- Department of Curriculum and Pedagogy, Faculty of Education, The University of British Columbia, Vancouver, BC, Canada
Agency is inherent in students’ ability to regulate, control, and monitor their own learning. A learners’ effectiveness in regulating their cognitive, affective, and behavioral processes as they interact within the learning environment is critical to their academic success. This article advances a theory of learner agency, or agency for learning (AFL), as an emergent capacity that is intentional, self-generated, and reactive to social factors in the learning context. This article further traces the development of the Agency for Learning Questionnaire (AFLQ) and examines the internal consistency, predictive validity, and psychometric properties covering four dimensions of agentic functioning including intentionality (planfulness, decision competence), forethought (intrinsic and extrinsic motivation), self-regulation, and self-efficacy. Results of this research demonstrate that the AFLQ provides a reliable, valid, multidimensional measure of AFL based on existing theoretical and empirical findings, advancing both theory and practice. Understanding how agency develops and emerges within learning environments is a key factor in identifying why learning occurs enabling educational psychologists to potentially identify, measure, and study agentic processes in the context of learning across a variety of research designs.
Introduction
Agency is the capability of individuals to make choices and to act on those choices in ways that make a difference in their lives (Martin, 2004). Agency is in operation only when individuals self-reflect and identify external influences that are most nurturing to the self. Students enact their agency to manage their cognitive, affective, and behavioral processes as they interact with environmental factors. Personal and social aspects of agency in self-regulation are integral to a student’s ability to regulate, control, and monitor their own learning. Although often mentioned as an important influence in the self-regulated learning (SRL) literature, agency has never been measured (see Zimmerman, 2000; Martin, 2004; Karoly et al., 2005).
Research in educational psychology over the past 20 years has done much to explain the role of the cognitive system in relation to perception, affect, motivation, and behavior (e.g. Wolters and Yu, 1996; Pintrich, 2004; Jarvenoja and Jarvela, 2009; Garcia-Martín and García-Sánchez, 2020). Efforts to understand how these processes integrate with each other and the impact of social and environmental influences are starting to emerge (e.g. deJong et al., 2005; Beishuzen, 2008; Greene et al., 2010). However, confusion among researchers exploring the relationship between metacognition, self-regulation, and SRL has contributed to a disjointed theoretical paradigm in which to empirically study these processes (see Dinsmore et al., 2008; Lajoie, 2008; Schunk, 2008). Dinsmore et al. (2008) conducted a detailed review of the literature on the conceptualizations of metacognition, self-regulation, and SRL, and discovered that surprisingly few researchers explicitly defined the constructs they were studying. Only 57% of studies explicitly defined self-regulation, 69% defined SRL, and 32% defined metacognition. Further, Dinsmore and colleagues exposed problems with construct definition, measurement alignment, and found noticeable variability in the degree to which measures were explicated by researchers. For example, they found that the scope of SRL identified in the literature was quite large and that typically the measures of SRL were also broad, often involving general measures of academic behavior (e.g. MSLQ; Pintrich et al., 1993). As a result of this broad operationalization it was unclear how “these broad measures that [sought] to generalize across multiple times and situations, as well as across cognitive, motivational, emotional, and behavioral domains, [could] fairly and accurately gauge monitoring or capture the dynamic interplay of person, environment, and behavior that is the hallmark of self-regulation” (p. 409). However, Dinsmore et al. found commonalities in the research literature on metacognition and self-regulation that revealed “a marriage between self-awareness and intention to act that aligns these bodies of work” (p. 409). A theory of human agency, as described by Bandura (2006), provides a conceptualization of this “marriage” and explains how these and other self-processes interact with personal, behavioral, and social-environmental factors to provide a more holistic and contextual view of learning.
Bandura’s Theory of Human Agency
Agency is “the power to originate action” (Bandura, 2001, p. 3). Agency in social cognitive theory (SCT; Bandura, 1986) is present in the ability of people to regulate and control their cognition, motivation, and behavior through the influence of existing self-beliefs (i.e. self-efficacy). SCT understands human functioning in terms of processes of triadic reciprocal causation among internal personal factors, behavioral patterns, and environmental influences – all operating as interacting determinants that affect one another bi-directionally (Bandura, 2006, 2001). In SCT, this is an agent that is both determined and determining. Agency arises within social structures and contexts, and once emergent may exert influence capable of altering social, cultural contexts, and structures (Bandura, 1986, 2000, 2001, 2006). In SCT, behavioral, cognitive, and other personal factors, as well as environmental influences operate as determinants of (causal influences on) each other. The regulation of personal processes is inherently an individual endeavor, however, the individual (self) does not operate in isolation and requires the meditative efforts of others and aspects of the sociocultural environment to develop and operate in a goal-directed manner. SCT considers the self-as-agent to encompass four core features of human agency (Figure 1)– intentionality, forethought, self-reactiveness (self-regulation), and self-reflectiveness (self-efficacy).
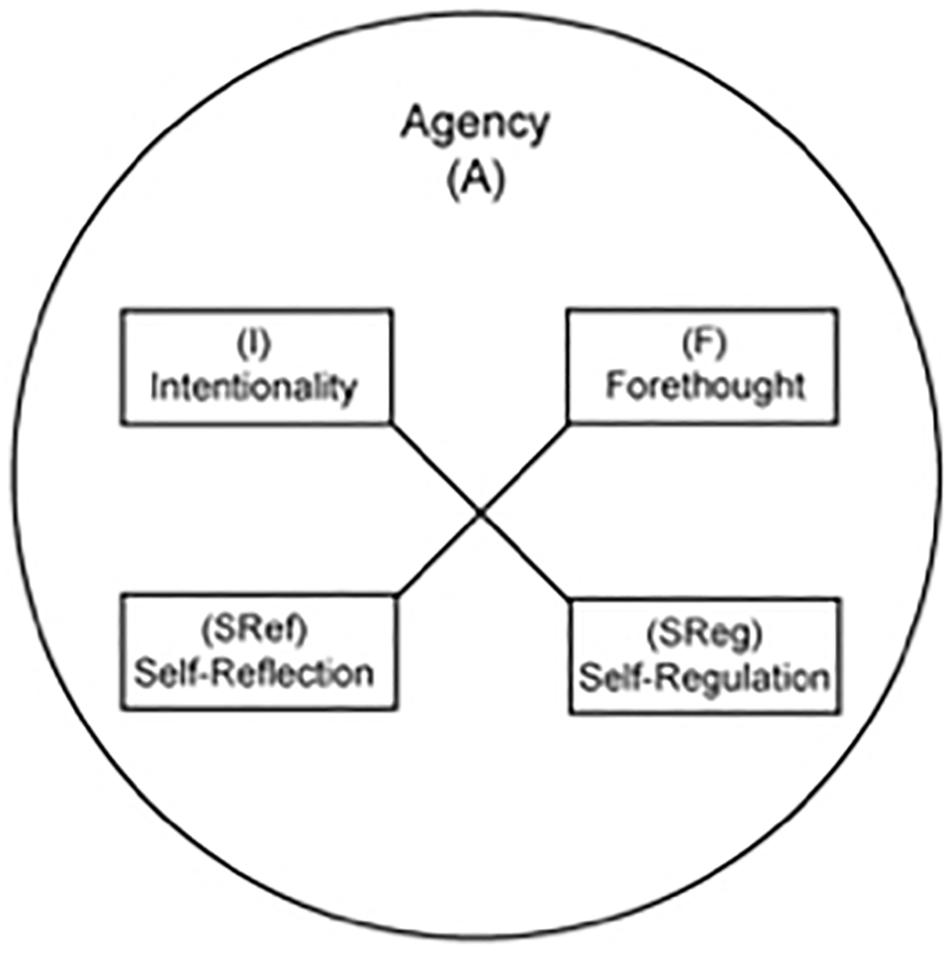
Figure 1. A visual conceptualization of agency. I is intentionality; F is forethought; SReg is self-regulation; SRef is self-reflection (self-efficacy); and A is agency. The solid lines represent intentional, conscious emergent influences.
