- 1Department of Psychology, Education and Child Studies, Erasmus University Rotterdam, Rotterdam, Netherlands
- 2Learning and Innovation Center, Avans University of Applied Sciences, Breda, Netherlands
- 3Department of Education, Utrecht University, Utrecht, Netherlands
Critical thinking is considered to be an important competence for students and graduates of higher education. Yet, it is largely unclear which teaching methods are most effective in supporting the acquisition of critical thinking skills, especially regarding one important aspect of critical thinking: avoiding biased reasoning. The present study examined whether creating desirable difficulties in instruction by prompting students to generate explanations of a problem-solution to themselves (i.e., self-explaining) is effective for fostering learning and transfer of unbiased reasoning. Seventy-nine first-year students of a Dutch Applied University of Sciences were first instructed on two categories of “heuristics and biases” tasks (syllogism and base-rate or Wason and conjunction). Thereafter, they practiced these either with (self-explaining condition) or without (no self-explaining condition) self-explanation prompts that asked them to motivate their answers. Performance was measured on a pretest, immediate posttest, and delayed (2 weeks later) posttest on all four task categories, to examine effects on learning (performance on practiced tasks) and transfer (performance on non-practiced tasks). Participants' learning and transfer performance improved to a comparable degree from pretest to immediate posttest in both conditions, and this higher level of performance was retained on the delayed posttest. Surprisingly, self-explanation prompts had a negative effect on posttest performance on practiced tasks when those were Wason and conjunction tasks, and self-explaining had no effect on transfer performance. These findings suggest that the benefits of explicit instruction and practice on learning and transfer of unbiased reasoning cannot be enhanced by increasing the difficulty of the practice tasks through self-explaining.
Introduction
Fostering students' critical thinking (CT) skills is an important educational objective, as these skills are essential for effective communication, reasoning and problem-solving abilities, and participation in a democratic society (Billings and Roberts, 2014). Therefore, it is alarming that many higher education students find it hard to think critically; their level of CT is often too low (Flores et al., 2012) and CT-skills do not seem to improve over their college years (e.g., Arum and Roksa, 2011). As early as 1910, John Dewey described the importance of critique and stated that everyone needs to engage in CT. A variety of CT definitions has been suggested since then, the most accepted definition in the field of educational assessment and instruction of which has been proposed by an expert Delphi Panel of the American Philosophical Association (APA; Facione, 1990). They characterized CT as “purposeful, self-regulatory judgment that results in interpretation, analysis, evaluation, and inference, as well as explanation of the evidential, conceptual, methodological, criteriological, or contextual considerations on which that judgment is based” (Facione, 1990, p. 2). Despite the variety of definitions of CT and the multitude of components, there appears to be agreement that one key aspect of CT is the ability to avoid biases in reasoning and decision-making (West et al., 2008), which we will refer to as unbiased reasoning from hereon. Bias is said to occur when a reasoning process results in a systematic deviation from a norm when choosing actions or estimating probabilities (Tversky and Kahneman, 1974; Stanovich et al., 2016). As biased reasoning can have serious consequences in situations in both daily life and the complex professional environments (e.g., economics, law, and medicine) in which the majority of higher education graduates end up working, it is essential to teach unbiased reasoning in higher education (e.g., Koehler et al., 2002; Rachlinski, 2004). However, it is still largely unclear how unbiased reasoning can be best taught, and especially how transfer can be fostered; that is, the ability to apply acquired knowledge and skills to new situations (e.g., Davies, 2013).
In line with findings of research on teaching CT in general (e.g., Abrami et al., 2014), previous research on unbiased reasoning has shown that providing students with explicit instructions and giving them the opportunity to practice what has been learned, improves performance on the learned tasks, but not transfer (e.g., Heijltjes et al., 2014b). This lack of transfer is a problem, as it is important that students can apply what has been learned to other situations. According to the desirable difficulties framework (e.g., Bjork, 1994; Bjork and Bjork, 2011; Soderstrom and Bjork, 2015; Fyfe and Rittle-Johnson, 2017), long-term performance and transfer can be enhanced by techniques that are effortful during learning and may seem to temporarily hold back performance gains. Conditions that support rapid improvement of performance (i.e., retrieval strength) often only support momentary performance gains and do not contribute to permanent changes needed for learning (Bjork and Bjork, 2011). To enhance long-term retention and transfer of learned skills, storage strength should be increased by effortful learning conditions that trigger deep processing (Yan et al., 2016). The active and deeper processing produced by encountering desirable difficulties can promote transfer to new situations (cf. germane load; Soderstrom and Bjork, 2015). If, however, the difficulties evoke learners to invest additional effort on processes that are not directly relevant for learning or the learners miss the relevant knowledge or skills to successfully deal with them, they become undesirable (McDaniel and Butler, 2010; Metcalfe, 2011).
Although conditions inducing the most immediate and observable signs of performance improvements are often preferred by both teachers and learners because they appear to be effective, it is important for teachers and students alike to search for conditions that confront students with desirable difficulties and thereby facilitate learning and transfer (Bjork et al., 2015). Such conditions include, for example, spacing learning sessions apart rather than massing them together (i.e., spacing effect), mixing practice-task categories rather than practicing one task-category before the next (i.e., interleaving effect), and testing learning material rather than simply restudying it (i.e., testing effect; e.g., Weissgerber et al., 2018). Another desirable difficulty is the active generation of an answer, solution, or procedure rather than the mere passive reception of it (i.e., generation effect; for a review see Bertsch et al., 2007). Generative processing of learning materials requires learners to invest additional effort on the learning processes and to be actively involved in these processes, such as encoding and retrieval processes (Yan et al., 2016). Therefore, generative learning activities contribute to the connection and entrenchment of new information from the to-be-learned materials to existing knowledge. As a result, understanding of the materials is stimulated and is more likely to be recallable at a later time or in a different context (Slamecka and Graf, 1978; DeWinstanley and Bjork, 2004; Bjork and Bjork, 2011; Fiorella and Mayer, 2016; McCurdy et al., 2017).
