Corrigendum: Establishment of wind gust estimation equation in Bohai Sea
- National Meteorological Center, China Meteorological Administration, Beijing, China
Wind gusts over the ocean can have a great influence on maritime transport and production operations. However, owing to the scarcity of marine observation data and the complex causes of wind gusts, the estimation and forecasting of gusts at sea are even more difficult. In this study, a gust estimation equation applicable to the Bohai Sea is established based on observation and reanalysis data, with representative observation stations on the coast and offshore in the Bohai Sea. During the process, partial correlation analysis and a significance test of correlation coefficient differences were used to further clarify the influence of average wind speed and vertical wind shear at different heights on wind gusts. The final gust estimation equation includes a mean wind speed term, a turbulence term, and a convection term, which are statistically significant contributors; in addition, there are clear physical meanings. Comparing two gust factor methods in this study, the average errors and fitting errors of the least squares gust estimation equation are the smallest. For wind gusts between 10 and 26 m/s, the fitting error in the gust estimation equation is 7.68–12.25% and 21.10–30.08% lower than those of the two gust factor methods in this study. The gust estimation equation better reflects the difference of wind gusts typical under different average wind speeds and vertical wind shear conditions, so a better prediction for wind gusts can be achieved.
Introduction
Wind gusts are strong, short-duration wind events that are measured by the average wind speed over several seconds. Wind gusts exceeding a certain threshold have a significant impact on housing, transportation, and/or maritime production (Sheridan, 2018; Teoh et al., 2019; Su et al., 2021). Accurate and timely wind gust forecast information can effectively reduce the loss of life and property. The formation mechanism of wind gusts is complex and is not only related to average wind speed and boundary layer turbulence but also affected by surface roughness and atmospheric stability (Harris and Kahl, 2017). For this reason, the prediction of wind gusts is a difficult problem in operational weather forecasting (Kahl, 2020). The wind gust factor was an early wind gust forecasting metric that was calculated as the ratio of the maximum wind speed to the average wind speed within a set time period. From this metric, the strength of wind speed pulsation was obtained, and the expected maximum wind gust value was estimated (Sherlock, 1952). The wind gust factor methodology is still widely used in practical operational forecasting owing to its simplicity and convenience (Cook et al., 2008; Blaes et al., 2014). However, because of the influence of different underlying surface conditions, altitude, and weather systems, the wind gust factor varies significantly. Therefore, in order to obtain more accurate wind gust forecasts, there has been considerable research on the factors driving wind gusts (Davis and Newstein, 1968; Krayer and Marshall, 1992; Ágústsson and Ólafsson, 2004; Yu and Chowdhury, 2009; Kahl et al., 2021). The wind gust factor methodology considers only the general relationship between the average wind speed and wind gusts and ignores the effects of the vertical distribution of upper-level wind speeds on surface wind gusts. When the vertical wind shear near the surface is large, strong turbulent momentum flux will occur, and this strong turbulent momentum flux will increase the surface wind gusts. Strong vertical wind shear in the troposphere is conducive to the development of convective weather, and when convective weather occurs, downdrafts in the troposphere reach the surface, thereby increasing the surface wind gusts (Brasseur, 2001; ECMWF, 2016; Minola et al., 2020). Therefore, by considering the effects of turbulence and convection on the average surface wind speed, which the wind gust factor method ignores, more accurate wind gust predictions can be obtained.
Compared with the average wind speed, strong wind gusts offshore have a greater impact on the safety of both maritime shipping and offshore production operations. However, the lack of observational data at sea, either high-altitude observations or ground-level observations, limits the accurate representation of the initial marine meteorological conditions in numerical models. This restricts the development of objective forecasting methods over the oceans. However, the underlying conditions at the sea surface are relatively uniform, which provides favorable conditions for the development of wind gust forecasting at sea (Leston et al., 2018).
The Bohai Sea is a relatively small inland sea in northern China, which has a substantial network of in situ observing sites, such as coastal observation sites, buoys, and oil platforms. Therefore, this study will use the gusts and mean wind observation data of these stations and the upper-air wind field data from reanalysis data to establish a gust estimation model that can be applied to the Bohai Sea. Through this model, the wind gusts can be more accurately estimated for varying average wind speeds and vertical wind shear conditions to provide a new method for wind gust forecasting in the Bohai Sea.