Intentionality
Intentionality is an awareness and will to act in a particular way based on an idea or mental state (Lewis, 1990; Owen, 2009; Brownell, 2013; Noctor, 2017). Intentions are actualized through goal setting and planning. Planfulness involves the extent to which individuals report making rational, rather than impulsive, decisions (Hitlin and Elder, 2007). Students exhibit planful competence if they have the capability to select social settings that best match their goals, values, and strengths (Shanahan, 2000; Brady and Gilligan, 2019). This competence helps students to project their agency in an organized way over time. The projection of agency is managed by forethought.
Forethought
Forethought involves the ability to anticipate the outcomes of actions. Through the exercise of forethought, students motivate themselves and guide their actions in anticipation of future events. Self-determination theory distinguishes between three different types of motivation orientation and intentionality based on different goals (Deci and Ryan, 2000). Students are intrinsically motivated if they do something because it is inherently interesting or enjoyable, extrinsically motivated if they are externally driven into action and a-motivated if they do not value the activity. Once motivated to act, students regulate their behavior to achieve their established goals (e.g. Brady and Gilligan, 2019; Garcia-Martín and García-Sánchez, 2020).
Self-Regulation
Self-regulation is “an active, constructive process whereby learners set goals for their learning and then attempt to monitor, regulate, and control their cognition, motivation, and behavior, guided and constrained by their goals and the contextual features in the environment” (Pintrich, 2000, p. 453). In the development of academic competence, the strategies learners select and use are critical if they are to achieve their desired outcome. Self-regulation for academic achievement implies a conscious awareness and involves selecting and deploying appropriate strategies in order to achieve explicit or implicit learning goals (Jain and Dowson, 2009; Jansen et al., 2019). Agency develops through continuous reflection and evaluation on task progress.
Self-Efficacy
Self-efficacy is a functional self-awareness in which students reflect on their personal efficacy, thoughts, actions, the meaning of their pursuits, and make corrective adjustments if necessary (Bandura, 2006). Self-efficacy inherently involves students judging the correctness of their plans against the outcomes of their actions. This “metacognitive capability to reflect upon oneself and the adequacy of one’s thoughts and actions is the most distinctly human core property of agency” (Bandura, 2006, p. 165). Self-efficacy is a self-reflective belief in one’s capability to succeed and is an essential condition of human functioning (Bandura, 1997). These “beliefs act as determinants of behavior by influencing the choices that individuals make, the effort they expend, the perseverance they exert in the face of difficulties, and the thought patterns and emotional reactions they experience” (Pajares, 1996, p. 325). Self-efficacy beliefs contribute to a student’s sustained interest, motivation, and performance in school (Panadero et al., 2017; Garcia-Martín and García-Sánchez, 2018).
Agency for Learning
Agency for Learning (AFL; Code, 2010) is a necessary extension of social cognitive theory as it situates agentic capabilities as mediating factors between the effects of personal, behavioral, and social-environmental aspects on the self. Individual agency is expressed or constrained in different contexts for different reasons, thus it is important to consider agency from the perspective of the learner in the context of learning – in situ. AFL posits that agency “is an emergent entity that is manifested in individual abilities to interact with personal, behavioral, environmental, and social factors in the learning context” (Code, 2010, p. 2). Ultimately, agency is an emergent capability that is intentional, self-generated, and has external sources of influence. Emergence refers to “the arising of novel and coherent structures, patterns, and properties during the process of self-organization” (Goldstein, 1999, p. 49). A capability is emergent if it has several component parts but is irreducible with respect to them (O’Connor and Wong, 2002; Martin, 2003). Since agency emerges through self-generated intentional action; it can only be explained by the interaction between its component influences. These component influences are represented by an individual’s intentionality, forethought, self-regulation, and self-efficacy.
Agency is integral to students’ ability to regulate, control, and monitor their own learning. Students enact agency through their ability to regulate their cognitive, affective, and behavioral processes as they interact with factors in the environment which, “entails not only a behavioral skill in self-managing environmental contingencies, but also the knowledge and the sense of personal agency to enact this skill in relevant contexts” (Zimmerman, 2000, p. 14). AFL implies a more unified approach to the study of learning processes in individual and social settings. AFL presents agentic capabilities as mediating factors between personal, environmental, and behavioral processes. AFL is situated within a social cognitive view and extends this view by incorporating aspects of developmental, historical, and sociocultural theorizing that emphasize the integral nature of agency on the regulating processes necessary for learning. AFL presents a more complete picture of how students regulate and use their influence to meet personal and collective goals and provides a framework that enables further study of learning in formal and informal educational contexts.
Purpose of This Research
Students enact agency through their ability to regulate their cognitive, affective, and behavioral processes as they interact with factors in the environment. Aspects of intentionality, forethought, self-regulation, and self-efficacy are often studied independently in the literature (e.g. Wolters and Yu, 1996; Little, 1998; Loedewyk and Winne, 2005; Gestsdottir and Lerner, 2007; Kitsantas, 2008; Panadero et al., 2017; Jansen et al., 2019) but are often neither theoretically or empirically linked. These agentic factors need to be studied and interpreted collectively to fully examine the role agency plays in learning. AFL presents a framework in which to study agentic processes and provides a means to re-interpret existing findings (Code, 2010). The purpose of this research is to develop a self-report instrument that measures the multidimensional aspects of agency in learning. Using classical test theory and item response analysis this study examines the internal consistency, predictive validity, and the psychometric properties of this new instrument, the Agency for Learning Questionnaire (AFLQ).
Item Generation and Refinement
A pool of items corresponding to the multidimensional aspects of agentic functioning was generated based on several existing instruments used in educational psychological research. These instruments were selected for inclusion based on three criteria: (1) the instruments were to be designed and validated for the university or college aged student, (2) the instruments were selected based on their theoretical foundations and similarities to modes of agentic functioning, and (3) validation evidence for the instruments must have been available in the literature – internal consistencies must have been reported in a validation study and be above acceptable levels α0 > 0.70 (Tabachnick and Fidell, 2006). An initial item pool was formed by selecting questions from these instruments which assessed along the four component elements of agency described by AFL theory. To select items appropriate for assessing a range of ability levels along the four dimensions, a selection method outlined by Fletcher and Nusbaum (2010) was used to reduce the number of items in the pool and come up with the final AFLQ. This procedure included an analysis of content (to reduce redundancies and maximize content validity), factor loadings (to ensure relationship with construct, factorial validity, and construct validity), and fit with the IRT model. To produce an instrument that will assess a wide range of abilities items must have at minimum good item discrimination (a > 0.75), assess difficulties between −2.0 < θ < 2.0, and test information I(θ) > 4.0. Each of the measures selected as a basis for the item pool are described below.