One promising strategy to promote generative learning, and thus to create desirable difficulty in instruction, is self-explaining (e.g., Fiorella and Mayer, 2016). Self-explaining involves the generation of explanations of a problem-solution to oneself rather than simply answering tasks passively. Indeed self-explaining has been shown to foster knowledge acquisition and to promote transfer in a variety of other domains (Lombrozo, 2006; Dunlosky et al., 2013; Wylie and Chi, 2014; Fiorella and Mayer, 2016; Rittle-Johnson and Loehr, 2017; for reviews see Bisra et al., 2018), but the effectivity in CT-instruction is not yet clear. Self-explaining is assumed to lead to the construction of meaningful knowledge structures (i.e., mindware), by investing effort in identifying knowledge gaps or faulty mental models and connecting new information to prior knowledge (e.g., Chi, 2000; Atkinson et al., 2003; Fiorella and Mayer, 2016), and seems especially effective in domains guided by general underlying principles (Rittle-Johnson and Loehr, 2017). Moreover, self-explaining might stimulate students to stop and think about new problem-solving strategies (Siegler, 2002) with engagement in more analytical and reflective reasoning, labeled as Type 2 processing, as a result. This type of processing is required to avoid biases in reasoning and decision-making. Biases often result from relying on Type 1 processing to solve problems, which is a relatively effortless, automatic, and intuitive type of processing. Although Type 1 processing may lead to efficient decision-making in many routine situations, it may open the door to errors that could have been prevented by engaging in Type 2 processing (e.g., Evans, 2008; Stanovich, 2011). As such, self-explaining might contribute to decoupling prior beliefs from available evidence, which is an essential aspect of unbiased reasoning. It is important to bear in mind, however, that the benefit of self-explaining only applies when students are able to provide self-explanations of sufficient quality (Schworm and Renkl, 2007).
Several studies demonstrated that prompting self-explaining fostered learning and/or transfer of certain aspects of CT-skills, such as argumentation (e.g., Schworm and Renkl, 2007), complex judgments (e.g., Helsdingen et al., 2011), or logical reasoning (e.g., Berry, 1983). Studies on the effect of self-explanation prompts on unbiased reasoning (Heijltjes et al., 2014a,b, 2015), however, showed mixed findings. One study found an effect on transfer performance on an immediate posttest (Heijltjes et al., 2014b), but this effect was short-lived (i.e., not retained on a delayed posttest) and not replicated in other studies (Heijltjes et al., 2014a, 2015). This lack of (prolonged) effects of self-explaining might have been due to the nature of the final tests, which were multiple-choice (MC) answers only. A study in which students had to motivate their MC-answers suggests that this might provide a better, more sensitive measure of the effects of self-explaining on transfer of unbiased reasoning (Hoogerheide et al., 2014). Therefore, the present study used MC-plus-motivation tests to investigate whether self-explaining is effective for fostering learning and transfer of unbiased reasoning.
Since it seems reasonable to assume, but is as yet unproven, that increasing the desirable difficulty of learning materials through self-explaining might foster learning and transfer of unbiased reasoning, the present study was conducted as part of an existing critical thinking course (i.e., classroom study) to examine the usefulness of this desirable difficulty in a real educational setting. We investigated the effects of self-explaining during practice with “heuristics and biases tasks” (e.g., Tversky and Kahneman, 1974) on learning and transfer, as assessed by final test tasks which required students to motivate their MC-answers. Based on the literature reviewed above, we hypothesized that explicit CT-instructions combined with practice on domain-specific cases would be effective for learning: therefore, we expect performance gains on practiced tasks from pretest to posttest as measured by MC-answers (Hypothesis 1). The more interesting question, however, is whether self-explaining during practice would lead to higher performance gains on practiced (i.e., learning; Hypothesis 2a) and non-practiced tasks (i.e., transfer; Hypothesis 2b) than not being prompted to self-explain during practice. As outlined before, we expect that beneficial effects of self-explaining on performance outcomes are more likely to be detected when participants are required to motivate their answer to MC-items. We hypothesized that self-explaining during practice would lead to higher total posttest scores (i.e., MC-plus-motivation) on practiced (i.e., learning; Hypothesis 3a) and non-practiced tasks (i.e., transfer; Hypothesis 3b). We expected this pattern of results to persist on the delayed posttest.
Furthermore, we explored perceived mental effort investment in the test items to get more insight into the effects of self-explaining on learning (Question 4a) and transfer performance (Question 4b). On the one hand, it can be expected that the acquisition of knowledge of rules and strategies would lower the cognitive load imposed by the task, and therefore participants might have to invest less mental effort on the posttests than on the pretest (Paas et al., 2003), especially after having engaged in self-explaining. On the other hand, as both our training-phase and the self-explanation prompts were designed to provoke Type 2 processing—which is more effortful than Type 1 processing (Evans, 2011)—participants might have been inclined to invest more effort on the posttests than on the pretest, especially on the non-practiced (i.e., transfer) tasks, on which participants had not acquired any knowledge during instruction. Finally, because the quality of self-explanations has been shown to be related to learning and transfer, we explored whether the quality of the self-explanations on the practice tasks correlated with the immediate and delayed posttest performance (Question 5).
Materials and Methods
We created an Open Science Framework (OSF) page for this project, where all materials, a detailed description of the procedure, and the dataset of the experiment are provided (osf.io/85ce9).
Participants and Design
Participants were all first-year “Safety and Security Management” students of a Dutch University of Applied Sciences (N = 88). Five participants missed the second session and four participants failed to complete the experiment due to technical problems. Therefore, the final sample consisted of 79 students (Mage = 19.16, SD = 1.61; 44 males). Because this study took place in a real educational setting and was part of an existing course, our sample was limited to the total number of students in this cohort. In response to a reviewer, we added a power function of our analyses using the G*Power software (Faul et al., 2009). The power of our 3 × 2 × 2 Mixed ANOVAs—under a fixed alpha level of 0.05, with a correlation between measures of 0.3, and with a sample size of 79—is estimated at 0.36, 0.99, and >0.99 for picking up a small, medium, and large interaction effect, respectively. Regarding our 2 × 2 × 2 Mixed ANOVAs, the power is estimated at 0.32, 0.96, and >0.99 for picking up a small, medium, and large interaction effect, respectively. The power of our study, thus, should be sufficient to pick up medium-sized effects, which is in line with the mean weighted medium effect size of self-explaining of previous studies as indicated in a recent meta-analysis (Bisra et al., 2018).
The experiment consisted of four phases: pretest, training-phase (CT-instructions plus practice), immediate posttest, and delayed posttest (see Table 1 for an overview). Participants were randomly assigned to one of two conditions: (1) Self-explaining condition (CT-instructions and CT-practice with self-explanation prompts; n = 39) and (2) No self-explaining condition (CT-instructions and CT-practice without self-explanation prompts; n = 40). Of the four task categories tested in the pretest and posttests participants received instruction and practice on two task categories (one involving statistical and one involving logical reasoning, see section CT-skills tests). To ensure that any condition effects would not be due to specific characteristics of the instructed and practiced tasks, half of the participants in each condition got instruction and practice on the first logical and the first probabilistic reasoning task category (i.e., syllogism and base-rate), and the other half on the second logical and the second probabilistic reasoning task category (i.e., Wason and conjunction).