Data
In this article, we use hourly wind speed data from surface observations taken by the China Meteorological Administration during 2017–2019 and wind field data on 1,000, 925, and 850 hPa from the ERA5 reanalysis data to establish an hourly wind gust forecast model for the Bohai Sea. The spatial resolution of the ERA5 reanalysis data is 0.25 × 0.25. A bilinear interpolation method is used to interpolate the upper-air data to the locations of the selected coastal and representative offshore stations shown in Figure 1. There are nine representative stations selected from the coastal and offshore areas in this study. The average elevation of the selected stations is 10 m, so the wind speed observation data do not need to be corrected in height. When using wind speed data to calculate wind gusts, the wind speed should be averaged over a 10-min interval (Harper et al., 2010; WMO, 2012). However, the numerical prediction model used in weather forecasting mainly outputs the wind speed at the forecast time. Therefore, in order to apply the established wind gust estimation equation in operational forecasting in the future, the average wind speed at the observation time is used instead of the 10-min average wind speed. In this study, it is a 2-min average and is recorded as integer values.
Factor Analysis
In general, the speed of wind gusts rises with the increase of the average wind speed. The observational data from 2017 to 2019 show that when the average wind speed is between 14 and 16 m/s, the corresponding average value of observed wind gusts is approximately 19 m/s, and when the average wind increases to 22–24 m/s, average wind gusts can reach 28 m/s. Since there is only one parameter, there is a direct relationship between the average wind speed and the gust prediction. However, in practice, different gusts can occur at any specific average wind speed (Figure 2). Therefore, as the average wind speed is an important factor in gust estimation, it alone is not an accurate predictor for the wind gust speed.
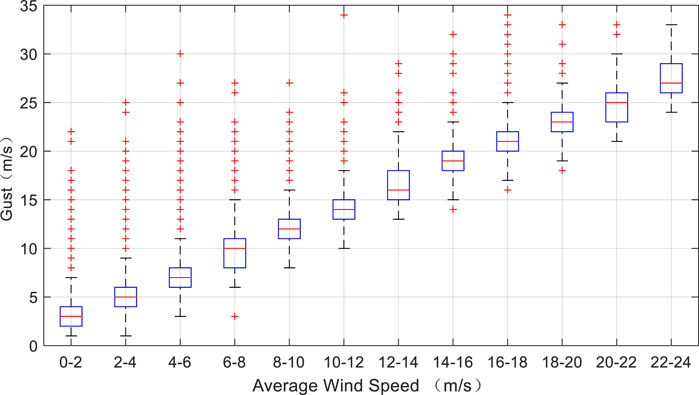
FIGURE 2. Boxplots of average wind speed and wind gust from 2017 to 2019. The boxplot corresponds to the minimum, 25th percentile, 50th percentile (red lines), 75th percentile, and maximum values from bottom to top, respectively. Red crosses in the figure represent outliers that are more than 1.5 quartiles away from the 75th percentile or 25th percentile of the box.
Turbulence is the main characteristic of the atmospheric boundary layer and plays an important role in the transfer of heat, momentum, and water vapor between the surface and the upper atmosphere (Gousseau et al., 2012). Strong vertical wind shear is conducive to the enhancement of turbulence (Kim et al., 2003). The height of 1,000 hPa is close to the ground, so this study attempts to use the difference between the wind speed of 1,000 hPa (F1000) and that at 10 m (F10) to estimate the vertical wind shear in the near-ground layer. Figure 3 is an array boxplot of different average wind speeds from 2017 to 2019 under the conditions of F1000-F10 > 0 and F1000-F10 ≤ 0. As is shown in Figure 3, by comparing the gust distribution in the two cases, it can be seen that under the same average wind speed, the larger gust is more likely to appear in the case of F1000-F10 > 0. The case of F1000-F10 > 0 may correspond to a stronger turbulence, which would be associated with larger surface wind gusts.
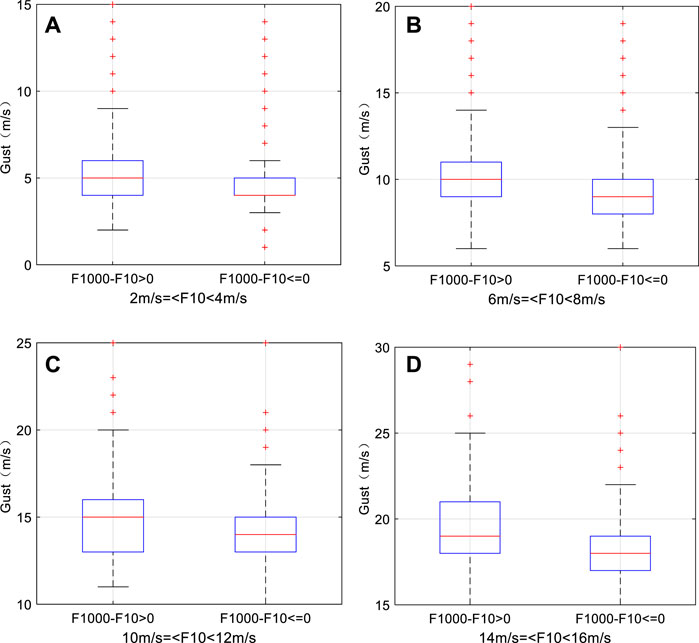
FIGURE 3. Boxplots of gusts under different average wind speeds when F1000-F10 > 0 and F1000-F10 ≤ 0 from 2017 to 2019: (A) 2 m/s ≤ F10 < 4 m/s; (B) 2 m/s ≤ F10 <4 m/s; (C) 2 m/s ≤ F10 < 4 m/s; and (D) 2 m/s ≤ F10 < 4 m/s.
