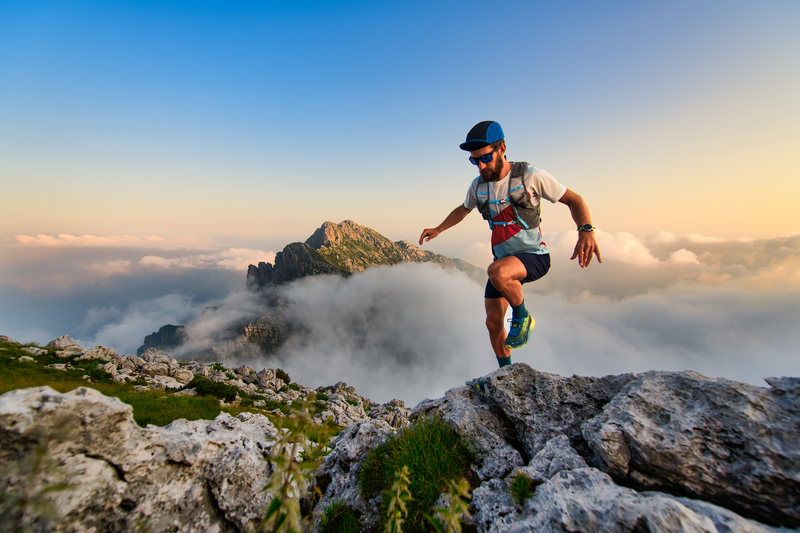
94% of researchers rate our articles as excellent or good
Learn more about the work of our research integrity team to safeguard the quality of each article we publish.
Find out more
ORIGINAL RESEARCH article
Front. Earth Sci. , 04 May 2023
Sec. Solid Earth Geophysics
Volume 11 - 2023 | https://doi.org/10.3389/feart.2023.1164751
This article is part of the Research Topic Advances in Petrophysics and Formation Evaluation of Unconventional Resources View all 9 articles
After complex geological transformation, such as sedimentation, diagenesis, and tectonic transformation, carbonate reservoirs have developed multiple reservoir spaces with significant heterogeneity and various pore-throat structures, including pores, vugs, and fractures. Due to these characteristics, many problems have been caused during the development of carbonate reservoirs, such as large differences between the initial production capacities of single wells and serious water channeling after injection, which have brought many challenges to the efficient development of carbonate reservoirs. Therefore, based on a reservoir evaluation method of petrophysical facies, this study classified and evaluated the pore-throat structures of Carboniferous carbonate reservoirs in the North Truwa oilfield of the Pre-Caspian Basin. Based on the data of the core, thin section, scanning electron microscope, high-pressure mercury injection, sedimentary facies, diagenetic facies, and the development of fractures and karst vugs, six reservoir petrophysical facies in the study area were classified and named, including the facies of favorable sedimentation-constructive diagenesis-fracture and vug, the facies of favorable sedimentation-constructive diagenesis-non-fracture or vug, the facies of favorable sedimentation-destructive diagenesis-fracture and vug, the facies of favorable sedimentation-destructive diagenesis-non-fracture or vug, the facies of unfavorable sedimentation-constructive diagenesis-non-fracture or vug, and the facies of unfavorable sedimentation-destructive diagenesis-non-fracture or vug. The recognition and division of diagenetic facies were based on the K-nearest neighbor. Four quantitative parameters were also selected to characterize the pore-throat structures, including displacement pressure (
The world’s oil and gas production of carbonate reservoirs accounts for about 60% of the world’s total oil and gas production (Chi et al., 2022; Farhadi et al., 2022; Meng et al., 2022; Zeng et al., 2022; Opoku et al., 2023). However, due to the significant heterogeneity (Ole et al., 2016; Chen et al., 2022; Skowera and Rusin, 2022), the single-well productions of reservoirs vary greatly, which can be measured by the permeability and production of reservoirs. Therefore, it is of great significance for the development of oil and gas fields to classify carbonate reservoirs and study their microscopic pore-throat-structure characteristics. At present, previous researchers have made some progress in carbonate reservoir classification and pore-throat structure evaluation. Based on the reservoir quality, Liu et al. (2019) carried out the classification and prediction of carbonate reservoirs with significant heterogeneity. According to the pore size distribution (PSD), Duan et al. (2021) established the relationship between fractal parameters and petrophysical properties and then introduced multifractal parameters to characterize pore-throat structures. Wang et al. (2020) revealed the diversity of pore structure, pore distribution, and pore-throat connectivity in complex carbonate reservoirs by scanning electron microscopy (SEM), micro-nano-computed tomography (CT), and nuclear magnetic resonance (NMR), laying a foundation for the evaluation of carbonate reservoirs by NMR logging. It can be seen that the existing technical methods still have some shortcomings. First of all, the method of reservoir classification is not rigorous enough, and the geological factors, such as sedimentation, diagenesis, and later transformation, are not comprehensively considered. Second, purely mathematical methods such as cluster analysis are mostly used for the characterization and classification of reservoir pore-throat structures. However, due to the lack of constraints from practical geological significance, the classification and evaluation of pore-throat structures are not clear. Finally, the identification and evaluation of reservoir pore-throat structures depend on special logging projects, such as NMR, while the conventional logging curves are failed to make full use of. As a result, it is difficult to implement large-scale identification and evaluation of reservoir pore-throat structures with actual well data of the oilfield. Taking the North Truwa oilfield in the Pre-Caspian Basin as an example, this study classified different petrophysical facies by favorable and unfavorable sedimentary facies, constructive and destructive diagenetic facies, and the development degree of the fracture and vug. Based on the aforementioned division results, this study established the classification and evaluation standards for pore-throat structures by the principal component analysis (PCA) and multi-information fusion and formed a set of characterization and evaluation methods for pore-throat structures in complex carbonate reservoirs, which is of great significance to the efficient development of carbonate oil fields.
