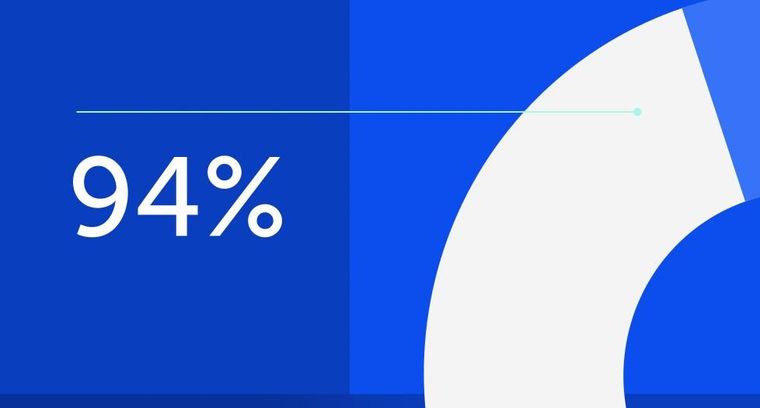
94% of researchers rate our articles as excellent or good
Learn more about the work of our research integrity team to safeguard the quality of each article we publish.
Find out more
ORIGINAL RESEARCH article
Front. Educ., 16 July 2020
Sec. Educational Psychology
Volume 5 - 2020 | https://doi.org/10.3389/feduc.2020.00126
Argumentation competence is an essential skill to be acquired in university education. However, there is a lack of advanced argumentation competence even for graduate students. To foster argumentation competence, typical interventions focus on example-based learning. Another approach is learning from advocatory errors. The combination of both approaches is presenting examples of erroneous arguments. Drawing on the concept of case-based learning, we developed a learning intervention that presents examples of argumentation errors in story-based designs, i.e., the erroneous examples are embedded in a story featuring the argumentation between two persons in an authentic setting. In this contribution, we report the results of two studies. In a first study, we compared an experimental condition receiving a story-based learning intervention with a control condition without a learning intervention. We found that learning from advocatory errors in a story-based design fosters students’ argumentation competence. In a second study, we compared two forms of instructional support (elaboration vs. testing prompts) against a control condition without instructional support. There was a significant increase in argumentation competence in both conditions with instructional support but not in the control condition. The results also support the cautious conclusion that elaboration prompts seem to be more effective than testing prompts. Overall, the results from both studies indicate that the story-based design is apt to foster students’ argumentation competence. We also considered the impact of prior argumentation competence and found in both studies that the present level of argumentation competence is a factor determining the argumentation competence after learning.
Acquiring scientific argumentation competence is a major goal of higher education study programs (Dietrich et al., 2015). In general, argumentation refers to the use of arguments for the sake of supporting a certain claim with a reason to persuade others of the claim’s validity (Lumer, 2007). In this way, Van Eemeren et al. (1996, p. 5) define argumentation as “a verbal and social activity of reason aimed at increasing (or decreasing) the acceptability of a controversial standpoint for the listener or reader by putting forward a constellation of propositions intended to justify (or refute) the standpoint before a rational judge.” An argument itself is a proposition consisting of a claim that is supported by a reason (Toulmin, 1958; Booth et al., 2008). In scientific contexts, we consider argumentation as the use of scientific evidence to support a claim to convince others of the claim’s validity. From a normative perspective, the main source of scientific evidence is the body of scientific knowledge that is (more or less) ratified by the scientific community and consists of either scientific theories, models, concepts, or empirical findings that result from the application of scientific methods. Usually, supporting evidence has to be integrated into a complex network of related scientific knowledge and has sometimes to be successfully de-contextualized (cf., Stark et al., 2009, p. 52). Besides, arguments are in part domain-specific (cf., Fischer et al., 2014). This notion dates back to the work of Toulmin (1958), who noted that some aspects of arguments may vary from discipline to discipline (Toulmin used the term field), whereas other aspects of arguments do not depend on the discipline. This is because some aspects of an argument may depend on contextually shared assumptions. Such assumptions are given, e.g., by a scientific community whose members put forward norms to which arguments must adhere. These argumentation norms, either stipulated by the scientific community or by substantial or logical requirements, suggest how scientific evidence is correctly used to support a claim. Besides such normative issues, arguments appear in two general types. Britt and Larson (2003) distinguish between claim-first and reason-first arguments which are hereafter referred to as Type 1 and Type 2 arguments (cf., Von der Mühlen et al., 2019). In Type 1 arguments the claim is mentioned first and followed by the supporting evidence. For example, in the argument “Extrovert people are more likely to have social contacts, because studies have found a statistically significant higher amount of social contacts with strangers for extrovert than for introvert people.” the claim about the social contacts is presented before the supporting evidence is mentioned. In contrast, in Type 2 arguments the supporting evidence is presented before the claim. For example, the former example in Type 2 form is “Studies found that extrovert people show a statistically higher amount of social contacts with strangers than introvert people, thus extroverts are more likely to have social contacts with strangers.” In the context of a competency-based approach, the use of scientific evidence to support claims can be described as a domain-specific disposition (cf., Klieme and Leutner, 2006; Dietrich et al., 2015) that is acquired within a study program. Thus, the correct use of argumentation is a competence which is hereafter referred to as argumentation competence. However, argumentation competence is mostly not explicitly taught resulting in students – and even graduates and experts – showing deficient arguments (cf., Astleitner et al., 2003). In traditional university education, especially in sciences like psychology and other social sciences, the traditional academic courses are not tailored to foster argumentation competence, and it cannot be expected that argumentation competence arises as a kind of epiphenomenon (Stark et al., 2009). As summarized in Fischer et al. (2014), students show deficits in the use of scientific evidence, make claims without any justification, or do not use scientific concepts to support their claims (Sadler, 2004). Other deficits refer to the quality of the arguments (Kelly and Takao, 2002) or the acknowledgment of different perspectives on the same topic (Sadler, 2004).
From a normative perspective, by defining an error as a deviation from a given norm (Mehl, 1994), the deficient use of arguments constitutes an error. In the case of argumentation, the error consists in violating the argumentation norms that govern the correct use of scientific evidence resulting in erroneous arguments. Stark (2005) classified students’ erroneous arguments into three different types. The first error type describes the erroneous reference to non-scientific everyday bodies of knowledge like, e.g., everyday observations and private experiences, beliefs, or implicit theories. An example of an erroneous argument of this type would be the justification of a claim about exam nerves with the report of their own classroom experience. The second error type describes the inappropriate choice of scientific theories, models, or concepts in the justification of a claim. An example of this error type would be the justification of claims about long-lasting knowledge restructuring processes with the theory of mental models, which are better explained with schema theory. In this example, an inappropriate theory is used for justification. Although both theories relate to memory processes, mental models are thought to cover short-term memory processes, e.g., in sentence comprehension, but not long-lasting knowledge restructuring processes for which schema theory is more appropriate. Besides using an inappropriate theory, erroneous arguments can also encompass essentially appropriate theories in combination with theories or models that fit better to the current claim. An example would be the use of Heider’s (1958) distinction between internal and external attribution processes to support an assertion about an attribution process whereas Kelley’s (1973) covariation principles would provide better support because this theory is more fine-grained. The third error type describes the erroneous reference to empirical findings. Examples for this may be the misinterpretation of correlational findings in terms of causality.
The findings regarding students’ deficits in argumentation competence suggest that interventions targeting the various argumentation errors are indicated. There is a vast literature focusing on intervention strategies relating to various instructional approaches and study types like, e.g., experiments and field studies, see Fischer et al. (2014) for an overview of recent studies. Two examples of recent studies are the contributions of Hefter et al. (2014) and Von der Mühlen et al. (2019). Drawing on the instructional method of example-based learning and the self-explanation principle (cf., Renkl, 2014), Hefter et al. (2014) developed a short-term intervention that fostered students’ argumentation skills. Von der Mühlen et al. (2019) developed an intervention based on the concept of constructivist learning environments (Jonassen, 1999). An instructional approach that focuses directly on possible errors is Oser’s (2007) learning from errors-approach. A special form of Oser’s (2007) approach is learning from advocatory errors in which learners are not required to make errors themselves. Instead, learning may occur by observing others making errors and receiving the correct solution. Wagner et al. (2014) demonstrated that learning from advocatory errors fosters preservice teacher’s argumentation competence. For instance, their findings suggest that, given appropriate instructional support, learning from advocatory errors enables teacher students to argue about problems like exam nerves drawing on scientific theories or models. Thus, learning from advocatory errors seems to be a promising approach to foster argumentation competence (cf., Klein et al., 2017).
In the following sections, we provide the theoretical foundations of learning from advocatory errors, i.e., the notion of negative knowledge, avoidance strategies, as well as the necessary prerequisites. After that, we elaborate on the use of learning from advocatory errors in fostering argumentation competence. Drawing on this, we develop the foundations for the kind of learning intervention we are going to propose in this contribution, i.e., a story-based design consisting in a combination of principles of example-based learning and anchored instruction. Regarding the learning intervention, we finally comment on a defining feature of the proposed intervention, i.e., the presentation of avoidance strategies as heuristics. To conclude the introduction section, we elaborate on two important issues that must be taken into account when studying the effects of learning interventions. The first issue refers to the form of instructional support that is necessary for successful learning from advocatory errors. The second refers to the role of prior knowledge for learning and evaluating the outcome of learning interventions.
Learning from advocatory errors is, as already mentioned, a special form of learning from errors that draws on the same basic learning process and preconditions. In the following, we firstly present the processes and conditions as well as the outcomes of learning from errors and elaborate on the peculiarities of advocatory learning from errors.
