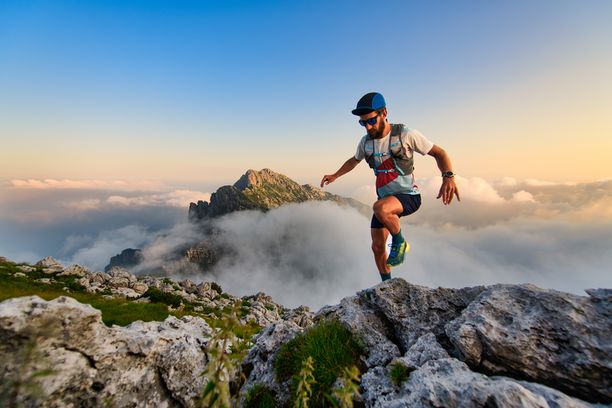
94% of researchers rate our articles as excellent or good
Learn more about the work of our research integrity team to safeguard the quality of each article we publish.
Find out more
PERSPECTIVE article
Front. Educ., 01 October 2019
Sec. Assessment, Testing and Applied Measurement
Volume 4 - 2019 | https://doi.org/10.3389/feduc.2019.00106
This article is part of the Research TopicAdvances in Classroom Assessment Theory and PracticeView all 7 articles
Assessment is prevalent throughout all levels of education in the United States. Even though educators are familiar with the idea of assessing students, familiarity does not necessarily ensure assessment literacy, or data-based decision making (DBDM) knowledge. Assessment literacy and DBDM are critical in education, and early childhood education in particular, to identify and intervene on potential barriers to students' development. Assessment produces data and evidence educators can use to inform intervention and instructional decisions to improve student outcomes. Given this importance and educators' varying levels of assessment literacy and DBDM knowledge, researchers, and educators should be willing to meet in the middle to support these practices and improve student outcomes. We provide perspective for half of the equation– how researchers can contribute– by addressing important considerations for the design and development of assessment measures, implications of these considerations for future measures, and a case example describing the design, and development of an assessment tool created to support DBDM for educators in early childhood education.
Educational assessment occurs throughout all levels of the education system in the United States (prekindergarten to grade 12 [PreK-12] and postsecondary/university levels). Assessments such as grade-level standardized tests, college entrance exams, and educator-developed measures each provide data to serve different purposes including: learning, achievement, and college readiness (e.g., Goertz and Duffy, 2003; U.S. Department of Education, 2016). Given assessment's prevalence, educators are not inexperienced with the idea of assessing students. However, assessment familiarity does not necessarily ensure assessment literacy or the knowledge needed to engage in using assessment data to inform instruction at the group or individual level. This process is generally referred to as data-based decision making (DBDM; Tilly, 2008).
Data-based decision making (DBDM) involves evaluating, administering, interpreting, and applying high-quality assessment tools and the data they produce, while assessment literacy entails having the fundamental measurement knowledge and skills to engage in such tasks (Stiggins, 1991; Stiggins and Duke, 2008; Brookhart, 2011; Popham, 2011; Mandinach and Jackson, 2012). Engaging in DBDM allows educators to measure student learning, inform instruction and intervention, and support educational decision-making.
While the importance of assessment literacy and DBDM resonates for educators across grade levels, here we argue the importance, and future of assessment literacy and DBDM in early childhood (defined here as preschool). This manuscript provides an overview of the fundamental need for assessment literacy and DBDM in early childhood education (ECE) and describes its future as a joint effort between the researchers who develop measures and the educators who use them. We include an example from our work in early childhood assessment. The manuscript concludes with considerations for the future development of measures to support early childhood educators' assessment literacy and support their DBDM practices.
Early childhood is a period of rapid development where young learners build foundational knowledge and skills in academic and social-emotional domains NRC, 2000; Shonkoff and Phillips, 2000. Early identification and intervention on potential barriers to children's development during this time allows for a better chance students will be successful as they grow (Missall et al., 2007; Welsh et al., 2010; e.g., Duncan and Sojourner, 2013). The likelihood of preschool services helping achieve these outcomes improves when instruction is guided by thorough screening assessments, and ongoing monitoring of student progress and intervention need. Thus, assessment and DBDM are integral components of educators' professional role (Young and Kim, 2010; Hopfenbeck, 2018).
