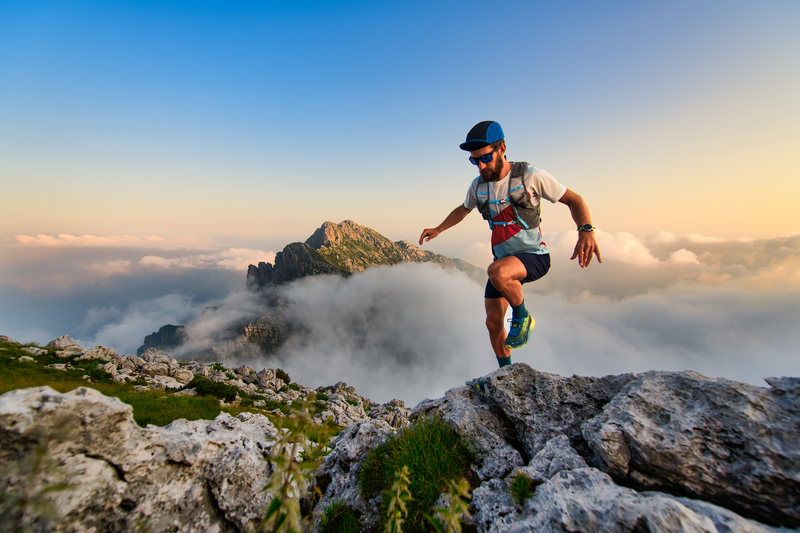
95% of researchers rate our articles as excellent or good
Learn more about the work of our research integrity team to safeguard the quality of each article we publish.
Find out more
ORIGINAL RESEARCH article
Front. Earth Sci. , 19 September 2023
Sec. Interdisciplinary Climate Studies
Volume 11 - 2023 | https://doi.org/10.3389/feart.2023.1231296
This study aims to assess the projected precipitation and temperature changes at the coastal karstic aquifer of Salento (Apulia, Southern Italy) under the Representative Concentration Pathway RCP4.5. For this purpose, an ensemble of twelve Regional Climate Models (RCMs) driven by several General Circulation Models (GCMs) were collected. Eight bias-correction (BC) methods were applied at daily time steps, and their results were assessed on monthly and annual time steps, using daily records from 19 and 11 precipitation and temperature (minimum and maximum) stations, respectively, for the period 1960–2005. Missing data in the observed dataset were filled-in applying the best performing techniques out of the 5 that were employed and tested. The Linear Scaling and the Power Transformation were found to be the most effective methods for precipitation BC at the case study, while all methods performed equally well in correcting air temperature datasets. Results of future climate projections show a decrease in precipitation of about 6% and an increase in temperature of 2°C until the end of this century, compared to the historical period (1971–2005). This study forms the first comprehensive attempt to test the scientific literature’s most widely used bias-correction methods over the study area. The case study may be considered a benchmark for circum-Mediterranean regions because of its high geomorphological and structural complexity, regional size, surface water scarcity, and significant water withdrawals for human activities.
Climate change impact studies on water resources systems need to be representative of the local climatic conditions. General Circulation Models (GCMs) are often affected by uncertainty predominantly due to low resolutions (approximately 100–250 Km) that inevitably lack regional details (Randall et al., 2007). Several downscaling methods have been developed to transform the large-scale information of GCMs to finer scales (25–50 Km), resulting in Regional Climate Models (RCMs) (Teutschbein and Seibert, 2012; Maraun, 2016). Mishra et al. (2023) demonstrated that increasing the horizontal resolution of RCMs (the high-resolution- 25 km-versus low-resolution- 50 km-) can improve the seasonal mean precipitation, temperature, circulation, frequency distribution of daily precipitation, and precipitation extremes over the complex region of India, although bias still occurs depending on the regions and the aspects investigated. Several authors discussed on the RCMs’ limitations (Christensen et al., 1998; Varis et al., 2004; Déqué, 2007; Teutschbein and Seibert, 2012), in terms of inaccurate seasonal precipitation patterns, overestimate wet days, or incorrectly yield extreme temperatures. For this reason, several bias-correction (BC) methods were developed to overcome the significant bias in RCMs, adapting simulated data to local observations in terms of mean and variance (scaling methods) or distribution probabilities. Lyra and Loukas (2023) implemented five BC methods to correct monthly precipitation and temperature data from an ensemble of GCM/RCM in the coastal agricultural Almyros Basin (Greece), demonstrating how bias-corrected simulated data still exhibits deviations from observations. They recommended the use of an integrated approach between BC methods and multi-model ensemble to define the best combination for hydrogeological modelling. Similar considerations were achieved by Tefera et al. (2023) in the Jemma sub-basin of the Upper Blue Nile Basin, which showed that BC methods affect the signal of climate change and extreme rainfall events with variable performance depending on the adopted technique. Recent studies utilise machine learning algorithms to minimise bias in RCMs simulations. Singh et al. (2023) used Autoencoder-Decoder and Residual Neural Network to successfully achieve bias-corrected simulated data of precipitation in India from CORDEX-SA domain and additionally to rescale the output to a finer resolution. Seo and Ahn (2023) compared the performance of empirical quantile mapping and the Long Short-Term Memory machine learning model for summertime daily rainfall simulation of Weather Research and Forecasting analysis in South Korea, concluding that despite quantile mapping performed better in terms of summertime mean and monthly rainfall, the machine learning algorithm reflects better the interannual precipitation variability. Therefore, using BC methods may introduce uncertainty in climate risk assessments due to the potential for diverse algorithms to yield varying impact results (Iizumi et al., 2017). Moreover, further uncertainty may be attributed to the accuracy of observation data (Kim et al., 2015) and the historical period used as a reference (Chen et al., 2015; Gampe et al., 2019; Li et al., 2010). The incompleteness of precipitation and temperature data is a frequent issue that can affect the quality and accuracy of subsequent analyses (Sattari et al., 2017; Armanuos et al., 2020). In the literature, missing data in time series may be managed on the basis of the two different categories in which they can be distinguished: very limited missing values and extensive or consecutive gaps (Aieb et al., 2019). For simplicity, very limited missing values can be excluded from the dataset (Song et al., 2008). However, this procedure could introduce an additional bias in successive analyses. For the latter category, many imputed missing value methods are available (Li et al., 2007), the effectiveness of which can vary depending on various factors, i.e., the percentage of missing values, the mechanism of data loss, the considered variables, and the respective correlations.
Through uncertainty analysis, Senatore et al. (2022) demonstrated in a catchment located in Southern Italy that the primary source of uncertainty is introduced by GCMs, followed by RCMs and applied BC methods. Noto et al. (2023b) argued that the intricate sequence of modelling procedures, i.e., emission scenarios, climate models, downscaling and/or bias-correction techniques, and hydrological models, involves a certain level of uncertainty that spreads throughout the entire process, resulting in divergent and occasionally unexpected outcomes. Thus, an ensemble of RCM simulations and field observation data may be considered together with different bias-correction methods to simultaneously evaluate the uncertainties of each simulated dataset and the performance of every technique (Fantini et al., 2018). This recommendation is particularly relevant when the hydrological variables are used to investigate future impacts in areas highly vulnerable to climate change, such as the Mediterranean basin (Giorgi and Lionello, 2008; IPCC, 2014).
The impacts of climate change in regions surrounding the Mediterranean are identified as the most critical (Noto et al., 2023a) since water shortage is a crucial obstacle. Generally, the coastal aquifers facing these areas are typically the ones where groundwater resources are needed for their economic sustainment, as they are by their nature geologically, structurally, or climatically characterised by a lack of water resources. The worrying trend of rising temperatures, increasing frequency and severity of drought periods, and decreasing amounts of annual rainfall will compromise the already critical condition of groundwater reserves with consequent socio-economic implications (Linares et al., 2020). From one perspective, these expected trends may affect groundwater availability. Sordo-Ward et al. (2019) pointed out that current water shortage problems are expected to exacerbate in the future in many southern European basins in Portugal, Spain, France, Switzerland, Italy, Greece, Macedonia, Bulgaria, and Turkey. By simulating the application of adaptation policies for water resources management for irrigation, they showed a potential improvement in facing the effects of long-term climate change. Pardo-Iguzquiza et al. (2019) investigated the effect of climate change on the karst aquifer recharge in the Sierra de las Nieves (southern Spain) for the period 2071–2,100 based on the RCP8.5 scenario, estimating a potential reduction in average recharge of around 53%.
Moreover, the economic sector may witness an increase in drought and heat stress, altering food provision and energy production and affecting human health. For example, Abd-Elmabod et al. (2020) evaluated the future impacts of climate change under the A1B scenario (i.e., a balanced scenario based on the assumption of similar improvement rates for all energy supply and end-use technologies) on land capability and yield reduction of wheat and sunflowers in Andalucia (Southern Spain) demonstrated a decrease in sunflowers production due to their sensitivity to projected increasing temperature. Lange (2019) suggested that the water, climate, development and political stability challenges of climate change in the MENA region (the Middle East, including the Eastern Mediterranean region and North Africa) require an integrated approach through the creation of a regional cooperation and a joint research and stakeholder community to plan adaptation and mitigation strategies (e.g., increased resource use efficiency, integrated technology assessments for power generation, and increased reliance on renewable/solar technologies) to address water and energy scarcity and ensure a secure and sustainable future for Mediterranean societies.
