- 1National Science Foundation (NSF) National Center for Atmospheric Research (NCAR), Boulder, CO, United States
- 2National Oceanic and Atmospheric Administration (NOAA) Global Systems Laboratory (GSL), Boulder, CO, United States
- 3Colorado University, Cooperative Institute for Research in Environmental Sciences (CIRES), Boulder, CO, United States
Quantitative precipitation forecasts (QPF) from numerical weather prediction models need systematic verification to enable rigorous assessment and informed use, as well as model improvements. The United States (US) National Oceanic and Atmospheric Administration (NOAA) recently made a major update to its regional tropical cyclone modeling capabilities, introducing two new operational configurations of the Hurricane Analysis and Forecast System (HAFS). NOAA performed multi-season retrospective forecasts using the HAFS configurations during the period that the Hurricane Weather and Forecasting (HWRF) model was operational, which was used to assess HAFS performance for key tropical cyclone forecast metrics. However, systematic QPF verification was not an integral part of the initial evaluation. The first systematic QPF evaluation of the operational HAFS version 1 configurations is presented here for the 2021 and 2022 season re-forecasts as well as the first HAFS operational season, 2023. A suite of techniques, tools, and metrics within the enhanced Model Evaluation Tools (METplus) software suite are used. This includes shifting forecasts to mitigate track errors, regridding model and observed fields to a storm relative coordinate system, as well as object oriented verification. The HAFS configurations have better performance than HWRF for equitable threat score (ETS), but larger over forecast biases than HWRF. Storm relative and object oriented verification show the HAFS configurations have larger precipitation areas and less intense precipitation near the TC center as compared to observations and HWRF. HAFS QPF performance decreased for the 2023 season, but the general spatial patterns of the model QPF were very similar to 2021-2022.
1 Introduction
Quantitative precipitation forecasts (QPF) from numerical weather prediction (NWP) models are used across a range of forecast and impact planning applications, as well as for model development cycles. Systematic QPF verification that examines overall performance, biases, and spatial patterns enables informed use for forecast applications and future model improvements. QPF verification on an event level is more challenging than model verification for continuous fields (e.g., temperature) because continuous fields often have less skewed distributions (e.g., more Gaussian) while precipitation is discontinuous with many zero values, highly right-skewed, with many small non-zero values and few large (but consequential) values (Rossa et al., 2008). Because precipitation has a highly skewed distribution and is spatiotemporally discontinuous, more advanced verification techniques, including spatially insensitive or spatially aware metrics are needed, particularly for event based precipitation verification (Ebert and McBride, 2000; Ebert and Gallus, 2009; Gilleland et al., 2009; Wolff et al., 2014; Clark et al., 2016; Matyas et al., 2018; Zick, 2020; Newman et al., 2023). Even with these challenges, bulk QPF verification for global and regional NWP forecasts is routinely done across major modeling centers (e.g., McBride and Ebert, 2000; Haiden et al., 2012).
However, QPF verification for specific features such as tropical cyclones (TCs) is even more challenging beyond general QPF verification. Feature specific spatial displacement error corrections and spatially aware verification techniques are more critical for TC QPF verification because TCs are relatively localized features with large spatial gradients of precipitation intensity that are tied to internal dynamics, and NWP forecasts often have large spatial displacement errors. Several TC specific QPF verification methodologies to address these challenges have been developed over the past one to 2 decades (Marchok et al., 2007; Cheung and Coauthors, 2018; Chen et al., 2018; Yu et al., 2020; Ko et al., 2020; Newman et al., 2023; Stackhouse et al., 2023). These methods and systems can be applied to large-sample TC QPF forecasts and provide useable information about QPF performance for model improvement (Newman et al., 2023).
Recently, the United States (US) National Oceanic and Atmospheric Administration (NOAA) National Centers for Environmental Prediction (NCEP) made a major update to its regional tropical cyclone (TC) NWP capabilities. Two configurations of the new Hurricane Analysis and Forecast System (HAFS) were made operational in advance of the 2023 season. Extensive model development and testing was performed by NCEP and the broader TC research community before the HAFS configurations were made operational (Dong et al., 2020; Hazelton and Coauthors, 2021; Zhang et al., 2023; Hazelton, 2022). The pre-implementation testing included a multi-season retrospective forecast evaluation with both HAFS configurations, with case samples corresponding to operational forecasts from the Hurricane Weather and Forecasting (HWRF) model. Accumulated precipitation was archived across forecast lead times from the models for the operational and retrospective forecasts, but was not systematically examined as part of the operational model implementation decision making process.
Here we present the first systematic, large-sample QPF verification of the HAFS and HWRF forecasts for the 2021 and 2022 season retrospective forecasts along with evaluation of the two operational HAFS configurations during the 2023 season, the first operational season for HAFS. We use a set of the TC and QPF specific tools within the enhanced Model Evaluation Tools (METplus) software system (Jensen et al., 2023; Brown and Coauthors, 2021). Newman et al. (2023) describe the development and application of the METplus TC and QPF tools for large-sample TC QPF verification. These tools include capabilities to shift model forecasts to mitigate track errors, regridding model and observed fields to a storm relative cylindrical coordinate system, as well as use of the object oriented verification using the MET Method for Object-Based Diagnostic Evaluation (Davis et al., 2006) tool applied to TC precipitation objects. This initial application of the suite of METplus QPF verification tools for TCs lays the foundation for future implementation of more comprehensive QPF verification within the HAFS workflow and broader TC research and forecasting communities, as HAFS and METplus are open-source, community capabilities.
2 Data and methods
2.1 Datasets
2.1.1 Models
Three NOAA NCEP operational regional hurricane models were used for this evaluation. The Hurricane Weather Research and Forecast (HWRF, Tallapragada, 2016; Mehra et al., 2018; Biswas, 2018) model became operational during the 2007 hurricane season (Tallapragada, 2016). Here we use the HWRF operational version for the 2021-2022 hurricane season. HWRF uses a triple-nested domain configuration with horizontal resolutions of 13.5 km for the parent domain, 4.5 km for the intermediate nest and 1.5 km for the inner nest. HWRF includes self-cycled hybrid Ensemble Kalman Filter (EnKF) data assimilation, ocean and wave coupling. The physics parameterizations used in the HWRF model are summarized in Table 1. Next, NOAA’s Unified Forecast System (UFS)-based hurricane application using the Finite-Volume Cubed-Sphere (FV3) dynamical core, the Hurricane Analysis and Forecast System (HAFS), became operational in June 2023. This new regional hurricane system is scheduled to fully replace the HWRF model, as well as the operational Hurricanes in a Multi-scale Ocean coupled Non-hydrostatic model (HMON; Mehra et al., 2018), with two distinct configurations. These configurations are referred to as HAFS-A and HAFS-B, using the 4-letter identifiers HFSA and HFSB, respectively. Both HAFS configurations are run with one moving nest, with horizontal grid spacing of 6 km for the parent and 2 km for the inner nest. Similar to HWRF, the HAFS system is run with ensemble variational data assimilation, ocean and wave coupling. Table 1 highlights the physics differences between the HAFS configurations. Most notable are the differences in the planetary boundary layer (PBL) scheme options and the microphysics schemes used. The use of distinctly different microphysics schemes in the HWRF, HFSA, and HFSB is an important consideration when evaluating the QPF performance of each system. A notable difference between the NOAA Geophysical Fluid Dynamics Laboratory (GFDL) microphysics used in HFSA and the Thompson microphysics used in HFSB is that the GFDL microphysics is a single-moment bulk cloud microphysics scheme whereas the Thompson microphysics is a double-moment scheme. The double-moment framework gives more flexibility for predicted particle size distribution, which may lead to better representation of precipitation processes. Note, the HWRF model uses the Ferrier-Aligo microphysics scheme, which is a single-moment scheme, with a single precipitation ice class (combined snow-graupel), diagnostic riming, and simplified sedimentation and may be the most different as compared to the other two microphysics schemes.
Retrospective forecasts of the North Atlantic basin 2021 and 2022 seasons for HFSA and HFSB, operational HWRF output from the North Atlantic basin 2021 and 2022 seasons, along with the operational output for the 2023 North Atlantic basin season for HFSA and HFSB are used in the evaluation. Finally, the parent domain was used for all three modeling systems. This was done to ensure consistency in horizontal resolution of the QPF fields from inner to outer regions of the storm.
2.1.2 Observations
QPF was verified against different observations over land and water. The quality and availability of observations over land and the ocean was a primary consideration. Over land, the Climatology-Calibrated Precipitation Analysis (CCPA; Hou et al., 2014) dataset was used for the verification. CCPA is a 5-km grid spacing, gauge corrected radar observation product that combines gauge analysis and stage IV data (Lin and Mitchell, 2005). Over water, model QPF was verified against the Integrated Multi-satellitE Retrievals for GPM (IMERG; Huffman et al., 2020; Qi et al., 2021). IMERG is a state-of-the-science 0.10° satellite precipitation product combining spaceborne radar, passive microwave, and geostationary satellite data. All models and observations were re-gridded to the common IMERG grid.
The best track analysis (Jarvinen et al., 1984; Rappaport and Coauthors, 2009; Landsea and Franklin, 2013), which is a subjective analysis of the track position and maximum wind speeds based on available observational data, was used for evaluation methods requiring track location. Best track files were obtained from the National Hurricane Center ftp server (https://ftp.nhc.noaa.gov/atcf/btk/).
2.2 Methodology
All large-sample verification results are a homogeneous sample that includes all forecast-observation pairs that have a best track and event equalization across all three models (e.g., all models have the same forecast valid times).
