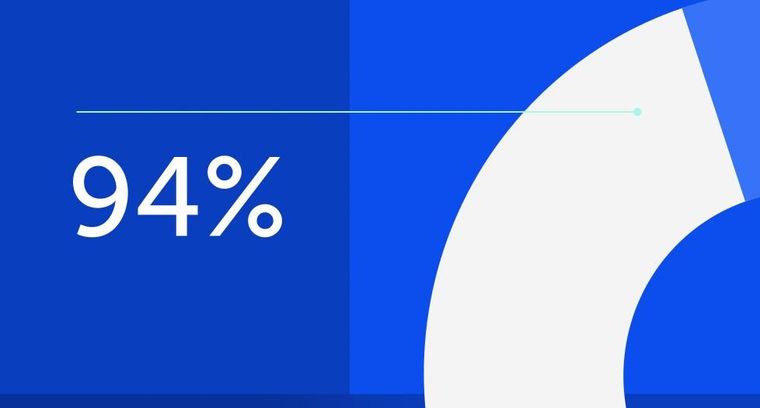
94% of researchers rate our articles as excellent or good
Learn more about the work of our research integrity team to safeguard the quality of each article we publish.
Find out more
METHODS article
Front. Educ., 25 June 2019
Sec. Educational Psychology
Volume 4 - 2019 | https://doi.org/10.3389/feduc.2019.00053
This article is part of the Research TopicEpistemological and Ethical Aspects of Research in the Social SciencesView all 13 articles
Methodological discussions often oversimplify by distinguishing between “the” quantitative and “the” qualitative paradigm and by arguing that quantitative research processes are organized in a linear, deductive way while qualitative research processes are organized in a circular and inductive way. When comparing two selected quantitative traditions (survey research and big data research) with three qualitative research traditions (qualitative content analysis, grounded theory and social-science hermeneutics), a much more complex picture is revealed: The only differentiation that can be upheld is how “objectivity” and “intersubjectivity” are defined. In contrast, all research traditions agree that partiality is endangering intersubjectivity and objectivity. Countermeasures are self-reflexion and transforming partiality into perspectivity by using social theory. Each research tradition suggests further countermeasures such as falsification, triangulation, parallel coding, theoretical sensitivity or interpretation groups. When looking at the overall organization of the research process, the distinction between qualitative and quantitative research cannot be upheld. Neither is there a continuum between quantitative research, content analysis, grounded theory and social-science hermeneutics. Rather, grounded theory starts inductively and with a general research question at the beginning of analysis which is focused during selective coding. The later research process is organized in a circular way, making strong use of theoretical sampling. All other traditions start research deductively and formulate the research question as precisely as possible at the beginning of the analysis and then organize the overall research process in a linear way. In contrast, data analysis is organized in a circular way. One consequence of this paper is that mixing and combining qualitative and quantitative methods becomes both easier (because the distinction is not as grand as it seems at first sight) and more difficult (because some tricky issues of mixing specific to mixing specific types of methods are usually not addressed in mixed methods discourse).
Since the 1920s, two distinct traditions of doing social science research have developed and consolidated (Kelle, 2008, p. 26 ff.; Baur et al., 2017, p. 3; Reichertz, 2019), which are typically depicted as the “qualitative” and the “quantitative” paradigm (Bryman, 1988). Both paradigms have a long tradition of demarcating themselves from each other by ignoring each other at best or criticizing as well as pejoratively devaluating the respective “other” tradition at worst (Baur et al., 2017, 2018, pp. 8–9; Kelle, 2017; Baur and Knoblauch, 2018). Regardless, few authors make the effort of actually defining the difference between the paradigms. Instead, most methodological texts in both research traditions make implicit assumptions about the properties of “qualitative” and “quantitative” research. If one sums up both these (a) implicit assumptions and (b) the few attempts of defining what “qualitative” and “quantitative” research is, the result is a rather crude and oversimplified picture.
“Qualitative research” is typically depicted as combination of the following elements (Ametowobla et al., 2017, pp. 737–776; Baur and Blasius, 2019):
– an “interpretative” epistemological stance (e.g., Knoblauch et al., 2018) which is associated e.g., with phenomenology or social constructivism (Knoblauch and Pfadenhauer, 2018) or some branches of pragmatism (Johnson et al., 2017);
– a research process that is circular or spiral (Strübing, 2014);
– single case studies (Baur and Lamnek, 2017a) or small theoretically and purposely drawn samples meaning that relatively few cases are analyzed (Behnke et al., 2010, pp. 194–210);
– for these cases, a lot of data are collected, e.g., by qualitative interviews (Helfferich, 2019), ethnography (Knoblauch and Vollmer, 2019) or so-called “natural” data, i.e., qualitative process-produced data such as visual data (Rose, 2016) or digital data such as web videos (Traue and Schünzel, 2019), websites (Schünzel and Traue, 2019) or blogs (Schmidt, 2019). In all these cases, this means that a lot of information per case is analyzed;
– both the data and the data collection process are open-ended and less structured than in quantitative research;
– data are typically prepared and organized either by hand or by using qualitative data analysis software (such as NVivo, MAXqda or Atlas/ti);
– data analysis procedures themselves are suitable for the more unstructured nature of the data.
In contrast, “quantitative research” is seen as a combination of (Ametowobla et al., 2017, pp. 752–754; Baur and Blasius, 2019):
– a “positivist” research stance;
– a linear research process (Baur, 2009a);
– large random samples meaning that many cases are analyzed;
– relatively little information per case, collected via (in comparison to qualitative research) few variables;
– data are collected in a highly structured format, e.g., using surveys (Groves et al., 2009; Blasius and Thiessen, 2012; Baur, 2014) or mass data (Baur, 2009a) which recently have also been called “big data” (Foster et al., 2017; König et al., 2018) and which may comprise e.g., webserver logs and log files (Schmitz and Yanenko, 2019), quantified user-generated information on the internet such as Twitter communication (Mayerl and Faas, 2019) as well as public administrational data (Baur, 2009b; Hartmann and Lengerer, 2019; Salheiser, 2019) and other social bookkeeping data (Baur, 2009a);
– the whole data collection process is highly structured and as standardized as possible;
– data are prepared by building a data base and analyzed using statistical packages (like R, STATA or SPSS) or advanced programming techniques (e.g., Python);
– data are analyzed using diverse statistical (Baur and Lamnek, 2017b) or text mining techniques (Riebling, 2017).
Once these supposed differences are spelled out, it immediately becomes obvious how oversimplified they are because in social science research practice, the distinction between the data types is much more fluent. For example, “big data” are usually mixed data, containing both standardized elements (Mayerl and Faas, 2019) such as log files (Schmitz and Yanenko, 2019) and qualitative elements such as texts (Nam, 2019) or videos (Traue and Schünzel, 2019). Accordingly, it is unclear, if text mining is really a “quantitative” method or rather a “qualitative” method. While the fluidity between “qualitative” and “quantitative” research becomes immediately obvious in big data analyses, this issue has also been lingering in “traditional” social science research for decades. For example, many quantitative researchers simultaneously analyse several thousand variables. Survey research has a long tradition of using qualitative methods for pretesting and evaluating survey questions (Langfeldt and Goltz, 2017; Uher, 2018). Almost all questionnaires contain open-ended questions with non-standardized answers which have to be coded afterwards (Züll and Menold, 2019), and if interviewees or interviewers do not agree with the questionnaire, they might add comments on the side—so-called marginalia (Edwards et al., 2017). During data analysis, results of statistical analyses are often “qualified” when interpreting results. While Kuckartz (2017) provides many current examples for qualification of quantitative data, a well-known older example is Pierre Bourdieu's analysis of social space by using correspondence analysis. Likewise, qualitative research has a long tradition of “quantification” of research results (Vogl, 2017), and similarly to text mining, it is unclear, if qualitative content analysis is a “quantitative” method or rather a “qualitative” method.
Despite these obvious overlaps and fluent borders between “qualitative” and “quantitative” research, the oversimplified view of two different “worlds” or “cultures” (Reichertz, 2019) of social science research practice is upheld in methodological discourse. Accordingly, methodological discourse has reacted increasingly by attempting to combine these traditions via mixed methods research since the early 1980s (Baur et al., 2017). However, although today many differentiated suggestions exist how to best organize a mixed methods research process (Schoonenboom and Johnson, 2017), mixed methods research in a way consolidates this simple distinction between “qualitative” and “quantitative” research, as in all attempts of mixing methods, qualitative and quantitative methods still seem distinct methods—which is exactly why it is assumed that they need to be “mixed.” Moreover, many qualitative researchers complain that current suggestions for mixing methods ignore important principles of qualitative research and instead enforce the quantitative research logic on qualitative research processes, thus robbing qualitative research of its hugest advantages and transforming it into a lacking version of quantitative research (Baur et al., 2017, for some problems arising when trying to take qualitative research logics seriously in mixed methods research, see Akremi, 2017; Baur and Hering, 2017; Hense, 2017).
In this paper, I will address this criticism by focusing on social science research design and the organization of the research process. I will show that the distinction between “qualitative” and “quantitative” research is oversimplified. I will do this by breaking up the debate about “the” qualitative and “the” quantitative research process up in two ways:
Firstly, if one looks closely, there is not “one way” of doing qualitative or quantitative research. Instead, in both research traditions, there are sub-schools, which are characterized by the same degree of ignoring themselves or infighting as can be observed between the qualitative and quantitative tradition.