In this context, Apulia (Southern Italy) can be considered a benchmark of such issues because it is a region with a geographical position and geomorphological characteristics prone to hydro-climatic hazards accelerated by prolonged drought periods and increasing temperatures. At the same time, the region lacks surface water bodies to cover domestic, agricultural, industrial, and environmental needs. Therefore, water demands are satisfied solely by groundwater abstracted from the regional karstic aquifer through numerous production wells. Groundwater overexploitation leads to aquifer depletion and dramatic quality deterioration due to seawater intrusion, accelerated by climate change, overall creating alarming conditions for water security. Despite the criticality of the condition, few studies are elaborated on assessing future climate scenarios and their relative impacts on the region. Kapur et al. (2007) predicted an increasing trend in temperature and evapotranspiration and a non-significant change in annual precipitation until 2,100, with consequent implications on agricultural productivity (i.e., increasing crop water demand, higher use of pesticides, reduced volume in the water supply system). The projected variations, also confirmed by D’Oria et al. (2018), should negatively impact water resources, especially in a complex semi-arid area of high-water demand that is covered by a highly vulnerable and already deteriorated aquifer system, where a deficient water budget has been established. High vulnerability, coupled with the alarming results of the reported climate parameters’ evolution and their consequent impacts on the region, calls for in-depth investigation at a local scale to substantiate and detail climate change projections that will drive awareness raise and support planning of mitigation and adaptation measures. To discuss the future hydrological impacts of climate change on a local scale, the Salento aquifer, a regional coastal karstic system located in the southern part of the Apulia region, was selected. Due to its vulnerability which is driven by its hydroclimatic conditions and hydrogeological setting, and the criticality of water security to the socio-economic welfare of the region, Salento may be considered a reference case study for the Mediterranean zone.
We tested five methodologies to infill missing data in the observations’ dataset, evaluating their performance to select the most efficient for precipitation and temperature variables. Secondly, projected daily precipitation and minimum and maximum temperature data were selected from an ensemble of twelve RCMs belonging to the EURO-CORDEX domain (Jacob et al., 2014). Using the filled daily meteorological observations (i.e., precipitation and temperature), eight BC methods were implemented to improve the RCMs’ simulations in terms of local climate variability representation. Although the BC methodologies adopted are now well established in the scientific literature, this study represents the first comprehensive attempt to examine the most appropriate and widely implemented techniques towards compiling a well-founded selection strategy. Two different approaches may be adopted to analyse the performance of BC methods in future climate scenarios. In climatology, the projected bias-corrected data over a future period are usually compared to the original incorrect simulations as the main focus is to investigate how BC algorithms influence historical and projected climate data (Dieng et al., 2022); in contrast, from a hydrogeological impact assessment point of view, these are typically compared with historical observations (Pfeifer et al., 2015; Arampatzis et al., 2018; Sperna Weiland et al., 2021). In this study, the corresponding bias-corrected results were compared with observed data on a monthly and annual step for the historical period (1971–2005) to assess RCM and BC performance. After selecting two-time windows for near to medium (2031–2060) and distant (2071–2,100) future, trend analyses were performed comparing the projected with the corresponding precipitation and temperature data of 1971–2005 on monthly and annual time steps. Finally, the potential impacts of precipitation and temperature projected changes over the study area were discussed in a qualitative aspect, as quantified analysis through hydrogeological modelling is out of the scope of this investigation.
This work aims to develop an integrated approach to investigate the potential implications of climate change on the hydrological cycle of coastal aquifers with a higher degree of reliability through a comprehensive assessment of climate projections and BC techniques. Moreover, it forms a prelude in estimating future meteorological time series, constituting the hydrological input data for subsequent analysis. Focusing on assessments based on local scale, this approach is proposed to be valid and of interest for catchments located in the Mediterranean basin. This is essential for developing an understanding of the system’s evolution, increasing institutional and societal awareness of the risks to which water resources are exposed, and designing feasible solutions that enhance resilience and safeguard their security.
Section 2 of the paper is devoted to a general description of the study area, the observational datasets used, and the methodologies adopted; Section 3 presents the results of infilling techniques and BC methods, whilst Section 4 summarises the main findings, looking at their potential effects on the water resource management of the Salento aquifer system.
Salento study area is part of the Apulia region, a peninsula located in southeastern Italy. It covers ca 2,760 km2 and extends from the Ionian to the Adriatic Sea (Figure 1). This domain is almost flat, with an average elevation of 100 m AMSL; a few gentle hills rise in the southern part, coinciding with the main recharge area. Ephemeral and poorly incised streams cross the area since they are mainly dry, and surface runoff is mainly occurring in response to intense precipitation events. The geological setting comprises multi-layered litho-stratigraphic units of Cretaceous age (De Filippis et al., 2019), dislocated in Horst and Graben structures. The deep aquifer, whose extension coincides with Salento, lays on the carbonate basement, with groundwater principally flowing in unconfined conditions and freshwater floating above salt water. The dominant hydraulic behaviour of the deep aquifer in response to recharge processes is the baseflow, which confers great inertia and high storage capacity (Balacco et al., 2022b). Mediterranean climate characterises the Salento area with mild, wet winters and hot, dry summers. Mean annual precipitation is about 600 mm (Portoghese et al., 2005), mostly occurring during the autumn-winter season. Several moderate to severe drought events have affected Salento in the last 70 years (Alfio et al., 2020). Agriculture and tourism are the main economic activity sectors in Salento that are both groundwater dependent, in absence of sufficient surface water resources. Consequently, due to the vulnerability of the karstic system to climate change, the major economic activities of the region are also climate dependent.
Daily historical climate observations were provided by the Civil Protection of the Apulia Government (www.protezionecivile.puglia.it) for 22 precipitation and 19 temperature stations from 1960 to 2005 (except SAL_MS6 and SAL_MS14, which date from 1971 to 2005). From this database, 19 precipitation (Pr) and 11 temperature (maximum–Tmax-, and minimum–Tmin-) stations were selected and used due to their consistent time series with less than 7% missing values. Figure 1 illustrates the spatial distribution of the selected meteorological stations over the study area, and the main characteristics detailing missing value percentages are reported in the Supplementary Material.
This study implements five imputation methods for missing values filling in daily Pr, Tmax, and Tmin datasets, evaluating their performances using Pearson’s coefficient (R), Nash-Sutcliffe Efficiency (NSE), and Similarity Index (SI). The adopted methods are briefly described in the following paragraphs. Hereafter, target and reference stations refer to the meteorological station with missing values and those selected to estimate them, respectively.
Mean Substitution method (MS) is the most straightforward imputation method. It replaces the daily missing value at the target station with the corresponding mean of the values recorded in the selected reference stations. Aissia et al. (2017) suggested that the MS technique applies when missing values are less than 10%.
Thiessen polygons (TH) is one of the most widely applied methods in hydrology, assigning to each meteorological station a weight factor proportionate to the area for which it is representative. It assumes that meteorological parameters are constant in each Thiessen polygon and equal to the figure recorded in the station. In this case, missing values correspond to the values from the polygon of the reference station in which the target station occurred.
In the Inverse Distance Weighting (IDW) method, missing values at the target station correspond to a weighted average of the data at the reference stations. The weighting parameters depend on the spatial distances from the target station. This method is appropriate for highly correlated data.
Multiple Imputation by Chained Equations (MICE) is an advanced method which accounts for multiple sets of plausible imputed values through a prediction model built on already available data in the reference stations (Zhang, 2016). This procedure relies on three main steps (Buuren and Groothuis-Oudshoorn, 2011), starting by substituting missing data at the target station with a set of imputed values drawn from a distribution fitted to the reference time series; the linear regression parameters of each set of the imputed dataset are then estimated and pooled following Rubin (1987), resulting in the final linear regression parameters with which missing values are imputed. Two MICE approaches were employed in this study, namely, the Predictive Mean Matching (MICE_pmm, Aguilera et al., 2020) and the Random Forest (MICE_rf, Jing et al., 2022).
The iterative procedure for selecting the reference stations and infilling missing values was performed in R software. A recording period without missing values for all stations (1971–1976 for precipitation and 2000–2002 for temperature) was identified since the analyses can be performed on a subset of the dataset without missing data. Successively, a rate of 10% of data was randomly assumed to be missing since the highest missing value percentage found in the dataset was about 7%. Missing data distribution in each target station was then filled by selecting a set of reference stations close to those affected by missing values according to climatic and physical behaviour. In fact, being a peninsula facing the Adriatic Sea on one side and the Ionian Sea on the other, the weather conditions are not influenced solely by altitude, as is generally the case inland, but also by other factors such as proximity to the sea. The temperatures in Salento are significantly influenced by the mitigating presence of the Ionian and Adriatic Seas, whose winds blowing on both sides establish distinct weather conditions. Consequently, the selection of reference stations for each target station was performed setting four climatic and physical criteria to be met: (i) a Pearson’s correlation coefficient R greater than 0.70 between the target and reference stations’ recordings, (ii) a distance of less than 20 Km between them, (iii) an altitude difference of less than 80 m, and (iv) exposure to the same sea (Adriatic or Ionian). As a result, the algorithm selects the nearest reference station that shares a comparable elevation with the target station, is exposed to the same sea, and exhibits a strong correlation with the target. An algorithm of 1,000 iterations was then applied for each method setting as convergence criterion a difference of less than 0.01 (mm for precipitation and °C for temperature data). Finally, the metrics (R, NSE, and SI) were calculated between observations and estimated data over the specified time interval to check the performance and determine the optimal data-filling approach.
In this study, simulated precipitation, minimum and maximum temperature raw data derived from the EURO-CORDEX initiative were used (Jacob et al., 2014), in which several GCMs, resulted from the CMIP5 project (Coupled Model Intercomparison Phase 5; Taylor et al., 2012), were dynamically downscaled and provided with a resolution of 0.11°. An ensemble of 12 RCMs was analysed to account for the uncertainty of the climate model projections. The selected RCMs have been widely applied in climate change impact assessment studies in the Mediterranean region (D’Oria et al., 2018; Senatore et al., 2022; Peres et al., 2020). This selection represents a sufficiently varied range of RCM and GCM combinations, as it encompasses 7 RCMs driven by 4 distinct GCMs. Only the results of the RCP4.5 intermediate scenario for 1960–2005 and 2006–2,100 were considered, as it refers to a stabilisation scenario assuming the invocation of climate policies to achieve the goal of limiting emissions and radiative forcing (Thomson et al., 2011). The more plausible outcomes are reflected by RCP4.5 considering the current (and, to a certain degree, pledged) policies (Hausfather and Peters, 2020). In contrast, RCP8.5 is a high emissions scenario, representative of the wide range of non-climate policy scenarios (van Vuuren et al., 2011). Table 1 lists the model used, renamed with an acronym comprising two letters referring to the GCM name and two to the RCM name.