2.2.1 Tropical cyclone specific processing
Following the QPF verification methodology described in Newman et al. (2023), TC-specific processing was employed. First, similar to Marchok et al. (2007), a track shift was applied to forecast data prior to grid-based verification in order to mitigate the effects of the track error. Figure 1A is a schematic representation of this process, where the model field, in this case precipitation, is shifted laterally to account for the track difference between the model forecast track as diagnosed by the GFDL vortex tracker (Marchok, 2021) and the best track analysis. A 600-km mask around the best track location at each valid time was applied to focus on the near-storm environment (Figure 1A). Additionally, Figure 1B demonstrates the mask designating the land boundaries, which was applied for the different observational datasets based on the storm location over land or water.
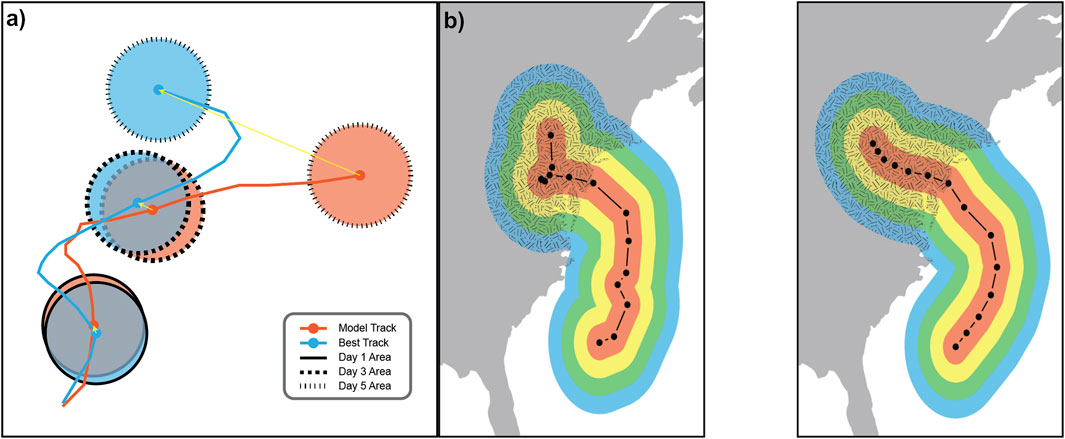
Figure 1. Schematic of (A) forecast field shifting using forecast minus analyzed track errors at three forecast valid times and (B) storm-relative distance masks within MET. User-specified range intervals (100 km) shown in colors are computed relative to the storm center (black line with circle markers, 12-h interval between markers) using the Gen-Vx-Mask Tool for both the model (left) and observations (right). Additional masking between the land (hatching colors over gray background) and water (colors over white background) is highlighted here. Original images from Newman et al. (2023), Figures 2, 4, ©American Meteorological Society. Used with permission.
2.2.2 METplus tools
The enhanced Model Evaluation Tools version 11.1.0 (METplus; Jensen et al., 2023), was utilized for the verification. Newman et al. (2023) describes the individual tools employed for TC QPF verification, including the Gen-Vx-Mask tool for creating a 600 km mask around the storm track at each forecast lead time and used for the grid-to-grid verification, the Regrid-Data-Plane tool for interpolating forecasts and observations to a common grid (which is required by the MODE tool), the Shift-Data-Plane tool for the track shifting methodology, the Pcp-Combine tool for generating precipitation accumulation intervals, the Grid-Stat tool for matching gridded forecast and observation grid points, the TC-RMW to regrid model and observation data onto a moving range-azimuth grid centered along the points of the storm track, and the MODE tool for identifying precipitation objects. The TC-RMW and MODE tools allow for storm-centric and object oriented approaches, which is a complementary approach to the track shifting methodology described in Section 2.2.1.
3 Results
3.1 Verification of 2021–2022 North Atlantic basin seasons
3.1.1 Description of storms
The 2021 North Atlantic basin hurricane season had twenty one named storms, with seven reaching hurricane strength, including four major hurricanes. Eight storms made landfall in the US, including six tropical storms and two hurricanes (Brennan, 2021). 101 deaths were attributed directly to TC impacts, many due to flooding, with nearly 80 billion dollars in US damage reported. (National Hurricane Center, 2022). Significant rainfall occurred for US landfalling storms. For example, Tropical Storm Fred impacted the eastern US, with catastrophic flooding occurring in parts of the southern Appalachian mountains with over 10.78 inches of rainfall at Mt. Mitchell, North Carolina (Berg, 2021), while Hurricane Nicholas brought heavy rainfall across the US states of Louisiana, Mississippi, Georgia, and Florida with a maximum total rainfall of 17.29 inches at Hammond, Louisiana (Latto and Berg, 2022).
The 2022 North Atlantic basin hurricane season had fourteen named storms, with nine reaching hurricane strength, including three major hurricanes. Four storms made landfall in the US, including one tropical storm and three hurricanes (Reinhart, 2022). 119 deaths were reported as a direct result of the tropical cyclones, with 116 billion dollars in damage in the US alone (National Hurricane Center, 2023). Several TCs had highly impactful rainfall effects, for example, Hurricane Ian brought widespread rainfall and flooding to Florida as well as the mid-Atlantic US states. The maximum storm total rainfall observed during Hurricane Ian was 26.95 inches in Grove City, Florida (Bucci et al., 2023).
3.1.2 Large sample verification
First, the track shifted grid-based QPF verification was compared against grid-based QPF verification of the same sample without track shifting applied to demonstrate the impact of track shifting on grid-based evaluation metrics. Figures 2A–D shows the equitable threat score (ETS) and frequency bias for 6 h accumulated precipitation across forecast lead times for low thresholds of greater than or equal to 0.1 inches (Figures 2A,C) and higher precipitation thresholds of greater than or equal to 2.5 inches (Figures 2B, D) with confidence intervals applied for the 95th percentile. For the ETS, a value of 0 indicates no skill and a value of 1 represents a perfect forecast as compared to random chance. The impact of the shifting is less evident for lower thresholds when there are many grid cells with precipitation than with the larger thresholds. The shifting mitigates average spatial error, thus helping to stabilize the skill scores at longer lead times due to ETS being a non-neighborhood metric, in particular for the larger precipitation thresholds. The overall lower magnitude of the ETS skill scores (ETS of less than 0.2) may be attributed to issues with ETS calculations of random chance adjustment with many rainy grid cells over a small domain (Wang, 2014). For example, the ETS scores for the full parent domain without storm area masking are higher than those in Figures 2A, B (not shown).
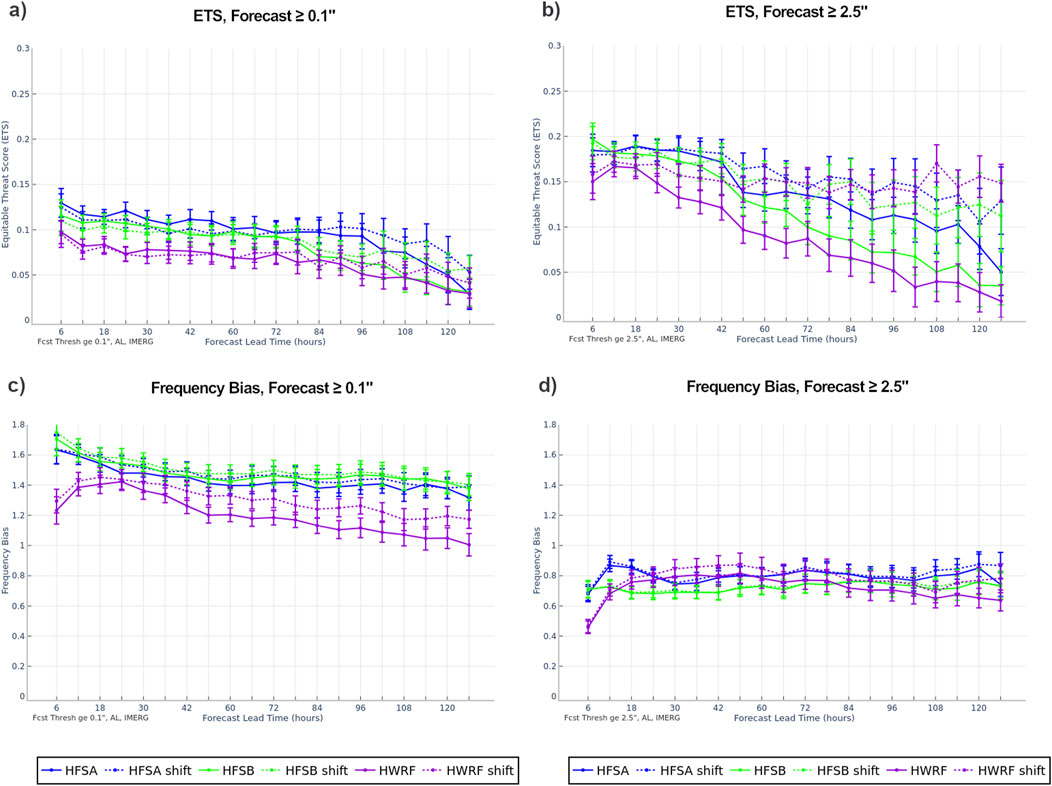
Figure 2. 6-h accumulated precipitation (A, B) ETS and (C, D) frequency bias for thresholds of greater than or equal to (A, C) 0.1 inch and (B, D) 2.5 inches for HFSA (blue), HFSB (green), and HWRF (purple) with bars denoting 95% parametric confidence intervals by lead time. Shifted (dashed) and unshifted (solid) track forecasts verified over water for all storms during the 2021-2022 North Atlantic basin hurricane seasons.
