- 1Department of Cardiology, The Affiliated Hospital of North Sichuan Medical College, Nanchong, Sichuan, China
- 2Department of Thoracic Surgery, Nan Chong Center Hospital, Nanchong, China
Objective: This study aims to evaluate whether radiomic features of pericoronary adipose tissue (PCAT) derived from coronary computed tomography angiography (CCTA) can better predict major adverse cardiovascular events (MACE) in patients with angina pectoris.
Methods: A single-center retrospective study included 239 patients with angina pectoris who underwent coronary CT examinations. Participants were divided into MACE (n = 46) and non-MACE (n = 193) groups based on the occurrence of MACE during follow-up, and further allocated into a training cohort (n = 167) and a validation cohort (n = 72) at a 7:3 ratio. Automatic segmentation of PCAT surrounding the proximal segments of the left anterior descending artery (LAD), left circumflex coronary artery (LCX), and right coronary artery (RCA) was performed for all patients. Radiomic features of the coronary arteries were extracted, screened, and integrated while quantifying the fat attenuation index (FAI) for the three vessels. Univariate and multivariate logistic regression analyses were utilized to select clinical predictors of adverse cardiovascular events. Subsequently, machine learning techniques were employed to construct models based on FAI, clinical features, and radiomic characteristics. The predictive performance of each model was assessed and compared using receiver operating characteristic (ROC) curves, calibration plots, and decision curve analysis for clinical utility.
Results: The radiomics model demonstrated superior performance in predicting MACE in patients with angina pectoris within both the training and validation cohorts, yielding areas under the curve (AUC) of 0.83 and 0.71, respectively, which significantly outperformed the FAI model (AUC = 0.71, 0.54) and the clinical model (AUC = 0.81, 0.67), with statistically significant differences in AUC (p < 0.05). Calibration curves for all three predictive models exhibited good fit (all p > 0.05). Decision curve analysis indicated that the radiomics model provided higher clinical benefit than the traditional clinical and FAI models.
Conclusion: The CCTA-based PCAT radiomics model is an effective tool for predicting MACE in patients with angina pectoris, assisting clinicians in optimizing risk stratification for individual patients. The CCTA-based radiomics model significantly surpasses traditional FAI and clinical models in predicting major adverse cardiovascular events in patients with angina pectoris.
1 Introduction
As public health awareness increases, the incidence of coronary artery disease (CAD) has seen a decline. However, it continues to pose a threat to human safety globally (1). According to recent statistics, half of the patients diagnosed with CAD suffer from angina pectoris (2). Angina is defined as chest pain, pressure, or discomfort located behind the sternum, which is often exacerbated by exertion and/or anxiety or other emotional or psychological stresses (3, 4). It is a highly prevalent condition that seriously endangers individuals’ health. With advancing age, the prevalence of angina increases, and concurrently, the risk of cardiovascular and cerebrovascular accidents such as congestive heart failure, myocardial infarction, and malignant arrhythmias also rises (5, 6). Therefore, identifying high-risk angina patients is crucial for effective risk stratification, patient management, and rational allocation of public health resources. Currently, the monitoring and assessment of the progression of angina primarily rely on traditional clinical factors such as age, risk factors, and medication history (7, 8). For patients with worsening chest pain symptoms or increased frequency of episodes, coronary CTA, a preferred non-invasive imaging method in clinical practice, is used for further examination. Based on the results of vascular stenosis, we then assess and predict the likelihood of patients experiencing major adverse cardiovascular events (9, 10). Recent research indicates that the formation and rupture of atherosclerotic plaques are inseparably linked to inflammation within the vessel (11). When vascular inflammation occurs, pro-inflammatory factors released diffuse into the PCAT via paracrine signaling, inhibiting preadipocyte differentiation and lipid accumulation (12–14). Researchers have discovered a new sensitive biomarker of coronary artery inflammation—the fat attenuation index (FAI)—which captures the CT attenuation gradient of PCAT, thereby revealing changes in the composition of PCAT induced by vascular inflammation (10). However, the pathophysiology of coronary vascular lesions is complex, and relying solely on FAI provides only a planar calculation of changes, failing to reflect the complexity of spatial variations (15). With the application of artificial intelligence in clinical radiology, advanced radiomics technology is playing an increasingly pivotal role in the medical field. Radiomics is a technique for fine phenotypic analysis of extracted tomographic images. In essence, this technology converts images into data (12, 13). It has been widely applied in the clinical diagnosis, prognosis, and postoperative monitoring of tumors (14, 16). Recently, the utility of radiomics combined with coronary CT in the cardiovascular domain has gained increasing attention. Numerous studies have also verified the superiority of radiomic features of pericoronary adipose tissue in diagnosing myocardial infarction and differentiating coronary heart disease (17, 18). However, the role of radiomics of pericoronary adipose tissue based on CCTA in predicting major clinical events has not yet been further investigated. Therefore, our study aims to explore the role of radiomic features based on CCTA in predicting major adverse cardiovascular events in patients with angina pectoris. In addition, we aim to compare it with traditional imaging techniques and clinical methods to determine whether it can improve and optimize the predictive performance for MACE in patients with angina pectoris.
2 Materials and methods
2.1 Study population
A retrospective collection was conducted on 239 patients with angina pectoris who underwent CCTA examinations at the Cardiac Center of the Affiliated Hospital of North Sichuan Medical College from February 2019 to December 2023. The diagnosis of angina pectoris was made by groups led independently by two cardiologists from our institution, based on medical history, physical examination, laboratory tests, and imaging studies. Exclusion criteria included a history of myocardial infarction or revascularization, poor CCTA image quality, and incomplete medical records. A detailed flowchart outlining the patient selection process and study design is provided in Figure 1. This study was approved by the Medical Ethics Committee of the Affiliated Hospital of North Sichuan Medical College (approval number: 2023ER218-1).