TABLE 1. List and acronyms of used GCM-RCM combinations. 1R Colin et al., 2010, Herrmann et al., 2011; 2R Rockel et al., 2008; 3R Christensen et al., 1998; 4R van Meijgaard et al., 2008; 5R Samuelsson et al., 2011, Kupiainen et al., 2011; 6R Jacob et al., 2012; 7R Skamarock et al., 2008; 1G Voldoire et al., 2013; 2G Hazeleger et al., 2010; 3G Giorgetta et al., 2013; 4G Dufresne et al., 2013.
Eight precipitation and seven temperature BC methods were applied to the selected RCMs data using historical daily climate observations from 1960 to 2005 (Table 2). The simulated values corresponding to the observed data positions were extracted from the RCM grid using the nearest-neighbour interpolation technique (Matiu and Hanzer, 2022). The results were analysed on a monthly and annual basis and compared to the corresponding precipitation and temperature data of 1960–2005. Taylor diagrams (Taylor, 2001) were used to analyse and depict the performance of each BC method since they incorporate correlation coefficient, centred root mean square difference and standard deviation into a single graph. BC methods were applied using the well-developed and tested climate4R (Iturbide et al., 2019) and downscaleR (Marsh et al., 2018) libraries for R software. The correction factor for daily data in all methods is based on a sliding window (Smitha et al., 2018), referring to 31 days. The BC methods briefly reported below are generally distinguished into (i) scaling and (ii) distribution adjustment techniques. Scaling methods include the Linear Scaling method (LS), the Local Intensity scaling of precipitation (LOCI), the Power Transformation of Precipitation (PTR), and the Variance scaling of temperature (VAR). In contrast, Empirical Quantile Mapping (EQM), Parametric Quantile Mapping (PQM), Generalised Quantile Mapping (GPQM), Detrended Quantile Matching (DQM), and Quantile Delta Mapping (QDM) belong to the second category.
The LS method consists of scaling simulated data with a multiplicative or additive factor calculated as the difference/ratio between the observed and the simulated mean in the reference period (Lender and Buishand, 2007). The multiplicative factor is typically used with variables with a lower bound (e.g., precipitation or wind speed), while the additive is preferably applicable to unbounded variables (e.g., temperature).
The LOCI technique (Schmidli et al., 2006) is an improvement of the LS approach because it involves the adjustment of the mean as well as both wet day frequencies and intensities of rainfall time series, by setting a common precipitation threshold for all considered stations (Pthres= 0.20 mm for the Salento case study) such that the number of simulated days exceeding this threshold matches the number of observed days.
While LS and LOCI account for the bias in the mean precipitation, the PTR and the VAR approach adjust the variance and the mean of raw RCM data. The PTR introduced by Leander and Buishand (2007) uses an exponential function permitting changes in the mean and the standard deviation of the dataset. It is designed only for precipitation data due to the power function applied. The VAR is the complementary approach for temperature data, developed to correct the mean and variance of normally distributed variables (Chen et al., 2011a; Chen et al., 2011b).
In contrast, the quantile mapping BC methods commonly correct bias between simulated and observed data, equating cumulative distribution functions (CDFs) of both datasets. The EQM method fits the CDF of simulated data to that of the observations, effectively correcting bias in the mean, standard deviation, and quantiles. This technique also adjusts the overestimation of wet or dry day frequency (defined as days with precipitation above or below Pthres in the observation dataset) following Themeßl et al. (2012) and Wilcke et al. (2013).
The PQM algorithm uses a theoretical distribution calibrated over the training period. Usually, the Gamma distribution applies to precipitation (Piani et al., 2009), while the Gaussian distribution is appropriate for temperature data (Collados-Lara et al., 2018). As for EQM, the overestimation of wet or dry day frequency is assumed considering the above-cited Pthres.
Gutjahr and Heinemann (2013) proposed the GPQM technique, which uses two theoretical distributions, i.e., the Gamma distribution to values under the threshold given by the 95th percentile and a general Pareto distribution to values above the threshold. This threshold is the 95th percentile of the observed and predicted wet-day distribution. For temperature data, the general Pareto distribution relates to values below the fifth percentile and the Normal distribution to the rest of the values. The wet-day frequency adjustment is also considered. This method aimed to adjust the extreme and non-extreme values separately.
The QDM method (Cannon et al., 2015) preserves the change signal in the simulated quantiles of variables, considering a bias-corrected value term obtained from the observations dataset and the relative change term (delta) obtained from the simulated data. Therefore, model projections are firstly detrended per quantile, and quantile mapping is applied to correct systematic distributional biases compared to the observations. Secondly, the removed projected trends are restored to the bias-corrected quantiles.
The DQM algorithm is similar to the previous method. It applies the EQM to the detrended series and then reapplies the mean trend to the bias-adjusted series. It preserves the long-term mean signal in a climate change context. More details and differences between QDM and DQM are explicitly provided by Cannon et al. (2015).
Choosing the most effective BC method is challenging because different statistical metrics may lead to inconsistent results (Gado et al., 2022). In this framework, the compromise programming proposed by Zeleny (1973) was applied to determine the most effective BC methods and evaluate the future scenario. It consists of measuring the distance of each method from the ideal value of the selected metrics and opting for the minimum one as the best method. The distance
where J is the number of the metrics used,
Five methods for imputed missing values in the hydrological time series of the Salento case study were evaluated in terms of the number of iterations for reaching the threshold value set in two successive steps of filling, and the main metrics (i.e., R, NSE, and SI). A preliminary analysis of the precipitation and temperature datasets preceded to calculate the percentage of missing values and investigate the presence of a potential trend. The rate of missing values in the dataset is very low, with a maximum value of 7% for precipitation and 5% for temperature. No trend was detected on an annual and monthly scale for both climate variables. Figure 2 presents the resulting performance of each missing value-filling methodology. The methodologies were applied on a daily scale in the reference period and evaluated monthly. In the case of precipitation, which is a random signal, the estimated daily values differed from the observed ones, particularly on days with higher precipitation. However, when examining the data on monthly aggregates, the results were accurate, which is further confirmed by the robust metrics acquired. Supplementary Figure S3 in Supplementary Material illustrates the monthly comparison between the observed and estimated amount of precipitation, as well as the maximum and minimum temperatures, for each of the data interpolation methods used during the reference period for Lecce meteorological station. It is worth noting that all methods perform effectively in the task of filling in missing data, as indicated by the significant R and SI coefficients calculated, along with the NSE coefficients. The number of iterations for reaching the convergence criterion of 0.01 mm for precipitation and 0.01°C for temperature is also minimal, especially for temperature. In the case of precipitation, some methods need more steps to reach convergence (Thiessen and IDW). Based on a combined evaluation of the metrics and the number of iterations to reach convergence, the MICE_pmm and the MS methods were selected for filling-in missing data in the precipitation and temperature daily time series, respectively.
FIGURE 2. Number of iteration boxplots and final R, NSE, and SI coefficients for each filling method referring, respectively, to Pr (top side), Tmax (middle side) and Tmin (bottom side).
In this study, an ensemble of 12 RCMs was analysed, and several BC methods were applied to account for uncertainty due to climate projections. Since the Salento aquifer has a great storage capacity and exhibits a slow response to precipitation and temperature variability (Balacco et al., 2022b), we focused on the monthly and annual assessment of the results. Furthermore, although the analyses were carried out for each station, the results reported refer to the ensemble’s average values for characterising the climate trend on a regional dimension.
AL_CN and WF_IP were excluded from precipitation analyses because their performance was significantly inconsistent compared to the observations, even after applying the BC methods. Although the seasonality is largely reproduced, the uncorrected monthly average precipitation of the other selected RCMs significantly deviates from the observed ones. In fact, raw data tend to overestimate spring-summer depths and underestimate autumn-winter ones.
Figure 3 shows the Taylor diagrams compiled by raw and bias-corrected RCM precipitation from 1971 to 2005 on a monthly interval. They are normalised to the standard deviation of the observed data and expressed as mm/month. Thus, bias-corrected RCMs showing high correlation coefficients and standard deviations closer to one represent datasets more similar to the observed patterns. Correlation coefficients of raw RCM datasets range between 0.15 (RC_IP) and 0.29 (CC_CN and RC_CN). After bias correction, they improve among each RCM and BC method, especially for LS, EQM, PQM, and PTR. The standard deviation varies between 0.58 mm/month (RC_IP) and 1.05 mm/month (RC_IC) for raw data, while LS, EQM, LOCI, and PTR display values closer to one after the bias correction of almost all RCMs. Taylor diagrams of maximum and minimum temperature are included in the Supplementary Material (Supplementary Figure S1, S2, respectively). They clearly demonstrate that all raw RCMs present high values of correlation coefficients, ranging between 0.94 and 0.96 and standard deviations varying from 0.80°C/month to 1.13°C/month. Applied BC methods improve the standard deviation more than the correlation coefficient. No significant difference in the performance between RCMs and BC methods was identified.
FIGURE 3. Taylor diagrams of monthly raw and bias-corrected RCMs precipitation data for the period 1971–2005.