The Pre-Caspian Basin is rich in oil and gas resources (Zhu et al., 2020; Zhanserkeyeva and Kassenov, 2022). So far, a series of large and extra-large carbonate oil and gas fields, such as Astrakhan, Kashagan, Tengiz, and Kenkiyak, have been discovered in the Lower Permian salt beds in this basin. The North Truwa oilfield is located in the central block of the eastern slope of the Yanbek–Zarkames palaeouplift (referred to as the central block) in the eastern margin of the Pre-Caspian Basin. In the oilfield, the strata are revealed by the drilling range from Quaternary to Devonian, in which the Lower Permian Kungurian developed salt dome structures. Based on the stratification of geological time and the boundary of salt rock deposits, the Carboniferous carbonate formation can be divided into two sets of oil layers, KT-I and KT-II, and the MKT interlayer is composed of terrigenous clastic rocks, with a thickness of about 350 m. The main oil-bearing series are located in the Middle and Upper pre-salt Carboniferous, including the Serpukhovian (the lower part of the KT-II), the Upper Carboniferous Rashkrian (the middle part of the KT-II), the Moscowian (including the upper part of KT-II, the MKT, and the middle and lower parts of KT-I), the Kasimovian (the middle and upper parts of KT-I), and the Gzhelian (the upper part of KT-I) from the bottom up. The thickness of KT-II is about 400 m, and it is in an unconformable contact with the overlying MKT and the underlying Visean. The thickness of KT-I is about 450 m, and it is in an unconformable contact with the overlying Permian Asselian and the underlying MKT (Figure 1).
FIGURE 1. Geologic overview of the North Truwa oilfield. (A) Location of the central block; (B) oilfield distribution; (C) stratigraphic histogram.
The North Truwa oilfield in the central block is located in the Zanarol carbonate platform and has experienced a complex sedimentary evolution process. From the later period of Early Visean to the early period of Early Moscowian (330.9–314.0 Ma, with a time limit of about 16.9 Ma), due to the large-scale and long-term decline of the global sea level, the KT-II layer was deposited under the regression and showed sedimentary characteristics of an open platform (Zhao et al., 2010; Yi et al., 2017; Li et al., 2020). In the middle period of the Middle Carboniferous Moscowian (314.0–310.0 Ma, with a time limit of 4.0 Ma), due to the large-scale rise of the global sea level, the KT-II carbonate platform was flooded, and as a result, the MKT sedimentary system of deep-water shelf and slope developed in the study area. The North Truwa oilfield in the eastern study area deposited a (deep-water) mixed shelf with large thickness, and the western study area was transformed into a slope (basin), with a significantly thinner thickness. From the Late Moscowian of the Middle Carboniferous to the Gzhelian of the Upper Carboniferous (310.0–298.8 Ma, with a time limit of 11.2 Ma), due to another long-term and large-scale global sea level decline, KT-I was deposited during this process, which was transformed from an open platform to a restricted evaporation platform. In general, the North Truwa oilfield has experienced the sedimentary evolution of open platform, mixed shelf, open platform, and evaporation-restricted platform and is mainly composed of open platform facies. Affected by the compression of Ural tectonic movement, it also developed facies of platform marginal reef and slope (Figure 2).
The understanding and achievements of the research on Carboniferous reservoirs in the North Truwa oilfield are mainly obtained from the analysis data of nine wells, including well CT-4, CT-10, CT-22, CT-41, CT-52, 5555, 5598, A-4, and A-7. The core intervals include KT-I and KT-II. Based on the data of the core, thin section, scanning electron microscope, conventional logging and imaging logging data, and the mathematical methods of K-nearest neighbor (KNN) and PCA, this study realized the effective classification and evaluation of carbonate reservoirs. The method flow is as follows:
(1) Identification of different sedimentary facies by detailed observation and analysis of the core and thin section data and classification of different sedimentary facies in single-well reservoirs by the logging curve shapes after the core location
(2) Based on the data of thin section and scanning electron microscope, the diagenesis, and the genetic patterns of pores, the lithofacies can be divided, and the diagenetic facies in the single-well reservoirs can be identified by the KNN algorithm
(3) Based on data from imaging logging, core observation, and thin section identification, the porosities of fractures and vugs can be calculated by conventional logging curves, and the development of fractures and vugs in single-well reservoirs can be identified
(4) Based on the sedimentary facies, diagenetic facies, and the development of fractures and vugs, the single-well petrophysical facies can be divided, and the classification and evaluation standards of pore-throat structures in different petrophysical facies are established by PCA combined with the pore-throat structure parameters
KNN is an effective mathematical algorithm. Each sample can be represented by its nearest k neighbors, and the new samples can be directly classified by the classification dataset in advance, without learning and training. If the neighbor of a sample to be divided is an object that has been correctly classified, the category of this sample can be determined by the category of the nearest sample or samples. Therefore, KNN is not affected by outliers and is suitable for classification problems with overlapping sample sets to be divided or overlapping class domains (Ma et al., 2022; Poggi et al., 2022; Zhang X. F. et al., 2022; Cao et al., 2023; Saravanan et al., 2023). The KNN algorithm is simple and direct. With this method, the samples with small sizes and characteristics can also be classified, but the number of sample types should be balanced.
The specific steps of KNN are as follows: we suppose there are
where
According to Eq. 1, the decision rule can be written as follows: if
PCA is a method of data dimensionality reduction, which to some extent reveals the hidden structure (principal component) of the best explanatory variables of data. By the projection method, the high-dimensional data can be projected to the low-dimensional space with as little information loss as possible so that the data dimension can be reduced, and the data structure can be simplified. The basic ideas of PCA are shown as follows (Hassan et al., 2022; Murshid et al., 2022): let the
(1) Based on the
(2) The original data are standardized; that is, the element xik is transformed in the sample set.
(3) The correlation coefficient matrix of the sample matrix is calculated.
(4) Corresponding to the correlation coefficient matrix
(5) The principal component contribution rate
The number of principal components, m, generally depends on the cumulative contribution rate. When the cumulative contribution rate of m principal components is greater than 85%, it can be considered that the current m principal components contain all the information of
Based on the theory of “facies control,” facies refers to rocks or rock combinations formed in the same sedimentary environment with the same rock composition and genetic significance. Hence, it can be seen that the petrophysical facies are mainly controlled by sedimentary facies, diagenetic facies, and the development of fractures and vugs (Yu et al., 2022), and their classification and naming should be affected by the three aforementioned factors (Amjad et al., 2021; Hou et al., 2022a; Mohsenipour et al., 2022; Yi et al., 2022). As the basis for petrophysical facies classification in carbonate reservoirs, the sedimentary facies, diagenetic facies, and fracture and vug development characteristics of the Carboniferous reservoirs in the North Truwa oilfield were researched in this study.