When a severe convective weather system occurs, downdrafts in the troposphere will transport momentum downward and affect surface wind gusts. However, severe convective weather has the characteristics of small spatial scale, short life, and strong suddenness. The prediction of severe convective weather is still a difficult problem (Grabowski et al., 2019), and it is even more difficult to quantitatively calculate the surface gusts caused by severe convective weather. In the gust forecast product of the European Center for Medium-Range Weather Forecasts (ECMWF) model, the maximum positive wind speed difference between 850 and 950 hPa is used to represent the gust caused by convective weather, so as to realize the quantitative calculation of convective gust (ECMWF, 2016). In this study, the vertical wind shears between the 850- and 1,000-hPa surfaces (F850-F1000) and between the 850 and 925-hPa surfaces (F850-F925) are used to characterize the effects of convection.
Correlation Analysis
Since the average surface wind speed and vertical wind shear both can affect the intensity of surface wind gusts, the relationship between them needs to be further clarified. The simple correlation coefficient only shows the degree of linear relation between two variables and does not fully clarify the relationship between those independent variables and the dependent variables in multiple regression analysis. Therefore, a partial correlation analysis needs to be performed, which considers other impact factors and temporarily ignores their influence, to study the relationship between the two remaining factors (Baka et al., 2004). When the partial correlation coefficient is first order, there are only two influence factors. The influence of one independent variable is being controlled, and the relationship between the other independent variable and the dependent variable is calculated according to the following (Hu and Wu, 2020):
where
where
The vertical wind shear is divided into four cases, and the partial correlation coefficients of the wind shear variables under the four different vertical wind shear conditions from 2017 to 2019 are calculated. By using partial correlation analysis to compare the effects of F850-F925 and F850-F1000 on surface gusts, it is found that the effects of F850-F925 is smaller than that of F850-F1000 (not show), so F850-F1000 is finally selected to represent the gust caused by convective weather (Table 1). The partial correlation coefficients in Table 1 are all significant at the 95% level. These results show that the partial correlation coefficient of the average wind speed is the largest regardless of the vertical wind shear conditions, indicating that the average wind speed is the most important factor affecting the surface wind gusts. When F1000-F10 > 0, the partial correlation coefficients are 0.314 and 0.346 (Case 1 and Case 2), which are much larger than 0.072 and 0.135 when F1000-F10 ≤ 0 (Case 3 and Case 4). When F850-F1000 > 0 (Case 1 and Case 3), the partial correlation coefficients are 0.07 and 0.103, which are also greater than 0.016 and 0.02 when F850-F1000 ≤ 0 (Case 2 and Case 4).
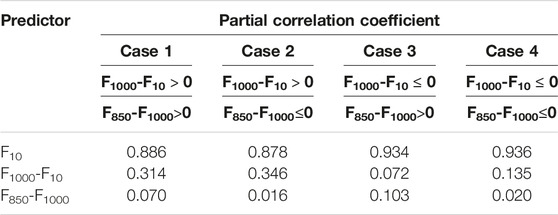
TABLE 1. Partial correlation coefficients of forecast factors under different vertical wind shear conditions from 2017 to 2019.
The partial correlation coefficients are calculated from the corresponding sample data. However, before comparing different correlation coefficients, a statistical test should be performed to make sure whether the difference between different correlation coefficients is statistically significant or possibly caused by sampling differences or random chance. In 1915, Fisher proposed the Fisher Z transform, which can convert correlation coefficients that do not conform to normal distribution into variables that conform to normal distribution (Needham, 1978). The formula is calculated as follows:
where
where Zr1 and Zr2 are the transformed correlation coefficients and VAR(Zr1–Zr2) is calculated by the following:
where n1 and n2 represent the sample sizes of variables z1 and z2 in the data, respectively. The numbers of samples under four different vertical wind shear conditions in this study are 85,453, 49,929, 24,365, and 10,333 (Case 1–Case 4, respectively). When the absolute value of Z exceeds 1.96, the differences in the correlation coefficients of the two variables are statistically significant at the 95% confidence level. When the absolute value of Z is less than 1.96, the correlation coefficients are not significantly different.