– More specifically, “quantitative research” can be at least differentiated into classical survey research (Groves et al., 2009; Blasius and Thiessen, 2012; Baur, 2014) and big data analysis of process-generated mass data (“Massendaten”) (Baur, 2009a). Survey data are a good example for research-elicited data, meaning that data are produced by researchers solely for research purposes which is why researchers (at least in theory) can control every step of the research process and therefore also the types of errors that occur. In contrast, process-produced mass data are not produced for research purposes but are a side product of social processes (Baur, 2009a). A classic example for process-produced mass data are public administrational data which are produced by governments, public administrations, companies and other organizations in order to conduct their everyday business (Baur, 2009b; Hartmann and Lengerer, 2019; Salheiser, 2019). For example, governments collect census data for planning purposes; pension funds collect data on their customers in order to assess who later has acquired which types of claims; companies collect data on their customers in order to send them bills etc. Digital data (Foster et al., 2017; König et al., 2018), too, are typically side-products of social processes and therefore count as process-produced data. For example, each time we access the internet, log files are created that protocol our internet activities (Schmitz and Yanenko, 2019), and in many social media, users will leave quantified information—a typical example is Twitter communication (Mayerl and Faas, 2019). Process-produced data can also be analyzed by researchers. In contrast to survey data, they have the advantage of being non-reactive, and for many research questions (e.g., in economic sociology) they are the only data type available (Baur, 2011). However, as they are not produced for research purposes, researchers cannot control the research process or types of errors that may occur during data collection—researchers can only assess how the data are biased before analyzing them (Baur, 2009a). Regardless of researchers using research-elicited or process-produced data, many quantitative researchers aim at replicating results in order to test, if earlier research can uphold scrutiny1. Therefore, one can distinguish between primary research (the original study conducted by the first researcher), replication (when a second researcher tries to produce the same results with the same or different data) and meta-analysis (where a researcher compares all results of various studies on a specific topic in order to summarize findings, see Weiß and Wagner, 2019). In contrast, for secondary analysis, researchers re-use an existing data set in order to answer a different research question than the primary researcher asked. As can be seen from this short overview, there are many diverging research traditions within quantitative research, and accordingly, there are many differences and unresolved issues between these traditions. However, for the purpose of this paper, I will subsume them under the term “quantitative research”, as I have shown in Baur (2009a) that at least regarding the overall organization of research processes, these various schools of quantitative research largely resemble each other.
– The situation is not as simple for “qualitative research”: Not only are there more than 50 traditions of qualitative research (Kuckartz, 2010), but these traditions widely diverge in their epistemological assumptions and the way they do research. In order to be able to better discuss these differences and commonalties, in this paper, I will focus on three qualitative research traditions, which have been selected for being as different as possible in the way they organize the research process, namely “qualitative content analysis” (Schreier, 2012; Kuckartz, 2014, 2018; Mayring, 2014; see also Ametowobla et al., 2017, pp. 776–786), “social-science hermeneutics” (“sozialwissenschaftliche Hermeneutik”), which is sometimes also called “hermeneutical sociology of knowledge” (“hermeneutische Wissenssoziologie”) (Reichertz, 2004a; Herbrik, 2018; Kurt and Herbrik, 2019; see also Ametowobla et al., 2017, pp. 786–790) and “grounded theory” (Corbin and Strauss, 1990; Strauss and Corbin, 1990; Clarke, 2005; Charmaz, 2006; Strübing, 2014, 2018, 2019). Please note that within these traditions, some authors try to combine and integrate these diverse qualitative approaches. However, in order to be able to explore the commonalities and differences better, I will focus on the more “pure,” i.e., original forms of these qualitative paradigms.
Secondly, while it is not possible of speaking of “the” qualitative and “the” quantitative research, it is neither possible of speaking of “the” research process in the sense that there is only one question to be asked when designing social inquiry. Instead, when it comes to discussing the differences between qualitative and quantitative research, at least six issues have to be discussed:
1. How is researchers' perspectivity handled during the research process?
2. How can intersubjectivity be achieved, and what does “objectivity” mean in this context?
3. When and how is the research question focused?
4. Does the research process start deductively or inductively?
5. Is the order of the diverse research phases (sampling, data collection, data preparation, data analysis) organized in a linear or circular way?
6. Is data analysis itself organized in a linear or circular way?
In the following sections, I will discuss for each of these six issues how the four research traditions (quantitative research, qualitative content analysis, grounded theory, social-science hermeneutics) handle them and how they resemble and differ from each other. I will conclude the paper by discussing what this means for the distinction between qualitative and quantitative research as well as mixed methods research.
There are many different types of philosophies of sciences and associated epistemologies, e.g., pragmatism (Johnson et al., 2017), phenomenology (Meidl, 2009, pp. 51–98), critical rationalism (Popper, 1935), critical theory (Adorno, 1962/1969/1993; Habermas, 1981), radical constructivism (von Glasersfeld, 1994), relationism (Kuhn, 1962), postmodernism (Lyotard, 1979/2009), anarchism (Feyerabend, 1975), epistemological historism (Hübner, 2002), fallibism (Lakatos, 1976) or evolutionary epistemology (Riedl, 1985). Moreover, debates within these different schools of thought are often rather refined and organized in sub-schools, as Johnson et al. (2017) illustrate for pragmatism. Regardless, current social science debates simply crudely distinguish between “positivism” and “constructivism”. While this is yet another oversimplification which would be worth deconstructing, for the context of this paper it suffices to note that this distinction is rooted in the demarcation between the natural sciences (“Naturwissenschaften”) and humanities (“Geisteswissenschaften”) in the nineteenth century. It has been the occasion of several debates on the nature of (social) science as well as the methodological and epistemological consequences to be drawn from this definition of (social) science (e.g., Merton, 1942/1973; Smelser, 1976; for an overview see Baur et al., 2018).
In current social science debates, the “quantitative paradigm” is often depicted as being “positivist”, while the “qualitative paradigm” is depicted as being “constructivist” or “interpretative” (e.g., Bryman, 1988) which has consequences on how we conceive social science research processes.
One of the issues debated is, whether social reality can be grasped “per se” as a fact. This so-called “positive stance” was taken e.g., by eighteenth and nineteenth century cameralistics and statistics who collected census and other public administrational data in order to improve governing practices and competition between nation states and who strongly believed that their statistical categories were exact images of social reality (Baur et al., 2018). This “positive stance” was also taken e.g., by the representatives of the German School of History who claimed that facts should speak for themselves and focused on a history of events (“Ereignisgeschichte”) (Baur, 2005 pp. 25–56).
The criticism of these research practices of both the natural sciences (exemplified by early statistics) and the humanities (exemplified by historical research) goes back to the nineteenth century. For example, early German-language sociologists such as Max Weber criticized both traditions because they argued that no “facts” exist that speak for themselves, as both the original data producers of sociological or historical data and the researchers using these data see them from a specific perspective and subjectively (re-)interpret them. In other words: Data are highly constructed. If researchers do not reflect this construction process, they unconsciously (re-)produce their own and the data producer's worldview. As in the nineteenth century, both statistical data and historical documents were mostly originally produced by or for the powerful, nineteenth century statistics and humanities unconsciously analyzed society from their own perspective and the perspective of the powerful (Baur, 2008, p. 192). Consequently, early historical science served to politically legitimate historically evolved orders (Wehler, 1980, p. 8, 44, 53–54).
These arguments are reflected in current debates, e.g., by the debates on how social-science methodology in general and statistics in particular are tools of power (e.g., Desrosières, 2002). They are also reflected in postmodern critiques that every research takes place from a specific worldview (“Standortgebundenheit der Forschung”), which is a particular problem for social science research, as researchers are always also part of the social realities they analyze, meaning that their particular subjectivity may distort research. More specifically, as academia today is dominated by white middle-class men from the Global North, social science research is systematically in danger of creating blind spots for other social realities (Connell, 2007; Mignolo, 2011; Shih and Lionnet, 2011)—an issue Merton (1942/1973) had already pointed out.
At the same time, it does not make sense to dissolve social science research in extreme “constructivism”, as this will make it impossible to assess the validity of research and to distinguish between solid research and “fake news” or “alternative facts” (Baur and Knoblauch, 2018).
In other words: The distinction between “positivism” and “constructivism” creates a dilemma between either denying the existence of different worldviews or abolishing the standards of good scientific practice. In order to avoid this deadlock, early German sociologists (e.g., Max Weber) and later generations of historians reframed this question: The problem is not, if subjectivity influences perception (it does!), but how it frames perception (Baur, 2005, 2008; Baur et al., 2018). In other words, one can distinguish between different types of subjectivity, which have different effects on the research process. In modern historical sciences, at least three forms of subjectivity are distinguished (Koselleck, 1977):
1. Partiality (“Parteilichkeit”): As shown above, subjectivity can distort research because researchers are so entangled in their own value system that they systematically misinterpret or even peculate data. This kind of subjectivity has to be avoided at all costs.
2. “Verstehen”: Subjectivity is necessary to understand the meaning of human action (and data in general), so in this sense, it is an important resource for social science research, especially in social-science hermeneutics.
3. Perspectivity (“Perspektivität”): Subjectivity is also a prerequisite for grasping reality. The first important steps in social science research are framing a research question as “relevant” and “interesting”, addressing this question from a certain theoretical stance and selecting data appropriate for answering that question.
Starting from this distinction, early German sociologists argued that—as one cannot avoid perspectivity—it is important to reflect it and make it explicit. And one does this by making strong use of social theory and methodology when designing and conducting social science research (Baur, 2008, pp. 192–193). The point about this is that social science research still creates blind spots (because reality can never be analyzed as a whole) but as these blind spots are made explicit, they become debatable and can be openly addressed in future research.