The calculated metrics for monthly precipitation and temperature data, i.e., RMSE, RHO, NSE, and BIAS, were reported in Supplementary Material, for brevity (Table 2–4). Regarding precipitation, results differ among each RCM and BC method. It is noted that LS, EQM, LOCI, and PTR generally show better coefficients compared to the other more sophisticated techniques, especially in terms of percent bias error. In contrast, temperature outcomes confirm that all methods perform well in adjusting raw simulated data, and no significant deviations between RCMs can be detected.
Figure 4 highlights the monthly precipitation mean values of raw and bias-corrected simulated data for 1971–2005. Raw and bias-corrected RCM simulations were compared with the historical observations (dashed line). The monthly precipitation averages of raw RCM data demonstrate that, in general, RCMs underestimate monthly precipitation, especially during autumn months, when the underestimation is considerable. After BC, the monthly bias-corrected precipitation of each ensemble member is comparable with that of the observation data, with the best fit presented for LS, EQM, and PTR techniques. GPQM, DQM, and QDM present more significant variability than the observed trend, with values usually overestimating monthly precipitation, especially during the wet period. Conversely to precipitation results, all 12 RCMs were considered for the temperature dataset. The monthly averages of the bias-corrected minimum and maximum temperature of each RCM perform well compared to the observed data for all BC methods (Figure 5). Raw RCM matched the observed data well (except for WF_IP), capturing at least the pattern; after the BC, bias was considerably reduced.
FIGURE 5. Average monthly maximum (left-hand side) and minimum temperature (right-hand side) of raw and bias-corrected RCM data for 1971–2005.
Compromise programming (CP) results were used to select the most suitable bias-corrected data for assessing projected precipitation and temperature trends. Figure 6 highlights the results of CP for precipitation in terms of mean values of Lcp. The most efficient methods are LS, PTR, and LOCI. Nevertheless, LOCI was excluded from the future trend analysis because there are still some considerable biases in monthly average precipitation after BC, as illustrated in Figure 4. Regarding temperature, all BC methods perform well. For the sake of brevity, LS and VAR methods were selected to discuss future trends in temperature.
Precipitation and minimum and maximum temperature datasets provided by each raw and bias-corrected RCM for the entire projection period 2006–2,100 were divided into two 30-year intervals (2031–2060, 2071–2,100) and compared with the reference period 1971–2005 on monthly and annual intervals. The correction factors assessed in the reference period for each BC method were used to correct the bias in the scenario period, as usually considered in climate studies. To evaluate the effect of the selected methods on future projections, the mean annual amount and the change signal were calculated for each RCM in the two 30-years periods from the observed reference. Specifically, the climate change signal refers to the percentage difference between future raw and bias-corrected RCMs output for precipitation, and deviations for temperature, from the reference period. Results reported in Table 3 and Table 4 refer to the average ensemble of all station time series. Figures 7, 8 show the boxplots and climate change signals of the annual precipitation of raw and bias-corrected RCMs, for brevity. Temperature results are instead shown in the Supplementary Material (Supplementary Figures S4–S7).
FIGURE 7. Boxplots of the annual precipitation of RCM simulations with (LS, PTR) and without (RCP4.5) bias correction for 2031–2060 (in blue) and 2071–2,100 (in orange). In the boxplots, whiskers indicate the minimum and maximum value of precipitation; the horizontal lines refer to the 25th percentile, median, and 75th percentile from the bottom to the top of each boxplot, and the point symbols represent outliers.
FIGURE 8. Climate change signals of annual precipitation of RCM simulations with (LS, PTR) and without (RCP4.5) bias correction for 2031–2060 (in blue) and 2071–2,100 (orange).
It has been revealed that, among the others, LS and PTR adapt simulated data of the selected RCMs to local observations in terms of monthly mean precipitation during the reference period (1971–2005). However, when evaluating the future projections, the raw RCMs consistently project a reduction in the mean value in both periods, except for RC_CN and RA_IC, which show higher mean precipitation (Figure 7). In contrast, bias-corrected simulated data overestimate the mean annual amount in most cases (e.g., CC_CN, CC_IC, CC_MP, HI_IC, RC_IP, RE_MP). Both methods appear to be appropriate in preserving the climate change signal of most of the RCMs in 2031–2060 and 2071–2,100 (Figure 8). Few exceptions are CC_CN, for which LS and PTR triggered a change in the negative climate change signal of raw data into a positive one in 2071–2,100, and RA_IC, which experienced a changing trend from positive to negative in 2031–2060. In general, all bias-corrected RCMs attenuate the climate change signals compared to raw data. Different results can be detected for minimum and maximum temperature, for which all raw simulations project an increase in the mean value in both periods compared to the reference period, except for RA_IC, RC_CN, and RC_IC which display a slight reduction in minimum temperature. However, when referring to bias-corrected data, LS and VAR tend to overestimate the mean annual temperature in most cases. LS and VAR preserve the positive climate change signals in both periods, except for RA_IC, RC_CN, RC_IC, and RC_MP for which BC methods triggered a change from negative to positive climate change signals.
Figure 9 shows the temporal variation in annual precipitation (blue line) on the top, and the monthly precipitation change on the bottom, resulting from the average of the 10 RCMs, bias-corrected with LS and PTR for 2031–2060 and 2071–2,100. The results indicate a wide interannual variation of average precipitation, while the 95% confidence interval (light blue area) range of about 200 mm reveals the considerable degree of uncertainty around projected annual precipitation, introduced by the different climate realisation presented from the RCM-GCM combinations. For the near to medium future, the data revealed that the annual precipitation trend was relatively stable, with no clear indication of a significant increase or decrease. This outcome was consistent across both LS and PTR methods, as indicated in Figure 9. In contrast, in 2071–2,100, a slightly positive trend in annual precipitation became more noticeable. However, bias-corrected data showed consistently lower values for both periods when compared to historical observations, pointing towards a potential decrease in precipitation over time. Table 3 summarises the above-presented results. Annual precipitation for the Salento study area decreases by 6.4% and 5.9% for LS and 5.9% and 4.7% for PTR in 2031–2060 and 2071–2,100, respectively, compared to the historical period (1971–2005). The monthly precipitation change is mainly negative in the short and long term, with some exceptions during the late spring-early summer period (April-June), which appears to be increasing (Figure 9).
FIGURE 9. Average annual precipitation and monthly precipitation change (bold blue line) with 95% confidence interval (light blue area) based on the data of the 10 RCMs, bias-corrected with LS and PTR methods for the period 2031–2060 and 2071–2,100, respectively. Orange line indicates the trend, and the light orange area refers to its 95% confidence interval. Annual precipitation for LS (top left-hand side), PTR (top right-hand side); monthly precipitation change for the two selected periods for LS (bottom left-hand side) and PTR (bottom right-hand side).
Table 4 summarises the annual average bias-corrected results of maximum and minimum temperatures for all BC techniques referring to the RCMs ensemble. Figure 10 highlights the temporal variation in maximum temperature (blue lines), resulting from the 12 RCMs’ average bias-corrected with LS (A) and VAR (B) for 2031–2060 and 2071–2,100, respectively. The linear trend (orange lines) outlines an increasing pattern for both periods. In contrast to precipitation results, air temperature simulations show a limited interannual variation of average temperature and moderate deviations in annual temperature projections resulting from all the selected RCMs (light blue area). The average annual maximum temperature resulting from bias-corrected data was around 22°C in 2031–2060°C and 22.6°C in 2071–2,100, demonstrating a high difference from the observed average in 1971–2005 (20.5°C). No significant deviation among the BC methods was detected (Table 4). Similarly, the annual minimum temperature resulting from bias-corrected data was around 14.1°C in 2031–2060°C and 14.8°C in 2071–2,100 compared to the value of 12.9°C in 1971–2005. Therefore, higher future maximum and minimum temperatures were estimated. The annual maximum and minimum temperatures increased compared to the reference period by 1.4°C and 1.3°C on average (2031–2060), respectively. Considerable increase was detected for the period 2071–2,100 (2.1°C and 1.9°C for maximum and minimum temperatures, respectively). On a monthly scale, maximum and minimum temperatures for almost all months in the two analysed time windows increase, especially during summer. For brevity, Figure 10 (bottom side) represents the monthly change results for the maximum temperature in the two periods and for LS and VAR since the results for the minimum temperature were similar.
FIGURE 10. Average maximum temperature and monthly maximum temperature change (bold blue line) and 95% confidence interval (light blue area) based on the data of the 12 RCMs, bias-corrected with LS and VAR methods for the period 2031–2060 and 2071–2,100, respectively. Orange line indicates the trend, and the light orange area refers to its 95% confidence interval. Annual precipitation for LS (top left-hand side), VAR (top right-hand side); monthly precipitation change for the two selected periods for LS (bottom left-hand side) and VAR (bottom right-hand side).
Figure 11 shows the projection of the total annual precipitation and minimum and maximum temperature in 2015–2,100 of the RCM ensemble’s average values bias-corrected through the LS method. The results are represented in terms of a 10-year moving average to mitigate natural variability and emphasise the climate change signal. The median values of the climate models (blue line) reveal no significant changes in precipitation time series in the future, whilst the variability between the RCMs (blue shadow) indicates high uncertainty in the climate projections. In contrast, the temperature projections suggest a progressive warming in the region under investigation.
FIGURE 11. Annual precipitation (left-hand side), annual maximum temperature (middle side) and minimum temperature (right-hand side) in terms of 10-year moving average in the period 2015–2,100 according to the RCP4.5 scenario.
The future patterns of precipitation and temperature were assessed for a Mediterranean aquifer, which is strongly affected by frequent and severe drought events (Alfio et al., 2020) and prone to hydro-climatic hazards. Several BC methods were applied to the RCMs to consider each simulated dataset’s uncertainties and the performance of every methodology. Then, the most suitable BC methods and RCMs for precipitation and temperature data were selected, and future trends until the end of this century were determined.