The above procedure is used to test the difference of correlation coefficients for the different vertical wind shear conditions. The results show that only two of the correlation coefficient differences are not statistically significant. The first is F10 for the two cases where F1000-F10 is nonpositive and the partial correlation coefficients are 0.934 and 0.936, and the second is F850-F1000 for the two cases where F1000-F10 is positive and the partial correlation coefficients are 0.016 and 0.020. The significance test shows that the correlation coefficient between different samples can be compared, and the larger the correlation coefficient is, the greater the influence on the gusts will be. When F1000-F10 > 0, the impact of F1000-F10 on gusts is greater than that of F1000-F10 ≤ 0. The corresponding physical meaning of this correlation is likely related to the fact that the positive vertical wind shear in the near-ground layer can stimulate strong turbulence, thereby enhancing gusts. When F850-F1000 is greater than 0, it also has a greater impact on gusts, and its corresponding physical meaning is that the positive vertical wind shear is more likely to cause downdrafts in the troposphere to affect the surface gusts.
Therefore, the influence of F1000-F10 ≤ 0 and F850-F1000 ≤ 0 will be ignored when establishing the gust estimation equation, and the equation established in this way has a clearer physical meaning. The influence of different factors on surface wind gusts is more clearly revealed using partial correlation analysis and the significance test of correlation coefficient differences, which provide a basis for the establishment of a wind gust estimation equation.
Estimation Equation
The multiple linear gust estimation equation established from a multiple regression analysis can be written in the form of a piecewise function, as follows:
where
where
As shown in Table 2, in the case when F1000-F10 ≤ 0 and F850-F1000 ≤ 0 (Case 4), the only significant influencing factor in the wind gust estimation equation is F10. In this case, the R2 of the estimation equation is 0.987, which is the largest of all estimation equations. R2 is the goodness of fit, and the closer the value is to 1, the better the fitting effect is. In this case, only using F10 as a predictor achieves a better forecast of the wind gusts, and the standard error of the estimated value is the smallest of all cases (1.437 m/s). When F1000-F10 > 0 and F850-F1000 > 0 (Case 1), there are three factors in the estimation equation. In this case, the
where
The wind gust estimation equation involves the wind speed on the 1,000-hPa surface. However, when the height of the 1,000-hPa surface is less than 10 m, F1000-F10 and F850-F1000 have no real physical meaning. Therefore, in this study, when the height of the 1,000-hPa surface is less than 10 m, the 1,000-hPa surface height data from the ERA5 reanalysis data is used to calculate the relationship between the 1,000- and 850-hPa wind speeds and the 10-m wind speed. However, the situation when the height of the 1,000-hPa surface is below 10 m and F1000-F10 is positive accounts for only ∼1.7% of all samples during 2017–2019, and the average value of F1000-F10 is ∼1.95 m/s. This situation primarily occurs when F10 is relatively small (1–3 m/s), with a frequency of roughly 51.6% of the time. The proportion of cases when the height of the 1,000-hPa surface is below 10 m and F850-F1000 is positive is approximately 2.2%, of which roughly 64.7% of the magnitudes of F850-F1000 are less than 5 m/s. In general, when the height of the 1000-hPa surface is below 10 m, F1000-F10 and F850-F1000 have relatively little meaning and have little effect on the wind gust estimates.
Perform Validations
In this study, according to the definition of the gust factor method, two wind gust factors are calculated using the hourly wind speed observation data from 2017 to 2019. The first is the gust factor calculated based on different average wind speeds (GF1), and the second is the gust factor calculated based on the overall sample (GF2). As shown in Figure 4, GF1 monotonically decreases with the increase of average wind speed. When the average wind speed is 0–2 m/s, GF1 is 3.6155. When the average wind speed increases to 22–24 m/s, GF1 is 1.2314. At each average wind speed, GF2 is 1.5.
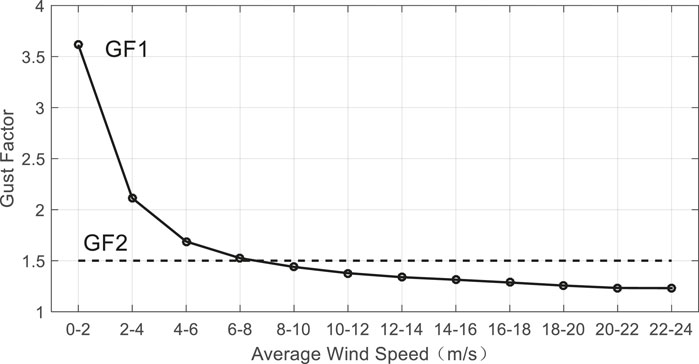
FIGURE 4. The gust factors as a function of average wind speed (GF1) and overall sample (GF2) calculated from the data from 2017 to 2019.