In learning from errors, learners’ performance of a task yield outcomes that do not fulfill a given norm (Mehl, 1994). The violation of a norm constitutes an error. Under the conditions that the learner becomes aware of and understands the error, and has the motivation to correct the error, learning progress is possible (Oser, 2007). The actual learning consists in the comparison of the error with the correct solution (cf., Wagner et al., 2014). As a result, the error is not discarded from memory, instead it is stored together with the correct solution. Thus, learning from errors results in the acquisition of negative knowledge, i.e., knowledge about what is wrong and what is to be avoided during task performance (Gartmeier et al., 2008). Avoidance strategies, i.e., strategies to avoid the error in the future, are an integral part of negative knowledge. The acquisition of negative knowledge and especially the acquisition of avoidance strategies should decrease the probability of committing the error in the future.
In learning form advocatory errors, learners acquire negative knowledge when observing the errors of others (Oser, 2007). This concept is similar to Bandura’s (1977) social-cognitive learning theory and draws on the notion that learning can occur by observing the social environment. More importantly, in Oser’s (2007) concept, the social environment refers not only to actual persons but is extended to include fictive actions, e.g., stories, novels, movies, etc. Stated otherwise, learning from advocatory errors can occur in an extended social environment. A necessary condition to enable learning from advocatory errors is the identification of the learners with the person making the error. This requirement is analogous to the requirement of an identification of the learners with the model in social-cognitive learning theory (Bandura, 1977). The context in which the error occurs should also be relevant for the learners (Oser, 2007). In the context of argumentation competence, learning from advocatory errors consists in observing erroneous arguments and their correction. Learning from advocatory errors has the advantage that it does not require the learners to commit a specific error. From an instructional point of view, this concept allows presenting specific errors to learners for which negative knowledge and avoidance strategies should be acquired.
Presenting specific errors instantiates a form of instructional means to trigger learning processes and may be considered as a form of learning from examples (Kopp et al., 2008). Such erroneous examples may especially be suited to foster conceptual understanding in the learning domain (Booth et al., 2013). Erroneous examples also represent a special form of case-based learning (e.g., Williams, 1992). Jonassen and Hernandez-Serrano (2002) state that cases, in the sense of instances of a paradigmatic example, are used in analogical reasoning when problems of the same type are present (cf., Kolodner, 1997). Such cases in turn can be presented in the form of stories. In combination with the notion of the extended social environment, these stories can be fictive. Therefore, we will hereafter use the terms story-based design to denote a fictive story containing an erroneous argumentation.
Regarding the content of the examples, Stark’s (2005) classification of argumentation errors provides a systematic presentation of common erroneous arguments. Additionally, as an argument is always related to specific content, such examples are cases of so-called double-content examples (Schworm and Renkl, 2007) consisting of two domains, i.e., the learning-domain and the exemplifying-domain. In this contribution, the learning-domain represents knowledge about argumentation errors whereas the exemplifying-domain represents knowledge about the content that is used to demonstrate the errors. Double-content examples have been successfully used in research on fostering argumentation competence (e.g., Schworm and Renkl, 2007; Hefter et al., 2014; Klopp and Stark, 2018).
To foster argumentation competence, a possible story-based design consists of a dialogue in which two persons are involved in argumentative discourse. To ease the identification of the learners with the protagonists shown in the story, learners and protagonists should be similar with respect to some relevant characteristics (cf., Bandura, 1977). For instance, when psychology students’ argumentation competence is to be fostered, the protagonists should also be psychology students. Additionally, drawing on principles of anchored instruction (Cognition and Technology Group at Vanderbilt, 1992), the story should be placed in an authentic setting to enhance the learner’s motivation. In this dialogue, the first protagonist uses an erroneous argument that, in turn, is corrected by the second protagonist. By correcting the erroneous argument, the learners (the reader of the dialogue) gain negative knowledge and avoidance strategies. This story of two psychology students could be narrated in the frame of a university lesson ensuring the relevance condition and the authenticity of the dialogue. Such a story-based design has been shown to foster the argumentation skills of students in the domain of education (Stark et al., 2009). Although the story-based design in this study did not directly feature advocatory errors, the learning intervention contained a special “elaboration tool.” Within this tool, argumentation errors according to Stark’s (2005) classification were modeled and it was demonstrated how scientific theories, models, and concepts as well as empirical evidence is applied to support the claim. The elaboration was also implemented in a story-based design.
However, as it is well-known in example-based learning (e.g., Renkl, 2014), providing examples does not ensure positive learning outcomes. As the story-based design is an instantiation of an example and thus simply providing an authentic and relevant story does not ensure successful learning. The drawback of example-based learning is triggering a shallow instead of a deep elaboration (Chi et al., 1989; Renkl, 2014). Examples are also conducive to illusions of understanding. To counter these effects, instructional support, e.g., in forms of prompts, is necessary.
As a part of negative knowledge, avoidance strategies are an important result of learning from advocatory errors. From an instructional point of view, the presentation of avoidance strategies is vital. A possible format to present avoidance strategies is heuristics. Heuristics are experience-based principles that help to solve analogous problems by ignoring unimportant information and focusing on relevant information (cf., Gigerenzer and Zimmer, 2014). Heuristics are cognitive devices that allow fast and reliable judgment (Gigerenzer and Brighton, 2009). Thus, avoidance strategies in the form of heuristics may especially be suited to provide a rule for the learner to avoid the error in the future. For example, a specific error from Stark’s (2005) classification is interpreting correlational findings in terms of causality. An erroneous argument with this error is the support of a claim about a causal relation with a correlational result. A possible heuristic to avoid this error would be: “Note that correlation does not equal causality!” In the context of argumentative reasoning, Wenglein et al. (2015) have shown that heuristics are beneficial for the use of correct evidence in argument construction. However, as the avoidance strategy is part of the example, it is also prone to shallow elaboration and illusions of understanding.
As it is well-known from previous studies (e.g., Kopp et al., 2008; Wagner et al., 2014), learning interventions drawing on learning from advocatory errors need instructional support to be effective. In the research of learning from examples, it is an established finding that instructional support is necessary to overcome shallow elaborations and illusions of understanding (Renkl, 2014). As the main part of the learning process consists in the elaboration of the contrast between the error and the correct solution, it is likely that the quality of the learners’ self-explanations of this contrast influences learning outcomes (Wagner et al., 2014). To foster argumentation competence prompts should refer to the argumentation principles to be learned, i.e., in this context the error and the avoidance strategy (Schworm and Renkl, 2007). Besides the prompts, Kopp et al. (2008) demonstrated that elaborated feedback explaining specifics of the error and the correct solution is necessary to ensure learning success.
The study of Wagner et al. (2014) indicated that the learning outcome is better when the instructional support refers to both, the presentation and the reconstruction of avoidance strategies. In this study, the presentation prompt contained a short description of the avoidance strategy. The participants were instructed to reflect on these strategies. Reconstruction prompts contained an open question to describe the avoidance strategy. Besides, feedback in the form of a sample solution was given. The prompts have in common that they firstly rehearse the avoidance strategy. Secondly, they prompt an elaboration of the avoidance strategy’s content associated with an elaboration of the contrast between the error and the correct solution. Wagner et al. (2014) experimentally varied the presentation of these prompts. In total, there were five conditions: a first condition with the presentation of both prompt types, a second and third condition with either the presentation or reconstruction prompts, respectively, a fourth condition in which the intervention without any prompts was given and a fifth control condition. The results indicate that in the first condition the learning outcome was highest, whereas the learning outcomes were almost equal in the second and third conditions, and lowest in the fourth condition. In the control condition, there was no learning gain at all. These results suggest that the elaboration triggered by the prompts is necessary for learning. Additionally, the results suggest that the more support triggering elaborations on the learning content is available, the larger the learning gain.
In the case of the Wagner et al. (2014) study, the elaboration triggered by the prompt requires that the learners retrieve the acquired negative knowledge and reflect on how to avoid the error. Another way to elicit retrieving negative knowledge and avoidance strategies and to initiate elaboration is by means of the testing effect. The testing effect means learning is improved when a test is taken on the previously learned content (e.g., Endres and Renkl, 2015). Thus, when prompts are designed according to the testing effect, they should ask participants questions regarding the previously learned content of the learning domain. The testing effect builds on the elaborative retrieval theory (cf., Carpenter, 2009; Halamish and Bjork, 2011; Rowland, 2014), which features two main cognitive processes. The first process is the retrieval induced by working on a testing question that elicits spreading activation in associative memory that in turn strengthens existing associations and builds up new associations to close memory content. The second process is the degree of semantic elaboration and the mental effort invested to that end. Especially the second process explains that a challenging task leads to more mental effort and consequently to more activation and finally, better learning outcomes (Endres and Renkl, 2015). Consequently, the testing task, i.e., in the context of this contribution the prompts, should require mental effort to be effective, and additionally, feedback about the correct response should also be given. Thus, testing on the content in the learning domain is also a promising way to construct prompts and the question arises what kind of prompts are more efficient in fostering learning from advocatory errors.
Besides the question of the effectiveness of different prompts, there is also the question of the quality of the elaboration of the prompts. Learners may either work on the prompts superficially or deeply. In the context of learning with examples, Chi et al. (1989) showed that successful learners produced higher quality self-explanations than unsuccessful learners. Stark (1999) and Renkl (2014) also demonstrated that the quality of the self-explanation fosters learning success. The question arises if the quality of the prompt elaboration has any effects on learning outcomes. As the prompts are tasks, a possible measure of the quality of task elaboration is if the task is solved correctly or not. Thus, do learners that perform better on the prompt tasks also have higher learning gains?
