computer- vs. human-controlled opponents: effects on presence, flow, and enjoyment. Comput. Hum. Behav. 24, 2274–2291. doi: 10.1016/j.chb.2007.11.002
Williams, R. B., and Clippinger, C. A. (2002). Aggression, competition and computer games: computer and human opponents. Comput. Hum. Behav. 18, 495–506. doi: 10.1016/S0747-5632(02)00009-2
Xue, S., Wu, M., Kolen, J., Aghdaie, N., and Zaman, K. A. (2017). “Dynamic difficulty adjustment for maximized engagement in digital games,” in Proceedings of the 26th International Conference on World Wide Web Companion, New York, NY: ACM, 465–471.
Yeo, G., and Neal, A. (2008). Subjective cognitive effort: a model of states, traits, and time. J. Appl. Psychol. 93, 617–631. doi: 10.1037/0021-9010.93.3.617
Yong, Y. (2018). EA Wants to Get Rid of Fair Matchmaking to Focus on Player Spending & Engagement. Avaliable at: https://www.youtube.com/watch?v=oC19cGJa-xw (accessed January 3, 2019)Google Scholar
Keywords: game-based learning, educational video games, serious games, artificial social competition, adaptivity, dynamic difficulty adjustment
Citation: Nebel S, Beege M, Schneider S and Rey GD (2020) Competitive Agents and Adaptive Difficulty Within Educational Video Games. Front. Educ. 5:129. doi: 10.3389/feduc.2020.00129
Received: 28 December 2019; Accepted: 29 June 2020;
Published: 21 July 2020.
Edited by:
Meryem Yilmaz Soylu, University of Nebraska–Lincoln, United StatesReviewed by:
Mei Yii Lim, Heriot-Watt University, United KingdomKazunori Terada, Gifu University, Japan
Copyright © 2020 Nebel, Beege, Schneider and Rey. This is an open-access article distributed under the terms of the Creative Commons Attribution License (CC BY). The use, distribution or reproduction in other forums is permitted, provided the original author(s) and the copyright owner(s) are credited and that the original publication in this journal is cited, in accordance with accepted academic practice. No use, distribution or reproduction is permitted which does not comply with these terms.
*Correspondence: Steve Nebel, c3RldmUubmViZWxAcGhpbC50dS1jaGVtbml0ei5kZQ==