Missing data in meteorological datasets remains a recurrent problem, and their accurate filling is a difficult task, especially when the available meteorological stations are scarce compared to the size of basins (Aguilera et al., 2020). We computed an iterative procedure by testing five techniques to fill-in missing data in the observed dataset, obtaining satisfactory results for all methods. This outcome is probably related to the pre-screening performed in selecting the reference stations, which required a higher correlation coefficient, a fair distance and elevation difference, and exposure to the same sea compared to the station containing missing values. Therefore, the criteria established for the selection of the reference stations in the context of the present study can be considered for precipitation and air temperature data imputation in areas with similar hydroclimatic setups. Finally, Multiple Imputation by Chain equations proved a suitable method for filling-in missing hydrological values. Turrado et al. (2014) obtained excellent results in estimating daily solar radiation in the Galicia catchment (Spain) compared with other methods like Ordinary Kriging; Wesonga (2015) used it to handle incomplete wind speed time series registered in the Entebbe International Airport (Uganda). Abdullah et al. (2022) studied extreme temperature and rainfall events in Bangladesh after using the MICE technique and implementing the predictive mean matching algorithm to fill in missing daily values. MS method was selected for filling-in missing data in the temperature daily time series; however, all tried methods performed equally well. Being aware that the evaluation of data-filling techniques should encompass both their ability to capture average values over time and their capacity to handle extreme events, in this research, however, the analysis of extreme events has been neglected, as the focus of the work is to understand the broader future trends monthly and yearly. Moreover, a substantial amount of data may be required to accurately analyse extreme events. In this case, the timeframe used for evaluating the filling-in method performance (1971–1976 for precipitation and 2000–2002 for temperature) is unfeasible for extreme event assessment.
The selected models’ ensemble can simulate temperature better than precipitation, and this outcome agrees with the results of Peres et al. (2020), which refers to the entire southern Italy. Thus, the whole ensemble was considered for temperature analyses. At the same time, we excluded AL_CN and WF_IP models from precipitation analysis since the climatic seasonality of the study area was not well reflected, even after the application of BC methods. This approach could be valid for climate change projection studies at regions in the mid-latitudes characterised by Mediterranean climate (i.e., mild, wet winters and hot, dry summers). However, its applicability should be thoroughly assessed.
Improving simulated raw precipitation data is more complex than temperature in the bias correction context. In this study, all BC methods slightly improve the RCM datasets, but there are significant deviations in reproduction of the observed characteristics, especially in the case of precipitation (Fang et al., 2015). In general, the identified RCMs are sufficiently representative of the precipitation and temperature conditions, although a significant variation exists among them compared to the observed time series. The LS and the PTR algorithms could finally be considered the best BC methodologies for precipitation time series for the case study, while quantile methods (i.e., GPQM, DQM, and QDM) tend to overestimate monthly precipitation, especially during the wet period. On the contrary, in the case of temperature datasets, all methods perform satisfactorily, and the results agree with the observation time series. Although BC methods adjust model output to fit observations and reduce systematic errors, the underlying assumptions of the various techniques are often questionable and, therefore, represent another source of uncertainty in climate change impact assessment studies. This is also because several BC methods were tailored to the specific characteristics of catchments up to basin scale, resulting watershed-dependent and, therefore, inappropriate in other contexts (Tumsa, 2022). According to Mendez et al. (2020), selecting appropriate methods in arid and semi-arid regions becomes more challenging due to the scarce, irregular, and random nature of precipitation patterns. Future precipitation and temperature outcomes demonstrate that BC methods tend to overestimate the annual mean, with differences that depend also on the analysed RCMs. Another source of uncertainty stems from their ability to preserve the climate change signal or to capture future extreme events. In this case, the climate change signal is sensitive only to RCMs since LS and PTR for precipitation and LS and VAR for temperature generally display comparable results. However, there are regions where climate change patterns and extreme event assessments are influenced by the choice of the BC methods. For example, Tefera et al. (2023) demonstrated in the Jemma sub-basin of the Upper Blue Nile Basin that the behavior of linear scaling and distribution mapping techniques differs in extreme events and climate change signals under different RCMs and emission scenarios. Despite the improvements in this field, it is challenging to handle with the cascade effect of uncertainty introduced by the entire modelling procedure, i.e., the choice of GCM/RCM simulations, the evaluation of the emission scenario, the selection of BC methods, the quality of the observed datasets (Noto et al., 2023b). Bias correction of RCM simulations is therefore indispensable, specifically when these datasets are used as input for hydrological modelling (Gado et al., 2022), although it is paramount to implement an integrated approach between BC methods and multi-model ensemble to address the existing uncertainties (Lyra and Loukas, 2023). Nevertheless, such a task is out of the scope of this paper, as further detailed investigations need to be carried out prior to successfully modelling it. At present state, the geological and structural complexity of the system and its high degree of anisotropy hinder development of a numerical hydrological model. This investigation aims to outline the preliminary tasks for defining future scenarios of the meteorological data to drive the climatic input data of a hydrological model. As an outcome of the generated climate projections in the study area, an overview of the potential impacts of future meteorological trends on water resources is discussed.
Results on future climate projections show a potential reduction in precipitation and an increase in temperature for the Salento study area until the end of this century compared to the historical period (1971–2005). In 2031–2060, an annual precipitation change of −6.1%, on average, is expected, while in 2071–2,100, a slight increase compared to the previous period is denoted, with a negative change of −5.3%, on average. The gradual temperature increase, which is detectable also during the historical period, agrees with the results of D’Oria et al. (2018). The average annual minimum and maximum temperatures vary by more than 2°C under the RCP4.5 scenario.
Precipitation and temperature projections are in agreement with those produced by Bucchignani et al. (2016) through the analysis of high-resolution simulations with COSMO-CLM over Italy. Concerning the study area, they suggested a moderate precipitation decrease during the winter-autumn period (from September to February) and a moderate-significant reduction during the rest of the months under the RCP4.5 scenario in 2071–2,100, compared to the reference period 1971–2010. Under the same conditions, an increase of more than 2°C was estimated for the mean temperature. These patterns are critical for all environments where inadequate attention is addressed to water resources management, especially so where highly vulnerable aquifer systems cater for almost all regional water demands, as in Salento. Just by the projected climate trends, it could be argued that precipitation regime variations and significantly rising temperatures should compromise aquifer recharge rates due to higher evapotranspiration levels and, at least in some periods, reduced precipitation.
In several catchments of the Mediterranean basin, like the Salento aquifer, the principal groundwater stress is linked to the agricultural and tourism sectors; water demand generally increases in summer, coinciding with the irrigation season and the concentration of thousands of tourists. The projected decrease in precipitation, especially during summer and early autumn, is expected to further increase the already elevated water demand. Consequently, anthropogenic pressures may worsen the already critical water shortages, which reflects the aquifer overexploitation that triggers groundwater quality degradation due to, amongst others, saline water intrusion. Land use changes may also impact on aquifer recharge. Inland and coastal urbanisation expansion for the development of tourism facilities convert large soil covered areas to impermeable or low permeability clusters that impede deep percolation and, therefore, potential aquifer recharge whilst increasing surface runoff and, consequently, flood risks. In addition, the concurrent increase in temperature increases water loss on such surfaces through evaporation. On the other hand, the higher pattern in minimum and maximum temperatures could affect the agricultural sector since the consequent increase in the potential evapotranspiration will likely determine a rise in crop water needs. Crops’ heat stress due to the maximum temperature increase could alter crop growth and yield, leading to significant economic losses and food production deficits.
Future groundwater availability in the Salento aquifer is expected to be compromised by the estimated decreasing precipitation and increasing temperature, as highlighted in previous studies (Kapur et al., 2007; Lionello et al., 2014; D’Oria et al., 2018). This is especially so, in the absence of any surface water bodies in the region, which sets all pressure on groundwater, thus exacerbating water deficit conditions and worsening its’ quality characteristics predominantly due to seawater intrusion. Wu et al. (2020) demonstrated that changes in groundwater could depend more on increased evapotranspiration than on reduced precipitation patterns, especially in dry areas. A fully coupled climate model applied to seven aquifers that have experienced severe groundwater heads decline demonstrated that groundwater depletion may result either from human pressures imposed on overexploited systems or adverse natural hydro-climatic drivers (i.e., higher evapotranspiration, snowmelt reduction) even in mildly exploited systems. Human imposed drivers may be more impactful on groundwater resources than climate change; however, coupling afore drivers certainly maximises impacts. Using the Landsat archive, Huntington et al. (2016) found a meaningful correlation between changes in annual vegetation vigor, precipitation, evaporative rate, groundwater depth, and land and water management, providing a helpful interpretation of the investigated groundwater-dependent ecosystem. It should be noted that the historical period selected for this study included severe and prolonged drought events and extensive aquifer overexploitation periods, which had already significantly affected groundwater quality and availability (Balacco et al., 2022a). Due to the coastal nature of the aquifer, groundwater suffered an increase in chloride concentrations, the initial values of which did not recover even following rainy periods (Alfio et al., 2020). Therefore, an eventual future reduction in rainfall compared to the historical reference period, albeit modest (c.a. 6%), could definitively compromise groundwater quality and availability, as the concomitant reduction in recharge and increased abstractions may accelerate the salinisation process.
Human pressures and projected climate change in the Mediterranean basin do not leave space for any future improvement in water resource abundance; therefore, perspectives on water resource availability are alarming, and management planning to strengthen resilience and ensure security is of utmost essence. Further investigations on the Salento aquifer are necessary to simulate the water balance in response to climate change and groundwater abstraction effects. Additional studies should be developed locally to quantify each factor influencing the aquifer behaviour to develop a strategic water resource management tool for complex systems.