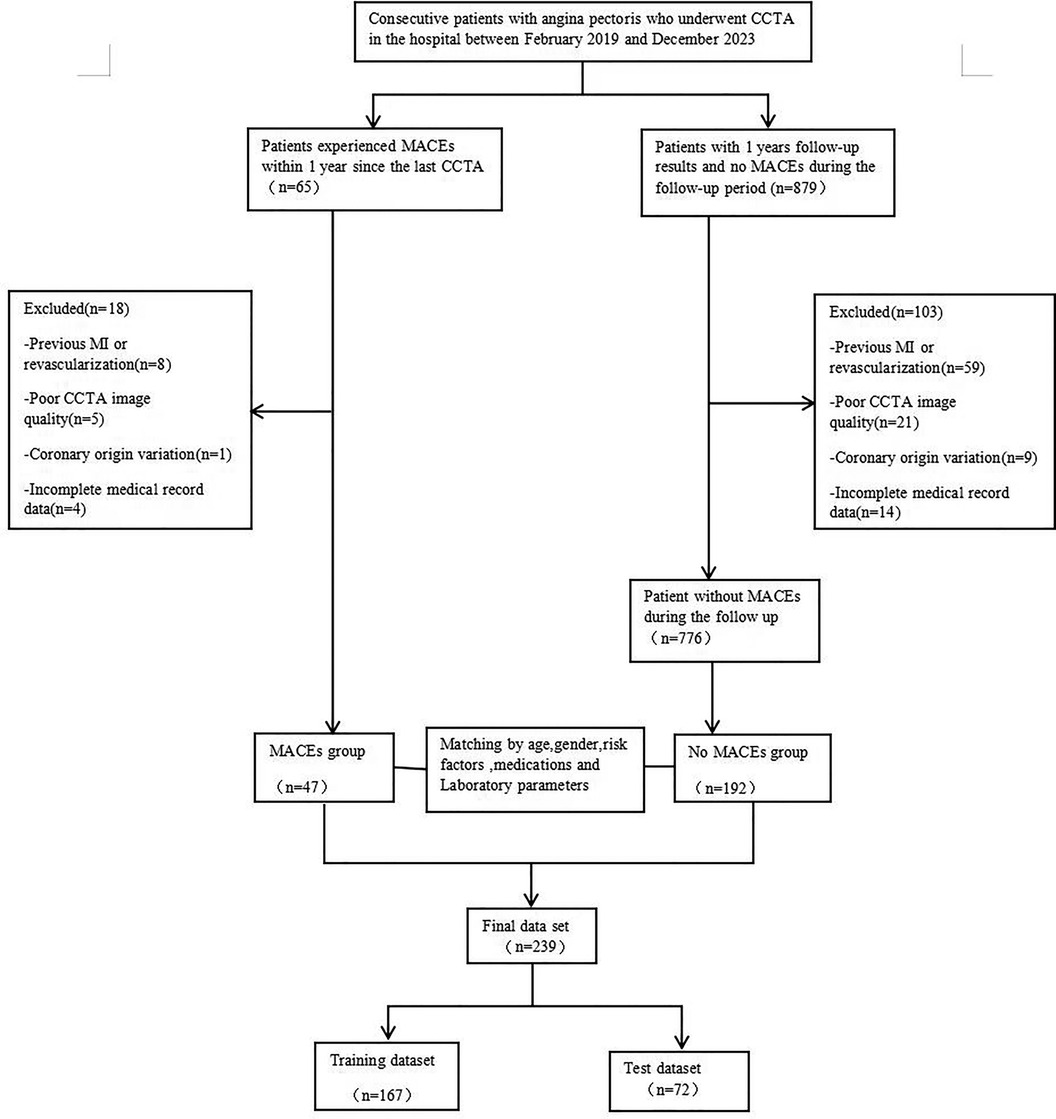
Figure 1. A flowchart of patient recruitment and study design. CCTA, coronary computed tomography angiography; MACE,Major adverse cardiovascular event;MI, myocardial infarction.
2.2 General data collection and clinical endpoints
Relevant clinical history data were collected for all patients, including demographic characteristics (gender, age, and body mass index), cardiovascular risk factors (smoking, hypertension, diabetes, hyperlipidemia, and medication history), Renal Function Measurement [Estimated glomerular filtration rate (eGFR), Serum creatinine (Scr) levels and blood urea nitrogen (BUN)] as well as laboratory and echocardiographic parameters.
The clinical endpoint was the occurrence of MACEs, defined as a composite of death, malignant arrhythmias, new-onset congestive heart failure, acute myocardial infarction, and cerebral infarction within one year.
Anti-Anginal Therapy Adjustments: During the follow-up period, modifications in anti-anginal therapy were documented for all patients. Adjustments included changes in the dosage or addition of medications such as beta-blockers, calcium channel blockers, and nitrates, based on patients’ symptoms and clinical assessments. These modifications were tracked and analyzed as part of the study.