Assessment produces data and evidence educators can use to inform intervention and instructional decisions to improve student outcomes (e.g., Shen and Cooley, 2008; Popham, 2011; Mandinach and Jackson, 2012). One example of this is the multi-tiered systems of support (MTSS) framework utilized in both K-12 (e.g., Fletcher and Vaughn, 2009; Johnson et al., 2009) and ECE settings (e.g., VanDerHeyden and Snyder, 2006; Bayat et al., 2010; Ball and Trammell, 2011; Carta and Young, 2019). The aim of MTSS is to more effectively educate students by focusing on early and immediate intervention to prevent future problems (Greenwood et al., 2011; Ball and Christ, 2012). The MTSS framework assumes common outcomes for all students through dynamic allocation of intervention resources using “tiered” approaches like those in public health interventions (Tilly, 2008). Universal screening identifies students who are successful with the general curriculum (i.e., Tier 1) or who may benefit from more intensive instruction (i.e., Tier 2/targeted services and Tier 3/intensive services). Progress monitoring assesses student's response to intervention. Depending on the student's performance, educators can change the intervention intensity.
An underlying assumption of MTSS is that educators are conducting ongoing assessments and progress monitoring of student performance. Thus, frameworks like MTSS can encourage sound empirical problem-solving by guiding early childhood educators in using data to make instructional decisions, which in turn improves student outcomes (Fuchs and Fuchs, 1986; VanDerHeyden et al., 2008; Greenwood et al., 2011; Ball and Christ, 2012; VanDerHeyden and Burns, 2018).
Additionally, assessment literacy and DBDM are important competencies because it is beneficial for educators to put time, energy, and resources toward psychometrically rigorous assessment tools. Such tools provide usable data on intervention needs and results, with relevance for future academic outcomes. Educators' use of weak, poorly designed tools that produce unreliable data can lead to potentially harmful decisions (Shonkoff and Phillips, 2000; NRC, 2008; NCII, 2013). Poorly-designed assessment tools are marked by little or no evidence of technical adequacy, limited evidence of validity, complex logistical demands, bias in evaluation of student subgroups, and failure to give educators timely access to data (Kerr et al., 2006; Lonigan, 2006; NRC, 2008; AERA, 2014). When educators are able to recognize poorly designed tools, they can allocate resources toward rigorous tools more likely to positively influence their DBDM practices and student outcomes.
Former United States secretary of education Arne Duncan was a strong advocate for DBDM, stating:
“I am a believer in the power of data to drive our decisions…It tells us where we are, where we need to go, and who is most at risk. Our best teachers today are using real time data in ways that would have been unimaginable just 5 years ago” (Duncan, 2009).
What needs to happen so Duncan's “best teachers” becomes all educators? We believe assessment literacy and DBDM need to become a shared responsibility between researchers and educators.
Assessment literacy is a critical competency for educators, but there is variation in their preparation, competence, and confidence (Volante and Fazio, 2007; Popham, 2011; Mandinach and Jackson, 2012). This is particularly true in ECE where our system is fragmented and less developed than the kindergarten to grade 12 (K-12) system (McConnell, 2019). Not all early childhood educators complete educator preparation programs. Those who do are still not necessarily equipped with the assessment and DBDM knowledge and skills needed in their future classrooms.
Since educator training and experience varies, we argue educators and the researchers developing measures should be prepared to meet one another in the middle. When both sides work to improve their respective contributions to assessment literacy and DBDM, there is increased likelihood for practices and student outcomes to improve. On the side of educators, this could mean increasing assessment literacy and DBDM knowledge through improved teacher education programs or offering professional development training protocols explicitly personalized to educators' interests and preferences (Fox et al., 2011; Artman-Meeker et al., 2015; Schildkamp and Poortman, 2015). For researchers creating measures, this could be addressed in the design of features and uses of a particular set of measures. We turn to the importance and implications of design details next.
Good design will lead to data collection requirements that can be implemented with fidelity in real-world settings, and reporting systems that assist educators in making decisions efficiently. Utility of measures can be enhanced through design and implementation of more adaptive user interfaces and reporting formats that provide information in intuitive, actionable, and supported ways. Such features can lead educators directly to analysis and decision-making. This approach requires researchers to consider how to make it easier for educators to use data to produce improved outcomes with appropriate resources and supports.
