- University of California, Riverside, Riverside, CA, United States
Research shows that students' non-academic attributes, such as forms of engagement, dispositions, and social and emotional skills, are associated with a range of outcomes—including academic performance. However, little research has investigated the effects of school peer non-academic attributes on academic performance. This study begins to address this gap in the research literature by examining the effects of 10 measures of school peer non-academic attributes. Importantly, most of the non-academic attributes examined in this study are malleable through school-based interventions. The results show that five measures of school peer attributes have medium to large effects on academic performance, including collaboration skills (a social-emotional characteristic), conscientiousness and belonging (dispositions), and cognitive and behavioral engagement. In contrast, the corresponding effects of students' own non-academic attributes were uniformly smaller. These findings indicate that the non-academic attributes of one's school peers play an important role in academic performance above and beyond one's own non-academic attributes. These results suggest that school-wide interventions and instructional practices designed to improve the non-academic skills of all students will provide additional benefits compared with interventions focusing on students with the most under-developed non-academic attributes.
Introduction
Research shows that students' non-academic attributes, also referred to as non-cognitive skills and twenty-first century skills, are associated with academic performance (Durlak et al., 2011; Farrington et al., 2012; OECD, 2013, 2015). Other research shows that the student composition of the school one attends, especially socioeconomic composition, can influence a range of student outcomes (Palardy, 2008, 2013, 2015b, in press; Borman and Dowling, 2010; Perry and McConney, 2010; Van Ewijk and Sleegers, 2010; Konstantopoulos and Borman, 2011). However, little research has specifically examined the associations between school peers' non-academic attributes and academic performance. This is a noteworthy gap in the literature because research suggests that school peers influence a range of student attitudes, aspirations, and outcomes, including behavior, academic performance, educational aspirations, and drug use (Hallinan and Williams, 1990; Mounts and Steinberg, 1995; Jang, 2002; Cook et al., 2007; Palardy, 2013). In addition to peer influences on one another through direct interactions, non-academic attributes of the student body may also contribute to student outcomes indirectly through various mechanisms, such as teachers' expectations, choices of instructional practices, academic culture, and disciplinary climate and practices (Coleman et al., 1966; Thrupp, 1999; Jussim and Harber, 2005; Palardy et al., 2015; Berkowitz et al., 2017).
The student and school non-academic risk factors examined in the present study include forms of student engagement at school, student dispositions, and student interpersonal and intrapersonal skills. Research suggests that these student skills tend to be malleable (Farrington et al., 2012; Gutman and Schoon, 2013). A recent study by Loeb et al. (2019) found that the growth rate of these skills varies substantially across schools, suggesting that schools play a central role in their development. Furthermore, the growth in these skills among schools is correlated with growth in achievement, suggesting that certain non-academic skills facilitate achievement (West et al., 2018). They also tend to impact educational and life outcomes (Heckman and Kautz, 2014). Malleability and impact make them excellent targets for interventions to reduce student risks.
Literature Review
This section outlines two research literatures. The first is on peer composition effects at school. Because very little research has focused specifically on peer non-academic attribute composition, this literature is general. The second literature on the associations between non-academic attributes and academic performance at school. While individual student attributes are not the primary focus of the current study, this literature is well-established and provides a context for interest in their peer composition effects.
Peer Composition Effects
The effects of peer composition at school has been an enduring topic in educational effectiveness and sociology of education studies for over 50 years (Coleman et al., 1966; Erbring and Young, 1979; Firebaugh, 1979; Willms, 1986; Harker and Tymms, 2004; Rumberger and Palardy, 2005; Ludtke et al., 2008; Perry and McConney, 2010; Van Ewijk and Sleegers, 2010; Konstantopoulos and Borman, 2011; Palardy and Rumberger, 2019; Palardy, in press). Peer composition effects, which are also referred to as school composition effects in the research literature, are conceptualized as being above and beyond or “controlling for” the students' own backgrounds (Willms, 1986; Raudenbush and Willms, 1995). Hence, these effects occur when the group mean of an individual measure is associated with an outcome even after controlling for individual effect (Firebaugh, 1979).
The associations between peer composition and student outcomes may be due to direct peer interactions at school or to indirect mechanisms such as teacher and school practices that tend to be concomitant with certain peer composition measures (Palardy, 2015a). Within the theoretical literature on child and adolescent development, peer composition at school can be considered a social or environmental factor within Bronfenbrenner's (1979) ecological framework. Because youths spend considerable time in schools, peer composition may influence a range of developmental outcomes. This is particularly the case in middle and high school, when peer effects tend to peak (Jang, 2002; Reynolds et al., 2014; Patacchini et al., 2017).
The literature on compositional effects has two intertwined strands. One strand focuses on theoretical and empirical work describing mechanisms through which school peer composition influences individual outcomes. The other strand focuses on methodological issues related to the measurement and statistical modeling of peer composition effects. The following sections reviews both of these strands.
Mechanisms Through Which Peer Composition Impacts Outcomes
The research literature on student body compositional effects in schools began with the landmark Coleman Report in 1966, which concluded that the mean socioeconomic status (SES) of the school had the largest effect on student achievement of any school factor (Coleman, 1990).1 Since then, a number of recent studies have drawn similar conclusions as the Coleman Report, including two recent reanalyses of Coleman's data using more sophisticated statistical models (Rumberger and Palardy, 2005; Borman and Dowling, 2010; Perry and McConney, 2010; Van Ewijk and Sleegers, 2010; Konstantopoulos and Borman, 2011; Palardy, 2013, in press).
There are two leading theories for the mechanisms through which peer composition impacts student outcomes. The first is through social interaction or peer influences at school (Erbring and Young, 1979; Willms, 1986). That is, peers tend to influence each other's attitudes and values, motivations and behaviors, and academic achievement and performance (Dreeben and Barr, 1988; Jencks and Mayer, 1990). Peer composition effects may also work through differential access to school resources, such as effective teachers, school structures (e.g., small classes), and school practices (e.g., academic press and disciplinary practices) (Willms, 1986; Dreeben and Barr, 1988; Greenwald et al., 1996; Lee and Smith, 1999; Rumberger and Palardy, 2005). Bowles and Gintis (1976) contend that schools tend to organize around their student composition, which plays a role in non-academic skill development that perpetuates social reproduction. For example, schools serving low-SES students tend to put a greater emphasis on conforming to rules and procedures and obedience to authority, whereas schools serving middle- and high-SES students put a greater emphasis on student initiative and creativity. These differential emphases tend to impair educational and career prospects in low-SES schools and enhance them in medium- and high SES schools (Bowles and Gintis, 1976; Thrupp, 1999; Palardy, 2013). Bowles and Gintis (1976) argue that, due in large part to this school composition-based organization, schooling tends to impact students' future employment opportunity and success more through the development of non-academic skills than cognitive skills. However, it is unclear whether the peer composition effects of non-academic factors are associated with academic performance, which is a focus of the current study.
Methodological Literature on Peer Composition
There is debate on the best approach for estimating peer composition effects. There is a consensus that model specification plays a central role in the magnitude and direction of estimates. Indeed, some have argued that the magnitude of composition effects tends to be overestimated and, in some cases, is a “phantom effect” due to model misspecification (Harker and Tymms, 2004). One issue that can lead to these problems is unreliability measurement of the corresponding student variable (e.g., unreliable measure of student SES when modeling peer composition SES). When reliability is low, the effect of the student measure on the outcome tends to be underestimated, which typically results in an overestimation of the peer composition effect. That is because the compositional effect is supposed to be above and beyond the student effect and a reliable measure of the student variable is needed to adequately control for the student effect. When it does not serve that role adequately, the part of the student effect that is unaccounted for is largely subsumed by the compositional effect. Marsh and his associates developed doubly latent models to overcome this issue, but the models require multiple measures of each student and compositional variable being tested (Ludtke et al., 2008; Marsh et al., 2009), and the complexity of these models often results in estimation problems (e.g., failure to reach convergence criteria).
Another model methodological issue that can result in inflated peer composition effects is not including a lagged covariate of the outcome in the model. That is because compositional effects are supposed to capture aspects of the school environment that impact the outcome, but student levels on the outcome upon entering the school cannot be attributed to the school environment. Using a lagged measure of the outcome to control for the outcome when students entered the school addresses this issue (Harker and Tymms, 2004).