The North Truwa oilfield is located in the shallow carbonate platform facies area at the eastern margin of the Pre-Caspian Basin. The sedimentary patterns of oil layers, KT-I and KT-II, during the Late Carboniferous have a certain inheritance. The KT-I oil layer in the study area is mainly composed of evaporation platform, limited platform, and open platform. The KT-II oil layer is mainly composed of an open platform, which can be divided into four subfacies: lagoon, flat, grain bank, and intertidal sea (Figure 2). The sedimentary subfacies can reflect the changes of sedimentary environments and lithology, and the thickness of the sedimentary subfacies is moderate. As a result, sedimentary subfacies identified by lithology are accurate (Arnsari et al., 2020; Gomes et al., 2020; Salman, 2021; Mohammadkhani et al., 2022; Wu et al., 2022; Balaky et al., 2023). Therefore, based on sedimentary subfacies, petrophysical facies can be divided in this study. These studies will be discussed as follows.
The top of the KT-I layer is mainly composed of dark gray micritic dolostone (Figure 3A) with occasional anhydrite (Figure 3C). There are few organisms and many cyanobacteria in the micritic dolostone; as a result, algal mats, algal clumps, and algal laminae are developed (Figure 3B). Based on these characteristics, it can be seen that during the sedimentation of KT-I, it was a shallow water environment, with poor connectivity to large-scale sea area, high evaporation, and high salinity, which shows typical characteristics of flat subfacies. The middle part of KT-I is mainly composed of bioclastic marlstone (Figure 3D) and mudstone. The bioclastic marlstone is formed with few particles, small organisms, and many biological fragments, and it is mainly supported by plaster (Figure 3E). It can be judged that the middle part of KT-I is located in low-lying areas within the restricted platform. It was flooded by seawater; as a result, it was developed with low environmental energy and still water sedimentation. Through comprehensive judgement, it can be seen that the middle part of KT-I is lagoon subfacies. The middle and lower parts of the KT-I layer are mainly composed of sparite bioclastic granular limestone (Figure 3F), and the particles are mainly composed of foraminifera, echinoderms, brachiopods, and mollusks, with high sorting. Most of the particles are filled with sparite cement, and there is less plaster (Figure 3G). It can be seen that the middle and lower parts of KT-I were formed in the seabed highland on the platform, with high water energy. Controlled by tidal and wave action, granular rocks were formed. As a result, it can be comprehensively judged that the sedimentary environment of the middle and lower parts of KT-I is grain beach subfacies. There are micritic carbonate interlayers of 2–3 m in the thicker granular carbonate rocks at the bottom of KT-I (Figure 3H), with low biological content and a large number of local algae (Figure 3I). These characteristics reflect the discontinuous distribution of grain banks in the space. Stable sedimentary environments with relatively deep water bodies can be developed between the beaches, namely, the inter-beach marine subfacies.
FIGURE 3. Typical characteristics of sedimentary facies in the North Truwa oilfield. (A) Well CT-4, 2341.29∼2341.38 m, micritic and powdercrystal dolomite with dissolved pores and caves; (B) well CT-4, 2341.29∼2341.32 m, micritic and powder crystal dolomite with intense dolomitization, fusulinids, and algae, ×40; (C) well A-1, 2616.00∼2316.03 m, gypsum rock associated with dolomite, ×40; (D) well CT-52, 2285.56∼2285.89 m, bioclastic granular marl without pores; (E) well CT-52, 2285.72∼2285.75 m, bioclastic wackestone with few grains and high marl content, ×40; (F) well CT-22, 2300.07∼2300.27 m, sparry bioclastic grainstone with few pores and one fracture; (G) well CT-22, 2300.14∼2300.17 m, sparry bioclastic grainstone with much bioclastic and little marl, ×40; (H) well CT-10, 2346.93∼2347.00 m, micritic limestone without pores; and (I) well CT-10, 2346.97∼2347.00 m, micrite limestone with micrite grains, ×40.
Conventional logging curves with high sensitivity to carbonate sedimentary facies include acoustic logging (AC), gamma-ray logging (GR), density logging (DEN), compensated neutron logging (CNC), and deep-lateral resistivity logging (RD). Based on the sedimentary facies division by core and thin section data, this study carried out the depth homing of core and thin sections and summarized the response characteristics and variation ranges of the four sedimentary subfacies, including lagoon, flat, grain bank, and intertidal sea on the logging curve (Table 1).
The reservoirs in the North Truwa oilfield mainly experienced five diagenesis, including compaction and pressure dissolution, argillization, multi-period cementation and filling, dissolution, and metasomatism. The diagenetic parameters in these diageneses are quite different. Based on the comprehensive diagenesis patterns, diagenesis intensity, pore genesis patterns, reservoir physical properties, and sedimentary environments, five diagenetic facies are divided, including early dissolution facies (DF1), early dolomitization dissolution facies (DF2), weak-cementation pore preservation facies (DF3), cementation facies (DF4), and compaction facies (DF5). The specific descriptions of these diagenetic facies are described as follows.
The early dissolution facies (DF1) represents the diagenetic facies significantly dissolved and transformed by meteoric water during the contemporaneous–quasicontemporaneous period. Most of the rock particles in the DF1 are in point-suspension contact. The intragranular dissolution pores, mold pores, and coelom pores are relatively developed. The multi-period cementation of dentate-powder crystal-fine crystal-coarse crystal calcite can be seen between the grains (Figure 4A). The diagenesis is mainly selective dissolution, which is mainly developed in the grain bank subfacies. The early dolomitization dissolution facies (DF2) represents the diagenetic facies formed by dolomitization and leaching and transformation of meteoric water during the contemporaneous–quasicontemporaneous period. The particles in the rocks are mainly in suspension contact, and intergranular pores and intergranular dissolution pores are widely developed (Figure 4B). The diageneses are mainly dolomitization and intergranular dissolution, which are mainly developed in the flat subfacies. The weak-cementation pore preservation facies (DF3) represents diagenetic facies with primary pores, which have hardly transformed by early karstification. Most of the rock particles are in point–line contact. The intergranular pores are widely developed, the connectivity between pores is high, and the cementation-filling effect is weak. The cements are mostly local patchy cements (Figure 4C), which are developed in the grain bank subfacies. The cementation facies (DF4) represents the diagenetic facies formed by the sediments in the pores consolidated by the cements precipitated from the pore water. The cements are mainly composed of sparry calcite with strong cementation and filling (Figure 4D), and they are mainly developed in the lagoon subfacies. The compaction facies (DF5) represents diagenetic facies composed of particles closely packed by the gravity of the overlying sediments during the shallow–medium burial period. The contact modes of rock particles include point contact, line contact, and mosaic contact, and the rocks are tight (Figures 4E, F), which are widely developed in the subfacies of lagoon, intertidal sea, and grain bank.