The frequency bias for the shifted and unshifted forecasts is shown in Figures 2C, D. Frequency bias is defined with a value of 1 representing an unbiased forecast, values greater than one indicating the precipitation is forecasted too frequently, and values less than one indicating the precipitation is not forecasted frequently enough. The general trend of over forecasting precipitation for lower thresholds (Figure 2C) and under forecasting for larger thresholds (Figure 2D) is evident for both the shifted and unshifted forecasts. Track shifting does not have a large impact on the frequency bias results, with the exception of the HWRF forecasts at the longest lead times. This could be attributable to large track errors at those longer lead times, but all three configurations have similar magnitude track errors (not shown). However, the storm structure in HWRF appears to be different from HFSA and HFSB (see Sections 3.1.3.1). The HWRF storm structure differences may have resulted in different precipitation feature shifting behavior along the boundaries of the 600-km mask when the larger shifts at longer lead times were applied. The relatively small impact from the track shifting methodology on the frequency bias results other than situations where the spatial errors are very large is expected because this statistic does not use spatial information, just the counts of rainy and non-rainy grid cells.
For the remainder of the multi-season analysis, only the shifted track forecasts will be shown. Figures 3A–F shows the ETS for 6 h accumulated precipitation for a variety of thresholds ranging from greater than or equal to 0.1 inches to greater than or equal to 5.0 inches for each of the 3 model configurations. The forecasts over land (Figures 3A–C), verified against the CCPA, demonstrate that the lowest skill occurs at the largest and smallest thresholds (greater than or equal to 5 and 0.1 inches, respectively). Particularly evident for the HAFS configurations, the intermediate precipitation thresholds of greater than or equal to 0.5 inch to 1.5 inches have precipitation forecasts with the highest skill. When comparing the trends of the HAFS configurations to those of the HWRF model, the HWRF model demonstrates more stable skill with increasing lead time, whereas the HAFS configurations skill drops relatively more with increasing lead time. For forecasts over water (Figures 3D–F), verified against IMERG, the lowest skill across all three model configurations occurs at the lowest threshold of greater than or equal to 0.1 inch. This is likely attributed to the ETS calculation itself as previously discussed. The track shifting over water results in a fairly constant ETS throughout the 5-day forecast period. Similar to the land-only verification results, the lower ETS values for the HAFS configurations are associated with the largest thresholds, with increasing ETS for the intermediate precipitation thresholds. The HWRF configuration performs more similarly across all precipitation thresholds above the lowest threshold.
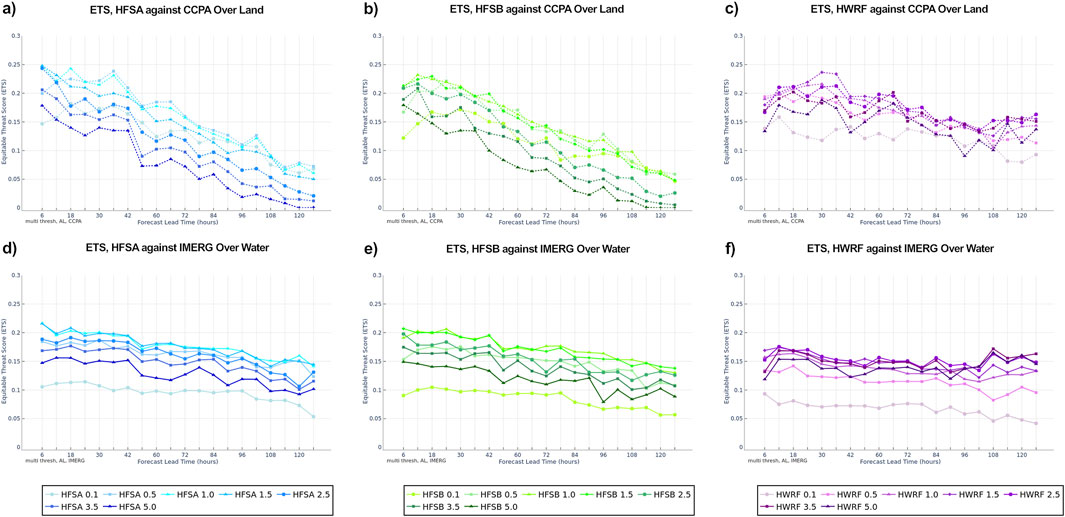
Figure 3. 6-h accumulated precipitation ETS for HFSA (a,d; blue), HFSB (b,e; green), and HWRF (c,f; purple) verified against CCPA over land (A–C) and IMERG over water (D–F) for all storms during the 2021-2022 North Atlantic basin hurricane season by lead time. Precipitation thresholds range from greater than or equal to 0.1 inch (lightest shade) to greater than or equal to 5.0 inches (darkest shade).
Frequency bias for track forecasts over land (Figures 4A–C), verified against CCPA, show that the model accumulated precipitation at the largest thresholds are forecasted well, with frequency bias values near 1. The smaller precipitation thresholds have overforecasted precipitation for all models and configurations. When verifying the track forecasts over water (Figures 4D–F), verified against IMERG, the largest precipitation thresholds are often under forecasted for all models and configurations, while the smallest precipitation thresholds are over forecasted by all models and configurations. For all models and configurations, the frequency bias behaviors remain relatively constant throughout the 5-day forecast period. One exception is in the first 12–18 h, the HAFS configurations have slightly decreasing frequency bias, while HWRF has increasing values, particularly at larger thresholds, which could be due to the differences in model initialization and spin-up.
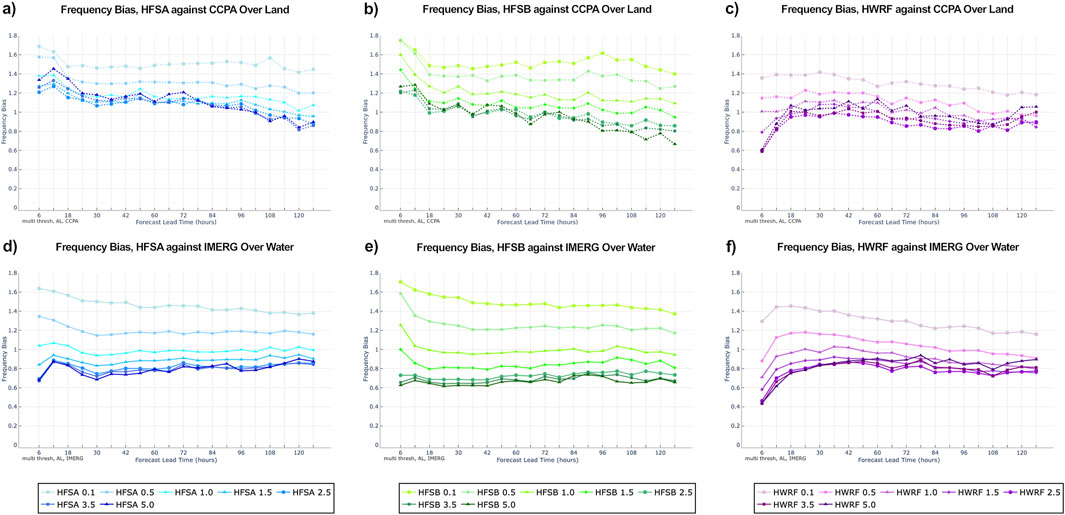
Figure 4. 6-h accumulated precipitation frequency bias for HFSA (a,d; blue), HFSB (b,e; green), and HWRF (c,f; purple) verified against CCPA over land (A–C) and IMERG over water (D–F) for all storms during the 2021-2022 North Atlantic basin hurricane season by lead time. Precipitation thresholds range from greater than or equal to 0.1 inch (lightest shade) to greater than or equal to 5.0 inches (darkest shade).
3.1.3 Hurricane Ian
Hurricane Ian occurred from 23 to 30 September 2022 and was the ninth named storm in the North Atlantic basin during the 2022 hurricane season. Ian made landfall in southwestern Florida as a category 4 intensity on the Saffir-Simpson scale on 28 September 2022 near Punta Gorda, Florida. Hurricane Ian was responsible directly for 66 deaths and an estimated 112 billion dollars in damage, making it Florida’s costliest hurricane and the third most costliest hurricane in US history. Storm total rainfall reports showed a maximum of 26.95 inches of rainfall at Grove City, Florida (Bucci et al., 2023).
3.1.3.1 Storm-centric verification
Mean 6-hourly precipitation accumulation (mm) for 16 initializations of Hurricane Ian at the 12-h lead time for over 25 September 2022 at 00 UTC to 28 September 2022 at 18 UTC are shown in Figures 5, 6. The 12 h forecast was chosen as a representative example of the storm in a time period where the forecast errors are not too large, but far enough away from initialization shock issues. This is the period when Ian was a tropical storm or hurricane in the Gulf of Mexico and making landfall in southwestern Florida. The analysis is done using the TC-RMW tool in METplus, which transforms the cartesian coordinates of the model domain into storm-relative coordinates normalized by the radius of maximum winds (RMW). The shading represents the precipitation accumulation in millimeters per 6 h. Relative to the IMERG analysis, the model configurations show a smaller storm and less precipitation in the eastern semicircle (Figure 5). The HAFS configurations, HFSA and HFSB (Figures 5B, C) are fairly similar in placement and intensity of the precipitation, with the HFSA producing slightly higher precipitation accumulations in the storm center as well as the western semicircle. The HWRF model shows more compact, intense precipitation accumulations closer to the center of the storm with less precipitation in the eastern semicircle than the HAFS configurations. A notable feature is the persistent outer band in the upper right quadrant around 5-10 RMW, which has the best placement in the HAFS configurations. We leave distance in units of RMW as this normalization ties precipitation features more directly to the dynamics of the storms. In some cases, it may be more informative to convert back to physical distance, which depends on the specific use-case and verification purpose.