If one reframes the question, the debate between “positivism” and “constructivism” implodes, as the comparison of the four research traditions reviewed in this paper illustrates: Quantitative research, qualitative content analysis, grounded theory and social-science hermeneutics all make a strong argument that social theory is absolutely necessary for guiding the research process2. In order to establish how social theory and empirical research should be linked, one first has to define what “social theory” actually is (Kalthoff, 2008). This is important as theories differ in their level of abstraction and at least three types of theories can be distinguished (Lindemann, 2008; Baur, 2009c; Baur and Ernst, 2011):
1. Social Theories (“Sozialtheorien”), such as analytical sociology, systems theory, communicative constructivism, actor network theory or figurational sociology, contain general concepts about what society is, which concepts are central to analysis (e.g., actions, interactions, communication), what the nature of reality is, what assumptions have to be made in order to grasp this reality and how—on this basis—theory and data can be linked on a general level.
2. Middle-range theories (“Theorien begrenzter Reichweite”) concentrate on a specific thematic field, a historical period and a geographical region. They model social processes just for this socio-historical context. For example, Esping-Andersen's (1990) model of welfare regimes argues that there have been typical patterns of welfare development in Western European and Northern American societies since about the 1880s. In contrast, in their study “Awareness of Dying”, Glaser and Strauss (1975), address topics of medical sociology and claim to have identified typical patterns that are valid for the U.S. in the 1960s and 1970s.
3. Theories of Society (“Gesellschaftstheorien”) try to characterize complete societies by integrating results from various studies to a larger theoretical picture, e.g., “Capitalism”, “Functionally Differentiated Society,” “Modernity,” and “Postmodernity.” In other words, theories of society build on middle-range theories and further abstract them. Middle-range theories and theories of society are closely entwined as an analysis of social reality demands “a permanent control of empirical studies by theory and vice versa a permanent review of these theories via empirical results” (Elias, 1987, p. 63). For example, in figurational sociology, the objective is to focus and advance sociological hypotheses and syntheses of isolated findings for the development of a “theory of the increasing social differentiation” (Elias, 1997, p. 369), of planned and unplanned social processes, and of integration and functional differentiation (Baur and Ernst, 2011).
These types of theories are entwined in a very typical way during the research process. Namely, all social science methodologies are constructed in a way that social theory is used to build, test and advance middle-range theories and theories of society (Lindemann, 2008; Baur, 2009c). Therefore, social theory is a prerequisite for social research as it helps researchers decide which data they need and which analysis procedure is appropriate for answering their research question (Baur, 2005, 2008). Social theory also allows researchers to link middle-range theories and theories of society with both methodology and research practice, as not all theories can make use of all research methods and data types (Baur, 2008). For example, rational choice theory needs data on individuals' thoughts and behavior, symbolic interactionism needs data on interactions, i.e., what is going on between individuals.
Due to the importance given to social theory, it is unsurprising that all research traditions stress that the theoretical perspective needs to be disclosed by explicating the study's social theoretical frame and defining central terms and terminology at the beginning of the research process (Weil et al., 2008). The dispute between the four methodological traditions discussed in this paper is whether one needs to have a specific middle-range theory in mind at the beginning of the research process or not. In quantitative research, specifying one or more middle-range theories in advance is necessary in order to formulate hypotheses to be tested. The opposing point of view is that of grounded theory which explicitly aims at developing new middle-range theories for new research topics and therefore by nature cannot have any middle-range theory in mind at the beginning of the research process. Qualitative content analysis and social-science hermeneutics are someway in between these extreme positions.
All in all, explicating one's social theoretical stance is a major measure against partiality, as assumptions are explicated and thus can be criticized. All research traditions analyzed for this paper also agree on a second measure against partiality: self-reflection. In addition, each research tradition has developed distinct methodologies in order to handle subjectivity and perspectivity, i.e., in order to avoid partiality crawling back in via the backdoor.
In the tradition of critical rationalism (Popper, 1935), quantitative research systematically aims at falsification. Ideally, different middle-range theories and hypotheses compete and are tested against each other. For example, survey methodology typically tries to test middle-range theories, meaning that at the beginning of the research process not only the general social theory but also middle-range theories must be known and clarified as well as possible. Then, these theories typically are formulated into hypotheses, which then are operationalized and can be falsified during the research process. This idea of testing theories can be seen in two typical ways of doing so:
(a) One can use statistical tests to falsify hypotheses.
(b) The other way of testing theories in quantitative research is using different middle-range theories and see which theory fits the data best.
Qualitative research has repeatedly stated that the point about qualitative research is that very often, no middle range theory exists (which would be needed for testing theories), and the aim of qualitative research is exactly to build these middle range theories. Therefore, in most qualitative research processes, testing theories with data is not a workable solution. Instead, qualitative research has suggested “triangulation” of theories, methods, researchers and data (Flick, 1992, 2017; Seale, 1999, pp. 51–72) as an alternative. Note that the idea of triangulating theories in qualitative research is very similar to the idea of testing theories against each other in quantitative research. But in contrast to quantitative research, in qualitative research theories are not necessarily spelled out in advance but instead are built during data analysis. Note also, that the idea that different researchers address the same problem with different data is also equal to the way quantitative research is organized in practice: In the ideal quantitative research process, each single study is just a small pebble in the overall mosaic, to be published e.g., in an academic paper. Then other researchers (e.g., from different institutions) can use other data (e.g., from a different data set) and see how well these fit the theory, i.e., they try to replicate results of the primary study, and the results of various replications can be then summarized in a meta-analysis (Weiß and Wagner, 2019).
In addition, qualitative content analysis has a strong tradition of handling researchers' perspectivity, not only by triangulating them but in fact by using different researchers to code in parallel e.g. the interview data and then comparing these codings. This procedure works better, the more dissimilar the researchers are concerning disciplinary, theoretical, methodological, political and socio-structural background, as contrasting researchers likely have also very different perspectives on the topic. If two such researchers independently coded the same text passages similarly, hopefully perspectivity can be ruled out. In contrast, if the same passages are coded differently by two persons, then one must interpret and take a closer look on how researchers' subjectivity and perspectivity might have influenced the coding process. All in all, qualitative content analysis makes use of research teams in a way that researchers first work independently and then results are compared. Note that this is similar to the way modern survey research works in practice: Here, questionnaires are typically developed and tested in teams, following the concept of the “Survey Life Cycle” (Groves et al., 2009), and a main means of evaluating survey questions is expert validation by other, external researchers. Similar, during data analysis, it is typical for quantitative researchers to re-analyze data that have already been analyzed by other teams. This is one of the reasons why archiving and documenting data is good-practice in survey methodology.
Social-science hermeneutics, too, have a strong tradition of researchers working together, but this co-operation and reciprocal control is organized in a different way: In contrast to qualitative content analysis and survey research (where researchers first work independently and then results are compared), the order of co-operation and independent research is reversed in social-science hermeneutics: The research team is used at the beginning of data analysis in so-called “data sessions” (“Datensitzungen”) (Reichertz, 2018). The team focusses on one section of the text and does a so-called “fine-grained analysis” (“Feinanalyse”). During these data sessions, the research teams collectively develops different interpretations or “readings” (“Lesarten”) (Kurt and Herbrik, 2019). In fact, these interpretations resemble hypothesis formation in quantitative research, and the following analysis steps also strongly resemble quantitative research, as after the data session, researchers can individually or collectively test the hypotheses (= interpretations). However, in hermeneutics, interpretations are not tested using statistical tests but using “sequential analysis” (“Sequenzanalyse”). During sequential analysis, the text is used as material for testing the hypotheses developed during data sessions: If an interpretation holds true, there should be other hints in the data that point to that interpretation, while other interpretations might be falsified by additional data (Lamnek, 2005, pp. 211–230, 531–546; Kurt and Herbrik, 2019).
Grounded theory handles subjectivity differently in so far that it has developed different procedures for theoretically grounding the research process and for building theoretical sensitivity (Strauss and Corbin, 1990). The starting point of discussions on theoretical sensitivity is that researchers—being human—cannot help but entering the field not only with their social theoretical perspective but also with their everyday knowledge and prejudices which may bias both their observations and their interpretations. This may also mislead researchers to gloss over inconsistencies or interesting points in their data too fast. Note that the problem formulated here is very similar to the idea of the “investigator bias” in experimental research. In order to tackle this tendency for misinterpretation, grounded theory states that researchers need to develop theoretical sensitivity, i.e., “to enter the research setting with as few predetermined ideas as possible (…). In this posture, the analyst is able to remain sensitive to the data by being able to record events and detect happenings without first having them filtered through and squared with pre-existing hypotheses and biases” (Glaser, 1978, p. 2–3). In order to develop and uphold this open-mindedness for new ideas, Strauss and Corbin (1990) suggest a number of specific procedures such as systematically asking questions or analyzing words, phrases and sentences. Grounded theory also suggests many ways of systematic comparisons such as “flip flop techniques,” “systematic comparison” and so on. Another modus operandi suggested is “raising the red flag.” These techniques for increasing theoretical sensitivity are meant as procedures that can be used if researchers are working on their own and do not have a team of coders who can code in parallel.
Summing up the argument so far, all qualitative and quantitative approaches analyzed suggest a strong use of social theory. Social theory transforms partiality into perspectivity by focusing on some aspects of (social) reality which then guide the research process. This necessarily creates blind spots. The difference between unreflected subjectivity and social theory is that by using social theory, blind spots are explicated. Consequently, both their assumptions and consequences can be discussed and criticized. All research traditions also have developed further methodologies in order to handle perspectivity in research practice. Two common ideas are working in teams and testing ideas that have been developed in earlier research. Regardless of research tradition, for these suggestions of using research teams for controlling partiality and handling perspectivity to be effective, it is necessary for the team members to be both knowledgeable about the topic and as different as possible concerning biographical experience (e.g., gender, age, disability, ethnicity, social status etc.) and theoretical stance. Note that none of these countermeasures guarantee impartiality—they just help to better handle it.
