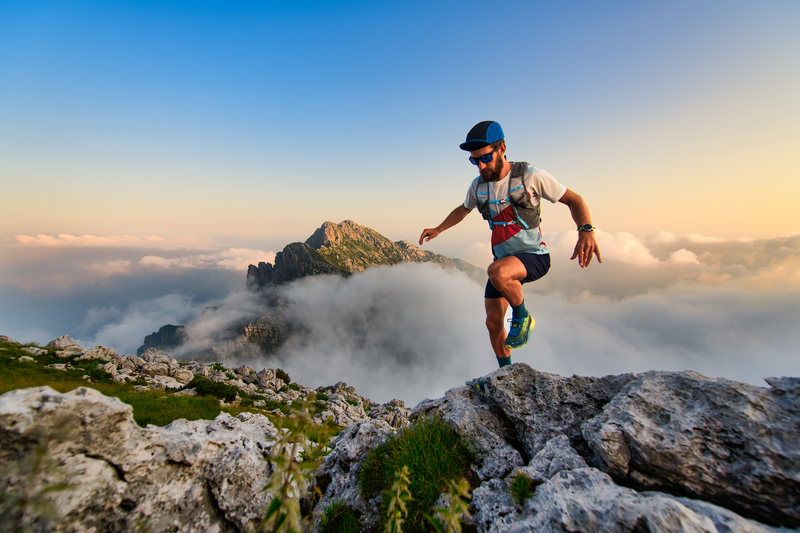
94% of researchers rate our articles as excellent or good
Learn more about the work of our research integrity team to safeguard the quality of each article we publish.
Find out more
ORIGINAL RESEARCH article
Front. Cardiovasc. Med. , 27 June 2023
Sec. Coronary Artery Disease
Volume 10 - 2023 | https://doi.org/10.3389/fcvm.2023.1140215
Objective: Dyslipidemia is a key risk factor for coronary artery disease (CAD). This study aimed to investigate the correlation between the atherogenic index of plasma (AIP) and the severity of CAD.
Methods: 2,491 patients were enrolled in this study and analyzed retrospectively, including 665 non-CAD patients as the control group and 1,826 CAD patients. The CAD patients were classified into three subgroups according to tertiles of SYNTAX score (SS). Non-high-density lipoprotein cholesterol (Non-HDL-C) was defined as serum total cholesterol (TC) minus serum high-density lipoprotein cholesterol (Non-HDL-C), atherogenic index (AI) was defined as the ratio of non-HDL-C to HDL-C; AIP was defined as the logarithm of the ratio of the concentration of triglyceride (TG) to HDL-C; lipoprotein combine index (LCI) was defined as the ratio of TC∗TG∗ low-density lipoprotein cholesterol (LDL)to HDL-C; Castelli Risk Index I (CRI I) was defined as the ratio of TC to HDL-C; Castelli Risk Index II (CRI II) was defined as the ratio of LDL-C to HDL-C.
Results: The levels of AIP (P < 0.001), AI (P < 0.001), and LCI (P = 0.013) were higher in the CAD group compared with the non-CAD group. The Spearman correlation analysis showed that AIP (r = 0.075, P < 0.001), AI (r = 0.132, P < 0.001), and LCI (r = 0.072, P = 0.001) were positively correlated with SS. The multivariate logistic regression model showed CRI I (OR: 1.11, 95% CI: 1.03–1.19, P = 0.005), CRI II (OR: 1.26, 95% CI: 1.15–1.39, P < 0.001), AI (OR: 1.28, 95% CI: 1.17–1.40, P < 0.001), AIP (OR: 2.06, 95% CI: 1.38–3.07, P < 0.001), and LCI (OR: 1.01, 95% CI: 1.01–1.02, P < 0.001) were independent predictors of severity of CAD After adjusting various confounders.
Conclusion: CRI I, CRI II, AIP, AI, and LCI were independent predictors of the severity of CAD, which could be used as a biomarker for the evaluation of the severity of CAD.