Materials
CT-Skills Tests
The pretest consisted of eight classic heuristics and biases tasks that reflected important aspects of CT across four categories (i.e., two of each category): (1) Syllogistic Reasoning tasks, which examine the tendency to be influenced by the believability of a conclusion when evaluating the logical validity of arguments (adapted from Evans, 2003); (2) Wason Selection tasks, that measure the tendency to verify rules rather than to falsify them (adapted from Stanovich, 2011); (3) Base-rate tasks, which measure the tendency to overrate individual-case evidence (e.g., from personal experience, a single case, or prior beliefs) and to underrate statistical information (Tversky and Kahneman, 1974; adapted from Fong et al., 1986); and (4) Conjunction tasks, that measure to what extent people neglect a fundamental rule in probability theory, that is, the conjunction rule [P(A&B) ≤ P(B)] which states that the probability of Event A and Event B both occurring must be lower than the probability of Event A or Event B occurring alone (adapted from Tversky and Kahneman, 1983). The syllogistic reasoning and Wason selection tasks involve logical reasoning (i.e., Wason selection tasks can be solved by applying modus ponens and modus tollens from syllogistic reasoning) and the base-rate and conjunction tasks involve statistical reasoning (i.e., both require knowledge of probability and data interpretation).
The content of the surface features (cover stories) of all test items was adapted to the study domain of the participants. A multiple-choice format with four answer options was used, with only one correct answer, except for one base-rate task where two answers were correct.
The immediate and delayed posttests were parallel versions of the pretest (i.e., structurally equivalent tasks but with different surface features). During the posttests, participants were additionally asked to motivate their MC-answers (“Why is this answer correct? Explain in steps how you have come to this answer.”) by typing their motivation in a text entry box below the MC-question. The posttest items on the practiced task categories served to assess differences in learning outcomes, whereas the posttest items on the non-practiced task categories served to assess transfer performance. The transfer task categories shared similar features with the learning categories, namely, one requiring knowledge and rules of logic (i.e., syllogisms rules) and one requiring knowledge and rules of statistics (i.e., probability and data interpretation).
CT-Instructions
The text-based CT-instructions consisted of a general instruction on deductive and inductive reasoning and explicit instructions on two of the four categories from the pretest, including two extensive worked examples (of the tasks seen in the pretest) of each category. Participants received the following hints stating that the principles used in these tasks can be applied at several reasoning tasks: “Remember that these reasoning schemes can be applied in several reasoning tasks” and “Remember that the correct calculation of probabilities is an important skill that can be applied in several reasoning tasks.”
CT-Practice
The CT-practice phase consisted of a case (315 words text)—on a topic that participants might encounter in their working-life—and four practice problems, two of each of the two task categories that students were given instructions on. In the self-explanation condition, participants were exposed to a self-explanation prompt after each of these tasks in which they were asked to explain how the answer was obtained: “Why is this answer correct? Explain in steps how you have come to this answer.”
Mental Effort
After each test item participants reported how much mental effort they invested in completing that item, on a 9-point rating scale ranging from (1) very, very low effort to (9) very, very high effort (Paas, 1992; Paas and Van Merriënboer, 1993).
Procedure
The study was run during the first two lessons of a CT-course in the Safety and Security Management study program of an institute of higher professional education and conducted in the classroom with an entire class of students present. Participants signed an informed consent form at the start of the experiment. All materials were delivered in a computer-based environment (Qualtrics platform) that was created for this experiment, except for the paper-based case during the CT-instructions. The Qualtrics program randomly assigned the participants to a condition/version. Participants could work at their own pace, were allowed to use scrap paper while solving the tasks, and time-on-task was logged during all phases.
The study consisted of two sessions. In session 1 (during the first lesson of the course, ca. 90 min.), participants first completed the pretest. Subsequently, they had to read the CT-instructions and the case, followed by the practice problems, which differed according to the assigned condition/version. At the end, participants completed the immediate posttest. After 2 weeks, session 2 (during the second lesson of the course, ca. 30 min.) was held in which participants completed the delayed posttest. Invested mental effort was rated after each test item on all CT-skills tests. Both the teacher and the experiment leader (first author of this paper) were present during all phases of the experiment.
Scoring
For selecting a correct MC-answer on the three CT-skills tests, 1 point was assigned, resulting in a maximum MC-score of four points on the learning (i.e., instructed/practiced task categories) items and four points on the transfer (i.e., task categories not instructed/practiced) items on each test. On the immediate and delayed posttest, participants were additionally asked to motivate their MC-answers. These motivations were scored based on a coding scheme that can be found on our OSF page. In addition to the MC-score (1 point), participants could earn a maximum of two points per question for the given motivation, resulting in a maximum total score (MC-plus-motivation) of three points per item. Because one syllogism task had to be removed from the tests due to an inconsistent variant in the delayed posttest (i.e., relatively easier form), participants who received instructions on the syllogistic reasoning and base-rate tasks, could attain a maximum total score of nine on the learning items and 12 on the transfer items on each posttest; and vice versa for the participants who received instructions on the Wason and conjunction tasks. For comparability, we computed percentage scores on the learning and transfer items instead of total scores. Two raters independently scored 25% of the immediate posttest. The intra-class correlation coefficient was 0.952 for the learning test items and 0.971 for the transfer test items. Because of these high inter-rater reliabilities the remainder of the tests was scored by one rater.
The quality of participants' explanations was determined on the basis of the self-explanations given during the practice tasks with a maximum of two points per task (cf. posttest explanation-scoring procedure). As there were four practice tasks, the maximum self-explanation score was eight (ranging from 0 to 8). Two raters independently scored 25% of the tasks. Because the inter-rater reliability was high (intra-class correlation coefficient of 0.899), the remainder of the tasks was scored by one rater.
Results
For all analyses in this paper a p-value of 0.05 was used as a threshold for statistical significance. Partial eta-squared () is reported as a measure of effect size for the ANOVAs, for which 0.01 is considered small, 0.06 medium, and 0.14 large, and Cohen's d is reported for the post-hoc tests, with values of 0.20, 0.50, and 0.80 representing a small, medium, and large effect size respectively (Cohen, 1988).
Preliminary analyses confirmed that there were no significant differences between the conditions before the start of the experiment in educational background, χ2(3) = 2.41, p = 0.493, gender, χ2(1) = 0.16, p = 0.900, or performance, time-on-task, and mental effort on the pretest (all Fs < 1, maximum ηp2 = 0.011). An independent-samples t-test indicated—surprisingly—that there were no significant differences in time-on-task (in seconds) spent on practice of the instruction tasks between the self-explaining condition (M = 409.25, SD = 273.45) and the no self-explaining condition (M = 404.89, SD = 267.13), t(77) = 0.07, p = 0.943, d = 0.016.
Test performance
Data are provided in Table 2 and test statistics in Table 3. Regarding the version of the instruction, only main effects of Version or interactions of Version with other factors are reported. The remaining results are provided in Table 3.