Gap in the Literature
Few studies have examined the effect of non-academic compositional effects on achievement, grades, or other measures of academic performance. One study examining elementary school classrooms found that peer externalizing behaviors impact achievement (Neidell and Waldfogel, 2010). In a study substantively close to the current study, West et al. (2016) found that some school aggregate measures of student non-academic skills are associated with mean school achievement in a sample of eighth graders attending Boston public schools. Furthermore, some of these compositional effects were much larger than the student-level effect, including growth mindset, suspension rate, and absence rate. These findings support the notion that peer composition measures of non-academic skills can impact student achievement and, in some cases, are larger than the corresponding student effect.
Non-academic Attributes and Academic Performance
Bowles and Gintis (1976) provided the early conceptualization and supporting research for how non-academic attributes influence educational and labor market success. They concluded that while an adequate level of cognitive skills is necessary, once reached, non-academic attributes are more essential. Over the past 40 years, a large body of research crosscutting education, economics, psychology, and other social sciences has documented a range of non-academic attributes that impact educational or life outcomes. This research largely supports Bowles and Gintis's conclusions (Farkas et al., 1990; Dweck, 1999; Bowles et al., 2001; Farkas, 2003; Cunha and Heckman, 2007; Duckworth et al., 2007; National Research Council, 2012). This review focuses on three types of non-academic attributes or skills that research suggests are most critical to academic performance at school: engagement, social, and emotional skills, and dispositions.
Engagement: Behavioral, Cognitive, and Emotional
Engagement at school is a multifaceted construction typically consisting of three major components: behavioral, cognitive, and emotional engagement (Fredricks et al., 2004). Each component has been defined and measured in various ways. This review focuses on the most common usages in the research literature.
Behavioral engagement most generally refers to student behavior and conduct at school related to conforming to norms, adhering to school rules, and exhibiting “problem behaviors,” such as getting in trouble, being late, and skipping school without permission (Finn, 1993; Finn et al., 1995; Finn and Rock, 1997; Palardy et al., 2015). Behavioral Engagement is predictive of achievement, grades, and high school completion (Finn, 1993; Finn et al., 1995; Fredricks et al., 2004; Gutman and Schoon, 2013). Fredricks et al. (2004, p. 70) concluded that “there is a consistent association between teacher and student reports of behavioral engagement and achievement across a variety of samples.” With regard to grades, however, the direct effect of behavioral engagement on academic performance may be overestimated because part of that effect is due to teachers punishing disruptive students by reducing their grade. That is, the total effect of behavioral engagement on grades consists of the direct effect of the behaviors (e.g., if a student is disruptive in class they may miss an opportunity to learn, which results in lower achievement and grades) and the indirect effect of punitive actions taken by the teacher (in addition to missing an opportunity to learn, the teacher punishes the student for disrupting class by lowering his or her grade).
Cognitive engagement pertains to efforts expended and strategies used to learn academic content and develop academic skills, particularly on complex content and skills that are difficult to master (Wang et al., 2011; Li and Lerner, 2013). Several studies have linked Cognitive Engagement with achievement. Specifically, students who use metacognitive strategies to regulate attention and effort, such as advanced organizers to relate new curricular content with prior knowledge, tend to exhibit higher achievement (Zimmerman, 1990; Boekaerts et al., 2000). Cognitive Engagement is also predictive of grades (Fredricks et al., 2004); however, as was the case for Behavioral Engagement, that effect may be overestimated because teachers often grade in part on effort and persistence, which are elements of Cognitive Engagement.
According to Fredricks et al. (2004, p. 60), “Emotional Engagement encompasses positive and negative reactions to teachers, classmates, academics, and school and is presumed to create ties to an institution and influence willingness to do the work.” Students may exhibit their level of Emotional Engagement through outward expressions (e.g., of interest, boredom, enthusiasm, etc.). Of the three forms of engagement, Emotional Engagement has by far the weakest link with academic outcomes. According to Fredricks et al. (2004), the studies that do find significant links tend to have measurement concerns, such as combining elements of emotional and behavioral engagement in the same measure. Emotional Engagement is predictive of dropout, however (Rumberger, 1987; Alexander et al., 1997).
Dispositions
Research has linked student dispositions (e.g., self-efficacy, sense of belonging, hope, and purpose) with academic performance. Self-efficacy is perhaps the most extensively studied of these. Bandura (1997) defined self-efficacy as the “beliefs in one's capabilities to organize and execute the courses of action required to manage prospective situations” (p. 2). It is associated with motivation, academic choices (e.g., whether to take courses or major in subjects perceived to be challenging), and achievement (Pajares, 1996). Sense of Belonging is the degree to which students feel accepted, respected, and supported in the school social environment (Goodenow and Grady, 1993). It has been linked with student grades, which may be due in part to its association with effort (Goodenow, 1993). Hope or optimism about the future is associated with a range of other dispositions and educational outcomes and is predictive of achievement even after controlling for other factors, such as intelligence, grades, and self-esteem (Snyder et al., 1997; Snyder, 2002). It is also predictive of well-being (Gilman et al., 2006). Damon et al. (2003) defined Sense of Purpose to be a stable intention to accomplish something that is both personally meaningful and has perceived impacts beyond oneself. It is associated with student achievement, an effect that seems to work through students' future orientation and coping strategies, although these mechanisms are not well-understood (Damon, 2008).
Social and Emotional Skills
Interpersonal or social skills are “learned behaviors that enable a person to interact effectively with others” (Gresham and Elliott, 1990, p. 1). This includes a variety of skills, many of which center around communication or facilitate gainful collaboration. Most of the research on the effects of interpersonal skills on student performance is in the broader area of social and emotional skills. However, few studies have examined the effects of individual facets of interpersonal skills, such as communication or collaboration skills (Gutman and Schoon, 2013). Intrapersonal skills are the self-directed mechanisms within a person's mind that facilitate self-regulation, planning, and awareness. One of the most studied of these is Conscientiousness—the tendency to be responsible, hardworking, organized, and punctual. A review of the research literature on non-academic skills concluded that, of the Big Five personality traits, Conscientiousness is the most strongly predictive of educational attainment, job performance, longevity, and delinquency (negative association) (Kautz et al., 2014). This literature also includes a substantial body of research specifically examining the association between Conscientiousness and academic performance. For example, recent meta-analyses found that the effect of conscientiousness on academic performance is both largely independent of intelligence and has a comparable effect on academic performance as intelligence (Poropat, 2009). Also, a review of the postsecondary research literature concluded that, of the Big Five personality traits, conscientiousness is the strongest predictor of academic performance in college (Trapmann et al., 2007).
As insinuated in this review, non-academic skills tend to be intercorrelated (Gutman and Schoon, 2013; Kautz et al., 2014). Hence, examining one skill at a time results in inflated estimates of the effects of individual factors on outcomes. Despite this methodological concern, very little correlational research has been conducted that estimates the effects of individual factors while controlling for the intercorrelations of others (Gutman and Schoon, 2013). Moreover, there are serious design challenges in conducting randomized experiments to estimate the causal effects of non-academic factors on outcomes. Therefore, more correlational work that examines individual factors while controlling for the intercorrelations of other factors is needed.
Research Questions
The present study uses a sample of students and schools from Sacramento, California, to estimate the effect size of 10 different student and corresponding peer composition non-academic risk factors on Academic Performance. The following research questions are addressed:
1. Do the effects of student's non-academic skills on Academic Performance vary across schools? This question addresses the degree to which the effects of non-academic skills on Academic Performance depends on the school one attends.
2. How does the magnitude of the effects of the student's own non-academic skills on Academic Performance compare with the magnitude of the effects of corresponding peer composition measures?
Methods
Data
This study uses a sample of 2,541 students attending 25 high schools in Sacramento, California. All students were tenth graders in 2014. This sample is part of the International Study of City Youth (ISCY), which was designed to examine school effectiveness in several cities worldwide (Lamb et al., 2015).2 Tenth graders were drawn from a representative sample of schools in Sacramento to facilitate student- and school-level analyses. In 2014, students were administered online versions of the Programme for International Student Assessment (PISA) achievement tests for reading and math as well as a questionnaire that with items focusing on non-academic skills, grades, plans, views, and outlooks.
Sacramento is served by five school districts, some of which include parts of surrounding suburbs. All five districts were asked to participate, and four agreed. ISCY staff provided districts with letters of introduction to students, parents, teachers, and school administrators, as well as permission forms, and the districts then recruited participants for the study. Some schools and students that were recruited elected not to participate. The final sample included 25 schools (18 traditional, 3 alternative, 2 charter, 1 magnet, and 1 private) and 2,541 students. The within-school samples ranged from 2 to 309 students. The final sample is fairly representative of the entire tenth-grade population in the four districts regarding race/ethnicity, with white and African American students being underrepresented, Asians and multiracial students overrepresented, and 12.3% of the students not reporting their race/ethnicity (see Table 1).