The gust estimation equation arising from the multiple regressions (MR) and the gust factors GF1 and GF2 are used to hind cast the data from 2017 to 2020 and compare their mean errors and fitting errors. The fitting error is estimated by the following:
where
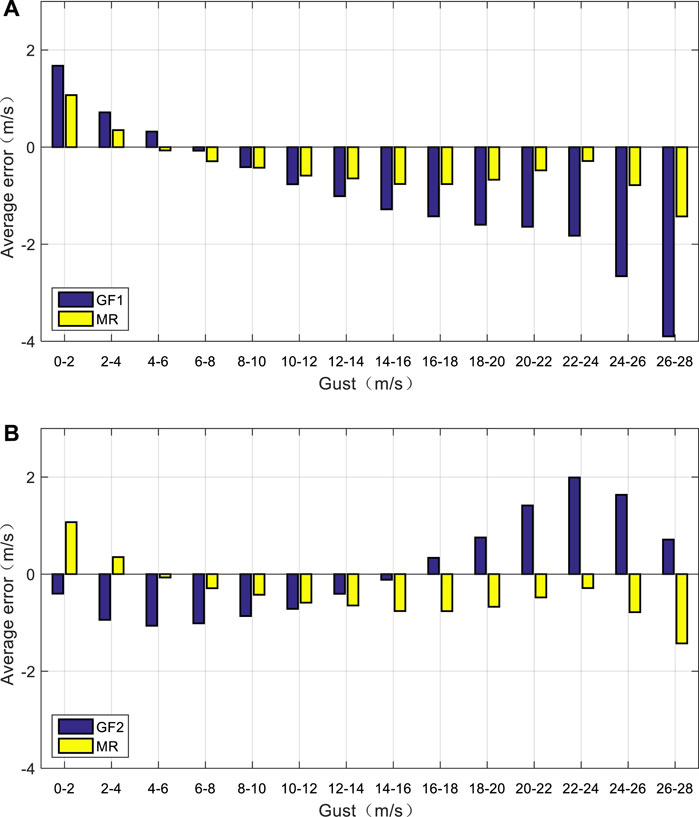
FIGURE 5. Comparison of mean error in the multiple regression model to that in the (A) gust factor and (B) gust factor 2 models from 2017 to 2019.
However, the mean error comparison does not prove that the estimation capability of the MR model is better than that of the GH1 or GF2 model. Table 3 shows the reduction of percentage in the fitting error of the MR model related to the fitting error in the GF1 and GF2 models. In Table 3, except when the observed wind gusts are between 2 and 4 m/s, the fitting error reduction rate of MR relative to that of the GF1 model is negative, and all others are positive. For wind gusts between 12 m/s and 22 m/s, the fitting error in the MR model is 10.99–12.25% lower than that of the GF1 model. For wind gusts between 4 and 26 m/s, the fitting error reduction of the MR model is more than 20% relative to that of the GF2 model.
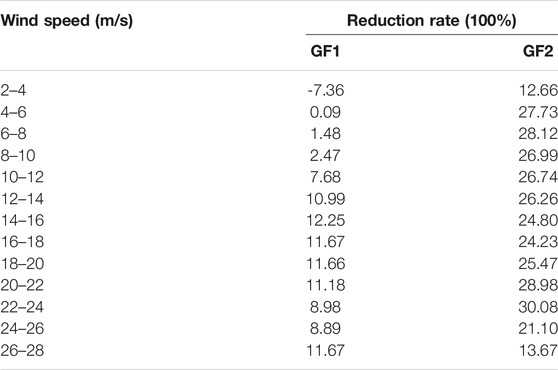
TABLE 3. Reduction rate of the multiple regression model relative to the gust factor 1 and gust factor 2 fitting error in 2017–2019.
Figure 6 shows the scatter diagrams of absolute errors of the MR and GF1 models. As can be seen, when the absolute error of GF1 is small, the absolute error of MR is also small, so that the scattered points near the origin are densely packed. As the absolute error of GF1 increases, the scattered points begin shifting to the left of the diagonal, indicating that the MR error is less than that in GF1. Figure 7 shows the scatter diagrams comparing the absolute error of the MR and GF2 models. The results in Figure 7 are basically the same as those in Figure 6; the absolute error in the MR model has fewer large deviations in the gust than the GF2 model does.