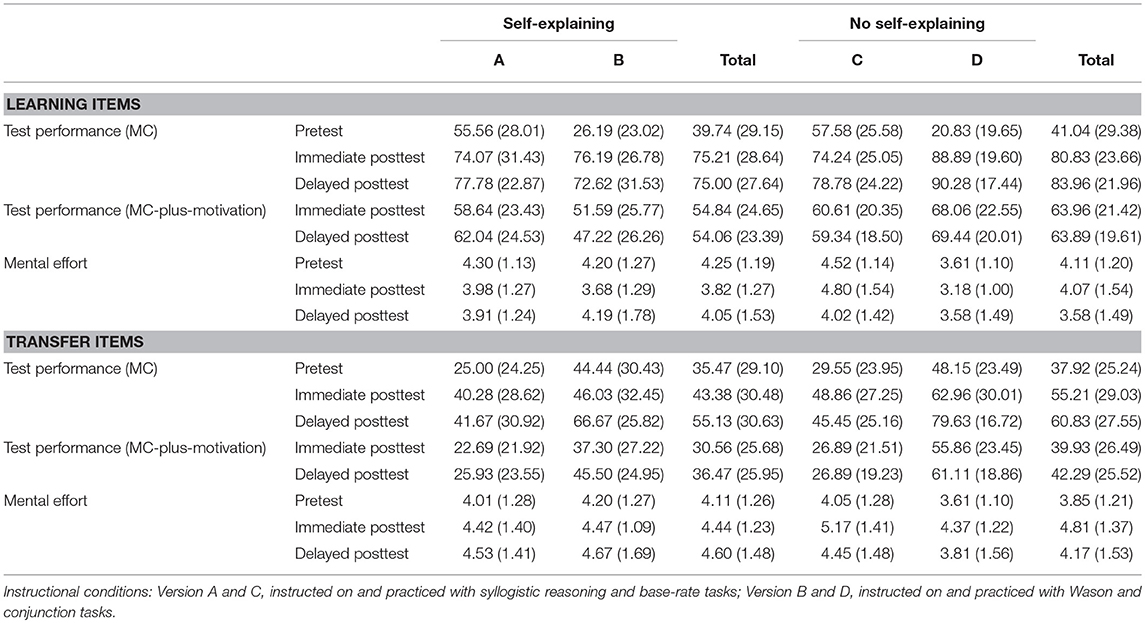
Table 2. Means (SD) of Test performance (multiple-choice % score), Test performance (multiple-choice plus motivation % score), and Mental effort (1–9) per Condition and Version.
Performance Gains on MC-Answers
To test hypotheses 1, 2a, and 2b, two 3 × 2 × 2 Mixed ANOVAs were conducted with Test Moment (pretest, immediate posttest, and delayed posttest) as within-subjects factor and Self-explaining (self-explaining and no self-explaining) and Version (syllogism and base-rate: SB, and Wason selection and conjunction: WC) as between-subjects factors.
Test Moment significantly affected learning (i.e., performance on practiced tasks): performance was lower on the pretest (M = 40.40, SD = 29.09) than on the immediate posttest (M = 78.06, SD = 26.22), p < 0.001, ηp2 = 0.647. Performance on the immediate posttest did not differ significantly from that on the delayed posttest (M = 79.54, SD = 25.17), p = 0.611, ηp2 = 0.003. Note though, that there was an interaction between Test Moment and Version; participants who received the SB-version showed an immediate to delayed posttest performance gain (Mimmediate = 74.16; Mdelayed = 78.28), whereas the WC-version showed a slight performance drop (Mimmediate = 82.54; Mdelayed = 81.45); however, follow-up tests showed that the gain/drop were non-significant, F(1, 38) = 13.12, p = 0.001, ηp2 = 0.257; F(1, 37) = 0.07, p = 0.794, ηp2 = 0.002. There was no main effect of Self-explaining nor an interaction between Test Moment and Self-explaining, indicating that prompting self-explanations did not affect learning gains.
There was a main effect of Test Moment on test performance on transfer (i.e., non-practiced) items. Performance was lower on the pretest (M = 36.71, SD = 27.07) than on the immediate posttest (M = 49.37, SD = 30.16), p < 0.001, = 0.169, which in turn was lower than on the delayed posttest (M = 58.02, SD = 29.07), p = 0.004, = 0.108. There was a main effect of Version: receiving the WC-version resulted in higher transfer performance (M = 57.98, SE = 3.46) than the SB-version (M = 38.47, SE = 3.42), indicating that transfer from WC-tasks to SB-tasks was higher than from SB-tasks to WC-tasks. Moreover, there was an interaction between Test Moment and Version. Follow-up analyses showed an effect of Test Moment for both the SB-version, F(2, 76) = 10.74, p < 0.001, , and the WC-version, F(2, 74) = 16.58, p < 0.001, ηp2 = 0.309. The pretest to immediate posttest performance gain was only significant for the SB-version, F(1, 38) = 16.32, p = 0.001, = 0.300, whereas the immediate to delayed posttest performance gain was only significant for the WC-version, F(1, 37) = 17.64, p < 0.001, ηp2 = 0.323. There was no main effect of Self-explaining nor a significant interaction between Test Moment and Self-explaining, indicating that prompting self-explanations did not affect transfer performance.
Effects of Self-Explaining on Learning Outcomes (MC-Plus-Motivation)
To test hypothesis 3a, we analyzed the data of the MC-plus-motivation scores on learning items using a 2 × 2 × 2 Mixed ANOVA with Test Moment (immediate posttest and delayed posttest) as within-subjects factor and Self-explaining (self-explaining and no self-explaining) and Version (syllogism and base-rate: SB, and Wason selection and conjunction: WC) as between-subjects factors (see Tables 2, 3 for data and test statistics, respectively). Pretest scores were not included in this analysis because the pretest only consisted of MC-questions. There was no main effect of Test Moment. Self-explaining significantly affected performance on learning items. Surprisingly, performance was higher in the no self-explaining condition (M = 64.36, SE = 3.26), compared to the self-explaining condition (M = 54.87, SE = 3.30). Note though, that there was an interaction between Self-explaining and Version. The effect of self-explaining was only found for the WC-version, F(1, 37) = 7.66, p = 0.009, ηp2 = 0.172; there was no main effect of self-explaining for the SB-version, F(1, 38) = 0.01, p = 0.953, ηp2 = 0.000. We did not found an interaction between Test Moment and Self-explaining.
Effects of Self-Explaining on Transfer Performance (MC-Plus-Motivation)
To test hypothesis 3b, we analyzed the data of the MC-plus-motivation scores on the transfer items using a 2 × 2 × 2 Mixed ANOVA with Test Moment (immediate posttest and delayed posttest) as within-subjects factor and Self-explaining (self-explaining and no self-explaining) and Version (syllogism and base-rate: SB, and Wason selection and conjunction: WC) as between-subjects factors (see Tables 2, 3 for data and test statistics, respectively). There were no main effects of Test Moment and Self-explaining nor an interaction between Test Moment and Self-explaining. Collectively, the results on the transfer items again suggest that transfer occurred to a comparable extent in the self-explaining condition and the no self-explaining condition. Note though, that there was a main effect of version of instruction. In line with the findings on the MC-scores data, performance was higher for the WC-version (M = 49.95, SE = 3.31) than the SB-version (M = 25.60, SE = 3.27), indicating that transfer was higher when instructed/practiced with the WC-tasks compared to the SB-tasks.