Coronary artery disease (CAD) is the most prevalent cardiovascular disorder, imposing a formidable risk to individuals in both developed and developing nations. It has been identified as a foremost cause of mortality worldwide, exerting a substantial burden on global public health (1). Coronary artery disease (CAD) is primarily an atherosclerotic condition influenced by various factors, including inflammation, endothelial dysfunction, and oxidative stress. The risk factors associated with CAD development are well-established. Dyslipidemia, particularly elevated levels of total cholesterol (TC), triglycerides (TG), low-density lipoprotein cholesterol (LDL-C), and decreased high-density lipoprotein cholesterol (HDL-C), has been extensively studied and strongly linked to the onset and progression of CAD. In clinical practice, LDL-C reduction has traditionally been the main focus for lipid-lowering therapy. However, residual cardiovascular risk persists even when LDL-C levels reached recommended targets (2). Therefore, we hypothesized that employing a combination of lipid indices may offer a more comprehensive assessment of lipid status as opposed to relying on a single lipid index.
Recently, composite lipid indices, including the atherogenic index of plasma (AIP, the logarithm of the ratio of TG to HDL-C levels), atherogenic index (AI, non-HDL-C/HDL-C), and lipoprotein combine index (LCI, TC∗TG∗LDL/HDL-C) have emerged as potential predictors of CAD risk, surpassing the predictive value of traditional single lipid parameters (3). AIP has been observed to exhibit a strong association and inverse correlation with the diameter of LDL-C particles, serving as an indirect indicator of small, low-density lipoprotein (sdLDL) levels (4). Thus, AIP may be a predictor of CAD. Studies have indicated a significant association between AIP and obesity (5), highlighting AIP as a novel lipid marker for individuals with Type 2 diabetes and CAD (3). Further, AIP is a strong predictor of CAD in post-menopausal women (6). The SYNTAX score (SS) is used to evaluate the degree of CAD and the severity of coronary stenosis (7). It is an anatomical scoring system based on coronary angiography. In a recent study, AIP emerged not only as a predictive factor for CAD but also exhibited a positive correlation with a high SYNTAX score, indicating its potential as a marker for evaluating CAD severity (8). However, there is limited research available on the correlation between AIP and SS, and the relationship has not been well established. Therefore, the primary objective of this study was to explore the association between AIP and both the incidence and severity of CAD. Additionally, the study aimed to explore the association of AIP with both the incidence and severity of CAD.
A total of 2,491 patients who underwent coronary angiography were enrolled in our study and were admitted into the Department of Cardiology, Zhongnan Hospital, Wuhan University from January 2014 to December 2019. CAD was defined as ≥50% stenosis of at least one epicardial major coronary artery (i.e., >2 mm in diameter) assessed by coronary angiography according to 2012 ACC/AHA guidelines (either stable or unstable) (9). There were 665 cases of non-CAD hospitalized patients in the control group and 1,826 cases in the CAD group.
Exclusion criteria: patients with lipid-affecting drug use history for more than one month before admission and incomplete lipid profile data, previous myocardial infarction, malignant tumors, severe liver, and renal dysfunction, Prior coronary stent implantation or coronary artery bypass grafting, thyroid dysfunction, decompensated heart failure or cardiomyopathy. This study was approved by the Medical Ethics Review Committee of Zhongnan Hospital. The informed consent was obtained from all participants.
Coronary angiography was performed by two experienced interventional cardiovascular physicians, and the results of the angiography were evaluated, the disagreements were resolved by the third cardiologist. Coronary angiography via radial or femoral approach according to Judkin’s method. Stenosis of the right coronary artery (RCA), left main coronary artery, anterior descending artery (LAD), and circumflex artery (LCX) was assessed.
Using the SYNTAX Score I to Assess the Severity of CAD. The SS was calculated using the SYNTAX scoring website (https://syntaxscore.org/). CAD patients were dissected into three subgroups according to the tertiles of their SYNTAX score I: mild group (0 ≤ SS < 6.5), moderate group (6.5 ≤ SS ≤ 14), and severe group (>14).