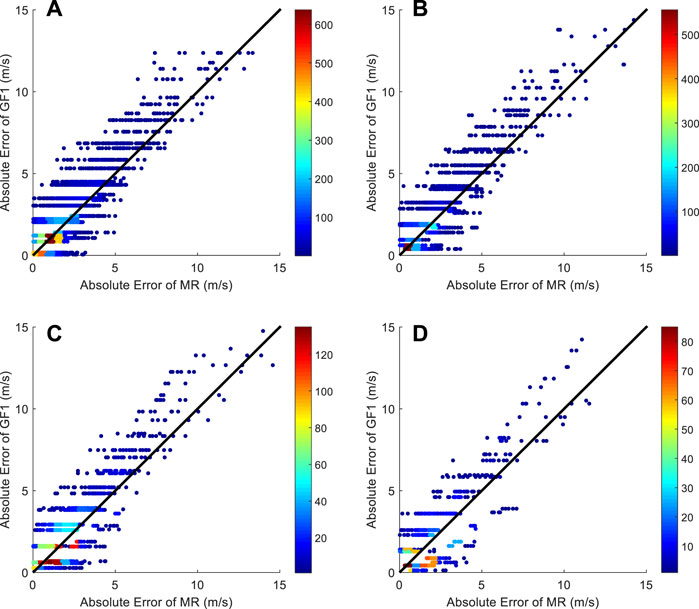
FIGURE 6. The scatter diagrams of absolute errors of the multiple regression method (MR) and the wind gust factor method (GF1) for four different observed gust magnitudes: (A) 14–16 m/s, (B) 16–18 m/s, (C) 18–20 m/s, (D) 20–22 m/s. The color in the scatter in the figure indicates the number of repeated scatter points.
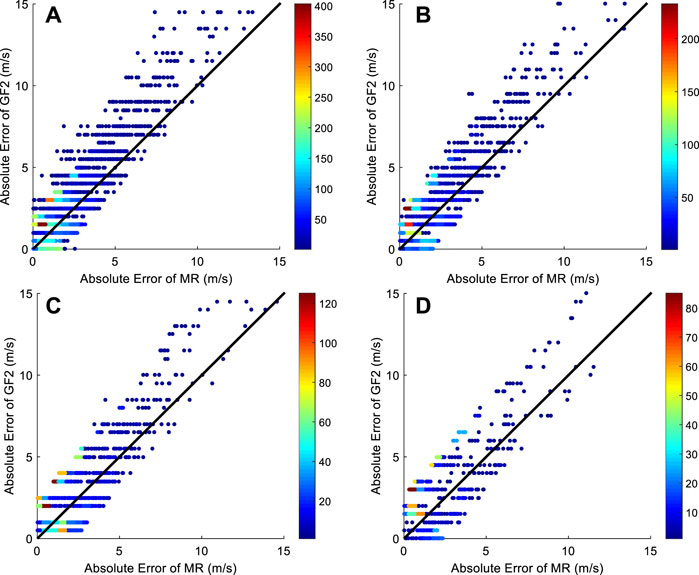
FIGURE 7. The scatter diagrams of absolute errors of the multiple regression method (MR) and the wind gust factor method (GF2) for four different observed gust magnitudes: (A) 14–16 m/s, (B) 16–18 m/s, (C) 18–20 m/s, (D) 20–22 m/s. The color in the scatter in the figure indicates the number of repeated scatter points.
By comparing the mean error, fitting error, and the scatter diagrams of the absolute error between the gust estimation equations method and the two gust factor methods, it is clear that the regression estimation equation is considerably better than the other two gust factor methods. This is because the regression considers both average wind speed and wind shear at different heights.
Summary and Discussion
In this study, based on both observational and ERA5 reanalysis data, the relationship among average wind speed, vertical wind shear at different levels in the lower atmosphere, and surface wind gusts is determined by the analysis of partial correlation and the development of wind gust estimation equation suitable for Bohai Sea using the least squares method. By comparing the gust estimation equation and the two gust coefficients, the results are as follows:
1. The average wind speed term F10 and the wind shear terms F1000-F10 and F850-F1000 are selected to establish an estimation equation for wind gusts in the Bohai Sea. While average wind speed is the dominant factor in the surface wind gust estimation, the near-surface wind shear is also an important term. Owing to the low frequency and intermittency of convective weather systems, the effects of convection as estimated using the wind shear across 850–1,000 hPa are relatively small.
2. Based on the significance test of the correlation coefficient difference, it is clear that the positive vertical wind shear has a greater impact on the ground gust than the negative vertical wind shear. The gust estimation equation ultimately includes the importance of vertical wind shear, which is more physically meaningful.
3. Compared with the two gust factor methods presented in this study (GF1 and GF2), the mean error and fitting error of the least squares regression gust estimation equation (MR) are the smallest. For wind gusts between 10 and 26 m/s, the fitting error in the gust estimation equation is 7.68–12.25% and 21.10–30.08% lower than those of the two gust factor methods in this study. The least squares regression gust estimation equation includes the effects of the average wind speed and different vertical wind shear conditions, so a better prediction for the wind gusts can be achieved.
The surface 10-m wind speed and 1,000- and 850-hPa wind speed data predicted using the numerical model can be applied in the gust estimation equation to realize the gust forecast. In this study, the gust estimation equation was established based on the Bohai Sea. If the gust estimation equation is applied to other sea areas, it is necessary to reselect representative stations to calculate the regression coefficients of different influencing factors. The regression coefficients of different sea areas will be slightly different. The gust estimation equation in this study considers only the average surface wind speed and vertical wind shear and does not consider the influence of temperature at different heights on gust. At the same time, the method is a linear regression method, which does not take the nonlinear relationship among different influencing factors and gusts into consideration. In future work, the influence of thermal factors will be considered, and the forecasting model of gust will be constructed using a nonlinear method, so as to further improve the forecasting ability of gust.