Mental Effort Investment
Again, data are provided in Table 2 and test statistics in Table 3. We exploratively analyzed the mental effort data (average mental effort invested per learning item) using two 3 × 2 × 2 Mixed ANOVAs with Test Moment (pretest, immediate posttest, and delayed posttest) as within-subjects factor and Self-explaining (self-explaining and no self-explaining) and Version (syllogism and base-rate: SB, and Wason selection and conjunction: WC) as between-subjects factors (Question 4a and 4b). Regarding the version of the instruction, only main effects of Version or interactions of Version with other factors are reported. The remaining results are available in Table 3. One participant had more than two missing values and was removed from the analysis.
There were no main effects of Test Moment or Self-explaining on effort invested in learning items, nor an interaction between Test Moment and Self-explaining. Note tough, that there was a main effect of version of instruction. Less effort investment on learning items was reported for the WC-version (M = 3.65, SE = 0.17) than the SB-version (M = 4.52, SE = 0.17). Moreover, there was an interaction between Self-explaining and Version. The effect of self-explaining was only found for the WC-version, F(1, 36) = 5.08, p = 0.030, ηp2 = 0.124; there was no main effect of self-explaining for the SB-version, F(1, 38) = 1.26, p = 0.268, = 0.032.
Regarding effort invested in transfer items, Mauchly's test indicated that the assumption of sphericity had been violated, χ = 7.45, p = 0.024, and therefore Huynh-Feldt corrected tests are reported (ε = 0.95). Mental effort was affected by Test Moment. Invested mental effort was lower on the pretest (M = 3.98, SE = 0.14) compared to the immediate posttest (M = 4.63, SE = 0.15), p < 0.001, ηp2 = 0.208, which did not differ from that on the delayed posttest (M = 4.38, SE = 0.17), p = 0.160, ηp2 = 0.026. There was no main effect of Self-explaining nor an interaction between Test Moment and Self-explaining.
Quality of Self-Explanations
Several authors have reported that self-explanations are only beneficial when the quality of the explanations is sufficient (e.g., Schworm and Renkl, 2007). To examine whether we could corroborate this finding, we conducted an exploratory analysis. Based on the quality of the self-explanations in the instruction tasks, we created three groups: (1) highest self-explanation scores (score ≥ 4; 25% of the total group), (2) scores between 2 and 3 (42% of the total group), and 3) lowest self-explanation scores (score ≤ 1; 33% of the total group). We examined whether the quality of the self-explanations was related to performance on the learning (practiced) items by conducting a Mixed ANOVA (on participants in the self-explanation condition) with Test Moment (immediate posttest and delayed posttest) as within-subjects factor and Quality of Self-explanations (high, medium, and low) as between-subjects factor. There was no main effect of Test Moment, F(1, 36) = 0.02, p = 0.881, ηp2 = 0.001, but there was a main effect of Quality of Self-explanations, F(2, 36) = 8.79, p = 0.001, ηp2 = 0.328. The group with the lowest self-explanation scores performed lower on learning items (M = 36.86, SE = 5.38) than the group with the medium self-explanation scores (M = 59.55, SE = 4.85), p < 0.001. The group with the medium self-explanation scores did not differ from the group with the highest self-explanation scores (M = 69.17, SE = 6.13), p = 0.226. No interaction between Test Moment and Quality of Self-explanations was found, F(2, 36) = 1.26, p = 0.297, ηp2 = 0.056.
A similar mixed ANOVA was conducted to explore whether the quality of the self-explanations was related to performance on the transfer (non-practiced) items. There was no main effect of Test Moment, F(1, 36) = 2.73, p = 0.107, ηp2 = 0.070, no main effect of Quality of Self-explanations, F(2, 36) = 0.01, p = 0.994, ηp2 = 0.000, nor an interaction between Test Moment and Quality of Self-explanations, F(2, 36) = 0.61, p = 0.550, ηp2 = 0.033.
Discussion
Previous research has shown that creating desirable difficulty in instruction by having learners generate explanations of a problem-solution to themselves (i.e., self-explaining) rather than simply answering tasks passively, is effective to foster learning and transfer in several domains (Fiorella and Mayer, 2016). Regarding unbiased reasoning, Heijltjes et al. (2014b) demonstrated that self-explaining during practice had a positive effect on transfer of unbiased reasoning, but this effect was short-lived and not replicated in other studies (Heijltjes et al., 2014a, 2015). However, these findings were based on MC-answers only, and there are indications that effects of self-explaining on transfer may be detected when more sensitive MC-plus-motivation tests are used (Hoogerheide et al., 2014). With the present experiment, we aimed to find out whether instruction followed by self-explaining during practice with heuristics and biases tasks would be effective for learning and transfer, using final tests that required participants to motivate their MC-answers.
Consistent with earlier research, our results corroborate the idea that explicit CT-instruction combined with practice is beneficial for learning to avoid biased reasoning (Hypothesis 1), as we found pretest to immediate posttest gains on practiced tasks, remaining stable on the delayed posttest after 2 weeks, as measured by performance on the MC-only questions. This is in line with the notion that the acquisition of relevant mindware contributes to an adequate use of Type 2 processing which can prevent biased reasoning (Stanovich et al., 2008). Contrary to earlier findings (e.g., Heijltjes et al., 2014b), our experiment seemed to provide some evidence that these instructions and practice tasks may also enhance transfer. However, this only applied to participants who practiced with the syllogism and base-rate version. For participants who received the other version, transfer performance gains were reached at a later stage. As such, this may mean that either transfer was easier from syllogism and base-rate to Wason and conjunction or, given that this pattern is not consistent across analyses, that our findings may reflect non-systematic variance. Another reason why caution is warranted in interpreting this finding is that the maximum scores differed per version, which—even though we used percentage scores—might be an issue for comparability.
As for our main question, we did not find any indications that prompting self-explanations to increase the difficulty of the practice tasks had a differential effect—compared to the control condition—on learning (Hypothesis 2a) or transfer (Hypothesis 2b) performance gains. Nor did the analyses of the MC-plus-motivation data show a benefit of prompting self-explanations during practice for learning (Hypothesis 3a) or transfer (Hypothesis 3b). Surprisingly, our findings even suggest that self-explaining during practice may actually be less beneficial for learning: participants who received self-explanation prompts benefitted less from the instructions than those who were not prompted; however, this was only the case for one of the versions, so again, this finding needs to be interpreted with caution.