A common finding in educational psychology is the importance of prior knowledge for learning (cf., Dochy, 1992; Hattie, 2009). Prior knowledge that is available to a learner before the learning task is the best predictor for future learning outcomes. It also determines to which information attention is paid to, which aspects are regarded as important, and what is understood (e.g., Alexander, 1996). The importance of prior knowledge was shown in several studies implementing example-based learning (c.f., Stark, 1999). Große and Renkl (2007) showed that prior knowledge is also important for learning with erroneous examples because it supports effective self-explanation processes resulting in higher learning outcomes. Prior knowledge also supports the learners’ understanding of what is wrong in a given situation and why it is wrong and therefore the potential of learning from errors can fully unfold (Siegler, 2002). As stated by Oser and Spychiger (2005), presenting errors only provides an opportunity for learning when the learner fully understands the error.
With regard to prior knowledge, the form of double-content examples implies that a distinction between prior knowledge concerning the learning domain and the exemplifying domain must be made. Concerning the learning domain, prior knowledge about argumentation errors is of primary relevance. The present level of argumentation competence, as a kind of applied knowledge, is a possible measure. Results of Von der Mühlen et al. (2019) suggest that prior argumentation competence affects learning gains. Concerning the content domain, domain-specific content knowledge could be relevant. For instance, for errors concerning the use of statistical results, prior knowledge in statistics may matter in understanding the argumentation error. Klopp et al. (2013) presented evidence that domain-specific prior knowledge is a factor determining the results of learning from advocatory errors. Taken together, the well-known importance of prior knowledge and the empirical evidence for its relevance in learning from errors and in learning argumentation skills imply the following: Firstly, the present level of argumentation competence should be controlled for and secondly, prior knowledge concerning the exemplifying domain should also be controlled for.
The present contribution has three goals. The first goal is a proof-of-concept, i.e., if a learning intervention in the form of the above presented story-based design in combination with instructional support fosters argumentation competence. The second goal is to examine the effectiveness of different kinds of instructional support in fostering argumentation competence. Because of the domain-specificity of argumentation, we refer to the domain of psychology and the argumentation competence of psychology students. The third goal is to investigate if the quality of elaboration of the instructional support measures is related to learning outcomes. To reach these three research goals, we conducted two studies.
To reach the first goal, we investigated in a first study if learning from advocatory errors provided in a story-based design fosters argumentation competence. In this Study 1, we compared an experimental condition (learning intervention) with instructional support in form of elaboration prompts with a control condition. Study 1 thus serves as a proof-of-concept of the story-based design and as a first evaluation of the learning intervention.
To reach the second goal, we investigated in a second study the effectiveness of two methods of instructional support, i.e., we compared learning outcomes of instructional support in form of elaborations prompts as in the study of Wagner et al. (2014) with testing prompts. In Study 2, we compared two experimental conditions that received a learning intervention with different types of instructional support with a control condition that received the learning intervention without any instructional support.
Concerning the third goal, we examined in both studies the effects of the instructional support on the learning outcomes, i.e., we investigated the relation of the quality of the prompt elaboration with the argumentation competence after the intervention.
Both studies have in common that they consider the same basic type of learning intervention. In Study 1, the focus is on a proof-of-concept of the story-based design. Therefore, the learning intervention in Study 1 is only compared with a control condition receiving a bogus intervention. Additionally, the learning intervention contained elaboration prompts whose effectiveness was evaluated before (see section “Introduction”; Wagner et al., 2014). In contrast, in Study 2 the focus is on the evaluation of different types of instructional support, i.e., elaboration vs. testing prompts. Therefore, in Study 2, two learning interventions with corresponding prompts were compared with a version of the learning intervention without prompts. Besides these main goals, we also control for possible effects of prior knowledge, i.e., in the context of these two studies the level of argumentation competence before the intervention and domain-specific prior knowledge. In this way, the effects of prior knowledge are exploratively scrutinized in each study.
As already mentioned before, the goal of Study 1 is to investigate if the story-based design is apt to foster argumentation competence by means of learning from advocatory errors. Additionally, in the experimental condition, the question if the quality of prompt elaboration is related with the argumentation competence after taking the intervention is investigated.
Drawing on the case-based story design presented above, we developed an intervention that features fictive, argumentative discourses in the form of a dialogue between two psychology students. The intervention was implemented in a computer-based version using the Sosci software (Leiner, 2019). In the following, the two psychology students are referenced as Protagonist 1 and Protagonist 2. Each of these discourses refers to one error type according to the classification of Stark (2005) and contains an example for a Type 1 and Type 2 argument. The discourses are structured as follows: In the first part of the dialogue, Protagonist 1 makes an erroneous Type 2 argument. In turn, Protagonist 2 points out that the argument contains an error and explains why the presented evidence does not support the claim. Protagonist 2 also presents an avoidance strategy in the form of a heuristic. Afterward, an elaboration prompt targeting the explanation of the erroneous argument is presented to the learner. In the second part of the dialogue, Protagonist 2 provides an erroneous Type 1 argument. Now, Protagonist 1 points out that Protagonist 2 made a violation of the heuristic he shortly provided before. Then Protagonist 1 explains why the argument of Protagonist 2 violated the heuristic. Afterward, an elaboration prompt targeting the application of the avoidance strategy is presented. Figure 1 provides an overview of the structure of the dialogues. In total, seven of these dialogues were presented. At the end of the intervention, a summary of all avoidance strategies was provided.
The content of the intervention targeted the error types presented by Stark (2005). According to the number of dialogues, seven specific errors were considered. Table 1 provides an overview of the errors, their respective type and contains a description of each error. To avoid effects of prior knowledge in the exemplifying domain, the content presented in the dialogues was fictive, i.e., we did not use real psychological work to construct the erroneous and correct arguments. However, all examples draw on general psychological knowledge and when empirical findings were presented, we used simulated data.
Elaboration prompts were given in the form of multiple-choice-tasks. Each prompt had three response alternatives of which one was the correct solution. To induce deep elaboration, the answer options were carefully designed so that all alternatives were plausible and the participants had to reflect the different options to find the correct one. The prompts were provided after the first and second parts of the dialogue. The first prompt asked how the error and the avoidance strategy are related (and therefore also relates indirectly to the contrast of the error and the correct solution), whereas the second prompt referred to the application of the avoidance strategy. The participants had to answer the elaboration prompts, otherwise they could not continue in the learning intervention. Drawing on Kopp et al. (2008), we provided feedback for each prompt. The participants received the correct answer and a short explanation of the answer and were instructed to compare their response with the correct response. In the control condition, a bogus intervention was used containing a text about the history of psychology. Integrated into the text were fake-prompts, i.e., prompts that asked the participants a question regarding the content they read. Feedback of the correct response to each prompt was supplied. The text in the bogus intervention did not refer to issues about argumentation and was approximately as long as the text of the learning intervention.
We hypothesize that (H1) learning from advocatory errors fosters argumentation competence. Because of the relevance of prior knowledge, we hypothesize that argumentation competence in the pretest has an impact on the argumentation competence in the posttest (H2), i.e., we will consider pretest argumentation competence to account for its effects. Additionally, as many of the argumentation errors in the intervention referred to statistical methods, we expect that prior knowledge in statistics will have an impact on argumentation competence in the pretest and the posttest, respectively (H3). Thus, prior knowledge in statistics will again be included in the model to account for its effects. Although not directly related to the research question put forward above, the hypotheses H2 and H3 are a kind of auxiliary hypotheses that serve to control for the effects of prior knowledge. From an explorative perspective, H2 and H3 enable us to scrutinize the effects of prior knowledge. Regarding the possible effects of the quality of prompt elaboration, we hypothesize that the quality of the prompt elaborations is positively related to the argumentation competence after the intervention (H4).
In total, N = 45 psychology students from a Southwestern German university participated in Study 1 (34 females). The mean age was 22.82 years (SD = 2.77). On average, the participants were in the fourth semester (MSemester = 4.33, SD = 2.92). The subjects were recruited through social networks and billboard postings. They received a 2 h time credit for the required participation in psychological experiments. The participants were randomly allocated to an experimental condition (EC; NEC = 25) and a control condition (CC; NCC = 20). The slightly different participant numbers in the EC and CC resulted from participants that agreed to take part and were allocated to a condition but did not show up. The experiment took place in group sessions with 10 participants at maximum. However, the participants worked on their own with the learning material.
Participants in the experimental condition received the learning intervention whereas the participants in the control condition received the bogus training intervention. The interventions were provided in the form of a computer program. The program’s design required the participants to fill in all required tasks, otherwise, they could not continue. So, no missing values are in the data set. A session was scheduled for 2 h, including pre- and posttest and the intervention. To ensure ecological validity, the time was not restricted. However, no participant exceeded the scheduled 2 h (Mtime = 79.39 min, SDtime = 20.07, Maxtime = 106.22).
The procedure was as follows: First, the participants answered some demographic questions and then took a test assessing prior knowledge in statistics. Afterward, the participants worked through the argumentation competence pretest. Next, in the EC the learning intervention, and in the CC the bogus intervention was presented. Finally, the participants took the argumentation competence posttest and answered four items measuring subjective learning success. The participants worked self-paced through all parts of the study. A student research assistant was present to answer participants’ general questions about the procedure but they did not answer any questions regarding the content of the intervention. Figure 2 gives a depiction of the procedure (in both studies).