This study is based solely on secondary data collected online by the ISCY staff and the staff of participating districts. Both written and informed consent was obtained from all participants and from the parents of non-adult participants, and data were groomed to maintain confidentiality. Ethics approval was not required for research using secondary data with these characteristics per the University of California—Riverside guidelines and national regulations.
Measures
The outcome variable measures student Academic Performance in terms of academic achievement and course grades. It was constructed using factor analysis from three measures: PISA reading and math achievement test scores and student's self-reported expectations for course grades. While self-reported grade expectations are subjective, meta-analyses shown they are the most potent predictor of future grades, even more so than actual grades (Hattie, 2008). However, other meta-analyses have found that the validity of self-reported grades depends in part on cognitive ability (Kuncel et al., 2005). Also, estimates of the effects of non-academic skills on Academic Performance based on grades alone can be biased because teachers often assign grades in part based on non-academic skills (e.g., classroom behavior, attitudes, and effort) rather than strictly on Academic Performance (Fredricks et al., 2004). Hence, including both types of measures in this latent variable alleviates some concerns about self-reported grades while creating a more holistic measure of Academic Performance. The factor loadings, shown in Table 2, indicate that achievement test scores are more strongly associated with Academic Performance than self-reported grade expectations (i.e., 0.83 vs. 0.62).
ISCY staff collected a large number of survey items related to non-academic skills, many of which were borrowed from PISA or other large-scale survey programs (Lamb et al., 2015). Principle component analysis (PCA) was used by ISCY staff to consolidate items into ten scales, each having three to five associated survey items. These scales are summarized in Table 2. The 10 non-academic scales measuring aspects of the student's disposition (Belonging, Self-efficacy, Hope, and Purpose), engagement (cognitive, behavioral, and emotional), intra-personal skills (Conscientiousness), interpersonal skills (Collaboration and Communication) and are similar to many of the scales discussed in the literature review section of this paper. SES was constructed by ISCY staff from student survey responses using the method outlined by Ganzeboom et al. (1992). It is based on parental reports of their educational attainment, income, and occupation and is highly similar to SES measures used in PISA and NCES databases. Cronbach's alpha for all but one scale was within the recommended range (0.7–0.9) (Streiner, 2003). The exception, Conscientiousness, was very close, with an alpha of 0.69. It would be substantially higher if the Homework Time variable were removed. However, it was retained because time spent on homework is considered an important aspect of consciousness related to schooling.
All 12 latent variables used in this study (10 non-academic measures, SES, and Academic Performance) were standardized to have a mean of zero and a standard deviation of one. Therefore, the effects of the non-academic measures on Academic Performance are in units of standard deviations. That is, the effects can be interpreted as the expected standard deviation change in Academic Performance per one standard deviation change in the non-academic measure, holding constant the other covariates in the model. This type of coefficient is referred to as a standardized effect size (ES) or beta coefficient. Some have suggested that the commonly used criteria for classifying strength of correlation coefficients is also applicable for classifying standardized effect sizes (i.e., small/weak < 0.2; 0.2 < medium/moderate < 0.5; 0.5 < large/strong) (see for example, Acock, 2014, p. 272). However, ESs vary considerably across academic disciplines and tend to be small for educational outcomes. Hence, it helps to interpret ESs relative to the effects of commonly used measures or well-documented interventions (e.g., in comparison to the effect of SES or class size reduction).
SES, ethnicity, and gender are used as control variables for student inputs. These demographic measures can vary considerably across schools, and research shows they are associated with student achievement and grades (Palardy, 2008; Borman and Dowling, 2010). Statistically controlling for student inputs serves the dual purposes of partialling out the effects of student background from the effects of the non-academic skills and also statistically equalizing schools on student inputs when modeling peer composition effects. Table 3 provides a lists of the variables used in this study, their labels, and descriptive statistics.
Statistical Modeling
The statistical models are specified to address the research questions and the nested structure of the data. Multilevel models (MLMs) are used because they are ideally suited for analyzing educational data that are hierarchical in structure, that is, students are nested in schools (Raudenbush and Bryk, 2002; Snijders and Bosker, 2012). When analyzed using traditional methods, nested data violate the assumption of statistical independence, which can lead to aggregation biases and tend to produce biased standard errors. The models used in this study have two levels. Level 1 is the within-school or student level for modeling the student non-academic effects on Academic Performance. Level 2 is the between-school level for modeling peer composition effects on Academic Performance. The following section outlines the model specifications used to address each research question.
Multilevel Equations
Research question 1 (RQ 1) is addressed using random coefficient models. The model includes only one student non-academic measure at a time to test whether the effect of each measure on Academic Performance varies among the sample of schools. The model can be represented by the following set of equations.
Level 1: Student Effects
Level 2: School Effects
Yij represents the Academic Performance outcome that was described previously. The subscripts denote the nested structure of the data, where i students are nested in j schools. β0j represents the random intercepts for each of the j schools, which is the school mean for Academic Performance adjusted for Xij, the non-academic measure in the model (note that all continuous level 1 variables were grand mean centered). β1j represents the linear relationship between the non-academic measure and Academic Performance. rij represents the student residuals, which are expected to be normally distributed with a mean of zero and a variance of σij.
At level 2, β0j and β1j are now the outcomes. γ00 represents the grand mean of the Academic Performance outcome adjusted for Xij, and u0j represents the residuals for the school means, which are expected to have a mean of zero, be normally distributed, and have a variance of τ00. γ10 is the mean of the j school slopes, and u1j is the residuals of those slopes, which is also expected to have a mean of zero and be normally distributed with a variance of τ11. The objective of this model is to test whether τ11 is significantly greater than zero. That tests whether the effects of student non-academic measures on Academic Performance vary across schools, which is RQ 1. This addresses whether some aspect of the schools likely moderates this association. For example, it could be that the association between student Behavioral Engagement and Academic Performance depends in part of the disciplinary policy of the school.
Addressing RQ 2 is the main objective of this study and addressing it requires running a series of models. The equation for the base model is provided here, while the extensions to the base model needed for the subsequent models are described. The methodological literature suggests that models that use unreliable measures of student non-academic risk factors and insufficient controls for student inputs tend to overestimate the compositional measures (Harker and Tymms, 2004). As mentioned earlier, non-academic measures have Cronbach's alpha levels ranging from 0.69 to 0.82, which correspond to acceptable-to-good internal consistency or reliability. However, this model does not include any control variables other than the student measure of the non-academic skill being tested. Therefore, the base model estimates of the non-academic effects are likely inflated and should be considered the upper boundaries of the effect sizes. As was the case for RQ 1, each model was repeatedly run—once for each non-academic compositional measure being tested.
Level 1: Student Model
Level 2: School Model
At level 1 the equation is the same as for RQ 1, described previously. At level 2 the intercept model now includes the compositional variable, X.j, which is the school mean for the student non-academic measure being tested. γ01 represents the linear relationship between the compositional measure and mean Academic Performance adjusted for the student non-academic measure in the level 1 model. The slopes are now fixed to be equal and therefore have zero residual variance. This is apparent in the equations because the residual term (u1j) is omitted. The reason for this specification is that the results for RQ 1 showed that none of the student non-academic measures varied significantly among the sample of schools. To address RQ 2, the magnitudes of β1j and γ01 are compared. The magnitudes of their effects are directly comparable because of the standardization method used, albeit, the effects or also sensitive to model specification.
Three subsequent models were fit, each with additional student covariate controls, which tend to reduce the effect size of both the non-academic student and peer composition effects. The first model in this sequence controls for student demographics (race/ethnicity, SES, and gender) and whether the student took or is taking remedial math or English coursework to improve basic skills. The remedial coursework indicators are included as proxies for prior achievement because the data source does not include actual measures. The controls included in this model are generally considered adequate for estimating the compositional effects. The second model in the sequence adds all the non-academic measures at the student-level. This can be considered extensive student controls and may result in conservative estimates of the individual non-academic effects at level 1 and the compositional non-academic effects at level 2. The third model in the sequence adds school-level SES composition. That is, the model includes all the demographic controls, all the student non-academic measures, and school SES composition. This third and last model is used to address RQ 2.
Missing Values
The percentage of missingness on the variables used in this study ranged from 0 to 14%. Missing values were imputed using the expectation maximization (EM) method (Little and Rubin, 1987). This method assumes a distribution for missing observations and imputes values based on the likelihood under that distribution, given all intercorrelations between the variables in the data set.