The findings of the present study are contrary to previous studies that demonstrated that self-explaining is effective for establishing both learning and transfer in a variety of domains (for a review see Fiorella and Mayer, 2016), but they are in line with the studies on unbiased reasoning (which assessed performance only by means of MC-answers) that demonstrated no positive effects (Heijltjes et al., 2014a, 2015) or only a short-lived effect of self-explaining on transfer (Heijltjes et al., 2014b). We did find that learners who gave lower quality self-explanations also performed worse on the learning items on the test (Question 5), which seems to corroborate the idea that a higher quality of self-explanations is related to higher performance (Schworm and Renkl, 2007), but it is possible that this finding reflects a priori knowledge or ability difference rather than an effect of the quality of self-explanations on performance. Thus, this study (with a more extensive performance measure) contributes to a small body of evidence that self-explanation prompts seem to have little or no benefit for acquiring unbiased reasoning skills.
One possible reason for the lack of a self-explanation effect could be the fact that the learners did not receive feedback on their self-explanations given in the practice phase. Providing feedback after students' self-explanations could have contributed to consolidating correct explanations and correcting or elaborating incorrect or incomplete explanations (e.g., Hattie and Timperley, 2007), which is of great importance in the domain of unbiased reasoning—arguably even more so than in other learning domains.
Another possibility might be that the nature of the tasks moderates effects of self-explaining. Contrary to previous studies, transfer on the tasks in the present study relies not only on deep understanding of the domain-specific knowledge involved in the task, but also on the ability to inhibit Type 1 processing and to switch to Type 2 processing. Possibly, prompting students to self-explain did not provoke the “stop and think” reaction that was needed for transfer above and beyond what the instructions already accomplished. Our findings regarding effort investment support this idea (i.e., higher effort investment on transfer items on the posttests compared to the pretest in both conditions), suggesting that our training-phase provoked Type 2 processing, but there was no (additional) effect of the self-explanation prompts on effort investment.
A strength of the present study worth mentioning, is that—contrary to previous studies (e.g., Chi et al., 1994)—both conditions spent equal time on the practice tasks. Hence, it could be hypothesized that the beneficial effects of self-explaining in these studies are not direct but caused by mediation: generating explanations usually requires more time and spending more time on subject matter increases performance. According to this hypothesis, the effect of self-explaining should disappear when time-on-task is equated between the conditions. Indeed, Matthews and Rittle-Johnson (2009) observed that solving tasks with self-explanations and solving more tasks without explanations in the same amount of time, resulted in equal final test performance. However, there are mixed results within the few studies that equated time-on-task, with some studies finding beneficial effects of self-explaining, while others did not (e.g., De Bruin et al., 2007; Matthews and Rittle-Johnson, 2009; De Koning et al., 2010; McEldoon et al., 2013) and most other studies on self-explaining did not (fully) report time-on-task (see Bisra et al., 2018). Thus, there is a definite need for more research that examines the interplay between self-explanation, time-on-task, and final test performance.
Another possibility why we did not find effects of self-explaining on learning of unbiased reasoning skills, however, is that our study was conducted as part of an existing course and the learning materials were part of the exam. Because of that, students of the control condition may have imposed desirable difficulties on themselves, for instance by covertly trying to come up with explanations for the questions. It seems likely that students would be more willing to invest effort when their performance on the learning materials actually matters (intrinsically or extrinsically) for them, which is often the case in field experiments conducted in real classrooms where the learning materials are related to the students' study domain. Therefore, it is possible that effects of desirable difficulties such as self-explaining found in the psychological laboratory—where students participate to earn required research credits and the learning materials are not part of their study program and sometimes even unrelated to their study domain—might not readily transfer to classroom studies. This would explain why previous studies, which are mostly laboratory studies, demonstrated effects of self-explaining and why these effects were mostly absent and in one case only short-lived in the classroom studies on unbiased reasoning (e.g., Heijltjes et al., 2014a,b, 2015). Moreover, this finding suggests a theoretical implication, namely that beneficial effects of creating desirable difficulty in instruction might become smaller when the willingness to invest increases and vice versa.
Future work might investigate why self-explanation prompts as used in the present study seem to have no additional effect after instruction and practice and whether strategies to improve students' quality of self-explanations would have beneficial effects on learning, and especially, transfer performance. Enhancing the quality of the self-explanations could be accomplished by, for example, providing students with a self-explanation training in advance, or by providing prompts that include some instructional assistance (cf. Berthold et al., 2009). Moreover, future research could investigate via classroom studies whether other desirable difficulties would be more beneficial for establishing learning and transfer of unbiased reasoning. In contrast to prompting self-explanations, other desirable difficulties such as creating task variability during practice and spacing of learning sessions apart, may result in beneficial effects since students of the control conditions cannot impose these desirable difficulties themselves (e.g., Weissgerber et al., 2018).
To conclude, based on the findings from the present study in combination with prior studies, prompting to self-explain during practice does not seem to be promising to enhance unbiased reasoning skills. This suggests that the nature of the task may be a boundary condition for effects of self-explaining on learning and transfer. Moreover, this study raises the question whether effects of self-explaining depend on the setting of the study, and thus contribute to knowledge about the usefulness of desirable difficulties in real educational settings. Considerably more research is needed to investigate how unbiased reasoning should be taught and especially how transfer can be fostered. This is important, because biased reasoning can have huge negative consequences in situations in both daily life and complex professional environments.
Ethics Statement
In accordance with the guidelines of the ethical committee at the Department of Psychology, Education and Child studies, Erasmus University Rotterdam, the study was exempt from ethical approval procedures because the materials and procedures were not invasive.
Author Contributions
LvP, PV, AH, and TvG contributed to the conception and design of the study. LvP, AH, and DK prepared the materials. LvP collected the data, organized the database, performed the statistical analysis, and wrote the original draft of the manuscript. PV, AH, EJ, and TvG provided critical revision of the manuscript. All authors read and approved the submitted version of the manuscript.
Conflict of Interest Statement
The authors declare that the research was conducted in the absence of any commercial or financial relationships that could be construed as a potential conflict of interest.
Acknowledgments
This research was funded by The Netherlands Organization for Scientific Research (project number 409-15-203). The authors would like to thank Stefan V. Kolenbrander for his help with running this study.
References
Abrami, P. C., Bernard, R. M., Borokhovski, E., Waddington, D. I., Wade, C. A., and Persson, T. (2014). Strategies for teaching students to think critically: a meta-analysis. Rev. Educ. Res. 85, 275–314. doi: 10.3102/0034654314551063
Arum, R., and Roksa, J. (2011). Limited learning on college campuses. Society 48, 203–207. doi: 10.1007/s12115-011-9417-8
Atkinson, R. K., Renkl, A., and Merrill, M. M. (2003). Transitioning from studying examples to solving problems: effects of self-explanation prompts and fading worked-out steps. J. Educ. Psychol. 95, 774–783. doi: 10.1037/0022-0663.95.4.774
Berry, D. C. (1983). Metacognitive experience and transfer of logical reasoning. Q. J. Exp. Psychol. 35, 39–49.