So far, I have argued that one way to address the distinction between “positivism” and “constructivism” is the issue of how to handle a researcher's subjectivity by transforming it into perspectivity, which in turn makes the specific blind spots created by a researcher's theoretical perspective obvious and opens them for theoretical and methodological reflexion. However, “positivism” and “constructivism” do not only address the relation of social science research to a researcher's personal, subjective perspective but also to the issue of how to achieve intersubjectivity—in other words, how to make research as “objective” as possible in order to be able to distinguish more and less valid research. In this sense, perspectivity is closely linked to the concept of “objectivity.”
Now, the debate on “objectivity” is a complicated issue, as the comparison of the four research traditions (quantitative research, qualitative content analysis, grounded theory, and social-science hermeneutics) reveals:
First of all, it is not clear at all what “objectivity” means in different research traditions. In quantitative research, “objectivity” and “intersubjectivity” are used synonymously and mean that independent researchers studying the same social phenomenon always come to the same results, as long as the social phenomenon remains stable. This concept of objectivity has consequences on the typical way quantitative inquiries are designed: The wish to ensure that researchers can actually independently come to the same result is the main reason why quantitative research tries to standardize everything that can be possibly standardized, as can be exemplified by survey research: sampling (random sampling), the measurement instruments (questionnaires), data collection (interviewer training, interview situation) as well as data analysis (statistics) (Baur et al., 2018). The idea is that by standardization, it does not matter who does the research and results become replicable. Qualitative content analysis tries to copy these procedures by techniques such as parallel coding discussed above. Other examples of aiming at getting as close to objectivity as possible are concepts like intercoder reliability.
However, this aim at achieving objectivity by controlling any effect a researcher's subjectivity might have on the research process does not work in practice at all: Despite all attempts of standardization, quantitative researchers have to make many theoretical and practical decisions during the research process and therefore interpret their data and results (Baur et al., 2018). This is true for all stages of the research process, starting from focussing the research question (Baur, 2008) to designing instruments and data collection (Kelle, 2018), data analysis (Akremi, 2018; Baur, 2018) and generalization using inductive statistics (Ziegler, 2018). In this sense, all quantitative research is “interpretative” as well (Knoblauch et al., 2018)—a fact, that is hidden by terminology: While qualitative research talks about “interpretation”, quantitative research talks about “error”, but this basically means the same, i.e., that regardless how much researchers might try, social reality cannot be “objectively” grasped by researchers. Instead, there is always a gap between what is represented in the data and what is “truly” happening (Baur and Knoblauch, 2018; Baur et al., 2018).
In order to react to this problem, within the quantitative paradigm, survey research has developed the concept of the “Total Survey Error” (TSE) in the last two decades (Groves et al., 2009). The key argument is that various types of errors might occur during the research process, and these various errors are often related in the sense that—if you reduce one error type—another error increases. For example, in order to minimize measurement error, it is typically recommended to ask many different and detailed questions, a classical example being a psychological test for diagnosis of mental disorders, which usually takes more than 1 hour to answer and is very precise. However, these kinds of questionnaires could not be used in surveys of the general population, as respondents typically are only willing to spend a limited time on answering survey questions. If the survey is too long, they will either outright decline to participate in the survey or drop out during processing the survey—which in turn results in unit or item nonresponse. Therefore, researchers typically ask fewer questions in surveys, which increases the likelihood of measurement error. In this example, there is a trade-off between measurement error (due to short questionnaires) and nonresponse error (due to long questionnaires). The other error types are likewise related. Therefore—while traditionally, these various errors were handled individually—modern survey methodology tries to incorporate them into one concept—the “Total Survey Error.” This means that researchers should take into account all errors and try to minimize the error as a whole. However, as errors can only be minimized and never completely deleted, logically, there will always remain a gap between “objective reality” and “measurement.” In other words, in research practice, survey methodology has long abolished the idea that it is possible to “objectively” measure reality. Instead, there might always be a difference between what truly happens and what the data convey (Baur, 2014, pp. 260–262).
The discussion about the Total Survey Error already suggests that trying to achieve “objectivity”—in the sense that everybody who does research may achieve the same result, if the research process is well-organized—only works to a specific point. While quantitative research tries to come as close to this goal as possible, there are many fields of social reality, where the attempt of achieving objectivity via standardization faces huge difficulties. Examples are cross-cultural and comparative research (Baur, 2014) and fields characterized by rapid social change (Kelle, 2017) because here concepts are not stable across contexts.
Based on these observations, most qualitative research traditions argue that a concept of objectivity in the quantitative sense does not make sense either for qualitative research or not at all—because it is never possible to achieve, as many social contexts are changing very fast and in fact so fast that it is difficult to build middle range theories that can be tested, as the object of research might have already changed before a researcher can replicate the study. In turn, most qualitative researchers define “objectivity” differently. For example, in social-science hermeneutics, “objectivity” means that researchers should reflect, document and explain how they arrived at their conclusions, i.e., how they collected and interpreted data (Lamnek, 2005, pp. 59–77). Seale (1999, pp. 141–158) calls this “reflexive methodological accounting.” The idea is that this makes it possible to criticize and validate research. While this is a more basic concept of “objectivity,” this is a concept that all researchers (including quantitative researchers) can agree on.
While I have, firstly, shown that “objectivity” can mean very different things, it is also, secondly, an oversimplification to claim that all quantitative researchers believe that “objectivity” (in the sense of the quantitative paradigm) can be actually achieved and that all qualitative researchers disbelieve this. As stated above, many quantitative researchers have long-ago given up the idea that there can be a “true,” “objective” measurement of reality. Moreover, there are many qualitative researchers who actually believe that “objectivity” (in the sense of the quantitative paradigm) can be achieved, and this believe in the possibility of “true measurement” can be found in all research traditions (Baur et al., 2018), e.g., within qualitative content analysis, Mayring (2014) does believe in “objectivity,” Kuckartz (2014) does not and takes a more interpretative stance. Similarly, within the hermeneutical tradition, Oevermann et al. (1979) as well as Wernet (2006) believe in “objectivity,” Maiwald (2018) and Herbrik (2018) take a more interpretative stance. All in all, the picture is much more complicated than it seems on first sight—something that is definitively worth exploring in more detail in future research.
A consequence of the above discussion is that all social science methodology needs to make strong use of social theory in order to guide a researcher's perspective, in other words: Research questions need to be focused and in fact, research becomes better, if and when it is focused, as it allows researchers to consciously collect and analyze exactly the data they need in order to answer the question. As I have shown in Baur (2009c, pp. 197–206), one can use social theory for focusing, and the research question has to be focused at least concerning four dimensions: (1) action sphere, (2) analysis level, (3) spatiality and (4) temporality with the two sub-dimensions (4a) pattern in time and duration. In additions, researchers have to decide, if there are interactions both within and between these dimensions, e.g., between long-term and short-term developments or between space and time. As a rule of thumb, if one wants to explore one of these dimensions in detail, it is advisable to reduce as much complexity for the other dimensions as possible.
While this need for focusing the research question is something that quantitative research, qualitative content analysis, grounded theory and social-science hermeneutics would agree on, they differ on the question, when the research question is focused:
Both quantitative research, qualitative content analysis and social-science hermeneutics formulate the research question as precisely as possible at the beginning of the analysis in order to know what types of data need to be collected and analyzed. Quantitative research needs to know e.g., what kinds of questions to ask in a questionnaire, and in order to be able do this, researchers need to know what they want to know. Similarly, social-science hermeneutics need to know which text passage is especially theoretical relevant and thus worthy of being analyzed in the first data session—usually, only a single or a few sentences are selected, and in order to do this selection, researchers, too, need to know what they want to know. While qualitative content analysis usually collects open-ended data such as interview data or documents, a (for qualitative research) relatively large amount of similarly types of e.g., interviews or documents is collected, and data collection is often divided up between team members—again, in order to be fruitful, this is only possible, if researchers, know what they want to know.
In contrast, grounded theory opposes this early focusing, arguing that researchers might miss the most innovative or important points of their research, if they focus too early, especially, if they enter a new research field they know very little about. Instead, grounded theory suggests that researchers should start with a very general research question at the beginning of the analysis, which is focused during data analysis. In order to enable a focusing grounded in data, grounded theory has developed an own technique for focusing the research process: selective coding (Corbin and Strauss, 1990).
Among other things, this has effects on the way social research results are written up, e.g., in a paper or book: While in quantitative research, qualitative content analysis and social-science hermeneutics, researchers can more or less decide how their text will be organized after they have focused their question, in grounded theory, the order of analysis and the order of writing may largely differ—deciding on how the final argument should be structured is an important part of selective coding and can only be decided on relatively late during research, i.e., after the research question has been focused.
These ideas of how social theory is used for handling objectivity and perspectivity as well as when and how the research question should be focused, strongly influence how the overall research process is designed. Concerning this overall design, the difference between qualitative and quantitative research seems clear:
In current methodological discourse, it is generally assumed that quantitative research is deductive. As depicted in Figure 1, “deduction” means that researchers start research by deriving hypotheses concerning the research from the selected theory. Researchers then collect and analyze data, in order to test their hypotheses (Hempel and Oppenheim, 1948).