After fasting for more than 10 h, blood samples were taken from the antecubital vein in the early morning. The serum was collected after the blood has spontaneously coagulated and been centrifuged. Serum lipids and high sensitive C-reactive protein (hs-CRP) were measured with an automatic biochemical analyzer (Beckman Coulter, AU5800, USA). Except for dsLDL and hs-CRP, the detection kits, calibrators and quality control products of other plasma lipids are all AU5800 supporting reagents. HDL-C and LDL-C were measured by the homogeneous method (direct method). TC and TG were measured by the enzymatic method. Lp(a) and hs-CRP was measured by the immunoturbidimetric assay (ITA) (the hs-CRP detection kits were from DiaSys Inc., Shanghai, China). sdLDL was mainly measured by the peroxidase method (the detection kits were from Zybio Inc., Chongqing, China). Non-HDL-C is calculated using the following formula:
AI, AIP, LCI, remnant lipoprotein cholesterol (RLP-C), Castelli risk index-I (CRI-I), and Castelli risk index-II (CRI-II) were expressed in mmol/L calculated by the following formulas:
According to the 2016 Chinese guidelines for the management of dyslipidemia in adults (10), plasma lipids were grouped according to the following criteria: (1) TC: normal: <5.2 mmol/L, marginal high: 5.2–6.2 mmol/L, and high: ≥6.2 mmol/L; (2) TG: normal: <1.7 mmol/L, marginal high: 1.7–2.3 mmol/L, and high: ≥2.3 mmol/L; (3) HDL-C: normal: ≥1.0 mmol/L, low: <1.0 mmol/L; (4) LDL-C: normal: <3.4 mmol/L, marginal high: 3.4–4.1 mmol/L, and high: ≥4.1 mmol/L; (5) Lp(a): normal: <140 mmol/L, and high: ≥140 mmol/L; (6) non-HDL-C: normal: <4.1 mmol/L, and high: ≥4.1 mmol/L; (7) ApoA-1: normal: <1.6 g/L, and high: ≥1.6 g/L; (8) ApoB: normal: <1.3 g/L, and high: ≥1.3 g/L.
Statistical analyses were carried out with statistical package for the social sciences version 23.0 software program (IBM Corp, Armonk, New York, USA). Continuous variables were given as mean ± SD (if normal distribution) and were compared using Student’s t-test. However, if variables were not normal distribution, expressed as medians (interquartile ranges). The Mann–Whitney U-test was used for comparison between two groups, and the Kruskal-Wallis test was used for comparison between multiple groups. The categorical variables were shown as percentages. The χ2-test was used to compare mean values of the categorical variables between the groups. The Kolmogorov–Smirnov test was used to assess whether the variables were normally distributed. The correlation of lipid indices with the CAD severity (mild, moderate, severe) was calculated with Spearman’s coefficient analysis. The critical correlation coefficient was calculated through calculator websites (https://mathcracker.com/critical-correlation-calculator). The univariate (i.e., unadjusted) and binary multivariate logistic regressions (i.e., adjusted) were used to estimate the associations of plasm lipids, CRI I, CRI II, AI, AIP and LCI with the incidence of CAD (i.e., the occurrence of CAD was used as the dependent variable for univariate and binary multivariate logistic regression analysis). This analysis was performed in the CAD group and the non-CAD group. The univariate and multivariate logistic regressions were used to estimate the associations of plasm lipids, CRI I, CRI II, AI, AIP, and LCI with the severity of CAD (mild, moderate, and severe). Taking the mild CAD group (0 ≤ syntax score <6.5) as the reference group. For variables with parallel line test P > 0.05, the multivariate ordinal logistic regression is used, and for variables with parallel line test P < 0.05, the disordered multivariate logistic regression is used. All plasma lipid parameters were included in the univariate logistic regression analysis. We included blood lipids as a categorical variable into logistic regression analysis, which can more clearly show the relationship between blood lipids and CAD and its severity. In multivariate logistic regression analysis, we included potential confounding factors into the analysis, mainly those with a P-value <0.5 in the baseline characteristics (i.e., age, gender, smoking, diabetes, hypertension, creatinine, uric acid, hs-CRP). The results were evaluated within a 95% confidence interval (CI) and at a significance level of two-sided P-value less than 0.05.
Table 1 shows the basic clinical characteristics and biochemical data of CAD and non-CAD patients. The values of CRI I (P < 0.001), CRI II (P < 0.001), AIP (P < 0.001), AI (P < 0.001), and LCI (P = 0.013) were significantly higher in CAD group than non-CAD group. Patients in the CAD group had higher age, Cr, UA, FBG, and hs-CRP, higher percentage of male patients, smoking, diabetes, and hypertension compared with the non-CAD group. TC, Lp(a), and TG/HDL were higher in CAD patients, while non-CAD patients had lower HDL, ApoA1, and ApoA1/ApoB levels.