Data Availability Statement
Publicly available datasets were analyzed in this study. The data can be found here: http://data.cma.cn/data/detail/dataCode/A.0012.0001.html https://www.ecmwf.int/en/forecasts/datasets/reanalysis-datasets/era5.
Author Contributions
HH: data analysis, method establishment, and article writing. BH: method guide. KD: method improvement. HQ: data collection and analysis.
Funding
The study received funding from the National Key Research and Development Plan of China (2021YFC3000905).
Conflict of Interest
The authors declare that the research was conducted in the absence of any commercial or financial relationships that could be construed as a potential conflict of interest.
Publisher’s Note
All claims expressed in this article are solely those of the authors and do not necessarily represent those of their affiliated organizations, or those of the publisher, the editors, and the reviewers. Any product that may be evaluated in this article, or claim that may be made by its manufacturer, is not guaranteed or endorsed by the publisher.
References
Ágústsson, H., and Ólafsson, H. (2004). Mean Gust Factors in Complex Terrain. Meteor. Z. 13, 149–155.
Al-Ashkar, I., Ibrahim, A., Ghazy, A., Attia, K., Al-Ghamdi, A. A., and Al-Dosary, M. A. (2021). Assessing the Correlations and Selection Criteria between Different Traits in Wheat Salt-Tolerant Genotypes. Saudi J. Biol. Sci. 28, 5414–5427. doi:10.1016/j.sjbs.2021.05.076
Ashok, K., Nakamura, H., and Yamagata, T. (2007). Impacts of ENSO and Indian Ocean Dipole Events on the Southern Hemisphere Storm-Track Activity during Austral Winter. J. Clim. 20, 3147–3163. doi:10.1175/jcli4155.1
Baka, K., Shibata, R., and Sibuya, M. (2004). “Partial Correlation and Conditional Correlation as Measures of Conditional Independence,”, 657–664.Aust. N. Z. J. Statistics46
Blaes, J. L., Glenn, D., Hawkins, D., Oliva, V., et al. (2014). “Developing a Dataset of Wind Gust Factors to Improve Forecasts of Wind Gusts in Tropical Cyclones,” in 39th National Weather Association Annual Meeting (Salt Lake City, UT: National Weather Association), P1.43.
Brasseur, O. (2001). Development and Application of a Physical Approach to Estimating Wind Gusts. Mon. Wea. Rev. 129, 5–25. doi:10.1175/1520-0493(2001)129<0005:daaoap>2.0.co;2
Cook, K. R., Gruenbacher, B., and Williams, L. D. (2008). Wichita,KS: National Weather Service, 21.Assessment of Science and Methodologies to Forecast Wind and Wind Gust Speed12th Annual High Plains Conf.
Davis, F. K., and Newstein, H. (1968). The Variation of Gust Factors with Mean Wind Speed and with Height. J. Appl. Meteor. 7, 372–378. doi:10.1175/1520-0450(1968)007<0372:tvogfw>2.0.co;2
Delsole, T. (2007). A Bayesian Framework for Multimodel Regression. J. Clim. 20, 2810–2826. doi:10.1175/jcli4179.1
European Center for Medium-Range Weather Forecasts (ECMWF) (2016). IFS Documentation CY45R2—Part IV: Physical Processes. IFS doc. Reading, UK: ECMWF.
Gousseau, P., Blocken, B., and Van Heijst, G. J. F. (2012). Large-eddy Simulation of Pollutant Dispersion Around a Cubical Building: Analysis of the Turbulent Mass Transport Mechanism by Unsteady Concentration and Velocity Statistics. Environ. Pollut. 167, 47–57. doi:10.1016/j.envpol.2012.03.021
Grabowski, W. W., Morrison, H., Shima, S.-I., Abade, G. C., Dziekan, P., and Pawlowska, H. (2019). Modeling of Cloud Microphysics: Can We Do Better? Bull. Amer. Meteor. Soc. 100, 655–672. doi:10.1175/bams-d-18-0005.1
Harper, B. A., Kepert, J. D., and Ginger, J. D. (2010). Guidelines for Converting between Various Wind Averaging Periods in Tropical Cyclone Conditions. Geneva: World Meteorological Organization Tech.Doc. WMO/TD-1555, 64.