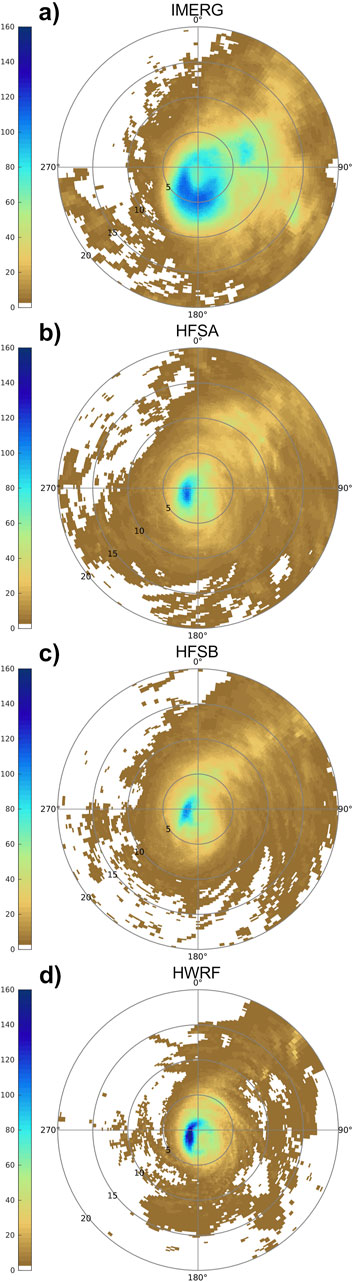
Figure 5. Mean 6-hourly precipitation accumulation (mm) for 16 initializations of Hurricane Ian at the 12-h lead time for (A) IMERG, (B) HFSA, (C) HFSB and (D) HWRF at the same valid times. Radii are normalized by the radius of maximum wind.
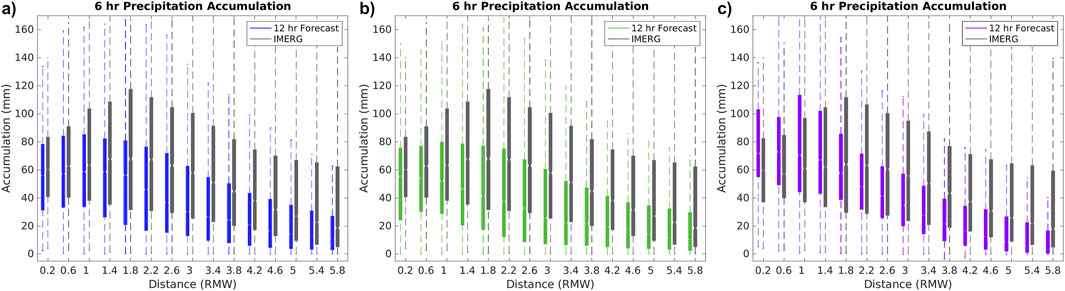
Figure 6. Boxplot of all 6-hourly precipitation accumulation (mm) grid points aggregated across 16 forecast initialization times from Hurricane Ian for the 12-h lead time forecasts for (A) HFSA, (B) HFSB and (C) HWRF compared to IMERG. For each box, the notches indicate the median and the bottom and top edges show the 25th and 75th percentiles, respectively. The whiskers extend to the most extreme data points not considered outliers.
The output from the TC-RMW tool was used in Figure 6, which are box plots of accumulated precipitation using distance bins of 0.4 RMW. Relative to IMERG, the HAFS configurations show lower precipitation accumulations, whereas the HWRF configuration has larger accumulations closer to the RMW with a steep drop after about 2-3 RMW. In general, the HAFS gradients, moving from the center, better match those of IMERG. Comparing the two HAFS configurations, the HFSA configuration shows a distribution and mean that is closer to IMERG, in particular out to 3 RMW.
3.1.3.2 Object based verification
The Method for Object-based Diagnostic Evaluation (MODE) within METplus is used for spatial verification of the precipitation field. The MODE object identification algorithm mimics the subjective matching of observed and forecasted objects by human forecasters using a multistep process and fuzzy logic engine (Davis et al., 2006). The 6 h accumulation for the 12 h forecast of Hurricane Ian valid at 06 UTC 28 September 2022 (18 UTC 27 September 2022 initialization) for a specific configuration of MODE is shown in Figure 7 for demonstration. Figure 7A is the forecasted accumulated precipitation from the HFSA configuration with MODE filtering applied. Similarly, Figure 7B shows the same field for the combined IMERG and CCPA datasets using the MODE filtering algorithm. The IMERG and CCPA observations were combined into a single observation based on the land mask in order to support the MODE object identification. Figure 7C shows the MODE object identification of the forecast objects in the shading and observed objects in the outline. This MODE analysis configuration was performed for all 12 h forecasts during the period of Hurricane Ian used in Section 3.1.3.1.
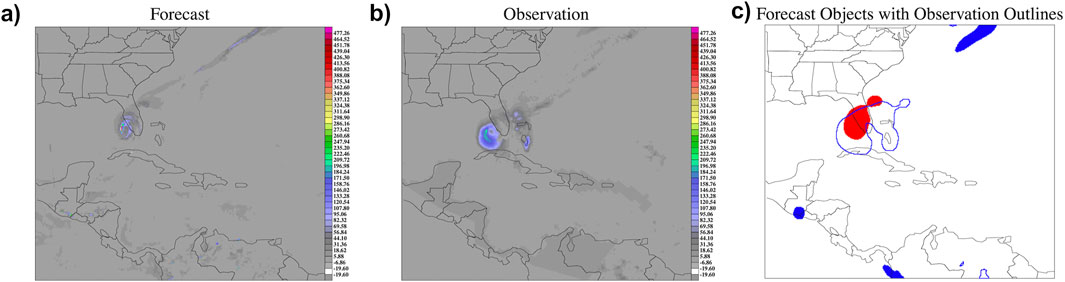
Figure 7. An example of MODE accumulated precipitation fields from the (A) 12 h HFSA forecast, (B) combined IMERG and CCPA observations, and (C) forecast objects for Hurricane Ian, identified as one object cluster in red with observation objects overlaid using blue outlines. The forecast valid at 06 UTC 28 September 2022 (18 UTC 27 September initialization) is shown.
All grid points with precipitation within the identified objects were used to calculate the frequency of 6 h precipitation accumulation (Figures 8A–C). The HAFS configurations both have a peak in the lightest precipitation and another area of precipitation greater than the combined IMERG and CCPA observations around 75–100 mm, most notably in the HFSA configuration. Conversely, the HWRF model is dominated by the heavy precipitation, as seen with the over forecast of precipitation accumulation near the storm center (Figures 6C, 8C), which could be due to the Ferrier-Aligo microphysics used in HWRF. The conclusions reached from the object based approach supports the findings using the storm-centric approaches in Section 3.1.3.1.
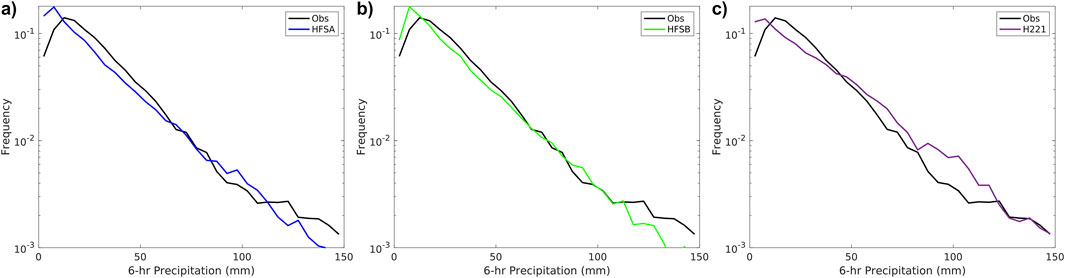
Figure 8. Log frequency of 6-h precipitation accumulations (mm) for (A) HFSA in blue, (B) HFSB in green, and (C) HWRF in purple compared to combined IMERG and CCPA observations in black.
3.2 Verification of 2023 North Atlantic basin season
3.2.1 Description of storms
The 2023 North Atlantic basin hurricane season had nineteen named storms, with seven reaching hurricane strength, including three major hurricanes. Three storms made landfall in the US, including two tropical storms and one hurricane (National Hurricane Center, 2024). 15 deaths were attributed directly to TC impacts during the 2023 season, with many associated with rainfall hazards (National Hurricane Center, 2024). Hurricane Franklin brought major rainfall associated impacts and damages to the Dominican Republic (Beven, 2024), and Hurricane Ophelia produced flooding throughout North Carolina with a maximum rainfall report of 9.51 inches near Greenville, North Carolina (Brown et al., 2024). The largest damage occurred in the US with Hurricane Idalia, described in Section 3.2.3.
3.2.2 Large sample verification
With a limited number of landfalling cases during the 2023 Atlantic basin hurricane season, the sample sizes were too small at the longest lead times to separate the land and water verification. Therefore, Figures 9, 10 show track shifted statistics from the combined forecasts over land and water, using the same observational datasets as before. Additionally, while the HWRF model was still run in limited capacity during the 2023 season, the gridded output was not available and therefore not included in this analysis. Figures 9A, B shows the ETS for 6 h precipitation accumulations for the same precipitation thresholds described in Section 3.1.2 and Figure 3. Again, the lowest ETS values are associated with the largest and smallest (greater than or equal to 0.1 inch) thresholds for both the HFSA and HFSB. Precipitation forecasts at the intermediate thresholds, ranging from greater than or equal to 0.5 inches to greater than or equal to 1.5 inches, consistently score higher. HFSB scores slightly higher than HFSA for lead times longer than 72 h. The impact of track shifting at longer lead times during the 2023 season did not prevent a decrease in ETS with lead time, as shown in the 2021-2022 season retrospective runs.