Table 2 shows the basic clinical characteristics and biochemical data of patients with mild, moderate and severe CAD. The AIP, AI, and LCI levels were compared among these three subgroups. The results demonstrated that CRI I (P < 0.001), CRI II (P < 0.001), AIP (P < 0.001), AI (P < 0.001), and LCI (P = 0.007) levels were higher in the moderate and severe group compared with the mild group,. Patients in the severe CAD group were older and had higher rates of diabetes, as well as increased levels of serum CR, UA, TC, LDL-C, ApoB, non-HDL-C, CRI I, CRI II, TG/HDL-C, AI, AIP, LCI was higher than mild CAD group.
In univariate logistic regression analysis, ApoA-1, Lp(a), ApoA-1/ApoB, CRI-I, CRI-II, TG/HDL-C, AI, AIP, and LCI were positively correlated with the risk of CAD (all P < 0.05), and HDL-C was negatively correlated with with the risk of CAD (P < 0.001). After adjusting potential confounding factors (age, gender, smoking, diabetes, hypertension, creatinine, uric acid, hs-CRP), except for TG/HDL-C (P = 0.138), other lipid parameters, AI (OR: 1.27, 95% CI:1.15–1.40, P < 0.001), AIP (OR: 2.78, 95% CI: 1.82–4.26, P < 0.001), LCI (OR: 1.01, 95% CI: 1.01–1.02, P < 0.001), CRI-I (OR: 1.10, 95% CI: 1.01–1.20, P = 0.037), and CRI II (OR: 1.24, 95% CI: 1.11–1.40, P < 0.001) were still positively correlated with the risk of CAD (all P < 0.05), and HDL-C was still negatively correlated with the risk of CAD (P < 0.001) (Table 3).
Spearman correlation analysis was performed on AIP, AI, LCI with CAD severity subgroups (mild, moderate, severe). The critical correlation coefficient calculated through calculator websites is 0.046 (n = 1,826, two-tailed α = 0.05). The results demonstrated that AIP (r = 0.075, P < 0.001), AI (r = 0.132, P < 0.001), LCI (r = 0.072, P = 0.001), CRI I (r = 0.132, P < 0.001), and CRI II (r = 0.128, P < 0.001) were positively correlated with SS subgroups.
In univariate logistic regression analysis, ApoA-1, Lp(a), RLP-C, Non-HDL-C, ApoA-1/ApoB, CRI-I, CRI-II, TG/HDL-C, RLP-C, AI, AIP, and LCI were positively correlated with the severity of CAD (all P < 0.05), and HDL-C was negatively correlated with the risk of CAD (P < 0.001). After adjusting for potential confounding factors (age, gender, smoking, diabetes, hypertension, creatinine, uric acid, hs-CRP), TC marginal high (OR: 1.68, 95% CI: 1.05–2.69, P = 0.031) or high (OR: 1.92, 95% CI: 1.25–2.95, P = 0.003) was positively correlated with CAD severity. Compared with patients with normal HDL-C, patients with subnormal HDL-C had an increased risk of severe CAD lesions (OR: 1.72, 95% CI: 1.33–2.23, P < 0.001). Meanwhile, Lp(a), RLP-C, ApoA1/ApoB, CRI-I (OR: 1.11, 95% CI: 1.03–1.19, P = 0.005), CRI II (OR: 1.26, 95% CI: 1.15–1.39, P < 0.001), AI (OR: 1.28, 95% CI: 1.17–1.40, P < 0.001), AIP (OR: 2.06, 95% CI: 1.38–3.07, P < 0.001), and LCI (OR: 1.01, 95% CI: 1.01–1.02, P < 0.001) were still positively correlated with the increased risk CAD severity (Table 4).
The present study demonstrated that: (1) The AIP, AI, and LCI in CAD patients were considerably greater than that in non-CAD patients; (2) The AIP, AI, and LCI were positively correlated with the severity of CAD and served as independent risk predictors of the incidence and severity of CAD. These results indicated that AIP, AI, and LCI could be used as biomarkers for the evaluation of severity in CAD patients.