Intention
The Melbourne Decision Making Questionnaire (MDMQ, Mann et al., 1997) and the Adolescent Decision-Making Questionnaire (ADMQ, Tunistra et al., 2000) measure decision making patterns based on decision conflict theory (see Janis and Mann, 1977). Decision conflict theory concerns decision-making styles through elements of self-confidence, vigilance, panic, evasiveness, and complacency. Janis and Mann (1977) classify these factors into two categories, adaptive and maladaptive decision-making patterns. An adaptive pattern involves carefully deliberated behavior such as vigilance and self-confident decision-making. Reported internal consistencies of the original scales that were used with several samples of university students are α = 0.63 for self-confidence and α = 0.80 for vigilance (Mann et al., 1997; Tunistra et al., 2000). Six items comprising the self-confidence subscale representing aspects of intentionality defined in AFL were adapted to measure decision competence (e.g. “The decisions I make turn out well”). Six items from the vigilance subscale representing aspects of planfulness in AFL were adapted to measure planful competence (e.g. “I try to be clear about my objectives before choosing”). Students indicated to what extent each of the statements corresponded to them using a five-point Likert scale (1 = does not correspond and 5 = corresponds exactly).
Intention and Forethought
The Academic Motivation Scale (AMS; Vallerand et al., 1992) measures academic motivation and intentionality based on the tenets of self-determination theory (see Deci and Ryan, 1985; Deci et al., 1991). The AMS is a 28-item inventory that measures intrinsic motivation (12 items), extrinsic motivation (12 items), and amotivation (4 items). Reported reliability estimates of the original French-Canadian version (EME, Vallerand et al., 1989) ranged from α = 0.76 to 0.86. For the translated English version reliability estimates ranged between α = 0.83 and 0.86. All items in the English version were added to the initial item pool. Students rated the degree to which each statement presently corresponded to the reasons they went to college on a five-point Likert scale (1 = does not correspond and 5 = corresponds exactly). An example of an intrinsic motivation item on this scale is “for the intense feelings I experience when I am communicating my own ideas to others” and extrinsic motivation item is “for the material and/or social benefits of being a University graduate.”
Self-Regulation
The volitional components inventory (VCI; Kuhl and Fuhrmann, 1998) is a 52-item inventory that measures competence in self-regulation (12 items, α = 81), self-control (8 items, α = 77), volitional development (12 items, α = 83), self-access (12 items, α = 82), and general life stress (8 items, α = 83). Only items from the self-regulation subscale of the VCI were added to the initial item pool. Students rated the degree to which each statement applied to them and their situation using a four-point Likert scale (1 = not at all and 4 = in full). An example of a self-regulation item is “when a task gets boring, I usually know how to make it interesting again.”
Self-Efficacy
The self-efficacy for self-regulated learning (SE-SRL) scale (11 items, α = 0.87) originally created by Bandura in 1989 and published by Zimmerman et al. (1992) was modified for use in this study. Students rated their degree of confidence on a list of tasks using a seven-point Likert scale (1 = not well at all and 7 = very well). An example of a self-efficacy item is “arrange a place to study without distractions.”
Study 1
Method
Participants (Sample 1, Year 1)
Data were collected via an anonymous Web survey from a convenience sample of second year undergraduate students (N = 1056) enrolled in five sections of organic chemistry taught by one of three instructors at a Canadian university. Students recruited consented to voluntary participation and received a bonus mark to their final course grade (0.5% bonus grade) and were given 8 weeks to complete the survey. The response rate was 73.5% (N = 776, 498 Female). Approximately 95% of the respondents were between the ages of 18 and 23.
Measures
An initial pool of 50 items was created from various instruments measuring agentic factors of intentionality and forethought (AMS; Vallerand et al., 1992), self-regulation (VCI; Kuhl and Fuhrmann, 1998), and self-efficacy (Zimmerman et al., 1992). The instructions and response scales were as follows.
• Intentionality and forethought (28 items): “Using the scale below, indicate to what extent each of the following items presently corresponds to one of the reasons why you go to college”; (1 = does not correspond and 5 = corresponds exactly).
• Self-regulation (11 items): “Please rate the degree to which each statement applies to you or your current situation, using the given scale”; (1 = not at all and 4 = in full).
• Self-reflectiveness (11 items): “Using the scale below, indicate to what extent each of the following statements presently corresponds to you”; (1 = not well at all and 7 = very well).
Data Analysis
A classical analysis, including an exploratory factor analysis (EFA) was conducted to assess the unidimensionality of each scale, a primary assumption required for unidimensional-IRT analysis (Hambleton et al., 1991). Following each scale’s classical analysis several IRT procedures were used. First, local item independence must be established. Local independence means that, when controlling for examinee trait level(s) the test items are not correlated (are independent). Local item independence is estimated using Yen’s (1993) Q3 statistic. Second, Yen’s (1981) Q1 and Drasgow et al.’s (1995) chi-square were used to determine the fit of the data to the IRT model chosen for this analysis. A 2PPC IRT model was selected because the items being analyzed are Likert-style. Finally, to analyze item parameters (difficulty and discrimination) and overall measurement characteristics, item response functions (IRF), item information functions (IIF), category response curves (CRC), test information functions (TIF), and standard error of measurement (SEM) graphs were used. The classical analytical procedures and factor analysis was performed. IRT analysis was performed using PARDUX (Burket, 2002), and graphing performed MODFIT (Stark, 2002).
Results
Academic Motivation Scale
Descriptive statistics
Table 1 presents the results of the classical analysis for the AMS. Items identified as poorly discriminating were 1, 5, 12, 19, and 26. Items 5, 12, 19, and 26 comprise the amotivation subscale. If CTT were the only procedure performed during this analysis it would be acceptable to remove these items completely. However, there was little additional information as to why the items on the amotivation subscale performed so poorly so further IRT analysis was warranted. The distribution of item correlations (CITC) was from −0.123 to 0.669.
Exploratory factor analysis
An EFA was used to assess latent dimensionality since the original validation of the AMS was preliminary (Vallerand et al., 1992) and further validation studies have demonstrated mixed results (e.g. Fairchild et al., 2005). Factors were extracted using Varimax rotation with Kaiser Normalization. The EFA on this data set revealed a three-factor structure (as based on the Scree plot): Intrinsic motivation (α = 0.92, CI95 = 0.91, 0.93), extrinsic motivation (α = 0.95, CI95 = 0.84, 0.87), and amotivation (α = 0.80, CI95 = 0.78, 0.83). All calculated internal consistencies were above the acceptable level of α0 > 0.70 (Tabachnick and Fidell, 2006). Several items identified in the literature as representing extrinsic motivation (items 3, 7, 10, 14, 17, 21, and 25) loaded on the first factor, intrinsic motivation. This finding is not surprising as there are known issues with the AMS and the simplex structure and motivation continuum on which it is based (see Fairchild et al., 2005). These challenges are either in the item construction or the theoretical foundation on which it is based (self-determination theory). From this point forward, these items were analyzed as part of the intrinsic motivation subscale.