Results
Before addressing the research questions, it is instructive to examine the descriptive statistics. Table 3 shows that the sample of students is ethnically diverse, with 87% of the students being non-white, including 32% Latinx, 22% Asian, 10% African American, and 13% Native American or Pacific Islander. This level of ethnic diversity is typical of urban school populations in California, where white students have long been a minority group, Latinxs have the highest representation, and Asian Americans often make up a substantial proportion of the student population.
Another preliminary result is for the unconditional model, which is useful for ascertaining whether the school means for Academic Performance vary significantly among the sample of schools and for generating the intra-class correlation coefficient (ICC) for the outcome. The results of the unconditional model indicate the school means vary significantly (τ00 = 0.18, p < 0.00). The ICC is 0.18, which means that 18.0% of the variance in Academic Performance is between school and 82% is among students within the same schools. This figure is consistent with achievement outcomes described in previous research (Raudenbush and Bryk, 2002; Snijders and Bosker, 2012).
Research Question 1
RQ 1 is addressed using random coefficient models. The results are not presented in any table because none of the non-academic skill effects varied across the sample of schools. This indicates those effects are quite similar among the schools used in this study and suggests that they are not moderated by school factors. It is worth noting that schools used in this study are similar in terms of the student bodies they serve. Furthermore, all schools are under the jurisdiction of the State of California, which regulates the curriculum and various school practices. These sample characteristics probably reduce variation in the non-academic effects among the schools. However, the sample also has a major strength for modeling school effects in that there is an average of over 100 students per school. That is substantially higher than most large-scale school databases collected by the National Center for Educational Statistics (NCES). For example, the National Educational Longitudinal Study (NELS: 1988), Educational Longitudinal Study (ELS: 2001), High School Longitudinal Study (HSLS: 2009), and Early Childhood Longitudinal Study (ECLS: 1998; ECLS: 2010), which have been widely used to study school effects, average 15–25 students per school. Research suggests that within-school sample sizes should be at least 30 to reliably estimate random slopes and variance components, which are two objectives of the present study (Kreft and De Leeuw, 1998; Maas and Hox, 2005).
Research Question 2
RQ 2 is addressed through a series of models summarized in Tables 4–6. Table 4 compares the student and peer composition effects with no control covariates in the model. Therefore, these estimates are likely inflated and should be considered an upper boundary in terms of effect sizes and statistical significance. All ten of the student measures are significantly associated with Academic Performance. The effect sizes (ESs) are relatively small, ranging from 0.05 to 0.21. Individually, the student measures account for small proportions of the variation in Academic Performance within schools (0–5%). Hence, it can be said that the student's non-academic skills are associated with their Academic Performance, but even at the upper boundaries, the ESs are small.
In general, the peer composition measures have much larger effects, but lower levels of statistical significance. This paradox is due to the fairly small sample of schools used in this study (N = 25), and hence, far lower statistical power compared with the student effects. Five of the ten peer composition measures are statistically significant. Of those, the ESs range from medium for Belonging (ES = 0.60) to large for Collaboration (ES = 1.36). The significant peer composition variables accounted for 22–61% of the between-school variance. While these estimates are likely inflated, they suggest the peer composition effects tend to be much larger than the student effects.
Table 5 shows the results for the models controlling for student demographics, including race/ethnicity, SES, and gender, and also remedial coursework in math and English. These controls account for 9% of the variance in Academic Performance among students attending the same school (i.e., the percentage of the within-school variance explained) and 37.3% of the variance between the school means. The first figure is similar to the percentage reported in a recent study using the Educational Longitudinal Study (ELS) of 2002 (11.6%), a nationally representative database of American high school students, but the second figure is substantially lower than for ELS (70.7%) (Palardy et al., 2015). That difference is likely due to the greater heterogeneity in student demographics among the schools in ELS.
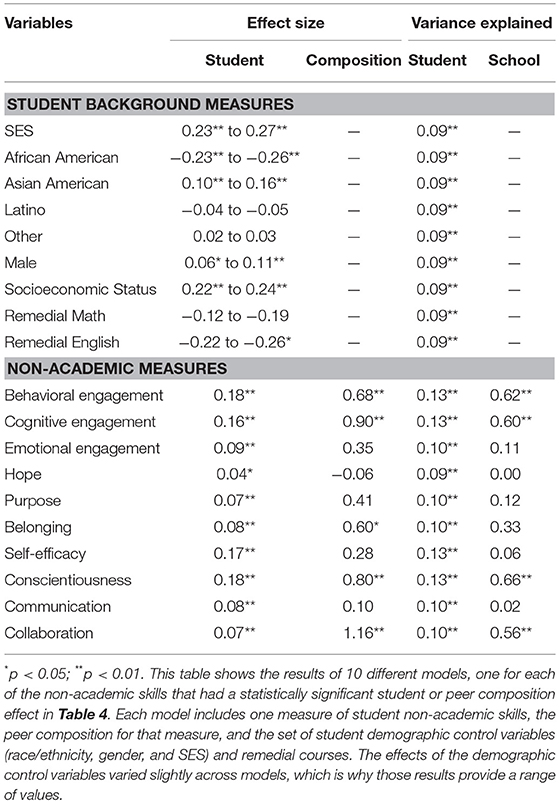
Table 5. Student and peer composition effects on academic performance, controlling for student demographics.
While all the same variables remain statistically significant after controlling for student demographics and remedial coursework, the ES of most student non-academic measures are reduced by about 10% (i.e., Table 5 results compared with Table 4). Controlling for student demographics reduces the effect sizes for the compositional measures by a greater percentage (~15–20%, on average). However, the ES for the significant compositional measures remains several times larger than for the corresponding student measures (0.04 to 0.18 vs. 0.60 to 1.16).
Table 6 shows the results after controlling for the student demographic variables and all ten student non-academic measures. The specification of this model represents an extension in the research literature on the effects of non-academic skills, as there was a call for work examining multiple non-academic skills at once to parse out their intercorrelations (Gutman and Schoon, 2013). This model does exactly that. The model also represents extensive controls for differences in student inputs, which account for 19% of the variance within schools and 54% between schools (the 54% figure is not shown in Table 6). The addition of the set of non-academic student controls further reduced the effect sizes for most student and peer composition measures on Academic Performance. Now only five of the ten student non-academic measures are significant at the student level, and the ESs were reduced by at least 15% for each measure and over 50% for most. Indeed, in three cases, the sign on the effect changed (e.g., from positive to negative) compared with the previously significant estimate shown in Table 5. These substantial changes in the effects due to controlling for all non-academic measures is not surprising, because their correlations range from 0.2 to 0.7. However, these intercorrelations raise concerns of collinearity when all measures are in the model together. To examine whether that was potentially biasing results, the variance inflation factor (VIF) was computed for each student covariate and all were <2.0, suggesting little concern for collinearity.
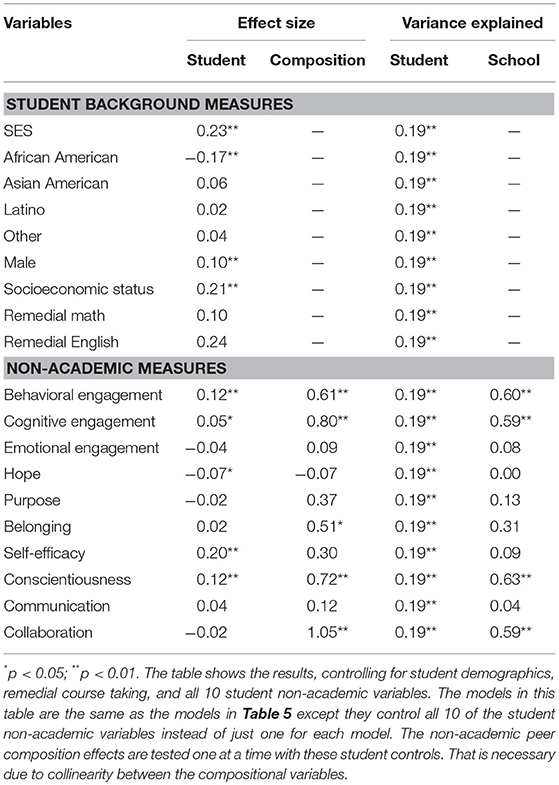
Table 6. Student and peer composition effects on academic performance, controlling for student demographics and all other non-academic variables.
For this model, only three non-academic student measures have ESs >0.10; Behavioral Engagement (ES = 0.12; p < 0.01), Self-efficacy (ES = 0.20; p < 0.01), and Conscientiousness (ES = 0.12; p < 0.01). Note that, while small, these ESs are not dismissible in education, as most student characteristics have small effects on outcomes. For example, the ES for SES, which is commonly considered the most robust demographic predictor of educational outcomes, is 0.20.