Berthold, K., Eysink, T. H. S., and Renkl, A. (2009). Assisting self-explanation prompts are more effective than open prompts when learning with multiple representations. Instruct. Sci. 37, 345–363. doi: 10.1007/s11251-008-9051-z
Bertsch, S., Pesta, B. J., Wiscott, R., and McDaniel, M. A. (2007). The generation effect: a meta-analytic review. Mem. Cogn. 35, 201–210. doi: 10.3758/BF03193441
Billings, L., and Roberts, T. (2014). Teaching Critical Thinking: Using Seminars for 21st Century Literacy. London: Routledge.
Bisra, K., Liu, Q., Nesbit, J. C., Salimi, F., and Winne, P. H. (2018). Inducing self-explanation: a meta-analysis. Educ. Psychol. Rev. Adv. 30, 703–725. doi: 10.1007/s10648-018-9434-x
Bjork, E. L., and Bjork, R. A. (2011). “Making things hard on yourself, but in a good way: creating desirable difficulties to enhance learning,” in Psychology and the Real World: Essays Illustrating Fundamental Contributions to Society, eds M. A. Gernsbacher, R. W. Pew, and J. R. Pomerantz (New York, NY: Worth Publishers), 59–68.
Bjork, E. L., Soderstrom, N. C., and Little, J. L. (2015). Can multiple-choice testing induce desirable difficulties? Evidence from the laboratory and the classroom. Am. J. Psychol. 128, 229–239. doi: 10.5406/amerjpsyc.128.2.0229
Bjork, R. A. (1994). “Memory and metamemory considerations in the training of human beings,” in Metacognition: Knowing About knowing, eds J. Metcalfe and A. Shimamura (Cambridge, MA: MIT Press), 185–205.
Chi, M. T. H. (2000). “Self-explaining expository texts: the dual processes of generating inferences and repairing mental models,” in Advances in Instructional Psychology, ed R. Glaser (Mahwah, NJ: Erlbaum), 161–238.
Chi, M. T. H., de Leeuw, N., Chiu, M., and LaVancher, C. (1994). Eliciting self-explanation improves understanding. Cogn. Sci. 18, 439–477.
Cohen, J. (1988). Statistical Power Analysis for the Behavioral Sciences, 2nd Edn. Hillsdale, NJ: Erlbaum.
Davies, M. (2013). Critical thinking and the disciplines reconsidered. High. Educ. Res. Dev. 32, 529–544. doi: 10.1080/07294360.2012.697878
De Bruin, A. B., Rikers, R. M., and Schmidt, H. G. (2007). The effect of self-explanation and prediction on the development of principled understanding of chess in novices. Contemp. Educ. Psychol. 32, 188–205. doi: 10.1016/j.cedpsych.2006.01.001
De Koning, B. B., Tabbers, H. K., Rikers, R. M. J. P., and Paas, F. (2010). Learning by generating vs. receiving instructional explanations: Two approaches to enhance attention cueing in animations. Comput. Educ. 55, 681–691. doi: 10.1016/j.compedu.2010.02.027
DeWinstanley, P. A., and Bjork, E. L. (2004). Processing strategies and the generation effect: implications for making a better reader. Mem. Cogn. 32, 945–955. doi: 10.3758/BF03196872
Dunlosky, J., Rawson, K. A., Marsh, E. J., Nathan, M. J., and Willingham, D. T. (2013). Improving students' learning with effective learning techniques: Promising directions from cognitive and educational psychology. Psychol. Sci. Publ. Interest 14, 4–58. doi: 10.1177/1529100612453266
Evans, J. S. (2003). In two minds: dual-process accounts of reasoning. Trends Cogn. Sci. 7, 454–459. doi: 10.1016/j.tics.2003.08.012
Evans, J. S. (2008). Dual-processing accounts of reasoning, judgment, and social cognition. Ann. Rev. Psychol. 59, 255–278. doi: 10.1146/annurev.psych.59.103006.093629
Evans, J. S. (2011). Dual-process theories of reasoning: contemporary issues and developmental applications. Dev. Rev. 31, 86–102. doi: 10.1016/j.dr.2011.07.007
Facione, P. A. (1990). Critical Thinking: A Statement of Expert Consensus for Purposes of Educational Assessment and Instruction. Millbrae, CA: The California Academic Press.
Faul, F., Erdfelder, E., Buchner, A., and Lang, A.-G. (2009). Statistical power analyses using G*Power 3.1: tests for correlation and regression analyses. Behav. Res. Methods 41, 1149–1160. doi: 10.3758/BRM.41.4.1149
Fiorella, L., and Mayer, R. E. (2016). Eight ways to promote generative learning. Educ. Psychol. Rev. 28, 717–741. doi: 10.1007/s10648-015-9348-9
Flores, K. L., Matkin, G. S., Burbach, M. E., Quinn, C. E., and Harding, H. (2012). Deficient critical thinking skills among college graduates: implications for leadership. Educ. Philos. Theory 44, 212–230. doi: 10.1111/j.1469-5812.2010.00672.x
Fong, G. T., Krantz, D. H., and Nisbett, R. E. (1986). The effects of statistical training on thinking about everyday problems. Cogn. Psychol. 18, 253–292. doi: 10.1016/0010-0285(86)90001-0
Fyfe, E. R., and Rittle-Johnson, B. (2017). Mathematics practice without feedback: a desirable difficulty in a classroom setting. Instruct. Sci. 45, 177–194. doi: 10.1007/s11251-016-9401-1
Hattie, J., and Timperley, H. (2007). The power of feedback. Rev. Educ. Res. 77, 81–112. doi: 10.3102/003465430298487
Heijltjes, A., Van Gog, T., Leppink, J., and Paas, F. (2014b). Improving critical thinking: Effects of dispositions and instructions on economics students' reasoning skills. Learn. Instruct. 29, 31–42. doi: 10.1016/j.learninstruc.2013.07.003
Heijltjes, A., Van Gog, T., Leppink, J., and Paas, F. (2015). Unraveling the effects of critical thinking instructions, practice, and self-explanation on students' reasoning performance. Instruct. Sci. 43, 487–506. doi: 10.1007/s11251-015-9347-8
Heijltjes, A., Van Gog, T., and Paas, F. (2014a). Improving students' critical thinking: empirical support for explicit instructions combined with practice. Appl. Cogn. Psychol. 28, 518–530. doi: 10.1002/acp.3025
Helsdingen, A. S., Van Gog, T., and van Merrienboer, J. J. G. (2011). The effects of practice schedule on learning a complex judgment task. Learn. Instruct. 21, 126–136. doi: 10.1016/j.learninstruc.2009.12.001
Hoogerheide, V., Loyens, S. M. M., and Van Gog, T. (2014). Effects of creating video-based modeling examples on learning and transfer. Learn. Instruct. 33, 108–119. doi: 10.1016/j.learninstruc.2014.04.005
Koehler, D. J., Brenner, L., and Griffin, D. (2002). “The calibration of expert judgment: Heuristics and biases beyond the labratory,” in Heuristics and Biases: The Psychology of Intuitive Judgment, eds T. Gilovich, D. W. Griffin, and D. Kahneman (New York, NY: Cambridge University Press), 686–715.