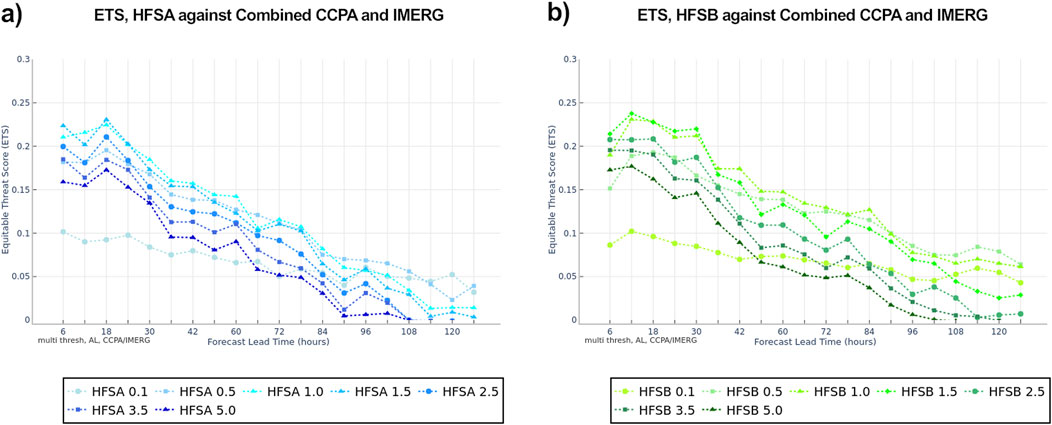
Figure 9. 6-h accumulated precipitation ETS for HFSA ((A) blue) and HFSB ((B) green) verified against both CCPA over land and IMERG over water for all storms during the 2023 North Atlantic basin hurricane season by lead time. Precipitation thresholds range from greater than or equal to 0.1 inch (lightest shade) to greater than or equal to 5.0 inches (darkest shade).
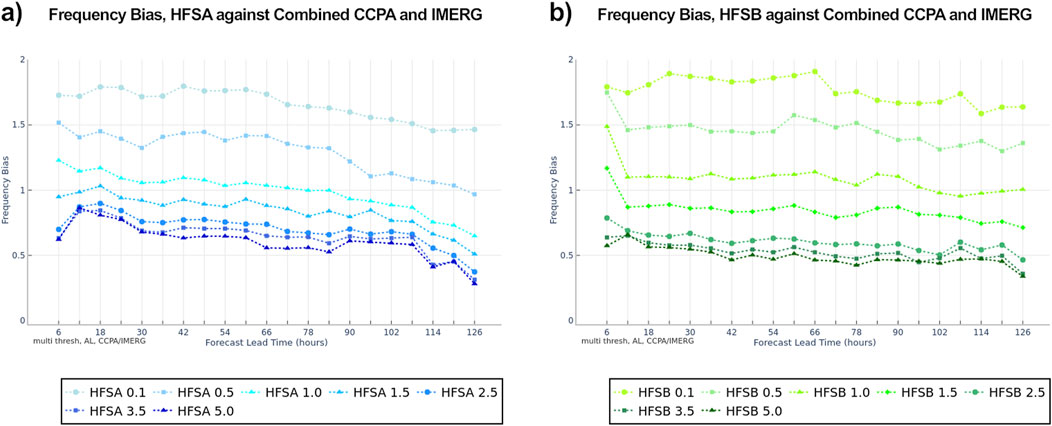
Figure 10. 6-h accumulated precipitation frequency bias for HFSA ((A) blue) and HFSB ((B) green) verified against both CCPA over land and IMERG over water for all storms during the 2023 North Atlantic basin hurricane season by lead time. Precipitation thresholds range from greater than or equal to 0.1 inch (lightest shade) to greater than or equal to 5.0 inches (darkest shade).
The frequency bias for the combined land and water verification is shown in Figures 10A, B. The models again tend to under forecast the largest precipitation thresholds, whereas the smallest thresholds tend to be over forecasted. Precipitation forecasts for thresholds of greater than or equal to 1.0–1.5 inch typically perform well with frequency bias values around 1. In general, the under forecasting of heavy precipitation is more extreme during the 2023 season than during the retrospective runs for the 2021-2022 seasons.
3.2.3 Hurricane Idalia
Hurricane Idalia occurred from 26 to 31 August 2023 and was the 10th named storm in the North Atlantic basin during the 2023 hurricane season. Idalia rapidly intensified in the Gulf of Mexico, making landfall in Florida’s big bend region as a category 3 hurricane. Idalia produced rainfall across the southeastern US states, with a maximum total rainfall of 13.55 inches reported at Holly Hill, SC. Idalia was responsible for 8 direct casualties, and an estimated damage of 3.6 billion US dollars. The rural region of Idalia’s landfall resulted in less damages than prior landfalling US TCs, primarily affecting the agricultural industry (Cangialosi and Alaka, 2024).
3.2.3.1 Storm-centric verification
Similar to Section 3.1.3.1, composites of 12 h forecasts of 6 h precipitation accumulation from 26 August 2023 at 18 UTC to 30 August 2023 at 18 UTC, which covers when Idalia was a tropical storm or hurricane, are shown in Figure 11. Both HAFS configurations show a smaller storm core with less precipitation than the IMERG observations. The HAFS configurations are similar to each other with placement of the most intense precipitation just to the east of IMERG. The HFSB configuration shows higher precipitation intensities compared to HFSA near the storm center.
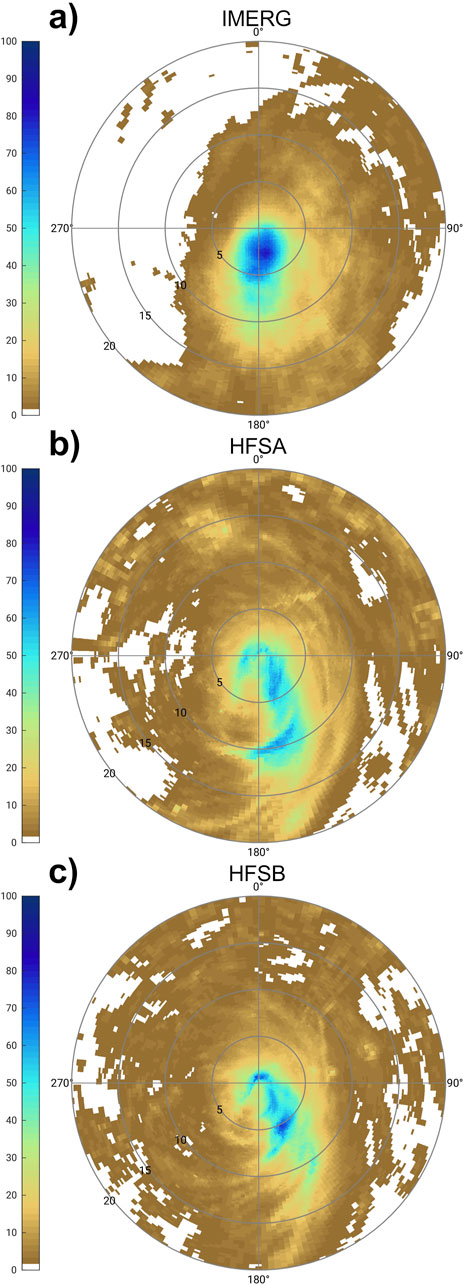
Figure 11. Mean 6-hourly precipitation accumulation (mm) for 17 initializations of Hurricane Idalia at the 12-h lead time for (A) IMERG, (B) HFSA, and (C) HFSB at the same valid times. Radii are normalized by the radius of maximum wind.
Box plots of precipitation accumulation using distance bins from the storm center normalized by RMW are shown in Figures 12A, B. Using the median of the distribution at each range bin, HFSA has an underestimation closer to the RMW, whereas HFSB has an overestimation around the RMW. Both HFSA and HFSB have slight underestimation of precipitation beyond 3 RMW.
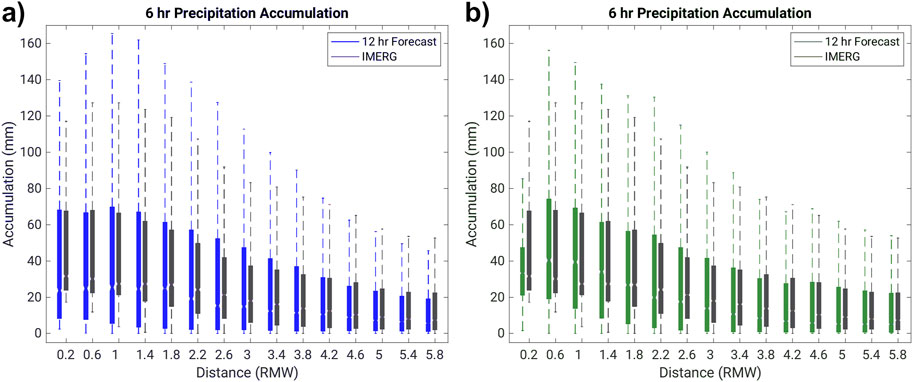
Figure 12. Boxplot of all 6-hourly precipitation accumulation (mm) grid points aggregated across 17 forecast initialization times from Hurricane Idalia for the 12-h lead time forecasts for (A) HFSA, and (B) HFSB compared to IMERG. For each box, the notches indicate the median and the bottom and top edges show the 25th and 75th percentiles, respectively. The whiskers extend to the most extreme data points not considered outliers.