In this research, future hydrological impacts of climate change have been evaluated at the regional coastal karstic aquifer of Salento. Historical precipitation and minimum and maximum temperature datasets were previously infilled by testing five methodologies. Based on the number of iterations, R, NSE, and SI, the MICE_pmm and the MS methods were selected for filling-in missing data in the precipitation and temperature daily time series, respectively. The trend analysis on both climate variables reveals that no significant annual or monthly trends are depicted in the reference period. An ensemble of 12 RCMs under the RCP4.5 scenario was analysed, and different scaling and distribution methods were applied daily. According to monthly and annual assessments of BC methods’ results, all RCMs were considered for the temperature dataset, whilst AL_CN and WF_IP were excluded from precipitation analyses. Additionally, the most suitable BC methods for the precipitation dataset were LS and PTR, while all methods are appropriate for temperature time series for the case study. Finally, future projections reveal a decreasing precipitation trend of about 6% and an increasing temperature pattern of 2°C compared to the historical period (1971–2005) until 2,100 for the Salento study area. These outcomes reveal a critical situation for the Salento aquifer since it can be expected a limited recharge rates due to higher evapotranspiration levels, reduced precipitation, and consequently increasing water demand. Without water resource alternatives, anthropogenic pressures could worsen current water shortages, potentially resulting in the deterioration of groundwater quality, including the intrusion of saline water. Therefore, water resource management in coastal basins expected to undergo similar conditions could represent a common challenge which requires focused attention, targeted solutions, and shared experiences among similar contexts. This research represents a starting point for further studies to determine a hydrogeological model of the study area with the ability to simulate the water balance in response to climate change and pumping effects. In this context, additional studies should be developed locally to quantify groundwater withdrawal and investigate its impacts on the aquifer status.
Publicly available datasets were analyzed in this study. This data can be found here: www.protezionecivile.puglia.it.
MA: Methodology, Data curation, Formal analysis, Writing original draft; VP: Conceptualization, Methodology. AP: Supervision; GB: Conceptualisation, Supervision. All authors contributed to the article and approved the submitted version.
This research is conducted in the context of MEDSAL Project© (www.medsal.net), which is part of the PRIMA Programme supported by the European Union’s Horizon 2020 Research and Innovation Programme and funded by the national funding agencies of GSRT (grant number 2018-7), BMBF, RPF, MIUR (grant number 1421), MHESR (grant number 2018-12), and TÜBITAK (grant number 118Y366).
We acknowledge the World Climate Research Programs Working Group on Coupled Modelling, the Working Group on Regional Climate, and all participating EURO-CORDEX modelling groups for producing and making available their RCMs output.
The authors declare that the research was conducted in the absence of any commercial or financial relationships that could be construed as a potential conflict of interest.
All claims expressed in this article are solely those of the authors and do not necessarily represent those of their affiliated organizations, or those of the publisher, the editors and the reviewers. Any product that may be evaluated in this article, or claim that may be made by its manufacturer, is not guaranteed or endorsed by the publisher.
The Supplementary Material for this article can be found online at: https://www.frontiersin.org/articles/10.3389/feart.2023.1231296/full#supplementary-material
Abdullah, A. Y. M., Bhuian, M. H., Kiselev, G., Dewan, A., Hassan, Q. K., and Rafiuddin, M. (2022). Extreme temperature and rainfall events in Bangladesh: A comparison between coastal and inland areas. Int. J. Climatol. 42 (6), 3253–3273. doi:10.1002/joc.6911
Abd-Elmabod, S. K., Muñoz-Rojas, M., Jordán, A., Anaya-Romero, M., Phillips, J. D., Jones, L., et al. (2020). Climate change impacts on agricultural suitability and yield reduction in a Mediterranean region. Geoderma 374, 114453. doi:10.1016/j.geoderma.2020.114453
Aguilera, H., Guardiola-Albert, C., and Serrano-Hidalgo, C. (2020). Estimating extremely large amounts of missing precipitation data. J. Hydroinformatics 22 (3), 578–592. doi:10.2166/hydro.2020.127
Aieb, A., Madani, K., Scarpa, M., Bonaccorso, B., and Lefsih, K. (2019). A new approach for processing climate missing databases applied to daily rainfall data in Soummam watershed, Algeria. Heliyon 5 (2), e01247. doi:10.1016/j.heliyon.2019.e01247
Aissia, M. A. B., Chebana, F., and Ouarda, T. B. M. J. (2017). Multivariate missing data in hydrology – review and applications. Adv. Water Resour. 110, 299–309. doi:10.1016/j.advwatres.2017.10.002
Alfio, M. R., Balacco, G., Parisi, A., Totaro, V., and Fidelibus, M. D. (2020). Drought Index as indicator of salinization of the Salento aquifer (southern Italy). Water 12, 1927. doi:10.3390/w12071927
Arampatzis, G., Panagopoulos, A., Pisinaras, V., Tziritis, E., and Wendland, F. (2018). Identifying potential effects of climate change on the development of water resources in Pinios River Basin, Central Greece. Appl. Water Sci. 8, 51. doi:10.1007/s13201-018-0690-1
Armanuos, A. M., Al-Ansari, N., and Yaseen, Z. M. (2020). Cross assessment of twenty-one different methods for missing precipitation data estimation. Atmosphere 11 (4), 389. doi:10.3390/atmos11040389
Balacco, G., Alfio, M. R., and Fidelibus, M. D. (2022a). Groundwater drought analysis under data scarcity: the case of the Salento aquifer (Italy). Sustainability 14 (2), 707. doi:10.3390/su14020707
Balacco, G., Alfio, M. R., Parisi, A., Panagopoulos, A., and Fidelibus, M. D. (2022b). Application of short time series analysis for the hydrodynamic characterization of a coastal karst aquifer: the Salento aquifer (southern Italy). J. Hydroinformatics 24 (2), 420–443. doi:10.2166/hydro.2022.135
Bucchignani, E., Montesarchio, M., Zollo, A. L., and Mercogliano, P. (2016). High-resolution climate simulations with COSMO-CLM over Italy: performance evaluation and climate projections for the 21st century. Int. J. Climatol. 36, 735–756. doi:10.1002/joc.4379
Cannon, A. J., Sobie, S. R., and Murdock, T. Q. (2015). Bias correction of GCM precipitation by quantile mapping: how well do methods preserve changes in quantiles and extremes? J. Clim. 28 (17), 6938–6959. doi:10.1175/jcli-d-14-00754.1
Chen, J., Brissette, F. P., and Leconte, R. (2011a). Uncertainty of downscaling method in quantifying the impact of climate change on hydrology. J. Hydrol. 401 (3–4), 190–202. doi:10.1016/j.jhydrol.2011.02.020
Chen, J., Brissette, F. P., and Lucas-Picher, P. (2015). Assessing the limits of bias-correcting climate model outputs for climate change impact studies. J. Geophys. Res. Atmos. 120, 1123–1136. doi:10.1002/2014JD022635
Chen, J., Brissette, F. P., Poulin, A., and Leconte, R. (2011b). Overall uncertainty study of the hydrological impacts of climate change for a Canadian watershed. Water Resour. Res. 47 (12), W12509. doi:10.1029/2011WR010602
Christensen, O. B., Christensen, J. H., Machenhauer, B., and Botzet, M. (1998). Very high-resolution regional climate simulations over Scandinavia-Present climate. J. Clim. 11, 3204–3229. doi:10.1175/1520-0442(1998)011<3204:VHRRCS>2.0.CO;2
Colin, J., De´que´, M., Radu, R., and Somot, S. (2010). Sensitivity study of heavy precipitation in limited area model climate simulations: influence of the size of the domain and the use of the spectral nudging technique. Tellus A 62, 591–604. doi:10.1111/j.1600-0870.2010.00467.x
Collados-Lara, A. J., Pulido-Velazquez, D., and Pardo-Igúzquiza, E. (2018). An integrated statistical method to generate potential future climate scenarios to analyse droughts. Water 10, 1224. doi:10.3390/w10091224
Déqué, M. (2007). Frequency of precipitation and temperature extremes over France in an anthropogenic scenario: model results and statistical correction according to observed values. Glob. Planet. Change. 57, 16–26. doi:10.1016/j.gloplacha.2006.11.030
De Filippis, G., Margiotta, S., Branca, C., and Negri, S. L. (2019). A modelling approach for assessing the hydrogeological equilibrium of the karst, coastal aquifer of the Salento peninsula southeastern Italy: evaluating the effects of a MAR facility for wastewater reuse. Geofluids 19, 1–19. doi:10.1155/2019/5714535
Dieng, D., Cannon, A. J., Laux, P., Hald, C., Adeyeri, O., Rahimi, J., et al. (2022). Multivariate bias-correction of high-resolution regional climate change simulations for west Africa: performance and climate change implications. J. Geophys. Res. Atmos. 127, e2021JD034836. doi:10.1029/2021JD034836
D’Oria, M., Tanda, M. G., and Todaro, V. (2018). Assessment of local climate change: historical trends and RCM multi-model projections over the Salento area (Italy). Water 10 (8), 978. doi:10.3390/w10080978
Dufresne, J.-L., Foujols, M.-A., Denvil, S., Caubel, A., Marti, O., Aumont, O., et al. (2013). Climate change projections using the IPSL-CM5 Earth system model: from CMIP3 to CMIP5. Clim. Dynam. 40, 2123–2165. doi:10.1007/s00382-012-1636-1
Fang, G. H., Yang, J., Chen, Y. N., and Zammit, C. (2015). Comparing bias correction methods in downscaling meteorological variables for a hydrologic impact study in an arid area in China. Earth Syst. Sci. 19, 2547–2559. doi:10.5194/hess-19-2547-2015