FIGURE 4. Typical diagenetic facies characteristics of the North Truwa oilfield. (A) Well CT-10, 2346.49∼2346.52 m, bioclastic calcareous siliceous rock with foraminiferal visceral foramen and siliceous and calcite cementation, ×12.5; (B) well CT-10, 2398.12∼2398.15 m, fine-powdery dolomite with fusulinids visceral foramen. It is filled with powdery dolomite, ×50; (C) well CT-10, 3122.52∼3122.55 m, sparry bioclastic limestone with residual intergranular pores and weak cementation, ×50; (D) well CT-10, 2350.64∼2350.67 m, sparry bioclastic limestone with fine bioclastic grains and intense calcite cementation, ×50; (E) well CT-10, 2346.11∼2346.14 m, micritic bioclastic limestone with micritic grains and intense compaction, ×12.5; and (F) well CT-10, 2347.52∼2347.55 m, bioclastic-bearing micritic limestone with micritic grains and intense compaction, ×50.
Well logging is widely used to identify carbonate sedimentary facies. However, due to the rapid vertical change, high longitudinal resolution is required during the identification of diagenetic facies (Zhao C. J. et al., 2022; Zhang J. F. et al., 2022; Zhao X. B. et al., 2022; Zhang L. Q. et al., 2022; Li et al., 2022; Wang et al., 2022). It is difficult to effectively identify the diagenetic facies by the common induction of log response values. Therefore, the KNN is chosen to identify and predict the diagenetic facies (Gao et al., 2021; Wang and Lu, 2021). While selecting logging curves, the well sections with poor borehole conditions or poor drilling fluid quality should be excluded. Also, inter-well standardization should be realized to eliminate errors caused by the environment and different logging tools. The crossplot of PE, CNC, DEN, and DT values of different diagenetic facies is shown in Figure 5. The difference of logging response provides a reliable basis for the identification of diagenetic facies by the KNN.
Vugs with different sizes in the Carboniferous reservoirs of the North Truwa oilfield are also widely developed, which are mainly composed of pore spaces formed by the significant non-fabric selective dissolution of crystalline dolomite, with irregular shape and pore size of more than 2 mm (Figures 6A–C, E). The individual vugs have various shapes, including round, elliptical, and irregular shapes, and can be locally distributed in honeycomb-like patches (Figure 6A), with a variety of sizes. The overall filling degree of these vugs is low. The reservoir fractures are mainly structural fractures, sutures, and dissolution fractures, among which the structural fractures are formed by tectonic stress, with flat and straight occurrence and groupings. The structural fractures are mostly formed with openings of less than 0.1 mm, and they are either unfilled or semi-filled (Figure 6F). The sutures are mainly formed by pressure dissolution, and the gaps can be seen on the suture cylinder surface, which are mostly partially filled or fully filled with asphalt, and the opening is usually less than 0.03 mm (Figure 6D). Dissolution fractures are the expanded fractures in the original shapes after the dissolution of diagenetic fluid. Most of the fractures are beaded, reticulated, irregularly curved, or serpentine, and are partially filled with calcite or dolomite in the later periods. The opening of these fractures changes greatly. They are mostly connected with vugs and various dissolved pores to form a typical fracture–cavity system, greatly improving the connectivity of the reservoirs (Figures 6C, E).
FIGURE 6. Types and characteristics of karst vugs and fractures in the North Truwa oilfield. (A) Well CT-22, 2350.45∼2350.62 m, dolomite with oil stains, dissolved caves, and pores. The dissolution caves and pores are honeycombed. The needle-like pores are widely developed with a diameter of 2–15 mm; (B) well CT-59, 2423.00∼2423.03 m, karst breccia limestone with 3.1 mm karst caves, weakly filled with weathered crustal clay, ×40; (C) well CT-4, 2345.28∼2345.31 m, residual bioclasticpowder crystal dolomite with unfilled dissolved pores and caves interconnected by beaded dissolution fractures, providing good connectivity, ×40; (D) well CT-22, 2370.88∼2370.91 m, micritic powder crystal dolomitic limestone with intergranular (dissolved) and intragranular pores show dolomitization along the stylolite and local dissolution, ×40; (E) well CT-4, 2341.66∼2341.69 m, bioclastic micritic dolomite with beaded and network dissolution fractures forms a fracture–cavity system with dissolved cavities and various dissolved pores, ×40; and (F) well 5555, 2345.72∼2345.75 m, residual bioclastic micritic and powder crystal dolomite show an intermittent structural fracture. The fracture is 0.03–0.1 mm wide and is partially filled with dolomite, ×40.
Image logging can accurately identify the reservoir spaces in the study area, such as pores, fractures, and vugs. In log imaging, the pores are shown as pieces of dark and fuzzy pattern (Figure 7A), which are generally distributed in the limestone reservoirs. Fractures are shown as large-amplitude dark sine strips (Figure 7B) in the imaging log. The higher the fracture angle, the greater the sine amplitude (Hou et al., 2022b). The pores are shown as dark blocks (Figure 7C) in the imaging log, which are generally distributed in the dolomite reservoirs with more physical properties.
FIGURE 7. Image logging of different reservoir space types in the North Truwa oilfield. (A) Well CT-1, 3,299∼3,304 m; (B) well CT-1, 2,311∼2,316 m; and (C) well CT-1, 2,349∼2,354 m.