2.3 CCTA acquisition
All recruited subjects underwent scanning with a 2*96-detector row CT scanner (Stellar Infinity detector, SOMATOM Force, Siemens Healthineers, Germany). The scanner operated with prospective ECG gating and tube voltage ranging from 70 to 150 kV, a rotation speed of 0.25 s, and a temporal resolution of 66 ms per segment, enabling free heart rate coronary CT. It also utilized Edge and UHR technology with a holographic photon detector that reduces electronic crosstalk between adjacent detectors, allowing the reconstruction of thin slices from a 0.6 mm acquisition layer thickness to 0.4 mm. Due to the frequent and substantial gas exchange during respiration, which can impair the field of view, patients were instructed to hold their breath as much as possible during the coronary CT scan. Additionally, patients were positioned supine, with as much of the anterior chest exposed as possible. The instrument scanned from the head downwards, capturing images from 1 cm below the tracheal bifurcation to the apex of the heart.
2.4 Quantification measurement of FAI
PCAT has become a new significant target for the detection of coronary artery disease, located at a radial distance from the vessel wall, approximately equivalent to the diameter of the vessel. FAI is determined by calculating the average attenuation value of the perivascular adipose tissue (i.e., the average CT value of PCAT), and to exclude non-adipose tissues, the CT value range is generally set between −190 and −30 HU in studies. In previous research, the FAI of the surrounding tissue near the proximal RCA has been found to be more representative of inflammation compared to other coronary artery fat attenuation tissues and is considered a good indicator of systemic inflammation (19, 20). Therefore, we will use software to automatically define and measure the proximal area of the RCA (10–50 mm from the RCA ostium), ensuring high standardization and repeatability. FAI for all included patients was measured using CoronaryDoc software version 6.21 (Shukun Technology Co., Ltd, Beijing, China).
2.5 PCAT segmentation and radiomic feature extraction
Recent studies have suggested that pericoronary adipose tissue (PCAT) surrounding coronary artery plaques may serve as a sensitive imaging biomarker for plaque vulnerability (17). To reflect the changes in coronary arteries during inflammation as comprehensively as possible, we included all three main epicardial vessels for segmentation. Based on the method by Oikonomou (20) et al., we utilized Shukun Technology software [Shukun Technology Co., Ltd, Beijing, China, Version: 6.21] to automatically track a 40 mm segment of interest around the proximal segments of the left anterior descending artery, left circumflex coronary artery, and right coronary artery. For LAD and LCX, a 40 mm segment following the left main bifurcation was analyzed. For the RCA, to avoid the influence of the aortic wall, we excluded the proximal 10 mm and focused on the segment from 10 mm downstream of the aortic root to 50 mm proximal to the RCA. Additionally, while segmenting the plaques in the corresponding coronary regions, we set the cross-sectional area of segmentation to be three times the diameter of the vessel lumen, further ensuring the comprehensiveness of the data. A total of 94 radiomic features were extracted from the PCAT surrounding each coronary artery plaque, including morphological features, first-order histogram features, and higher-order texture features, resulting in a total of 94*3 radiomic features per patient.
2.6 Feature selection and prediction model building
This study attempted to develop three models to determine the prediction of MACE in patients with angina pectoris; the building process for each is detailed below.
2.6.1 Radiomics model
First, the acquired raw imaging data underwent z-score normalization to exclude variables with zero variance and to center the feature values at zero. The normalized radiomic features were then subjected to Mann-Whitney U-tests and selection. Only radiomic features with p-values < 0.05 were retained. Second, we calculated the correlation between features using Spearman's rank correlation coefficient and excluded radiomic features with a correlation coefficient >0.9. Third, the remaining radiomic features underwent final selection using the Least Absolute Shrinkage and Selection Operator (LASSO) algorithm, setting irrelevant feature coefficients precisely to zero while retaining non-zero coefficient features for model fitting (21). After this series of selections, we input the final radiomic features into machine learning (random forest) to construct a predictive risk model.
2.6.2 Clinical model
Independent clinical predictors, including cardiovascular risk factors, laboratory, and echocardiographic parameters, etc., were initially screened using univariate Logistic regression. Variables with P < 0.05 in the univariate analysis were used for subsequent multivariate Logistic analysis to determine the features most correlated with MACE. Clinical features were also input into machine learning (random forest) to develop a clinical prediction model.
2.6.3 FAI model
The construction of the FAI model was nearly identical to the previous two methods. The extracted FAI values around the proximal RCA were used to establish the prediction model, using the same machine learning model. To avoid overfitting and balance the limited sample size, all three ML models were calibrated using a 10-fold cross-validation method (22).
2.7 Statistical analysis
Statistical analyses in this study were performed using R Studio (version 4.0.3) and Python (version 0.13.2) software packages. Continuous variables were assessed using the Mann-Whitney U-test or Student's t-test. Categorical variables were compared using the chi-square test or Fisher's exact test. For continuous (quantitative) data, the Shapiro-Wilk normality test was used to determine the normality of sample data. If the data followed a normal distribution, it was presented as mean ± standard deviation (X ± S), and comparisons between two groups were made using independent sample t-tests; if not normally distributed, data were presented as median (25th percentile, 75th percentile), and comparisons between two groups were made using the Wilcoxon test. A two-sided p-value < 0.05 was considered statistically significant. Receiver operating characteristic curves were used to evaluate the efficacy of the three types of models in predicting the occurrence of MACE in patients with angina pectoris. Calibration curves were plotted to assess the consistency between the predicted probabilities by the models and the actual probabilities. Clinical utility was evaluated through decision curve analysis (DCA), which quantifies net benefit across different threshold probabilities for the three predictive models.