Argumentation competence in the pre- and posttest (ACT1 and ACT2) was assessed by means of a multiple-choice-test. The argumentation competence test covered type 1 and type 2 arguments. Each item had three answer options with one option being the correct argument and an “I don’t know” option. The sequence of the answer options was randomized, only the “I don’t know” option was always the last option. The sequence of the items was the same for all participants. Because type 1 and type 2 arguments differ and require a different instruction, the test was split with regard to the argument types. The items were designed to cover the topics and errors presented in the learning intervention. The answer options were designed in such a way that the two incorrect options reflected possible errors. The pre- and posttest did not contain the same items but were parallelized with regard to the item content. As in the intervention, the items in the ACT did not draw on real psychological examples but used constructed, fictive examples based on general psychological knowledge. Empirical findings in the items were constructed using simulated data.
The pre- and posttest contained 19 items in total (the Supplementary Material contains an example item. In each test, 15 items referred to type 1 arguments and four items to type 2 arguments. Both subscales were added to a total score as a measure of overall argumentation competence. Each correct answered item was scored with a 1, so that the sum reflects the number of correctly answered items. The “I don’t know” option was counted as an incorrect answer. The maximum number of points is 19. Criterion-referenced reliability of the test was calculated with the method provided in Subkoviak (1976) that yields a coefficient of agreement cr that ranges from 0 to 1. The criterion-referenced reliability of the pretest was crACT1 = 0.75 and of the posttest crACT2 = 0.85.
Because some errors in the learning domain referred to the use of statistical procedures (see Table 1), we assessed prior knowledge in statistics by means of a multiple-choice-test. The test consisted of 21 items covering basic statistical subjects such as samples, correlation and causality, effect size, significance, and the association of sample size and significance. Each item had three answer options with one option being the correct answer and an “I don’t know” option. The sequence of the answer options was randomized, only the “I don’t know” option was always the last option. The sequence of the items was the same for all participants. Each correct answered item was scored with a 1, an incorrect answer with a 0, so that the sum reflects the number of correctly answered items. The “I don’t know” option was counted as an incorrect answer. The criterion-referenced reliability for the prior knowledge in statistics test was crPKS = 0.73 (the Supplementary Material contains an example item).
To measure the prompt elaboration, the answer to the multiple-choice prompts during the intervention was recorded. A correct answer was coded as 1 point and a false answer was coded as 0 points. To get an overall measure of prompt elaboration, we calculated the sum score. As only the EC received the intervention, this score was only calculated for participants in the EC. A correct answer to the prompt was scored as 1, an incorrect answer was scored as 0. Afterward, the sum score was calculated. Because there are two prompts in each of the seven dialogues, the maximum number of points is 14.
Perceived learning success was measured by means of four self-constructed items in combination with a six-point rating scale (the items are provided in Supplementary Material). The theoretical range of the SLS scale is 4–24. Internal consistency in terms of Cronbach’s α was.93. Subjective learning success is used as a manipulation check, so there should be a significant difference between EC and CC.
The data were analyzed by means of linear models. Additionally, we used t-tests. To check the internal validity of the results, we used a linear model with ACT1 as the dependent variable and EC and PKS as explanatory variables (LM1). Additionally, we compared SLS between the experimental conditions as a kind of manipulation check with a t-test. With regard to the intervention, we set up a linear model with ACT2 as the dependent variable and with EC, PKS, and ACT1 as explanatory variables (LM2). In these models, the experimental condition was entered as a dummy-coded variable with the reference group being the CC. To test if there is a change in ACT in each condition, we set up a third linear model (LM3), in which the difference between ACT2 and ACT1 in regressed on the EC and PKS. For LM3, we compute the estimated marginal means (EMM; Searle et al., 1980). The EMM represent the mean difference predicted by LM3. By means of a Wald test, we test if the EMM differ from zero, i.e., we test if the mean difference in each condition is different from zero. The p-values for this test are adjusted to avoid α-error inflation. In the last linear model, LM4, we regress ACT2 on QPE while controlling for ACT1 and PKS, i.e., QPE, ACT1, and PKS are the explanatory variables in the model. Because only the participants in EC2 received the intervention with the prompts, LM4 is only calculated for this subsample. Regarding possible interactions, we checked for all possible interaction terms between the explanatory variables. If an interaction proved significant, the interaction term and all lower-order terms were included in the model, non-significant interaction terms were discarded (cf., Fox, 2016).
The various linear models relate to the research hypothesis. LM2 is to scrutinize the hypothesis about the learning intervention H2, whereas LM4 is to scrutinize the hypothesis H4 about the effects of the prompt elaboration. Regarding the auxiliary hypotheses H2 and H3, hypothesis H3 is incorporated in each linear model. Hypothesis H3 is not considered in LM3 because in this model the difference between ACT2 and ACT1 is regressed on EC and PKS and consequently, ACT1 cannot be controlled for by entering as a covariate. Figure 3 provides an overview of the analysis step and the various linear models and other statistical used in each step. Additionally, Figure 3 provides an overview of the statistical analysis and also shows to which hypothesis each analysis step relates.
Figure 3. Analysis steps in each study. The figure shows the statistical models and corresponding hypotheses in each analysis step. The linear models are provided in a simplified form, the left-hand side indicates the dependent variables and the right-hand side the regressors, variable names are the same as in “Materials and Methods” section in both studies respectively. Note that in this figure the experimental condition (EC) in Study 2 is considered in a generic form as a single variable and not as a dummy-coded variable with two manifestations. Further abbreviations: EMMs: estimated marginal means, GLH: general linear hypothesis.
As a measure of the effect size of each explanatory variable, we used η2 (cf., Richardson, 2011). We consider η2-values of 0.01, 0.06, and 0.14 as small, medium, and large effects, respectively. Additionally, for the change in argumentation competence between the pre-and posttest, we use Cohen’s d as a measure of effect size, with 0.20 being a small, 0.50 being a medium, and 0.80 being a large effect (cf., Cohen, 1988). Moreover, we use a Monte Carlo simulation to investigate if the reduction in argumentation competence in the control condition (see the “Results” section) affected the conclusion about the effectiveness of the learning intervention.
All analyses were realized with R (R Core Team, 2019, version 3.6.1) in combination with the packages car (Fox and Weisberg, 2019; version 3.0.3), lsr (Navarro, 2015; version 0.5), psych (Revelle, 2018, Version 1.8.12), and MASS (Venables and Ripley, 2002; version 7.3-51.4). A self-written R-script was used to calculate the criterion-referenced reliability according to Subkoviak (1976).
Regarding the sample size, we used G-Power software (Faul et al., 2007). To get an approximate estimate of the required sample size, we assumed a large effect size in combination with a linear model with one categorical explanatory variable with two groups and two metric explanatory variables. We also assumed an α = 0.05 significance level and a power of 0.80. In such a setting, a sample size of N = 51 participants would be necessary. To account for a possible dropout, we considered a total sample size of N = 54 to be uniformly allocated to each condition. Although our actual sample size is below this level, the difference between the actual and necessary sample size is rather small and should thus be without consequences.
Descriptive statistics for all variables are provided in Table 2. Table 3 contains the results of LM1 and LM2, and Table 6 presents the results of LM4. Checking the possible interaction terms in each model revealed that they are all non-significant, so we omitted the interaction terms. Concerning the internal validity, LM1 indicated the expected significant effect of PKS on ACT1, there was no effect of the experimental condition on ACT1. However, looking at the descriptive statistics reveals that in the control condition pretest argumentation competence was somewhat higher than in the experimental condition.
Regarding the SLS manipulation check, a t-test (with correction for unequal variances) indicated a significant and large difference between the experimental and control condition, t(31.86) = 5.54, p < 0.001, d = 1.71, indicating that the intervention triggered learning in the EC.
Concerning the effects of the learning intervention, LM2 indicated a large, significant effect of the experimental condition and a significant effect of the argumentation competence in the pretest. In contrast to LM1, in LM2 there was no significant effect of PKS. The EMM for LM3 are given in Table 4. The EMM shows that there is a significant and medium increase in ACT, in the EC, but also an almost significant, and small, decrease of ACT in the CC.
The nearly significant decline in ACT in the CC weakens the results regarding the effectiveness of the learning intervention. To scrutinize the result of the effectiveness of the learning intervention, we consider a scenario in which there is no decrease in ACT in the CC. In this scenario, we assume for the CC a population in which the population means and variances of ACT1 and ACT 2 are equalACT1. In this hypothetical population, there is no decrease in ACT. Additionally, to account for the effects of ACT1 and PKS on ACT2, we assume that the population covariance of ACT1, ACT2, and PKS are equal to the sample covariances in the CC. By means of a Monte Carlo simulation, we simulated 1000 data sets with the before described population setting in the CC. To these simulated data, we added the actual data from the EC. For these 1000 datasets, we calculated the LM2. To judge the robustness of the results concerning the effectiveness of the intervention, we scrutinize the power of the dummy regression coefficient indicating the effect of the EC on ACT2. We define the power as the portion of p-values of the dummy regression coefficient for which p ≤ 0.05. The results of this Monte Carlo simulation are presented in Table 5. The mean of the dummy regression coefficient, which represents the average effect, is considerably smaller than the regression coefficient in LM2. But the power indicates that in 98.4% of the simulated cases, there is a difference between the EC and the CC. Thus, the simulation of the hypothetical scenario in which there is no decrease in ACT in the CC also supports the hypothesis of the effectiveness of the learning intervention. Additionally, the mean of the effect size ηp2 was 0.12 (SD = 0.03) indicating an almost large effect on average.
Concerning LM4, the results in Table 6 show that there is a positive and large effect of the QPE on ACT in the EC.