Fantini, A., Raffaele, F., Torma, C., Bacer, S., Coppola, E., Giorgi, F., et al. (2018). Assessment of multiple daily precipitation statistics in ERA-Interim driven Med-CORDEX and EURO-CORDEX experiments against high resolution observations. Clim. Dyn. 51, 877–900. doi:10.1007/s00382-016-3453-4
Gado, T. A., Mohameden, M. B., and Rashwan, I. M. H. (2022). Bias correction of regional climate model simulations for the impact assessment of the climate change in Egypt. Environ. Sci. Pollut. Res. 29, 20200–20220. doi:10.1007/s11356-021-17189-9
Gampe, D., Schmid, J., and Ludwing, R. (2019). Impact of reference dataset selection on RCM evaluation, bias correction, and resulting climate change signals of precipitation. J. Hydrometeorolo. 20 (9), 1813–1828. doi:10.1175/JHM-D-18-0108.1
Giorgetta, M. A., Jungclaus, J., Reick, C. H., Legutke, S., Bader, J., B¨ottinger, M., et al. (2013). Climate and carbon cycle changes from 1850 to 2100 in MPI-ESM simulations for the coupled model Intercomparison project phase 5: climate changes in MPI-ESM. Adv. Model. Earth Sy. 5, 572–597. doi:10.1002/jame.20038
Giorgi, F., and Lionello, P. (2008). Climate change projections for the Mediterranean region. Glob. Planet. Change 63 (2–3), 90–104. doi:10.1016/j.gloplacha.2007.09.005
Gutjahr, O., and Heinemann, G. (2013). Comparing precipitation bias correction methods for high-resolution regional climate simulations using COSMO-CLM. Theor. Appl. Climatol. 114 (4), 511–529. doi:10.1007/s00704-013-0834-z
Hausfather, Z., and Peters, G. P. (2020). Emissions–the ‘business as usual’story is misleading. Nature 577 (7792), 618–620. doi:10.1038/d41586-020-00177-3
Hazeleger, W., Severijns, C., Semmler, T., S¸tef˘anescu, S., Yang, S., Wang, X., et al. (2010). EC-earth: A seamless earth-system prediction approach in action. B. Am. Meteorol. Soc. 91, 1357–1364. doi:10.1175/2010BAMS2877.1
Herrmann, M., Somot, S., Calmanti, S., Dubois, C., and Sevault, F. (2011). Representation of spatial and temporal variability of daily wind speed and of intense wind events over the mediterranean sea using dynamical downscaling: impact of the regional climate model configuration. Nat. Hazards Earth Syst. Sci. 11, 1983–2001. doi:10.5194/nhess-11-1983-2011
Huntington, J., McGwire, K., Morton, C., Snyder, K., Peterson, S., Erickson, T., et al. (2016). Assessing the role of climate and resource management on groundwater dependent ecosystem changes in arid environments with the Landsat archive. Remote Sens. Environ. 185, 186–197. doi:10.1016/j.rse.2016.07.004
Iizumi, T., Takikawa, H., Hirabayashi, Y., Hanasaki, N., and Nishimori, M. (2017). Contributions of different bias-correction methods and reference meteorological forcing data sets to uncertainty in projected temperature and precipitation extremes. J. Geophys. Res. Atmos. 122, 7800–7819. doi:10.1002/2017JD026613
Iturbide, M., Bedia, J., Herrera, S., Baño-Medina, J., Fernández, J., Frías, M. D., et al. (2019). The R-based climate4R open framework for reproducible climate data access and post-processing. Environ. Model. Softw. 111, 42–54. doi:10.1016/j.envsoft.2018.09.009
IPCC (2014). in Climate change 2014: Synthesis report. Contribution of working groups I, II and III to the fifth assessment report of the intergovernmental panel on climate change [core writing team. Editors Pachauri, R. K., and Meyer, L. A. (Geneva, Switzerland: IPCC), 151.
Jacob, D., Elizalde, A., Haensler, A., Hagemann, S., Kumar, P., Podzun, R., et al. (2012). Assessing the transferability of the regional climate model REMO to different coordinated regional climate downscaling experiment (CORDEX) regions. Atmosphere 3, 181–199. doi:10.3390/atmos3010181
Jacob, D., Petersen, J., Eggert, B., Alias, A., Christensen, O. B., Bouwer, L. M., et al. (2014). EURO-CORDEX: new high-resolution climate change projections for European impact research. Reg. Environ. Change 14 (2), 563–578. doi:10.1007/s10113-013-0499-2
Jing, X., Luo, J., Wang, J., Ganggang, Z., and Na, W. (2022). A multi-imputation method to deal with hydro-meteorological missing values by integrating Chain equations and random forest. Water Resour. Manage 36, 1159–1173. doi:10.1007/s11269-021-03037-5
Kapur, B., Steduto, P., and Todorovic, M. (2007). Prediction of climatic change for the next 100 Years in the Apulia region, southern Italy. South. Italy, Ital. J. Agron./Riv. Agron. 4, 365–371. doi:10.4081/ija.2007.365
Kim, K. B., Kwon, H. H., and Han, D. (2015). Bias correction methods for regional climate model simulations considering the distributional parametric uncertainty underlying the observations. J. Hydrol. 530, 568–579. doi:10.1016/j.jhydrol.2015.10.015
Kupiainen, M., Samuelsson, P., Jones, C., Jansson, C., Wille´n, U., Hansson, U., et al. (2011). Rossby Centre regional atmospheric model. RCA4. Rossby Centre Newsletter, June.
Lange, M. A. (2019). Impacts of climate change on the eastern mediterranean and the Middle East and North Africa region and the water–energy nexus. Atmosphere 10, 455. doi:10.3390/atmos10080455
Leander, R., and Buishand, T. A. (2007). Resampling of regional climate model output for the simulation of extreme river flows. J. Hydrol. 332 (3–4), 487–496. doi:10.1016/j.jhydrol.2006.08.006
Li, H., Sheffield, J., and Wood, E. F. (2010). Bias correction of monthly precipitation and temperature fields from intergovernmental panel on climate change AR4 models using equidistant quantile matching. J. Geophys. Res. Atmos. 115, D10101. doi:10.1029/2009JD012882
Li, J., Ruhe, G., Al-Emran, A., and Richter, M. M. (2007). A flexible method for software effort estimation by analogy. Empir. Softw. Eng. 12, 65–106. doi:10.1007/s10664-006-7552-4
Linares, C., Díaz, J., Negev, M., Sánchez Martínez, G., Debono, R., and Paz, S. (2020). Impacts of climate change on the public health of the Mediterranean Basin population - current situation, projections, preparedness and adaptation. Environ. Res. 182, 109107. doi:10.1016/j.envres.2019.109107
Lionello, P., Congedi, L., Reale, M., Scarascia, L., and Tanzarella, A. (2014). Sensitivity of typical Mediterranean crops to past and future evolution of seasonal temperature and precipitation in Apulia. Reg. Environ. Change 14, 2025–2038. doi:10.1007/s10113-013-0482-y
Lyra, A., and Loukas, A. (2023). Simulation and evaluation of water resources management scenarios under climate change for adaptive management of coastal agricultural watersheds. Water Resour. Manage 37, 2625–2642. doi:10.1007/s11269-022-03392-x
Maraun, D. (2016). Bias correcting climate change simulations—a critical review. Curr. Clim. Change Rep. 2 (3), 211–220. doi:10.1007/s40641-016-0050-x
Marsh, C. J., Barwell, L. J., Gavish, Y., and Kunin, W. E. (2018). downscale: an R Package for downscaling species occupancy from coarse-grain data to predict occupancy at fine-grain sizes. J. Stat. Softw. 86, 1–20. Code Snippet 3. doi:10.18637/jss.v086.c03
Matiu, M., and Hanzer, F. (2022). Bias adjustment and downscaling of snow cover fraction projections from regional climate models using remote sensing for the European Alps. Hydrol. Earth Syst. Sci. 26, 3037–3054. doi:10.5194/hess-26-3037-2022
Mendez, M., Maathuis, B., Hein-Griggs, D., and Alvarado-Gamboa, L. F. (2020). Performance evaluation of bias correction methods for climate change monthly precipitation projections over Costa Rica. WaterSwitzerl. 12 (2), 482. doi:10.3390/w12020482
Mishra, A. K., Dubey, A. K., and Dinesh, A. S. (2023). Diagnosing whether the increasing horizontal resolution of regional climate model inevitably capable of adding value: investigation for Indian summer monsoon. Clim. Dyn. 60, 1925–1945. doi:10.1007/s00382-022-06424-9
Noto, L. V., Cipolla, G., Francipane, A., and Pumo, D. (2023a). Climate change in the Mediterranean basin (Part I): induced alterations on climate forcings and hydrological processes. Water Resour. Manage 37, 2287–2305. doi:10.1007/s11269-022-03400-0
Noto, L. V., Cipolla, G., Pumo, D., and Francipane, A. (2023b). Climate change in the Mediterranean basin (Part II): A review of challenges and uncertainties in climate change modeling and impact analyses. Water Resour. Manage 37, 2307–2323. doi:10.1007/s11269-023-03444-w
Pardo-Igúzquiza, E., Collados-Lara, A. J., and Pulido-Velazquez, D. (2019). Potential future impact of climate change on recharge in the Sierra de las Nieves (southern Spain) high-relief karst aquifer using regional climate models and statistical corrections. Environ. Earth Sci. 78, 598. doi:10.1007/s12665-019-8594-4
Peres, D. J., Senatore, A., Nanni, P., Cancelliere, A., Mendicino, G., and Bonaccorso, B. (2020). Evaluation of EURO-CORDEX (coordinated regional climate downscaling experiment for the euro-mediterranean area) historical simulations by high-quality observational datasets in southern Italy: insights on drought assessment. Nat. Hazards Earth Syst. Sci. 20, 3057–3082. doi:10.5194/nhess-20-3057-2020
Pfeifer, S., Bülow, K., Gobiet, A., Hänsler, A., Mudelsee, M., Otto, J., et al. (2015). Robustness of ensemble climate projections analyzed with climate signal maps: seasonal and extreme precipitation for Germany. Atmosphere 6, 677–698. doi:10.3390/atmos6050677
Piani, C., Haerter, J. O., and Coppola, E. (2009). Statistical bias correction for daily precipitation in regional climate models over Europe. Theor. Appl. Climatol. 99, 187–192. doi:10.1007/s00704-009-0134-9
Portoghese, I., Uricchio, V., and Vurro, M. (2005). A GIS tool for hydrogeological water balance evaluation on a regional scale in semi-arid environments. Comput. Geosci. 31, 15–27. doi:10.1016/j.cageo.2004.09.001
Randall, D., Wood, R., Bony, S., Colman, R., Fichefet, T., Fyfe, J., et al. (2007). “Cilmate models and their evaluation,” in Climate change 2007: The physical science basis. Contribution of working group I to the fourth assessment report of the intergovernmental panel on climate change Editors S. Solomon, D. Qin, M. Manning, Z. Chen, M. Marquis, and K. B. Averyt (Cambridge & New York, NY: Cambridge University Press).