3 Results
3.1 General baseline information
Table 1 summarizes the clinical baseline characteristics of the 239 patients in both the training and validation sets. Among the included patients with angina pectoris, 47 (19.7%) experienced MACEs during the follow-up period: 9 (3.8%) had a new acute ischemic stroke, 10 (4.2%) developed congestive heart failure, 8 (3.3%) suffered malignant arrhythmias, and 20 (8.4%) had a new acute myocardial infarction. Statistical testing showed that clinical features were well-matched between the MACE and non-MACE groups in both the training and validation datasets.
3.2 Feature selection and prediction model building
Radiomic features were extracted from regions of interest (ROIs) corresponding to the three main coronary arteries of patients, including morphological features (24), first-order histogram features (18), and higher-order texture features (51). Following standardization and redundancy reduction via Spearman correlation analysis, 11 radiomic features remained.
After final selection through LASSO regression, the four most relevant features were chosen for the construction of the radiomic feature model. Figure 2 depicts the selection process of the radiomic features in this study. All independent clinical predictors underwent univariate logistic regression analysis, and variables with p < 0.05 were included in subsequent analyses. A stepwise elimination approach was then used to construct a multivariate logistic regression analysis, ultimately identifying age as an independent predictor of major cardiovascular adverse events in patients with angina pectoris (P < 0.05). Based on this result, a clinical model was constructed using machine learning methods, with detailed results presented in Table 2. Lastly, the same methodology was applied to build the FAI model using the extracted fat attenuation index values.
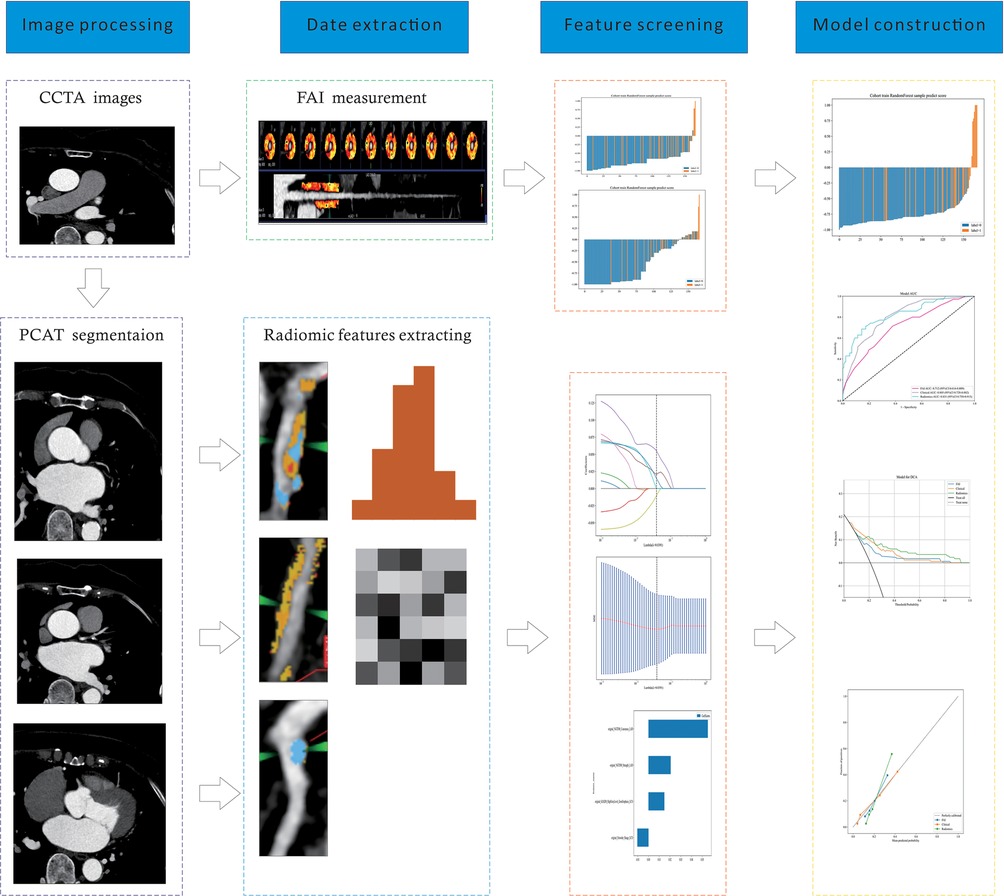
Figure 2. A flow chart of prediction model development process. CCTA, coronary computed tomography angiography; PCAT, pericoronary adipose tissue; FAI, fat attenuation index.
3.3 Efficacy evaluation
ROC curves for the three models were plotted in both the training and validation sets to illustrate their predictive performances (see Figure 3). The radiomics model showed superior efficacy with AUCs of 0.83 [95% CI: 0.750–0.913] and 0.71 [95% CI: 0.539–0.871], compared to the clinical feature model (AUC = 0.81 [95%CI: 0.728–0.882], AUC = 0.67 [95%CI: 0.526–0.812]) and the FAI model (AUC = 0.71 [95%CI: 0.614–0.809], AUC = 0.54 [95%CI: 0.348–0.733]). The predictive abilities of the three models were quantified by measuring accuracy, specificity, sensitivity, positive predictive value, and negative predictive value (Table 3). Decision curves demonstrated the clinical utility of the three predictive models by comparing net benefits at different threshold probabilities in the dataset, with results indicating that the net benefit of predicting MACE in angina pectoris was greater with the radiomics model than with the other models (see Figure 4). Calibration curves for the three predictive models showed good agreement between predicted and observed probabilities of adverse events in angina pectoris (see Figure 4).