Regarding H1, the results indicate that learning from advocatory errors fosters argumentation competence. In the posttest, the linear model indicated a clear effect of the experimental condition. This conclusion is further supported by the indication of internal validity, the positive results regarding the manipulation check, and the simulation of the hypothetical scenario without a decrease of argumentation competence in the control condition. However, the decrease of argumentation competence in the control condition remains a small drawback of the results. As this difference in the pretest is statistically non-significant, the higher level of pretest argumentation competence in the control condition may simply reflect a kind of a naturally occurring fluctuation due to sampling errors. Especially since the total sample size is rather small, statistics like the mean may not be quite stable. Additionally, there may have been demotivating effects of the bogus intervention in the control condition. Psychology students in Germany have to pass 30 h of compulsory participation in psychological experiments. Thus, having low subjective learning success, may decrease the participants’ motivation yielding them to arbitrarily answering the posttest argumentation test just to acquire the required participation credit. However, the variance explained by the experimental condition is rather large. Assuming that a certain portion of this explained variance is due to demotivating effects of the control condition would probably not yield a significant decrease. This view is also supported by the results of the simulation. Taken together, the hypothesis that learning from advocatory errors in the form of case-based stories fosters argumentation competence is supported. But besides this support for H1, a remarkable finding is the rather low increase in argumentation competence in the experimental condition.
The results also lent support to the hypothesis H2. The linear model for the posttest indicates an effect of the pretest argumentation competence on the posttest argumentation competence. Thus, this hypothesis is supported and indicates the well-known Matthew effect of prior knowledge that was also expected from the results of Von der Mühlen et al. (2019).
With regard to H3, there is a significant effect of prior knowledge in statistics at the pretest, whereas there is no significant effect of prior knowledge in statistics in the posttest. This is a somewhat unexpected finding, indicating that, indeed, prior knowledge in statistics affects argumentation competence (at least, as long as statistical knowledge is needed for constructing the argument). At the same time, the findings indicate that the repetition of statistics basics levels out effects of prior knowledge so that prior knowledge in statistics does not affect the posttest argumentation competence. However, the findings did not indicate how prior knowledge in statistics is involved in the learning processes.
With regard to H4, the results show that, as we have expected, the quality of the prompt elaboration has a large effect on the argumentation competence after the intervention, and is this in line with the results found in the literature cited above.
A limitation of Study 1 is certainly the unequal distribution of Type 1 and 2 arguments in the argumentation competence test. Although it could be argued that Type 1 arguments are more natural (cf., Von der Mühlen et al., 2019), the typical use of arguments in discourses and texts often require type 2 arguments. Thus, to ensure the validity of the argumentation competence test, an even distribution of both argument types should be sought.
The goal of Study 2 is to investigate the effectiveness of learning from advocatory errors further and to conceptually replicate the findings from Study 1. We also aim at investigating the effectiveness of different prompt types, i.e., prompts drawing on the testing effect versus elaboration prompts. Moreover, the question if the quality of prompt elaboration has effects on the argumentation competence after taking the intervention is considered again, for each type of prompt separately.
For the learning intervention in Study 2, the same kind of dialogue as in Study 1 was used but the dialogues were adapted. Firstly, the strict order of Type 2 arguments in the first part and Type 1 arguments in the second part of the dialogue was skipped to provide more authentic dialogues. The framing of the dialogues aimed at an even distribution of Type 1 and 2 arguments. Moreover, the errors “False generalization of results” and “Neglecting the context” (see Table 1) from the intervention were conflated because of their similarity. Thus, six dialogues were finally presented covering the argumentation errors provided in Table 7. The intervention in Study 1 was realized as a printed booklet. Because participants were asked to write in the booklets; each participant received their copy.
In the first part of the dialogue, an erroneous argument was used by Protagonist 1. In turn, Protagonist 2 hinted at the error and provided the contrast between the error and the correct argument. Finally, Protagonist 2 also provided a heuristic with the avoidance strategy. In the second part, Protagonist 2 presented an erroneous argument with the same error as in the first part. This argument was corrected by Protagonist 1 and the application of the heuristic was explained.
The learning intervention was set up in three versions. In the first version, the prompts drew on the testing effect and consisted of multiple-choice questions asking for the previously learned content. In total, three prompts were implemented. The prompts were given after the first part and the second part of a dialogue, respectively. The first prompt asked a question regarding the error, whereas the second and third prompt asked a question for the avoidance strategy. Care was taken that the questions were general and did not refer to the specific content of the dialogue but rather to the error or the avoidance strategy, respectively. Each prompt consisted of a multiple-choice question. All prompts had three answer options. Only one option was the correct answer. Feedback of response was provided and the participants were instructed to compare their response with the correct one.
In the second version, elaboration prompts were provided targeting the error featured in the dialogue. In the first part of the dialogue, the prompts referred to the contrast of the error and the correct solution. The explanation that was provided in the first dialogue part described the error and the correct argument only superficially. The prompt asked to provide a thorough explanation. In the second part of each dialogue, the explanation of the avoidance strategy’s application was superficial and a second prompt again asked to provide a thorough explanation. Afterward, a third prompt followed asking again a question regarding the application of the avoidance strategy. All prompts were designed as a multiple-choice question with one correct answer option. The first two prompts had three answer options, whereas the third prompt had three answer options. After each prompt, feedback of correct response was provided and the participants were advised to compare the correct solution with their own. After each prompt (in the first and in the second version), the printed advice to not turn to the next page before the task was completed was given; the solution of the prompt was provided on the next page.
The third version consisted of the text of the first version but without any prompts. In all versions, an introduction to the material and task to be done by the participants was provided.
The hypotheses in Study 2 are largely similar to those in Study 1. Firstly, we hypothesize that (H1) learning from advocatory errors fosters argumentation competence. Especially, we hypothesize that the learning intervention with prompts fosters argumentation competence. Additionally, we also want to exploratively investigate if both types of prompts differ in their effectiveness in fostering argumentation competence. As in Study 1, concerning the importance of prior knowledge, we hypothesize that the argumentation competence in the pretest has an impact on the argumentation competence in the posttest (H2). Drawing on the results of Study 1, we hypothesize that prior knowledge in statistics will have an impact on pretest argumentation competence, whereas there is no effect of prior knowledge in statistics on the posttest argumentation competence (H3). Again, H2 and H3 are auxiliary hypotheses. As in Study 1, regarding the possible effects of the quality of prompt elaboration, we hypothesize that the quality of the prompt elaborations has a positive effect on the argumentation competence after the intervention (H4).
In total, 85 psychology students (N = 15 males) from the same southwestern Germany university took part and were recruited in the same way as in Study 1. The mean age was 21.72 years (SD = 3.24). On average, the participants were in the third semester (MSemester = 3.08, SD = 1.40). They received a 2 h time credit for the required participation in psychological experiments. The participants were randomly allocated to a first experimental condition (EC1; NEC1 = 29), a second experimental condition (EC2; NEC2 = 29), and a control condition (CC; NCC = 27). The slightly different participant numbers between both ECs and the CC resulted from two participants that agreed to participate and were allocated to the CC but did not show up. The experiment took place in group sessions with 5 participants at maximum. The participants worked individually with the learning material without interacting with each other.
In the first experimental condition (EC1), participants received the learning intervention with the testing prompts. In the second experimental condition (EC2), participants received the learning intervention with elaboration prompts. In the control condition (CC), participants only received the learning intervention without instructional support.
The procedure was basically the same as in Study 1. In total, 120 min were scheduled for all tests and the intervention, no participant took longer. The participants worked self-paced through the material. First, the participants received the pretest booklet which contained some demographic questions, followed by the prior knowledge in statistics and the argumentation competence test. After the pretest, the participant requested the intervention from a student research assistant that guided each session. Finally, after finishing the intervention, the participant requested the posttest from the research assistant.
For assessing the argumentation competence in the pre- and posttest (ACT1 and ACT2), the same construction as in Study 1 was used but the test was completely revised. Firstly, the revised test accounted for the conflation of the errors “False generalization of results” and “Neglecting the context.” Secondly, the representation of Type 2 arguments was enhanced. The new test had 22 items in total: 12 items referring to Type 1 and ten items referring to Type 2 arguments. Correct responses were coded as 1 point and incorrect responses as 0 points; the maximum number of points was 22. The pre- and posttest were different but both tests were parallelized. Criterion-referenced reliability in the pretest was crpre = 0.88, and in the posttest crpost = 0.94.
The prior knowledge in statistics test was essentially the same as in Study 1, but four items that did not directly relate to the content of the learning intervention were discarded. Thus, the final PKS test had 17 items. Correct responses were coded as 1 point and incorrect responses as 0 points; the maximum number of points was 17. The criterion-referenced reliability was crspk = 0.71.
The same procedure as in Study 1 was applied to get an overall measure of prompt elaboration. As only the EC1 and EC2 received the intervention, this score was only calculated for participants in both conditions. Because there were three prompts in six dialogues, the maximal number of points was 18.
The same models (LM1-LM3) and tests as in Study 1 were used. Because there are three experimental conditions in Study 1, there are now two dummy variables in the linear models. The reference category was the CC, so the regression coefficients for the dummy variables indicate the difference between each EC and the CC while accounting for the other explanatory variables. To test if the two types of prompts have different effects, we used a general linear hypothesis (e.g., Fox, 2016, ch. 9) to test the equality of the two dummy regression coefficients. Regarding LM4, the model in Study 1 checks in a first step if there is an interaction between QPE and the experimental condition. As this was the case, we calculated a model with ACT2 as dependent variables and QPE, ACT1, and PKS as an explanatory model for the EC1 and EC2 separately. As the participants in the CC did not receive any prompts, this subsample is not considered in LM4. The same software as in Study 1 was used. Again, all linear models were checked for interaction and significant interactions were retained. Regarding LM4, a significant interaction of QPE and EC would indicate different effects of QPE in both EC. Therefore, in the first step we LM4a with the interaction of QPE and EC. As the analysis revealed a significant interaction, we calculated LM4b. In LM4b, we regressed ACT2 on ACT1 and PKS in each condition separately. Again, Figure 3, provides an overview of the statistical analysis in Study 2.