Several features of assessments can be considered to promote educators' use of DBDM. Through a systematic literature review and focus groups (Hoogland et al., 2016) identified prerequisites for the implementation of data-based decision making, such as a school's DBDM culture, teacher knowledge and skills, and collaboration. Here we expand upon their assessment instruments and processes prerequisite by discussing how it relates to measure design and development.
Historically, researchers developing measures have been held to standards of psychometric rigor, including evidence of measure validity, and reliability (AERA, 2014). It is critically important the measures and data educators use to inform their instruction have good reliability and validity evidence that allows interpretations to align with the intended uses. To achieve these standards, measures need to be “precise” and sensitive to change over time. One method to improve the precision of assessments is to use an item response theory (IRT) framework (Wilson, 2005; Rodriguez, 2010) to develop items and measures. Within an IRT framework, test items that represent a continuum of ability within the test domain are developed and then calibrated. In an IRT model items are scaled on a logit metric and error is reduced by utilizing adaptive models that allow students to interact with items at or near their true ability (e.g., computer adaptive testing).
Social validity, coined by Wolf (1978), refers to the importance and significance of a program (Schwartz and Baer, 1991). It is used in intervention research to assess three components; (a) social significance of intervention goals, (b) social acceptability of intervention procedures, and (c) social importance of outcomes (Wolf, 1978). These three elements can also be applied to educational assessments: (a) social significance—is the measure assessing a socially important behavior/construct?; (b) social acceptability—is the measure—including test items—deemed appropriate by the users?; and (c) social importance—are the users satisfied with the outcome of the measure? (e.g., in the context of DBDM the utility of the measure identifying current student achievement or monitoring progress). If educators deem an assessment to be socially acceptable and to produce socially important and significant results, the likelihood of making instructional decisions that will improve student outcomes increases (Gresham, 2004). One way to increase social validity is by collaborating with educators in the design and implementation of DBDM tools.
Despite early childhood educators reporting the importance of collecting data, efforts can be periodic, and non-systematic (e.g., Cooke et al., 1991; Sandall et al., 2004). One frequently reported barrier is lack of time available to collect, analyze, and interpret data (e.g., Means et al., 2011; Pinkelman et al., 2015; Wilson and Landa, 2019). To increase the likelihood of early childhood educators using DBDM, measures need to be accessible, easy-to-use, feasible for use in applied settings, time efficient, and resource efficient. General Outcome Measures (GOMs) are one example. These are assessments that reflect the overall content of a domain or curriculum and provide information regarding current achievement and progress over time, both at an individual, and group level (Fuchs and Deno, 1991; Fuchs and Fuchs, 2007). In addition, they are easy and quick to administer, and have rigorous psychometric properties, making them ideal assessments for DBDM.
With increased demands on educators' time, implementing strategies that are easy to use, fast to implement, and result in desired student outcomes is imperative. Recently, researchers have focused on incorporating technology into DBDM (e.g., cloud-based systems: Buzhardt et al., 2010; Johnson, 2017 and app-based: Kincaid et al., 2018).
Technology provides potential solutions to facilitate assessment processes. It can improve the efficiency with which educators can collect data and increase the effectiveness with which they interpret the results and implement interventions (Ysseldyke and McLeod, 2007). Improving the speed with which educators can collect data and receive feedback reduces time constraints and burdens (Sweller, 1988; Ysseldyke and McLeod, 2007). As a result, there is potential for data collection efficiency to improve and the frequency with which educators can monitor student progress to increase. Collecting data using technology-enabled devices means responses can be automatically entered, scored, and analyzed. This both reduces the likelihood of errors and eliminates the duplication of data collection efforts common with paper-and-pencil measures. Providing access to data in real-time supports educators in determining intervention effectiveness and using data to make meaningful, quick, and accurate instructional decisions (Feldman and Tung, 2001; Kerr et al., 2006).
Educators also need to be able to make accurate decisions from data. Hojnoski et al. (2009) found interpretative accuracy—using two data points to draw a conclusion—was higher for methods that were rated as less preferred and acceptable. These results highlight the delicate balance between social validity and presentation methods that promote accurate decision-making that needs to be considered when developing measures. A potential way to achieve this balance is to present data in multiple ways (e.g., tables, line graphs, and narrative summaries, etc.). Kerr et al. (2006) found positive academic outcomes were greatest when educators had access to multiple sources and types of data for analysis and instructional decision making. Additionally, educators need data analyzed and summarized in an actionable way (Feldman and Tung, 2001). Educators may have a variety of preferences and needs for how data are summarized. These needs may depend on the type of decisions they are looking to make (e.g., group instructional changes, small group progress, or effectiveness of an intervention on student progress), or based on the educator's skill and training in using data (Schildkamp and Poortman, 2015).