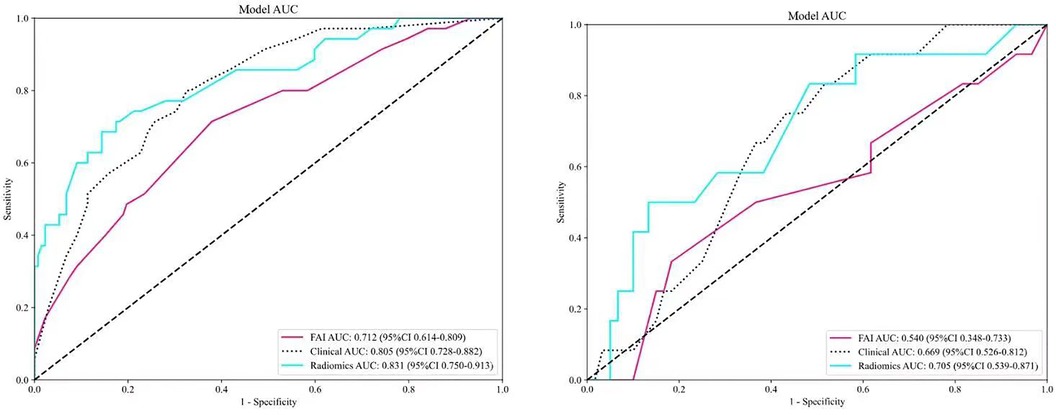
Figure 3. Comparison of receiver operating characteristic (ROC) curves for the FAI model (red lines), clinical model (blue dotted lines) and radiomics model (blue solid lines).
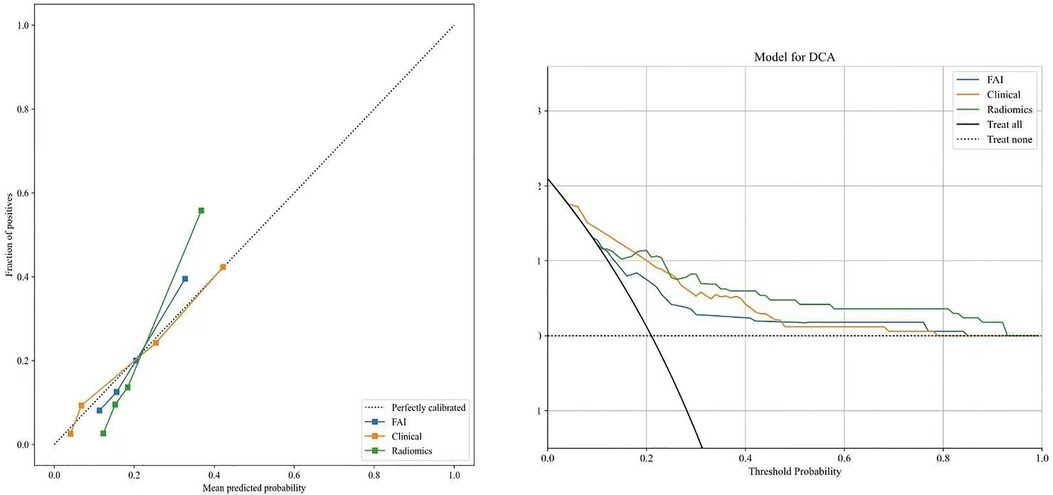
Figure 4. Decision curve analysis and calibration curves of the FAI model (blue line), clinical model(orange line) and radiomics model(green line).
4 Discussion
With the deepening research on coronary artery disease, a variety of effective consensus treatment methods have emerged. Conservative treatment remains a baseline strategy aimed at symptom management, preventing the progression of disease, and averting adverse events, particularly myocardial infarction. Invasive treatments such as percutaneous coronary intervention (PCI) or coronary artery bypass grafting (CABG) can complement conservative measures (1, 23). In our study, patients with angina were initially managed with conservative treatments, including anti-anginal therapy, aimed at symptom relief and prevention of disease progression. Modifications to anti-anginal therapy were made on a case-by-case basis during the follow-up period, depending on changes in symptomatology, although detailed tracking of therapy modifications was not a primary focus of this study. Invasive treatments, such as PCI or CABG, were performed for a subset of patients based on baseline CCTA findings. These procedures were undertaken when significant coronary stenosis or high-risk plaque features were identified, necessitating further intervention to mitigate the risk of MACE. During the follow-up period, 15% of patients underwent PCI and 5% underwent CABG. The general discussion of treatment strategies in this manuscript is based on established literature on coronary artery disease management. Our study reflects real-world clinical decision-making, where a combination of conservative and invasive strategies was employed depending on the individual patient's risk profile and clinical presentation. However, numerous patients still suffer serious cardiovascular adverse events, and the specific risk factors and likelihood of occurrence are not entirely clear. Therefore, researchers are constructing predictive models by exploring the factors related to major cardiovascular adverse events in ST-elevation myocardial infarction to further assess risks and guide treatment (24). An increasing number of patients presenting with angina pectoris for consultation and treatment also frequently experience severe and irreversible cerebrovascular accidents during the progression of their disease (2, 3). However, current research into the major adverse cardiovascular events associated with angina pectoris is not in-depth, and early identification of high-risk factors for MACE events in patients with angina pectoris, constructing evaluation systems suitable for specific populations, and managing individuals are crucial. Consequently, this study attempts to optimize individualized risk prediction through the establishment of a radiomics model, offering a novel spatial perspective. Clinically, CCTA serves as a frontline diagnostic tool for assessing coronary artery disease patients and is an excellent means to determine arterial plaque burden and disease progression. PCAT is directly adjacent to the coronary vessels and interacts with the formation and progression of atherosclerotic plaques in a bidirectional manner. PCAT can sensitively respond to vascular inflammation, causing changes in the size, shape, and distribution of adipocytes in pericoronary adipose tissue (13), and FAI can quantify these PCAT changes. Therefore, observers use CCTA to quantitatively assess coronary lumen stenosis, coronary plaques, and high-risk plaque characteristics, while also qualitatively evaluating low-attenuation plaques, napkin-ring signs, and spotty calcifications (20). CCTA's recognition of features such as high-risk plaques is applicable to plaques forming later in the disease process, while early inflammation, plaque structure, and luminal microenvironment analysis require the introduction of plaque radiomic characterization for assessment (13, 25). Thus, the FAI model in this study indeed exhibited a certain gap in predictive capability compared to the emerging radiomics model. Age is recognized as a primary risk factor for the development of atherosclerotic cardiovascular diseases and coronary heart disease. Studies have shown that age correlates more strongly with the risk of cardiovascular disease events in men than any other factor, and in women, it is second only to hypertension (26, 27). To further analyze the factor of age, scholars have found that patients under the age of 65 have a higher incidence of chest pain, while those over 65 experience a reduction in chest pain (28). However, mortality rates also increase with age. This leads patients to often overlook the high risk associated with age due to the alleviation of chest pain symptoms. When investigating the underlying reasons, the telomere hypothesis has been proposed. Telomere shortening is considered a marker of the aging process and can lead to arterial atherosclerosis, thereby causing