For wells without imaging log data, conventional logging data can be used to calculate reservoir fracture porosity and vug porosity. Xavier and Lucia (2018) proposed to calculate the interparticle porosity by the AC difference (i.e., slowness). Therefore, pore–vug porosity can be calculated by subtracting the interparticle porosity from the total porosity, as follows:
For pore-pattern reservoirs, the reservoir resistivity has a good relationship with the AC. However, due to the fractures, the reservoir conductivity is greatly increased with few changes in the reservoir porosity, so the relationship between resistivity and AC is quite different in the fractured reservoir. The AC can reflect the porosity of the reservoir matrix, which is less affected by the lithology and fluid in the reservoir, and can be used to calculate the reservoir matrix resistivity (Figure 8):
where
where
FIGURE 8. Calculation models of matrix resistivity for reservoirs with different lithologies in the North Truwa Oilfield. (A) Well CT-4, 2,300∼2,302 m, dolomite reservoir without fractures and cavities; (B) well CT-4, 2,357–2,360 m, limestone reservoir without fractures and cavities.
Considering the complexity of the naming scheme after the classification of petrophysical facies by the “facies control” method, the author believed that the superimposed petrophysical facies can be classified and summarized by favorable and unfavorable sedimentary subfacies, constructive and destructive diagenetic facies, and fracture development or not. Among them, the favorable sedimentary subfacies are grain bank and dolomite flat, and the unfavorable sedimentary subfacies are intertidal sea and lagoon. The constructive diagenetic facies include early dissolution facies (DF1), early dolomitization dissolution facies (DF2), and weak-cementation pore preservation facies (DF3), and the destructive diagenetic facies include cementation facies (DF4) and compaction facies (DF5). The development of fractures and vugs can be calculated by the logging data. Considering the specific combination relationship between some diagenetic facies and sedimentary subfacies (early dolomitization dissolution facies only developed in favorable sedimentary facies, such as dolomite flat subfacies and fractures and vugs, generally do not develop in unfavorable sedimentary facies), the reservoirs in the North Truwa oilfield can be divided into six petrophysical facies, including favorable sedimentation-constructive diagenesis-fracture–cavity facies, favorable sedimentation-constructive diagenesis-non-fracture–cavity facies, favorable sedimentation-destructive diagenesis-fracture-fracture–cavity facies, favorable sedimentation-destructive diagenesis-non-fracture–cavity facies, unfavorable sedimentation-constructive diagenesis-non-fracture-vug facies, and unfavorable sedimentation-destructive diagenesis-non-fracture-vug facies (Table 2; Figure 9).
FIGURE 9. Pore-throat radius and capillary pressure characteristics of different petrophysical facies.
When studying the characteristics of pore-throat structures with different petrophysical facies, the following points can be seen. First, the characteristics of pore-throat structures in rocks are important for the classification of petrophysical facies, which can correct the deviation of the classification results based on geological factors such as sedimentary facies, diagenetic facies, and the development of fractures and karst vugs. Second, the range of quantitative parameters (such as average pore-throat radius) characterizing the pore-throat structures of different petrophysical facies overlaps, as a result, single parameters of pore-throat structure cannot be distinguished. Finally, the characteristics of the pore-throat structure of individual core samples are between two different petrophysical facies, and there is no direct evidence to identify their petrophysical facies patterns, such as thin section photos and scanning electron microscopy. Therefore, based on the multi-information fusion technology, this study selected the pore-throat structure parameters, such as displacement pressure (
PCA can effectively identify different pore-throat structures. Finally, the sum of the total eigenvalues (
After the calculation, the discriminant standards of six pore-throat structures are shown in Table 3, including the facies of favorable sedimentation-constructive diagenesis-fracture and vug, the facies of favorable sedimentation-constructive diagenesis-non-fracture or vug, the facies of favorable sedimentation-destructive diagenesis-non-fracture or vug, the facies of unfavorable sedimentation-constructive diagenesis-non-fracture or vug, and the facies of unfavorable sedimentation-destructive diagenesis-non-fracture or vug. Based on the identification index P, different pore-throat structures can be quantitatively classified.
TABLE 3. Quantitative classification standards of pore-throat structures with different petrophysical facies in the North Truwa oilfield.
Taking well CT-4 in the North Truwa oilfield as an example, the well took cores from 2,293∼2,297 m and 2,322∼2,354 m intervals of the KT-I oil layer. Based on core observation and thin section identification, petrophysical facies research was conducted in combination with the characteristics of logging curves. The results showed that the well section was divided into three sedimentary subfacies types from top to bottom, namely, grain beach, flat, and lagoon, and three diagenetic facies types, namely, DF4, DF2, and DF5, at 2,321∼2,325 m. The porosity development of fractures and karst cavities calculated in the well section of 2,337∼2,356 m is consistent with the core photos. Five petrophysical facies, namely, PF1, PF2, PF3, PF4, and PF5, are comprehensively divided, and two petrophysical facies, namely, PF1 and PF3, are developed as oil layers. The petrophysical facies of the reservoir at 2322.3∼2324.1 m well depth is classified as PF1 and those of 2341.2∼2353.7 m depth are PF1 (81%) and PF3 (19%) (Figure 10). The well was perforated in 2008, and daily oil productions of the two sections were 3.25 tons and 61.32 tons, decreasing to 2.41 tons and 11.98 tons 2 years later, respectively. Then, the well was reinjected in 2016. The relative water absorptions were 44% and 56% in 2016 and 0% and 100% in 2017. The results show that the storage capacity of PF1 is better than PF3. This is a critical guarantee for the high and stable production of the reservoir. After water injection, the water is quickly absorbed by PF1 and then the absorption ceased soon, stably replenishing the formation energy. In general, the production performance is well matched with the results of petrophysical facies division.
(1) Under complex sedimentary evolution and diagenetic transformation, the North Truwa oilfield developed two favorable sedimentary subfacies, grain bank and flat, and two unfavorable sedimentary subfacies, lagoon and intertidal sea. After five types of diagenesis, such as compaction and pressure dissolution, argillization, multi-period cementation and filling, dissolution, and metasomatism, five diagenetic facies can be divided, including three constructive diagenetic facies, early dissolution facies (DF1), early dolomitization dissolution facies (DF2), and weak-cemented pore preservation facies (DF3), and two destructive diagenetic facies, such as cemented facies (DF4) and compacted facies (DF5). The core observation and thin section identification results show that fractures and karst vugs are mainly developed in favorable sedimentary subfacies.