In contrast, it is generally assumed that qualitative research systematically makes use of inductivism. As illustrated in Figure 1, “induction” starts from the data and then analyses which theory would best fit the data (Strauss and Corbin, 1990).
This simple distinction is another oversimplification in several ways:
First, the idea of induction and deductions has been supplemented by idea of “abduction” (Peirce, 1878/1931; Reichertz, 2004b, 2010, 2013), which resembles induction in the sense that both start analysis from data and conclude from data to theory (in contrast to deduction). However, induction only draws on existing theories—if no theory is known that fits or can model the data analysis, induction fails. Researchers can only invent [sic!] a new theory—and this is called abduction (Reichertz, 2004b, 2010, 2013). Grounded theory (Corbin and Strauss, 1990) and social-science hermeneutics (Reichertz, 2004b, 2010, 2013) are the only of the four research traditions which explicitly stress the necessity and importance of abduction, especially as it is the only way of really creating new knowledge. However, in research practice, all researchers in all research traditions need to work abductively at some point, e.g., when they study a completely new social phenomenon (where no prior knowledge can exist).
Secondly, no actual research process is purely deductive, inductive or abductive:
– In actual research processes, what usually happens when researchers start their research deductively, is that they build a theory, collect and test data—and then research results differ from what was expected. This does not mean that the researcher made a mistake or that research is “bad”—on the contrary: If one assumes that only research questions that can actually yield new results are worthy of being explored, then it is to be expected that results differ from what researchers have deduced from their data. Similarly, if one truly tries to falsify data, it must be possible that the data can actually contradict the theory. The point for the debate about induction and deduction is that researchers usually never end analysis here. Instead, they will take a closer look at the data, re-analyze them and look for other explanations for their results, i.e., they will check, if a different theory than the one considered originally might fit the data better. In the moment they are doing this, they change from the logic of deduction to the logic of induction.
– Likewise, if researchers start data analysis inductively, this means that they start interpreting the data and muse, if there is any theory that might fit the data. Once they have identified theories in line with the data, researchers usually go on testing these theories by using further data. An example is the sequence of data sessions and sequence analysis in social-science hermeneutics discussed above. However, in the moment researchers start testing their hypothesis, they have switched from induction to deduction.
– Similarly, if researchers start abductively, after abduction, they have a theory that can be tested by deduction.
In further research, researchers will typically switch from induction to deduction and back several time, regardless which paradigm they work with. So in principle, all social science research makes use of both induction and deduction. If the existing theories do not fit the data, researchers will additionally make use of abduction.
Rather, the difference between the traditions lies is how they begin research:
Quantitative researchers have no choice but to start deductively as they need to know what standardized data they need to collect (e.g., which questions to ask in a survey) and which population the random sample needs to be drawn from—and in order to do so, they need to exactly know what they want to know, i.e., which hypothesis to test. While this is often depicted as an advantage, it is actually a problem when researchers are analyzing unfamiliar fields or if social phenomena are so new that researchers do not know, which theory is appropriate for answering the question.
As suggested by the discussion so far, social-science hermeneutics and grounded theory usually start with induction and then later switch to deduction: Grounded theory specifically aims at building theories for unfamiliar fields, which is exactly one of the reasons why the research question is focused only later in research. In contrast, social-science hermeneutics focus the research question early but only develop hypotheses inductively from the material during data sessions. This illustrates that the logics of deduction and induction are not necessarily linked to the issue when and how the research question is focused.
In contrast, qualitative content analysis also starts deductively. This shows that the simple idea that qualitative research uses induction and quantitative research uses deduction cannot be upheld. On the contrary, there is some qualitative research that starts deductively while other qualitative research might start inductively. Regardless of the logic of beginning, qualitative and quantitative research will swap between the logics in the course of the further research process.
The question, if the research process is deductive or inductive is often mingled with the question, if the research process is linear or circular. However, these are not the same things: “Deduction” and “induction” describe ways of linking theory and data. “Linearity” and “circularity” address the issue, how different research phases are ordered, namely, if (a) posing and focusing the research question; (b) sampling; (c) designing instruments; (d) collecting data; (e) preparing data; (f) analyzing data; (g) generalizing results; and (h) archiving data follow one after the other (“linearity”), or if they are iterated (“circularity”). Again, it is generally assumed that quantitative research is organized in a linear way, while qualitative research is organized in a circular way.
Indeed, quantitative research is and always has to be organized in a linear way. This is a direct result of the quantitative concept of objectivity, deduction in combination with the idea of making use of numbers: As stated above, in order to ensure that researchers influence the research process as little as possible but also in order to enable a strong division of labor, research instruments are developed using a prescribed order. Many quantitative techniques do not work, if one deviates from this model. For example, in order to generalize results using inductive statistics, the sample has to be a random sample. A sample is only random, if a population is defined first, then the sample is randomly drawn from this population, and only then data are collected and all units drawn actually participate. If there is unit or item nonresponse, there might be a systematic error (meaning that the sample becomes a nonprobability sample and thus making it impossible to use inductive statistics for generalization). Recruiting additional cases later does not resolve this problem because it contradicts the logic of random sampling (Baur and Florian, 2008; Baur et al., 2018). All in all, this means that both sampling and the development of the instrument must be conducted before data collection. Then, all data must be collected and thereafter analyzed in a bunch. So, the logics of trying to formulate the hypotheses as standardized as possible has the result that quantitative research must be linear in the sense of research phases being organized step-by-step.
Although the need of linearity sometimes is depicted as an advantage, the contrary is true: Often, linearity is a problem because very often, researchers only realize during the actual research that they have made mistakes or false assumptions or forgotten important aspects of the phenomenon under investigation. In circular research processes, these can be easily corrected. However, in linear research processes, this is not possible without setting up a whole new study. That this is not just a general statement but an actual problem that quantitative researchers perceive themselves is reflected in the fact that psychometrics has been using iterative processes of item generation, testing and selection as established practice for several decades. In the last two decades, sociological survey methodologists, too, have tried to derive as much as possible from linearity by developing the concept of the “Survey Life Cycle” (Groves et al., 2009). While in traditional survey methodology, sampling and instrument development were subsequent phases, now at least during instrument development, feedback loops are built in. Panel and trend designs even allow for making slight adjustments both of the instruments and the sample after data analysis in later waves. Regardless, a true circularity is not possible in the logic of quantitative research processes—in principle, the research process concerning the order of building instruments, sampling, data collection and data analysis is linear.
Linearity is also a characteristic of qualitative content analysis, which starts with sampling and collecting data (for example by conducting interviews or sampling texts), then preparing them in a qualitative data analysis software, coding them and afterwards structuring the data. In social-science hermeneutics, too, the overall research process is linear in the sense that usually first data are collected, then transcribed and then analyzed. Similar to survey research, both qualitative content analysis and social-science hermeneutics might build circular elements into the research process later, e.g., by collecting more data or sampling new cases—still, all in all, all these research processes remain linear in nature, which contrasts common-sense knowledge on qualitative data analysis.
Of the four research traditions analyzed, the only research process truly circular is that of grounded theory. In fact, grounded theory argues most explicitly that linearity is inefficient because a lot of time is wasted on things researchers relatively soon realize they do not need to know and because linearity forces researchers to spend a lot of time before they can actually get started. Thus, grounded theory not only propagates circularity but has also developed suggestions of how to organize this circularity in research practice. In this regard, the key concept is “theoretical sampling,” which states that researchers should start analysis as soon as possible with one single case. The first case sampled is ideally the critical case (i.e., a case that should not exist in theory but exists empirically) or the case from which researchers can learn the most given their current understanding. Then data for this case only are collected and immediately analyzed. Depending on what has been learned from the first case, researchers select the second case that likely contrasts the most with the first case. This process is based on the idea that one can learn more from new cases, if they provide as different information as possible. Then data are collected only for the second case and analyzed immediately, then a third contrasting case is selected and so on, until results are “theoretically saturated,” i.e., no new ideas or information arises. Theoretical sampling not only allows for developing and adjusting the sampling plan during data analysis but also allows to change the data collection or analysis methods used. For example, researchers could start with qualitative interviews and then later change to ethnography or other kinds of data which will be analyzed. So all in all, given the ways in which the research phases follow each other, it is only grounded theory that differs from the other traditions.
The distinction between linear and circular research processes becomes completely blurred when looking at data analysis. On paper, all qualitative traditions discussed in this paper openly build in circular elements into their data analysis: Researchers using qualitative content analysis conduct different rounds coding the data. Grounded theory, as stated above, as a matter of principle does not only change between different phases of data collection but also differentiates between open, axial and selective coding. Hermeneutics are also circular in the sense that once the different interpretations are developed, the material is tested in different ways.
Quantitative data analysis seems to be completely different, on first sight, as it appears to be linear: If you follow the textbook, quantitative researchers should develop hypotheses at the beginning of the research process, then design their instruments, plan how to analyze them, sample, collect and prepare data. Next, researchers will use statistics to test the hypotheses—and until this step, good quantitative research practice also follows the book.
However, as stated above, what usually happens is that researchers do not achieve the results as expected—and in fact, this is a desirable result, because otherwise research would never produce new insights, and in the sense of quantitative logics, it should be possible to falsify results.
Still, have you ever read a paper that said “I have done an analysis and did not get the results I wanted or expected … so I am finished now! Sorry!” or “I have falsified my hypotheses and now we do not know anything because what we thought we knew has been falsified”?