Assessment of local dependence
Parameter estimations for the AMS are in Supplementary Table S1. Separate IRT analyses were performed on each of the three AMS scales. The success of an IRT analysis and the use of the 2PPC model depends on the assumption that the latent variable being measured is unidimensional and locally dependent. When items on a scale are locally dependent, TIF and reliabilities may be overestimated. Local dependence also indicates that the items may be assessing the same content. Yen’s Q3 statistic is a common way to infer this relationship. There were several clear violations of local independence where Q3 comparisons were significant at p < 0.01. The following groups of items on the intrinsic motivation scale were locally dependent: 6, 13, 11; 18, 16, 23; and 18, 25. The following groups of items on the extrinsic motivation scale were locally dependent: 3, 10, 7; 14, 7, 21; 14, 21; 1, 22, 7; 28, 14, 28; and 21, 28. No items on the amotivation scale were locally dependent. Items identified as locally dependent had the language revised to more accurately reflect the construct for Sample 2 in hopes of improving independence.
Estimation of IRT model-fit parameters
Yen’s Q1 chi-square goodness-of-fit test was computed for each item on the AMS. A Q1 value indicates whether the IRT model selected for the analysis was appropriate. Bolded items in Supplementary Table S1 indicate that these items do not fit the specified IRT model and have Q1 values outside the accepted range of |z| = 4.6. Items 5, 12, and 26 were removed due to their lack of fit to the IRT model as they had a χ2/df > 3. Items 19 and 27 could not be estimated because of an estimation failure and were removed from subsequent analysis. It was noted that items 5, 12, 19, and 26 comprise the amotivation subscale. Additional items with low discrimination values (a < 0.75) had the language revised to more accurately reflect the construct for Sample 2.
Self-Regulation Scale of the Volitional Components Inventory (SR-VCI)
Descriptive statistics
Results of the classical analysis of the SR-VCI are in Table 2. None of the items on this scale had a CITC < 0.25, had good overall discrimination, and differentiated well between students who rated the degree to which each statement applied to them. Item response frequencies are also listed in Table 2.
Exploratory factor analysis
An EFA was performed to assess latent dimensionality of the SR-VCI given that the population used for this study was culturally different than the original validation study (Kuhl and Fuhrmann, 1998) and only selected items from the VCI were used. Factors were extracted using Varimax rotation with Kaiser Normalization. The EFA on this data set revealed a one-factor structure for self-regulation (as based on the Scree plot). The internal consistency estimate for the SR-VCI subscale (α = 0.87, CI95 = 0.85, 0.88) was above the acceptable level of α0 > 0.70 (Tabachnick and Fidell, 2006).
Assessment of local dependence
Parameter estimations of items on the SR-VCI are presented in Supplementary Table S2. There were two violations of local independence on the SR-VCI where Q3 comparisons are significant at p < 0.01. The following grouped items are locally dependent: 32, 40; and 32, 41. The language of these items were revised to more accurately reflect the construct for Sample 2 in hopes of improving dimensionality.
Estimation of IRT model-fit parameters
Bolded items in Supplementary Table S2 indicate that these items do not fit the specified IRT model and have values outside the accepted range of |z| = 4.6. Items 28 and 41 were removed due to their lack of fit to the 2PPC model.
Self-Efficacy for Self-Regulated Learning
Descriptive statistics
Results of the classical analysis of the SE-SRL are in Table 3. None of the items on this scale had a CITC < 0.25, had good overall discrimination, and differentiated well between students who stated how confident they felt performing a particular task. Item response frequencies are also listed in Table 3.
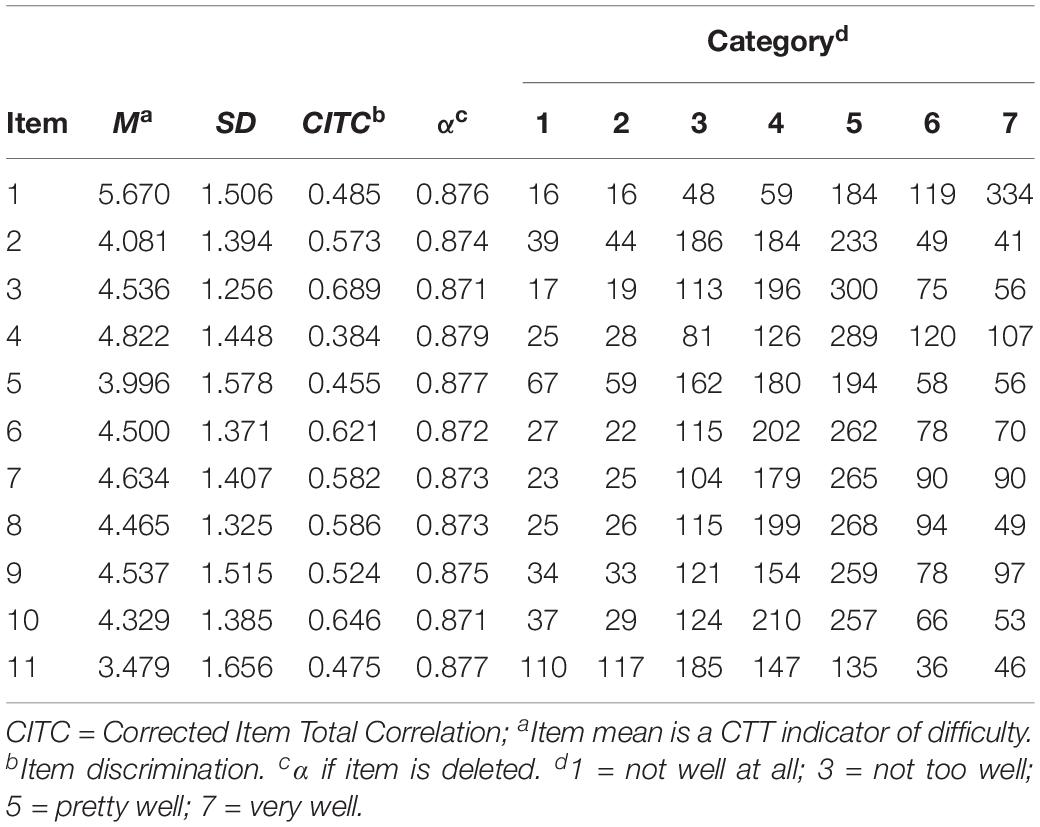
Table 3. Classical item analysis of self-efficacy for self-regulated learning scale (α = 0.87, CI95 = 0.85, 0.88).
Exploratory factor analysis
An EFA was used to assess latent dimensionality of the SE-SRL given that the population used for this study was post-secondary students and the original validation study was with school age children (Zimmerman et al., 1992). Factors were extracted using Varimax rotation with Kaiser Normalization. The EFA of this data set revealed a one-factor structure of SE-SRL (as based on the Scree plot). The internal consistency estimates for the SE-SRL subscale (α = 0.87, CI95 = 0.85, 0.88) were above the acceptable level of α0 > 0.70 (Tabachnick and Fidell, 2006).
Assessment of local dependence
Parameter estimations of items on the SE-SRL are presented in Supplementary Table S3. There was one violation of dimensionality where the Q3 comparison is significant at p < 0.01. Items 6 and 7 are locally dependent. The language of these items was revised to more accurately reflect the construct for Sample 2 to improve dimensionality.
Estimation of IRT model-fit parameters
All items listed in Supplementary Table S3 fit the specified IRT model and did not have values outside the accepted Q1 range of |z| = 4.6. Items 1, 4, 5, 8, 9, and 11 had low discrimination values (a < 0.75) therefore the language of these items were revised to more accurately reflect the construct for Sample 2.