3.2.4 Hurricane Lee
Hurricane Lee occurred from 5 to 16 September 2023 and was the 13th named storm in the North Atlantic basin during the 2023 hurricane season. Lee formed in the eastern Atlantic and rapidly intensified to a category 5 hurricane, remaining over water before making landfall in Nova Scotia as a post tropical system. The rainfall impacts occurred in eastern Maine and New Brunswick, Canada (Blake and Nepaul, 2024).
3.2.4.1 Storm-centric verification
For Hurricane Lee, 6 h accumulated precipitation for 12 h forecast composites are shown in Figure 13 covering 07 September 2023 at 00 UTC to 15 September 2023 at 06 UTC. This corresponds to the period when Lee was a tropical storm or hurricane in the North Atlantic basin. The HAFS configurations have a similar placement shape to the precipitation with more precipitation in the southeast quadrant. However, the intensity of the precipitation relative to IMERG is too weak in the southeast quadrant and stronger than IMERG in both configurations. The HFSA configuration also shows slightly higher precipitation accumulations relative to the HFSB configuration.
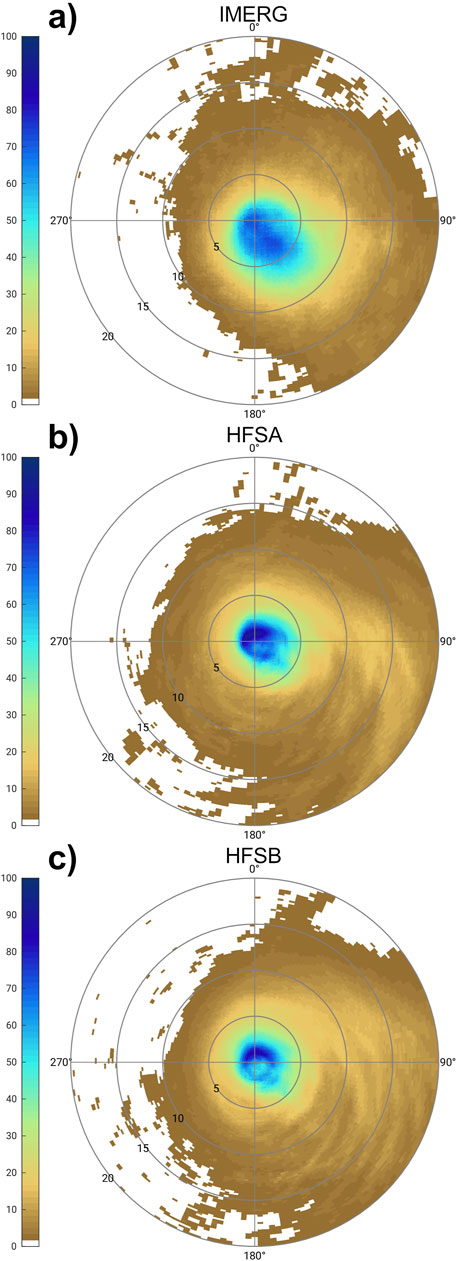
Figure 13. Mean 6-hourly precipitation accumulation (mm) for 34 initializations of Hurricane Lee at the 12-h lead time for (A) IMERG, (B) HFSA, and (C) HFSB at the same valid times. Radii are normalized by the radius of maximum wind.
The precipitation accumulation box plots shown in Figure 14 further demonstrate HFSA over estimated precipitation near RMW (Figure 14A) using the median of the distributions at each range bin, whereas the mean precipitation accumulations for HFSB near RMW closely match those of IMERG (Figure 14B). Beyond 1 RMW, the HAFS configurations are more similar.
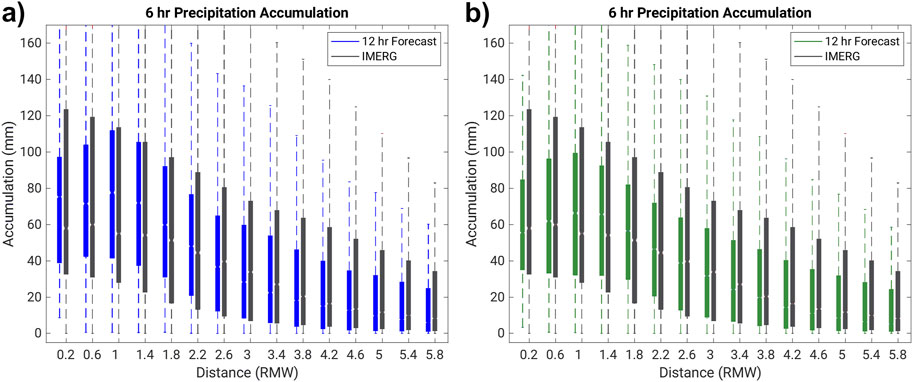
Figure 14. Boxplot of all 6-hourly precipitation accumulation (mm) grid points aggregated across 34 forecast initialization times from Hurricane Lee for the 12-h lead time forecasts for (A) HFSA and (B) HFSB compared to IMERG. For each box, the notches indicate the median and the bottom and top edges show the 25th and 75th percentiles, respectively. The whiskers extend to the most extreme data points not considered outliers.
4 Summary and discussion
Here we performed the first multi-season verification of QPF from the new NOAA operational regional TC forecasting system, HAFS. We use state-of-the-science methods to mitigate track errors for traditional grid-to-grid comparison methods, and spatially aware verification using both a storm-relative coordinate system and an object oriented approach (Newman et al., 2023).
Comparisons between the HAFS configurations and the HWRF model show that the more complex microphysics in the HAFS configurations better represent the tropical cyclone precipitation and features of TCs than the legacy Ferrier-Aligo microphysics scheme used in the HWRF model from the 2021-2022 North Atlantic basin retrospective forecasts. Generally, the HAFS version 1 configurations tend to over forecast precipitation for smaller thresholds and under forecast precipitation for larger thresholds. During the 2023 season, the HAFSv1 configurations demonstrated the same trends as the 2021-2022 seasonal retrospective, which includes over forecasting light precipitation and under forecasting larger accumulation thresholds, along with similar spatial patterns and gradients moving away from the storm center. However, the HAFSv1 configurations exhibited larger underestimation of higher thresholds relative to the 2021-2022 seasons. This could be from a variety of reasons (e.g., unique differences in 2023 such as record SSTs) and may be worth further investigation. Performance of the HAFS configurations varies across the case studies, with HFSA performing better for Idalia while HFSB performs better for Ian and Lee.
There are several considerations and avenues for future work within this specific type of analysis and TC QPF verification more generally. We see future research opportunities using these types of large-sample and case study QPF approaches for further process-oriented studies (e.g., Ko et al., 2020) to better understand relationships between model QPF spatial patterns and rapid intensification/rapid weakening forecasts, representation of internal core dynamics, and improved use of HAFS forecasts for inland freshwater flood forecasting. For our specific methodology, additional metrics or modifications to our existing metrics are needed when assessing ETS or possibly other scores over smaller verification domains when a large number of precipitating grid cells are present in the verification domain. Examination of or inclusion of different spatial interpolation techniques (e.g., Accadia et al., 2003) should be done, as well as in-depth examination of inherent intensity differences across models due to resolution or other factors, as well as across observations. Finally, improved automation and inclusion of QPF verification into the HAFS verification workflow is underway to enable near-real time TC QPF verification.
Data availability statement
The data analyzed in this study is subject to the following licenses/restrictions: Available upon request. Requests to access these datasets should be directed to Kathryn Newman, a25ld21hbkB1Y2FyLmVkdQ==.
Author contributions
KN: Conceptualization, Data curation, Formal Analysis, Funding acquisition, Investigation, Methodology, Project administration, Software, Supervision, Validation, Visualization, Writing–original draft, Writing–review and editing. BN: Data curation, Formal Analysis, Investigation, Methodology, Software, Validation, Visualization, Writing–review and editing. MB: Investigation, Writing–review and editing. LP: Funding acquisition, Investigation, Project administration, Resources, Writing–review and editing.
Funding
The author(s) declare that financial support was received for the research, authorship, and/or publication of this article. This work was supported by NOAA OAR (W8R2Q80) and under NSF NCAR Cooperative Agreement No. 1852977.
Acknowledgments
This work was supported by the Developmental Testbed Center, which is funded by NOAA Oceanic and Atmospheric Research, NOAA National Weather Service, United States Air Force, and the NSF NCAR. The National Center for Atmospheric Research is a major facility sponsored by the National Science Foundation under Cooperative Agreement No. 1852977. The authors thank the NOAA/NCEP/EMC and NOAA/AOML/HRD teams for model development, conducting the HAFS retrospective runs, and providing the operational HAFS and HWRF datasets for this evaluation. We also thank the three reviewers, as their suggestions improved the final manuscript.
Conflict of interest
The authors declare that the research was conducted in the absence of any commercial or financial relationships that could be construed as a potential conflict of interest.
The reviewer [AH] declared a past collaboration with the author [KMN] to the handling editor.
Publisher’s note
All claims expressed in this article are solely those of the authors and do not necessarily represent those of their affiliated organizations, or those of the publisher, the editors and the reviewers. Any product that may be evaluated in this article, or claim that may be made by its manufacturer, is not guaranteed or endorsed by the publisher.