Among the peer composition measures, the same five variables were statistically significant as shown in Tables 5, 6, but all had reductions in their ESs within the 10–20% range. Although 54% of the between-school variance in Academic Performance was accounted for by the student variables, none of the compositional effects changed substantially when the set of student non-academic measures was added to the model, and all ESs remained medium to large (0.51 to 1.05). The compositional measures now account for 31 to 63% of the between-school variance after controlling for the student variables.
In comparing the effect sizes of the student non-academic risk factors and peer composition effects, one issue is that there are no school-level control variables. One may argue that there should be controls for the intercorrelations between the compositional effects. To that end, the model with all five significant peer composition measures was run, but there was severe collinearity among the effects, with all variables having VIF values between 6 and 12. The intercorrelations between the five significant peer composition measures range from 0.4 to 0.9. With only 25 schools and most of the between-school variance accounted for by extensive student controls, including all the significant compositional effects is impractical for this database.
Addressing RQ 2, compared with the magnitude of their student effects, all the non-academic measures have a compositional effect several times larger. Even if the student ESs from Table 4, which tested only one student measure at a time, are compared with the peer composition ESs in Table 6, which controls for a large number of student measures, the peer composition ESs are still several times larger.
Discussion
Of the 10 non-academic measures considered in the study, five were significantly associated with Academic Performance: Behavioral Engagement, Cognitive Engagement, Conscientiousness, Self-efficacy, and Hope (see Table 6). Those estimates control demographic background, low prior achievement, and the other non-academic skills. However, the sign on the effect for Hope switched to negative after controlling for the other non-academic risk factors, suggesting its effect is unstable and unreliable. Students with attendance and disciplinary problems (low Behavioral Engagement), students who do not try hard or who quit when facing challenges or frustration, or who turn in work late (low Cognitive Engagement and Conscientiousness), and students who lack confidence in their ability to resolve problems and do well in school (low Self-efficacy), are all at risk for poor Academic Performance. These findings are supported by previous research (see Farrington et al., 2012 and Gutman and Schoon, 2013, for recent summaries of the research literature).
A challenge in comparing the magnitude of the effects found in this study with previous research is that measures of non-academic skills and Academic Performance outcomes vary across studies, and studies report widely varying effect sizes for the same non-academic skill. For example, in their summary of the effect of academic perseverance (a construct similar to grit) on grades, Farrington et al. (2012) reported that correlations ranged from very weak (0.06) to moderate (0.38) across studies cited. Moreover, the association between non-academic skills and grades tends to be stronger than between non-academic skills and achievement test scores (Farrington et al., 2012). These issues confound ES comparisons with the present study. However, one recent study of non-academic effects had similarities to the present study in terms of sample, measures, and outcome (West et al., 2016). That study examined the effects of a range of self-reported ratings of non-academic skills on achievement for a large sample (N = 1,368) of urban eighth graders attending 32 public schools in Boston. While slightly different measures of non-academic skills were used compared with the current study, the results are remarkably similar, with effect sizes all in the range of 0.07-0.17 compared to 0.05–0.213. The similarity in the effect sizes from these two studies lends credibility to the student estimates of non-academic effects in the present study.
Of the ten compositional measures considered, five also had significant associations with Academic Performance: Behavioral and Cognitive Engagement, Belonging, Conscientiousness, and Collaboration (see Table 6). In each case, the effect size of the compositional measure is several times larger than the individual effect. West et al. (2016) also estimated aggregate school effects of their non-academic factors. Their results again show similarities with the findings of the present study. For example, the school aggregate of attendance rate, which is a component of the Behavioral Engagement measure used in the present study, and school aggregate of growth mindset both have effects several times larger than the effect of the corresponding student measure4. These results suggest it is plausible that non-academic compositional effects are much larger than their corresponding individual effect for at least some measures.
Interpreting the Peer Composition Effects
The results suggest that the non-academic skills of one's school peers have a stronger association with Academic Performance than one's own level of non-academic skills. This means that attending a school where the student body is highly rated in terms of Collaboration, Conscientiousness, Behavioral Engagement, Cognitive Engagement, and Belonging matters more to a student's Academic Performance than his or her own levels on those and other non-academic skills.
Collaboration had the largest effect on Academic Performance of the five significant non-academic compositional measures (ES = 1.05, see Table 6). The items of the measurement model for Collaboration (see Table 2) consist of working well with others, understanding others, being fair, and helping others. Note that these behaviors are not associated with individual students' Academic Performance (ES = −0.02, p > 0.10); however, as a collective student body behavior—a school culture, if you will—it has a large impact on Academic Performance. That suggests that being collaborative is only beneficial when peers and coworkers are also collaborative. The underlying mechanism for this effect may be relational trust, which tends to have positive effects on academic and social outcomes in schools (Bryk and Schneider, 2002). Within an organization, the essence of relational trust is the level of confidence individuals have that others will meet role expectations (Couch and Jones, 1997). Without sufficient confidence that others will fulfill role expectations, students may perceive collaboration at school as being burdened with additional group work without reciprocal benefits. An antecedent for relational trust is a common understanding of role expectations (Couch and Jones, 1997). This suggests that student and teacher role expectations related to collaborative work must be clear and widely accepted for a productive collaborative school environment to take hold.
Belonging pertains to feeling safe, happy, and liked at school. High values on the peer composition measure for Belonging reflect schools where higher percentages of the students want to be there for social reasons. Rotermund (2010) found that Belonging is an antecedent for other non-academic effects at the individual level, such as engagement. It may play a similar role as a peer composition effect, as it is highly correlated with the peer composition for Cognitive Engagement (r = 0.61). That is, schools with a low mean on Belonging may have difficulty facilitating Collaboration and Cognitive Engagement because students do not feel accepted. A post-hoc analysis also showed that the peer composition measure of Belonging is a statistically significant antecedent of Collaboration (r = 0.83) and Conscientiousness (r = 0.62). More research is needed to better understand the mediating and moderating relationships among these peer composition measures and Academic Performance.
Conscientiousness is a Big Five personality trait that implies a persistent desire to do tasks well and take obligations seriously. In the school context, it concerns the degree to which students make an effort to learn in class, persist when the material becomes difficult, and turn assignments in on time. Of the Big Five traits, research has found it to have the largest effect on academic performance in school (Trapmann et al., 2007; Poropat, 2009; Kautz et al., 2014). Yet, the peer composition effect appears to be substantially larger than the robust individual effect. The large compositional effect is likely due to both peer and school effects. There is likely a direct effect in that students tend to adopt the attitudes and behaviors of their peers. However, the student-level results for Conscientiousness show that raising one's level of Conscientiousness has only a moderate effect on Academic Performance, so there must be more to the peer composition effect than just raising individual Conscientiousness. There is also likely an indirect effect for Conscientiousness peer composition in that schools that are high on the compositional measure can provide more advanced curricula and use more rigorous instructional practices because conscientious students are equipped to respond well to and benefit from those academic challenges. Conversely, at schools low on Conscientiousness composition, imposing advanced curricula and rigorous instructional practices may be counterproductive, leading to student resistance and teacher frustration that tends to undermine academic performance (Thrupp, 1999; Kautz et al., 2014).
Behavioral Engagement pertains to the degree to which students avoid problem behaviors at school, such as being late for school, skipping school without permission, and getting into trouble at school. As is the case with the other measures of peer composition, the total effect likely has direct and indirect components. There is likely a direct peer influence, as students tend to adopt the behaviors and attitudes of their peers. Another direct peer influence is Behavioral Engagement peer composition because disengageed and disruptive peers tend to interfer with learning among their classmates. There is also likely an indirect school effect in that schools with low Behavioral Engagement composition tend to emphasize reducing the disorder, which will require disciplinary attention from school personnel, drawing time and energy away from academic activities. That is, schools that are rated low on Behavioral Engagement composition may respond with policies and practices that emphasize order and discipline over the development of academic and non-academic skills (Bowles and Gintis, 1976; Thrupp, 1999).
Implications for Educational Research, Policy, and Practice
Previous research has identified a substantial number of non-academic risk factors that are associated with student academic performance and other outcomes. The substantial number of risk factors that have been identified makes it impractical for schools to address but a small fraction of them. Moreover, many non-academic skills have conceptual and measurement overlap. For research on non-academic skills to be useful for informing policy and practice, a small number of malleable skills with the largest effects must be identified, along with effective interventions for improving them. Unfortunately, research on the effects of non-academic skills tends to model one skill at a time, which likely results in inflated estimates of the effects and provides little guidance for policy and practice regarding which skills matter most when all are considered at once (Gutman and Schoon, 2013). This study represents a departure from that norm, as student non-academic skills were modeled both individually and together, controlling for their intercorrelations. The results suggest that three individual measures have the strongest associations with Academic Performance: Behavioral Engagement (ES = 0.12), Self-efficacy (ES = 0.20), and Conscientiousness (ES = 0.12). Past research shows these three factors are malleable and may be improved through school interventions (Farrington et al., 2012; Gutman and Schoon, 2013). Therefore, the results of this study suggest that effective interventions targeting these factors will have the greatest impact on Academic Performance.