Summary
Intentionality and Forethought
A three-factor model was revealed in the EFA of the AMS: Intrinsic motivation (α = 0.92, CI95 = 0.91, 0.93), extrinsic motivation (α = 0.95, CI95 = 0.84, 0.87), and amotivation (α = 0.80, CI95 = 0.78, 0.83). Several items theoretically associated with extrinsic motivation (7 items) loaded on the intrinsic motivation factor. There were eight violations of local dependence on the extrinsic motivation scale and four violations on the intrinsic motivation scales. These items were revised for Sample 2. The four items on the amotivation scale were removed due to their lack of fit to the IRT model. Two items on the amotivation scale were also identified as poorly calibrated as they had a χ2/df > 3. Five items on the extrinsic motivation scale reported poor discrimination (a < 0.75). The plots of the TIF and SEM for intrinsic motivation had the best measurement precision with I(θ) > 6.0 between theta values of −2.5 < θ < 2.5. The plots of the TIF and SEM for extrinsic motivation had the best measurement precision with I(θ) > 6.0 between theta values of −3.0 < θ < 1.0.
Self-Regulation
A single factor model was revealed in the EFA for SR-VCI: Self-regulation (α = 0.87, CI95 = 0.85, 0.88). There were two violations of local dependence. Two items on the scale did not fit the IRT model and were removed. Five items had low discrimination values (a < 0.75). The plots of the TIF and SEM for self-regulation had the best measurement precision with I(θ) > 4.0 between theta values of −3.0 < θ < 3.0.
Self-Efficacy
A single factor model was revealed in the EFA for SE-SRL: Self-efficacy (α = 0.87, CI95 = 0.85, 0.88). There was one violation of local dependence. Six items had low discrimination values (a < 0.75). The plots of the TIF and SEM for self-efficacy had the best measurement precision with I(θ) > 6.0 between theta values of −3.0 < θ < 3.0.
Study 2
The following changes were made to the item pool for administration to Sample 2. First, all items that violated the local dependence assumption and had low discrimination values were revised. Second, all items that did not fit the IRT model were removed. Third, items on the intentionality, forethought, and self-regulation scales were converted to a single Likert style inventory where students indicate to what extent each of the statements corresponds to them, using a 5-point scale (1 = does not correspond and 5 = corresponds exactly). To improve the discrimination of items on the self-efficacy scale the original Likert scale was changed from 7 options to 5 (1 = not confident and 5 = extremely confident). The instructions for each subscale were adapted to reflect the aforementioned changes. Finally, since the intentionality scale did not reveal any aspects of planfulness in the EFA, 12 items from the MDMQ (Mann et al., 1997) were modified to meet this requirement. An example of a planfulness item is “I consider how best to carry out a decision.” After these changes, there were 61 items remaining in the pool.
Method
Participants (Sample 2, Year 2)
Data were collected via an anonymous Web survey from a convenience sample of second year undergraduate students (N = 1097) enrolled in five sections of organic chemistry taught by one of three instructors at a Canadian university. Students recruited consented for voluntary participation were given a bonus mark for participating (0.25% bonus grade) and had 2 weeks to complete the survey. The response rate was 77.5% (N = 850, 517 female). Approximately 93% of the respondents were between the ages of 18 and 23.
Measures
The instructions and response scales were as follows.
• Intentionality (12 items) and self-regulation (11): “Using the scale below indicate to what extent each of the following items presently corresponds to you”; (1 = does not correspond and 5 = corresponds exactly).
• Forethought (27): “Using the scale below indicate to what extent each of the following items presently corresponds to one of the reasons why you go to University”; (1 = does not correspond and 5 = corresponds exactly).
• Self-reflectiveness (11 items): “Using the scale below rate your degree of confidence with each of the tasks”; (1 = not confident and 5 = extremely confident).
Data Analysis
The same analytical procedures from Study 1 were used with Study 2.
Results
Intention
Descriptive statistics
Results of the classical analysis of the intentionality scale are in Table 4. None of the items on this scale had a CITC < 0.25 indicating that all items had good overall discrimination and differentiated well between students of differing abilities. Item response frequencies are also listed in Table 4.
Exploratory factor analysis
An EFA was used to assess latent dimensionality given that the population used for this study was post-secondary students as opposed to school age children and adults in the original validation studies (Mann et al., 1997; Tunistra et al., 2000), and only selected items from these instruments were modified. Factors were extracted using Varimax rotation with Kaiser Normalization. The EFA on this data set revealed a two-factor structure of intentionality (as based on the Scree plot): Planfulness (α = 0.82, CI0.95 = 0.80, 0.84) and decision confidence (α = 0.82, CI0.95 = 0.80, 0.84). Internal consistency estimates for the subscales were above the acceptable level of α0 > 0.70 (Tabachnick and Fidell, 2006). Correlations between the planfulness and decision confidence subscales are significant at r = 0.357, p < 0.01. From this point forward analyses of each factor on the intentionality scale were completed separately.
Assessment of local dependence
Parameter estimations are presented in Supplementary Table S4. Items 4 and 5 violated the unidimensionality assumption where the Q3 comparison was significant at p < 0.01. Item 5 had the greater discrimination value and were kept for the final instrument.
Estimation of IRT model-fit parameters
Bolded items in Supplementary Table S4 indicate that these items do not fit the specified IRT model and had values outside the accepted range of |z| = 4.6. Items 4r, 5r, 10, 16, and 17r were removed due to their lack of fit to the IRT model as they had a χ2/df > 3.
Forethought
Descriptive statistics
Results of the classical analysis of the Forethought scale are in Table 5. None of the items on this scale had a CITC < 0.25, indicating that all items had good overall discrimination and differentiated well between students of differing abilities. Item response frequencies are also listed in Table 5.
Exploratory factor analysis
Based on findings from Sample 1, the following items were revised: 3, 7, 10, 14, 17, 21, and 25. An EFA was used to assess latent dimensionality because so many items were revised. Factors were extracted using Varimax rotation with Kaiser Normalization. The EFA on this data set revealed a two-factor structure (as based on the Scree plot): Intrinsic motivation (α = 0.92, CI95 = 0.91, 0.93) and extrinsic motivation (α = 0.95, CI95 = 0.84, 0.87). All calculated internal consistencies were above the acceptance threshold of α0 > 0.70 (Tabachnick and Fidell, 2006).
Correlations among the extrinsic and intrinsic motivation were r = 0.217, p < 0.01. Several items in the literature that represented extrinsic motivation (items 5, 10, 12, 18, 19, 24, 26, and 27) loaded on factor 1, intrinsic motivation. These items were removed from subsequent analysis because of this theoretical discrepancy.
Assessment of local dependence
Parameter estimations are presented in Supplementary Table S5. There were four violations of dimensionality (items 16, 20; and 11, 25) where Q3 comparisons were significant at p < 0.01. Items 20 and 25 were kept for the final instrument because they had the highest discrimination for each pair.
Estimation of IRT model-fit parameters
Bolded items in Supplementary Table S5 indicated that these items do not fit the specified IRT model and had values outside the accepted range of |z| = 4.6. Items 11, 17, 22, and 25 were removed due to their lack of fit to the IRT model.
Self-Regulation
Descriptive statistics
Results of the classical analysis of the self-regulation scale are in Table 6. None of the items on this scale had a CITC < 0.25, indicating that all had good overall discrimination and differentiated well between students of differing abilities. Item response frequencies are also listed in Table 6.