References
Accadia, C., Mariani, S., Casaioli, M., Lavagnini, A., and Speranza, A. (2003). Sensitivity of precipitation forecast skill scores to bilinear interpolation and a simple nearest-neighbor average method on high-resolution verification grids. Wea. Forecast. 18, 918–932. doi:10.1175/1520-0434(2003)018<0918:sopfss>2.0.co;2
Aligo, E. A., Ferrier, B., and Carley, J. R. (2018). Modified NAM microphysics for forecasts of deep convective storms. Mon. Wea. Rev. 146, 4115–4153. doi:10.1175/MWR-D-17-0277.1
Bender, M. A., Ginis, I., Tuleya, R., Thomas, B., and Marchok, T. (2007). The operational GFDL coupled hurricane–ocean prediction system and a summary of its performance. Mon. Wea. Rev. 135, 3965–3989. doi:10.1175/2007MWR2032.1
Berg, R. (2021). National hurricane center tropical cyclone report, tropical storm fred. Miami, FL: National Oceanic and Atmospheric Administration National Hurricane Center. Available at: https://www.nhc.noaa.gov/data/tcr/AL062021_Fred.pdf (accessed on March 15, 2024).
Beven, J. L. (2024). National hurricane center tropical cyclone report, hurricane Franklin. Miami, FL: National Oceanic and Atmospheric Administration, National Hurricane Center. Available at: https://www.nhc.noaa.gov/data/tcr/AL082023_Franklin.pdf (accessed on March 15, 2024).
Biswas, M.Coauthors (2018). Hurricane weather research and forecasting (HWRF) model: 2017 scientific documentation. NCAR Tech. Note NCAR/TN-5441STR, 111. doi:10.5065/D6MK6BPR
Blake, E., and Nepaul, H. (2024). National hurricane center tropical cyclone report, hurricane lee. Miami, FL: National Oceanic and Atmospheric Administration, National Hurricane Center. Available at: https://www.nhc.noaa.gov/data/tcr/AL132023_Lee.pdf (Accessed March 15, 2024).
Brennan, M. (2021). “2021 NHC analysis and forecast challenges,” in HFIP annual meeting. Silver Spring, MD: National Oceanic and Atmospheric Administration National Weather Service. Available at: https://hfip.org/sites/default/files/events/269/200-brennan-2021-nhc-challenges.pdf (Accessed February 23, 2024).
Brown, B.Coauthors (2021). The model evaluation tools (MET): more than a decade of community-supported forecast verification. Bull. Amer. Meteor. Soc. 102, E782–E807. doi:10.1175/BAMS-D-19-0093.1
Brown, D. P., Hagen, A., and Alaka, L. (2024). National hurricane center tropical cyclone report, tropical storm Ophelia. Miami, FL: National Oceanic and Atmospheric Administration, National Hurricane Center. Available at: https://www.nhc.noaa.gov/data/tcr/AL162023_Ophelia.pdf (Accessed March 15, 2024).
Bucci, L., Alaka, L., Hagen, A., Delgado, S., and Beven, J. (2023). National hurricane center tropical cyclone report, hurricane ian. Miami, FL: National Oceanic and Atmospheric Administration, National Hurricane Center. Available at: https://www.nhc.noaa.gov/data/tcr/AL092022_Ian.pdf (Accessed March 15, 2024).
Cangialosi, J. P., and Alaka, L. (2024). National hurricane center tropical cyclone report, hurricane Idalia. Miami, FL: National Oceanic and Atmospheric Administration, National Hurricane Center. Available at: https://www.nhc.noaa.gov/data/tcr/AL102023_Idalia.pdf (Accessed March 15, 2024).
Chen, X., Hazelton, A., Marks, F. D., Alaka, G. J., and Zhang, C. (2023). Performance of an improved TKE-based eddy-diffusivity mass-flux (EDMF) PBL scheme in 2021 hurricane forecasts from the hurricane analysis and forecast system. Wea. Forecast. 38, 321–336. doi:10.1175/WAF-D-22-0140.1
Chen, Y., Ebert, E. E., Davidson, N. E., and Walsh, K. J. E. (2018). Application of Contiguous Rain Area (CRA) methods to tropical cyclone rainfall forecast verification. Earth Space Sci. 5, 736–752. doi:10.1029/2018EA000412
Cheung, K.Coauthors (2018). Recent advances in research and forecasting of tropical cyclone rainfall. Trop. Cyclone Res. Rev. 7, 106–127. doi:10.6057/2018TCRR02.03
Clark, P., Roberts, N., Lean, H., Ballard, S. P., and CharltonPerez, C. (2016). Convection-permitting models: a step-change in rainfall forecasting. Meteor. Appl. 23, 165–181. doi:10.1002/met.1538
Davis, C., Brown, B., and Bullock, R. (2006). Object-based verification of precipitation forecasts. Part I: methodology and application to mesoscale rain areas. Mon. Wea. Rev. 134, 1772–1784. doi:10.1175/MWR3145.1
Dong, J., Liu, B., Zhang, Z., Wang, W., Mehra, A., Hazelton, A. T., et al. (2020). The evaluation of real-time hurricane analysis and forecast system (HAFS) stand-alone regional (sar) model performance for the 2019 atlantic hurricane season. Atmosphere 11, 617. doi:10.3390/atmos11060617
Ebert, E. E., and Gallus, W. A. (2009). Toward better understanding of the contiguous rain area (CRA) method for spatial forecast verification. Wea. Forecast. 24, 1401–1415. doi:10.1175/2009WAF2222252.1
Ebert, E. E., and McBride, J. L. (2000). Verification of precipitation in weather systems: determination of systematic errors. J. hydrology 239 (1-4), 179–202. doi:10.1016/s0022-1694(00)00343-7
Ek, M. B., Mitchell, K. E., Lin, Y., Rogers, E., Grunmann, P., Koren, V., et al. (2003). Implementation of Noah land surface model advances in the National Centers for Environmental Prediction operational mesoscale Eta model. J. Geophys. Res. Atmos. 108, D22. doi:10.1029/2002jd003296
Gilleland, E., Ahijevych, D., Brown, B., Casati, B., and Ebert, E. (2009). Intercomparison of spatial forecast verification methods. Wea. Forecast. 24, 1416–1430. doi:10.1175/2009WAF2222269.1
Haiden, T., Rodwell, M., Richardson, D., Okagaki, A., Robinson, T., and Hewson, T. (2012). Intercomparison of global model precipitation forecast skill in 2010/11 using the SEEPS score. Mon. Wea. Rev. 140, 2720–2733. doi:10.1175/mwr-d-11-00301.1
Han, J., and Bretherton, C. S. (2019). TKE-based moist eddy-diffusivity mass-flux (EDMF) parameterization for vertical turbulent mixing. Wea. Forecast. 34, 869–886. doi:10.1175/WAF-D-18-0146.1
Han, J., and Pan, H. (2011). Revision of convection and vertical diffusion schemes in the NCEP global forecast system. Wea. Forecast. 26, 520–533. doi:10.1175/WAF-D-10-05038.1
Han, J., Wang, W., Kwon, Y. C., Hong, S.-Y., Tallapragada, V., and Yang, F. (2017). Updates in the NCEP GFS cumulus convection schemes with scale and aerosol awareness. Weather Forecast 32, 2005–2017. doi:10.1175/WAF-D-17-0046.1
Han, J., Witek, M. L., Teixeira, J., Sun, R., Pan, H., Fletcher, J. K., et al. (2016). Implementation in the NCEP GFS of a hybrid eddy-diffusivity mass-flux (EDMF) boundary layer parameterization with dissipative heating and modified stable boundary layer mixing. Wea. Forecast. 31, 341–352. doi:10.1175/WAF-D-15-0053.1
Hazelton, A.Coauthors (2021). 2019 atlantic hurricane forecasts from the global-nested hurricane analysis and forecast system: composite statistics and key events. Wea. Forecast. 36, 519–538. doi:10.1175/WAF-D-20-0044.1
Hazelton, A.Coauthors (2022). Performance of 2020 real-time atlantic hurricane forecasts from high-resolution global-nested hurricane models: HAFS-globalnest and GFDL T-SHiELD. Wea. Forecast. 37, 143–161. doi:10.1175/WAF-D-21-0102.1
Hou, D.Coauthors (2014). Climatology-calibrated precipitation analysis at fine scales: statistical adjustment of stage IV toward CPC gauge-based analysis. J. Hydrometeor. 15, 2542–2557. doi:10.1175/JHM-D-11-0140.1
Huffman, G. J., Bolvin, D. T., Braithwaite, D., Hsu, K. L., Joyce, R. J., et al. (2020). “Integrated multi-satellite Retrievals for the global precipitation measurement (GPM) mission (IMERG),” in Satellite precipitation measurement. Advances in global change research. Editors V. Levizzani, C. Kidd, D. B. Kirschbaum, C. D. Kummerow, K. Nakamura, and F. J. Turk (Cham: Springer), 67, 343–353. doi:10.1007/978-3-030-24568-9_19
Iacono, M. J., Delamere, J. S., Mlawer, E. J., Shephard, M. W., Clough, S. A., and Collins, W. D. (2008). Radiative forcing by long-lived greenhouse gases: calculations with the AER radiative transfer models. J. Geophys. Res. Atmos. 113, D13. doi:10.1029/2008jd009944
Jarvinen, B. R., Neumann, C. J., and Davis, M. A. S. (1984). A tropical cyclone data tape for the North Atlantic Basin, 1886–1983: contents, limitations, and uses. NOAA Tech. Memo. NWS NHC 22. Coral Gables.