Technology affords educators opportunities to customize data reports by applying filters, aggregations, and visualizations. Leveraging technology-based adaptations and solutions to assessment systems to improve efficiency and effectiveness of the DBDM process can increase the likelihood educators can quickly and accurately use information to make timely instructional decisions and improve student outcomes (Wayman and Stringfield, 2006).
Assessment practices vary with a range of purposes, audiences, and intended uses. To the extent assessment is expected to contribute to improved outcomes, it is likely specific prerequisites must be met. The categories of design requirements described previously can be appended to any measure design and evaluation process with reasonable expectation the result will be more likely to contribute to overall improvement of student and educator performance.
Assessment practices must produce information directly relevant to mid- and long-term, socially valued outcomes. This conceptual and statistical alignment must be present at all levels of assessment, from evaluations of intervention need, to measures of individuals' response to intervention, to outcomes for individuals and groups. Only when assessment results meet rigorous psychometric standards and are aligned in this way can interventions be arrayed to achieve expected effects. Additionally, assessment practices must produce actionable information that leads, with little ambiguity, to specific interventions. This is a “last mile” of measure design and development; only when educators have interventions that reliably show effects on future measures, and only when those measures are aligned with socially important outcomes, can we have confidence that assessment results lead to achievement gains.
To this point, we've detailed ways assessment literacy and DBDM can progress in education and their critical need in ECE. One strategic approach to realize these aims during the design process is engaging researchers with educators who will use the tool. Here we describe an exemplar of this process to illustrate how researchers and educators can work together to design tools and DBDM supports.
Early literacy and language development is an important hallmark of early childhood development (NELP, 2008). Psychometrically rigorous assessments designed for use in MTSS are available in the field (e.g., Individual Growth and Development Indicators [IGDIs], McConnell et al., 2011; Phonological Awareness Literacy Screener [PALS-PreK], Invernizzi et al., 2004; Preschool Early Literacy Indicators [PELI], Kaminski et al., 2013), however few pair their rigorous design process with complementary explicit design to support DBDM.
The IGDIs took on this need, developing a tablet application designed to facilitate DBDM. The app, IGDI-Automated Performance Evaluation of Early Language and Literacy (APEL) is designed to support educators in assessing students within a MTSS framework and immediately providing information to help them act on student scores. When educators use IGDI-APEL they assess preschool-age students on five measures of language and early literacy through a yoked tablet experience. Figure 1 shows a sample of the main classroom screen on which educators can interact with their students' scores.
Figure 1. Screenshot of the IGDI-APEL main classroom screen. It shows a list of students in the class with their scores and tier designations on the five early literacy and language measures.
IGDI-APEL was strategically designed in partnership with educators through a 4-year Institute of Education Sciences grant. For 3 years of the project, local early childhood educators were engaged through focus groups, surveys, and informal discussions to provide feedback about the app. They provided recommendations on features, functionality, and utility such as how to improve the graph's readability, the benefits of automatic scoring in comparison paper-pencil assessments, and the ease of use in their busy classrooms. Including end users as meaningful contributors to the design team allowed the app to integrate requirements of psychometric rigor and functional utility. This helped ensure the final product would be something educators would want to use and could do so successfully, while also maintaining psychometric integrity.
To successfully support educators' use of IGDIs data, it was important to consider how to support them in their classroom experiences. These educators are pressed for time and have dramatic variability in education and training levels. Given these constraints, the app needed to be efficient, provide real-time data, and tailor to each educator's level. IGDI-APEL generates scores in real-time to encourage educators to immediately consider the student's performance. Additionally, once three data points are available, trend and slope information are available and alert boxes appear to facilitate the experience for educators unsure how to interpret the graphs (Figure 2 shows a graph of classroom performance on the Sound Identification measure). Further, if the educator is ready to implement an intervention, the app can guide them to resources inventorying evidence-based practices to pair student performance with empirically proven interventions. These in-app experiences leverage concepts presented in behavioral economics, where optimized behaviors are “nudged” toward reinforcement (Wilkinson and Klaes, 2017). This reinforcement cycle is solidified when an educator actively engages the app, makes an intentional instructional change, and observes a change in student performance. The behavioral momentum within this cycle supports the educator continuing the process with additional students, and in engaging with more resources to support DBDM.