Exploratory factor analysis
All items were revised to include a Likert scale that was the same as the intentionality and forethought subscales. An EFA was used to assess latent dimensionality as a result of this change. Factors were extracted using Varimax rotation with Kaiser Normalization. The EFA on this data set revealed a one-factor structure (as based on the Scree plot): Self-regulation. In addition, internal consistency estimate for the self-regulation subscale (α = 0.91, CI95 = 0.90, 0.92) was above acceptable levels α0 > 0.70 (Tabachnick and Fidell, 2006). Note that the internal consistency increased as a result of changing the Likert scale.
Assessment of local dependence
Parameter estimations of items on the self-regulation scale are presented in Supplementary Table S6. There were no significant violations of local independence as assessed by Q3 comparisons at p < 0.01.
Estimation of IRT model-fit parameters
All items listed in Supplementary Table S6 have good discrimination (a < 0.9) and fit the 2PPC IRT model selected. Item 3 could not be estimated by PARDUX due to an estimation failure.
Self-Efficacy
Descriptive statistics
Results of the classical analysis of the self-reflectiveness scale are in Table 7. None of the items on this scale had a CITC < 0.25 indicating that all items had good overall discrimination and differentiated well between students who felt confident in performing a particular task. Item response frequencies are also listed in Table 7.
Exploratory factor analysis
An EFA was used to assess latent dimensionality because the Likert scale was changed following the analysis with Sample 1 to a five-point scale where students indicated their degree of confidence with each of the tasks (1 = not confident and 5 = extremely confident). Factors were extracted using Varimax rotation with Kaiser Normalization. The EFA on this data set revealed a one-factor structure of self-efficacy (as based on the Scree plot). The internal consistency estimates for the self-reflectiveness subscale (α = 0.88, CI95 = 0.86, 0.89) were above the acceptable level of α0 > 0.70 (Tabachnick and Fidell, 2006).
Assessment of local dependence
Parameter estimations of items on the self-reflectiveness scale are presented in Supplementary Table S7. There was one violation of local independence (items 3 and 4) where the Q3 comparison was significant at p < 0.01. Item 3 was chosen for the final instrument because it had the greatest discrimination.
Estimation of IRT model-fit parameters
All items listed in Supplementary Table S7 fit the specified IRT model and did not have values outside the accepted Q1 range of |z| = 4.6. Items identified during the Sample 1 analysis as having low discriminations, items 4 and 8, have improved. However, items 1, 5, and 11 still have low discrimination values and were removed from the final instrument.
Summary
Intention
A two-factor structure was revealed in the EFA of the intentionality scale: planfulness (6 items, α = 0.82, CI0.95 = 0.80, 0.84) and decision confidence (6 items, α = 0.82, CI0.95 = 0.80, 0.84). A total of five items were removed due to their lack of fit to the IRT model: two from the planfulness scale, and three from the decision confidence scale. Three of these items were also identified as being poorly calibrated as they had a χ2/df > 3. The plots of the TIF and SEM for planfulness had the best measurement precision with I(θ) > 4 between theta values of −3.5 < θ < 2.0. The plots of the TIF and SEM for decision confidence had the best measurement precision with I(θ) > 4 at theta of −3 and between theta values of −0.5 < θ < 0.5. This scale should be assessed with a different sample of students and refined to improve its measurement accuracy at a broader range of abilities.
Forethought
Based on the findings from Sample 1, 7 items were revised. A two-factor structure was revealed in the EFA of the forethought scale: Intrinsic motivation (α = 0.92, CI95 = 0.91, 0.93) and extrinsic motivation (α = 0.95, CI95 = 0.84, 0.87). However, 8 of the items that loaded on intrinsic motivation were theoretically supposed to be associated with extrinsic motivation. These 8 items were removed from analysis because of this disparity. Three items on these subscales were removed due to their lack of fit to the IRT model. Two of these three items were also identified as poorly calibrated as they had a χ2/df > 3. After all of the removals, there were 7 items left on the intrinsic motivation scale and 9 items on the extrinsic motivation scale. The plots of the TIF and SEM for intrinsic motivation were similar to Sample 1 with the best measurement precision with I(θ) > 6 between theta values of −3.0 < θ < 2.5. The plots of the TIF and SEM for extrinsic motivation were improved over Sample 1 with the best measurement precision with I(θ) > 4 between theta values of −2.0 < θ < 2.
Self-Regulation
Items on the self-regulation scale were revised to include the same Likert scale as the intentionality and forethought items. A single factor model was revealed in the EFA: Self-regulation (α = 0.91, CI95 = 0.90, 0.92). There was a slight increase in internal consistency from Sample 1 and there were no violations of dimensionality. All items on this subscale fit the IRT model however one item could not be estimated due to an estimation failure. The plots of the TIF and SEM for self-regulation were improved over Sample 1 with the best measurement precision with I(θ) > 6 between theta values of −2.0 < θ < 2.0.
Self-Efficacy
Items on the self-efficacy scale were revised to include a 5-point Likert scale asking students to rate their degree of confidence in completing a task. A single factor model was revealed in the EFA: Self-efficacy (α = 0.88, CI95 = 0.86, 0.89). Of the six items identified in Sample 1 as having low discrimination values (a < 0.75) three of them improved with Sample 2. The remaining three items were removed from the final instrument. The plots of the TIF and SEM for self-efficacy improved over Sample 1 with the best measurement precision with I(θ) > 6 between theta values of −3.0 < θ < 3.0.
The Agency for Learning Questionnaire
Following a process of selection outlined by Fletcher and Nusbaum (2010), two versions of the AFLQ are presented in Appendix A, B. Since Fletcher and Nusbaum (2010) used two independent data samples for cross validation which had a Ntotal = 742 further data collection for Sample 2 of the ALFQ research presented here was not warranted since Ntotal = 850. The Agency for Learning Questionnaire – Short Form (AFLQ-S, α = 0.90, CI95 = 0.89, 0.91) and the Agency for Learning Questionnaire – Long Form (AFLQ-L, α = 0.93, CI95 = 0.92, 0.96). The AFLQ-S has 28 items that measure agentic functioning along six dimensions: Intentionality – Planfulness (5 items), Intentionality – Decision Confidence (3 items), Forethought – Intrinsic Motivation (5 items), Forethought – Extrinsic Motivation (5 items), Self-Regulation – Self-Regulation (5 items), and Self-Reflectiveness – Self-Efficacy (5 items). The items on the AFLQ-S were selected based on their high discrimination values (a > 1.0). The AFLQ-L has 42 items that measure agentic functioning along six dimensions Intentionality – Planfulness (5 items), Intentionality – Decision Confidence (3 items), Forethought – Intrinsic Motivation (7 items), Forethought – Extrinsic Motivation (9 items), Self-Regulation – Self-Regulation (10 items), and Self-Reflectiveness – Self-Efficacy (8 items).
Predictive Validity Analyses
Previous analyses have shown that self-reports of motivation and SRL in university students tend to be negatively skewed (e.g. Pintrich et al., 1993). The means reported throughout this analysis show some evidence of being skewed. The means for planfulness (M = 3.73; SD = 0.627), decision confidence (M = 3.31; SD = 0.768), intrinsic motivation (M = 3.53; SD = 0.756), extrinsic motivation (M = 3.45; SD = 0.697), self-regulation (M = 2.92; SD = 0.756), and self-efficacy (M = 3.70; SD = 0.882) are all over 2.5 giving this distribution of scores a negative skew. These findings are consistent with previous work in this area. Correlations among the AFLQ-L scales suggest that the scales are related measures of the agentic processes. The planfulness, decision confidence, intrinsic motivation, extrinsic motivation, self-regulation, and self-efficacy scales were all positively correlated with one another, with r ranging from 0.11 to 0.57 at p < 0.01.