cardiovascular disease (29). As age increases, telomeres become progressively shorter, but it appears that patients with coronary heart disease who have a more balanced nutritional diet may have elongated telomeres (29). Through the analysis and selection of clinical factor correlations, this study also confirmed that age has the closest relationship with the occurrence of adverse cardiovascular events in patients with angina pectoris, and the clinical model established as such also exhibited good predictive capacity. Radiomics is a rapidly developing new technology that extracts and analyzes imaging features by segmenting images of the ROI, considering the complex spatial relationships between voxels (12, 13). It can also reflect more persistent changes in the perivascular space caused by vascular inflammation, such as fibrosis and neovascularization (15). In this study, images from the region of interest in coronary CT were segmented and extracted. After analyzing and filtering the PCAT from the proximal parts of the three main coronary arteries, four most robust radiomic features were obtained. These features were used to construct the radiomics model, demonstrating its good efficacy in predicting major adverse cardiovascular events in patients with angina pectoris. Although we did not directly link radiomic features with histopathological analysis, precluding determination of their specific correlation with histopathological changes, radiomic features are big data parameters derived from pixel distribution, serving as an interpretation of the structure underlying the images. They are also an important supplement to data providing information about the imaging phenotype, potentially including a wealth of information (30, 31).
Our study also has some limitations. On one hand, the sample size included in the study is relatively limited, with a correspondingly low incidence rate of events. The presence of a low prevalence binary predictive variable (MACEs negative or positive) may lead to issues of complete or quasi-complete separation in logistic regression (32). Therefore, larger populations and external validations are needed for future studies. On the other hand, all patients’ imaging pictures were taken from the same CT scanner and settings. Since image acquisition, reconstruction, and analysis can affect the reproducibility of imaging features, no studies have explored how these settings may affect radiomic parameters, and our model will need to be validated in different CT scanners in the future (33–35). Renal function, as measured by eGFR, serum creatinine, and BUN, was found to differ between the MACE and non-MACE groups. Patients in the MACE group exhibited lower eGFR and higher serum creatinine and BUN levels, consistent with the known association between impaired renal function and increased cardiovascular risk. However, despite these differences, the inclusion of renal function parameters in our predictive model did not significantly enhance its performance in predicting MACE. This may be due to the relatively preserved renal function in the majority of our cohort, or the small sample size. Nevertheless, renal impairment remains an important risk factor for cardiovascular events, and future studies with larger populations may explore this relationship in greater detail.
5 Conclusion
In summary, the PCAT radiomics model based on coronary CT can effectively predict the occurrence of major adverse cardiovascular events in patients with angina pectoris. This user-friendly tool can help clinicians optimize risk stratification and management of individual patients.
Data availability statement
The original contributions presented in the study are included in the article/Supplementary Material, further inquiries can be directed to the corresponding author.
Ethics statement
The studies involving humans were approved by the Medical Ethics Committee of the Affiliated Hospital of North Sichuan Medical College (approval number: 2023ER218-1). The studies were conducted in accordance with the local legislation and institutional requirements. The participants provided their written informed consent to participate in this study.
Author contributions
WZ: Writing – original draft. YL: Writing – original draft. HL: Writing – original draft. ZZ: Writing – original draft. NY: Writing – original draft. YL: Writing – original draft. XF: Writing – original draft. YY: Writing – review & editing.
Funding
The author(s) declare financial support was received for the research, authorship, and/or publication of this article. The National Natural Science Youth Foundation project (81700417).
Conflict of interest
The authors declare that the research was conducted in the absence of any commercial or financial relationships that could be construed as a potential conflict of interest.
Publisher's note
All claims expressed in this article are solely those of the authors and do not necessarily represent those of their affiliated organizations, or those of the publisher, the editors and the reviewers. Any product that may be evaluated in this article, or claim that may be made by its manufacturer, is not guaranteed or endorsed by the publisher.