Lombrozo, T. (2006). The structure and function of explanations. Trends Cogn. Sci. 10, 464–470. doi: 10.1016/j.tics.2006.08.004
Matthews, P., and Rittle-Johnson, B. (2009). In pursuit of knowledge: comparing self-explanations, concepts, and procedures as pedagogical tools. J. Exp. Child Psychol. 104, 1–21. doi: 10.1016/j.jecp.2008.08.004
McCurdy, M. P., Leach, R. C., and Leshikar, E. D. (2017). The generation effect revisited: fewer generation constraints enhances item and context memory. J. Mem. Lang. 92, 202–216. doi: 10.1016/j.jml.2016.06.007
McDaniel, M. A., and Butler, A. C. (2010). “A contextual framework for understanding when difficulties are desirable,” in Successful Remembering and Successful Forgetting: A Festschrift in Honor of Robert A. Bjork, ed A. S. Benjamin (London: Psychology Press), 175–198.
McEldoon, K. L., Durkin, K. L., and Rittle-Johnson, B. (2013). Is self-explanation worth the time? A comparison to additional practice. Br. J. Educ. Psychol. 83, 615–632. doi: 10.1111/j.2044-8279.2012.02083.x
Metcalfe, J. (2011). “Desirable difficulties and studying in the region of proximal learning” in Successful Remembering and Successful Forgetting: A Festschrift in Honor of Robert A. Bjork, ed A. S. Benjamin (London: Psychology Press), 259–276.
Paas, F. (1992). Training strategies for attaining transfer or problem solving skills in statistics: a cognitive-load approach. J. Educ. Psychol. 84, 429–434. doi: 10.1037/0022-0663.84.4.429
Paas, F., Renkl, A., and Sweller, J. (2003). Cognitive load theory and instructional design: recent developments. Educ. Psychol. 38, 1–4. doi: 10.1207/S15326985EP3801_1
Paas, F., and Van Merriënboer, J. J. G. (1993). The efficiency of instructional conditions: an approach to combine mental efforts and performance measures. Hum. Fact. 35, 737–743. doi: 10.1177/001872089303500412
Rachlinski, J. J. (2004). “Heuristics, biases, and governance,” in Blackwell Handbook of Judgment and Decision Making, eds D. J. Koehler and N. Harvey (Malden, MA: Blackwell Publishing Ltd.), 567–584.
Rittle-Johnson, B., and Loehr, A. (2017). Eliciting explanations: constraints on when self-explanation aids learning. Psychonom. Bull. Rev. 24, 1501–1510. doi: 10.3758/s13423-016-1079-5
Schworm, S., and Renkl, A. (2007). Learning argumentation skills through the use of prompts for self-explaining examples. J. Educ. Psychol. 99, 285–296. doi: 10.1037/0022-0663.99.2.285
Siegler, R. S. (2002). “Microgenetic studies of self-explanations,” in Microdevelopment: Transition Processs in Development and Learning, eds N. Grannot and J. Parziale (New York, NY: Cambridge University Press), 31–58.
Slamecka, N. J., and Graf, P. (1978). The generation effect: delineation of a phenomenon. J. Exp. Psychol. Hum. Learn. Mem. 4, 592–604. doi: 10.1037/0278-7393.4.6.592
Soderstrom, N. C., and Bjork, R. A. (2015). Learning versus performance: an integrative review. Perspect. Psychol. Sci. 10, 176–199. doi: 10.1177/1745691615569000
Stanovich, K. E. (2011). Rationality and the Reflective Mind. New York, NY: Oxford University Press.
Stanovich, K. E., Toplak, M. E., and West, R. F. (2008). The development of rational thought: a taxonomy of heuristics and biases. Adv. Child Dev. Behav. 36, 251–285. doi: 10.1016/S0065-2407(08)00006-2
Stanovich, K. E., West, R. K., and Toplak, M. E. (2016). The Rationality Quotient: Toward a Test of Rational Thinking. Cambridge, MA: MIT Press.
Tversky, A., and Kahneman, D. (1974). Judgment under uncertainty: heuristics and biases. Sciences 185, 1124–1131. doi: 10.1126/science.185.4157.1124
Tversky, A., and Kahneman, D. (1983). Extensional versus intuitive reasoning: the conjunction fallacy in probability judgment. Psychol. Rev. 90, 293–315. doi: 10.1037/0033-295X.90.4.293
Weissgerber, S. C., Reinhard, M. A., and Schindler, S. (2018). Learning the hard way: need for cognition influences attitudes toward and self-reported use of desirable difficulties. Educ. Psychol. 38, 176–202. doi: 10.1080/01443410.2017.1387644
West, R. F., Toplak, M. E., and Stanovich, K. E. (2008). Heuristics and biases as measures of critical thinking: associations with cognitive ability and thinking dispositions. J. Educ. Psychol. 100, 930–941. doi: 10.1037/a0012842
Wylie, R., and Chi, M. T. H. (2014). “The self-explanation principle in multimedia learning,” in Cambridge Handbook of Multimedia Learning, ed R. E.Mayer (New York, NY: Cambridge University Press), 413–432.
Yan, V. X., Clark, C. M., and Bjork, R. A. (2016). “Memory and metamemory considerations in the instruction of human beings revisited: implications for optimizing online learning,” in From the Laboratory to the Classroom: Translating the Learning Sciences for Teachers, eds J. C. Horvath, J. Lodge, and J. A. C. Hattie (Abingdon: Routledge), 61–78.
Keywords: critical thinking, reasoning, heuristics and biases, instructional design, desirable difficulties, self-explaining
Citation: van Peppen LM, Verkoeijen PPJL, Heijltjes AEG, Janssen EM, Koopmans D and van Gog T (2018) Effects of Self-Explaining on Learning and Transfer of Critical Thinking Skills. Front. Educ. 3:100. doi: 10.3389/feduc.2018.00100
Received: 10 June 2018; Accepted: 26 October 2018;
Published: 19 November 2018.
Edited by:
Ralf Rummer, University of Kassel, GermanyCopyright © 2018 van Peppen, Verkoeijen, Heijltjes, Janssen, Koopmans and van Gog. This is an open-access article distributed under the terms of the Creative Commons Attribution License (CC BY). The use, distribution or reproduction in other forums is permitted, provided the original author(s) and the copyright owner(s) are credited and that the original publication in this journal is cited, in accordance with accepted academic practice. No use, distribution or reproduction is permitted which does not comply with these terms.
*Correspondence: Lara M. van Peppen, vanpeppen@essb.eur.nl