Regarding the sample size, we draw on the simulation results of Study 1 and assumed the smallest possible effect size that is classified as a large effect in combination with a linear model with one categorical explanatory variable and three groups and two metric exploratory variables. Additionally, we assumed an α = 0.05 significant level and a power of.95. In this setting, a sample size of N = 84 would be necessary. Thus, 29 participants per experimental condition are necessary. To compensate for possible dropouts, 32 participants per condition were planned. As the actual number of students that volunteered is close to the planned number, the achieved power is as likely as intended.
The descriptive statistics are shown in Table 8. The regression results for LM1 and LM2 are shown in Table 9. Table 10 contains the results relating to LM3 and the EMM, and Table 11 contains the results of LM4. Initially, all linear models were checked for possible interaction terms. In LM2, the interaction of PKS with ACT1 revealed to be significant, so it was included in the model. Regarding the internal validity, the regression results of LM1 shows that there is no effect of the experimental condition in the pretest. Consequently, the internal validity of the experimental setting can be assumed. LM1 also indicated a medium effect of PKS on ACT1.
With regard to the effects of the learning environment, the regression results for LM2 indicate significant effects of each experimental condition, with both effects being small. The regression coefficients indicate a slightly larger effect of EC2, but the general linear hypothesis for testing the equality of both dummy regression coefficients indicate that they do not differ, F(1, 79) = 0.30, p = 0.584. Thus, the effects of experimental conditions do not differ. LM2 also indicates the effects of ACT1 and PKS on ACT2. The results for the effect of PKS in LM2 are in contrast to the result from Study 1.
With regard to LM3, Table 10 indicates that the EMM are different from zero for both EC but not for the CC. The EMM in both EC indicate a small effect. Thus, these results support the hypothesis that instructional support is necessary. Regarding H4, the first LM4 indicates that there is a significant interaction between the EC and QPE in the overall model, indicating that the effects of QPE on ACT2 are different in both experimental conditions. In EC1, there was no effect of QPE on ACT2, whereas in EC2, there was a significant and large effect of QPE on ACT2.
The results support our hypothesis H1, that learning from advocatory errors in combination with instructional support fosters argumentation competence, whereas learning from advocatory errors without instructional support does not foster argumentation competence, as indicated by the estimated marginal means. But the results did not indicate which kind of instructional support is superior due to a lack of a significant difference between the experimental conditions. Descriptively, learning from advocatory errors that is supported by especially targeted elaboration prompts seems superior to learning that is supported with testing prompts. But the lack of a significant difference between these two conditions does not allow a profound conclusion in this way. Thus, an assertion about the effectiveness of the various prompts is not possible. A noticeable finding – and a replication of the results from Study 1 in the experimental condition – is the small amount of increase in argumentation competence in both experimental conditions which is firstly shown by the descriptive statistics and secondly by the estimated marginal means for the difference in argumentation competence.
However, the interpretation of the effectiveness of the prompts drawing on the testing effect should be taken with care. The dummy regression coefficient representing the effect of the testing prompts is very close to the significance level and the effects size is small. For the evaluation of both prompt types, H4 matters, too. As there was an interaction effect of the experimental condition and the quality of prompt elaboration, the effects of the quality of prompt elaboration on posttest argumentation competence differ. Whereas for the testing prompts, there was no effect of the quality of prompt elaboration, but there was a large effect for the elaboration prompts. Taken together, a cautious interpretation regarding the testing prompts is advisable. A cautious interpretation would be that the elaboration prompts do indeed foster argumentation competence when learning from advocatory errors, whereas the testing prompts are only likely to do so.
With regard to H2, the results support the importance of the present level of argumentation competence, i.e., the level of argumentation competence in the pretest, on the argumentation competence in the posttest – and along with this on the learning outcomes. Again, this indicates a Matthew effect regarding the present level of argumentation skills. Regarding H3, the results of Study 2 are different from the results of Study 1, i.e., the prior knowledge in statistics had effects on argumentation competence in the pre- and posttest. Moreover, in the posttest, there was a significant interaction between prior knowledge in statistics and pretest argumentation competence. This interaction suggests that in the learning process, a low level of pretest argumentation competence can be compensated by an adequate level of statistical knowledge and vice versa. Nevertheless, this finding of the interaction effect should be replicated and not generalized too far. However, the results regarding H3 show that it is important to consider the present level of argumentation competence and prior knowledge when evaluating learning interventions.
A shortcoming of this study is that there is no indication of subjective learning success. On the one hand, measuring subjective learning success would have allowed gaining insight into the individual perception of the instructional support. On the other hand, as all participants received a learning intervention – the only difference being the kind of instructional support – it is unclear if considerable differences in subjective learning success would emerge. Because of the same reason, subjective learning success would also not be a good indicator of the internal validity of the study.
The goal of these two studies was first to demonstrate that learning from advocatory errors in a story-based design fosters argumentation competence and second, to scrutinize the role of various kinds of instructional support. With regard to the first goal, Study 1 indicates the effectiveness of the story-based approach to learning from advocatory errors. This result is reflected in Study 2 in which the conditions with instructional support were effective, too, but not the condition without instructional support. However, as already mentioned, the learning gains in both studies were rather small. In both studies, the participants answered on average two to three questions more correctly after learning than they did before. There are several reasons for this, at the first sight, disappointing results. Firstly, the participants had no opportunity to practice the newly acquired knowledge. Although the prompts provided a limited practice opportunity, this may not be considered as practice. Given the importance of practice in the classical accounts to learning and instruction (e.g., Gagné, 1985), practicing to recognize errors and to apply avoidance strategies seems to be necessary. Practice is also important from the perspective of expertise development (e.g., Ericsson and Krampe, 1993). Secondly, there is also the issue of transfer, which is also related to the issue of practice. A narrow transfer was implemented in the story-based design, each error and the respective avoidance strategy was explained with two examples in the both parts of each dialogue. In the context of the cognitive flexibility theory (e.g., Spiro and Jehng, 1990), this procedure initiates multiperspectivity that should enable transfer. However, as argumentation requires some kind of de-contextualization to support a claim with scientific evidence, two examples may simply be too few to acquire the necessary expertise. The kind of argument may also matter. Type 1 arguments, in which a claim is supported by an empirical finding, may easily be evaluated for issues, e.g., a significant group difference due to large samples size but small effect sizes may easily be recognized as an erroneous argument (e.g., see the error “Misinterpreting significant results”). Type 2 arguments in which the scientific evidence consists in a substantive evaluation of findings or theories may be harder to evaluate (e.g., see the errors “False generalizability of results” and “Neglecting the context”). Considering the evaluation (or construction) of arguments as a problem-solving process, Type 1 arguments may require far less practice to elaborate their deep and surface structure that enable analogical transfer than Type 2 arguments (cf., Holyoak and Koh, 1987). Additionally, Britt and Larson (2003) demonstrated that Type 1 arguments are better recalled than Type 2 arguments and also that Type 1 arguments are read faster. Thus, the position of the claim and the reason may affect cognitive learning processes in many ways.
Up to now, we have only considered – more or less – cognitive factors that may affect the learning process. Another perspective on the rather low gains in argumentation competence is an assessment perspective. As indicated by the pretest results, in both studies the empirical mean of the pretest argumentation competence is over the theoretical mean of the test and thus, the participants answered more than half of the questions correctly. Stated otherwise, the participants already had – in sense of the used test –a relatively high level of argumentation competence. Thus, it is likely that the average learning gains are rather low due to an upcoming ceiling effect.
Another factor, which should be considered when interpreting the results, is the motivation of the participants. As already mentioned above in the discussion of the decline of argumentation competence in the control condition in Study 1, participants usually take part in a study because of external requirements and not because they are intrinsically motivated. Drawing on the distinction of intrinsic and extrinsic motivation (e.g., Deci and Ryan, 2000), it is more likely that students are extrinsically motivated to participate with the consequence that they only invest minimal effort resulting in rather low learning outcomes. Along with this is also the question of interest, which is also a major predictor for learning (cf., Schiefele et al., 1992; Ainley et al., 2002). It is plausible that students, especially in the beginning phase of their university education, are not aware of the importance of scientific argumentation and have more interest in psychological knowledge than in acquiring and/or improving scientific argumentation competence.
Notwithstanding the before discussed cognitive and motivational factors, the statistical results in combination with the effect size indicate the presence of gains in argumentation competence. In summary, the effectiveness of learning from advocatory errors approach to foster argumentation competence was demonstrated.
Concerning the second goal, we asked for the effectiveness of different kinds of instructional support. Because of the present findings, this goal has to be considered in combination with the third goal, i.e., the question about the relation between the quality of prompt elaboration and argumentation and its relations with the learning outcomes. As stated above, as an instantiation of example-based learning, learning from advocatory errors needs instructional support to be effective. This was demonstrated in Study 2, in which successful learning occurred in both conditions with instructional support, but not in the condition without instructional support. The results provided evidence that both prompt types did not differ in their effectiveness. However, as already discussed above, because of the statistical results, this finding should be cautiously interpreted. Taken for granted that the two prompt types did indeed produce the same learning, this result is in line with the theory behind the testing effect. As the testing prompts were designed to be cognitively demanding, the results that they are as effective as the elaboration prompts seem straightforward (cf., Endres and Renkl, 2015).