Dyslipidemia is a traditional cardiovascular risk factor, it is the key driver for CAD occurrence and progression (11). A previous study has demonstrated that lipid metabolism disorder is characterized by low LDL-C and high TG for most Chinese patients (12). High TG and low HDL levels are and common lipid phenotype that is strongly correlated with cardiovascular disease. It has been well documented that triglyceride-rich lipoproteins (TRL) are involved in atherogenesis by many mechanisms. A previous randomized controlled trial (RCT) demonstrated that elevated RLP-C and TRL are causally associated with low-grade inflammation, whereas elevated LDL-C is not (13). This may be due to the fact that TRL and its remnants can increase the level of cellular adhesion molecules in plasma, thereby promoting the adhesion of leukocytes and monocytes to atherosclerotic lesions, which may trigger an inflammatory response (14, 15). In addition, TRL accumulation in the plasma causes hyperviscosity through fibrinolysis inhibition, and a procoagulant state by amplifying the coagulation cascade through upregulated platelet aggregation, thrombus formation, and enhanced expression of the plasminogen activating inhibitor-1 (16). HDL facilitates cholesterol efflux from lipid-rich macrophages within atherosclerotic plaques in the arterial walls. This efflux is mediated by the interactions of apolipoprotein A-I (apoA-I) with adenosine triphosphate-binding cassette transporter A1 (ABCA1). The cholesterol is then transported to the liver for metabolism or biliary excretion (17). Furthermore, HDL has anti-inflammatory and anti-oxidative effects by regulating endothelial homeostasis and anti-thrombus through attenuation of platelets aggregation and adhesion responses (18).Based on the mechanisms and functions of triglycerides (TG) and high-density lipoprotein cholesterol (HDL-C) in atherosclerosis, the atherogenic index of plasma (AIP), which is the logarithmic transformation of TG/HDL, has been found to be associated with the incidence of CVD. Additionally, AIP has been negatively correlated with LDL particle diameter and can serve as a surrogate marker for small dense LDL (sdLDL) particles, which are also associated with CVD (19). Therefore, it is more reasonable to use AIP to predict CAD risk.
Growing evidence has shown that AIP was strongly associated with numerous cardiovascular risk factors, such as BMI, visceral fat, TG, and glucose (20, 21). AIP was closely related to obesity, hypertension, DM, insulin resistance, and metabolic syndrome (5, 22). Furthermore, it has been suggested that AIP was a reliable indicator in the prediction of atherosclerosis and cardiovascular complications. Cai et al. observed a significant decrease in the atherogenic index of plasma (AIP) in the control group compared to the CAD group (23). Moreover, an observational study showed a strong correlation between the AIP and the severity of CAD (24). In another study, AIP was significantly related to the progression of coronary artery calcification in Korean adults without CVD, the incidence of coronary artery calcification progression increased gradually with rising AIP through 4.2 year-follow-up (25). Furthermore, a study has demonstrated that an increased AIP may predict the complexity of percutaneous coronary intervention in chronic total occlusion patients and additionally may help to improve the therapeutic intervention of chronic total occlusion (26). A previous study, which included 1,347 non-CAD and 2,253 CAD patients, suggested that AIP and SYNTAX scores were positively correlated and both could be used to predict the severity of CAD (8).
In the current study, AIP was elevated in CAD patients compared with non-CAD patients, and AIP was positively correlated with SS, which indicated a positive correlation between AIP and the severity of CAD. The results were consistent with those of previous studies (8). In addition, AI and LCI were found to be significantly higher in CAD than in non-CAD patients in our study, suggesting that these two lipid indices may also be positively associated with CAD severity. AI represents the ratio of all the atherogenic particles (i.e., non-HDL-C) and all the anti-atherosclerosis particles (e.g., HDL-C); this index has been shown to relate broadly to diseases associated with dyslipidemia, including metabolic syndrome, insulin resistance, and CAD (27, 28). LCI is a non-traditional lipid profile and could be used as a risk factor for acute coronary syndrome (29), Oguntola et al. found that LCI correlated with atherosclerotic vascular disease (30). The current study demonstrated a positive correlation between the severity of CAD and three lipid indices. After adjusting various confounders, AI, AIP, and LCI were still independent risk factors for CAD and SS. Moreover, as AI, AIP, and LCI increase, there is a corresponding worsening of coronary artery stenosis. In this study, the utilization of three composite lipid indices aimed to enhance the prediction of both CAD risk and CAD severity, thereby providing a more comprehensive and accurate assessment. On the other hand, this method will be more feasible and more economical in clinical practice.