Figure 2. Screenshot of a group classroom graph for one measure—Sound Identification. Stars indicate seasonal screening points and circles indicate progress monitoring points. The colors associate with MTSS tiers- green for Tier 1, yellow for Tier 2, and red for Tier 3. The group classroom graph is one of the resources available to educators in the app in addition to individual graphs, group tables and videos on psychometric concepts.
In concert, these features have a strong theoretical foundation for driving changes in teacher behavior, and secondarily, student outcomes. Appropriately examining the empirical effectiveness of the system requires studies that systematically evaluate the features designed to improve efficiency, data interaction, and intervention selection through robust methods (i.e., random-control trials). Specifically, evidence to support the degree to which teacher behavior meaningfully changed and student outcomes improved is an important factor in ensuring the design specifications translate to practical improvements in practice. Our future papers will address results of IGDI-APEL's empirical effectiveness.
Assessment literacy and DBDM are critical competencies for educators and ones that can be further developed as a result of efforts from both researchers and educators. For researchers, educators' assessment literacy and DBDM knowledge and skills need to be at the forefront as they design measures and data reporting formats to support educators' practices. Ideally, supporting DBDM use through improvements in measure design and the presentation of scores will result in easy to use, efficient, and feasible measures that produce meaningful data presented in a user-friendly way.
In the end, the goal of researchers and educators is the same– produce improved assessment practices through assessment literacy and DBDM that directly contribute to improved instruction and intervention opportunities and better student outcomes. While we have focused on the contributions researchers can bring to measure development, these suggestions alone will not solve the need for increased educator competencies in assessment literacy and DBDM. We are hopeful the outlined suggestions can drive future work of researchers to support educators, as well as student outcomes, but also acknowledge there is more work to be done when it comes to training and preparing early childhood educators.
KW and SM contributed to the conception and design of the article. KW organized the outline of the article. KW, SM, ME, EL, and AW-H wrote sections of the manuscript. All authors contributed to manuscript revision and read and approved the submitted version.
This work was supported by the Institute of Education Sciences, Grant Number: R305A140065. All statements offered in this manuscript are the opinion of the authors and official endorsement by IES should not be inferred.
SM and AW-H have developed assessment tools and related resources known as Individual Growth and Development Indicators and Automated Performance Evaluation of Early Language and Literacy. This intellectual property is subject of technology commercialization by the University of Minnesota, and portions have been licensed to Renaissance Learning Inc., a company which may commercially benefit from the results of this research. These relationships have been reviewed and are being managed by the University of Minnesota in accordance with its conflict of interest policies.
The remaining authors declare that the research was conducted in the absence of any commercial or financial relationships that could be construed as a potential conflict of interest.
AERA, APA, and NCME. (2014). Standards for Educational and Psychological Testing. Washington DC: American Educational Research Association.
Artman-Meeker, K., Fettig, A., Barton, E. E., Penney, A., and Zeng, S. (2015). Applying an evidence-based framework to the early childhood coaching literature. Topics Early Child. Spec. Educ. 35, 183–196. doi: 10.1177/0271121415595550
Ball, C. R., and Christ, T. J. (2012). Supporting valid decision making: Uses and misuses of assessment data within the context of RTI. Psychol. Schools 49, 231–244. doi: 10.1002/pits.21592
Ball, C. R., and Trammell, B. A. (2011). Response-to-intervention in high-risk preschools: critical issues for implementation. Psychol. Sch. 48, 502–512. doi: 10.1002/pits.20572
Bayat, M., Mindes, G., and Covitt, S. (2010). What does RTI (response to intervention) look like in preschool? Early Child. Edu. J. 37, 493–500. doi: 10.1007/s10643-010-0372-6
Brookhart, S. (2011). Educational assessment knowledge and skills for teachers. Edu. Measure. Issues Pract. 30, 3–12. doi: 10.1111/j.1745-3992.2010.00195.x
Buzhardt, J., Greenwood, C., Walker, D., Carta, J., Terry, B., and Garrett, M. (2010). A web-based tool to support data-based early intervention decision making. Topics Early Child. Spec. Educ. 29, 201–213. doi: 10.1177/0271121409353350
Carta, J. J., and Young, R. M. (Eds.). (2019). Multi-Tiered Systems of Support for Young Children: Driving Change in Early Education. Baltimore, MD: Brookes Publishing.