That you have very likely never read a paper like this, is because quantitative data analysis is not as strictly deductive-linear as it pretends to be in methods textbooks. But in fact, quantitative data analysis is much more organized in a circular way, similar to the qualitative research traditions. More specifically, when quantitative researchers do not achieve the results they expected, they switch to induction and/or abduction—data analysis now becomes circular in the sense that researchers analyze the dataset in different rounds. After the first round of unexpected results, researchers might e.g., either conduct a more detailed analysis of a specific variable or subgroup which is more interesting, or they might use different statistical procedures to find clues why the results were different than expected. The only difference to qualitative research is that quantitative researchers have to limit their analysis to the data they have—if information is not contained in the data set, they would need to conduct a whole new study. Regardless, the important point for this paper is that—while the overall research process can be either organized in a linear or circular way, during data analysis, all social science research is organized in a circular way in research practice, whether researchers admit this or not.
In this paper, I have shown how four research traditions (quantitative research, qualitative content analysis, grounded theory, social-science hermeneutics) handle six issues to be resolved when deciding on a social science research design, namely: How is researchers' perspectivity handled during the research process? How can intersubjectivity be achieved, and what does “objectivity” mean in this context? When and how is the research question is focused? Does the research process start deductively or inductively? Are the diverse research phases (sampling, data collection, data preparation, data analysis) organized in a linear or circular way? Is data analysis organized in a linear or circular way? For each of these issues, I have discussed how the four traditions resemble and differ from each other. Table 1 sums up the various positions.
Table 1. Commonalities and differences between research traditions concerning some aspects of the research process.
When regarding the whole picture depicted in Table 1, it is possible to state that the common-sense knowledge that “quantitative research” organizes its research process deductively, tests theories, does objective, positivist research and organizes the research process in a linear way while “qualitative research” organizes its research process inductively, develops theories, has a constructivist stance on research and organizes the research process in a circular way, cannot be upheld for at least three reasons:
1. Quantitative research is not as objective, deductive and linear as it is often depicted in literature. It is much more necessary to interpret in all phases of quantitative research as quantitative researchers usually admit. During data analysis, quantitative research has always iterated between deduction, induction and abduction, and concerning the overall organization of the research process, quantitative research has recently tried to dissolve linearity as much as possible, as exemplified in the concept of the “Survey Life Cycle.”
2. For all these issues, there are some qualitative traditions that resemble quantitative research more than quantitative research. As this is not a new revelation, the distinction between “qualitative” and “qualitative” research has often been depicted as continuum, resulting in an order (from strong “quantitativeness” to strong “qualitativeness”) from quantitative methods, qualitative content analysis, grounded theory and social-science hermeneutics. The general argument is that qualitative content analysis is “almost” quantitative research, while social-science hermeneutics is one of the “truest” forms of qualitative research.
3. However, neither can a continuum between qualitative and quantitative research be upheld, i.e., one can neither claim that qualitative content analysis is per se closer to quantitative research than social-science hermeneutics nor is grounded theory positioned in the middle. Rather, depending on the debated issue concerning the research process, social-science hermeneutics might resemble quantitative research much more than qualitative content analysis. For example, while it is true that both quantitative research and qualitative content analysis organize the overall research process more linearly than grounded theory and social-science hermeneutics do, when it comes to handling theory, social-science hermeneutics are “stricter” than the other two qualitative traditions in the sense that they systematically test theories.
To conclude, the oversimplified distinction between “qualitative” and “quantitative” research cannot be upheld. This is both a chance and a challenge for mixed methods research. On the bright sight, mixing and combining qualitative and quantitative methods becomes easier because the distinction is not as grand as it seems at first sight and the boundaries between research traditions are much more blurred. It thus might be easier to focus on practical issues of mixing instead of epistemological debates. On the dark sight, mixing becomes more difficult because some tricky issues of mixing specific types of methods are usually not addressed in current mixed methods discourse. More specifically, mixed methods research so far has strongly focussed on mixing traditions that can be easily mixed due to some similarities in the research process, e.g., quantitative research and qualitative content analysis. When the discussion presented here is taken seriously, it would be much more fruitful to discuss how to combine quantitative research e.g., with grounded theory and social-science hermeneutics because in these traditions the research process is more circular and this circularity is part of their strength. To effectively use the potential of these paradigms, it would be necessary to implement these circular elements in mixed methods research.
The author confirms being the sole contributor of this work and has approved it for publication.
The author declares that the research was conducted in the absence of any commercial or financial relationships that could be construed as a potential conflict of interest.
1. ^Note that for many social phenomena, replication is not possible due to the nature of the research object, e.g. for macro-social or fast-changing social phenomena (Kelle, 2017) – see below for more details.
2. ^To clarify a common misconception of qualitative research: When qualitative researchers demand that research should be ‘open-ended' (‘Offenheit'), they do not mean that they are not using theory but that they are using an inductive analytical stance (see below).
Adorno, T. W. (1962/1969/1993). “Zur Logik der Sozialwissenschaften,” in Der Positivismusstreit in der Deutschen Soziologie, eds T.W. Adorno, R. Dahrendorf, R. Pilot, H. Albert, J. Habermas, and K. R. Popper (München: dtv), 125–143.
Akremi, L. (2017). Mixed-Methods-Sampling als Mittel zur Abgrenzung eines unscharfen und heterogenen Forschungsfeldes. Am Beispiel der Klassifizierung von Zukunftsängsten im dystopischen Spielfilm. Kölner Z. Soz. Sozialpsychol. 69 (Suppl. 2), 261–286. doi: 10.1007/s11577-017-0460-3
Akremi, L. (2018). “Interpretativität quantitativer Auswertung. Über multivariate Verfahren zur Erfassung von Sinnstrukturen,” in Handbuch Interpretativ forschen, eds L. Akremi, N. Baur, H. Knoblauch, and B. Traue (Weinheim; München: Beltz Juventa), 361–408.
Ametowobla, D., Baur, N., and Norkus, M. (2017). “Analyseverfahren in der empirischen Organisationsforschung,” in Handbuch Empirische Organisationsforschung, eds S. Liebig, W. Matiaske, and S. Rosenbohm (Wiesbaden: Springer), 749–796. doi: 10.1007/978-3-658-08493-6_33
Baur, N. (2005). Verlaufsmusteranalyse: Methodologische Konsequenzen der Zeitlichkeit sozialen Handelns. Wiesbaden: VS-Verlag für Sozialwissenschaften. doi: 10.1007/978-3-322-90815-5
Baur, N. (2008). Taking perspectivity seriously: a suggestion of a conceptual framework for linking theory and methods in longitudinal and comparative research. Hist. Soc. Res. 33(4), 191–213. doi: 10.12759/hsr.33.2008.4.191-213
Baur, N. (2009a). Measurement and selection bias in longitudinal data: A framework for re-opening the discussion on data quality and generalizability of social bookkeeping data. Hist. Soc. Res. 34(3), 9–50. doi: 10.12759/hsr.34.2009.3.9-50
Baur, N. (ed.). (2009b). Social Bookkeeping Data: Data Quality and Data Management. Historical Social Research 129. Mannheim and Köln: GESIS.
Baur, N. (2009c). Problems of linking theory and data in historical sociology and longitudinal research. Hist. Soc. Res. 34(1), 7–21. doi: 10.12759/hsr.34.2009.1.7-21
Baur, N. (2011). Mixing process-generated data in market sociology. Qual. Quant. 45(6), 1233–1251. doi: 10.1007/s11135-009-9288-x
Baur, N. (2014). Comparing societies and cultures: Challenges of cross-cultural survey research as an approach to spatial analysis. Hist. Soc. Res. 39(2), 257–291. doi: 10.12759/hsr.39.2014.2.257-291
Baur, N. (2018). “Kausalität und Interpretativität. Über den Versuch der quantitativen Sozialforschung, zu Erklären, ohne zu Verstehen,” in Handbuch Interpretativ forschen, eds L. Akremi, N. Baur, H. Knoblauch, and B. Traue (Weinheim; München: Beltz Juventa), 306–360.
Baur, N., and Blasius, J. (2019). “Methoden der empirischen Sozialforschung. Ein Überblick,” in Handbuch Methoden der empirischen Sozialforschung, eds N. Baur and J. Blasius (Wiesbaden: Springer), 1–30.
Baur, N., and Ernst, S. (2011). Towards a process-oriented methodology. Modern social science research methods and Nobert Elias' figurational sociology. Sociol. Rev. 59(777), 117–139. doi: 10.1111/j.1467-954X.2011.01981.x
Baur, N., and Florian, M. (2008). “Stichprobenprobleme bei Online-Umfragen,” in Sozialforschung im Internet. Methodologie und Praxis der Online-Befragung, eds N. Jackob, H. Schoen, and T. Zerback (Wiesbaden: VS-Verlag), 106–125.
Baur, N., and Hering, L. (2017). Die Kombination von ethnografischer Beobachtung und standardisierter Befragung. Mixed-Methods-Designs jenseits der Kombination von qualitativen Interviews mit quantitativen Surveys. Kölner Z. Soz. Sozialpsychol. 69(Suppl. 2), 387–414. doi: 10.1007/s11577-017-0468-8
Baur, N., Kelle, U., and Kuckartz, U. (2017). Mixed Methods – Stand der Debatte und aktuelle Problemlagen. Kölner Z. Soz. Sozialpsychol. 69(Suppl. 2), 1–37. doi: 10.1007/s11577-017-0450-5
Baur, N., and Knoblauch, H. (2018). Die Interpretativität des Quantitativen, oder: zur Konvergenz von qualitativer und quantitativer empirischer Sozialforschung. Soziologie 47(4), 439–461.