This study has several important limitations. First, we did not establish the optimal diagnostic threshold values for these three indices. Further investigation in clinical practice is warranted to determine these threshold values. Second, it is important to acknowledge that this study was conducted in a single center and relied on retrospective data. Therefore, the potential influence of selection bias cannot be completely ruled out, and the results may not fully generalize to the broader population. Lastly, it is worth noting that the SS was determined based on the results of coronary angiography, and objective factors such as the spatial resolution of the coronary angiography system and the size of the catheter used could potentially impact the findings of this study.
The CRI I, CRI II, AI, AIP, and LCI in the CAD group were significantly elevated compared to the non-CAD group, the CRI I, CRI II, AI, AIP, and LCI positively correlated with the CAD severity, which may be used as a noninvasive biomarker for the evaluation of the complexity of CAD in clinical practice. In addition, CRI I, CRI II, AI, AIP, and LCI could serve as independent predictive factors for CAD and CAD severity.
The raw data supporting the conclusions of this article will be made available by the authors, without undue reservation.
The studies involving human participants were reviewed and approved by Medical Ethics Review Committee of Zhongnan hospital. The patients/participants provided their written informed consent to participate in this study.
YL and YF designed the study and drafted the manuscript. YL, YF, SL, MY, and JL collected the data and helped implement and analyze the project. MZ and JW interpreted and reviewed the results, revised the manuscript, and confirmed the final published version. All of the authors read and approved the final manuscript. All authors contributed to the article and approved the submitted version.
This study was supported by the Translational Medicine and Interdisciplinary Research Joint Fund of Zhongnan Hospital of Wuhan University (grant number ZNJC202201).
Thanks to all the members of the research group and friends who have helped us in this process.
The authors declare that the research was conducted in the absence of any commercial or financial relationships that could be construed as a potential conflict of interest.
All claims expressed in this article are solely those of the authors and do not necessarily represent those of their affiliated organizations, or those of the publisher, the editors and the reviewers. Any product that may be evaluated in this article, or claim that may be made by its manufacturer, is not guaranteed or endorsed by the publisher.
1. GBD 2013 Mortality and Causes of Death Collaborators. Global, regional, and national age-sex specific all-cause and cause-specific mortality for 240 causes of death, 1990–2013: a systematic analysis for the global burden of disease study 2013. Lancet. (2015)385(9963):117–71. doi: 10.1016/S0140-6736(14)61682-2
2. Matsuura Y, Kanter JE, Bornfeldt KE. Highlighting residual atherosclerotic cardiovascular disease risk. Arterioscler Thromb Vasc Biol. (2019) 39(1):e1–e9. doi: 10.1161/ATVBAHA.118.311999
3. Zhou K, Qin Z, Tian J, Cui K, Yan Y, Lyu S. The atherogenic index of plasma: a powerful and reliable predictor for coronary artery disease in patients with type 2 diabetes. Angiology. (2021) 72(10):934–41. doi: 10.1177/00033197211012129
4. Dobiasova M, Frohlich J. The plasma parameter log (TG/HDL-C) as an atherogenic index: correlation with lipoprotein particle size and esterification rate in apoB-lipoprotein-depleted plasma [FER(HDL)]. Clin Biochem. (2001) 34(7):583–8. doi: 10.1016/s0009-9120(01)00263-6
5. Zhu X, Yu L, Zhou H, Ma Q, Zhou X, Lei T, et al. Atherogenic index of plasma is a novel and better biomarker associated with obesity: a population-based cross-sectional study in China. Lipids Health Dis. (2018) 17(1):37. doi: 10.1186/s12944-018-0686-8