(2) The sedimentary subfacies of the carbonate reservoirs in the study area can be divided by core observation and logging curves. The diagenetic facies can be effectively identified and divided by the KNN. Based on the data of image logging and conventional logging, the development of reservoir fractures and vugs can be identified, and six petrophysical facies can also be classified, including the facies of favorable sediment-constructive diagenesis-fracture and vug, the facies of favorable sediment-constructive diagenesis-non fracture or vug facies, the facies of favorable sediment-destructive diagenesis-fracture and vug, the facies of favorable sedimentation-destructive diagenesis-non fracture of vug, the facies of unfavorable sedimentation-constructive diagenesis-non fracture or vug, and the facies of unfavorable sedimentation-destructive diagenesis-non fracture or vug.
(3) The identification standards of pore-throat structures with different petrophysical facies can be established by PCA, which can cover 86% of the information of pore-throat structure parameters of each rock and realize the effective classification of petrophysical facies. PF1 reservoir has strong reserves capacity, and the formation energy can be rapidly replenished after water injection development. It is an important object to implement water-driven production and ensure efficient reservoir development.
The raw data supporting the conclusion of this article will be made available by the authors, without undue reservation.
JH and LZ contributed in writing, reviewing, and editing, data curation, and writing—original draft preparation; WZ, ZF, XW, and XZ contributed in formal analysis, validation, and reviewing.
The authors thank all editors and reviewers for their helpful comments and suggestions.
Authors JH, LZ, WZ, ZF, XW, and XZ are employed by PetroChina Co. Ltd.
Author ZF declared a shared affiliation with the reviewer JZ.
The authors declare that this study was financially supported by the Scientific Research and Technology Development Project of CNPC (2022DJ3210). The funder had the following involvement in the study: data collection and analysis.
All claims expressed in this article are solely those of the authors and do not necessarily represent those of their affiliated organizations, or those of the publisher, the editors, and the reviewers. Any product that may be evaluated in this article, or claim that may be made by its manufacturer, is not guaranteed or endorsed by the publisher.
Akbar, T. A., Javed, A., Ullah, S., Ullah, W., Pervez, A., Akbar, R. A., et al. (2022). Principal component analysis (PCA)–Geographic information system (GIS) modeling for groundwater and associated health risks in abbottabad, Pakistan. Sustainability 14, 14572. doi:10.3390/SU142114572
Amjad, A., Chen, S. C., and Munawar, S. (2021). Integration of cluster analysis and rock physics for the identification of potential hydrocarbon reservoir. Nat. Resour. Res. (Dordr. Neth.) 30, 1395–1409. (prepublish). doi:10.1007/S11053-020-09800-6
Ansari, A. H., Pandey, S. K., Kamlesh, K., Shailesh, A., Shamim, A., and Mayank, S. (2020). Palaeoredox link with the late neoproterozoic–early cambrian bilara carbonate deposition, marwar supergroup, India. Carbonates Evaporites 35, 38. doi:10.1007/s13146-020-00574-9
Balaky, S. M., Al-Dabagh, M. M., Asaad, I. S., Tamar-Agha, M., Ali, M. S., and Radwan, A. E. (2023). Sedimentological and petrophysical heterogeneities controls on reservoir characterization of the Upper Triassic shallow marine carbonate Kurra Chine Formation, Northern Iraq: Integration of outcrop and subsurface data. Mar. Pet. Geol. 149, 106085. doi:10.1016/J.MARPETGEO.2022.106085
Cao, Z., Cheng, J. J., Han, X. D., Li, L. S., Wang, J., Fan, Q. W., et al. (2023). Rapid classification of coal by laser-induced breakdown spectroscopy (LIBS) with K-nearest neighbor (KNN) chemometrics. Instrum. Sci. Technol. 51 (1), 59–67. doi:10.1080/10739149.2022.2087185
Chen, X. Y., Sotomayor, M., Alshaer, H. J., Song, H. F., Panthi, K., Balhoff, M., et al. (2022). Enhanced oil recovery with anionic and zwitterionic surfactants in carbonate rocks: A CT-aided investigation. Fuel 311, 122589. doi:10.1016/J.FUEL.2021.122589
Chi, P., Sun, J. M., Wang, Z. Y., Ju, R. K., Wei, B. J., and Duan, Y. X. (2022). Simulation of flow characteristics and development of permeability model in fractured-vuggy carbonate reservoir. J. Pet. Sci. Eng. 219, 111098. doi:10.1016/J.PETROL.2022.111098
Duan, R. Q., Xu, Z. F., Dong, Y. H., and Liu, W. J. (2021). Characterization and classification of pore structures in deeply buried carbonate rocks based on mono- and multifractal methods. J. Pet. Sci. Eng. 203, 108606. doi:10.1016/J.PETROL.2021.108606
Farhadi, H., Ayatollahi, S., and Fatemi, M. (2022). Impact of rock morphology on the dominating enhanced oil recovery mechanisms by low salinity water flooding in carbonate rocks. Fuel 324 (PC), 124769. doi:10.1016/J.FUEL.2022.124769
Gao, Z. W., Shi, J., Xie, Q. C., Zhou, Y., and Zhou, S. X. (2021). Classification and evaluation of tight sandstone reservoirs based on diagenetic facies: A case study on chang 6 reservoir in the center-west ordos basin. J. Pet. Explor. Prod. Technol. 12, 1203–1216. prepublish. doi:10.1007/S13202-021-01383-Z
Gomes, J. P., Bunevich, R. B., Tedeschi, L. R., Tucker, M. E., and Whitaker, F. F. (2020). Facies classification and patterns of lacustrine carbonate deposition of the barra velha formation, santos basin, Brazilian pre-salt. Mar. Pet. Geol. 113, 104176. doi:10.1016/j.marpetgeo.2019.104176