But this is in turn in contrast to the findings regarding the quality of the prompt elaboration and its relation with the learning outcome, which constituted the third goal of this study. The present findings suggest that the quality of the elaboration for the testing prompt did not affect the posttest argumentation competence, whereas there was an effect on the quality of the prompt elaboration for the elaboration prompts. A possibility is that the implementation of prompts as a multiple-choice task was unfavorable. A study of Greving and Richter (2018) showed that the testing effect disappeared when the testing tasks were in multiple-choice format. Thus, the form in which the testing prompts were presented may be problematic.
As a general result, we can infer that learning from advocatory errors is indeed a viable means to foster argumentation competence. However, instructional support is vital for learning success. As Study 2 revealed, if no instructional support is provided, the acquisition of argumentation competence will not occur. Regarding the type of instructional support, this study especially indicates the effectiveness of the elaboration prompts. The effectiveness of the prompts designed after the testing effect should be further investigated.
Both studies have some limitations. The first limitation refers to the kind of arguments that we considered in this contribution. We only used simple arguments consisting of a claim and a reason. However, typical arguments in scientific argumentation contain additional elements like warrants and qualifiers (cf., Toulmin, 1958). Thus, the results from the reported studies do not necessarily generalize to more complex arguments. A second issue regarding the generalization of the results refers to the measurement of argumentation competence. As argumentation competence was measured by means of a multiple-choice-test, the generalizability to a “real-world” argumentation setting may be questionable. The distractors in the multiple-choice task were designed to capture the argumentation errors in the intervention. Thus, the used argumentation competence measures are tailored to the means of the studies and the covered argumentation errors. But these errors are certainly not an extensive collection of all possible argumentation errors and therefore, the transfer of the newly acquired (or improved) argumentation competence to other errors is uncertain. Besides, the multiple-choice tasks required the evaluation of ready-made reasons or claims, respectively. Thus, the results do not allow a prediction if the argumentation errors are avoided when students are constructing their arguments.
A second and methodical limitation concerns the role of prior knowledge. Notwithstanding the importance of prior knowledge for learning and the consequential need to statistically control the effects of prior knowledge in the evaluation of interventions, the measurement of prior knowledge itself may have distorting consequences. As the discussion about the testing effect makes clear, testing may activate existing knowledge. Thus, activating the prior knowledge may have effects on the learning outcomes which would not appear if there was no testing in advance. From a methodological point of view, there is a dilemma to control for the effects but the measurement necessary for controlling itself may have consequences. A way out of this dilemma would be to compare conditions with and without prior knowledge assessment.
A last and rather minor limitation refers to the use of fictive examples in the dialogue. Although the content for the exemplifying domain was constructed to avoid possible effects of domain-specific prior knowledge, the construction of the examples rested on the properties of psychological knowledge in general. Thus, participants with a larger psychological knowledge base might have advantages compared to participants with a smaller psychological knowledge base.
These limitations should be seen as a set of desiderata for future research. Future research could take into account more complex types of arguments and could investigate the role of prior knowledge assessment on the learning outcome by means of an adequate experimental setting.
The datasets for this manuscript are not publicly available because of legal reasons. Participants gave their consent to store, process and analyze the data as well as to the publication of the analysis results. Participants were assured that the data will not be distributed. Requests to access the datasets should be directed to EK, ZS5rbG9wcEBteC51bmktc2FhcmxhbmQuZGU=.
Ethical review and approval was not required for the study on human participants in accordance with the local legislation and institutional requirements. The participants provided their written informed consent to participate in this study.
EK made the conceptualization and planned the studies, developed the materials, analyzed the data, and wrote up the manuscript. RS assisted in the development of the materials and supervised the conceptualization, read and commented on the draft of the manuscript, and reviewed the analysis results. All authors contributed to the article and approved the submitted version.
We acknowledge support by the Deutsche Forschungsgemeinschaft (DFG, German Research Foundation) and Saarland University within the funding programme Open Access Publishing.
The authors declare that the research was conducted in the absence of any commercial or financial relationships that could be construed as a potential conflict of interest.
We thank Katharina Scholtes and Sina Wilhelm for their help in the preparation of the manuscript.
The Supplementary Material for this article can be found online at: https://www.frontiersin.org/articles/10.3389/feduc.2020.00126/full#supplementary-material
Ainley, M., Hidi, S., and Berndorff, D. (2002). Interest, learning, and the psychological processes that mediate their relationship. J. Educ. Psychol. 94, 545–561. doi: 10.1037/0022-0663.94.3.545
Alexander, P. A. (1996). The past, present, and future of knowledge research: A reexamination of the role of knowledge in learning and instruction. Edu. Psychol. 31, 89–92. doi: 10.1080/00461520.1996.10524941
Astleitner, H., Brünken, R., and Leutner, D. (2003). The quality of instructional materials for argumentative knowledge construction. J. Instr. Psychol. 30, 3–11.
Booth, J., Lange, K., Koedinger, K., and Newton, K. (2013). Using example problems to improve student learning in algebra: differentiating between correct and incorrect examples. Learn. Instr. 25, 24–35.
Booth, W., Colomb, G., and Williams, J. (2008). The Craft of Research. Chicago, IL: The Chicago University Press.
Britt, M. A., and Larson, A. (2003). Constructing representations of arguments. J. Mem. Lang. 48, 749–810.
Carpenter, S. (2009). Cue strength as a moderator of the testing effect: the benefits of elaborative retrieval. J. Exp. Psychol. Learn. Mem. Cogn. 35, 1563–1569. doi: 10.1037/a0017021
Chi, M. T. H., Bassok, M., Lewis, M. W., Reimann, P., and Glaser, R. (1989). Self-explanations: how students’ study and use examples in learning to solve problems. Cogn. Sci. 13, 145–182. doi: 10.1207/s15516709cog1302_1
Cognition and Technology Group at Vanderbilt (1992). The jasper series as an example of anchored instruction: theory, program, description, and assessment data. Educ. Psychol. 27, 291–315. doi: 10.1207/s15326985ep2703_3
Cohen, J. (1988). Statistical Power Analysis for the Behavioral Sciences. Hillsdale, MI: Lawrence Erlbaum.
Deci, E. L., and Ryan, R. M. (2000). The ‘what’ and ‘why’ of goal pursuits: human needs and the self-determination of behavior. Psychol. Inq. 11, 227–268. doi: 10.1207/s15327965pli1104_01
Dietrich, H., Zhang, Y., Klopp, E., Brünken, R., Krause, U.-M., Spinath, F. M., et al. (2015). Scientific competencies in the social sciences. Psychol. Learn. Teach. 14, 115–130.
Dochy, F. J. R. C. (1992). Assessment of Prior Knowledge as a Determinant for Future Learning. Utrecht: Lemma.
Endres, T., and Renkl, A. (2015). Mechanisms behind the testing effect: an empirical investigation of retrieval practice in meaningful learning. Front. Psychol. 6:1054. doi: 10.3389/fpsyg.2015.01054
Ericsson, K. A., and Krampe, R. T. (1993). The role of deliberate practice in the acquisition of expert performance. Psychol. Rev. 100, 363–406. doi: 10.1037/0033-295x.100.3.363
Faul, F., Erdfelder, E., Lang, A.-G., and Buchner, A. (2007). G∗Power 3: a flexible statistical power analysis program for the social, behavioral, and biomedical sciences. Behav. Res. Methods 39, 175–191. doi: 10.3758/bf03193146
Fischer, F., Kollar, I., Ufer, S., Sodian, B., Hussmann, H., Pekrun, R., et al. (2014). Scientific reasoning and argumentation: advancing an interdisciplinary research agenda in education. Front. Learn. Res. 4:28–45. doi: 10.14786/flr.v2i2.96
Fox, J. (2016). Applied Linear Regression and Generalized Linear Regression, 3rd Edn. Thousand Oaks, CA: Sage.
Fox, J., and Weisberg, S. (2019). An R Companion to Applied Regression, 3rd Edn. Thousand Oaks, CA: Sage.
Gagné, R. M. (1985). The Conditions of Learning and Theory of Instruction. London: Holt Rinehart and Winston.
Gartmeier, M., Bauer, J., Gruber, H., and Heid, H. (2008). Negative knowledge: understanding professional learning and expertise. Voc. Learn. 1, 87–103. doi: 10.1007/s12186-008-9006-1
Gigerenzer, G., and Brighton, H. (2009). Homo heuristicus: why biased minds make better inferences. Top. Cogn. Sci. 1, 107–143. doi: 10.1111/j.1756-8765.2008.01006.x
Gigerenzer, G., and Zimmer, A. (2014). “Heuristik. [Heuristic.],” in Dorsch – Lexikon der Psychologie [Dorsch’s Lexicon of Psychology], 18th Edn, ed. M. A. Wirtz (Hrsg.) (Bern: Verlag Hogrefe Verlag), 691.
Greving, S., and Richter, T. (2018). Examining the testing effect in university teaching: retrievability and question format matter. Front. Psychol. 9:2412. doi: 10.3389/fpsyg.2018.02412
Große, C. S., and Renkl, A. (2007). Finding and fixing errors in worked examples: can this foster learning outcomes? Learn. Instruc. 17, 612–634. doi: 10.1016/j.learninstruc.2007.09.008
Halamish, V., and Bjork, R. A. (2011). When does testing enhance retention? A distributionbased interpretation of retrieval as a memory modifier. J. Exp. Psychol. Learn. Mem. Cogn. 37, 801–812. doi: 10.1037/a0023219
Hattie, J. (2009). Visible Learning. A Synthesis of Over 800 Meta-Analyses Relating to Achievement. London: Routledge.