6. Wu TT, Gao Y, Zheng YY, Ma YT, Xie X. Atherogenic index of plasma (AIP): a novel predictive indicator for the coronary artery disease in postmenopausal women. Lipids Health Dis. (2018) 17(1):197. doi: 10.1186/s12944-018-0828-z
7. Neumann FJ, Sousa-Uva M, Ahlsson A, Alfonso F, Banning AP, Benedetto U, et al. 2018 ESC/EACTS guidelines on myocardial revascularization. Eur Heart J. (2019)40(2):87–165.doi: 10.1093/eurheartj/ehy394
8. Wang L, Chen F, Xiaoqi C, Yujun C, Zijie L. Atherogenic index of plasma is an independent risk factor for coronary artery disease and a higher SYNTAX score. Angiology. (2021) 72(2):181–6. doi: 10.1177/0003319720949804
9. Fihn SD, Gardin JM, Abrams J, Berra K, Blankenship JC, Dallas AP, et al. 2012 ACCF/AHA/ACP/AATS/PCNA/SCAI/STS guideline for the diagnosis and management of patients with stable ischemic heart disease: a report of the American college of cardiology foundation/American heart association task force on practice guidelines, and the American college of physicians, American association for thoracic surgery, preventive cardiovascular nurses association, society for cardiovascular angiography and interventions, and society of thoracic surgeons. Circulation. (2012)126(25):e354–e471.doi: 10.1161/CIR.0b013e318277d6a0
10. 2016 Chinese Guidelines for the management of dyslipidemia in adults. J Geriatr Cardiol. (2018)15(1):1–29.doi: 10.11909/j.issn.1671-5411.2018.01.011
11. Wilson P, Polonsky TS, Miedema MD, Khera A, Kosinski AS, Kuvin JT. Systematic review for the 2018 AHA/ACC/AACVPR/AAPA/ABC/ACPM/ADA/AGS/APhA/ASPC/NLA/PCNA guideline on the management of blood cholesterol: a report of the American college of cardiology/American heart association task force on clinical practice guidelines. J Am Coll Cardiol. (2019) 73(24):3210–27. doi: 10.1016/j.jacc.2018.11.004
12. Huang Y, Gao L, Xie X, Tan SC. Epidemiology of dyslipidemia in Chinese adults: meta-analysis of prevalence, awareness, treatment, and control. Popul Health Metr. (2014) 12(1):28. doi: 10.1186/s12963-014-0028-7
13. Varbo A, Benn M, Tybjaerg-Hansen A, Nordestgaard BG. Elevated remnant cholesterol causes both low-grade inflammation and ischemic heart disease, whereas elevated low-density lipoprotein cholesterol causes ischemic heart disease without inflammation. Circulation. (2013) 128(12):1298–309. doi: 10.1161/CIRCULATIONAHA.113.003008
14. Doi H, Kugiyama K, Oka H, Sugiyama S, Ogata N, Koide SI, et al. Remnant lipoproteins induce proatherothrombogenic molecules in endothelial cells through a redox-sensitive mechanism. Circulation. (2000) 102(6):670–6. doi: 10.1161/01.cir.102.6.670
15. Wang YI, Bettaieb A, Sun C, DeVerse JS, Radecke CE, Mathew S, et al. Triglyceride-rich lipoprotein modulates endothelial vascular cell adhesion molecule (VCAM)-1 expression via differential regulation of endoplasmic reticulum stress. PLoS One. (2013) 8(10):e78322. doi: 10.1371/journal.pone.0078322
16. Castillo-Nunez Y, Morales-Villegas E, Aguilar-Salinas CA. Triglyceride-rich lipoproteins: their role in atherosclerosis. Rev Invest Clin. (2022) 74(2):61–70. doi: 10.24875/RIC.21000416
17. Annema W, von Eckardstein A. High-density lipoproteins. Multifunctional but vulnerable protections from atherosclerosis. Circ J. (2013) 77(10):2432–48. doi: 10.1253/circj.cj-13-1025
18. Wong N, Nicholls SJ, Tan J, Bursill CA. The role of high-density lipoproteins in diabetes and its vascular complications. Int J Mol Sci. (2018) 19(6):1680. doi: 10.3390/ijms19061680
19. Arai H, Kokubo Y, Watanabe M, Sawamura T, Ito Y, Minagawa A, et al. Small dense low-density lipoproteins cholesterol can predict incident cardiovascular disease in an urban Japanese cohort: the Suita study. J Atheroscler Thromb. (2013) 20(2):195–203. doi: 10.5551/jat.14936
20. Bo MS, Cheah WL, Lwin S, Moe NT, Win TT, Aung M. Understanding the relationship between atherogenic index of plasma and cardiovascular disease risk factors among staff of an university in Malaysia. J Nutr Metab. (2018)2018:7027624.doi: 10.1155/2018/7027624