The magnitude of the peer composition effects relative to the individual effects suggests that raising the school averages should be the primary objective of any policy or intervention. That objective can be accomplished be focusing the greatest attention on efforts to improve students with the most underdeveloped non-academic skills. However, focusing only on underdeveloped students is unlikely to be optimal, as it neglects to improve the non-academic skills of the majority of the students. A more effective method for raising the school averages is a school-wide and multi-tiered approach that provides supports for improvement to all students, while matching the intensity with student needs. This approach is consistent with the model of school-wide positive behavioral support (Durlak et al., 2010, 2011; see Bradshaw et al., 2010). Additionally, the results of this study suggest that programs and interventions that focus on creating a school culture that values Collaboration, Conscientiousness, Behavioral Engagement, Cognitive Engagement, and Belonging are likely to be most effective for raising Academic Performance.
Limitations of the Study
The data sample used in this study has strengths and weaknesses for addressing the objectives of the study. One strength is the wide range of non-academic factors that were reliably measured. Another strength is the size of the within-school sample, averaging over 100 students per school. That is several times the average in large-scale high school databases collected by the U.S. government (e.g., ELS: 2002). The larger within-school sample size provides the higher statistical power needed to test whether effects vary across schools, which is the objective for research question 1 of this study. However, the small number of schools (N = 25) is a limitation. While the sample represents excellent coverage of high schools in Sacramento, it has limitations with regard to the statistical power of the peer composition effects, which may result in false negatives on statistical tests. This could be why two peer composition measures (Purpose and Self-efficacy) have non-significant effects even though their effect sizes are medium (0.37 and 0.30). It is important to note that despite this limitation, 5 of the 10 peer composition effects are statistically significant due to their substantial effect sizes.
The moderate school sample size prevented the modeling of all peer compositional effects simultaneously, which may have resulted in inflated effects due to the intercorrelations between the peer composition measures. One specific case, the overlap in peer composition measures of Conscientiousness and Cognitive Engagement, merits elaboration because their distinctness is questionable. Conscientiousness generally applies to a person's behaviors and attitudes, while Cognitive Engagement pertains specifically to academic behaviors at school. However, when Conscientiousness is measured in the school context, the distinction between the two constructs becomes subtle, as their measurement items are similar (see Table 2). Additionally, the strength of their correlation is high at the compositional level, higher than at the individual level (0.85 vs. 0.66), further supporting the notion of less distinction at the compositional level in school settings. This calls into question whether both compositional measures are necessary, which is particularly important when moving from research to policy and practice because the fewer compositional factors a school needs to be concerned with, the easier it is to focus on improving them.
Table 1 shows that the sample characteristics are quite similar to the student populations of the four Sacramento districts from which they were drawn. Furthermore, the sample is similar to many urban districts in Western and Southwest United States, especially urban districts in California. Therefore, the results are most applicable to students and schools in those settings. However, it is unclear whether the results generalize to the broader population of U.S. high schools, schools serving lower grade levels, or schools in other countries.
Summary and Conclusions
A generation ago, Bowles and Gintis (1976) concluded that non-academic risk factors tend to have a greater effect on employment, well-being, and other life outcomes than do academic skills. They also argued that schools play a greater role in impacting life outcomes through socialization processes that impact non-academic development than through academic development. Over the past generation, a body of research has accumulated largely supporting Bowles and Gintis's contentions (Farrington et al., 2012; Gutman and Schoon, 2013; Kautz et al., 2014). The present study contributes to this body of work by exploring a range of non-academic compositional risk factors and indexing their effect sizes relative to student non-academic factors.
The results show a distinct pattern of much larger effects for peer composition than for individual non-academic attributes. Five compositional risk factors (Collaboration, Cognitive Engagement, Conscientiousness, Behavioral Engagement, and Belonging) were found to have medium-to-large effects on Academic Performance, whereas all student risk factors were small (ES < 0.2) or not statistically significant.
These findings are consistent with developmental theory positing that context plays a central role in child development (Bronfenbrenner, 1979; Reschly and Coolong-Chaffin, 2016). Namely, interventions that focus solely on students with the lowest non-academic skills are unlikely to be optimally effective for raising student academic performance. That is because such interventions are not optimal for improving the school context as captured by school means on peer non-academic attributes. Multi-tiered interventions that focus on raising school-wide peer composition on non-academic attributes are likely to be most effective for improving academic performance. The findings also help establish which individual and peer composition attributes matter most to academic performance and therefore should be targeted for interventions. That is important because of the substantial number of non-academic risk factors that have been identified in the research literature.
Author Contributions
GP is the sole author and wrote the entire manuscript and conducted all data analyses.
Conflict of Interest Statement
The author declares that the research was conducted in the absence of any commercial or financial relationships that could be construed as a potential conflict of interest.
Footnotes
1. ^Socioeconomic or racial peer composition are often framed in terms of school segregation.
2. ^The author acknowledges Professor Russell Rumberger for his generosity in providing the data used in this study. The data are not currently available for public use but may be in the future.
3. ^The results from West et al. (2016) that are being compared are summarized in their Table 6, column 3, on page 157. Their estimates are based on separate regressions for each non-academic skill on student achievement with school fixed effects and no student control covariates. Given their model specification, their student non-academic ESs are most comparable to column 1 of Table 4 in the present study.
4. ^West et al. (2016) did not control for the individual effect of the non-academic measures or other aspects of student background when estimating peer composition effects. Hence, compositional effects may be overestimated. However, the authors of that study did not claim to be estimating compositional effects. This model specification issue is noted as a caveat for comparisons between the studies.
References
Alexander, K. L., Entwisle, D. R., and Horsey, C. S. (1997). From first grade forward: early foundations of high school dropout. Sociol. Educ. 70, 87–107. doi: 10.2307/2673158
Berkowitz, R., Moore, H., Astor, R. A., and Benbenishty, R. (2017). A research synthesis of the associations between socioeconomic background, inequality, school climate, and academic achievement. Rev. Educ. Res. 87, 425–469. doi: 10.3102/0034654316669821
Boekaerts, M., Pintrich, P. R., and Zeidner, M. (eds.). (2000). Handbook of Self-Regulation. San Diego, CA: Academic Press.
Borman, G. D., and Dowling, M. (2010). Schools and inequality: a multilevel analysis of coleman's equality of opportunity data. Teach. Coll. Rec. 112, 1201–1246.
Bowles, S., Gintis, H., and Osborne, M. (2001). The determinants of earnings: a behavioral approach. J. Econ. Lit. 39, 1137–1176. doi: 10.1257/jel.39.4.1137
Bradshaw, C. P., Mitchell, M. M., and Leaf, P. J. (2010). Examining the effects of schoolwide positive behavioral interventions and supports on student outcomes: results from a randomized controlled effectiveness trial in elementary schools. J. Posit. Behav. Interv. 12, 133–148. doi: 10.1177/1098300709334798
Bronfenbrenner, U. (1979). The Ecology of Human Development. Cambridge, MA: Harvard University Press.
Bryk, A. S., and Schneider, B. (2002). Trust in Schools: A core Resource for Improvement. Russell Sage Foundation.
Coleman, J. S., Campbell, C., Hobson, J., McPartland, F., Mood, F., and Weinfeld, York, R. (1966). Equality of Educational Opportunity. Washington, DC: U.S. Government Printing Office.
Cook, T. D., Deng, Y., and Morgano, E. (2007). Friendship influences during early adolescence: the special role of friends' grade point average. J. Res. Adolesc. 17, 325–356. doi: 10.1111/j.1532-7795.2007.00525.x
Couch, L. L., and Jones, W. H. (1997). Measuring levels of trust. J. Res. Person. 31, 319–336. doi: 10.1006/jrpe.1997.2186
Cunha, F., and Heckman, J. (2007). The technology of skill formation. Am. Econ. Rev. 97, 31–47. doi: 10.1257/aer.97.2.31
Damon, W. (2008). The Path to Purpose: How Young People Find Their Calling in Life. New York, NY: Free Press.