In terms of predictive validity of the scales on the AFLQ-L (Table 8), planfulness (r = 0.121, p < 0.01), decision confidence (r = 0.136, p < 0.01), intrinsic motivation (r = 0.141, p < 0.01), self-regulation (r = 0.114, p < 0.01), and self-efficacy (r = 0.263, p < 0.01) showed significant correlations with academic achievement at p < 0.01. Extrinsic motivation also showed a significant correlation with academic achievement (r = 0.088) at p < 0.05. These correlations are in the expected direction and are consistent with the literature. Students who said they approached their course work in a planful manner, also reported they were confident in their decisions, motivated to learn (either intrinsically or extrinsically), were good self-regulators, and had high self-efficacy for academic tasks were likely to achieve good grades. Overall, these student reports indicate a high degree of AFL.
Concluding Discussion
Results of this study indicate that the scales on the AFLQ used with two convenience samples of university students have excellent internal consistency, significant predictive validity, and strong psychometric properties. To further validate this instrument, alternative populations should be studied and non-convenience samples should be used. There are several implications to the development of the AFLQ as a multidimensional assessment of agency. First, this research provides a valid, multidimensional measure of AFL based on existing theoretical and empirical findings. Building upon existing research contributes to the content validity necessary to conduct further research with this instrument. Second, the AFLQ enables educational psychologists to potentially identify, measure, and study agentic processes in the context of learning and within particular experimental and developmental designs. Understanding how agency develops and emerges within learning environments is a key factor in identifying why learning occurs. Finally, this research enables further exploration into the role of agentic processes and how they operate collectively, perhaps function as significant predictors of academic achievement, and interact with various personal, behavioral, and social-environmental processes.
Students enact agency through their ability to regulate their cognitive, affective, and behavioral processes as they interact with factors in the environment. The multidimensional aspects of agency including intentionality, forethought, self-regulation, and self-reflectiveness have often been studied independently in the literature. However, in order to examine the role these processes play in learning they need to be studied and interpreted collectively. AFL (Code, 2010) provides a framework in which to explore the relationship of agency to various personal, behavioral, and social-environmental factors. Building upon this framework, the AFLQ provides a means to empirically investigate these processes within the learning context.
Data Availability Statement
The datasets generated for this study are available on request to the corresponding author.
Ethics Statement
The studies involving human participants were reviewed and approved by the Simon Fraser University and The University of British Columbia. The participants provided their written informed consent to participate in this study.
Author Contributions
The author confirms being the sole contributor of this work and has approved it for publication.
Conflict of Interest
The author declares that the research was conducted in the absence of any commercial or financial relationships that could be construed as a potential conflict of interest.
Acknowledgments
Research presented in this article is based on JC’s thesis (Code, 2010) at the Simon Fraser University completed under the supervision of Drs. John C. Nesbit, Jack Martin, and Kadriye Ercikan.
Supplementary Material
The Supplementary Material for this article can be found online at: https://www.frontiersin.org/articles/10.3389/feduc.2020.00019/full#supplementary-material
References
Bandura, A. (2000). Exercise of human agency through collective efficacy. Curr. Dir. Psychol. Sci. 9, 75–78. doi: 10.1111/1467-8721.00064
Bandura, A. (2001). Social cognitive theory: an agentic perspective. Annu. Rev. Psychol. 52, 1–26. doi: 10.1146/annurev.psych.52.1.1
Bandura, A. (2006). Toward a psychology of human agency. Persp. Psychol. Sci. 1, 164–180. doi: 10.1111/j.1745-6916.2006.00011.x
Beishuzen, J. (2008). Does a community of learners foster self-regulated learning? Technol. Pedag. Educ. 17, 183–193. doi: 10.1080/14759390802383769
Brady, E., and Gilligan, R. (2019). The role of agency in shaping the educational journeys of care-experiences adults: insights from a life course study of education and care. Child. Soc. 34, 121–135. doi: 10.1111/chso.12361
Brownell, P. (2013). “Assimilating/integrative: the case of contemporary gestalt therapy,” in Abnormal psychology. Abnormal psychology across the ages, Vol. 1. History and conceptualizations, Vol. 2. Disorders and treatments, Vol. 3. Trends and future directions, ed. T. G. Plante (Santa Barbara, CA: Praeger/ABC-CLIO), 221–234.
Code, J. (2010). Assessing agency for learning. Ph.D Doctoral Dissertation, Simon Fraser University, Burnaby, BC.
Deci, E., and Ryan, R. (1985). Intrinsic Motivation and Self-Determination in Human Behavior. New York, NY: Plenum.
Deci, E., and Ryan, R. (2000). Self-determination theory and the facilitation of intrinsic motivation, social development, and well-being. Am. Psychol. 55, 68–78.
Deci, E. L., Vallerand, R. J., Pelletier, L. G., and Ryan, R. M. (1991). Motivation in education: the self-determination perspective. Educ. Psychol. 26, 325–346.
deJong, F., Kolloffel, B., van der Meijden, H., Staarman, J. K., and Janssen, J. (2005). Regulative processes in individual, 3D and computer supported cooperative learning contexts. Comput. Hum. Behav. 21, 645–670.
Dinsmore, D. L., Alexander, P. A., and Loughlin, S. M. (2008). Focusing the conceptual lens on metacognition, self-regulation, and self-regulated learning. Educ. Psychol. Rev. 20, 391–409.
Drasgow, F., Levine, M. V., Tsien, S., Williams, B., and Mead, A. D. (1995). Fitting polytomous item response theory models to multiple choice tests. Appl. Psychol. Measure. 19, 143–165.
Fairchild, A. J., Horst, S. J., Finney, S. J., and Barron, K. E. (2005). Evaluating existing and new validity evidence for the Academic Motivation Scale. Contemp. Educ. Psychol. 30, 331–358.
Fletcher, T. D., and Nusbaum, D. N. (2010). Development of the competetive work environment scale: a multidimensional climate construct. Educ. Psychol. Measure. 70, 105–124.
Garcia-Martín, J., and García-Sánchez, J. N. (2018). The instructional effectiveness of two virtual approaches: processes and product. Rev. Psicodidáctica 23, 117–127. doi: 10.1016/j.psicod.2018.02.002
Garcia-Martín, J., and García-Sánchez, J. N. (2020). The effectiveness of four instructional approaches used in a MOOC promoting personal skills for success in life. Rev. Psicodidáctica 25, 36–44. doi: 10.1016/j.psicoe.2019.08.001
Gestsdottir, S., and Lerner, R. M. (2007). Intentional self-regulation and positive youth development in early adolescence: findings from the 4-H study of positive youth development. Dev. Psychol. 43, 508–521. doi: 10.1037/0012-1649.43.2.508
Greene, J., Bolick, C., and Robertson, J. (2010). Fostering historical knowledge and thinking skills using hypermedia learning environments: the role of self-regulated learning. Comput. Educ. 54, 230–243.
Hambleton, R. K., Swaminathan, H., and Rogers, H. J. (1991). Fundamentals of Item Response Theory. Newbury Park, CA: SAGE Publications, Inc.