Hassan, W. M., Al, D. A., Al, A. L., Bhat, R. S., and El, A. A. (2022). Author Correction: Discriminant analysis and binary logistic regression enable more accurate prediction of autism spectrum disorder than principal component analysis. Sci. Rep. 12, 19049. doi:10.1038/S41598-022-23620-Z
Hou, J., Zhao, L., Zeng, X., Zhao, W. Q., Chen, Y. F., Li, J. X., et al. (2022a). Characterization and evaluation of carbonate reservoir pore structure based on machine learning. Energies (Basel, Switz.) 15 (19), 7126. doi:10.3390/EN15197126
Hou, J., Lin, Y. P., Zhao, W. Q., Luo, M., Li, J. X., and Wang, S. Q. (2022b). Logging evaluation of carboniferous carbonate reservoir in North truva oilfield, Kazakhstan. Mar. Orig. Pet. Geol. 27, 103–112. doi:10.3969/j.issn.1672-9854.2022.01.011
Li, W. Q., Mu, L. X., Zhao, L., Li, J. X., Wang, S. Q., Fan, Z. F., et al. (2020). Pore-throat structure characteristics and its impact on the porosity and permeability relationship of Carboniferous carbonate reservoirs in eastern edge of Pre-Caspian Basin. Pet. Explor. Dev. 47 (05), 1027–1041. doi:10.1016/s1876-3804(20)60114-8
Li, Z. H., Zhang, L. Q., Yuan, W. F., Chen, X., Zhang, L., and Li, M. Q. (2022). Logging identification for diagenetic facies of tight sandstone reservoirs: A case study in the lower jurassic ahe formation, kuqa depression of tarim basin. Mar. Pet. Geol. 139, 105601. prepublish. doi:10.1016/J.MARPETGEO.2022.105601
Liu, H. Y., Tian, Z. Y., Liu, B., Guo, R., Shi, K. B., and Ye, Y. F. (2019). Classification and prediction of giant thick strongly heterogeneous carbonate reservoirs in the Middle East area:a case study of Mid-Cretaceous Mishrif Formation in the W oilfield of Iraq. Acta Pet. Sin. 40 (06), 677–691. doi:10.7623/syxb201906004
Ma, R. J., Kong, W., Chen, T., Shu, R., and Huang, G. H. (2022). KNN based denoising algorithm for photon-counting LiDAR: Numerical simulation and parameter optimization design. Remote Sens. 14 (24), 6236. doi:10.3390/RS14246236
Manz, T. A. (2022). Apples to apples comparison of standardized to unstandardized principal component analysis of methods that assign partial atomic charges in molecules. RSC Adv. 12, 31617–31628. doi:10.1039/D2RA06349B
Meng, J. Z., Chen, S. L., Wang, J. X., Chen, Z., and Zhang, J. Y. (2022). Development and application of carbonate dissolution test equipment under thermal–hydraulic–chemical coupling condition. Materials 15 (20), 7383. doi:10.3390/MA15207383
Mohammadkhani, H., Hosseini-Barzi, M., Sadeghi, A., and Pomar, L. (2022). Middle Miocene short-lived Tethyan seaway through the Zagros foreland basin: Facies analysis and paleoenvironmental reconstruction of mixed siliciclastic-carbonate deposits of Mishan Formation, Dezful Embayment, SW Iran. Mar. Pet. Geol. 140, 105649. doi:10.1016/J.MARPETGEO.2022.105649
Mohsenipour, A., Soleimani, B., Zahmatkesh, I., and Veisi, I. (2022). Determination of reservoir rock typing using integrating geological and petrophysical methods in one of the oil field in south-west of Iran. Carbonates Evaporites 37 (2), 31. doi:10.1007/S13146-022-00782-5
Murshid, N., Mouhtady, O., Abusamha, M., Obeid, E., Kharboutly, Y., Chaouk, H., et al. (2022). Metal oxide hydrogel composites for remediation of dye-contaminated wastewater: Principal component analysis. Gels 8, 702. doi:10.3390/GELS8110702
Ole, P. W., Giulio, C., Sima, J., and David, C. P. (2016). The characteristics of open fractures in carbonate reservoirs and their impact on fluid flow: A discussion. Pet. Geosci. 22 (1), 91–104. doi:10.1144/petgeo2015-003
Opoku, B. L., Rafati, R., and Sharifi, H. A. (2023). Modelling of carbonate rock wettability based on surface charge and calcite dissolution. Fuel 331 (P2), 125856. doi:10.1016/J.FUEL.2022.125856
Poggi, B., Babatounde, C., Vittori, E., and Antoine, S. T. (2022). Efficient WSN node placement by coupling KNN machine learning for signal estimations and I-HBIA metaheuristic algorithm for node position optimization. Sensors 22, 9927. doi:10.3390/S22249927
Ramesh, A., and Satyavani, N. (2022). Seismic image enhancement from principal component analysis: A case study from KG basin. J. Geol. Soc. India 98 (11), 1547–1552. doi:10.1007/S12594-022-2210-Z
Salman, A. M. (2021). Diagenetic characteristics and sequence stratigraphy on carbonate deposits: A case study from wadi el dakhl, west gulf of suez, Egypt. Arab. J. Geosci. 14 (12), 1133. doi:10.1007/S12517-021-07472-X
Saravanan, A., Parida, S., Murugan, M., Reddy, M., Sreenivasa, E. P. V., and Kumar, D. S. (2023). Thermal performance prediction of a solar air heater with a C-shape finned absorber plate using RF, LR and KNN models of Machine learning. Therm. Sci. Eng. Prog. 38, 101630. doi:10.1016/J.TSEP.2022.101630
Skowera, K., and Rusin, Z. (2022). Differential analysis of volumetric strain method characterization in the context of phase change of water in carbonate rocks. Materials 15, 308. doi:10.3390/MA15010308
Wang, Y., and Lu, Y. (2021). Diagenetic facies prediction using a LDA-assisted SSOM method for the Eocene beach-bar sandstones of Dongying Depression, East China. J. Pet. Sci. Eng. 196, 108040. prepublish. doi:10.1016/j.petrol.2020.108040
Wang, M. Q., Xie, J., Guo, F. J., Zhou, Y. W., Yang, X. D., and Meng, Z. A. (2020). Determination of NMR T2 cutoff and CT scanning for pore structure evaluation in mixed siliciclastic–carbonate rocks before and after acidification. Energies (Basel, Switz.) 13 (6), 1338. doi:10.3390/en13061338
Wang, W. G., Lin, C. Y., Zhang, X. G., Dong, C. M., Ren, L. H., and Lin, J. L. (2022). Seismic diagenetic facies prediction of tight sandstone in the offshore sparse well area: An example from the Xihu Depression of the East China Sea Basin. J. Pet. Sci. Eng. 216, 110825. doi:10.1016/J.PETROL.2022.110825