Rockel, B., Will, A., and Hence, A. (2008). The regional climate model COSMO-CLM (CCLM). Meteorol. Z 17, 347–348. doi:10.1127/0941-2948/2008/0309
Samuelsson, P., Jones, C., Wille´n, U., Ullerstig, A., Gollvik, S., Hansson, U., et al. (2011). The rossby centre regional climate model RCA3: model description and performance. Tellus A 63, 4–23. doi:10.1111/j.1600-0870.2010.00478.x
Sattari, M. T., Rezazadeh-Joudi, A., and Kusiak, A. (2017). Assessment of different methods for estimation of missing data in precipitation studies. Hydrology Res. 48 (4), 1032–1044. doi:10.2166/nh.2016.364
Schmidli, J., Frei, C., and Vidale, P. L. (2006). Downscaling from GCM precipitation: A benchmark for dynamical and statistical downscaling methods. Int. J. Climatol. 26 (5), 679–689. doi:10.1002/joc.1287
Senatore, A., Fuoco, D., Maiolo, M., Mendicino, G., Smiatek, G., and Kunstmann, H. (2022). Evaluating the uncertainty of climate model structure and bias correction on the hydrological impact of projected climate change in a Mediterranean catchment. J. Hydrology Regional Stud. 42, 101120. doi:10.1016/j.ejrh.2022.101120
Seo, G.-Y., and Ahn, J.-B. (2023). Comparison of bias correction methods for summertime daily rainfall in South Korea using quantile mapping and machine learning model. Atmosphere 14, 1057. doi:10.3390/atmos14071057
Singh, D., Choi, Y., Dimri, R., Ghahremanloo, M., and Pouyaei, A. (2023). An Intercomparison of deep-learning methods for super-resolution bias-correction (SRBC) of Indian summer monsoon rainfall (ISMR) using CORDEX-SA simulations. Asia-Pac J. Atmos. Sci. 59, 495–508. doi:10.1007/s13143-023-00330-8
Skamarock, W. C., Klemp, J. B., Dudhia, J., Gill, D. O., Duda, D. M. B. M. G., Huang, X-Y., et al. (2008). A description of the advanced research WRF version 3. (No. NCAR/TN-475+STR). University Corporation for Atmospheric Research. doi:10.5065/D68S4MVH
Smitha, P. S., Narasimhan, B., Sudheer, K. P., and Annamalai, H. (2018). An improved bias correction method of daily rainfall data using a sliding window technique for climate change impact assessment. J. Hydrology 556, 100–118. doi:10.1016/j.jhydrol.2017.11.010
Song, Q., Shepperd, M., Chen, X., and Liu, J. (2008). Can k-NN imputation improve the performance of C4.5 with small software project data sets? A comparative evaluation. J. Syst. Softw. 81 (12), 2361–2370. doi:10.1016/j.jss.2008.05.008
Sordo-Ward, A., Granados, A., Iglesias, A., Garrote, L., and Bejarano, M. D. (2019). Adaptation effort and performance of water management strategies to face climate change impacts in six representative basins of southern europe. Water 11, 1078. doi:10.3390/w11051078
Sperna Weiland, F. C., Visser, R. D., Greve, P., Bisselink, B., Brunner, L., and Weerts, A. H. (2021). Estimating regionalized hydrological impacts of climate change over europe by performance-based weighting of CORDEX projections. Front. Water 3, 713537. doi:10.3389/frwa.2021.713537
Taylor, K. E. (2001). Summarizing multiple aspects of model performance in a single diagram. J. Geophys. Res. Atmos. 106 (D7), 7183–7192.
Taylor, K. E., Stouffer, R. J., and Meehl, G. A. (2012). An overview of CMIP5 and the experiment design. Bull. Am. Meteorol. Soc. 93, 485–498. doi:10.1175/BAMS-D-11-00094.1
Tefera, G. W., Dile, Y. T., and Ray, R. L. (2023). Evaluating the impact of statistical bias correction on climate change signal and extreme indices in the Jemma sub-basin of blue Nile Basin. Sustainability 15, 10513. doi:10.3390/su151310513
Teutschbein, C., and Seibert, J. (2012). Bias correction of regional climate model simulations for hydrological climate-change impact studies: review and evaluation of different methods. J. Hydrol. 456, 12–29. doi:10.1016/j.jhydrol.2012.05.052
Themeßl, M. J., Gobiet, A., and Heinrich, G. (2012). Empirical-statistical downscaling and error correction of regional climate models and its impact on the climate change signal. Clim. Change 112, 449–468. doi:10.1007/s10584-011-0224-4
Thomson, A. M., Calvin, K. V., Smith, S. J., Kyle, G. P., Volke, A., Patel, P., et al. (2011). RCP4.5: A pathway for stabilization of radiative forcing by 2100. Clim. Change 109, 77–94. doi:10.1007/s10584-011-0151-4
Tumsa, B. C. (2022). Performance assessment of six bias correction methods using observed and RCM data at upper Awash basin, Oromia, Ethiopia. J. Water Clim. Change 13 (2), 664–683. doi:10.2166/wcc.2021.181
Turrado, C. C., López Mdel, C., Lasheras, F. S., Gómez, B. A., Rollé, J. L., and Juez, F. J. (2014). Missing data imputation of solar radiation data under different atmospheric conditions. Sensors (Basel) 14 (11), 20382–20399. doi:10.3390/s141120382
van Buuren, S., and Groothuis-Oudshoorn, K. (2011). Mice: multivariate imputation by chained equations in R. J. Stat. Softw. 45 (3), 1–67. doi:10.18637/jss.v045.i03
van Meijgaard, E., van Ulft, B., van de Berg, W. J., Bosveld, F. C., van den Hurk, B., Lenderink, G., et al. (2008). The KNMI regional atmospheric climate model RACMO version 2.1 (KNMI TR-302). Technical Report TR-302.
van Vuuren, D. P., Edmonds, J., Kainuma, M., Riahi, K., Thomson, A., Hibbard, K., et al. (2011). The representative concentration pathways: an overview. Clim. Change 109, 5–31. doi:10.1007/s10584-011-0148-z
Varis, O., Kajander, T., and Lemmelä, R. (2004). Climate and water: from climate models to water resources management and vice versa. Clim. Change 66 (3), 321–344. doi:10.1023/B:CLIM.0000044622.42657.d4
Voldoire, A., Sanchez-Gomez, E., Salas y M´elia, D., Decharme, B., Cassou, C., Sénési, S., et al. (2013). The CNRM-cm5.1 global climate model: description and basic evaluation. Clim. Dyn. 40, 2091–2121. doi:10.1007/s00382-011-1259-y
Wesonga, R. (2015). On multivariate imputation and forecasting of decadal wind speed missing data. Springerplus 4, 12. doi:10.1186/s40064-014-0774-9
Wilcke, R. A. I., Mendlik, T., and Gobiet, A. (2013). Multi-variable error correction of regional climate models. Clim. Change 120, 871–887. doi:10.1007/s10584-013-0845-x
Wu, W. Y., Lo, M. H., Wada, Y., Famiglietti, J. S., Reager, J. T., Yeh, P. J. F., et al. (2020). Divergent effects of climate change on future groundwater availability in key mid-latitude aquifers. Nat. Commun. 11, 3710. doi:10.1038/s41467-020-17581-y
Zeleny, M. (1973). “Compromise programming,” in Multiple criteria decision making. Editors J. L. Cochrane,, and M. Zeleny (Columbia, SC: University of South Carolina Press), 262–301.
Keywords: climate change, bias-correction, missing data, RCM, daily precipitation, daily temperature
Citation: Alfio MR, Pisinaras V, Panagopoulos A and Balacco G (2023) A comprehensive assessment of RCP4.5 projections and bias-correction techniques in a complex coastal karstic aquifer in the Mediterranean. Front. Earth Sci. 11:1231296. doi: 10.3389/feart.2023.1231296
Received: 30 May 2023; Accepted: 11 September 2023;
Published: 19 September 2023.
Edited by:
Tomas Halenka, Charles University, CzechiaCopyright © 2023 Alfio, Pisinaras, Panagopoulos and Balacco. This is an open-access article distributed under the terms of the Creative Commons Attribution License (CC BY). The use, distribution or reproduction in other forums is permitted, provided the original author(s) and the copyright owner(s) are credited and that the original publication in this journal is cited, in accordance with accepted academic practice. No use, distribution or reproduction is permitted which does not comply with these terms.
*Correspondence: Maria Rosaria Alfio, bWFyaWFyb3NhcmlhLmFsZmlvQHBvbGliYS5pdA==
Disclaimer: All claims expressed in this article are solely those of the authors and do not necessarily represent those of their affiliated organizations, or those of the publisher, the editors and the reviewers. Any product that may be evaluated in this article or claim that may be made by its manufacturer is not guaranteed or endorsed by the publisher.
Research integrity at Frontiers
Learn more about the work of our research integrity team to safeguard the quality of each article we publish.