Cooke, N., Heward, W. L., Test, D. W., Spooner, F., and Courson, F. H. (1991). Student performance data in the classroom: Measurement and evaluation of student progress. Teach. Edu. Special Edu. 14,155–161. doi: 10.1177/088840649101400301
Duncan, A. (2009). “Robust data gives us the roadmap to reform,” in Speech Made at the Fourth Annual IES Research Conference (Washington, DC). Available online at: http://www.ed.gov/news/speeches/~2009/06/06-82009.html
Duncan, G. J., and Sojourner, A. J. (2013). Can intensive early childhood intervention programs eliminate income-based cognitive and achievement gaps? J. Human Resour. 48, 945–968. doi: 10.3368/jhr.48.4.945
Feldman, J., and Tung, R. (2001). “Whole school reform: how schools use the data-based inquiry and decision making process,” in Paper Presented at the 82ndAnnual Meeting of the American Educational Research Association (Seattle, WA).
Fletcher, J. M., and Vaughn, S. (2009). Response to intervention: preventing and remediating academic difficulties. Child Dev. Perspect. 3, 30–37. doi: 10.1111/j.1750-8606.2008.00072.x
Fox, L., Hemmeter, M. L., Snyder, P., Binder, D. P., and Clarke, S. (2011). Coaching early childhood special educators to implement a comprehensive model for promoting young children's social competence. Topics Early Child. Spec. Educ. 31, 178–192. doi: 10.1177/0271121411404440
Fuchs, L. S., and Deno, S. L. (1991). Paradigmatic distinctions between instructionally relevant measurement models. Except. Child. 57, 488–500. doi: 10.1177/001440299105700603
Fuchs, L. S., and Fuchs, D. (1986). Effects of systematic formative evaluation: a meta-analysis. Except. Child. 53, 199–208. doi: 10.1177/001440298605300301
Fuchs, L. S., and Fuchs, D. (2007). “The role of assessment in the three-tier approach to reading instruction” in Evidence-based Reading Practices for Response to Intervention, eds D. Haager, J. Klingner, and S. Vaughn (Baltimore, MD: Brookes Publishing, 29–44.
Goertz, M., and Duffy, M. (2003). Mapping the landscape of high-stakes testing and accountability programs. Theory Pract. 42, 4–12. doi: 10.1207/s15430421tip4201_2
Greenwood, C., Bradfield, T., Kaminski, R., Linas, M., Carta, J. J., and Nylander, D. (2011). The response to intervention (RTI) approach in early childhood. Focus Except. Child. 43, 1–24. doi: 10.17161/foec.v43i9.6912
Gresham, F. M. (2004). Current status and future directions of school-based behavioral interventions. School Psych. Rev. 33, 326–343.
Hojnoski, R. L., Caskie, G. I., Gischlar, K. L., Key, J. M., Barry, A., and Hughes, C. L. (2009). Data display preference, acceptability, and accuracy among urban head start teachers. J. Early Interv. 32, 38–53. doi: 10.1177/1053815109355982
Hoogland, I., Schildkamp, K., van der Kleij, F., Heitink, M., Kippers, W., Veldkamp, B., et al. (2016). Prerequisites for data-based decision making in the classroom: research evidence and practical illustrations. Teach. Teach. Edu. 60, 377–386. doi: 10.1016/j.tate.2016.07.012
Hopfenbeck, T. N. (2018). ‘Assessors for learning’: understanding teachers in contexts. Assess. Edu. Principles Policy Pract. 25, 439–441. doi: 10.1080/0969594X.2018.1528684
Invernizzi, M., Sullivan, A., Meier, J., and Swank, L. (2004). Phonological Awareness Literacy Screening: Preschool (PALS-PreK). Charlottesville, VA: University of Virginia.
Johnson, E., Smith, L., and Harris, M. (2009). How RTI Works in Secondary Schools. Thousand Oaks. CA: Corwin Press.
Johnson, L. D. (2017). Exploring cloud computing tools to enhance team-based problem solving for challenging behavior. Topics Early Child. Spec. Educ. 37, 176–188. doi: 10.1177/0271121417715318
Kaminski, R. A., Bravo Aguayo, K. A., Good, R. H., and Abbott, M. A. (2013). Preschool Early Literacy Indicators Assessment Manual. Eugene, OR: Dynamic Measurement Group.