Hefter, M. H., Berthold, K., Renkl, A., Rieß, W., Schmid, S., and Fries, S. (2014). Effects of a training intervention to foster argumentation skills while processing conflicting scientific positions. Instr. Sci. 42, 929–947. doi: 10.1007/s11251-014-9320-y
Holyoak, K., and Koh, K. (1987). Surface and structural similarity in analogical transfer. Mem. Cogn. 15, 332–340. doi: 10.3758/bf03197035
Jonassen, D. (1999). “Designing constructivist learning environments,” in Instructional Design Theories and Models, ed. C. M. Reigeluth (Hillsdale, NJ: Lawrence Erlbaum), 215–239.
Jonassen, D., and Hernandez-Serrano, J. (2002). Case-based reasoning and instructional design: using stories to support problem solving. Educ. Technol. Res. Dev. 50, 65–77.
Kelly, G. J., and Takao, A. (2002). Epistemic levels in argument: an analysis of university oceanography students’ use of evidence in writing. Sci. Educ. 86, 314–342. doi: 10.1002/sce.10024
Klein, M., Wagner, K., Klopp, E., and Stark, R. (2017). Fostering of applicable educational knowledge in student teachers: effects of an error-based seminar concept and instructional support during testing on qualities of applicable knowledge. J. Educ. Res. Online 9, 88–114.
Klieme, E., and Leutner, D. (2006). Kompetenzmodelle zur Erfassung individueller Lernergebnisse und zur Bilanzierung von Bildungsprozessen. Beschreibung eines neu eingerichteten Schwerpunktprogramms der DFG [Competence models for assessing individual learning outcomes and evaluating educational processes. Description of a new priority program of the German Research Foundation (DFG)]. Zeitschrift für Pädagogik 52, 876–903.
Klopp, E., and Stark, R. (2018). Learning scientific explanations by means of worked examples – promoting psychology students’ explanation competence. Psychol. Learn. Teach. 17, 144–165. doi: 10.1177/1475725718757171
Klopp, E., Stark, R., Kopp, V., and Fischer, M. R. (2013). Psychological factors affecting medical students’ learning with erroneous worked examples. J. Educ. Learn. 2, 158–170.
Kolodner, J. (1997). Educational implications of analogy: a view from case-based reasoning. Am. Psychol. 52, 57–66. doi: 10.1037/0003-066x.52.1.57
Kopp, V., Stark, R., and Fischer, M. R. (2008). Fostering diagnostic knowledge through computer-supported, case-based worked examples: effects of erroneous examples and feedback. Med. Educ. 42, 823–829. doi: 10.1111/j.1365-2923.2008.03122.x
Leiner, D. J. (2019). SoSci Survey (Version 3.1.06) [Computer software]. Available online at https://www.soscisurvey.de (accessed January 6, 2020).
Lumer, C. (2007). “Überreden ist gut, Überzeugen ist besser! Argumentation und Ethos in der Rhetorik. [Persuading is good, convincing is better! Argumentation and ethos in rhetoric],” in Persuasion und Wissenschaft: Aktuelle Fragestellungen von Rhetorik und Argumentationstheorie [Persuasion and science: Current Issues in Rhetoric and Argumentation Theory.], eds G. Kreuzbauer, N. Gratzl, and E. Hiebl (Vienna: LIT-Verlag), 7–13.
Mehl, K. (1994). Über einen Funktionalen Aspekt von Handlungsfehlern. Was Lernt man aus Fehlern? [On a Functional Aspect of Erronoeus Actions. What to Learn From Errors?] Fortschritte der Psychologie [Advances in Psychology], Vol. 8. Münster: LIT Verlag.
Navarro, D. J. (2015). Learning Statistics with R: A Tutorial for Psychology Students and Other Beginners. (Version 0.5). Adelaide, SA: University of Adelaide.
Oser, F. (2007). “Aus Fehlern lernen. [Leanring from errors.],” in Pädagogische Theorien des Lernens [Pedagogical Theories of Learning], eds M. Göhlich, Ch Wulf, and J. Zirfas (Weinheim: Beltz), 203–212.
Oser, F., and Spychiger, M. (2005). Lernen ist schmerzhaft. Zur Theorie des Negativen Wissens und zur Praxis der Fehlerkultur. Weinheim: Beltz.
R Core Team (2019). R: A Language and Environment for Statistical Computing. Vienna: R Foundation for Statistical Computing.
Renkl, A. (2014). Toward an instructionally oriented theory of example-based learning. Cogn. Sci. 38, 1–37. doi: 10.1111/cogs.12086
Revelle, W. (2018). psych: Procedures for Personality and Psychological Research Version=1.8.12. Available online at: https://CRAN.R-project.org/package=psych (accessed January 08, 2020).
Richardson, J. (2011). Eta squared and partial eta squared as measures of effect size in educational research. Educ. Res. Rev. 6, 135–147. doi: 10.1016/j.edurev.2010.12.001
Rowland, C. (2014). The effect of testing versus restudy on retention: a meta-analytic review of the testing effect. Psychol. Bull. 140, 1432–1463. doi: 10.1037/a0037559
Sadler, T. D. (2004). Informal reasoning regarding socio-scientific issues: a critical review of research. J. Res. Sci. Teach. 41, 513–536. doi: 10.1002/tea.20009
Schiefele, U., Krapp, A., and Winteler, A. (1992). “Interest as a predictor of academic achievement: a meta-analysis of research,” in The Role of Interest in Learning and Development, eds K. A. Renninger, S. Hidi, and A. Krapp (Hillsdale, NJ: Erlbaum), 183–211.
Schworm, S., and Renkl, A. (2007). Learning argumentation skills through the use of prompts for self-explaining examples. J. Educ. Psychol. 99, 285–296. doi: 10.1037/0022-0663.99.2.285
Searle, S., Speed, F., and Milliken, G. (1980). Population marginal means in the linear model: an alternative to least squares means. Am. Stat. 34, 216–221. doi: 10.1080/00031305.1980.10483031
Siegler, R. S. (2002). “Microgenetic studies of self-explanations,” in Microdevelopment: Transition Processes in Development and Learning, eds N. Granott and J. Parziale (New York, NY: Cambridge University), 31–58. doi: 10.1017/CBO9780511489709.002
Spiro, R. J., and Jehng, J. (1990). “Cognitive flexibility and hypertext: theory and technology for the non-linear and multidimensional traversal of complex subject matter,” in Cognition, Education, and Multimedia, eds D. Nix and R. Spiro (Hillsdale, NJ: Erlbaum).
Stark, R. (1999). Lernen mit Lösungsbeispielen. [Learning with Worked Examples.]. Göttingen: Hogrefe.
Stark, R. (2005). “Constructing arguments in educational discourses,” in Bridging Individual, Organisational, and Cultural Aspects of Professional Learning, eds H. Gruber, C. Harteis, R. Mulder, and M. Rehrl (Hrsg.) (Regensburg: S. Roderer), 64–71.
Stark, R., Puhl, T., and Krause, U.-M. (2009). Improving scientific argumentation skills by a problem-based learning environment: effects of an elaboration tool and relevance of student characteristics. Eval. Res. Educ. 22, 51–68. doi: 10.1080/09500790903082362
Subkoviak, M. J. (1976). Estimating reliability from a single administration of a criterion-referenced test. J. Educ. Meas. 13, 265–276. doi: 10.1111/j.1745-3984.1976.tb00017.x
Van Eemeren, F., Grootendorst, R., Henkemans, F., Blair, J., Johnson, R., Krabbe, E., et al. (1996). Fundamental of Argumentation Theory. Hillsdale, NJ: Erlbaum.
Von der Mühlen, S., Richter, T., Schmid, S., and Berthold, K. (2019). How to improve argumentation comprehension in university students: experimental test of a training approach. Instr. Sci. 47, 215–237. doi: 10.1007/s11251-018-9471-3
Wagner, K., Klein, M., Klopp, E., and Stark, R. (2014). Instruktionale Unterstützung beim Lernen aus advokatorischen Fehlern in der Lehramtsausbildung: Effekte auf die Anwendung wissenschaftlichen Wissens. Psychologie in Erziehung und Unterricht 61, 287–301.
Wenglein, S., Bauer, J., Heininger, S., and Prenzel, M. (2015). Kompetenz angehender Lehrkräfte zum Argumentieren mit Evidenz: Erhöht ein Training von Heuristiken die Argumentationsqualität? Unterrichtswissenschaft 43, 209–224.
Keywords: argumentation, competence, learning from advocatory errors, example-based learning, heuristics
Citation: Klopp E and Stark R (2020) Learning to Argue From Others’ Erroneous Arguments – Fostering Argumentation Competence Through Learning From Advocatory Errors. Front. Educ. 5:126. doi: 10.3389/feduc.2020.00126
Received: 25 March 2020; Accepted: 26 June 2020;
Published: 16 July 2020.
Edited by:
Lawrence Jun Zhang, University of Auckland, New ZealandReviewed by:
Shu-ming Wang, Chinese Culture University, TaiwanCopyright © 2020 Klopp and Stark. This is an open-access article distributed under the terms of the Creative Commons Attribution License (CC BY). The use, distribution or reproduction in other forums is permitted, provided the original author(s) and the copyright owner(s) are credited and that the original publication in this journal is cited, in accordance with accepted academic practice. No use, distribution or reproduction is permitted which does not comply with these terms.
*Correspondence: Eric Klopp, ZS5rbG9wcEBteC51bmktc2FhcmxhbmQuZGU=
Disclaimer: All claims expressed in this article are solely those of the authors and do not necessarily represent those of their affiliated organizations, or those of the publisher, the editors and the reviewers. Any product that may be evaluated in this article or claim that may be made by its manufacturer is not guaranteed or endorsed by the publisher.
Research integrity at Frontiers
Learn more about the work of our research integrity team to safeguard the quality of each article we publish.