References
1. Timmis A, Vardas P, Townsend N, Torbica A, Katus H, De Smedt D, et al. European society of cardiology: cardiovascular disease statistics 2021. Eur Heart J. (2022) 43(8):716–99. doi: 10.1093/eurheartj/ehab892
2. Tsao CW, Aday AW, Almarzooq ZI, Anderson CAM, Arora P, Avery CL, et al. Heart disease and stroke statistics—2023 update: a report from the American Heart Association. Circulation. (2023) 147(8):e93–e621. doi: 10.1161/cir.0000000000001123
3. Kloner RA, Chaitman B. Angina and its management. J Cardiovasc Pharmacol Ther. (2017) 22(3):199–209. doi: 10.1177/1074248416679733
4. Ford TJ, Berry C. Angina: contemporary diagnosis and management. Heart. (2020) 106(5):387–98. doi: 10.1136/heartjnl-2018-314661
5. Padala SK, Lavelle MP, Sidhu MS, Cabral KP, Morrone D, Boden WE, et al. Antianginal therapy for stable ischemic heart disease: a contemporary review. J Cardiovasc Pharmacol Ther. (2017) 22(6):499–510. doi: 10.1177/1074248417698224
6. Ford TJ, Rocchiccioli P, Good R, McEntegart M, Eteiba H, Watkins S, et al. Systemic microvascular dysfunction in microvascular and vasospastic angina. Eur Heart J. (2018) 39(46):4086–97. doi: 10.1093/eurheartj/ehy529
7. Montalescot G, Sechtem U, Achenbach S, Andreotti F, Arden C, Budaj A, et al. 2013 ESC guidelines on the management of stable coronary artery disease: the task force on the management of stable coronary artery disease of the European Society of Cardiology. Eur Heart J. (2013) 34(38):2949–3003. doi: 10.1093/eurheartj/eht296
8. Shaya GE, Leucker TM, Jones SR, Martin SS, Toth PP. Coronary heart disease risk: low-density lipoprotein and beyond. Trends Cardiovasc Med. (2022) 32(4):181–94. doi: 10.1016/j.tcm.2021.04.002
9. Douglas PS, Hoffmann U, Patel MR, Mark DB, Al-Khalidi HR, Cavanaugh B, et al. Outcomes of anatomical versus functional testing for coronary artery disease. N Engl J Med. (2015) 372(14):1291–300. doi: 10.1056/NEJMoa1415516
10. SCOT-HEART investigators. CT coronary angiography in patients with suspected angina due to coronary heart disease (SCOT-HEART): an open-label, parallel-group, multicentre trial. Lancet. (2015) 385(9985):2383–91. doi: 10.1016/S0140-6736(15)60291-4
11. Libby P, Loscalzo J, Ridker PM, Farkouh ME, Hsue PY, Fuster V, et al. Inflammation, immunity, and infection in atherothrombosis. J Am Coll Cardiol. (2018) 72(17):2071–81. doi: 10.1016/j.jacc.2018.08.1043
12. Oikonomou EK, Siddique M, Antoniades C. Artificial intelligence in medical imaging: a radiomic guide to precision phenotyping of cardiovascular disease. Cardiovasc Res. (2020) 116(13):2040–54. doi: 10.1093/cvr/cvaa021
13. Murgia A, Balestrieri A, Crivelli P, Suri JS, Conti M, Cademartiri F, et al. Cardiac computed tomography radiomics: an emerging tool for the non -invasive assessment of coronary atherosclerosis. Cardiovasc Diagn Ther. (2020) 10(6):2005–17. doi: 10.21037/cdt-20-156
14. Nie P, Yang G, Wang Z, Yan L, Miao W, Hao D, et al. A CT-based radiomics nomogram for differentiation of renal angiomyolipoma without visible fat from homogeneous clear cell renal cell carcinoma. Eur Radiol. (2020) 30(2):1274–84. doi: 10.1007/s00330-019-06427-x
15. Oikonomou EK, Antoniades C. The role of adipose tissue in cardiovascular health and disease. Nat Rev Cardiol. (2019) 16(2):83–99. doi: 10.1038/s41569-018-0097-6
16. Wang Y, Liu W, Yu Y, Liu JJ, Xue HD, Qi YF, et al. CT radiomics nomogram for the preoperative prediction of lymph node metastasis in gastric cancer. Eur Radiol. (2020) 30(2):976–86. doi: 10.1007/s00330-019-06398-z
17. Lin A, Kolossváry M, Yuvaraj J, Cadet S, McElhinney PA, Jiang C, et al. Myocardial infarction associates with a distinct pericoronary adipose tissue radiomic phenotype. JACC Cardiovascular Iimaging. (2020) 13(11):2371–83. doi: 10.1016/j.jcmg.2020.06.033
18. Si N, Shi K, Li N, Dong X, Zhu C, Guo Y, et al. Identification of patients with acute myocardial infarction based on coronary CT angiography: the value of pericoronary adipose tissue radiomics. Eur Radiol. (2022) 32(10):6868–77. doi: 10.1007/s00330-022-08812-5
19. Goeller M, Tamarappoo BK, Kwan AC, Cadet S, Commandeur F, Razipour A, et al. Relationship between changes in pericoronary adipose tissue attenuation and coronary plaque burden quantified from coronary computed tomography angiography. Eur Heart J Cardiovasc Imaging. (2019) 20(6):636–43. doi: 10.1093/ehjci/jez013
20. Oikonomou EK, Marwan M, Desai MY, Mancio J, Alashi A, Hutt Centeno E, et al. Non-invasive detection of coronary inflammation using computed tomography and prediction of residual cardiovascular risk (the CRISP CT study): a post-hoc analysis of prospective outcome data. Lancet. (2018) 392(10151):929–39. doi: 10.1016/s0140-6736(18)31114-0