Kerr, K. A., Marsh, J. A., Ikemoto, G. S., Darilek, H., and Barney, H. (2006). Strategies to promote data use for instructional improvement: actions, outcomes, and lessons from three urban districts. Am. J. Edu. 112, 496–520. doi: 10.1086/505057
Kincaid, A. P., McConnell, S., and Wackerle-Hollman, A. K. (2018). Assessing early literacy growth in preschoolers using individual growth and development indicators. Assess. Effect. Inter. doi: 10.1177/1534508418799173. [Epub ahead of print].
Lonigan, C. J. (2006). Development, assessment, and promotion of preliteracy skills. Early Educ. Dev. 17, 91–114. doi: 10.1207/s15566935eed1701_5
Mandinach, E. B., and Jackson, S. S. (2012). Transforming Teaching and Learning Through Data-driven Decision Making. Thousand Oaks, CA: Corwin Press.
McConnell, S. R. (2019). “The path forward for Multi-tiered Systems of Support in early education,” in Multi-Tiered Systems of Support for Young Children: Driving Change in Early Education, eds J. J. Carta and R. M. Young (Baltimore, MD: Brookes Publishing), 253–267.
McConnell, S. R., Wackerle-Hollman, A. K., Bradfield, T. A., and Rodriguez, M. C. (2011). Individual Growth and Development Indicators: Early Literacy Plus. St. Paul, MN: Early Learning Labs.
Means, B., Chen, E., DeBarger, A., and Padilla, C. (2011). Teachers' Ability to Use Data to Inform Instruction: Challenges and Supports. Washington, DC: U.S. Department of Education, Office of Planning, Evaluation, and Policy Development.
Missall, K., Reschly, A., Betts, J., McConnell, S., Heistad, D., Pickart, M., et al. (2007). Examination of the predictive validity of preschool early literacy skills. School Psych. Rev. 36, 433–452.
NCII (2013). Data-Based Individualization: A Framework for Intensive Intervention. Washington, DC: Office of Special Education Programs, U.S. Department of Education.
NELP (2008). Developing early Literacy: Report of the National Early Literacy Panel. Executive Summary. Washington, DC: National Institute for Literacy.
NRC (2000). Eager to Learn: Educating Our Preschoolers. Washington, DC: The National Academies Press.
NRC (2008). Early Childhood Assessment: Why, What, and How. Washington, DC: The National Academies Press.
Pinkelman, S. E., McIntosh, K., Rasplica, C. K., Berg, T., and Strickland-Cohen, M. K. (2015). Perceived enablers and barriers related to sustainability of school-wide positive behavioral interventions and supports. Behav. Disord. 40, 171–183. doi: 10.17988/0198-7429-40.3.171
Popham, J. (2011). Assessment literacy overlooked: a teacher educator's confession. Teach. Educ. 46, 265–273. doi: 10.1080/08878730.2011.605048
Rodriguez, M. C. (2010). Building a Validity Framework for Second-Generation IGDIs (Technical Reports of the Center for Response to Intervention in Early Childhood). Minneapolis: University of Minnesota.
Sandall, S. R., Schwartz, I. S., and Lacroix, B. (2004). Interventionists' perspectives about data collection in integrated early childhood classrooms. J. Early Interv. 26, 161–174. doi: 10.1177/105381510402600301
Schildkamp, K., and Poortman, C. (2015). Factors influencing the functioning of data teams. Teach. Coll. Rec. 117, 1–42.
Schwartz, I. S., and Baer, D. M. (1991). Social validity assessments: Is current practice state of the art? J. Appl. Behav. Anal. 24, 189–204. doi: 10.1901/jaba.1991.24-189
Shen, J., and Cooley, V. E. (2008). Critical issues in using data for decision-making. Int. J. Leader. Educ. 11, 319–329. doi: 10.1080/13603120701721839
Shonkoff, J. P., and Phillips, D. A. (Eds.). (2000). “From neurons to neighborhoods: the science of early childhood development,” in Board on Children, Youth, and Families, Commission on Behavioral and Social Sciences and Education (Washington, DC: National Academy Press).