Baur, N., Knoblauch, H., Akremi, L., and Traue, B. (2018). “Qualitativ – Quantitativ – Interpretativ: Zum Verhältnis Methodologischer Paradigmen in der Empirischen Sozialforschung,” in Handbuch Interpretativ forschen, eds L. Akremi, N. Baur, H. Knoblauch, and B. Traue (Weinheim; München: Beltz Juventa), 246–284.
Baur, N., and Lamnek, S. (2017a): “Einzelfallanalyse,” in Qualitative Medienforschung, eds L. Mikos and C. Wegener (Konstanz: UVK), 274–284.
Baur, N., and Lamnek, S. (2017b). “Multivariate analysis,” in The Blackwell Encyclopedia of Sociology, ed G. Ritzer (Oxford: Blackwell Publishing Ltd.). doi: 10.1111/b.9781405124331.2007.x
Behnke, J., Baur, N., and Behnke, N. (2010). Empirische Methoden der Politikwissenschaft. Paderborn: Schöningh.
Charmaz, K. (2006). Constructing Grounded Theory. A Practical Guide through Qualitative Analysis. London: Sage.
Clarke, A. E. (2005). Situational Analysis. Grounded Theory After the Postmodern Turn. Thousand Oaks, CA; London; New Delhi: Sage.
Corbin, J. M., and Strauss, A. (1990). Grounded theory research: procedures, canons, and evaluative criteria. Qual. Sociol. 13(1), 3–21. doi: 10.1007/BF00988593
Desrosières, A. (2002). The Politics of Large Numbers. A History of Statistical Reasoning. Harvard: Harvard University Press.
Edwards, R., Goodwin, J., O'Connor, H., and Phoenix, A. (2017). Working With Paradata, Marginalia and Fieldnotes: The Centrality of By-Products of Social Research. Cheltenham: Edward Elgar. doi: 10.4337/9781784715250
Elias, N. (1987). Engagement und Distanzierung. Arbeiten zur Wissenssoziologie I. Frankfurt a.M.: Suhrkamp.
Elias, N. (1997). Towards a theory of social processes. Br. J. Sociol. 48(3), 355–383. doi: 10.2307/591136
Esping-Andersen, G. (1990). The Three Worlds of Welfare Capitalism. Cambridge; Oxford: Polity and Blackwell.
Flick, U. (1992). Triangulation revisited – strategy of or alternative to validation of qualitative data. J. Theor. Soc. Behav. 2, 175–197. doi: 10.1111/j.1468-5914.1992.tb00215.x
Flick, U. (2017). Mantras and myths: the disenchantment of mixed-methods research and revisiting triangulation as a perspective. Qual. Inq. 23(1), 46–57. doi: 10.1177/1077800416655827
Foster, I., Ghani, R., Jarmin, R. S., Kreuter, F., and Lane, J. (eds.). (2017). Big data and Social Science. A Practical Guide to Methods and Tools. Boca Raton, FL: CRC Press.
Glaser, B. G. (1978). Theoretical Sensitivity: Advances in the Methodology of Grounded Theory. Mill Valley, CA: The Sociology Press.
Groves, R. M., Fowler, F. J., Couper, M., Lepkowski, J. M., Singer, E., and Tourangeau, R. (2009). Survey Methodology. Hoboken, NJ: Wiley.
Hartmann, P., and Lengerer, A. (2019). “Verwaltungsdaten und Daten der amtlichen Statistik,” in Handbuch Methoden der empirischen Sozialforschung, eds N. Baur and J. Blasius (Wiesbaden: Springer), 1223–1232.
Helfferich, C. (2019). “Leitfaden- und Experteninterviews,” in Handbuch Methoden der empirischen Sozialforschung, eds N. Baur and J. Blasius (Wiesbaden: Springer), 669–686. doi: 10.1007/978-3-531-18939-0_39
Hempel, C. G., and Oppenheim, P. (1948): Studies in the logic of explanation. Philos. Sci. 15, 135–175. doi: 10.1086/286983
Hense, A. (2017). Sequentielles Mixed-Methods-Sampling: Wie quantitative Sekundärdaten qualitative Stichprobenpläne und theoretisches Sampling unterstützen können. Kölner Z. Soz. Sozialpsychol. 69(Suppl. 2), 237–259. doi: 10.1007/s11577-017-0459-9
Herbrik, R. (2018). “Hermeneutische Wissenssoziologie (sozialwissenschaftliche Hermeneutik). Das Beispiel der kommunikativen Konstruktion normativer, sozialer Fiktionen, wie z. B. ‘Nachhaltigkeit’ oder: Es könnte auch immer anders sein,” in Handbuch Interpretativ forschen, eds L. Akremi, N. Baur, H. Knoblauch, and B. Traue (Weinheim; München: Beltz Juventa), 659–680.
Johnson, R. B., de Waal, C., Stefurak, T., and Hildebrand, D. L. (2017). Understanding the philosophical positions of classical and neopragmatists for mixed methods research. Kölner Z. Soz. Sozialpsychol. 69(Suppl. 2), 63–86. doi: 10.1007/s11577-017-0452-3
Kalthoff, H. (2008). “Zur Dialektik von qualitativer Forschung und soziologischer Theoriebildung”, Theoretische Empirie, eds H. Kalthoff, S. Hirschauer, and G. Lindemann (Frankfurt a.M.: Suhrkamp), 8–34.
Kelle, U. (2008). Die Integration qualitativer und quantitativer Methoden in der empirischen Sozialforschung. Wiesbaden: Springer.
Kelle, U. (2017). Die Integration qualitativer und quantitativer Forschung – theoretische Grundlagen von ‘Mixed Methods’. Kölner Z. Soz. Sozialpsychol. 69(Suppl. 2), 39–61. doi: 10.1007/s11577-017-0451-4
Kelle, U. (2018). “Datenerhebung in der quantitativen Forschung. Eine interpretative Perspektive auf Fehlerquellen im standardisierten Interview,” in Handbuch Interpretativ forschen, eds L. Akremi, N. Baur, H. Knoblauch, and B. Traue (Weinheim; München: Beltz Juventa), 285–305.
Knoblauch, H., Baur, N., Traue, B., and Akremi, L. (2018). “Was heißt, Interpretativ forschen?” in Handbuch Interpretativ Forschen, eds L. Akremi, N. Baur, H. Knoblauch, and B. Traue (Weinheim; München: Beltz Juventa), 9–35.
Knoblauch, H., and Pfadenhauer, M. (eds.). (2018). Social Constructivism as Paradigm? The Legacy of the Social Construction of Reality. London: Routledge.
Knoblauch, H., and Vollmer, T. (2019). “Ethnografie,” in Handbuch Methoden der empirischen Sozialforschung, eds N. Baur and J. Blasius (Wiesbaden: Springer), 599–618. doi: 10.1007/978-3-658-21308-4_40
König, C., Schröder, J., and Wiegand, E. (2018). Big Data. Chancen, Risiken, Entwicklungstendenzen. Wiesbaden: Springer. doi: 10.1007/978-3-658-20083-1
Koselleck, R. (1977). “Standortbindung und Zeitlichkeit. Ein Beitrag zur historiographischen Erschließung der geschichtlichen Welt,” in Objektivität und Parteilichkeit in der Geschichtswissenschaft, eds R. Koselleck, W.J. Mommsen, and J. Rüsen (München: dtv), 17–46. Reprinted 1979 in Vergangene Zukunft. Zur Semantik geschichtlicher Zeiten, ed R. Koselleck (Frankfurt a.M.: Suhrkamp), 176–207.
Kuckartz, U. (2010). Einführung in die Computergestützte Analyse Qualitativer Daten. Wiesbaden: Springer. doi: 10.1007/978-3-531-92126-6
Kuckartz, U. (2014). Qualitative Text Analysis. A Guide to Methods, Practice and Using Software. London; Thousand Oaks, CA; New Delhi; Singapore: Sage.
Kuckartz, U. (2017). Datenanalyse in der Mixed-Methods-Forschung. Strategien der Integration von qualitativen und quantitativen Daten und Ergebnissen. Kölner Z. Soz. Sozialpsychol. 69(Suppl. 2), 157–183. doi: 10.1007/s11577-017-0456-z
Kuckartz, U. (2018). “Qualitative Inhaltsanalyse. Am Beispiel einer Studie zu Klimabewusstsein und individuellem Verhalten,” in Handbuch Interpretativ forschen, eds L. Akremi, N. Baur, H. Knoblauch, and B. Traue (Weinheim; München: Beltz Juventa), 506–535.
Kuhn, T. S. (1962). The Structure of Scientific Revolutions. Chicago, IL: University of Chicago Press.
Kurt, R., and Herbrik, R. (2019). “Sozialwissenschaftliche Hermeneutik und hermeneutische Wissenssoziologie,” in Handbuch Methoden der empirischen Sozialforschung, eds N. Baur and J. Blasius (Wiesbaden: Springer), 545–564. doi: 10.1007/978-3-658-21308-4_37
Langfeldt, B., and Goltz, E. (2017). Die Funktion qualitativer Vorstudien bei der Entwicklung standardisierter Erhebungsinstrumente. Ein Beispiel aus der Evaluationsforschung in militärischem Kontext. Kölner Z. Soz. Sozialpsychol. 69(Suppl. 2), 313–335. doi: 10.1007/s11577-017-0462-1
Lindemann, G. (2008). “Theoriekonstruktion und empirische Forschung,” in Theoretische Empirie, eds H. Kalthoff, S. Hirschauer, and G. Lindemann (Frankfurt a.M.: Suhrkamp), 165–187.