Hitlin, S., and Elder, G. H. Jr. (2007). “Agency: an empirical model of an abstract concept,” in Constructing Adulthood: Agency and Subjectivity in Adolescence and Adulthood, ed. R. Macmillan (New York, NY: Elsevier), 33–67.
Jain, S., and Dowson, M. (2009). Mathematics anxiety as a function of multidimensional self-regulation and self-efficacy. Contemp. Educ. Psychol. 34, 240–249.
Janis, I., and Mann, L. (1977). Decision Making: A Psychological Analysis of Conflict, Choice and Commitment. New York, NY: The Free Press.
Jansen, R., van Leeuwen, A., Janssen, J., Jak, S., and Kester, L. (2019). Self-regulated learning partially mediates the effect of self-regulated learning interventions on achievement in higher education: a meta-analysis. Educ. Res. Rev. 28:100292. doi: 10.1016/j.edurev.2019.100292
Jarvenoja, H., and Jarvela, S. (2009). Emotion control in collaborative learning situations: do students regulate emotions evoked by social challenges? Br. J. Educ. Psychol. 79, 463–481. doi: 10.1348/000709909X402811
Karoly, P., Boekaerts, M., and Maes, S. (2005). Toward consensus in the psychology of self-regulation: how far have we come? How far do we have yet to travel? Appl. Psychol. 54, 300–311.
Kitsantas, A. (2008). Self-regulation and ability predictors of academic success dusing college: a predicitve validity study. J. Adv. Acad. 20, 42–68.
Kuhl, J., and Fuhrmann, A. (1998). “Decomposing self-regulation and self-control: the volitional components inventory,” in Motivation and Self-Regulation Across the Life Span, eds J. Heckhausen, and C. S. Dweck (New York, NY: Cambridge University Press), 15–19.
Lajoie, S. P. (2008). Metacognition, self-regulation, and self-regulated learning: a rose by any other name? Educ. Psychol. Rev. 20, 469–475.
Lewis, M. (1990). The development of intentionality and the role of consciousness. Psychol. Inq. 1, 231–247.
Little, T. (1998). “Sociocultural influences on the development of children’s action-control beliefs,” in Motivation and Self-Regulation Across the Life Span, eds J. Heckhausen, and C. Dweck (New York, NY: Cambridge University Press), 281–315.
Loedewyk, K., and Winne, P. (2005). Relations among the structure of learning tasks, achievement, and changes in self-efficacy in secondary students. J. Educ. Psychol. 97, 3–12.
Mann, L., Burnett, P., Radford, M., and Ford, S. (1997). The melbourne decision making questionnaire: an instrument for measuring patterns for coping with decisional conflict. J. Behav. Decis. Making 10, 1–19.
Martin, J. (2004). Self-regulated learning, social cognitive theory, and agency. Educ. Psychol. 39, 135–145.
Noctor, C. (2017). I Share Therefore I Am: A Narrative Inquiry Concerning Young Adults’ Experience of Personal Self-Disclosure on Facebook. Dublin, UK: Dublin City University.
O’Connor, T., and Wong, H. Y. (2002). “Emergent properties,” in Stanford Encyclopaedia of Philosophy, ed. E. N. Zalta (Stanford, CA: The Metaphysics Research Lab, Stanford University).
Owen, I. R. (2009). The intentionality model: a theoretical integration of psychodynamic talking and relating with cognitive–behavioral interventions. J. Psychother. Integr. 19, 173–186.
Pajares, F. (1996). Self-efficacy beliefs and mathematical problem-solving of gifted students. Contemp. Educ. Psychol. 21, 325–344. doi: 10.1006/ceps.1996.0025
Panadero, E., Jonsson, A., and Botella, J. (2017). Effects of self-assessment on self-regulated learning and self-effiacy: four meta-analyses. Educ. Res. Rev. 22, 74–98.
Pintrich, P. (2000). “The role of goal orientation in self-regulated learning,” in Handbook of Self-Regulations, eds M. Boekaerts, P. Pintrich, and M. Zeidner (San Diego, CA: Academic Press), 451–502.
Pintrich, P. (2004). A conceptual framework for assessing motivation and self-regulated learning in college students. Educ. Psychol. Rev. 16, 385–407.
Pintrich, P., Smith, D. A. F., Garcia, T., and McKeachie, W. J. (1993). Reliability and predictive validity of the motivated strategies for learning questionnaire (MSLQ). Educ. Psychol. Measure. 53, 801–813.
Schunk, D. H. (2008). Metacognition, self-regulation, and self-regulated learning: research recommendations. Educ. Psychol. Rev. 20, 463–467. doi: 10.2174/1874434600903010076
Shanahan, M. J. (2000). Pathways to adulthood in changing societies: variability and mechanisms in life course perspective. Ann. Rev. Sociol. 26, 667–692.
Tabachnick, B., and Fidell, L. (2006). Using Multivariate Statistics, 5th Edn. New York, NY: Harper & Row.
Tunistra, J., van Sonderen, F. L. P., Groothoff, J. W., van den Huevel, W. J. A., and Post, D. (2000). Reliability, validity and structure of the adolescent decision making questionnaire among adolescents in the Netherlands. Pers. Individ. Differ. 28, 273–285.
Vallerand, R. J., Blais, M. R., Briere, N. M., and Pelletier, L. G. (1989). Construction et validation de l’Echelle de Motivation en Education (EME). Can. J. Behav. Sci. 21, 323–349.
Vallerand, R. J., Pelletier, L. G., Blais, M. R., Briere, N. M., Senecal, C., and Vallieres, E. F. (1992). The academic motivation scale: a measure if intrinsic, extrinsic, and amotivation in education. Educ. Psychol. Measure. 52, 1003–1017.
Wolters, C., and Yu, S. (1996). The relation between goal orientation and students’ motivational beliefs and self-regulated learning. Learn. Individ. Differ. 8, 211–238.
Yen, W. M. (1981). Using simulation results to choose a latent trait model. Appl. Psychol. Measure. 5, 245–262. doi: 10.3389/fpsyg.2017.00806
Yen, W. M. (1993). Scaling performance assessments: strategies for managing local item dependence. J. Educ. Measure. 30, 197–213.
Zimmerman, B. (2000). “Attaining self-regulation: a social cognitive perspective,” in Handbook of Self-Regulation, eds M. Boekaerts, P. Pintrich, and M. Zeidner (San Diego, CA: Academic Press).
Appendix A
Appendix B
Keywords: learner agency, self-efficacy, self-regulated learning, motivation, intention, AFLQ
Citation: Code J (2020) Agency for Learning: Intention, Motivation, Self-Efficacy and Self-Regulation. Front. Educ. 5:19. doi: 10.3389/feduc.2020.00019
Received: 20 October 2019; Accepted: 10 February 2020;
Published: 26 February 2020.
Edited by:
Michael S. Dempsey, Boston University, United StatesReviewed by:
Pedro Daniel Ferreira, University of Porto, PortugalJudit García-Martín, University of Salamanca, Spain
Copyright © 2020 Code. This is an open-access article distributed under the terms of the Creative Commons Attribution License (CC BY). The use, distribution or reproduction in other forums is permitted, provided the original author(s) and the copyright owner(s) are credited and that the original publication in this journal is cited, in accordance with accepted academic practice. No use, distribution or reproduction is permitted which does not comply with these terms.
*Correspondence: Jillianne Code, amlsbGlhbm5lLmNvZGVAdWJjLmNh