Stiggins, R., and Duke, D. (2008). Effective instructional leadership requires assessment leadership. Phi Delta Kappan 90, 285–291. doi: 10.1177/003172170809000410
Sweller, J. (1988). Cognitive load during problem solving: effects on learning. Cogn. Sci. 12, 257–285. doi: 10.1207/s15516709cog1202_4
Tilly, W. D. (2008). “The evolution of school psychology to a science-based practice: Problem solving and the three-tiered model,” in Best Practices in School Psychology V, eds A. Thomas and J. Grimes (Washington, DC: National Association of School Psychologists, 17–36.
U.S. Department of Education (2016). “Every student succeeds act,” in Assessments under Title I, Part A and Title I, Part B: Summary of Final Regulations. Available online at: https://www2.ed.gov/policy/elsec/leg/essa/essaassessmentfactsheet1207.pdf
VanDerHeyden, A. M., and Burns, M. K. (2018). Improving decision making in school psychology: making a difference in the lives of students, not just a prediction about their lives. School Psych. Rev. 47, 385–395. doi: 10.17105/SPR-2018-0042.V47-4
VanDerHeyden, A. M., and Snyder, P. (2006). Integrating frameworks from early childhood intervention and school psychology to accelerate growth for all young children. School Psych. Rev. 35, 519–534.
VanDerHeyden, A. M., Snyder, P. A., Broussard, C., and Ramsdell, K. (2008). Measuring response to early literacy intervention with preschoolers at risk. Topics Early Child. Spec. Educ. 27, 232–249. doi: 10.1177/0271121407311240
Volante, L., and Fazio, X. (2007). Exploring teacher candidates' assessment literacy: implications for teacher education reform and professional development. Canadian J. Edu. 30, 749–770. doi: 10.2307/20466661
Wayman, J. C., and Stringfield, S. (2006). Technology-supported involvement of entire faculties in examination of student data for instructional improvement. Am. J. Edu. 112, 549–571. doi: 10.1086/505059
Welsh, J. A., Nix, R. L., Blair, C., Bierman, K. L., and Nelson, K. E. (2010). The development of cognitive skills and gains in academic school readiness for children from low-income families. J. Educ. Psychol. 102, 43–53. doi: 10.1037/a0016738
Wilson, K. P., and Landa, R. J. (2019). Barriers to educator implementation of a classroom-based intervention for preschoolers with autism spectrum disorder. Front. Edu. 4:27. doi: 10.3389/feduc.2019.00027
Wilson, M. (2005). Constructing Measures: An Item Response Modeling Approach. Mahwah, NJ: Lawrence Erlbaum.
Wolf, M. M. (1978). Social validity: the case for subjective measurement or how applied behavior analysis is finding its heart. J. Appl. Behav. Anal. 11, 203–214. doi: 10.1901/jaba.1978.11-203
Young, V., and Kim, D. (2010). “Using assessments for instructional improvement,” in Educational Policy Analysis Archives. Available online at: http://epaa.asu.edu/ojs/article/view/809 doi: 10.14507/epaa.v18n19.2010
Keywords: assessment literacy, data-based decision making, early childhood, educational assessment, early childhood educators
Citation: Will KK, McConnell SR, Elmquist M, Lease EM and Wackerle-Hollman A (2019) Meeting in the Middle: Future Directions for Researchers to Support Educators' Assessment Literacy and Data-Based Decision Making. Front. Educ. 4:106. doi: 10.3389/feduc.2019.00106
Received: 27 April 2019; Accepted: 12 September 2019;
Published: 01 October 2019.
Edited by:
Sarah M. Bonner, Hunter College (CUNY), United StatesReviewed by:
Jana Groß Ophoff, University of Tübingen, GermanyCopyright © 2019 Will, McConnell, Elmquist, Lease and Wackerle-Hollman. This is an open-access article distributed under the terms of the Creative Commons Attribution License (CC BY). The use, distribution or reproduction in other forums is permitted, provided the original author(s) and the copyright owner(s) are credited and that the original publication in this journal is cited, in accordance with accepted academic practice. No use, distribution or reproduction is permitted which does not comply with these terms.
*Correspondence: Kelsey K. Will, d2lsbDM1NTlAdW1uLmVkdQ==
Disclaimer: All claims expressed in this article are solely those of the authors and do not necessarily represent those of their affiliated organizations, or those of the publisher, the editors and the reviewers. Any product that may be evaluated in this article or claim that may be made by its manufacturer is not guaranteed or endorsed by the publisher.
Research integrity at Frontiers
Learn more about the work of our research integrity team to safeguard the quality of each article we publish.