Harris, A. R., and Kahl, J. D. W. (2017). Gust Factors: Meteorologically Stratified Climatology, Data Artifacts, and Utility in Forecasting Peak Gusts. Appl. Meteor. Climatol. 56, 3151–3166. doi:10.1175/jamc-d-17-0133.1
Hu, C. C., and Wu, C. C. (2020). Ensemble Sensitivity Analysis of Tropical Cyclone Intensification Rate during the Development Stage. J. Atmos. Sci. 77, 3387–3405. doi:10.1175/jas-d-19-0196.1
Kahl, J. D. W. (2020). Forecasting Peak Wind Gusts Using Meteorologically Stratified Gust Factors and MOS Guidance. Weather Forecast. 35, 1129–1143. doi:10.1175/waf-d-20-0045.1
Kahl, J. D. W., Selbig, B. R., and Harris, A. R. (2021). Meteorologically Stratified Gust Factors for Forecasting Peak Wind Gusts across the United States. Am. Meteorological Soc. 102, 1665–1671. doi:10.1175/bams-d-21-0013.1
Kim, S. W., Park, S. U., and Moeng, C. H. (2003). Entrainment Processes in the Convective Boundary Layer with Varying Wind Shear. Boundary-Layer Meteorol. 108, 221–245. doi:10.1023/a:1024170229293
Krayer, W. R., and Marshall, R. D. (1992). Gust Factors Applied to Hurricane Winds. Bull. Amer. Meteor. Soc. 73, 613–618. doi:10.1175/1520-0477(1992)073<0613:gfathw>2.0.co;2
Lala, J., Tilahun, S., and Block, P. (2020). Predicting Rainy Season Onset in the Ethiopian Highland for Agricultural Planning. J. Hydrometeorol. 21, 1675–1688. doi:10.1175/jhm-d-20-0058.1
Letson, F., Pryor, S. C., Barthelmie, R. C., and Hu, W. (2018). Observed Gust Wind Speeds in the Coterminous United States,and Their Relationship to Local and Regional Drivers. Wind Eng.Ind.Aerodyn. 173, 199–209. doi:10.1016/j.jweia.2017.12.008
Minola, L., Zhang, F., and Azorin-Molina, C. (2020). Near-surface Mean and Gust Wind Speeds in ERA5 across Sweden:towards an Improved Gust Parametrization. Clim. Dyn. 55, 887–907. doi:10.1007/s00382-020-05302-6
Needham, R. M., and Schroeder, M. (1978). Using Encryption for Authentication in Large Networks of Computers. Commun. ACM 21, 993–999. doi:10.1145/359657.359659
Sheridan, P. (2018). Current Gust Forecasting Techniques, Developments and Challenges. Adv. Sci. Res. 15, 159–172. doi:10.5194/asr-15-159-2018
Sherlock, R. H. (1952). Variation of Wind Velocity and Gust with Height. Proc. Amer. Soc. Civ. Eng. 78, 26. (Separate No.126; Paper 2553).
Smoliak, B. V., Wallace, J. M., and Lin, P. (2015). Dynamical Adjustment of the Northern Hemisphere Surface Air Temperature Field: Methodology and Application to Observations. J. Clim. 28, 1613–1629. doi:10.1175/jcli-d-14-00111.1
Su, N., Peng, S., and Hong, N. (2021). Stochastic Dynamic Transient Gusty Wind Effect on the Sliding and Overturning of Quayside Container Cranes. Struct. Infrastruct. Eng. 17, 1271–1283. doi:10.1080/15732479.2020.1809465
Teoh, Y. E., Alipour, A., and Cancelli, A. (2019). Probabilistic Performance Assess¬ment of Power Distribution Infrastructure under Wind Events. Eng. Struct. 197, 109199.1–109199.16. doi:10.1016/j.engstruct.2019.05.041
WMO (2012). Measurement of Surface Wind. Guide to Meteorological Instruments and Methods of Observation. Geneva: World Meteorological Organization. WMO-No. 8, 2008 edition Updated in 2010.
Keywords: wind gust, estimation equation, partial correlation analysis, multiple regression, marine weather forecast
Citation: Hu H, Huang B, Dai K and Qu H (2022) Establishment of Wind Gust Estimation Equation in Bohai Sea. Front. Earth Sci. 10:920048. doi: 10.3389/feart.2022.920048
Received: 14 April 2022; Accepted: 16 June 2022;
Published: 22 July 2022.
Edited by:
Guihua Wang, Fudan University, ChinaReviewed by:
Zhengkun Qin, Nanjing University of Information Science and Technology, ChinaDelei Li, Institute of Oceanology (CAS), China
Copyright © 2022 Hu, Huang, Dai and Qu. This is an open-access article distributed under the terms of the Creative Commons Attribution License (CC BY). The use, distribution or reproduction in other forums is permitted, provided the original author(s) and the copyright owner(s) are credited and that the original publication in this journal is cited, in accordance with accepted academic practice. No use, distribution or reproduction is permitted which does not comply with these terms.
*Correspondence: Bin Huang, hbzbj199928@163.com; Kan Dai, daikan1998@163.com