21. Niroumand S, Khajedaluee M, Khadem-Rezaiyan M, Abrishami M, Juya M, Khodaee G, et al. Atherogenic index of plasma (AIP): a marker of cardiovascular disease. Med J Islam Repub Iran. (2015) 29:240. PMID: 26793631.26793631
22. Pourfarzam M, Zadhoush F, Sadeghi M. The difference in correlation between insulin resistance index and chronic inflammation in type 2 diabetes with and without metabolic syndrome. Adv Biomed Res. (2016) 5:153. doi: 10.4103/2277-9175.188489
23. Cai G, Shi G, Xue S, Lu W. The atherogenic index of plasma is a strong and independent predictor for coronary artery disease in the Chinese Han population. Medicine (Baltimore). (2017) 96(37):e8058. doi: 10.1097/MD.0000000000008058
24. Cai G, Liu W, Lv S, Wang X, Guo Y, Yan Z, et al. Gender-specific associations between atherogenic index of plasma and the presence and severity of acute coronary syndrome in very young adults: a hospital-based observational study. Lipids Health Dis. (2019) 18(1):99. doi: 10.1186/s12944-019-1043-2
25. Nam JS, Kim MK, Nam JY, Park K, Kang S, Ahn CW, et al. Association between atherogenic index of plasma and coronary artery calcification progression in Korean adults. Lipids Health Dis. (2020) 19(1):157. doi: 10.1186/s12944-020-01317-4
26. Guelker JE, Bufe A, Blockhaus C, Kroeger K, Rock T, Akin I, et al. The atherogenic index of plasma and its impact on recanalization of chronic total occlusion. Cardiol J. (2020) 27(6):756–61. doi: 10.5603/CJ.a2018.0064
27. Kim SW, Jee JH, Kim HJ, Jin SM, Suh S, Bae JC, et al. Non-HDL-cholesterol/HDL-cholesterol is a better predictor of metabolic syndrome and insulin resistance than apolipoprotein B/apolipoprotein A1. Int J Cardiol. (2013) 168(3):2678–83. doi: 10.1016/j.ijcard.2013.03.027
28. Eliasson B, Gudbjornsdottir S, Zethelius B, Eeg-Olofsson K, Cederholm J. LDL-cholesterol versus non-HDL-to-HDL-cholesterol ratio and risk for coronary heart disease in type 2 diabetes. Eur J Prev Cardiol. (2014) 21(11):1420–8. doi: 10.1177/2047487313494292
29. Si Y, Liu J, Han C, Wang R, Liu T, Sun L. The correlation of retinol-binding protein-4 and lipoprotein combine index with the prevalence and diagnosis of acute coronary syndrome. Heart Vessels. (2020) 35(11):1494–501. doi: 10.1007/s00380-020-01627-8
Keywords: atherogenic index of plasma, atherogenic index, lipoprotein combine index, coronary artery disease, biomarker
Citation: Li Y, Feng Y, Li S, Ma Y, Lin J, Wan J and Zhao M (2023) The atherogenic index of plasma (AIP) is a predictor for the severity of coronary artery disease. Front. Cardiovasc. Med. 10:1140215. doi: 10.3389/fcvm.2023.1140215
Received: 9 January 2023; Accepted: 14 June 2023;
Published: 27 June 2023.
Edited by:
Jason P. Glotzbach, The University of Utah, United StatesReviewed by:
Alexander V Sorokin, National Heart, Lung, and Blood Institute (NIH), United States© 2023 Li, Feng, Li, Ma, Lin, Wan and Zhao. This is an open-access article distributed under the terms of the Creative Commons Attribution License (CC BY). The use, distribution or reproduction in other forums is permitted, provided the original author(s) and the copyright owner(s) are credited and that the original publication in this journal is cited, in accordance with accepted academic practice. No use, distribution or reproduction is permitted which does not comply with these terms.
*Correspondence: Jing Wan d2FuamluZ196bkAxNjMuY29t Min Zhao emhhb21pbjI4QDE2My5jb20=
†These authors have contributed equally to this work and share first authorship
Disclaimer: All claims expressed in this article are solely those of the authors and do not necessarily represent those of their affiliated organizations, or those of the publisher, the editors and the reviewers. Any product that may be evaluated in this article or claim that may be made by its manufacturer is not guaranteed or endorsed by the publisher.
Research integrity at Frontiers
Learn more about the work of our research integrity team to safeguard the quality of each article we publish.