Damon, W., Menon, J., and Bronk, K. C. (2003). The development of purpose during adolescence. Appl. Dev. Sci. 7, 119–128. doi: 10.1207/S1532480XADS0703_2
Dreeben, R., and Barr, R. (1988). Classroom composition and the design of instruction. Sociol. Educ. 61, 129–142. doi: 10.2307/2112622
Duckworth, A. L., Peterson, C., Matthews, M. D., and Kelly, D. R. (2007). Grit: perseverance and passion for long-term goals. J. Pers. Soc. Psychol. 92, 1087–1101. doi: 10.1037/0022-3514.92.6.1087
Durlak, J. A., Weissberg, R. P., Dymnicki, A. B., Taylor, R. D., and Schellinger, K. B. (2011). The impact of enhancing students' social and emotional learning: a meta-analysis of school-based universal interventions. Child Dev. 82, 405–432. doi: 10.1111/j.1467-8624.2010.01564.x
Durlak, J. A., Weissberg, R. P., and Pachan, M. (2010). A meta-analysis of after-school programs that seek to promote personal and social skills in children and adolescents. Am. J. Commun. Psychol. 45, 294–309. doi: 10.1007/s10464-010-9300-6
Dweck, C. S. (1999). Self-Theories: Their Role in Motivation, Personality and Development. Philadelphia, PA: Psychology Press.
Erbring, L., and Young, A. A. (1979). Individuals and social structure: contextual effects as endogenous feedback. Sociol. Methods Res. 7, 396–430. doi: 10.1177/004912417900700404
Farkas, G. (2003). Cognitive skills and non-academic traits and behaviors in stratification processes. Annu. Rev. Sociol. 29, 541–562. doi: 10.1146/annurev.soc.29.010202.100023
Farkas, G., Grobe, R., Sheehan, D., and Shuan, Y. (1990). Cultural resources and school success: gender, ethnicity, and poverty groups within an urban school district. Am. Sociol. Rev. 55, 127–142. doi: 10.2307/2095708
Farrington, C. E., Roderick, M., Allensworth, E., Ngaoka, J., Keyes, T. S., Johnson, D. W., et al. (2012). Teaching Adolescents to Become Learners: The Role of non-Academic Factors in Shaping School Performance. Chicago, IL: Consortium on Chicago School Research, University of Chicago. Retrieved from: https://ccsr.uchicago.edu/publications/teaching-adolescents-become-learners-role-non-academic-factors-shaping-school (accessed December 15, 2017).
Finn, J. D. (1993). School Engagement and Students at Risk. Washington, DC: National Center for Education Statistics.
Finn, J. D., Pannozzo, G. M., and Voelkl, K. E. (1995). Disruptive and inattentive withdrawn behavior and achievement among fourth graders. Elementary Sch. J. 95, 421–454. doi: 10.1086/461853
Finn, J. D., and Rock, D. A. (1997). Academic success among students at risk for school failure. J. Appl. Psychol. 82, 221–234. doi: 10.1037/0021-9010.82.2.221
Firebaugh, G. (1979). Assessing group effects: a comparison of two methods. Sociol. Methods Res. 7, 384–395. doi: 10.1177/004912417900700402
Fredricks, J. A., Blumenfeld, P. C., and Paris, A. H. (2004). School engagement: potential of the concept, state of the evidence. Rev. Educ. Res. 74, 59–109. doi: 10.3102/00346543074001059
Ganzeboom, H. B. G., De Graaf, P. M., and Treiman, D. J. (1992). A standard international socio-economic index of occupational status. Soc. Sci. Res. 21, 1–56. doi: 10.1016/0049-089X(92)90017-B
Gilman, R., Dooley, J., and Florell, D. (2006). Relative levels of hope and their relationship with academic and psychological indicators among adolescents. J. Soc. Clin. Psychol. 25, 166–178. doi: 10.1521/jscp.2006.25.2.166
Goodenow, C. (1993). The psychological sense of school membership among adolescents: scale development and educational correlates. Psychol. Sch. 30, 79–90.
Goodenow, C., and Grady, K. E. (1993). The relationship of school Belonging and friends' values to academic motivation among urban adolescent students. J. Exp. Educ. 62, 60–71. doi: 10.1080/00220973.1993.9943831
Greenwald, R., Hedges, L. V., and Laine, R. D. (1996). The effect of school resources on student achievement. Rev. Educ. Res. 66, 361–396. doi: 10.2307/1170528
Gresham, F. M., and Elliott, S. N. (1990). Social Skills Rating System: Preschool, Elementary Level. Minneapolis, MN: American Guidance Service.
Gutman, L. M., and Schoon, I. (2013). The Impact of Non-academic Skills on Outcomes for Young People. London: Education Endowment Foundation.
Hallinan, M. T., and Williams, R. A. (1990). Students' characteristics and the peer-influence process. Sociol. Educ. 63, 122–132. doi: 10.2307/2112858
Harker, R., and Tymms, P. (2004). The effects of student composition on school outcomes. Sch. Effect. Sch. Improv. 15, 177–200. doi: 10.1076/sesi.15.2.177.30432
Hattie, J. (2008). Visible Learning: A Synthesis of Over 800 Meta-analyses Relating to Achievement. Abingdon: Routledge. doi: 10.4324/9780203887332
Heckman, J. J., and Kautz, T. (2014). “Fostering and measuring skills: interventions that improve character and cognition,” in The Myth of Achievement Tests: The GED and the Role of Character in American Life, eds J. Heckman, J. E. Humphries, and T. Kautz (Chicago, IL: University of Chicago Press), 1–71. doi: 10.3386/w19656
Jang, S. J. (2002). The effects of family, school, peers, and attitudes on adolescents' drug use: do they vary with age? Justice Q. 19, 97–126. doi: 10.1080/07418820200095181
Jencks, C., and Mayer, S. E. (1990). The social consequences of growing up in a poor neighborhood,” in Inner-City Poverty in the United States, eds L. Lynn, and M. G. H. McGeary (Washington, DC: National Academy Press, 111–186.
Jussim, L., and Harber, K. D. (2005). Teacher expectations and self-fulfilling prophecies: knowns and unknowns, resolved and unresolved controversies. Pers. Soc. Psychol. Rev. 9, 131–155. doi: 10.1207/s15327957pspr0902_3
Kautz, T., Heckman, J. J., Diris, R., Weel, B., and Borghans, L. (2014). Fostering and measuring skills: improving cognitive and non-academic skills to promote lifetime success. OECD Education Working Papers. Paris: OECD Publishing. doi: 10.3386/w20749
Konstantopoulos, S., and Borman, G. D. (2011). Family background and school effects on student achievement: a multilevel re-analysis of the Coleman data. Teach. Coll. Rec. 113, 97–132.
Kreft, I. G. G., and De Leeuw, J. (1998). Introducing Multilevel Modeling. Newbury Park, CA: Sage. doi: 10.4135/9781849209366
Kuncel, N. R., Cred,é, M., and Thomas, L. L. (2005). The validity of self-reported grade point averages, class ranks, and test scores: a meta-analysis and review of the literature. Rev. Educ. Res. 75, 63–82. doi: 10.3102/00346543075001063
Lamb, S., Jackson, J., and Rumberger, R. (2015). Measuring 21th Century Skills in ISCY. Melbourne, VIC: ISCY.
Lee, V. E., and Smith, J. B. (1999). Social support and achievement for young adolescents in Chicago: the role of school academic press. Am. Educ. Res. J. 36, 907–945. doi: 10.3102/00028312036004907
Li, Y., and Lerner, R. M. (2013). Interrelations of behavioral, emotional, and cognitive school engagement in high school students. J. Youth Adolesc. 42, 20–32. doi: 10.1007/s10964-012-9857-5
Little, R. J. A., and Rubin, D. B. (1987). Statistical Analysis With Missing Data. New York, NY: Wiley.
Loeb, S., Christian, M. S., Hough, H., Meyer, M. S., Rice, A. B., and West, M. R. (2019). School differences in social-emotional learning gains: findings from the first large-scale panel survey of students. J. Educ. Behav. Statist. 44, 1–36. doi: 10.3102/1076998619845162
Ludtke, O., Marsh, H. W., Robitzsch, A., Trautwein, U., Asparouhov, T., and Muthén, B. (2008). The multilevel latent covariate model: a new, more reliable approach to group-level effects in contextual studies. Psychol. Methods 13:203. doi: 10.1037/a0012869
Maas, C. J., and Hox, J. J. (2005). Sufficient sample sizes for multilevel modeling. Methodology 1, 86–92. doi: 10.1027/1614-2241.1.3.86
Marsh, H. W., Ludtke, O., Robitzsch, A., Trautwein, U., Asparouhov, T., Muthen, B., et al. (2009). Doubly-latent models of school contextual effects: integrating multilevel and structural equation approaches to control measurement and sampling error. Multiv. Behav. Res. 44, 764–802. doi: 10.1080/00273170903333665
Mounts, N. S., and Steinberg, L. (1995). An ecological analysis of peer influence on adolescent grade point average and drug use. Dev. Psychol. 31, 915–922. doi: 10.1037/0012-1649.31.6.915
National Research Council (2012). “Education for Life and Work: Developing Transferable Knowledge and Skills in the 21st century. Committee on Defining Deeper Learning and 21st century skills,” in Board on Testing and Assessment, Division of Behavioral and Social Sciences and Education, eds J. W. Pellegrino and M. L. Hilton (Washington, DC: National Academies Press).