Maiwald, K.-O. (2018). “Objektive Hermeneutik. Von Keksen, inzestuöser Verführung und dem Problem, die Generationendifferenz zu denken – exemplarische Sequenzanalyse einer Interaktion in einem Fernsehwerbefilm,” in Handbuch Interpretativ Forschen, eds L. Akremi, N. Baur, H. Knoblauch, and B. Traue (Weinheim; München: Beltz Juventa), 442–478.
Mayerl, J., and Faas, T. (2019). “Quantitative Analyse von Twitter und anderer usergenerierter Kommunikation,” in Handbuch Methoden der empirischen Sozialforschung, eds N. Baur and J. Blasius (Wiesbaden: Springer), 1027–1040. doi: 10.1007/978-3-658-21308-4_73
Mayring, P. (2014). Qualitative Content Analysis. Theoretical Foundation, Basic Procedures and Software Solution. Klagenfurt: Beltz.
Merton, R. K. (1942/1973). “The normative structure of science,” in The Sociology of Science: Theoretical and Empirical Investigations, ed R. K. Merton (Chicago, IL: University of Chicago Press), 267–278.
Mignolo, W. (2011). “I am where I think: Remapping the Order of Knowing,” in The Creolization of Theory, eds F. Lionnet and S.-M. Shih (Durham; London: Duke University Press), 159–192. doi: 10.1215/9780822393320-007
Nam, S.-H. (2019). “Qualitative Analyse von Chats und anderer usergenerierter Kommunikation,” in Handbuch Methoden der empirischen Sozialforschung, eds N. Baur and J. Blasius (Wiesbaden: Springer), 1041–1052. doi: 10.1007/978-3-658-21308-4_74
Oevermann, U., Allert, T., Konau, E., and Krambeck, J. (1979): “Die Methodologie einer ‘objektiven Hermeneutik’ und ihre allgemeine forschungslogische Bedeutung in den Sozialwissenschaften,” in Interpretative Verfahren in den Sozial- und Textwissenschaften, ed H.-G. Soeffner (Stuttgart: Metzler), 352–434.
Peirce, C. S. (1878/1931). “Deduction, induction, and hypothesis,” in Collected Papers of Charles Sanders Peirce, Vol. 2, eds C. S. Peirce, C. Hartshorne, and P. Weiss, P. (Cambridge: Belknap Press of Harvard University Press), 619–644.
Popper, K. R. (1935): Logik der Forschung. Zur Erkenntnistheorie der modernen Naturwissenschaft. Wien: Springer. doi: 10.1007/978-3-7091-4177-9
Reichertz, J. (2004b). “Abduction, deduction and induction in qualitative research,” in Companion to Qualitative Research, eds U. Flick et al. (London: Sage), 159–165.
Reichertz, J. (2010). “Abduction: The logic of discovery of Grounded Theory,” in The Sage Handbook of Grounded Theory, eds A. Bryant and K. Charmaz (London: Sage), 214–229.
Reichertz, J. (2013). Die Abduktion in der qualitativen Sozialforschung. Über die Entdeckung des Neuen. Wiesbaden: Springer VS.
Reichertz, J. (2018). “Interpretieren in Interpretationsgruppen. Versprechungen, Formen, Bedingungen, Probleme,” in Handbuch Interpretativ forschen, eds L. Akremi, N. Baur, H. Knoblauch, and B. Traue (Weinheim; München: Beltz Juventa), 72–107.
Reichertz, J. (2019). “Empirische Sozialforschung und soziologische Theorie,” in Handbuch Methoden der empirischen Sozialforschung, eds N. Baur and J. Blasius (Wiesbaden: Springer), 31–48. doi: 10.1007/978-3-658-21308-4_2
Riebling, J. (2017). Methode und Methodologie quantitativer Textanalyse. [dissertation]. Bamberg: Otto-Friedrich Universität Bamberg
Riedl, R. (1985). Die Spaltung des Weltbildes. Biologische Grundlagen des Erklärens und Verstehens. Berlin and Hamburg: Paul Parey.
Salheiser, A. (2019). “Natürliche Daten: Dokumente,” in Handbuch Methoden der empirischen Sozialforschung, eds N. Baur and J. Blasius (Wiesbaden: Springer), 1119–1134. doi: 10.1007/978-3-658-21308-4_80
Schmidt, J.-H. (2019). “Blogs,” in Handbuch Methoden der empirischen Sozialforschung, eds N. Baur and J. Blasius (Wiesbaden: Springer), 1015–1026.
Schmitz, A., and Yanenko, O. (2019). “Web Server Logs und Logfiles,” in Handbuch Methoden der empirischen Sozialforschung, eds N. Baur, and J. Blasius (Wiesbaden: Springer), 991–1000.
Schoonenboom, J., and Johnson, R. B. (2017). How to construct a mixed methods research design. Kölner Z. Soz. Sozialpsychol. 69(Suppl. 2), 107–131. doi: 10.1007/s11577-017-0454-1
Schünzel, A., and Traue, B. (2019). “Websites,” in Handbuch Methoden der empirischen Sozialforschung, eds N. Baur and J. Blasius (Wiesbaden: Springer), 1001–1014. doi: 10.1007/978-3-658-21308-4_71
Shih, S.-M., and Lionnet, F. (2011). “The creolization of theory,” in The Creolization of Theory, eds F. Lionnet and S.-M. Shih (Durham; London: Duke University Press), 1–36.
Smelser, N. J. (1976). Comparative Methods in the Social Sciences. Englewood Cliffs, NJ: Prentice-Hall.
Strauss, A., and Corbin, J. M. (1990). Basics of Qualitative Research: Grounded Theory Procedures and Techniques. Thousand Oaks, CA: Sage.
Strübing, J. (2014). Grounded Theory. Zur sozialtheoretischen und epistemologischen Fundierung eines pragmatistischen Forschungsstils. Wiesbaden: Springer VS.
Strübing, J. (2018). “Situationsanalyse. Eine pragmatistische Erweiterung der Grounded Theory unter dem Eindruck der Postmoderne,” in Handbuch Interpretativ forschen, eds L. Akremi, N. Baur, H. Knoblauch, and B. Traue (Weinheim; München: Beltz Juventa), 681–707.
Strübing, J. (2019). “Grounded theory,” in Handbuch Methoden der empirischen Sozialforschung, eds N. Baur and J. Blasius (Wiesbaden: Springer), 525–544.
Traue, B., and Schünzel, A. (2019). “YouTube und andere Webvideos,” in Handbuch Methoden der empirischen Sozialforschung, eds N. Baur and J. Blasius (Wiesbaden: Springer), 1065–1078.
Uher, J. (2018). Data generation methods across the empirical sciences: Differences in the study phenomena's accessibility and the processes of data encoding. Qual. Quant. Int. J. Methodol. 53(1), 221–246. doi: 10.1007/s11135-018-0744-3
Vogl, S. (2017). Quantifizierung. Datentransformation von qualitativen Daten in quantitative Daten in Mixed-Methods-Studien. Kölner Z. Soz. Sozialpsychol. 69(Suppl. 2), 287–312. doi: 10.1007/s11577-017-0461-2
von Glasersfeld, E. (1994). “Einführung in den radikalen Konstruktivismus,” in Die erfundene Wirklichkeit, ed P. Watzlawick (München: Piper), 16–38.
Wehler, H.-U. (1980). Historische Sozialwissenschaft und Geschichtsschreibung. Göttingen: Vandenhoeck and Ruprecht.
Weil, S., Eberle, T. S., and Flick, U. (2008). Between Reflexivity and Consolidation—Qualitative Research in the Mirror of Handbooks. Forum Qual. Soc. Res. 9(3). Available online at: http://nbn-resolving.de/urn:nbn:de:0114-fqs0803280
Weiß, B., and Wagner, M. (2019). “Meta-analyse,” in Handbuch Methoden der empirischen Sozialforschung, eds N. Baur and J. Blasius (Wiesbaden: Springer), 1511–1520.
Ziegler, M. (2018). “Interpretativität und schließende Statistik. Das Verhältnis von sozialem Kontext und Generalisierungsstrategien der quantitativen Sozialforschung,” in Handbuch Interpretativ forschen, eds L. Akremi, N. Baur, H. Knoblauch, and B. Traue (Weinheim; München: Beltz Juventa), 409–441.
Keywords: research process, mixed methods, survey research, big data, qualitative content analysis, grounded theory, social-science hermeneutics, objectivity
Citation: Baur N (2019) Linearity vs. Circularity? On Some Common Misconceptions on the Differences in the Research Process in Qualitative and Quantitative Research. Front. Educ. 4:53. doi: 10.3389/feduc.2019.00053
Received: 30 June 2018; Accepted: 22 May 2019;
Published: 25 June 2019.
Edited by:
Douglas F. Kauffman, Medical University of the Americas–Nevis, United StatesReviewed by:
Jana Uher, University of Greenwich, United KingdomCopyright © 2019 Baur. This is an open-access article distributed under the terms of the Creative Commons Attribution License (CC BY). The use, distribution or reproduction in other forums is permitted, provided the original author(s) and the copyright owner(s) are credited and that the original publication in this journal is cited, in accordance with accepted academic practice. No use, distribution or reproduction is permitted which does not comply with these terms.
*Correspondence: Nina Baur, bmluYS5iYXVyQHR1LWJlcmxpbi5kZQ==
Disclaimer: All claims expressed in this article are solely those of the authors and do not necessarily represent those of their affiliated organizations, or those of the publisher, the editors and the reviewers. Any product that may be evaluated in this article or claim that may be made by its manufacturer is not guaranteed or endorsed by the publisher.
Research integrity at Frontiers
Learn more about the work of our research integrity team to safeguard the quality of each article we publish.