Wu, J., Liang, C., Yang, R. C., and Xie, J. (2022). Variation of lacustrine carbonate deposition in the Eocene Dongying Depression and its comparison with Holocene environments. Geol. Mag. 159 (6), 963–980. doi:10.1017/S0016756822000103
Xavier, J., and Lucia, F. J. (2018). Matrix microcrystalline structure and acoustic properties of oomoldic dolograinstone. Geophysics 83, MR199–MR210. doi:10.1190/geo2017-0061.1
Yi, S., Huang, W. H., Jin, Z. K., and Gao, B. S. (2017). Characteristics of carbonate microfacies and sedimentary environment of the east margin of Caspian Basin in the carboniferous KT⁃II layer: A case from zanazor area. Acta Sedimentol. Sin. 35 (01), 139–150. doi:10.14027/j.cnki.cjxb.2017.01.014
Yi, S. B., Pan, S. L., Zuo, H. Y., Wu, Y. H., Song, G. J., and Gou, Q. Y. (2022). Research on rock physics modeling methods for fractured shale reservoirs. Energies (Basel, Switz.) 16 (1), 226. doi:10.3390/EN16010226
Yu, Z. C., Wang, Z. Z., Jiang, Q. P., Wang, J., Feng, Y. L., Zheng, J. R., et al. (2022). Evaluation of low permeability conglomerate reservoirs based on petrophysical facies: A case study from the triassic baikouquan formation, northern mahu sag, junggar basin, China. J. Pet. Sci. Eng. 219, 111082. doi:10.1016/J.PETROL.2022.111082
Zeng, X., Li, W. Q., Hou, J., Zhao, W. Q., Liu, Y. Y., and Kang, Y. B. (2022). Fractal characteristics of pore-throats structure and quality evaluation of carbonate reservoirs in eastern margin of Pre-Caspian Basin. Energies (Basel, Switz.) 15 (17), 6357. doi:10.3390/EN15176357
Zhang, J. F., Yan, D. T., Wang, H., Zhou, S. D., Deng, Y., and Liang, W. L. (2022). Prediction of diagenetic facies via well logs and petrophysical properties in tight sandstone from zhu-III sag: Pearl river mouth basin, south China sea. J. Energy Eng. 148 (6). doi:10.1061/(ASCE)EY.1943-7897.0000862
Zhang, L. Q., Li, J. J., Wang, W., Li, C. Y., Zhang, Y. J., Jiang, S., et al. (2022). Diagenetic facies characteristics and quantitative prediction via wireline logs based on machine learning: A case of lianggaoshan tight sandstone, fuling area, southeastern sichuan basin, southwest China. Front. Earth Sci. 10. doi:10.3389/FEART.2022.1018442
Zhang, X. F., Xia, W. M., Cao, C. J., Che, P. P., Pan, H., and Chen, Y. Q. (2022). Graphene doping to enhance the mechanical energy conversion performances of GR/KNN/P(VDF-TrFE) flexible piezoelectric sensors. PCCP Phys. Chem. Chem. Phys. 25, 1257–1268. doi:10.1039/D2CP05091A
Zhanserkeyeva, A. A., and Kassenov, A. K. (2022). Prospect evaluation based on integrated petroleum system analysis: Block E case study, south-eastern edge of Precaspian Basin (Kazakhstan). J. Pet. Explor. Prod. Technol. 12, 2625–2642. prepublish. doi:10.1007/S13202-022-01466-5
Zhao, L., Li, J. X., Li, K. C., Fan, Z. F., Song, H., and Cheng, X. B. (2010). Development and genetic mechanism of complex carbonate reservoir fractures:A case from the Zanarol Oilfield, Kazakhstan. Pet. Explor. Dev. 37 (03), 304–309. doi:10.1016/s1876-3804(10)60034-1
Zhao, C. J., Jiang, Y. L., and Wang, L. J. (2022). Data-driven diagenetic facies classification and well-logging identification based on machine learning methods: A case study on xujiahe tight sandstone in sichuan basin. J. Pet. Sci. Eng. 217, 110798. doi:10.1016/J.PETROL.2022.110798
Zhao, X. B., Yao, G. Q., Chen, X. J., Zhang, R. X., Lan, Z. J., and Wang, G. C. (2022). Diagenetic facies classification and characterization of a high-temperature and high-pressure tight gas sandstone reservoir: A case study in the ledong area, yinggehai basin. Mar. Pet. Geol. 140, 105665. doi:10.1016/J.MARPETGEO.2022.105665
Zhu, X. E., Jin, Z. K., Liang, T., Yi, S., Wei, K., Gao, B. S., et al. (2020). Depositional environment, diagenetic evolution, and their impact on the reservoir quality of the carboniferous KT-Ⅱ carbonate in the zhanazhol reservoir, Pre-Caspian Basin, Kazakhstan. Mar. Pet. Geol. 117, 104411. prepublish. doi:10.1016/j.marpetgeo.2020.104411
Keywords: carbonate reservoir, pore-throat structure, petrophysical facies, logging, sedimentation, diagenesis
Citation: Hou J, Zhao L, Zhao W, Feng Z, Wang X and Zeng X (2023) Evaluation of pore-throat structures of carbonate reservoirs based on petrophysical facies division. Front. Earth Sci. 11:1164751. doi: 10.3389/feart.2023.1164751
Received: 13 February 2023; Accepted: 24 March 2023;
Published: 04 May 2023.
Edited by:
Xinmin Ge, China University of Petroleum (East China), ChinaCopyright © 2023 Hou, Zhao, Zhao, Feng, Wang and Zeng. This is an open-access article distributed under the terms of the Creative Commons Attribution License (CC BY). The use, distribution or reproduction in other forums is permitted, provided the original author(s) and the copyright owner(s) are credited and that the original publication in this journal is cited, in accordance with accepted academic practice. No use, distribution or reproduction is permitted which does not comply with these terms.
*Correspondence: Lun Zhao, zhaolun@petrochina.com.cn
Disclaimer: All claims expressed in this article are solely those of the authors and do not necessarily represent those of their affiliated organizations, or those of the publisher, the editors and the reviewers. Any product that may be evaluated in this article or claim that may be made by its manufacturer is not guaranteed or endorsed by the publisher.
Research integrity at Frontiers
Learn more about the work of our research integrity team to safeguard the quality of each article we publish.