21. Chen L, Shen Y, Huang X, Li H, Li J, Wei R, et al. MRI-based radiomics for differentiating orbital cavernous hemangioma and orbital schwannoma. Front Med (Lausanne). (2021) 8:795038. doi: 10.3389/fmed.2021.795038
22. Kim J, Sandri BJ, Rao RB, Lock EF. Bayesian Predictive modeling of multi-source multi-way data. Comput Stat Data Anal. (2023) 186:107783. doi: 10.1016/j.csda.2023.107783
23. Doenst T, Thiele H, Haasenritter J, Wahlers T, Massberg S, Haverich A. The treatment of coronary artery disease—current status six decades after the first bypass operation. Dtsch Arztebl Int. (2022) 119(42):716–23. doi: 10.3238/arztebl.m2022.0277
24. Ma Q, Ma Y, Wang X, Li S, Yu T, Duan W, et al. A radiomic nomogram for prediction of major adverse cardiac events in ST-segment elevation myocardial infarction. Eur Radiol. (2021) 31(2):1140–50. doi: 10.1007/s00330-020-07176-y
25. Nakanishi R, Motoyama S, Leipsic J, Budoff MJ. How accurate is atherosclerosis imaging by coronary computed tomography angiography? J Cardiovasc Comput Tomogr. (2019) 13(5):254–60. doi: 10.1016/j.jcct.2019.06.005
26. Duggan JP, Peters AS, Trachiotis GD, Antevil JL. Epidemiology of coronary artery disease. Surg Clin North Am. (2022) 102(3):499–516. doi: 10.1016/j.suc.2022.01.007
27. Nakazato R, Arsanjani R, Achenbach S, Gransar H, Cheng VY, Dunning A, et al. Age-related risk of major adverse cardiac event risk and coronary artery disease extent and severity by coronary CT angiography: results from 15 187 patients from the international multisite CONFIRM study. Eur Heart J Cardiovasc Imaging. (2014) 15(5):586–94. doi: 10.1093/ehjci/jet132
28. Canto JG, Rogers WJ, Goldberg RJ, Peterson ED, Wenger NK, Vaccarino V, et al. Association of age and sex with myocardial infarction symptom presentation and in-hospital mortality. JAMA. (2012) 307(8):813–22. doi: 10.1001/jama.2012.199
29. Kalstad AA, Tveit S, Myhre PL, Laake K, Opstad TB, Tveit A, et al. Leukocyte telomere length and serum polyunsaturated fatty acids, dietary habits, cardiovascular risk factors and features of myocardial infarction in elderly patients. BMC Geriatr. (2019) 19(1):376. doi: 10.1186/s12877-019-1383-9
30. Aerts HJ. The potential of radiomic-based phenotyping in precision medicine. JAMA Oncol. (2016) 2(12):1636–42. doi: 10.1001/jamaoncol.2016.2631
31. Castellano G, Bonilha L, Li LM, Cendes F. Texture analysis of medical images. Clin Radiol. (2004) 59(12):1061–9. doi: 10.1016/j.crad.2004.07.008
32. Ogundimu EO, Altman DG, Collins GS. Adequate sample size for developing prediction models is not simply related to events per variable. J Clin Epidemiol. (2016) 76:175–82. doi: 10.1016/j.jclinepi.2016.02.031
33. Kolossváry M, Karády J, Szilveszter B, Kitslaar P, Hoffmann U, Merkely B, et al. Radiomic features are superior to conventional quantitative computed tomographic metrics to identify coronary plaques with napkin-ring sign. Circ Cardiovasc Iimaging. (2017) 10(12):e006843. doi: 10.1161/CIRCIMAGING.117.006843
34. Serruys PW, Kotoku N, Nørgaard BL, Garg S, Nieman K, Dweck MR, et al. Computed tomographic angiography in coronary artery disease. EuroIntervention. (2023) 18(16):e1307–27. doi: 10.4244/EIJ-D-22-00776
Keywords: coronary computed tomography angiography, major adverse cardiovascular event, pericoronary adipose tissue, angina pectoris, radiomics
Citation: Zhan W, Luo Y, Luo H, Zhou Z, Yin N, Li Y, Feng X and Yang Y (2024) Predicting major adverse cardiovascular events in angina patients using radiomic features of pericoronary adipose tissue based on CCTA. Front. Cardiovasc. Med. 11:1462451. doi: 10.3389/fcvm.2024.1462451
Received: 17 July 2024; Accepted: 23 September 2024;
Published: 31 October 2024.
Edited by:
Massimiliano Ruscica, University of Milan, ItalyReviewed by:
Gabriele Tumminello, IRCCS Ca 'Granda Foundation Maggiore Policlinico Hospital, ItalyGloria Santangelo, IRCCS Ca 'Granda Foundation Maggiore Policlinico Hospital, Italy
Copyright: © 2024 Zhan, Luo, Luo, Zhou, Yin, Li, Feng and Yang. This is an open-access article distributed under the terms of the Creative Commons Attribution License (CC BY). The use, distribution or reproduction in other forums is permitted, provided the original author(s) and the copyright owner(s) are credited and that the original publication in this journal is cited, in accordance with accepted academic practice. No use, distribution or reproduction is permitted which does not comply with these terms.
*Correspondence: Ying Yang, MTg1ODI1NDcxNzVAMTYzLmNvbQ==