Neidell, M., and Waldfogel, J. (2010). Cognitive and non-academic peer effects in early education. Rev. Econ. Statist. 92, 562–576. doi: 10.1162/REST_a_00012
OECD (2013). PISA 2012 Results: Ready to Learn: Students' Engagement, Drive and Self-Beliefs (Volume III): Preliminary Version. Paris: OECD. doi: 10.1787/9789264201170-en
OECD (2015). Skills for Social Progress: The Power of Social and Emotional Skills. Paris: OECDPublishing. Retrieved from: http://dx.doi.org/10.1787/9789264226159-en. doi: 10.1787/9789264226159-en
Pajares, F. (1996). Self-efficacy beliefs in academic settings. Rev. Educ. Res. 66, 543–578. doi: 10.3102/00346543066004543
Palardy, G. J. (2008). Differential school effects among low, middle, and high social class composition schools: a multilevel, multiple group latent growth curve analysis. Sch. Effect. Sch. Improv. 19, 21–49. doi: 10.1080/09243450801936845
Palardy, G. J. (2013). High school socioeconomic segregation and student attainment. Am. Educ. Res. J. 50, 714–754. doi: 10.3102/0002831213481240
Palardy, G. J. (2015a). The effects of classroom context, teacher qualifications and effectiveness on achievement gaps in first grade. Teach. Coll. Rec. 117, 1–48.
Palardy, G. J. (2015b). High school socioeconomic composition and student college choice: multilevel mediation via school organizational habitus, practices, and peer influences. Sch. Effect. Sch. Improv. 26, 398–423. doi: 10.1080/09243453.2014.965182
Palardy, G. J. (in press). “The effects of high school socioeconomic composition on achievement, engagement, graduation, college-going behaviors in the U.S. ” in International Perspectives in Educational Effectiveness Research, eds J. Hall, P. Sammons, A. Lindorff (London: Springer), 108–134.
Palardy, G. J., and Rumberger, R. W. (2019). “Problem behaviors at school: demographic differences and the role of 21st century skills and peer composition in Sacramento,” in Resisting Education: A Cross-National Study on Systems and School Effects, eds J. Demanet and M. Van Houtte (Cham: Springer), 53–80.
Palardy, G. J., Rumberger, R. W., and Butler, T. (2015). The effect of high school socioeconomic, racial, and linguistic segregation on academic performance and school behaviors. Teacher College Record 117, 1–46.
Patacchini, E., Rainone, E., and Zenou, Y. (2017). Heterogeneous peer effects in education. J. Econ. Behav. Organ. 134, 190–227. doi: 10.1016/j.jebo.2016.10.020
Perry, L. B., and McConney, A. (2010). Does the SES of the school matter? An examination of socioeconomic status and student achievement using PISA 2003. Teachers College Record 112, 1137–1162.
Poropat, A. E. (2009). A meta-analysis of the five-factor model of personality and academic performance. Psychol. Bull. 135:322. doi: 10.1037/a0014996
Raudenbush, S. W., and Bryk, A. (2002). Hierarchical Linear Models: Applications and Data Analysis Methods, 2nd Edn. Thousand Oaks, CA: Sage.
Raudenbush, S. W., and Willms, J. D. (1995). The estimation of school effects. J. Educ. Behav. Statist. 20, 307–335. doi: 10.3102/10769986020004307
Reschly, A. L., and Coolong-Chaffin, M. (2016). “Contextual influences and response to intervention,” in Handbook of Response to Intervention: The Science and Practice of Multi-Tiered Systems of Support, S. Jimerson, K. Burns, and A. M. VanDerHeyden (Boston, MA, Springer), 441–453. doi: 10.1007/978-1-4899-7568-3_26
Reynolds, D., Sammons, P., De Fraine, B., Van Damme, J., Townsend, T., Teddlie, C., et al. (2014). Educational effectiveness research (EER): A state-of-the-art review. Sch. Effect. Sch. Improv. 25, 197–230. doi: 10.1080/09243453.2014.885450
Rotermund, S. L. (2010). The Role of Psychological Antecedents and Student Engagement in a Process Model of High School Dropout. Doctoral dissertation. University of California, Santa Barbara, CA.
Rumberger, R. W. (1987). High school dropouts: a review of issues and evidence. Rev. Educ. Res. 57, 101–121. doi: 10.3102/00346543057002101
Rumberger, R. W., and Palardy, G. J. (2005). Does segregation still matter? The impact of social composition on academic achievement in high school. Teach. Coll. Rec. 107, 1999–2045.
Snijders, T. A. B., and Bosker, R. J. (2012). Multilevel Analysis: An Introduction to Basic and Advanced Multilevel Modeling, 2nd Edn. London: Sage.
Snyder, C. R. (2002). Hope theory: rainbows in the mind. Psychol. Inquiry 13, 249–275. doi: 10.1207/S15327965PLI1304_01
Snyder, C. R., Cheavens, J., and Sympson, S. C. (1997). Hope: An individual motive for social commerce. Group Dyn. 1:107. doi: 10.1037/1089-2699.1.2.107
Streiner, D. L. (2003). Starting at the beginning: an introduction to coefficient alpha and internal consistency. J. Pers. Assess. 80, 99–103. doi: 10.1207/S15327752JPA8001_18
Thrupp, M. (1999). Schools Making a Difference: Let's be Realistic! Buckingham: Open University Press.
Trapmann, S., Hell, B., Hirn, J. O. W., and Schuler, H. (2007). Meta-analysis of the relationship between the Big Five and academic success at university. Zeitschrift für Psychol. 215, 132–151. doi: 10.1027/0044-3409.215.2.132
Van Ewijk, R., and Sleegers, P. (2010). The effect of peer socioeconomic status on student achievement: a meta-analysis. Educ. Res. Rev. 5, 134–150. doi: 10.1016/j.edurev.2010.02.001
Wang, M. T., Willett, J. B., and Eccles, J. S. (2011). The assessment of school engagement: examining dimensionality and measurement invariance by gender and race/ethnicity. J. Sch. Psychol. 49, 465–480. doi: 10.1016/j.jsp.2011.04.001
West, M. R., Buckley, K., Krachman, S. B., and Bookman, N. (2018). Development and implementation of student social-emotional surveys in the CORE districts. J. Appl. Dev. Psychol. 55, 119–129. doi: 10.1016/j.appdev.2017.06.001
West, M. R., Kraft, M. A., Finn, A. S., Martin, R. E., Duckworth, A. L., Gabrieli, C. F., et al. (2016). Promise and paradox: measuring students' non-academic skills and the impact of schooling. Educ. Eval. Policy Anal. 38, 148–170. doi: 10.3102/0162373715597298
Willms, J. D. (1986). Social class segregation and its relationship to pupils' examination results in Scotland. Am. Sociol. Rev. 51, 224–241. doi: 10.2307/2095518
Keywords: school composition effects, peer influences, non-academic skills, non-cognitive skills, social-emotional skills, school engagement, student dispositions, academic performance
Citation: Palardy GJ (2019) School Peer Non-academic Skills and Academic Performance in High School. Front. Educ. 4:57. doi: 10.3389/feduc.2019.00057
Received: 06 August 2018; Accepted: 28 May 2019;
Published: 14 June 2019.
Edited by:
James Elliot Hall, University of Southampton, United KingdomReviewed by:
Geoff Anthony Lindsay, University of Warwick, United KingdomAntonio Valle, University of A Coruña, Spain
Copyright © 2019 Palardy. This is an open-access article distributed under the terms of the Creative Commons Attribution License (CC BY). The use, distribution or reproduction in other forums is permitted, provided the original author(s) and the copyright owner(s) are credited and that the original publication in this journal is cited, in accordance with accepted academic practice. No use, distribution or reproduction is permitted which does not comply with these terms.
*Correspondence: Gregory J. Palardy, gjpalardy@gmail.com