Jensen, T., Prestopnik, J., Soh, H., Goodrich, L., Brown, B., Bullock, R., et al. (2023). The MET version 11.1.0 user’s guide. Boulder, CO: Developmental Testbed Center. Available at: https://github.com/dtcenter/MET/releases.
Ko, M.-C., Marks, F. D., Alaka, G. J., and Gopalakrishnan, S. G. (2020). Evaluation of hurricane harvey (2017) rainfall in deterministic and probabilistic HWRF forecasts. Atmosphere 11, 666. doi:10.3390/atmos11060666
Landsea, C. W., and Franklin, J. L. (2013). Atlantic hurricane database uncertainty and presentation of a new database format. Mon. Wea. Rev. 141, 3576–3592. doi:10.1175/MWR-D-12-00254.1
Latto, A. S., and Berg, R. (2022). National hurricane center tropical cyclone report, hurricane Nicholas. Miami, FL: National Oceanic and Atmospheric Administration, National Hurricane Center. Available at: https://www.nhc.noaa.gov/data/tcr/AL142021_Nicholas.pdf (accessed on March 15, 2024).
Lin, Y., and Mitchell, K. E. (2005). “The NCEP Stage II/IV hourly precipitation analyses: development and applications,” in Preprints, 19th conf. On hydrology, 1.2. San Diego, CA: Amer. Meteor. Soc. Available at: https://ams.confex.com/ams/pdfpapers/83847.pdf.
Marchok, T. (2021). Important factors in the tracking of tropical cyclones in operational models. J. Appl. Meteor. Climatol. 60, 1265–1284. doi:10.1175/JAMC-D-20-0175.1
Marchok, T., Rogers, R., and Tuleya, R. (2007). Validation schemes for tropical cyclone quantitative precipitation forecasts: evaluation of operational models for U.S. Landfalling cases. Wea. Forecast. 22, 726–746. doi:10.1175/WAF1024.1
Matyas, C. J., Zick, S. E., and Tang, J. (2018). Using an object-based approach to quantify the spatial structure of reflectivity regions in hurricane isabel (2003). Part I: comparisons between radar observations and model simulations. Mon. Wea Rev. 146, 1319–1340. doi:10.1175/mwr-d-17-0077.1
McBride, J. L., and Ebert, E. E. (2000). Verification of quantitative preci-pitation forecasts from operational numerical weather predic-tion models over Australia. Weather Forecast. 15, 103–121. doi:10.1175/1520-0434(2000)015<0103:voqpff>2.0.co;2
Mehra, A., Tallapragada, V., Zhang, Z., Liu, B., Zhu, L., Wang, W., et al. (2018). Advancing the state of the art in operational tropical cyclone forecasting at NCEP. Trop. Cyclone Res. Rev. 7 (1), 51–56. doi:10.6057/2018TCRR01.06
National Hurricane Center (NHC) (2022). 2021 atlantic hurricane season summary table. Miami, FL: National Oceanic and Atmospheric Administration, National Hurricane Center. Available at: https://www.nhc.noaa.gov/data/tcr/2021_Atlantic_Hurricane_Season_Summary_Table.pdf (accessed on March 15, 2024).
National Hurricane Center (NHC) (2024). 2023 atlantic hurricane season reports. Miami, FL: National Oceanic and Atmospheric Administration, National Hurricane Center. Available at: https://www.nhc.noaa.gov/data/tcr/index.php?season=2023&basin=atl (accessed on March 15, 2024).
National Hurricane Center (NHC) (2023). 2022 atlantic hurricane season summary table. Miami, FL: National Oceanic and Atmospheric Administration, National Hurricane Center. Available at: https://www.nhc.noaa.gov/data/tcr/2022_Atlantic_Hurricane_Season_Summary_Table.pdf (accessed on March 15, 2024).
Newman, K. M., Brown, B., Gotway, J. H., Bernardet, L., Biswas, M., Jensen, T., et al. (2023). Advancing tropical cyclone precipitation forecast verification methods and tools. Wea. Forecast. 38, 1589–1603. doi:10.1175/WAF-D-23-0001.1
Qi, W., Yong, B., and Gourley, J. (2021). Monitoring the super typhoon Lekima by GPM-based near-real-time satellite precipitation estimates. J. Hydrol. 603, 126968. doi:10.1016/j.jhydrol.2021.126968
Rappaport, E. N.Coauthors (2009). Advances and challenges at the national hurricane center. Wea. Forecast. 24, 395–419. doi:10.1175/2008WAF2222128.1
Reinhart, B. (2022). NHC analysis and forecast challenges. Available at: https://hfip.org/sites/default/files/events/286/1030-reinhart-nhc-season-summary-and-forecast-challengespptx.pdf (Accessed February 23, 2024).
Rossa, A., Nurmi, P., and Ebert, E. (2008). “Overview of methods for the verification of quantitative precipitation forecasts,” in Precipitation: advances in measurement, estimation and prediction. Berlin, Heidelberg: Springer Berlin Heidelberg, 419–452.
Stackhouse, S. D., Zick, S. E., Matyas, C. J., Wood, K. M., Hazelton, A. T., and Alaka, G. J. (2023). Evaluation of experimental high-resolution model forecasts of tropical cyclone precipitation using object-based metrics. Wea. Forecast. 38, 2111–2134. doi:10.1175/WAF-D-22-0223.1
Tallapragada, V. (2016). “Overview of the NOAA/NCEP operational hurricane weather research and forecast (HWRF) modelling system,” in Advanced numerical modeling and data assimilation techniques for tropical cyclone prediction. Editors U. C. Mohanty, and S. G. Gopalakrishnan (Dordrecht: Springer). doi:10.5822/978-94-024-0896-6_3
Thompson, G., Rasmussen, R. M., and Manning, K. (2004). Explicit forecasts of winter precipitation using an improved bulk microphysics scheme. Part I: description and sensitivity analysis. Mon. Wea. Rev. 132, 519–542. doi:10.1175/1520-0493(2004)132<0519:EFOWPU>2.0.CO;2
Wang, C. (2014). On the calculation and correction of equitable threat score for model quantitative precipitation forecasts for small verification areas: the example of taiwan. Wea. Forecast. 29, 788–798. doi:10.1175/WAF-D-13-00087.1
Wang, W., Liu, B., Zhang, Z., Mehra, A., and Tallapragada, V. (2022). Improving low-level wind simulations of tropical cyclones by a regional HAFS. Res. Act. Earth Syst. Model, 9–10.
Wang, W., Sippel, J. A., Abarca, S., Zhu, L., Liu, B., Zhang, Z., et al. (2018). Improving NCEP HWRF simulations of surface wind and inflow angle in the eyewall area. Wea. Forecast. 33, 887–898. doi:10.1175/WAF-D-17-0115.1
Wolff, J. K., Harrold, M., Fowler, T., Gotway, J. H., Nance, L., and Brown, B. G. (2014). Beyond the basics: evaluating model-based precipitation forecasts using traditional, spatial, and object-based methods. Wea. Forecast. 29, 1451–1472. doi:10.1175/WAF-D-13-00135.1
Yu, Z., Chen, Y. J., Ebert, B., Davidson, N. E., Xiao, Y., Yu, H., et al. (2020). Benchmark rainfall verification of landfall tropical cyclone forecasts by operational ACCESS-TC over China. M 27. doi:10.1002/met.1842
Zhang, Z., Wang, W., Doyle, J. D., Moskaitis, J., Komaromi, W. A., Heming, J., et al. (2023). A review of recent advances (2018-2021) on tropical cyclone intensity change from operational perspectives, Part 1: dynamical model guidance. Trop. Cyclone Res. Rev. 12, 30–49. doi:10.1016/j.tcrr.2023.05.004
Zheng, W., Ek, M., Mitchell, K., Wei, H., and Meng, J. (2017). Improving the stable surface layer in the NCEP global forecast system. Mon. Wea. Rev. 145, 3969–3987. doi:10.1175/MWR-D-16-0438.1
Zhou, L., Harris, L., Chen, J., Gao, K., Guo, H., Xiang, B., et al. (2022). Improving global weather prediction in GFDL SHiELD through an upgraded GFDL cloud microphysics scheme. J. Adv. Model. Earth Syst. 14, e2021MS002971. doi:10.1029/2021MS002971
Keywords: HAFS, tropical cyclones, verification, quantitative precipitation forecasts, numerical modeling
Citation: Newman KM, Nelson B, Biswas M and Pan L (2024) Multi-season evaluation of hurricane analysis and forecast system (HAFS) quantitative precipitation forecasts. Front. Earth Sci. 12:1417705. doi: 10.3389/feart.2024.1417705
Received: 15 April 2024; Accepted: 09 October 2024;
Published: 05 November 2024.
Edited by:
Zhan Zhang, NCEP Environmental Modeling Center (EMC), United StatesReviewed by:
Srinivas Desamsetti, National Centre for Medium Range Weather Forecasting, IndiaAndrew Hazelton, University of Miami, United States
Timothy Marchok, NOAA Geophysical Fluid Dynamics Laboratory, United States
Copyright © 2024 Newman, Nelson, Biswas and Pan. This is an open-access article distributed under the terms of the Creative Commons Attribution License (CC BY). The use, distribution or reproduction in other forums is permitted, provided the original author(s) and the copyright owner(s) are credited and that the original publication in this journal is cited, in accordance with accepted academic practice. No use, distribution or reproduction is permitted which does not comply with these terms.
*Correspondence: Kathryn M. Newman, a25ld21hbkB1Y2FyLmVkdQ==